DOI:
10.1039/D0LC01006E
(Paper)
Lab Chip, 2021,
21, 196-204
Hyperspectral imaging-based exosome microarray for rapid molecular profiling of extracellular vesicles†
Received
5th October 2020
, Accepted 20th November 2020
First published on 23rd November 2020
Abstract
One of the challenges of exploiting extracellular vesicles (EVs) as a disease biomarker is to differentiate EVs released by similar cell types or phenotypes. This paper reports a high-throughput and label-free EV microarray technology to differentiate EVs by simultaneous characterization of a panel of EV membrane proteins. The EsupplV microarray platform, which consists of an array of antibodies printed on a photonic crystal biosensor and a microscopic hyperspectral imaging technique, can rapidly assess the binding of the EV membrane proteins with their corresponding antibodies. The EV microarray assay requires only a 2 μL sample volume and a detection time of less than 2 h. The EV microarray assay was validated by not only quantifying seven membrane proteins carried by macrophage-derived EVs but also distinguishing the EVs secreted by three macrophage phenotypes. In particular, the EV microarray technology can generate a molecular fingerprint of target EVs that can be used to identify the EVs' parental cells, and thus has utility for basic science research as well as for point-of-care disease diagnostics and therapeutics.
Introduction
Extracellular vesicles (EVs), such as sub-100 nm exosomes, are membrane-bound nanovesicles actively secreted by a wide variety of cell types into their extracellular environment and are considered as important mediators of intercellular communication.1–5 Most EVs are complex and carry often highly heterogeneous molecular cargos, including proteins, lipids, and microRNAs that tend to reflect their parental cells. The rich and unique molecular information provided by EVs can be exploited to retrieve the originating cells or tissues and determine their physiological conditions. Because they can be isolated and enriched circulating EVs from clinical samples have led EVs to be categorized as an important type of high-quality biomarker for disease diagnostics, prognostics, and therapeutics6–12 in contrast to protein biomarkers that could be overwhelmed by interfering molecules of a global analysis. To fully exploit their diagnostic and therapeutic potential, EVs need to be quantified for their membrane proteins with high accuracy, throughput, and efficiency. However, existing EV analysis methods are usually based on time-consuming, expensive immunoblotting or enzyme-linked immunosorbent assays or western blotting assays.13,14 Fluorescence-based EV detection methods using microbeads and magnetic particles have multiplexing capability but require laborious assays.15 Therefore, significant efforts have been made to improve the EV analysis through the development of low-cost, rapid, and high-throughput EV sensors and systems.16–25 Our recent development of an economical label-free photonic crystal (PC) biosensor has enabled new diagnostic potential by distinguishing EV populations derived from the host cells and invasive parasites by a specific surface antigen whose expression level varies significantly between two different populations of EVs.17
Despite the progress, one remaining challenge for the broader exploitation of EVs in disease diagnosis and therapy is the need for EV analysis tools that can differentiate EVs released by similar cell types or phenotypes. To address this challenge, we propose a label-free EV microarray to discriminate EVs released by closely related cell types, such as murine macrophages. As a type of white blood cell, macrophages are critical components of the immune response and macrophage dysfunction can cause severe diseases. Macrophages can undergo specific differentiation and differentiate into phenotypes with distinct functions in response to their local tissue environments.26 Because of their remarkable plasticity, the macrophages were chosen as the EVs' parental cells. Upon activation by external stimuli, the macrophages can express widely divergent phenotypes ranging from M1 macrophages, which arise from pro-inflammatory environments, to M2 macrophages, which predominate in the TH2 immune response environment.27,28 These patterns of macrophage activation are driven by highly dynamic transcriptional changes, a product of which is the differential surface expression of epitopes taken to be characteristic of the M1 and M2 phenotypes. Although not well documented, we expected differences in cell surface markers to also be observed in the EVs released by naïve, M1, and M2 macrophages, and thus we hypothesized that these differences could be used to identify the phenotypic state of the parental cell.
This paper reports the rapid profiling of macrophage EVs based on membrane proteins using a PC-based EV microarray in conjunction with microscopic hyperspectral imaging technology.29–32 The EV assay utilizes the label-free optical sensing mechanism for multiplexed analysis, and the microarray format increases the throughput via simultaneous characterization of multiple immunobinding reactions.16,33,34 Here, we demonstrate parallel detection of seven membrane proteins of macrophage-derived EVs to distinguish the EVs from different cell origins. Twelve replicas are produced for each target protein to assess statistical significance. The EVs secreted by divergent macrophage phenotypes are characterized to investigate the EV microarray technology because the macrophage phenotypes have been well studied.35–37
Results and discussion
EV samples extracted from macrophages
The EVs used in this study were secreted by murine macrophages (J774A.1 cell line). The macrophages were cultured and activated to two extreme phenotypes, the M1 and M2 macrophages. To produce M1 and M2 macrophages, the naïve macrophages were cultured and activated by lipopolysaccharide (LPS) and interlekin-4 (IL-4), respectively. The macrophage phenotypes were incubated in three different cell culture flasks. Fig. 1a shows that EVnaïve, EVM1, and EVM2 originated from the parent macrophages. These macrophages carry membrane antigens that are specific to the phenotype. The EVs were collected from the cell culture flasks and purified by differential centrifugation as previously described.17Fig. 1b summarizes the major steps to collect, enrich, and resuspend the EVs from the macrophage cultures. The concentration of EVs was confirmed using nanoparticle tracking analysis as shown in Fig. S1 in the ESI.†
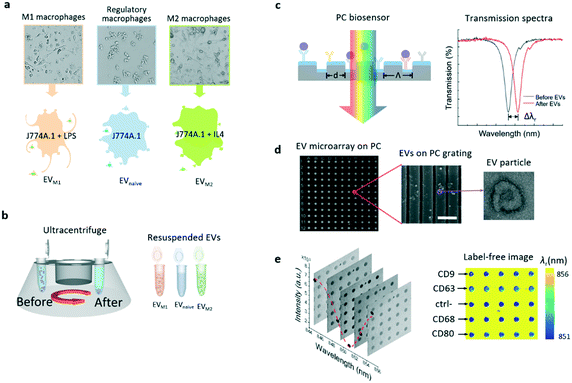 |
| Fig. 1 Schematic flowchart of EV isolation and detection using the PC biosensor. a Secretion of EVnaïve, EVM1, and EVM2 from macrophage phenotypes. b EVs are separated from spent macrophage culture media using an ultracentrifuge. The extracted EVs are re-suspended in buffer for the label-free EV microarray analysis. c Schematic diagram of the label-free detection of EVs using the PC biosensor. The antibody can serve as the ligand to detect the target EVs (purple dots). The spectral feature of measured PC transmittance shifts after the binding of EVs. d SEM images of the EV microarray (left) and PC grating with EVs (center, scale bar: 800 nm), and TEM image of an EV particle (right). e Transmission images (left) and label-free image of the EV microarray. | |
Label-free EV microarray built on a PC biosensor
The PC biosensor was designed based on a one-dimensional (1D) grating formed on a plastic substrate coated with a thin titanium oxide (TiO2) layer. The cross section of the biosensor is schematically shown in Fig. 1c. The PC substrate exhibits narrowband optical resonances owing to the guided mode resonance effect.38,39 The resonance is present as a dip in the transmission spectrum of the PC substrate (right panel in Fig. 1c). The spectral signatures, including the resonance wavelength (λr) and linewidth, have been exploited for the detection of chemicals and biomolecules.17,30,40–42 On the surface of the PC biosensor, the absorption of the analyte, in this case, EVs but may include nucleotides, proteins or cells, can result in a resonance wavelength shift (Δλr) and the shift is proportional to the analyte concentration.
Details of the PC fabrication process are described in the Methods and materials section. In brief, the plastic 1D grating structure was patterned using the nano-replica molding method.43,44 A 100 nm-thick TiO2 layer was then deposited on the grating by electron beam evaporation. For the detection of EVs, the PC biosensors were coated using a combination of antibodies against canonical EV markers and antibodies predicted to bind specifically to macrophage membrane antigens. As EV membranes are derived from parental cell membranes, it would be reasonable to expect these parental cell markers to be present on EVs. Fig. 1d shows the scanning electron microscopy (SEM) images of the EV microarray on a PC surface (left) and the EVs captured on the PC grating (center). The EVs were also characterized using transmission electron microscopy (TEM) as shown in the TEM image on the right side of Fig. 1d. The EV particle exhibits the well-known doughnut morphology. The binding of the EV and antibody leads to a redshift of the transmission dip by the amount of Δλr as shown in Fig. 1d.
To achieve the simultaneous measurement of multiple membrane proteins carried by the EVs, the EV microarray was prepared in two steps. Firstly, an 11 × 12 array of microwells was patterned in a layer of photoresist on the surface of the PC substrate (Fig. 2a). Each microwell had a diameter, depth, and period of 60 μm, 25 μm, and 150 μm, respectively, and the bottom of the microwell was the PC grating as shown in Fig. 2b. Secondly, to print specific antibodies on the grating surface in the microwells using a molecular printer,45,46 the grating surface was functionalized with aldehyde functional groups to immobilize antibodies. The antibodies were printed using a biomolecule printer in an environmental chamber with a constant relative humidity of 60%. The detailed processes of the microarray fabrication, surface functionalization, and antibody printing are described in the Methods and materials section. Fig. 2c lists the antibodies, as well as negative control spots, printed onto the microarray. From rows 1 to 12, each row was printed with the same material. The capture antibodies that are specific to a group of membrane proteins (CD9, CD63, CD68, CD80, CD81, CD86, and MHC-II) were printed. The combination of these protein markers was chosen based on their heterogeneity between the naïve, M1, and M2 macrophages.47 A blank row was assigned as the reference spots in every other antibody-coated row. Fig. 2d shows how the antibody-induced resonance wavelength shift was calculated. For one row coated with a specific antibody, the λr value of each microwell was obtained by subtracting the λr value of the nearby reference microwell. For example, the λr values of microwells A2 and B2 were subtracted from the λr values of microwells A1 and B1, respectively, and then, the mean value of the total 11 referenced microwells in the same row was calculated to represent the Δλr of the specific antibody. Subsequently, the resonance wavelength shifts caused by the blocking and EV detection steps were obtained using the λr value in the same microwell as the baseline.
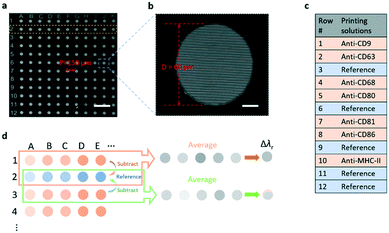 |
| Fig. 2 EV microarray fabricated on a PC sensor substrate. a SEM image of the array of 60 μm-diameter microwells patterned on the PC using photolithography. Scale bar: 300 μm. b Zoomed-in SEM image of a single microwell with the grating pattern at the bottom of the well. Scale bar: 15 μm. c List of the printed antibodies and reference spots with the corresponding row numbers. d Method to calculate the shift of resonance wavelength after the printing of each antibody. | |
Hyperspectral imaging microscopy
To measure the λr values of the entire EV microarray rapidly, a hyperspectral microscopic imaging system was developed as shown in Fig. 3a, and is described in detail in the Methods and materials section. In brief, the imaging system was built on an inverted microscope and a tunable monochromatic light source. The wavelength of the monochromatic illumination was selected using a fiber-coupled monochromator. The illumination light was collimated and polarized before it passed through the entire EV microarray. To complete one measurement, the wavelength was scanned in the vicinity of the PC resonance from 830 nm to 870 nm with an incremental of 1 nm wavelength. For each wavelength, the transmission image of the EV microarray was recorded using a charge-coupled device (CCD) camera. Fig. 3b shows the serial monochromatic images captured to assemble a hyperspectral image data cube, which contains the spectrally dispersed intensity maps of the entire EV microarray. Following data acquisition, the data cube was processed by fitting the transmission spectrum of each pixel to construct a label-free image. Fig. 3c plots the transmission intensity as a function of wavelength for the pixel located at (x, y) of the EV microarray from 846 nm to 856 nm wavelength. The resonance wavelength of this pixel, λr(x, y), was determined by fitting the transmission curve. After the λr values of 1000 × 1000 pixels are calculated, the label-free image could be created. Fig. 3d shows the pixelated label-free image consisting of the λr(x, y) values around one microwell. The blue spot and yellow area are the regions inside the microwell and photoresist layer, respectively.
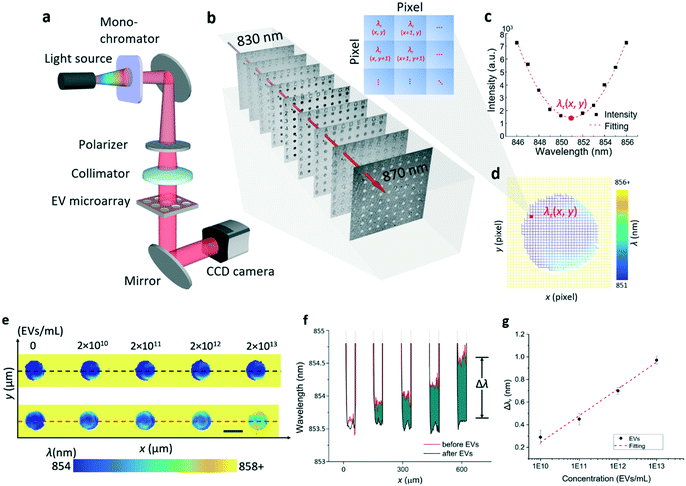 |
| Fig. 3 Hyperspectral imaging of the label-free EV microarray. a Schematic diagram of the hyperspectral imaging-based detection setup. b Intensity images captured at nine different wavelengths ranging from 830 nm to 870 nm. Each image consists of 1000 × 1000 pixels with a spatial pixel resolution of 1.85 μm. c Reconstructed transmission spectrum at a given pixel in the area of interest. The resonance wavelength of this pixel, λr(x, y), is determined by a curve fitting algorithm. d Label-free image around one microwell. e Label-free images of the EV microarray. The top panel shows the microarray before printing EVs. The lower panel shows the microarray after printing different concentrations of EVs. Scale bar: 60 μm. f Profile plot before and after EV treatment (black dashed line and red dashed line in e). Δλ represents the wavelength shift induced by the binding of EVs. g. Dose–response curve for the detection of EVs using a CD63 coated PC sensor. The Δλr values were fitted using a linear function. | |
To characterize and calibrate the PC biosensor, we measured the macrophage-derived EVs at four concentrations ranging from 2 × 1010 EVs per mL to 2 × 1013 EVs per mL. The samples were consecutively diluted in phosphate buffered saline (PBS) by a factor of ten. The PC sensor was functionalized using the CD63 antibody. Fig. 3e shows the scheme used to find Δλr values caused by the binding of the EVs to the CD63 antibody. The top panel shows the label-free images of five microwells before the printing of EVs. The bottom panel shows the label-free images of microwells, where the EVs at different concentrations were immobilized. The label-free image shows that the resonance wavelength increases with the increase of the EV concentration. Fig. 3f plots the distribution profile of λr taken along a line across the microwells. The black and red lines are the λr profiles measured before and after the binding of EVs, respectively. The difference (green area) between the black and red lines corresponds to the EV-induced Δλr. Fig. 3g shows the dose–response relationship of the EV detection by plotting Δλr as a function of the EV concentration. The limit of detection (LOD) of this label-free EV assay was determined using the concentration representing the mean Δλr value of ten negative control spots plus three times their standard deviation. Based on the fitted dose–response curve, the LOD was calculated to be 2.18 × 109 EVs per mL, which falls in the range of the clinically relevant EV concentrations (from 1 × 108 to 3 × 1012 exosomes per mL).34,48,49
Label-free EV microarray assay
The major steps of the label-free EV assay are summarized in Fig. 4a. Before the EV detection, the surface of the EV microarrays was functionalized using a reported four-step process.17 The first step (step 1 in Fig. 4a) involves coating an amine polymer layer (polyvinylamine (PVAm)) and a subsequent bifunctional linker (glutaraldehyde (GA)). The PVAm/GA treated sensors were soaked in PBS and measured using the hyperspectral microscopic imaging system to ensure the λr uniformity of microwells. Next, the capture antibodies were printed at the concentration of 0.5 mg mL−1 (step 2) and then were incubated for 4 hours at room temperature. After the incubation, the microarray was raised using PBS to remove excessive antibodies and blocked using bovine serum albumin (BSA) to prevent non-specific binding (step 3). The label-free images of EV microarrays were measured after each step. It is worth noting that the time used to prepare the EV microarray can be excluded from the EV detection time at step 4.
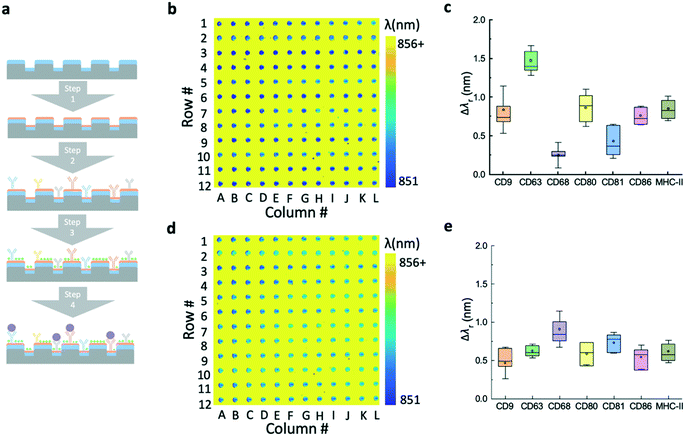 |
| Fig. 4 Preparation of the label-free microarray for multiplexed EV analysis. a Major step of the label-free EV microarray assay. The surface functionalization, printing of multiple antibodies, and blocking processes are summarized in steps 1–3. Step 4 illustrates the analysis of EVs using the printed microarray. b Label-free image of the EV microarray after the printing of seven antibodies. Rows # 3, 6, 9, 11, and 12 are the reference spots without antibodies. c Box plot of the average resonance wavelength shifts for each antibody. d Label-free image obtained after the BSA blocking. This image serves as the baseline to calculate the wavelength shifts induced by the binding of EVs. e Resonance wavelength shifts calculated by subtracting the resonance wavelengths before from after the BSA blocking. | |
Fig. 4b shows the label-free image measured immediately after the printing of antibodies. The pseudo-color plot represents the resonance wavelength for the pixels inside the microwells. Since the region outside the microwell was covered by the photoresist, the high refractive index of the photoresist can cause a larger shift than that of the biomolecules. The resulting yellow color indicates the high contrast of the resonance wavelength of the photoresist by comparing with the area of the microarray. The box plot in Fig. 4c shows the resonance wavelength shifts for the panel of antibodies, including CD9, CD63, CD68, CD80, CD81, CD86, and MHC-II. The bars represent the mean values and the 25th and 75th percentiles and the lines are the mean values ±1.5 times the interquartile range. For each antibody, the Δλr value was calculated by subtracting the reference value from the nearby reference spot and averaging the values of all 12 spots in the row. Fig. 4d shows the label-free microarray image measured after BSA blocking and before EV detection. The box plot in Fig. 4e shows the Δλr values caused by the blocking step. Here, the Δλr values were calculated by subtraction of the λr spots by the previous step value and averaging the values of all 12 spots in the row. The Δλr value of the BSA blocking step is higher if the Δλr of the antibody step is lower, and vice versa.
Characterization of EVs released by different macrophage phenotypes
The label-free EV microarray assay was used to characterize the EVs derived from the naïve, M1, and M2 macrophages. The concentration of the EVnaïve, EVM1, and EVM2 samples was 2 × 1013 EVs per mL. Fig. 5a shows the label-free image for the binding of EVnaïve. Fig. 5b compares the Δλr values of each antibody. The label-free image measured after the BSA blocking step was used as the baseline to calculate the Δλr values. For EVnaïve, CD68 and CD80 exhibit the highest and lowest Δλr values of 1.75 nm and 0.32 nm, respectively. Fig. 6a and b compare the characterization of multiple membrane proteins of EVs secreted by M1 and M2 macrophages. As shown in Fig. 6a, EVM1 presents the highest and lowest wavelength shift for CD80 (Δλr = 1.5 nm) and CD9 (Δλr = 0.3 nm), respectively. As shown in Fig. 6b, EVM2 presents the highest and lowest wavelength shift for CD80 (Δλr = 1.2 nm) and MHC-II (Δλr = 0.1 nm), respectively. The label-free images of the binding of M1 and M2 macrophage-derived vesicles on the EV microarray are given in the ESI† S4.
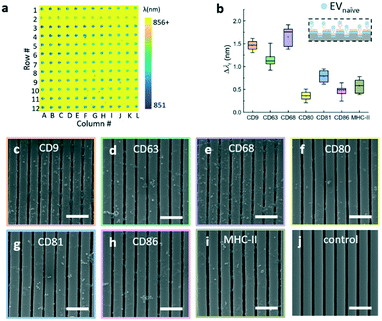 |
| Fig. 5 Characterization of multiple membrane proteins of EVs secreted by naïve macrophages. a Label-free image of the binding of naïve macrophage-derived vesicles on the EV microarray. b Box plot of the average resonance wavelength shifts caused by the binding of EVnaïve to the antibody panel. c–j SEM images of the EV immobilizations on the antibody coated PC sensor and a negative control sample. Scale bar: 1000 nm. | |
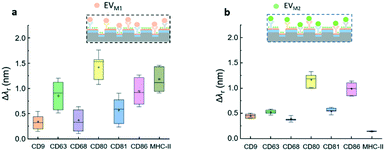 |
| Fig. 6 Characterization of multiple membrane proteins of EVs secreted by M1 and M2 macrophages. a Statistical analysis of the binding results of EVM1. b Statistical analysis of the binding results of EVM2. | |
Fig. 7a compares the measured EV membrane protein distribution profiles, indicating that EVM1 carries a significantly higher amount of CD80 than EVnaïve. In contrast, the amounts of CD68 and CD9 on EVM1 were dramatically decreased. However, EVM2 also carries more CD80 but less MHC-II. Based on the profile of the Δλr values for the antigens carried by the EVs, it is possible to differentiate the macrophage phenotypes. The radar chart in Fig. 7b shows the profiling of membrane proteins of EVnaïve, EVM1 and EVM2. The blue area represents EVnaïve and the red area and green area show EVM1 and EVM2, respectively. The length of a spoke is proportional to the Δλr value of the specific antibody. All data points are normalized to the maximum Δλr value. The radar chart shows the clearly distinguishable profiles of membrane proteins on EVs secreted by three different macrophage phenotypes.
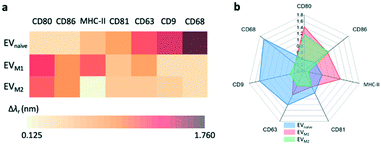 |
| Fig. 7 Profiling of membrane proteins on different EVs. a Distribution profiles of membrane proteins on the EVs from naïve, M1, and M2 macrophages, respectively. b Radar chart of wavelength shifts measured for the binding between antibodies and EVnaïve, EVM1, and EVM2, respectively. | |
Conclusions
Our data show that we can use a PC biosensor to identify EVs released by macrophages and discriminate between EVs from polarized and non-polarized parental macrophages based on these differential motifs. Polarization (to either the M1 or M2 phenotype) was characterized by a significant decrease in CD68+ EVs and an increase in CD80+ and CD86+ EVs. CD68 is generally considered a marker of cells with monocyte lineage, including macrophages, and its abundance increases in J774A.1 macrophages following polarization to both the M1 and M2 phenotypes.50 Supporting our findings, levels of CD68 in EVs secreted by murine bone-marrow derived macrophages decrease following activation.51 Low EV CD68 abundance in EVs released by polarized cells might be expected if high parental cell abundance is required to fulfil endogenous function.52 CD80 and CD86 are co-stimulatory molecules expressed on antigen-presenting cells such as macrophages; CD80+/CD86+ macrophages are associated with the M1 phenotype and CD80−/CD86− with the M2 phenotype. Whilst an increase in CD80+/CD86+ EVs was observed following LPS treatment, surprisingly polarization to the M2 phenotype also saw an increase in CD80+/CD86+ EVs perhaps reflecting a mechanism by which surface CD80 and CD86 are shed following M2 polarization. Whilst motifs discriminating EVs from polarized and naïve macrophages were evident, differentiating EVs released by M1 versus M2 macrophages may prove more difficult but our data indicate that MHC II may be a useful epitope. It is well established that EVs released by activated antigen presenting cells express MHC II that functionally presents antigen53–55 and dendritic cell EV MHC II expression is strongly increased by LPS treatment.56 Our data confirm this observation in LPS-treated macrophages and further show reduced MHC II EV binding in IL-4-treated macrophages, suggesting that MHC II expression in macrophage EVs allows differentiation of M1 and M2 parental cell phenotypes.
This work demonstrated an EV microarray assay that can characterize a panel of EV membrane proteins and distinguish EVs derived from similar originating cells. The EV microarray was built upon the PC label-free biosensor for rapid analysis of EVs based on the binding affinity between EV membrane proteins and their specific antibodies, which were printed on the microarray. The microarray-based assay enabled quantitative profiling of EV populations released by different macrophage phenotypes in in vitro culture. The advantages of the label-free EV microarray assay include the low sample volume of 2 μL, low-cost and disposable sensor, short detection time of 30 min, and improved EV sensitivity of 2 × 109 EVs per mL. The PC-based microarray biosensors are more compact and less expensive than the surface plasmon EV sensor used in previous work. Compared with the plasmonic sensor, the PC sensor's narrowband resonance mode with a spectral linewidth of 5 nm allowed the detection of EVs without using a tag or label for enhanced sensor output.
Future work plans to improve the EV microarray technology from the following aspects. First, the EV microarray sensor will be integrated with an EV extraction and purification function, such as on-chip centrifugation, immunoprecipitation using magnetic beads, and filter-based ultrafiltration, to transform the EV microarray into a point-of-care testing tool. Second, the number of target protein markers will be expanded by increasing the array density and the field of view of the hyperspectral imaging setup. The analysis of a greater number of EV markers will result in the proteome profiling capability for future studies of EVs' roles in cell–cell signal transduction. Lastly, the antibody microarray will be combined with a DNA microarray on the same PC biosensor chip to measure both protein and nucleic acid biomarkers, such as microRNAs, carried by EVs. A fully integrated EV analysis system will have great potential for in vitro disease diagnostics and therapeutics.
Methods and materials
Preparation of EV samples
Murine macrophage cells (J774A.1 cell line, ATCC) were cultured at 37 °C with 5% CO2 in Dulbecco's modified Eagle medium (DMEM) containing 10% fetal bovine serum, 100 units of penicillin, 100 μg mL−1 streptomycin, and 2 mM L-glutamine (Sigma-Aldrich, Inc.). To produce polarized macrophages, the murine macrophages were chemically activated during cell culture. The M1 and M2 macrophages were activated by adding 100 ng mL−1 LPS (Sigma-Aldrich) and 20 ng mL−1 IL-4 (BioLegend, Inc.) into the culture media, respectively. After being cultured at 37 °C with 5% CO2 for 24 h, we gently rotated the flask with the remaining medium to wash off the dead cells, and then aspirated the medium. The macrophages were washed with 10 mL DPBS (Dulbecco's phosphate buffered saline, Thermo Fisher Scientific), the flask was gently rotated to wash off the dead cells, and then the DPBS was aspirated. After adding DPBS (10 mL) into the flask, the cells were detached using a cell scraper. The solution was centrifuged at 1000 rpm for 5 min. After aspirating the DPBS supernatant, the cell pellet was resuspended in 600 μL culture medium and a 100 μL cell suspension was transferred to a new flask with 10 mL DMEM for cell culture. Usually, the macrophages can reach ∼90% confluency on day three of sub-culturing. To extract EVs, the culture medium was collected and filtered through a 0.22 μm filter to remove cells and debris, and then loaded into an ultracentrifuge tube. The sample was centrifuged at 120
000g for 90 min to collect EVs. Then the pellet was resuspended with PBS and transferred into a 1.5 ml Beckman ultracentrifuge tube. Then, they were centrifuged at 55
000 rpm for 2 hours and the pellet was resuspended with PBS. All the samples were centrifuged at 4 °C. The harvested EVs were stored in a −80 °C freezer for future use. The concentrations of the resuspended EV samples were measured using a nanoparticle tracking analysis (NanoSight LM10, Malvern Instruments).
Fabrication of the PC-based EV microarray
The nano-replica molding technique was used to generate the sub-micron grating pattern. The detailed process is given in Fig. S2 and S3 of the ESI.† Briefly, the PC grating was replicated from a silicon mold to a glass coverslip using an ultraviolet (UV)-curable polymer. The UV-curable polymer grating was coated using TiO2 to form the waveguide layer which was deposited on the polymer grating using an electron beam evaporator. To tune the resonance wavelength near 850 nm, the thickness of the TiO2 layer was chosen to be 150 nm and the refractive index of the TiO2 film was 2.2. After the PC device was characterized, the array of microwells was created on the PC by patterning a 1.5 μm-thick layer of photoresist (AZ 5214E, MicroChemicals, GmbH) using photolithography.
Surface functionalization
Before the printing of antibodies, the sensor surface was coated with aldehyde functional groups to immobilize antibodies. To do so, the sensor was soaked in a diluted PVAm solution for 12 h, followed by triple washing using deionized (DI) water. Next, the PVAm-coated sensor was dipped into GA solution (25% in water; Sigma-Aldrich) and incubated for 4 h, followed by DI water rinsing. The GA treatment enabled the covalent attachment of antibodies to the sensor surface. Next, the panel of antibodies were printed using a contact biomolecule printer (Nano eNabler, BioForce Nanosciences, Inc.).45,46 The purified anti-mouse CD9, CD63, CD68, CD80, CD81, CD86, and MHC-II (BioLegend) were dissolved in PBS buffer at 0.5 mg mL−1. The antibodies were mixed with the printing buffer (BioForce Nanosciences, Inc.) at a ratio of 50
:
50% (v/v). In each microwell, the sample volume was approximately 6 pL. The printed antibody samples were incubated for 4 hours at room temperature and at a relative humidity of 50%. Following the incubation, the microarray sensor was dipped into the BSA solution (0.5 mg mL−1) to block unoccupied binding sites. Then, the functionalized microarray chip was attached to the LiterSlip™ cover glass (Electron Microscopy Sciences). The EV sample with a volume of approximately 2 μL was pipetted between the microarray and cover glass for the label-free imaging analysis.
Hyperspectral imaging setup
The hyperspectral imaging setup was built on an inverted microscope (IX-81, Olympus, Corp.) with the addition of a monochromatic light source. A fiber-coupled monochromator (Mini-Chrom, Optometrics, Corp.) was used to filter the broadband emission of a tungsten halogen lamp (HL-2000-HP, Ocean Optics, Inc.) and generate monochromatic light. The spectral bandwidth of the monochromatic light was chosen to be approximately 1 nm. The monochromatic excitation was collimated using a fiber tip collimator (F230SMA-850, Thorlabs, Inc.) and then polarized using a linear polarizer (WP25L-UB, Thorlabs, Inc.). The diameter of the excitation beam was adjusted to cover the entire EV microarray with the polarization perpendicular to the grating direction. Transmitted light through the PC biosensor was collected using a 4× objective lens and imaged using an EMCCD camera (C9100, Hamamatsu Photonics). Each captured image consisted of 1000 × 1000 pixels and corresponded to a field of view of 2 × 2 mm2. To generate a label-free image, the monochromator was scanned from 830 nm to 870 nm with an increment of 0.5 nm. At each wavelength, the transmission image was recorded and normalized to the intensity of the monochromatic excitation. After the wavelength scan, the hyperspectral data cube with dimensions of 1000 × 1000 × 80 was assembled. At a given spatial pixel (x, y), the transmission spectrum in the wavelength range of 830 nm to 870 nm was interpolated and fitted to determine the resonance wavelength λr(x, y). Then, the label-free image of the EV microarray was generated by plotting the resonance wavelength of each pixel.
Conflicts of interest
There are no conflicts to declare.
Acknowledgements
This work was supported by the United States National Science Foundation under Grant No. ECCS 17–11839 and ECCS 16–53673. Any opinions, findings, and conclusions or recommendations expressed in this material are those of the authors and do not necessarily reflect the views of the National Science Foundation. This work was also supported by an NIH R21 award (AI117204) to MJK. YW acknowledges the Catron Center for Solar Energy Research for the Carton Graduate Fellowship.
References
- J. Meldolesi, Curr. Biol., 2018, 28, R435–R444 CrossRef CAS
.
- M. Tkach and C. Thery, Cell, 2016, 164, 1226–1232 CrossRef CAS
.
- R. M. Johnstone, Blood Cells, Mol., Dis., 2006, 36, 315–321 CrossRef CAS
.
- J. Zhang, S. Li, L. Li, M. Li, C. Guo, J. Yao and S. Mi, Genomics, Proteomics Bioinf., 2015, 13, 17–24 CrossRef CAS
.
- A. S. Azmi, B. Bao and F. H. Sarkar, Cancer Metastasis Rev., 2013, 32, 623–642 CrossRef CAS
.
- J. S. Schorey and S. Bhatnagar, Traffic, 2008, 9, 871–881 CrossRef CAS
.
- J. De Toro, L. Herschlik, C. Waldner and C. Mongini, Front. Immunol., 2015, 6, 203 Search PubMed
.
- R. J. Simpson, J. W. E. Lim, R. L. Moritz and S. Mathivanan, Expert Rev. Proteomics, 2009, 6, 267–283 CrossRef CAS
.
- F. Properzi, M. Logozzi and S. Fais, Biomarkers Med., 2013, 7, 769–778 CrossRef CAS
.
- L. Barile and G. Vassalli, Pharmacol. Ther., 2017, 174, 63–78 CrossRef CAS
.
- A. I. Masyuk, T. V. Masyuk and N. F. Larusso, J. Hepatol., 2013, 59, 621–625 CrossRef CAS
.
- H. Shao, J. Chung, L. Balaj, A. Charest, D. D. Bigner, B. S. Carter, F. H. Hochberg, X. O. Breakefield, R. Weissleder and H. Lee, Nat. Med., 2012, 18, 1835–1840 CrossRef CAS
.
- J. Lee, H. Kim, Y. Heo, Y. K. Yoo, S. I. Han, C. Kim, D. Hur, H. Kim, J. Y. Kang and J. H. Lee, Analyst, 2019, 145, 157–164 RSC
.
- H. Kalra, C. G. Adda, M. Liem, C. S. Ang, A. Mechler, R. J. Simpson, M. D. Hulett and S. Mathivanan, Proteomics, 2013, 13, 3354–3364 CrossRef CAS
.
- T. A. Hartjes, S. Mytnyk, G. W. Jenster, V. van Steijn and M. E. van Royen, Bioengineering, 2019, 6(1), 7 CrossRef CAS
.
- H. Im, H. L. Shao, Y. I. Park, V. M. Peterson, C. M. Castro, R. Weissleder and H. Lee, Nat. Biotechnol., 2014, 32, 490–495 CrossRef CAS
.
- Y. Wang, W. Yuan, M. Kimber, M. Lu and L. Dong, ACS Sens., 2018, 3, 1616–1621 CrossRef CAS
.
- T. Goda, K. Masuno, J. Nishida, N. Kosaka, T. Ochiya, A. Matsumoto and Y. Miyahara, Chem. Commun., 2012, 48, 11942–11944 RSC
.
- L. Zhu, K. Wang, J. Cui, H. Liu, X. Bu, H. L. Ma, W. Wang, H. Gong, C. Lausted, L. Hood, G. Yang and Z. Hu, Anal. Chem., 2014, 86, 8857–8864 CrossRef CAS
.
- I. Zubiri, M. Posada-Ayala, A. Sanz-Maroto, E. Calvo, M. Martin-Lorenzo, L. Gonzalez-Calero, F. de la Cuesta, J. A. Lopez, B. Fernandez-Fernandez, A. Ortiz, F. Vivanco and G. Alvarez-Llamas, J. Proteomics, 2014, 96, 92–102 CrossRef CAS
.
- J. Su, ACS Photonics, 2015, 2, 1241–1245 CrossRef CAS
.
- S. Wang, L. Zhang, S. Wan, S. Cansiz, C. Cui, Y. Liu, R. Cai, C. Hong, I. T. Teng, M. Shi, Y. Wu, Y. Dong and W. Tan, ACS Nano, 2017, 11, 3943–3949 CrossRef CAS
.
- C. Liu, J. Zhao, F. Tian, L. Cai, W. Zhang, Q. Feng, J. Chang, F. Wan, Y. Yang, B. Dai, Y. Cong, B. Ding, J. Sun and W. Tan, Nat. Biomed. Eng., 2019, 3, 183–193 CrossRef CAS
.
- F. Tian, C. Liu, L. Lin, Q. Chen and J. Sun, TrAC, Trends Anal. Chem., 2019, 117, 128–145 CrossRef CAS
.
- M. He and Y. Zeng, J. Lab. Autom., 2016, 21, 599–608 CrossRef CAS
.
- D. M. Mosser and J. P. Edwards, Nat. Rev. Immunol., 2008, 8, 958–969 CrossRef CAS
.
- P. J. Murray, Annu. Rev. Physiol., 2017, 79, 541–566 CrossRef CAS
.
- T. Lawrence and G. Natoli, Nat. Rev. Immunol., 2011, 11, 750–761 CrossRef CAS
.
- V. Chaudhery, S. George, M. Lu, A. Pokhriyal and B. T. Cunningham, Sensors, 2013, 13, 5561–5584 CrossRef CAS
.
- W. Chen, K. D. Long, M. Lu, V. Chaudhery, H. Yu, J. S. Choi, J. Polans, Y. Zhuo, B. A. C. Harley and B. T. Cunningham, Analyst, 2013, 138, 5886–5894 RSC
.
- Y. Zhuo and B. T. Cunningham, Sensors, 2015, 15, 21613–21635 CrossRef CAS
.
- Y. Zhuo, H. Hu, W. Chen, M. Lu, L. Tian, H. J. Yu, K. D. Long, E. Chow, W. P. King, S. Singamaneni and B. T. Cunningham, Analyst, 2014, 139, 1007–1015 RSC
.
- L. Wu and X. G. Qu, Chem. Soc. Rev., 2015, 44, 2963–2997 RSC
.
- J. Conde-Vancells, E. Rodriguez-Suarez, N. Embade, D. Gil, R. Matthiesen, M. Valle, F. Elortza, S. C. Lu, J. M. Mato and J. M. Falcon-Perez, J. Proteome Res., 2008, 7, 5157–5166 CrossRef CAS
.
- N. De Silva, M. Samblas, J. A. Martinez and F. I. Milagro, J. Physiol. Biochem., 2018, 74, 559–568 CrossRef CAS
.
- M. Shokooh-Saremi and R. Magnusson, Opt. Lett., 2014, 39, 6958–6961 CrossRef
.
- H. Shao, J. Chung, L. Balaj, A. Charest, D. D. Bigner, B. S. Carter, F. H. Hochberg, X. O. Breakefield, R. Weissleder and H. Lee, Nat. Med., 2012, 18, 1835 CrossRef CAS
.
- S. S. Wang and R. Magnusson, Appl. Opt., 1993, 32, 2606–2613 CrossRef CAS
.
- S. S. Wang, R. Magnusson, J. S. Bagby and M. G. Moharam, J. Opt. Soc. Am. A, 1990, 7, 1470–1474 CrossRef
.
- Y. Wang, M. A. Ali, E. K. C. Chow, L. Dong and M. Lu, Biosens. Bioelectron., 2018, 107, 224–229 CrossRef CAS
.
- Y. Wang, Y. Huang, J. Sun, S. Pandey and M. Lu, Opt. Express, 2015, 23, 28567–28573 CrossRef CAS
.
- B. T. Cunningham, B. Lin, J. Qiu, P. Li, J. Pepper and B. Hugh, Sens. Actuators, B, 2002, 85, 219–226 CrossRef CAS
.
- I. D. Block, L. L. Chan and B. T. Cunningham, Microelectron. Eng., 2007, 84, 603–608 CrossRef CAS
.
- H. Schift, J. Vac. Sci. Technol., B: Microelectron. Nanometer Struct.--Process., Meas., Phenom., 2008, 26, 458–480 CrossRef CAS
.
- S. Nettikadan, K. Radke, J. Johnson, J. T. Xu, M. Lynch, C. Mosher and E. Henderson, Mol. Cell. Proteomics, 2006, 5, 895–901 CrossRef CAS
.
- J. Xu, M. Lynch, S. Nettikadan, C. Mosher, S. Vegasandra and E. Henderson, Sens. Actuators, B, 2006, 113, 1034–1041 CrossRef CAS
.
- F. O. Martinez and S. Gordon, F1000Prime Rep., 2014, 6, 13 Search PubMed
.
- A. A. Sina, R. Vaidyanathan, S. Dey, L. G. Carrascosa, M. J. Shiddiky and M. Trau, Sci. Rep., 2016, 6, 30460 CrossRef CAS
.
- J. de Vrij, S. L. Maas, M. van Nispen, M. Sena-Esteves, R. W. Limpens, A. J. Koster, S. Leenstra, M. L. Lamfers and M. L. Broekman, Nanomedicine, 2013, 8, 1443–1458 CrossRef CAS
.
- K. Hassani and M. Olivier, PLoS Neglected Trop. Dis., 2013, 7, e2185 CrossRef CAS
.
- C. Wang, C. Zhang, L. Liu, B. Chen, Y. Li, J. Du and X. A, Mol. Ther., 2017, 25, 192–204 CrossRef CAS
.
- D. A. Chistiakov, M. C. Killingsworth, V. A. Myasoedova, A. N. Orekhov and Y. V. Bobryshev, Lab. Invest., 2017, 97, 4–13 CrossRef CAS
.
- G. Raposo, H. W. Nijman, W. Stoorvogel, R. Liejendekker, C. V. Harding, C. J. Melief and H. J. Geuze, J. Exp. Med., 1996, 183, 1161–1172 CrossRef CAS
.
- L. Zitvogel, A. Regnault, A. Lozier, J. Wolfers, C. Flament, D. Tenza, P. Ricciardi-Castagnoli, G. Raposo and S. Amigorena, Nat. Med., 1998, 4, 594–600 CrossRef CAS
.
- C. Thery, L. Duban, E. Segura, P. Veron, O. Lantz and S. Amigorena, Nat. Immunol., 2002, 3, 1156–1162 CrossRef CAS
.
- E. Segura, S. Amigorena and C. Thery, Blood Cells, Mol., Dis., 2005, 35, 89–93 CrossRef CAS
.
Footnote |
† Electronic supplementary information (ESI) available. See DOI: 10.1039/d0lc01006e |
|
This journal is © The Royal Society of Chemistry 2021 |
Click here to see how this site uses Cookies. View our privacy policy here.