The protein–water nuclear Overhauser effect (NOE) as an indirect microscope for molecular surface mapping of interaction patterns†
Received
27th August 2019
, Accepted 20th November 2019
First published on 30th November 2019
Abstract
In this computational study, the intermolecular solute–solvent Nuclear Overhauser Effect (NOE) of the model protein ubiquitin in different chemical environments (free, bound to a partner protein and encapsulated) is investigated. Short-ranged NOE observables such as the NOE/ROE ratio reveal hydration phenomena on absolute timescales such as fast hydration sites and slow water clefts. We demonstrate the ability of solute–solvent NOE differences measured of the same protein in different chemical environments to reveal hydration changes on the relative timescale. The resulting NOE/ROE-surface maps are shown to be a central key for analyzing biologically relevant chemical influences such as complexation and confinement: the presence of a complexing macromolecule or a confining surface wall modulates the water mobility in the vicinity of the probe protein, hence revealing which residues of said protein are proximate to the foreign interface and which are chemically unaffected. This way, hydration phenomena can serve to indirectly map the precise influence (position) of other molecules or interfaces onto the protein surface. This proposed one-protein many-solvents approach may offer experimental benefits over classical one-protein other-protein pseudo-intermolecular transient NOEs. Furthermore, combined influences such as complexation and confinement may exert non-additive influences on the protein compared to a reference state. We offer a mathematical method to disentangle the influence of these two different chemical environments.
1 Introduction
In order to understand the actual mechanisms underlying the function of biomolecules, we need to study the natural environment they operate in, i.e. aqueous solution1,2 and confinement in a biological cell, surrounded by a high concentration of other solutes.3,4 Many contemporary hydration dynamics measurement methods such as time-resolved fluorescence spectroscopy5 or ODNP have been used to study local hydration dynamics near globular,6 fibrous7 and membrane-bound8 proteins but require the insertion of an artificial probe (chromophore or stable radical) into the native protein type. In contrast, intermolecular protein–water NOEs provide an intrinsic probe to measure site-resolved hydration dynamics of proteins.9,10 Another advantage of the protein NOE is that hydrogen atoms cover the entire surface of the protein, allowing for a comprehensive mapping of hydration dynamics. Taking the example of the popular model protein UBQ,9–11 one can probe up to 629 hydrogen atoms (about 315 surface protons12) but only one intrinsic UV/Vis chromophore, TYR-59,13–15 which is furthermore buried in the protein scaffold16,17 and subject to intramolecular quenching mechanisms.18,19 A list of the abbreviations used in this article is given in Table 1.
Table 1 List of abbreviations used in the article
DMAG |
1-Decanoyl-rac-glycerol |
LDAO |
Lauryldimethylamine-N-oxide |
NOE |
Nuclear Overhauser effect |
NOESY |
Nuclear Overhauser effect correlation spectroscopy |
NQR |
Nuclear quadrupole resonance |
ODNP |
Overhauser dynamic nuclear polarization |
RDF |
Radial distribution function |
RM |
Reverse micelle |
ROE |
Rotating-frame nuclear Overhauser effect |
ROESY |
Rotating-frame nuclear Overhauser effect correlation spectroscopy |
SDF |
Spectral density function |
TCF |
Time-correlation function |
UBQ |
Ubiquitin |
In essence, the TCF relevant to dipole–dipole magnetization transfer NOE captures the average dynamics of the vectors rIS joining a protein atom (typically hydrogen) with spin I and the water protons with spin S:
|  | (1) |
where
rIS is the distance between the two spins and
θIS is the angle swept by the connecting vector during time
t. The Fourier transform of
GIS(
t) yields the SDF
J(
ω)
| 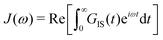 | (2) |
whose linear combinations can be measured experimentally as the self- and cross-relaxation rates
ρ and
σ:
20 | 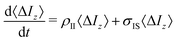 | (3) |
| 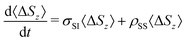 | (4) |
with
| σNOE(ω) = 0.6J(2ω) − 0.1J(0) | (5) |
| σROE(ω) = 0.3J(ω) + 0.2J(0) | (6) |
| ρ(ω) = 0.6J(2ω) + 0.3J(ω) + 0.1J(0) | (7) |
where
ω is the Larmor frequency in the homo-nuclear case. The intramolecular NOESY signal is exploited regularly in organic-chemical and biochemical structure elucidation due to its strict 1/
r6 distance dependence. In the past, this inspired experimentalists to tacitly assume the 1/
r6 short-range dependency for intermolecular NOEs as well.
21–25 However, in this case, one reference spin
I interacts with many solvent spins
S. For instance, using above
σNOE relation and assuming exponential correlation decay
| 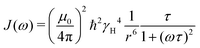 | (8) |
a measured protein–water
σNOE rate of 0.01 m s
−1 between an UBQ surface proton and water would yield a spin–spin vector relaxation time
τ close to the P2 tumbling time of the protein itself and would thus refer to the extreme case of a water molecule sticking strongly to the surface.
12 It was shown that intermolecular NOEs lead to distance dependencies 1/
rn with
n ranging from 1 to 3.
26,27 Using these long-ranged distance dependencies in above example, we obtain more realistic water residence times of tens of picoseconds.
28
In light of this difference, the explanatory qualities of intermolecular NOE studies have been called into question.29–32 Halle first described the long-ranged nature of the intermolecular SDF in his groundbreaking work using simple step functions to describe the RDFs of interacting nuclei.29 Halle's finding holds even when accounting for local structure by using more sophisticated model functions,33 RDFs from MD simulations26,34 or calculating the SDF directly from MD simulations.27,34 While carrying chemically specific structural information, the actual NOESY signal is contaminated by many unspecific long-ranged spin interactions29,33 with the range depending on the frequency of the spectrometer26 which casts doubt on the locality of the intermolecular NOE.
However, more recent computational studies by our group have shown that while absolute NOE entities (σNOE, σROE, ρ,…) are in fact long-ranged, relative observables like the ratio σNOE/σROE correlate well with the actual site-specific hydration dynamics12 due to relative error compensation eliminating chemically unspecific long-ranged contributions.35 While the first hydration shell shows a mere overall retardation of a factor of about 5 around proteins28,30,36,37 and 3 around interface walls,38,39 NMR possesses the unique ability to separate contributions by chemical identity and reveal a surprisingly large range of dynamics. We reported comprehensive hydration data12,35 mapped on each protein hydrogen and were thus able to point out three water pockets holding slow structural water in the protein UBQ as opposed to the two previously known ones.37,40
Additionally, comparative NOE/ROE ratio calculations Δconfined(σNOE/σROE) of UBQ dissolved in diluted bulk water and UBQ in a living-matter mimic (macromolecular crowding by other proteins, encapsulation in a RM or both, hitherto referred to as confined system)
|  | (9) |
showed that the NOE/ROE ratio picks up the slow dynamics of water molecules captured interstitially between the measured protein and a foreign interface and is thus able to map the inherent heterogeneity of confined systems by measuring the same protein–water cross-peak of a protein hydrogen in a confined environment and in diluted bulk solution both with NOESY and ROESY, form the ratio and subtract them.
35 This encapsulation process corresponds to paths B → E symbolized by green arrows in
Fig. 1.
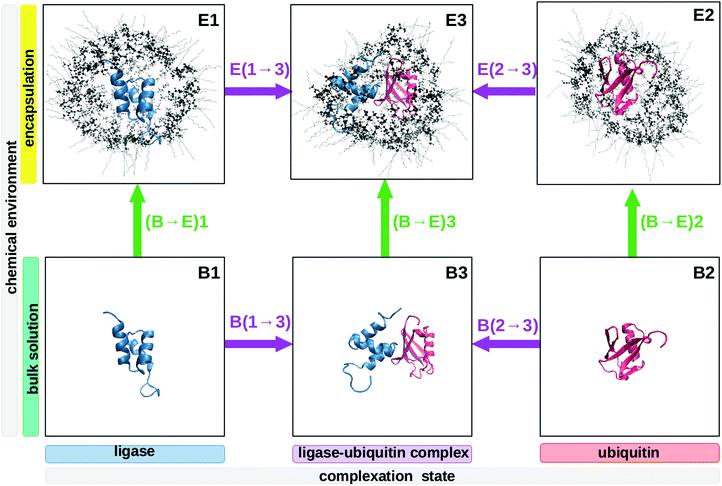 |
| Fig. 1 Overview of the six systems necessary to analyze the combined effect of complex formation (paths 1 → 3 and 2 → 3, purple arrows) and encapsulation (paths (B → E)1, (B → E)2, (B → E)3, green arrows). The combined effect is described either by pathway B(1, 2 → 3); (B → E)3 (complex formation prior to encapsulation) or, alternatively, by path (B → E)1, 2; E(1, 2 → 3) (encapsulation before complex formation). | |
Note that proteins in bulk solution are prone to chemical proton exchange and exchange-relayed NOE, making experimental interpretation difficult and dependent on assumptions when separating magnetization transfers into the actual dipole–dipole coupling signal and the exchange contributions on the time scale. Quite generally, protein hydration dynamics in bulk solutions are fast41 and may be difficult to measure, requiring e.g. hyperpolarized solvent NMR spectroscopy.42 Water dynamics in RMs, however, are slow in the high-field NMR43 and hydrogen exchange is slowed down drastically.9
Typically, a σNOE/σROE term varies from −0.5 to 1.12 Hence, our ratio difference varies from −1.5 = −0.5 − (+1) (hydration dynamics at this site is much slower in confinement than in bulk) to 1.5 = +1 −(−0.5) (much faster in confinement than in bulk). In practice, the difference is limited up to ≈0 since solute and solvent molecular motion are retarded in confined systems compared to bulk solutions.44,45
Instead of the ratio of two observables σNOE and σROE, one may also measure just one observable per sample, the NOE enhancement, and form the difference.35 This may be experimentally advantageous for various reasons, e.g. σROE is often merely estimated,46 though interpretation may be more complicated due to spin diffusion and hydrogen exchanges. See the ESI† document for the equivalence of using either the NOE to ROE ratio or the NOE enhancement.
Since the intermolecular solute–solvent NOE is able to map anisotropic hydration dynamics brought about by confinement effects, we raise the question whether it is also able to map hydration dynamics changes brought about by complex formations, e.g. between two proteins. These specific protein–protein interactions are important in many biological processes involving molecular recognition,47 for instance enzymatic catalysis48 and docking.49
Thus we performed molecular dynamics simulations, allowing us to use explicit atomistic time-resolved data. Despite the high computational effort, we avoided the far more common analytical models and their intrinsic assumptions like strictly exponentially TCFs, which were experimentally found to be non-applicable to some molecular liquids.51,52 We selected UBQ, a protein involved in many protein–protein complex formations in protein-degradation pathways.53 UBQ is a small protein (76 amino acids) of near-spherical geometry and a stable conformation. Due to its robustness and availability, it is widely used in theoretical and experimental proof-of-concept studies, e.g.ref. 9 and 10. We selected the ubiquitin protein ligase Cbl-b54 as its partner. They form a complex as depicted in the bottom row of Fig. 1.
2 Methods
This section details the computational experiments carried out. Fig. 2 summarizes the work-flows employed.
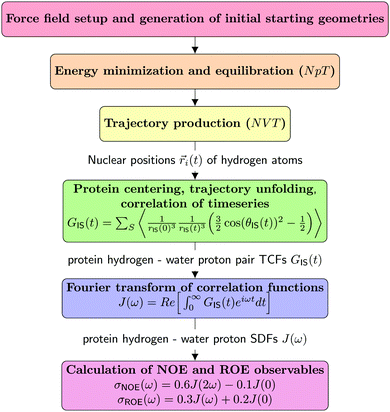 |
| Fig. 2 Schematic representation of the MD/computational spectroscopy work-flows performed in the frame of this study. | |
2.1 Molecules and force field
The geometries of the three proteins studied were taken from the RCSB database:55
• ligase: entry 2OOA54
• ubiquitin: entry 1UBQ40
• ligase–ubiquitin complex: entry 2OOB54
The proteins were parametrized using appropriate CHARMM36 atom types.56–59
We picked Berendsen's SPC/E water model60 to represent the explicit solvent. This water model has been shown to reproduce experimental observables like dielectric relaxation,61 terahertz spectra,62 neutron scattering63 and, most importantly, NMR data64 better than the TIP family of water models. It was demonstrated to combine well with the CHARMM force field.65
In the RM systems, we used isooctane as the immersion medium surrounding the RM. While this phase fills most of the simulation box in our systems, it is not relevant to the post-production analysis per se. Hence, both isooctane and the aliphatic tails of the surfactant were modelled as coarse-grained to save computational resources with one particle representing a CH, CH2 or CH3 group. For this aliphatic phase, we set the negligible atomic charges strictly to zero. All other parameters were selected from van Gunsterens GROMOS 45A3 force field which was designed specifically for aliphatic aggregates like lipids and micelles.66 In practice, it has been shown to combine excellently with the polar phase described above, yielding stable RMs which accurately reproduce dielectric absorptions,67 terahertz bands68 and NQR relaxation times.69
We picked a novel surfactant consisting of a 1
:
2 mixture of the zwitterionic LDAO and the monoglyceride DMAG. This mixture was demonstrated by Wand et al. to safely encapsulate a variety of proteins and even t-RNA, conserving the macromolecular structures faithfully.70,71 The aliphatic tails were modelled analogously to isooctane described above, the polar heads of the LDAO and DMAG surfactants were modelled atomistically with appropriate CHARMM36 atom types56–59 except for the charge, which was derived from quantum-chemical calculations. For details regarding the surfactant force field creation, we refer the reader to ref. 72.
Recent works showed that current biomolecular force fields are optimized to model simply one protein in aqueous solution, yielding considerable artifacts when using several proteins capable of interacting with one another.73,74 These simulations lead to erroneous solvation energies73 and exaggerated protein aggregation behaviour.74 By extension, this defect is also true for RMs where the studied protein may interact with the surfactant wall. Best and Mittal pointed out that these errors can be counteracted fairly well when scaling the Lennard-Jones interaction of protein–water atom pairs by a factor λ:73
| 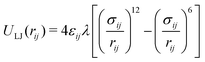 | (10) |
In essence, one makes the protein surface stickier for water molecules, efficiently increasing hydration. Setting
λ = 1.1 already improves the simulation accuracy considerably.
73 Thus, in order to avoid conclusions based on simulational artifacts, we created RM simulations using different levels of Mittals scaling
λ = 1.1:
1. The RM systems with standard force field parameters (unscaled system)
2. RM systems with the protein–water interactions scaled up as proposed by Best and Mittal (half-scaled system)
3. As above, but with the water–surfactant interactions scaled up as well (fully scaled system)
Overall, we find that our results are consistent across all three levels of λ-scaling, thus we do not discuss this aspect further.
2.2 Simulation setup and trajectory production
Table 2 summarizes the systems used in the frame of this work. For each system, we created five independent replica using numerical seeds to ensure statistical stability in the following analysis. Furthermore, note that for the confined systems, we employed three different levels of λ-scaling as described above. This ensures independence from force field artifacts known to occur in simulations with multiple biological interfaces.73,74
Table 2 Overview of the systems simulated. Both the ligase and the ubiquitin–ligase complex simulations were produced for the purposes of this study. The ubiquitin bulk system was created for this project as well, but the ubiquitin micelle simulations were reused from ref. 50
System |
# H2O |
# 2OOA |
# 1UBQ |
# 2OOB |
# Na+ |
# LDAO |
# DMAG |
# isooctane |
Ligase bulk |
6500 |
1 |
— |
— |
3 |
— |
— |
— |
Ligase confined |
1500 |
1 |
— |
— |
3 |
50 |
100 |
9000 |
Ubiquitin bulk |
6500 |
— |
1 |
— |
— |
— |
— |
— |
Ubiquitin confined |
1500 |
— |
1 |
— |
— |
50 |
100 |
9000 |
Complex bulk |
6500 |
— |
— |
1 |
3 |
— |
— |
— |
Complex confined |
1500 |
— |
— |
1 |
3 |
50 |
100 |
9000 |
The starting geometries of the simulation boxes were constructed using the program PACKMOL.75 In the simple case of the bulk systems, the protein was put into the box center and surrounded by the specified amount of water molecules and counter ions. In the case of an RM, the protein was set into the box center, then surrounded by a sphere of water molecules and counter ions. This sphere was covered by a layer of properly oriented surfactant molecules. The cubic simulation box containing the proto-micelle in its center was then filled with the proper amount of isooctane molecules.
The following molecular dynamics simulation steps were performed using DOMDEC CHARMM.76,77 The initial PACKMOL geometries were energetically minimized by 2000 steps of steepest descent. The resulting simulation box was then equilibrated as a toroidal isobaric–isothermal (NpT) ensemble at T = 300 K for 4 ns. The converged cubic box length was then used to start the actual production of the trajectory as a toroidal isochoric–isothermal (NVT) ensemble. The temperature was kept constant at T = 300 K using the Nosè-Hoover thermostat.78,79 The equations of motion were integrated using a leap-frog scheme.80 High-frequency vibrations of covalent bonds involving hydrogen atoms were constrained using the SHAKE algorithm81 which allowed for a generous time step of 2 fs, a write frequency of 1 ps and a total trajectory length of 450 ns. Non-bonded interactions were smoothly switched off between 10 and 12 Å. The long-ranged latter interactions were calculated using the Particle Mesh Ewald method82,83 with cubic splines of order 6 on a 128 × 128 × 128 grid with κ = 0.41 Å−1 (tinfoil boundary conditions).
For the subsequent computational NOE analysis, we used 60 independent trajectories, a total of 21
000 ns of simulational data.
2.3 Post-production analysis
The resulting CHARMM trajectories were read into a Python3 program with the MDAnalysis module.84,85 The dipole–dipole TCF was evaluated using accelerated Cython functions. All pairs formed by a protein hydrogen atom (397 in the ligase, 629 in ubiquitin and 1026 in the ubiquitin–ligase complex) and a water hydrogen atom (13
000 in the bulk systems and 3000 in the RM systems) were analyzed, totaling between 1
191
000 and 13
338
000 interacting pairs per trajectory depending on the system. In total, we have thus recorded 225
720
000 protein–water correlation functions. Furthermore, we calculated the correlation functions in a radially resolved manner to examine long-range contributions, using an increment of 0.1 nm up to 3.0 nm.
In essence, the same TCF construction method presented in ref. 12 was employed in this study. In all systems, we have chosen a trajectory length of 350 ns and a TCF length of 50 ns with 500 equally spaced starting points and a time step of 10 ps. We discarded the initial 100 ns of each trajectory to avoid slow equilibration artifacts, in particular systematic protein motion within the RMs.67 At each starting point, the protein was centered in the simulation box, ensuring minimum distance of the protein and the water molecules. For every starting point, the trajectory was unfolded allowing a natural diffusive motion of water, correcting toroidal coordinate jumps caused by periodic boundary conditions. This is essential for correctness because the collision-induced dipole–dipole TCF tracks the diffusive motion of the spin pair, hence spatial context matters.
The resulting TCFs were averaged over the five independent replica to minimize the influence of random simulation events, then real-part Fourier transformed using the numpy.fft module to obtain the spectral density function, which in turn served as a base to calculate the frequency-dependent self- (ρ(ω)) and cross-correlation rates (σNOE(ω), σROE(ω)). The rates used in the main article were taken at ν = 600 MHz, a common operating frequency in 1H-NMR spectrometers.
3 Results and discussion
3.1 Ubiquitin–ligase complex in liquid-bulk solution
Protein–protein interactions are profoundly important in biological processes47 such as molecular docking49 and enzymatic catalysis,48 hence inspiring interest in protein–protein geometry in solution. The difference in hydration dynamics was calculated as a difference of NOESY and ROESY signals from the same protein–water cross-peak |  | (11) |
for each protein hydrogen. This corresponds to the complex formation paths 1 → 3 and 2 → 3 in Fig. 1 (purple arrows). Note that a difference of NOEs refers to forming the ratio of the hydration dynamics, since the context between translational diffusion and σNOE/σROE is logarithmic.12 The resulting maps thus represent the relative timescale.
The result of this procedure is shown in Fig. 3. Using the example of UBQ, the ligase selectively coordinates the β-sheets over the opposite alpha-helix, as is typical for UBQ complexes.54,86–88 Local hydration anisotropies like water pockets do not appear in this difference if their hydration dynamics do not change systematically upon complexation. Thus, we see that Δcomplex(σNOE/σROE) is able to map spatial anisotropy brought about by complex formation as well.
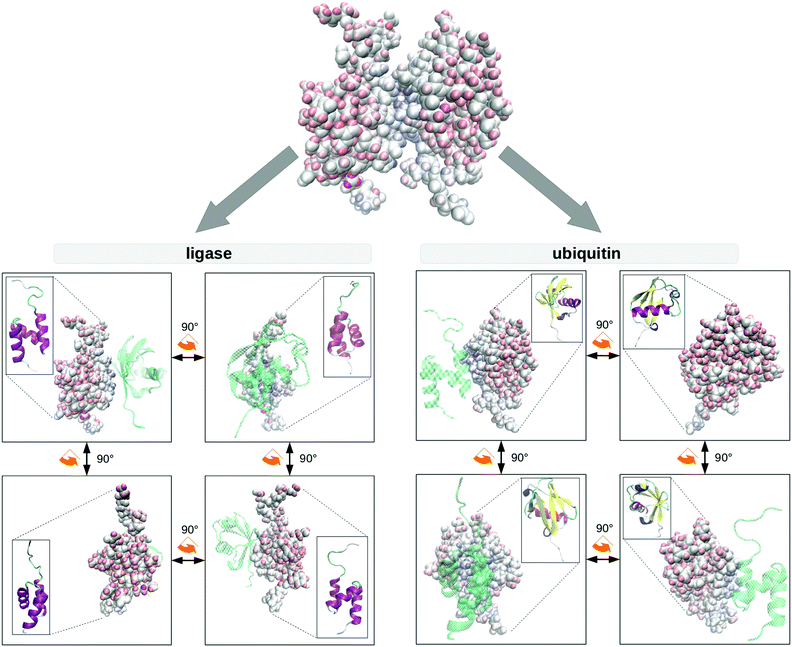 |
| Fig. 3
Δ
complex(σNOE/σROE) calculated for the UBQ–ligase complex. The top figure shows this difference for each protein hydrogen with colors indicating neutral (red) to negative (blue) values. This corresponds to unaffected hydration dynamics versus strongly retarded ones. To present this entity for each partner in the complex, the two sub-panels resolve Δcomplex(σNOE/σROE) in detail while representing the partner as a green ribbon cartoon shadow. Note that in precisely those regions where the two partners cover each other, the hydration water limited to interstitial molecules exhibits strongly retarded dynamics, thus reflecting complex formation in an indirect, but spatially resolved way. | |
Note that position of the complex partner is revealed by an indirect measure, i.e. the hydration dynamics, standing for the spatially resolved protein–water interaction. This is in sharp contrast to classical NOE studies of complex formation via direct intermolecular NOEs between the complex partners. This indirect method offers potential experimental advantages:
• Instead of relying on an isolated spin pair I and S residing on different complex partners, the interaction with the singular S spin is replaced by a set of interactions with many water spins S.
• As a further consequence, the indirect way of probing interactions within the complex can be applied to short-lived complexes as well. The direct measurement of the transient NOE between complex partners is of quasi-intramolecular character and thus limited to complexes surviving on the NMR time scale. The protein–water NOE, on the other hand, always represents an average hydration dynamics not requiring stable complexes.
3.2 Encapsulated proteins
At this point, it is important to remember that the biomolecules forming the machinery of life exist and function in the context of a biological cell, crowded by other cell contents. This environment significantly reduces the volume available to biomolecules and thus alters their behaviour.3,4 Aside from unspecific excluded volume effects, directed interaction with other biological interfaces44,89,90 impacts protein enthalpy terms91 and dynamics45,92 which in turn alters the equilibria and reaction rates molecules partake in compared to usually studied diluted buffer solutions.4,93 These confinement conditions influence protein stability, aggregation behaviour, folding rates3,4,94–98 and hydrogen exchange rates,41,70,71 while the solvating water exhibits greater viscosity,43,99–108 a smaller dielectric constant67,109–116 and normal50,117 to elevated72 collective dynamics. Depending on the system studied, protein secondary structures are reinforced118–121 or destabilized.121,122
Thusly motivated, we simulated the three protein systems encapsulated in RMs as shown in the top row of Fig. 1. Of course, these RMs do not resemble actual biological cells in size, wall curvature or wall composition, but they do model some aspects of cellular environments, for instance the presence of a volume limiting wall. In previous work, we have shown that Δconfined(σNOE/σROE) is capable of spatially revealing the specific influence of the cell wall on one encapsulated protein.35 We confirm these previous findings in Fig. 4 where Δconfined(σNOE/σROE) specifically resolves the sites in proximity to the cell wall. For instance, UBQ preferentially interacts with the micelle wall via its β-sheets. This is the same position containing the hydrophobic patch (Leu-8, Ile-44, Val-70), the preferential region for UBQ complex formation53,123 as can be seen in Fig. 3. The secondary structure stays intact during this interaction as was found empirically.11 Experimentally, protein–surfactant interactions have been known to occur124 and have been described both by the groups of Wand125,126 and Flynn.127–129 In particular, Flynn studied encapsulated UBQ using HSQC, NMR chemical shift perturbation and Lipari–Szabo order parameters and reported interactions between the β-sheet and the surfactant wall.127–129 While the group of Flynn used another surfactant in their experiments, their findings regarding UBQ qualitatively agree with ours.
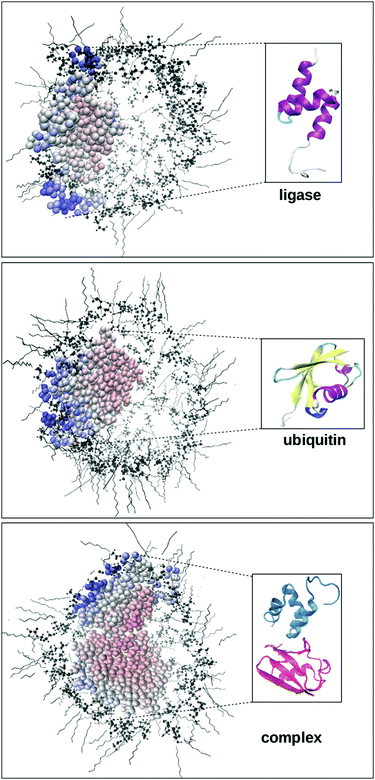 |
| Fig. 4 The difference Δconfined(σNOE/σROE) between the unconfined and the confined protein (ligase, top; UBQ, middle; complex, bottom) can be used to detect contact with the cellular wall. Available experimental NOE data for UBQ encapsulated in AOT confirm slow water dynamics at the β-sheets and fast water dynamics at the α-helix.9,10 Note that in the complex (bottom figure), the NOE/ROE difference caused by complex formation as discussed above does not show up. This is because we form the differences corresponding to paths (B → E)1, (B → E)2 and (B → E)3 in Fig. 1 corresponding to the green arrows. In these paths, the complex binding state (unbound vs. bound) does not change for the sample proteins, thus the influences of complex formation are effectively ruled out. | |
Surfactant–wall interactions can profoundly impact enzymatic activity. For instance, Cabral et al. found that encapsulation of cutinase inhibits its catalytic activity.130–132 Furthermore, note how the protein sites facing the RM boundary and thus seeing extremely retarded interstitial water show very low NOE/ROE ratios. In fact, the existence of such slowed-down mono-layers of water has been connected recently to the cryopreservation of biomolecules.133,134
3.3 Distinction of different chemical influences
In the encapsulated complex system E3, mimicking protein–protein interaction in a biological cell, we now face retarding influences brought about by both the complex partner and the cellular wall as expressed by eqn (9) and (11) (position in the cell vs. complex binding state). Fig. 5 displays ΔΔconfined,complex(σNOE/σROE) for the system studied here.
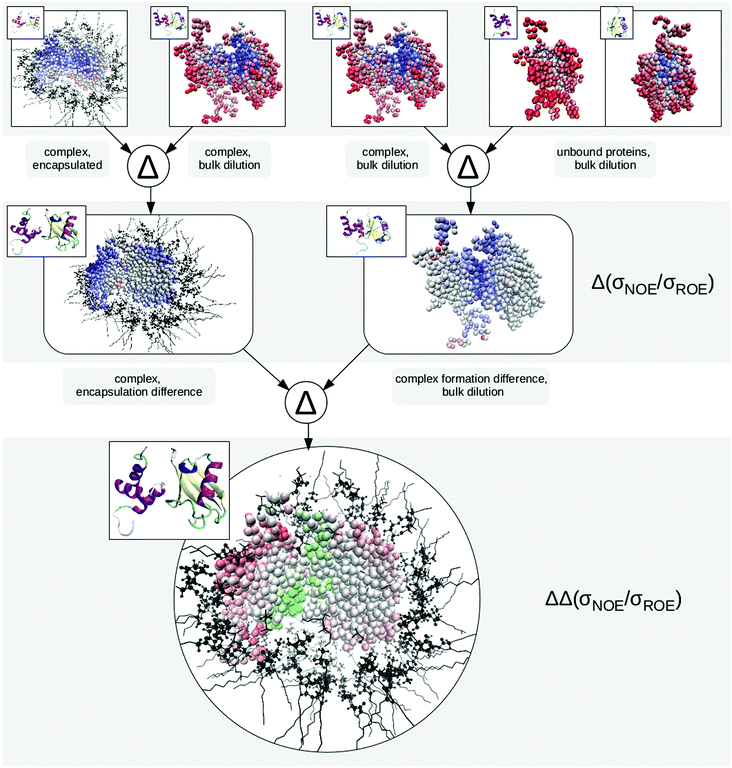 |
| Fig. 5 Expression tree showing the calculation of ΔΔconfined,complex (eqn (12)) in a pictorial way. The top row presents the protons of the studied proteins, coloured by their σNOE/σROE from high (red, fast dynamics) to low (blue, slow dynamics) values. In case of the unbound proteins, the few slow hydration sites correspond to water pockets. In the next row, we form the encapsulation difference of the protein–ligase complex (left-hand side) as well as the complex formation difference of the complex in bulk dilution. Red indicates faster hydration dynamics while blue marks retarded sites. In case of the encapsulation difference, this retardation occurs at the sites facing the RM wall, while for the complex formation, water caught between the two proteins causes the strong difference. Forming the difference of differences, we obtain the proton map at the bottom. Note how it clearly distinguishes the protein regions (a) facing the complex partner, (b) facing the cell wall or (c) facing neither. Values below zero are marked in red and are indicative of the first subtraction term (in this case encapsulation of the complex) while positive values are marked in green and point to the second subtraction term being dominant (complex formation in free bulk solution). Neutral (white) values indicate equal influence of both leading to cancellation. | |
Forming the difference between the encapsulated complex E3 and the diluted unbound proteins B1 and B2 would yield an unspecific retardation map. In the specific example of UBQ, we cannot tell whether the complex partner or the cell wall coordinates the β-sheets, as both foreign interfaces have shown a tendency to do so above. Thus, in order to disentangle the two different influences, we need to consider paths in Fig. 1. In formal language, this corresponds to taking the double difference
| 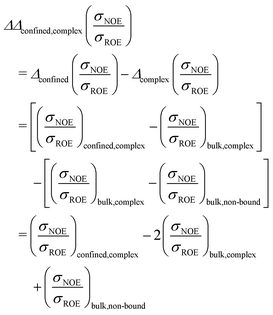 | (12) |
This corresponds to forming the complex, then encapsulating it (pathway B(1, 2 → 3), (B → E)3). Alternatively, one may first encapsulate the unbound protein, followed by complexation within the capsule, though we discourage forming
ΔΔconfined,complex this way. This double difference is not a state function and calculating another path will result in a different surface pattern. We discuss the path dependence in the ESI
† in detail.
In total, this calculation requires three different samples of the protein to be measured in NOESY and ROESY experiments (or in NOE enhancement experiments): In term (1), the protein (e.g. UBQ) is in complex with its partner (e.g. ligase) and confined in a capsule (system E3); in term (2) the protein complex is dissolved in a diluted buffer (system B3); term (3) requires the protein to be without binding partner, dissolved in solution (system B1 or B2).
From this calculation procedure, which can be done both for computational and empirical data, it now becomes clear which part of UBQ interacts with the ligase and which interacts with the micelle wall. Thus we are now in a position to discriminate whether a surface proton is influenced by the proximity of the complex partner or the cellular wall.
Eqn (12) may also be generalized and applied to discern any two different influences, for instance two different possible binding partners or the influence of a specific binding partner vs. the influence of an unspecific crowder.
4 Conclusions
Intermolecular solute–solvent NOE in principle contains hydration structure and dynamics information. While the raw NOESY observable is contaminated with a multitude of chemically unspecific long-ranged interactions, short ranged observables such as the ratio NOE/ROE reveal local hydration phenomena on the absolute timescale such as fast surface hydration and slow water trapped in pockets. In this study, we focus on differences of NOE signals from the same protein in different environments, thus accessing relative timescales instead: the absolute hydration dynamics does not matter, but the extent of change does. For example, on a relative timescale, a very slow hydration site and a very fast hydration site slowed by the same factor appear as equal. Experiments measuring the relative time scale require both a test system and a reference system.
Generally, retardations are caused by the proximity of foreign interfaces, which trap water interstitially between their surface and the probe protein, causing it to slow down. Thus, the surface map of the relative timescale (i.e. differences of NOE/ROE) is able to spatially resolve the presence of this interface. We show this for a protein complex of UBQ with a ligase. The coordination of a protein site by a different biomacromolecule leads to hydration retardation, which is measurable via indirect protein–water NOEs. This approach may in future offer experimental advantages over traditional transient direct NOEs between complex partners. As a second test case, nanoconfined UBQ feels the surfactant wall proximity due to strongly retarded water specifically at the β-sheets.
Both informations can be combined to detect, e.g., which site of a protein preferentially interacts with the cell wall and which site interacts with the binding partner. Thus we propose the intermolecular solute–solvent NOE, represented either by the NOE/ROE ratio or by the NOE enhancement, as an experimental means to actually map the influences of the cell and the other cell contents on the hydrated surface of the protein and thus elucidate the intracellular processes directly.
Conflicts of interest
There are no conflicts to declare.
Acknowledgements
We thank Dr Dennis Kurzbach for providing an exemplary experimental protein–water σNOE relaxation rate discussed in the introductory section and Fiona Kearns for proofreading the manuscript.
References
- P. Ball, Chem. Rev., 2008, 108, 74–108 CrossRef CAS.
- J. L. Finney, Faraday Discuss., 1996, 103, 1–18 RSC.
- R. J. Ellis, Trends Biochem. Sci., 2001, 26, 597–604 CrossRef CAS.
- A. P. Minton, J. Cell Sci., 2006, 119, 2863–2869 CrossRef CAS PubMed.
- S. K. Pal and A. H. Zewail, Chem. Rev., 2004, 104, 2099–2123 CrossRef CAS PubMed.
- B. D. Armstrong, J. Choi, C. Lopez, D. A. Wesener, W. Hubbell, S. Cavagnero and S. Han, J. Am. Chem. Soc., 2011, 133, 5987–5995 CrossRef CAS PubMed.
- J. H. Ortony, B. Qiao, C. J. Newcomb, T. J. Keller, L. C. Palmer, E. Deiss-Yehiely, M. O. de la Cruz, S. Han and S. I. Stupp, J. Am. Chem. Soc., 2017, 139, 8915–8921 CrossRef CAS PubMed.
- O. Fisette, C. Päslack, R. Barnes, J. M. Isas, R. Langen, M. Heyden, S. Han and L. V. Schäfer, J. Am. Chem. Soc., 2016, 138, 11526–11535 CrossRef CAS PubMed.
- N. V. Nucci, M. S. Pometun and A. J. Wand, Nat. Struct. Mol. Biol., 2011, 18, 245–249 CrossRef CAS PubMed.
- N. V. Nucci, M. S. Pometun and A. J. Wand, J. Am. Chem. Soc., 2011, 133, 12326 CrossRef CAS PubMed.
- C. R. Babu, P. F. Flynn and A. J. Wand, J. Am. Chem. Soc., 2001, 123, 2691–2692 CrossRef CAS PubMed.
- D. Braun, M. Schmollngruber and O. Steinhauser, J. Phys. Chem. Lett., 2017, 8, 3421 CrossRef CAS PubMed.
- J. M. Antosiewicz and D. Shugar, Biophys. Rev., 2016, 8, 151–161 CrossRef CAS PubMed.
- J. M. Antosiewicz and D. Shugar, Biophys. Rev., 2016, 8, 163–177 CrossRef CAS PubMed.
- L. H. Fornander, B. Feng, T. Beke-Somfai and B. Norden, J. Phys. Chem. B, 2014, 118, 9247–9257 CrossRef CAS PubMed.
- P. D. Cary, D. S. King, C. Crane-Robinson, E. M. Bradbury, A. Rabbani, G. H. Goodwin and E. W. Johns, Eur. J. Biochem., 1980, 112, 577–580 CrossRef CAS PubMed.
- S. Vijay-Kumar, C. E. Bugg, K. D. Wilkinson and W. J. Cook, Proc. Natl. Acad. Sci. U. S. A., 1985, 82, 3582–3585 CrossRef CAS.
- J. Jenson, G. Goldstein and E. Breslow, Biochim. Biophys. Acta, Protein Struct., 1980, 624, 378–385 CrossRef CAS.
- M. Noronha, J. C. Lima, M. Bastos, H. Santos and A. L. Macanita, Biophys. J., 2004, 87, 2609–2620 CrossRef CAS.
- I. Solomon, Phys. Rev., 1955, 99, 559 CrossRef CAS.
- G. Otting, E. Liepinsh and K. Wüthrich, Science, 1991, 254, 974–980 CrossRef CAS.
- G. Otting and K. Wüthrich, J. Am. Chem. Soc., 1989, 111, 1871–1875 CrossRef CAS.
- K. Wüthrich, G. Otting and E. Liepinsh, Faraday Discuss., 1992, 93, 35–45 RSC.
- G. Otting and E. Liepinsh, Acc. Chem. Res., 1995, 28, 171–177 CrossRef CAS.
- G. Otting, Prog. Nucl. Magn. Reson. Spectrosc., 1997, 31, 259–285 CrossRef CAS.
- S. Gabl, O. Steinhauser and H. Weingärtner, Angew. Chem., Int. Ed., 2013, 52, 9242–9246 CrossRef CAS PubMed.
- D. Braun and O. Steinhauser, Phys. Chem. Chem. Phys., 2015, 17, 8509–8517 RSC.
- V. P. Denisov and B. Halle, Faraday Discuss., 1996, 103, 227–244 RSC.
- B. Halle, J. Chem. Phys., 2003, 119, 12373 CrossRef.
- B. Halle, Philos. Trans. R. Soc. London, Ser. B, 2004, 359, 1207–1224 CrossRef CAS.
- K. Modig, E. Liepinsh, G. Otting and B. Halle, J. Am. Chem. Soc., 2004, 126, 102–114 CrossRef CAS.
- C. Mattea, J. Qvist and B. Halle, Biophys. J., 2008, 95, 2951–2963 CrossRef CAS PubMed.
- D. Frezzato, F. Rastrelli and A. Bagno, J. Phys. Chem. B, 2006, 110, 5676–5689 CrossRef CAS PubMed.
- S. Gabl, C. Schröder, D. Braun, H. Weingärtner and O. Steinhauser, J. Chem. Phys., 2014, 140, 184503 CrossRef.
- P. Honegger and O. Steinhauser, Phys. Chem. Chem. Phys., 2019, 21, 14571–14582 RSC.
- B. Halle, T. Andersson, S. Forsen and B. Lindman, J. Am. Chem. Soc., 1981, 103, 500–508 CrossRef CAS.
- V. P. Denisov and B. Halle, J. Mol. Biol., 1995, 245, 682–697 CrossRef CAS.
- B. Halle and G. Carlstöm, J. Phys. Chem., 1981, 85, 2142–2147 CrossRef CAS.
- G. Carlström and B. Halle, Langmuir, 1988, 4, 1346–1352 CrossRef.
- S. Vijay-Kumar, C. E. Bugg and W. J. Cook, J. Mol. Biol., 1987, 194, 531–544 CrossRef CAS.
- C. Jorge, B. S. Marques, K. G. Valentine and A. J. Wand, Methods Enzymol., 2019, 615, 77 Search PubMed.
-
P. Kadeřávek, F. Ferrage, G. Bodenhausen and D. Kurzbach, Chem. – Eur. J., 2018, 24, 13418–13423.
- M. D. Fayer and N. E. Levinger, Annu. Rev. Anal. Chem., 2010, 3, 89–107 CrossRef CAS PubMed.
- I. Yu, T. Mori, T. Ando, R. Harada, J. Jung, Y. Sugita and M. Feig, eLife, 2016, 5, e19274 CrossRef PubMed.
- G. Nawrocki, A. Karaboga, Y. Sugita and M. Feig, Phys. Chem. Chem. Phys., 2019, 21, 876–883 RSC.
- P. N. Gallo, J. C. Iovine and N. V. Nucci, Methods, 2018, 148, 146–153 CrossRef CAS PubMed.
- S. J. Wodak and J. Janin, Adv. Prot. Chem., 2002, 61, 9–73 CrossRef PubMed.
- R. Wolfenden, Biophys. Chem., 2003, 105, 559–572 CrossRef CAS.
- B. Q. Wei, W. A. Baase, L. H. Weaver, B. W. Matthews and B. K. Shoichet, J. Mol. Biol., 2002, 322, 339–355 CrossRef CAS.
- P. Honegger and O. Steinhauser, Phys. Chem. Chem. Phys., 2018, 20, 22932–22945 RSC.
- M. Roos, M. Hofmann, S. Link, M. Ott, J. Balbach, E. Rössler, K. Saalwächter and A. Krushelnitsky, J. Biomol. NMR, 2015, 63, 403–415 CrossRef CAS PubMed.
- T. Blochowicz, C. Tschirwitz, S. Benkhof and E. Rössler, J. Chem. Phys., 2003, 118, 7544–7555 CrossRef CAS.
- A. Hershko and A. Ciechanover, Annu.
Rev. Biochem., 1998, 67, 425–479 CrossRef CAS PubMed.
- P. Peschard, G. Kozlov, T. Lin, I. A. Mirza, A. M. Berghuis, S. Lipkowitz, M. Park and K. Gehring, Mol. Cell, 2007, 27, 474–485 CrossRef CAS PubMed.
- H. Berman, K. Henrick and H. Nakamura, Nat. Struct. Biol., 2003, 10, 980 CrossRef CAS PubMed.
- D. Beglov and B. Roux, J. Chem. Phys., 1994, 100, 9050 CrossRef CAS.
- A. D. MacKerell Jr., D. Bashford, M. Bellott, R. L. Dunbrack Jr., J. D. Evanseck, M. J. Field, S. Fischer, J. Gao, H. Guo, S. Ha, D. Joseph-McCarthy, L. Kuchnir, K. Kuczera, F. T. K. Lau, C. Mattos, S. Michnick, T. Ngo, D. T. Nguyen, B. Prodhom, W. E. Reiher, B. Roux, M. Schienkrich, J. C. Smith, R. Stote, J. Straub, M. Watanabe, J. Wlorklewicz-Kuczera, D. Yin and M. Karplus, J. Phys. Chem. B, 1998, 102(18), 3586–3616 CrossRef.
- A. D. MacKerell Jr., M. Feig and C. L. Brooks III, J. Am. Chem. Soc., 2004, 126, 698–699 CrossRef.
- R. B. Best, X. Zhu, J. Shim, P. E. Lopes, J. Mittal, M. Feig and A. D. MacKerell Jr., J. Chem. Theory Comput., 2012, 8(9), 3257–3273 CrossRef CAS PubMed.
- H. J. C. Berendsen, J. R. Grigera and T. P. Straatsma, J. Phys. Chem., 1987, 91, 6269 CrossRef CAS.
- D. Braun, S. Boresch and O. Steinhauser, J. Chem. Phys., 2014, 140, 064107 CrossRef PubMed.
- M. Sega and C. Schröder, J. Phys. Chem. A, 2015, 119, 1539–1547 CrossRef CAS PubMed.
- M. Tarek and D. J. Tobias, Biophys. J., 2000, 79, 3244–3257 CrossRef CAS PubMed.
- M. Marchi, F. Sterpone and M. Ceccarelli, J. Am. Chem. Soc., 2002, 124, 6787–6791 CrossRef CAS PubMed.
- G. Schiro, F.-X. G. Y. Fichou, K. Wood, F. Gabel, M. Moulin, M. Härtlein, M. Heyden, J.-P. Colletier, A. Orecchini, A. Paciaroni, J. Wuttke, D. J. Tobias and M. Weik, Nat. Commun., 2015, 6, 6490 CrossRef CAS.
- L. D. Schuler, X. Daura and W. F. V. Gunsteren, J. Comput. Chem., 2001, 22, 1205–1218 CrossRef CAS.
- M. Schmollngruber, D. Braun, D. Oser and O. Steinhauser, Phys. Chem. Chem. Phys., 2016, 18, 3606–3617 RSC.
- M. Schmollngruber, D. Braun and O. Steinhauser, J. Chem. Phys., 2016, 145, 214702 CrossRef PubMed.
- D. Braun, M. Schmollngruber and O. Steinhauser, Phys. Chem. Chem. Phys., 2016, 18, 24620–24630 RSC.
- I. Dodevski, N. V. Nucci, K. G. Valentine, G. K. Sidhu, E. S. O'Brien, A. Pardi and A. J. Wand, J. Am. Chem. Soc., 2014, 136, 3465–3474 CrossRef CAS PubMed.
- I. Dodevski, N. V. Nucci, K. G. Valentine, G. K. Sidhu, E. S. O'Brien, A. Pardi and A. J. Wand, J. Magn. Reson., 2014, 241, 137–147 CrossRef PubMed.
- P. Honegger, M. Schmollngruber and O. Steinhauser, Phys. Chem. Chem. Phys., 2018, 20, 11454–11469 RSC.
- R. B. Best, W. Zheng and J. Mittal, J. Chem. Theory Comput., 2014, 10, 5113–5124 CrossRef CAS PubMed.
- D. Petrov and B. Zagrovic, PLoS Comput. Biol., 2014, 10, e1003638 CrossRef.
- L. Martinez, R. Andrade, E. G. Birgin and J. M. Martinez, J. Comput. Chem., 2009, 30(13), 2157–2164 CrossRef CAS PubMed.
- B. R. Brooks, C. L. Brooks III, A. D. MacKerell Jr, L. Nilsson, R. J. Petrella, B. Roux, Y. Won, G. Archontis, C. Bartels, S. Boresch, A. Caflisch, L. Caves, Q. Cui, A. R. Dinner, M. Feig, S. Fischer, J. Gao, M. Hodoscek, W. Im, K. Kuczera, T. Lazaridis, J. Ma, V. Ovchinnikov, E. Paci, R. W. Pastor, C. B. Post, J. Z. Pu, M. Schaefer, B. Tidor, R. M. Venable, H. L. Woodcock, X. Wu, W. Yang, D. M. York and M. Karplus, J. Comput. Chem., 2009, 30, 1545 CrossRef CAS PubMed.
- A.-P. Hynninen and M. F. Crowley, J. Comput. Chem., 2014, 35(5), 406–413 CrossRef CAS PubMed.
- S. Nose, J. Chem. Phys., 1984, 81, 511 CrossRef CAS.
- W. G. Hoover, Phys. Rev. A: At., Mol., Opt. Phys., 1985, 31, 1695 CrossRef PubMed.
- R. W. Hockney, Methods Comput. Phys., 1970, 9, 136 Search PubMed.
- J.-P. Ryckaert, G. Ciccotti and H. J. C. Berendsen, J. Comput. Phys., 1977, 23, 327 CrossRef CAS.
- T. Darden, D. York and L. Pedersen, J. Chem. Phys., 1993, 98, 10089 CrossRef CAS.
- U. Essmann, L. Perera, M. L. Berkowitz, T. Darden, H. Lee and L. Pedersen, J. Chem. Phys., 1995, 103, 8577 CrossRef CAS.
- N. Michaud-Agrawal, E. J. Denning, T. B. Woolf and O. Beckstein, J. Comput. Chem., 2011, 32, 2319–2327 CrossRef CAS PubMed.
-
R. J. Gowers, M. Linke, J. Barnoud, T. J. E. Reddy, M. N. Melo, S. L. Seyler, D. L. Dotson, J. Domanski, S. Buchoux, I. M. Kenney and O. Beckstein, Proceedings of the 15th Python in Science Conference, 2016, pp. 102–109.
- W. I. Sundquist, H. L. Schubert, B. N. Kelly, G. C. Hill, J. M. Holton and C. P. Hill, Mol. Cell, 2004, 13, 783–789 CrossRef CAS PubMed.
- H. Teo, D. B. Veprintsev and R. L. Williams, J. Biol. Chem., 2004, 279, 28689–28696 CrossRef CAS PubMed.
- G. Prag, S. Lee, R. Mattera, C. N. Arighi, B. M. Beach, J. S. Bonifacino and J. H. Hurley, Proc. Natl. Acad. Sci. U. S. A., 2005, 102, 2334–2339 CrossRef CAS PubMed.
- M. Candotti and M. Orozco, PLoS Comput. Biol., 2016, 12, e1005040 CrossRef PubMed.
- M. Feig and Y. Sugita, J. Phys. Chem. B, 2012, 116, 599–605 CrossRef CAS PubMed.
- M. Senske, L. Törk, B. Born, M. Havenith, C. Herrmann and S. Ebbinghaus, J. Am. Chem. Soc., 2014, 136, 9036–9041 CrossRef CAS PubMed.
- M. Roos, M. Ott, M. Hofmann, S. Link, E. Rössler, J. Balbach, A. Krushelnitsky and K. Saalwächter, J. Am. Chem. Soc., 2016, 138, 10365–10372 CrossRef CAS PubMed.
- D. Hall and A. Minton, Biochim. Biophys. Acta, 2003, 1649, 127–139 CrossRef CAS.
- H.-X. Zhou, Arch. Biochem. Biophys., 2008, 469, 76–82 CrossRef CAS PubMed.
- A. V. Martinez, E. Malolepsza, L. Dominguez, Q. Lu and J. E. Straub, J. Phys. Chem. B, 2014, 119, 9084–9090 CrossRef.
- B. van den Berg, R. J. Ellis and C. M. Dobson, EMBO J., 1999, 18, 6927–6933 CrossRef CAS.
- L. A. Munishkina, E. M. Cooper, V. N. Uversky and A. L. Fink, J. Mol. Recognit., 2004, 17, 456–464 CrossRef CAS.
- H. X. Zhou, G. Rivas and A. P. Minton, Annu. Rev. Biophys., 2008, 37, 375–397 CrossRef CAS PubMed.
- A. Patra, T. Q. Luong, R. K. Mitra and M. Havenith, Phys. Chem. Chem. Phys., 2014, 16, 12875–12883 RSC.
- T. Kinugasaa, A. Kondo, S. Nishimuraa, Y. Miyauchia, Y. Nishii, K. Watanabe and H. Takeuchi, Colloids Surf., A, 2002, 204, 193–199 CrossRef.
- S. Abel, F. Sterpone, S. Bandyopadhyay and M. Marchi, J. Phys. Chem. B, 2004, 108, 19458–19466 CrossRef CAS.
- J. Faeder and B. M. Ladanyi, J. Phys. Chem. B, 2000, 104, 1033–1046 CrossRef CAS.
- P. A. Pieniazek, Y.-S. Lin, J. Chowdhary, B. M. Ladanyi and J. L. Skinner, J. Phys. Chem. B, 2009, 113, 15017–15028 CrossRef CAS PubMed.
- E. Negro, R. Latsuzbaia, A. H. de Vries and G. J. M. Koper, Phys. Chem. Chem. Phys., 2014, 10, 8685–8697 CAS.
- M. H. H. Pomata, D. Laria, M. S. Skaf and M. D. Elola, J. Chem. Phys., 2008, 129, 244503 CrossRef.
- E. N. Brodskaya and G. V. Mudzhikova, Mol. Phys., 2006, 104, 3635 CrossRef CAS.
- D. Cringus, A. Bakulin, J. Lindner, P. Vöhringer, M. S. Pshenichnikov and D. A. Wiersma, J. Phys. Chem. B, 2007, 111, 14193–14207 CrossRef CAS PubMed.
- P. Zinsli, J. Phys. Chem., 1979, 83, 3223–3231 CrossRef CAS.
- M. D'Angelo, D. Fioretto, G. Onori, L. Palmieri and A. Santucci, Phys. Rev. E: Stat. Phys., Plasmas, Fluids, Relat. Interdiscip. Top., 1995, 52, 4620 CrossRef PubMed.
- M. D'Angelo, D. Fioretto, G. Onori and A. Santucci, J. Mol. Struct., 1996, 383, 157–163 CrossRef.
- M. D'Angelo, D. Fioretto, G. Onori, L. Palmieri and A. Santucci, Phys. Rev. E: Stat. Phys., Plasmas, Fluids, Relat. Interdiscip. Top., 1996, 54, 993 CrossRef PubMed.
- D. Fioretto, M. Freda, S. Mannaioli, G. Onori and A. Santucci, J. Phys. Chem. B, 1999, 103, 2631–2635 CrossRef CAS.
- M. Freda, G. Onori, A. Paciaroni and A. Santucci, J. Mol. Liq., 2002, 101, 55–68 CrossRef CAS.
- M. Freda, G. Onori, A. Paciaroni and A. Santucci, Phys. Rev. E: Stat.,
Nonlinear, Soft Matter Phys., 2003, 68, 021406 CrossRef PubMed.
-
S. Balakrishnan, Structure and dynamics of fluids in confinement: a case study of water, protein and ionic liquid in reverse micelles and microemulsions, Ruhr-Universität Bochum, 2007 Search PubMed.
- M. Belletete, M. Lachapelle and G. Durocher, J. Phys. Chem., 1990, 94, 5337–5341 CrossRef CAS.
- J. Faeder and B. M. Ladanyi, J. Phys. Chem. B, 2001, 105, 11148–11158 CrossRef CAS.
- H. Workman and P. F. Flynn, J. Am. Chem. Soc., 2009, 131, 3806–3807 CrossRef CAS PubMed.
- S. Mukherjee, P. Chowdhury and F. Gai, J. Phys. Chem. B, 2006, 110, 11615–11619 CrossRef CAS.
- S. Abel, M. Waks, W. Urbach and M. Marchi, J. Am. Chem. Soc., 2006, 128, 382–383 CrossRef CAS.
- A. V. Martinez, S. C. DeSensi, L. Dominguez, E. Rivera and J. E. Straub, J. Chem. Phys., 2011, 134, 055107 CrossRef PubMed.
- S. Abel, M. Waks and M. Marchi, Eur. Phys. J. E: Soft Matter Biol. Phys., 2010, 32, 399 CrossRef CAS PubMed.
- J. Tian and A. E. Garcia, J. Chem. Phys., 2011, 134, 225101 CrossRef PubMed.
- A. Shioi, M. Harada, H. Takahashi and M. Adachi, Langmuir, 1997, 13, 609–616 CrossRef CAS.
- R. W. Peterson, M. S. Pometun, Z. Shi and A. J. Wand, J. Protein Sci., 2005, 14, 2919–2921 CrossRef CAS PubMed.
- Z. Shi, R. W. Peterson and A. J. Wand, Langmuir, 2005, 21, 10632–10637 CrossRef CAS PubMed.
- W. D. V. Horn, M. E. Oglivie and P. F. Flynn, J. Am. Chem. Soc., 2009, 131, 8030 CrossRef PubMed.
- W. D. V. Horn, A. K. Simorellis and P. F. Flynn, J. Am. Chem. Soc., 2005, 127, 13553 CrossRef PubMed.
- A. K. Simorellis and P. F. Flynn, J. Am. Chem. Soc., 2006, 128, 9580–9581 CrossRef CAS PubMed.
- E. P. Melo, C. M. L. Carvalho, M. R. Aires-Barros, S. M. B. Costa and J. M. S. Cabral, Biotechnol. Bioeng., 1998, 58, 380–386 CrossRef CAS PubMed.
- E. P. Melo, R. P. Baptista and J. M. S. Cabral, J. Mol. Catal. B: Enzym., 2003, 22, 299–306 CrossRef CAS.
- E. P. Melo, S. M. Costa, J. M. S. Cabral, P. Fojan and S. B. Petersen, Phys. Lipids, 2003, 124, 37–47 CrossRef CAS.
- C. Olsson, H. Jansson and J. Swenson, J. Phys. Chem. B, 2016, 120, 4723–4731 CrossRef CAS PubMed.
- D. Corradini, E. G. Strekalova, H. E. Stanley and P. Gallo, Sci. Rep., 2013, 3, 1218 CrossRef PubMed.
Footnote |
† Electronic supplementary information (ESI) available. See DOI: 10.1039/c9cp04752b |
|
This journal is © the Owner Societies 2020 |
Click here to see how this site uses Cookies. View our privacy policy here.