DOI:
10.1039/C8SC03831G
(Edge Article)
Chem. Sci., 2019,
10, 542-547
A computationally designed binding mode flip leads to a novel class of potent tri-vector cyclophilin inhibitors†
Received
27th August 2018
, Accepted 14th October 2018
First published on 23rd October 2018
Abstract
Cyclophilins (Cyps) are a major family of drug targets that are challenging to prosecute with small molecules because the shallow nature and high degree of conservation of the active site across human isoforms offers limited opportunities for potent and selective inhibition. Herein a computational approach based on molecular dynamics simulations and free energy calculations was combined with biophysical assays and X-ray crystallography to explore a flip in the binding mode of a reported urea-based Cyp inhibitor. This approach enabled access to a distal pocket that is poorly conserved among key Cyp isoforms, and led to the discovery of a new family of sub-micromolar cell-active inhibitors that offer unprecedented opportunities for the development of next-generation drug therapies based on Cyp inhibition. The computational approach is applicable to a broad range of organic functional groups and could prove widely enabling in molecular design.
Introduction
In the absence of innovative treatments, the societal burden of neurodegenerative disorders is projected to grow substantially over the next few decades worldwide. A growing body of evidence points to the central role of the opening of the mitochondrial permeability transition pore (mPTP) in neurodegenerative cell death.1 The enzyme cyclophilin D (CypD) is an essential regulator of the mPTP opening, and blockage of CypD function is neuroprotective in models of Alzheimer's and Parkinson's diseases.2–4 Besides neurodegenerative disorders, CypD inhibition is protective in animal models of acute kidney injury, a condition that accounts for a fifth of emergency hospitalizations in the USA.5 Other related cyclophilin isoforms CypA and CypB are overexpressed in malignant cancers,6 and CypA inhibition has potent antiviral and anti-inflammatory effects.7 Cyp inhibition may also protect the liver from fibrosis subsequent to non-alcoholic steatohepatitis (NASH), a common indication for liver transplantation after chronic hepatitis C.8
The majority of existing Cyp inhibitors are derived from the natural products cyclosporin A (CsA) and sanglifehrin A.9 These scaffolds achieve potent inhibition but are complex to synthesize, lack sub-type selectivity, show undesirable side-effects, and have poor CNS activity. Extensive efforts have been pursued to simplify these scaffolds into more synthetically tractable variants.10–13 There is also considerable interest in developing small molecule Cyp inhibitors that may be more easily optimized for use as therapeutics.14–20 However, there are few reports of well characterized small molecules that bind strongly to Cyps.21,22 Current small molecule design strategies typically feature linear (thio)ureas15,23 or amides24 as scaffolds. Compound 1 provides an example that exploits interactions with residues in the highly conserved Pro and Abu pockets (Fig. 1A). Fig. 1A also highlights a less conserved distal three o'clock pocket that is thought to offer prospects for potency and selectivity improvements,25 but to date it is unclear how this pocket can be engaged using current scaffolds.
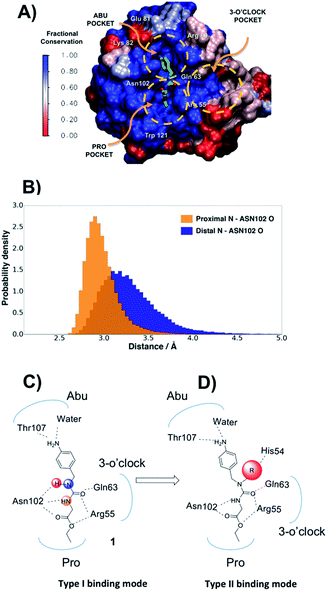 |
| Fig. 1 (A) Surface representation of the PPIAse domain coloured by residue conservation across human Cyp A–D isoforms. The location of Abu, Pro and 3 o'clock pockets are highlighted and compound 1 is shown in coloured sticks. (B) Distribution of distances between urea nitrogen atoms in 1 and Asn102 backbone oxygen observed in MD simulations. (C) Interactions of 1 with CypA residues in a canonical type-I binding mode. (D) Hypothesized type-II binding mode for alkylated urea variants of 1. | |
Herein we report a multidisciplinary approach to redesigning linear Cyp inhibitor scaffolds into the first class of tri-vector inhibitors that simultaneously target the Pro, Abu and three o'clock pockets.
Results and discussion
Molecular dynamics simulations suggest a new ligand optimisation strategy
The protein X-ray structure of 1 in complex with CypA suggests that both urea nitrogen atoms are hydrogen-bonded to the backbone oxygen of Asn102.21 However, molecular dynamics (MD) simulations revealed that the nitrogen atom distal to the ester moiety in 1 is only weakly interacting with Asn102 and readily moves away from this residue (Fig. 1B, C and S1†), suggesting that alkylation of that nitrogen to introduce a new vector in the scaffold could be tolerated. Ab initio calculations on model ureas suggest a modest energetic preference for the Z,Z urea conformer.26 This led to the hypothesis that a suitably chosen R group could stabilize an alkylated urea in an E,Z conformer able to adopt a novel type-II binding mode that would enable access to the 3 o'clock pocket (Fig. 1D).
A general synthetic route to tri-vector cyclophilin ligands
The tri-vector design hypothesis was pursued by synthesis of a series of alkylated urea ester ligands. The majority of the compounds were prepared according to Scheme 1 (steps a–d, see ESI† for details). Substituted amino derivatives were typically prepared from 4-nitrophenyl derivatives by reductive amination,27 or nucleophilic substitution.28 The urea moiety was prepared by condensation of the resulting amines with ethyl isocyanatoacetate,15 and the desired products were obtained by subsequent reduction of the nitro group.29
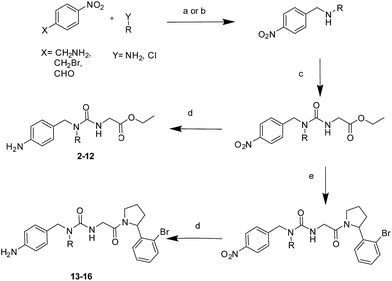 |
| Scheme 1 (a) NaBH4, EtOH, RT, 24 hours, yields: 93–95%; (b) K2CO3, MeCN, 70 °C, 6 hours, yields: 95–99%; (c) ethyl isocyanatoacetate, DCM, RT, overnight, yields: 81–99%; (d) Fe, CaCl2, EtOH/H2O, 90 °C, 4 hours, yields: 17–98%. (e) LiOH·H2O, THF/MeOH/H2O, RT, 2 hours, then 2-(2-bromophenyl)-pyrrolidine, HATU, DIPEA, DMF, RT, overnight, yields: 9–46% over two steps. | |
A free-energy perturbation (FEP) protocol guides the selection of substituents
MD simulations cannot readily sample the desired type-I to type-II binding mode flip because rotational barriers of ca. 15 kcal mol−1 separate Z,Z and E,Z conformers.30 Free Energy Perturbation (FEP) calculation methods have shown potential for optimization of ligand potency and selectivity.31–38 Here the selection of potential R groups was guided by a novel FEP protocol designed to compute the energetics of this binding mode flip (Fig. 2A). The setup, execution and analysis of the FEP calculations were carried out with the software FESetup,39 SOMD,40–42 and freenrgworkflows.43 The selection of R groups for computational and experimental evaluation was driven by the following considerations: lack of obvious steric or electrostatic mismatches, polar or non-polar interactions with 3 o'clock pocket residues, ease of synthesis, diversity of structure–activity relationships, and validation of FEP-calculated energetics.
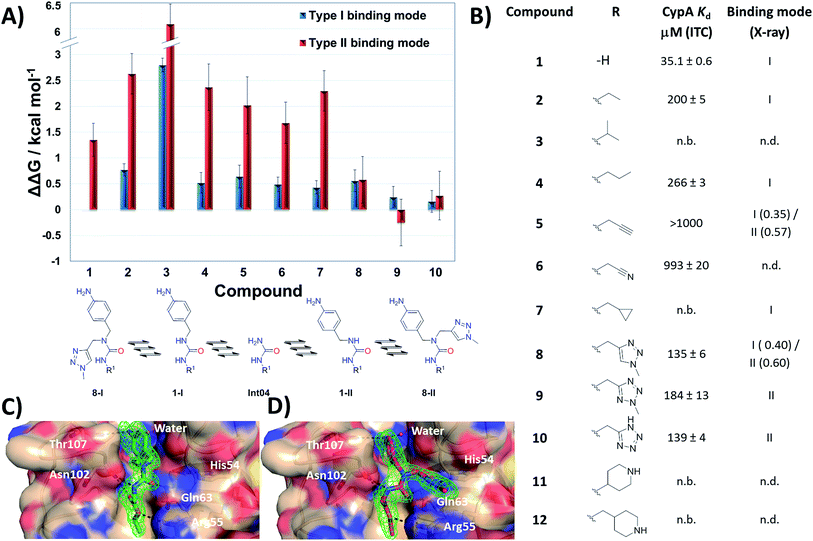 |
| Fig. 2 (A) FEP-calculated binding energetics. The figures are relative to 1 in a type-I binding mode and were obtained from automated analysis of FEP maps (Fig. S2†). A partial map depicted below the table illustrates how molecule Int04 is used to connect other compounds in a type-I or II binding mode. (B) ITC-derived dissociation constants and observed binding mode in X-ray crystallography derived CypA structures. Uncertainties on Kd values from fit to a one-site binding model. Partial occupancies are given for compounds refined in multiple binding modes. n.b.: no binding observed at the maximum concentration tested. n.d.: not determined. (C) X-ray crystal structure of CypA: 2 depicting a type-I binding mode. (D) X-ray crystal structure of CypA: 9 depicting a type-II binding mode. Fo − Fc electron density omit maps are shown as a green mesh at 2.5σ contour (see ESI† for details). | |
Compound 1 was predicted to favour a type-I binding mode by ca. 1 kcal mol−1, in line with the preferences observed in X-ray structures (Fig. 2A).21 Non-polar substitutions (2, 3, 4, 5, 7) were predicted to worsen free energies of binding relative to 1 and maintain preference for a type-I binding mode. By contrast nitrogen-rich 5-membered rings (8, 9, 10) were predicted to show a similar or slight preference for type-II binding mode.
Ligand binding to CypA was characterized by isothermal titration calorimetry (ITC) measurements (Fig. 2B). Substitutions with small linear alkyl groups (2, 4) were tolerated but weakened binding, whereas branched alkyls (3) were inactive, in line with the FEP calculations. Alkyne, cyano and cyclopropyl derivatives (5, 6, 7) were computed to bind with potencies comparable to linear alkyl groups, but turned out to be very weak binders in the ITC assay. Nitrogen rich five-membered rings (8, 9, 10) showed more favourable binding constants, in line with the FEP calculations. Piperidines 11 and 12 had not been evaluated by FEP calculations and turned out to be inactive.
Evidence of binding mode preferences was sought by soaking CypA crystals with compounds 2–12. Seven X-ray structures of CypA complexes were determined. Compounds 2, 4, 7 were found to adopt a type-I binding mode in line with the FEP calculations (Fig. 2C and ESI†). Compound 5 was observed to adopt dual occupancy, whereas the FEP calculations anticipated a type-I binding mode. Compound 8 was observed to adopt dual occupancy, whereas 9 and 10 were only observed in a type-II binding mode in the X-ray refined structures. The FEP calculations suggest both binding modes are feasible for these three compounds. In 8–10 the type-II binding mode is stabilized by hydrogen-bonding interactions between nitrogen atoms in the five-membered rings and His54. This binding mode flip from type-I to type-II is accompanied by a slight rearrangement in the orientation of the urea carbonyl to maintain optimal placement of the aniline ring in the Abu pocket. Strikingly the 5-membered ring methyl groups in 8 and 9 point into the 3 o'clock pocket of CypA and provide a clear vector for further derivatization (Fig. 2D).
The tri-vector design generalises to other ligand families and Cyp isoforms
With the binding mode flip hypothesis validated for the urea ester series in CypA, attention turned to transferability of the strategy to other ligand families and Cyp isoforms. FEP calculations suggested that a type-II binding mode preference was maintained for nitrogen-rich heterocycles in the context of a urea arylpyrrolidine scaffold for both CypA and CypD isoforms (Fig. S3–S5†).21 Thus alkylated variants 13–16 were prepared from 4-nitrophenyl ester intermediates (Scheme 1, steps e and d). Compounds 13–15 feature similar R groups to 5, 8 and 9, whereas 16 was prepared to assess viability of further extension into the three o'clock pocket via appendage of an ester group to a triazole. The X-ray crystallography derived structure of 15 in complex with CypA confirmed that the type-II binding mode has been maintained (Fig. 3A). Thus 13–16 were assayed by surface plasmon resonance (SPR) against CypA, CypB and CypD isoforms. The compounds bind to all three isoforms with Kd values in the low μM to mid nM range (Fig. 3B). Compound 15 binds particularly well to CypD, with a potency (ca. 70 nM) approaching that of CsA (ca. 20 nM). For comparison the unsubstituted (R = H) urea has been reported to inhibit CypD with an IC50 of 1.1 ± 0.2 μM.15 Although 13–15 only access the entrance of the three o'clock pocket, the SPR assay suggests this is sufficient to endow a degree of isoform selectivity. Compound 16 binds slightly worse to CypA and CypB but modestly better to CypD with respect to 14. Thus further lead optimization efforts to tune desired selectivity profiles appear auspicious.
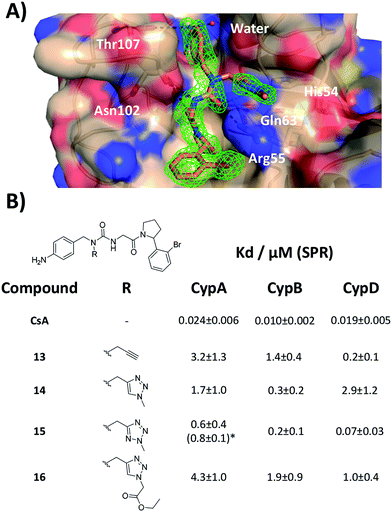 |
| Fig. 3 (A) X-ray crystal structure of CypA in complex with 15. (B) SPR-derived dissociation constants for alkylated urea arylpyrrolidine ligands. Values are the mean ± 1σ from three repeat experiments. * ITC-derived Kd value (see ESI† for details). | |
Tri-vector ligands show improved efficacy and decreased toxicity over cyclosporine in cell assays
Additional evidence for the potential of this tri-vector design was sought by evaluating 13–15 in cellular assays. CypA has previously been shown to be necessary for the prolactin-induced activation of Janus-activated kinase 2 in human breast cancer cells, with CsA treatment causing inhibition of growth of the triple negative MDA-MB-231 breast-cancer cell line.44 Compounds 13 and 15 showed evidence of dose-dependent growth inhibition at low micromolar concentration resulting in GI50 values similar (13) or two-fold better (15) than those measured for CsA. By contrast 14 was inactive (Fig. 4A and B). Thus alkylation of the urea moiety does not appear to prevent cell penetration. Negligible cell death was observed for compounds 13 and 15 suggesting low toxicity in comparison with CsA. This indicates that in MDA-MB-231_NLG cells growth inhibition by compounds 13 and 15 is due to reduced proliferation, whereas growth inhibition by CsA is also a consequence of cell death (Fig. 4C). Additionally 13–15 showed no growth inhibition or induction of cell death in the non-tumorigenic fibroblast IMR-90 cell line. By contrast CsA induced significant growth inhibition and cell death (Fig. 4D).
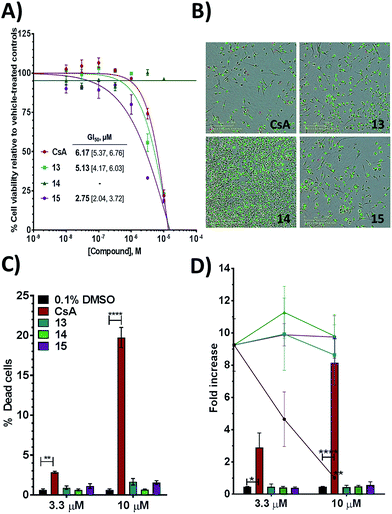 |
| Fig. 4 (A) Growth inhibition for MDA-MB-231_NLG cells and mean GI50 with 95% CI [lower limit, upper limit]. (B) MDA-MB-231_NLG cells after 120 hours treatment; red nuclei indicate dead cells. Area shown is 0.64 mm2, scale bar is 200 μm. (C) % dead MDA-MB-231_NLG cells. (D) Fold increase of IMR-90 cell confluence (line graphs) and number of dead IMR-90 cells (bar graphs). Values in panels A, C, D represent mean ± SEM of three independent experiments each performed in triplicate with 120 hours of treatment. | |
Thus, further evaluation of the present tri-vector Cyp inhibitors in a range of in vitro and in vivo disease models implicating Cyps seems to be warranted, particularly in instances where CsA toxicity and off-target effects have been a cause for concern. Alternatives to the aniline moiety in 15 to alleviate toxicity concerns have been suggested elsewhere.21,45 Immunosuppression via inhibition of calcineurin phosphatase activity is a well-known feature of CsA. The tri-vector Cyp inhibitors are unlikely to possess this capability given that the parent urea-arylpyrrolidine compounds they are derived from lack calcineurin inhibition properties.21
Conclusions
In summary, this work has validated a strategy for the redesign of existing cyclophilin inhibitors into a novel class of inhibitors that can simultaneously target three pockets on the surfaces of cyclophilins. This breakthrough has led to significantly more potent cyclophilin inhibitors and also opens up new horizons to optimise isoform-selectivity. Nanomolar acylurea-based cyclophilin inhibitors have been reported previously,46 but subsequent work was unable to reproduce these findings or to detect evidence of binding to cyclophilins for these molecules.21 Members of the tri-vector class of cyclophilin inhibitors (13, 15) show improved efficacy and reduced toxicity in cell assays over the drug cyclosporine A. They are thus already attractive leads for new drug therapies based on Cyp inhibition. To our knowledge this is the first example of the validation of an FEP methodology to computationally design a large scale binding mode flip in a protein–ligand complex. The approach is readily applicable to a broad range of commonly encountered organic functional groups that exhibit E/Z conformational equilibria. We anticipate that this methodology may be of widespread utility to facilitate ligand optimisations in structure-based drug design efforts.
Conflicts of interest
There are no conflicts to declare.
Acknowledgements
JM is supported by a University Research Fellowship from the Royal Society. The research leading to these results has received funding from the European Research Council under the European Union's Seventh Framework Programme (FP7/2007–2013)/ERC grant agreement no. 336289 and Horizon 2020 Marie Sklodowska-Curie grant agreement no. 655667 awarded to JJJ. This work was supported by Wellcome Trust Multi-User Equipment grant 101527/Z/13/Z and the Diamond Light Source for beamtime (BAG proposals MX13550 and MX18515). This project made use of software and facilities provided by CCPBioSim and HECBioSim supported by EPSRC (grants no. EP/M022609/1 and EP/L000253/1).
Notes and references
- T. Nakagawa, S. Shimizu, T. Watanabe, O. Yamaguchi, K. Otsu, H. Yamagata, H. Inohara, T. Kubo and Y. Tsujimoto, Nature, 2005, 434, 652–658 CrossRef CAS PubMed.
- H. Du, L. Guo, F. Fang, D. Chen, A. A. Sosunov, G. M. McKhann, Y. Yan, C. Wang, H. Zhang, J. D. Molkentin, F. J. Gunn-Moore, J. P. Vonsattel, O. Arancio, J. X. Chen and S. D. Yan, Nat. Med., 2008, 14, 1097–1105 CrossRef CAS PubMed.
- M. R. H. Ludtmann, P. R. Angelova, M. H. Horrocks, M. L. Choi, M. Rodrigues, A. Y. Baev, A. V. Berezhnov, Z. Yao, D. Little, B. Banushi, A. S. Al-Menhali, R. T. Ranasinghe, D. R. Whiten, R. Yapom, K. S. Dolt, M. J. Devine, P. Gissen, T. Kunath, M. Jaganjac, E. V. Pavlov, D. Klenerman, A. Y. Abramov and S. Gandhi, Nat. Commun., 2018, 9, 2293 CrossRef PubMed.
- J. Lee, Bioorg. Med. Chem. Lett., 2016, 26, 714–720 CrossRef CAS PubMed.
- R. Murugan and J. A. Kellum, Nat. Rev. Nephrol., 2011, 7, 209–217 CrossRef CAS PubMed.
- P. Lavin and M. Mc Gee, Curr. Mol. Pharmacol., 2015, 9, 148–164 CrossRef PubMed.
- H. Tang, Viruses, 2010, 2, 1621–1634 CrossRef CAS PubMed.
- Z. Younossi, Q. M. Anstee, M. Marietti, T. Hardy, L. Henry, M. Eslam, J. George and E. Bugianesi, Nat. Rev. Gastroenterol. Hepatol., 2018, 15, 11–20 CrossRef PubMed.
- Z. K. Sweeney, J. Fu and B. Wiedmann, J. Med. Chem., 2014, 57, 7145–7159 CrossRef CAS PubMed.
- B. M. Dunyak and J. E. Gestwicki, J. Med. Chem., 2016, 59, 9622–9644 CrossRef CAS PubMed.
- V. A. Steadman, S. B. Pettit, K. G. Poullennec, L. Lazarides, A. J. Keats, D. K. Dean, S. J. Stanway, C. A. Austin, J. A. Sanvoisin, G. M. Watt, H. G. Fliri, A. C. Liclican, D. Jin, M. H. Wong, S. A. Leavitt, Y. J. Lee, Y. Tian, C. R. Frey, T. C. Appleby, U. Schmitz, P. Jansa, R. L. Mackman and B. E. Schultz, J. Med. Chem., 2017, 60, 1000–1017 CrossRef CAS PubMed.
- R. Sedrani, J. Kallen, L. M. M. Cabrejas, C. D. Papageorgiou, F. Senia, S. Rohrbach, D. Wagner, B. Thai, A. M. J. Eme, J. France, L. Oberer, G. Rihs, G. Zenke and J. Wagner, J. Am. Chem. Soc., 2003, 125, 3849–3859 CrossRef CAS PubMed.
- X. Y. Wu, J. L. Stockdill, P. Wang and S. J. Danishefsky, J. Am. Chem. Soc., 2010, 132, 4098–4100 CrossRef CAS PubMed.
- J. Li, Z. Tan, S. Tang, I. Hewlett, R. Pang, M. He, S. He, B. Tian, K. Chen and M. Yang, Bioorg. Med. Chem., 2009, 17, 3177–3188 CrossRef CAS PubMed.
- E. R. Shore, M. Awais, N. M. Kershaw, R. R. Gibson, S. Pandalaneni, D. Latawiec, L. Wen, M. A. Javed, D. N. Criddle, N. Berry, P. M. O'Neill, L. Y. Lian and R. Sutton, J. Med. Chem., 2016, 59, 2596–2611 CrossRef CAS PubMed.
- S. Daum, M. Schumann, S. Mathea, T. Aumuller, M. A. Balsley, S. L. Constant, B. F. de Lacroix, F. Kruska, M. Braun and C. Schiene-Fischer, Biochemistry, 2009, 48, 6268–6277 CrossRef CAS PubMed.
- K. R. Valasani, Q. Sun, D. Fang, Z. Zhang, Q. Yu, Y. Guo, J. Li, A. Roy and S. ShiDu Yan, ACS Med. Chem. Lett., 2016, 7, 294–299 CrossRef CAS PubMed.
- K. R. Valasani, J. R. Vangavaragu, V. W. Day and S. S. Yan, J. Chem. Inf. Model., 2014, 54, 902–912 CrossRef CAS PubMed.
- M. Nagaraju, L. C. McGowan and D. Hamelberg, J. Chem. Inf. Model., 2013, 53, 403–410 CrossRef CAS PubMed.
- S. V. Sambasivarao and O. Acevedo, J. Chem. Inf. Model., 2011, 51, 475–482 CrossRef CAS PubMed.
- A. Ahmed-Belkacem, L. Colliandre, N. Ahnou, Q. Nevers, M. Gelin, Y. Bessin, R. Brillet, O. Cala, D. Douguet, W. Bourguet, I. Krimm, J. M. Pawlotsky and J. F. Guichou, Nat. Commun., 2016, 7, 12777 CrossRef CAS PubMed.
- P. Taylor, Prog. Biophys. Mol. Biol., 1997, 67, 155–181 CrossRef CAS PubMed.
- J.-F. Guichou, J. Viaud, C. Mettling, G. Subra, Y.-L. Lin and A. Chavanieu, J. Med. Chem., 2006, 49, 900–910 CrossRef CAS PubMed.
- K. I. Cho, A. Orry, S. E. Park and P. A. Ferreira, ACS Chem. Neurosci., 2015, 6, 1476–1485 CrossRef CAS PubMed.
- T. L. Davis, J. R. Walker, V. Campagna-Slater, P. J. Finerty, R. Paramanathan, G. Bernstein, F. MacKenzie, W. Tempel, H. Ouyang, W. H. Lee, E. Z. Eisenmesser and S. Dhe-Paganon, PLoS Biol., 2010, 8, e1000439 CrossRef PubMed.
- J. P. Terhorst and W. L. Jorgensen, J. Chem. Theory Comput., 2010, 6, 2762–2769 CrossRef CAS PubMed.
- S. Castellano, S. Taliani, M. Viviano, C. Milite, E. Da Pozzo, B. Costa, E. Barresi, A. Bruno, S. Cosconati, L. Marinelli, G. Greco, E. Novellino, G. Sbardella, F. Da Settimo and C. Martini, J. Med. Chem., 2014, 57, 2413–2428 CrossRef CAS PubMed.
- A. De Simone, D. Russo, G. F. Ruda, A. Micoli, M. Ferraro, R. M. Di Martino, G. Ottonello, M. Summa, A. Armirotti, T. Bandiera, A. Cavalli and G. Bottegoni, J. Med. Chem., 2017, 60, 2287–2304 CrossRef CAS PubMed.
- K. Rangappa, S. Chandrappa, K. Vinaya and T. Ramakrishnappa, Synlett, 2010, 2010, 3019–3022 CrossRef.
- J. R. Loeffler, E. S. Ehmki, J. E. Fuchs and K. R. Liedl, J. Comput.-Aided Mol. Des., 2016, 30, 391–400 CrossRef CAS PubMed.
- W. G. Lee, R. Gallardo-Macias, K. M. Frey, K. A. Spasov, M. Bollini, K. S. Anderson and W. L. Jorgensen, J. Am. Chem. Soc., 2013, 135, 16705–16713 CrossRef CAS PubMed.
- L. Wang, Y. J. Wu, Y. Q. Deng, B. Kim, L. Pierce, G. Krilov, D. Lupyan, S. Robinson, M. K. Dahlgren, J. Greenwood, D. L. Romero, C. Masse, J. L. Knight, T. Steinbrecher, T. Beuming, W. Damm, E. Harder, W. Sherman, M. Brewer, R. Wester, M. Murcko, L. Frye, R. Farid, T. Lin, D. L. Mobley, W. L. Jorgensen, B. J. Berne, R. A. Friesner and R. Abel, J. Am. Chem. Soc., 2015, 137, 2695–2703 CrossRef CAS PubMed.
- M. Aldeghi, A. Heifetz, M. J. Bodkin, S. Knapp and P. C. Biggin, J. Am. Chem. Soc., 2017, 139, 946–957 CrossRef CAS PubMed.
- W. L. Jorgensen, Acc. Chem. Res., 2009, 42, 724–733 CrossRef CAS PubMed.
- J. Michel, N. Foloppe and J. W. Essex, Mol. Inform., 2010, 29, 570–578 CrossRef CAS PubMed.
- J. D. Chodera, D. L. Mobley, M. R. Shirts, R. W. Dixon, K. Branson and V. S. Pande, Curr. Opin. Struct. Biol., 2011, 21, 150–160 CrossRef CAS PubMed.
- Z. Cournia, B. Allen and W. Sherman, J. Chem. Inf. Model., 2017, 57, 2911–2937 CrossRef CAS PubMed.
- M. Aldeghi, A. Heifetz, M. J. Bodkin, S. Knapp and P. C. Biggin, Chem. Sci., 2016, 7, 207–218 RSC.
- H. H. Loeffler, J. Michel and C. Woods, J. Chem. Inf. Model., 2015, 55, 2485–2490 CrossRef CAS PubMed.
- G. Calabro, C. J. Woods, F. Powlesland, A. S. Mey, A. J. Mulholland and J. Michel, J. Phys. Chem. B, 2016, 120, 5340–5350 CrossRef CAS PubMed.
-
C. J. Woods, A. S. J. S. Mey, C. G. S. Bosisio and J. Michel, http://www.siremol.org.
- P. Eastman and V. S. Pande, Comput. Sci. Eng., 2015, 12, 34–39 Search PubMed.
- A. Mey, J. J. Jimenez and J. Michel, J. Comput.-Aided Mol. Des., 2018, 32, 199–210 CrossRef CAS PubMed.
- J. Zheng, J. E. Koblinski, L. V. Dutson, Y. B. Feeney and C. V. Clevenger, Cancer Res., 2008, 68, 7769–7778 CrossRef CAS PubMed.
- C. Georgiou, I. McNae, M. Wear, H. Ioannidis, J. Michel and M. Walkinshaw, J. Mol. Biol., 2017, 429, 2556–2570 CrossRef CAS PubMed.
- S. Ni, Y. Yuan, J. Huang, X. Mao, M. Lv, J. Zhu, X. Shen, J. Pei, L. Lai, H. Jiang and J. Li, J. Med. Chem., 2009, 52, 5295–5298 CrossRef CAS PubMed.
Footnote |
† Electronic supplementary information (ESI) available: Chemical synthesis and characterization of novel compounds. Computational, biochemical, biophysical and cellular assays protocols. The crystal structures of 2, 4, 5, 7, 8, 9, 10, 15 in complex with CypA have been deposited in the Protein Data Bank (PDB codes 6GJJ, 6GJM, 6GJY, 6GJP, 6GJI, 6GJR, 6GJL, 6GJN respectively). See DOI: 10.1039/c8sc03831g |
|
This journal is © The Royal Society of Chemistry 2019 |