DOI:
10.1039/C9RA06519A
(Paper)
RSC Adv., 2019,
9, 29511-29521
Reverse Monte Carlo modeling for local structures of noble metal nanoparticles using high-energy XRD and EXAFS†
Received
20th August 2019
, Accepted 12th September 2019
First published on 18th September 2019
Abstract
Reverse Monte Carlo (RMC) modeling based on the total structure factor S(Q) obtained from high-energy X-ray diffraction (HEXRD) and the k3χ(k) obtained from extended X-ray absorption fine structure (EXAFS) measurements was employed to determine the 3-dimensional (3D) atomic-scale structure of Pt, Pd, and Rh nanoparticles, with sizes less than 5 nm, synthesized by photoreduction. The total structure factor and Fourier-transformed PDF showed that the first nearest neighbor peak is in accordance with that obtained from conventional EXAFS analysis. RMC constructed 3D models were analyzed in terms of prime structural characteristics such as metal-to-metal bond lengths, first-shell coordination numbers and bond angle distributions. The first-shell coordination numbers and bond angle distributions for the RMC-simulated metal nanoparticles indicated a face-centered cubic (fcc) structure with appropriate number density. Modeling disorder effects in these RMC-simulated metal nanoparticles also revealed substantial differences in bond-length distributions for respective nanoparticles.
1. Introduction
Metal nanoparticles such as platinum (Pt), palladium (Pd) and rhodium (Rh) have been extensively investigated with regard to different potential applications in biotechnology, catalysis, and optoelectronics.1–3 The photochemical reduction of metal ions in solutions is one of the promising methods to prepare small metal nanoparticles,4–6 since the morphologies (such as size, shape, etc.) of metal nanoparticles is regulated by the protecting reagents as well as by the conditions of photoirradiation (for example, irradiation time, irradiation intensity, and so on). For small metal nanoparticles in which the number of atoms in the surface layer is comparable to the bulk, the compositions of the bulk and the surface are expected to be strongly correlated. Thus, in order to fully understand the properties and their relationship to the functions of nanoparticles, it is important to characterize not only the surface or the initial composition but also the 3-dimensional (3D) atomic structures of nanoparticles. This may have a profound effect on their structure–sensitive properties, including the catalytic reactivity.
Determining the 3D atomic arrangement inside metallic nanoparticles is significant in a diversity of scientific and technological areas. This is still challenging because of the functional disordered nanomaterials. In some cases, if metallic nanoparticles are crystallized, single crystal X-ray methods can be used to determine their structures, or atomic resolution tomographic images of individual nanoparticles can be obtained. However, in general, additional methods for quantitative and rapid 3D atomic structural characterization of disordered nanomaterials are needed because traditional techniques such as powder X-ray diffraction (XRD), high-resolution transmission electron microscopy (HRTEM),7,8 X-ray absorption fine structure (XAFS),9,10 small-angle X-ray scattering (SAXS),11,12 grazing incident small-angle X-ray scattering (GISAXS),13,14 differential scanning calorimetry (DSC),15,16 and others, are well suited to bulk-like crystalline materials for the determination of phase behavior, particle size, and so on. XAFS techniques such as XANES and EXAFS have widely been used to probe the valence state and nearest-atomic neighbor arrangement, but they are not sensitive to the longer range atomic structure in nanomaterials.
Over the past few decades, synchrotron radiation based high-energy X-ray diffraction (HEXRD) coupled to atomic pair distribution function (PDF) analysis has emerged as an important tool to reveal the 3D atomic arrangement of unsupported or supported metal nanoparticles,17–35 which have a pronounced effect on their structure and shape as well as on their functionality in applications such as catalysis. For example, Petkov and co-workers reported the 3D atomic structure of nanosized Ru18–20 and Au21–23 and binary alloy24–26 particles, indicating that these nanoparticles less than 10 nm in diameter could be considered as nonperiodic, dense packing of metallic atoms that shows characteristic of the fcc lattice with some surface atomic disorder. Here it may be noted that atoms at the nanoparticle surfaces have incomplete coordination spheres, experience significant surface relaxation and surface environment effects. Therefore, atoms at the nanoparticle surfaces and atoms inside the nanoparticles, that have complete coordination polyhedral and are largely not affected by nanoparticle extended surface related effects, may not exhibit the same structural patterns. Chupas et al.27–29 also demonstrated in situ time-resolved PDF methods to monitor the structural evolution associated with the formation of supported Pt nanoparticles as well as their structural changes induced by the CO and H2 adsorption by means of differential PDF techniques, which allow the atom–atom correlations that arise from the supported Pt nanoparticles to be separated from those of the bulk support. Other previous studies30–35 have also used finite cluster models built by applying crystallographic symmetry and periodic boundary conditions to a small unit cell.
In addition, to obtain a more definitive picture of the atomic-scale structure of nanoparticles, 3D atomic configurations were constructed by RMC modeling method based on experimental HEXRD.36,37 RMC simulations are an established and ideal technique for modeling structure functions of highly disordered materials that requires no crystallographic information.18–26,38–40 Coordination number (CN) and bond angle distributions are calculated from the RMC-generated configurations as well, further showing a disordered crystalline structure in detail. Recently, we have reported the 3D atomic structures of Ru nanoparticles in several diameters by means of HEXRD techniques with the aid of RMC simulation.41 The CN and bond angle analysis of the RMC-generated model of Ru nanoparticles revealed that the fcc-type nanoparticles dominate low activation energy packing sites for the catalytic activity of CO oxidation particularly on the surface.
On the other hand, application of the RMC simulations for EXAFS data analysis has been firstly reported by Gurman and McGreevy42 and several studies have been carried out for disordered molecular and finite-size systems,43–51 e.g., bcc-Fe,45 undercooled Cu46 and Ni,47 bulk metallic glasses,48 binary oxide glasses,49 liquid iodine,50 and poly-atomic molecules in gas phase.51 In contrast, RMC is rarely applied to discrete systems composed of molecules and small clusters. For complex structures, RMC refinements using a combined real-space fit of the X-ray total scattering PDF and EXAFS have been developed to incorporate an explicit treatment of both single- and multiple-scattering contributions to EXAFS.45,51–53
In this work, we have examined a combination of two synchrotron X-ray techniques, HEXRD and EXAFS, together with complementary HRTEM measurement to characterize the atomic-scale 3D structure details of Pt, Pd, and Rh nanoparticles prepared by the photoreduction. The RMC modeling of the experimental HEXRD and EXAFS data has revealed atomic-scale configurations where the surface of these nanoparticles with a diameter less than 5 nm exhibits fcc-type structure with some distinct disorders in terms of the CN and bond angle distributions.
2. Experimental methods
2.1. Materials
Hexachloroplatinic(IV) acid (H2PtCl6·6H2O, Nacalai Tesque), palladium(II) chloride (PdCl2, Nacalai Tesque), rhodium(III) chloride hydrate (RhCl3·3H2O, Aldrich), poly(N-vinyl-2-pyrrolidone) (PVP, K-30, average M.W. = 40
000, Tokyo Kasei Kogyo Co.), ethanol (guaranteed reagent, 99.5%, Nacalai Tesque), and distilled water were used without further purification. Benzophenone ((C6H5)2CO, guaranteed reagent) was used as a photoactivator which was purchased from Nacalai Tesque.
2.2. Sample preparation
Colloidal dispersions of Pt, Pd, and Rh nanoparticles ([M] = 24.4 mM, M = Pt, Pd and Rh) were prepared by the photoreduction of the ionic precursors (H2PtCl6·6H2O, PdCl2, RhCl3·3H2O) in water/ethanol(1/1, v/v) solution of PVP in the presence of benzophenone as a photoactivator, as described previously.10,12 In a typical procedure for the preparation of the Pt nanoparticles, 0.223 g of PVP (2.01 mmol of monomeric unit) and 0.06 g of benzophenone was added to the 10 mL of ethanol solution, and stirred to dissolve PVP and benzophenone completely. 10 mL of aqueous solution containing 0.488 mmol of H2PtCl6·6H2O was subsequently added and mixed for 1 min. The concentration of [Pt] and [benzophenone] in the solution was 24.4 and 16.5 mM, respectively. N2 gas was bubbled into the mixed solution, and vigorous stirring was carried out during 5 min to remove the dissolved O2. The mixed solution was then photoirradiated by a 500 W superhigh-pressure mercury lamp with continuous stirring using a magnetic stirrer. After the photoreduction was complete in the reduction time of up to 5 hours, the colloidal dispersions of Pt nanoparticles was evaporated to give a dried PVP-protected Pt nanoparticles. It is noted that PVP played an important role of the capping agent of the Pt nanoparticles. The other colloidal dispersions of Pd and Rh nanoparticles ([Pd] = [Rh] = 24.4 mM) were prepared by the photoreduction with benzophenone, according to the similar procedure to produce the corresponding powder samples. The size of the obtained nanoparticles was determined from HRTEM images, which were captured using a JEOL JEM-2000FX microscope operating at 200 kV. The aqueous ethanol solution of nanoparticles was diluted and drop-cast onto a carbon-coated copper grid followed by drying in vacuo. The average diameter and distributions were estimated by measuring about 200 particles each in arbitrarily chosen areas on the enlarged photograph. Representative HRTEM images are shown in Fig. S1 (see the ESI†). It is found that these nanoparticles appear to be rounded in shape and highly monodisperse, and the average particle diameters of Pt, Pd, and Rh nanoparticles are 2.5, 2.0, 2.2 nm, respectively. The HEXRD and EXAFS experiments were performed on the same samples, and the results were compared.
2.3. High-energy X-ray diffraction and data analysis
High-energy X-ray diffraction (HEXRD) experiments were performed at room temperature using a two-axis diffractometer available at the BL04B2 beamline of SPring-8 at the Japan Synchrotron Radiation Research Institute (JASRI), Japan.54 The incident X-ray beams of 61.37 keV with a wavelength of 0.0202 nm were monochromatized using an Si(220) monochromator. The powder samples of Pt, Pd, and Rh nanoparticles were either loaded into quartz capillaries with 1.1 mm OD and ∼0.7 mm ID, or they were sandwiched between Kapton films, and they were measured in transmission geometry. PVP was used as the reference material for background correction. HEXRD data was collected over quite a wide region (0–25 Å−1) of reciprocal space vectors, Q. Here, Q = 4π(sin
θ)/λ, where 2θ is the scattering angle between the incoming and the outgoing X-rays and λ is the wavelength of X-ray radiation. The observed X-ray intensity was corrected for background, polarization and absorption.
The pair distribution function (PDF) method, which was initially used for studying short-range ordered materials, such as liquids and glasses, has recently been increasingly applied to nanomaterials. It uses the whole measured spectrum, including both Bragg and diffuse components, to extract the Faber–Ziman total scattering structure factor, S(Q), using standard analysis procedures.55 The S(Q) is related to the coherent part (Icoh(Q)) of the diffraction data as follows:
|
 | (1) |
where
ci and
fi are the atomic concentration and X-ray atomic scattering factor, respectively, for the atomic species of type i. The great advantage of atomic PDFs in studying materials of a limited length of structural coherence is that the total scattering, including Bragg scattering as well as diffuse scattering, is taken into account. In this way, both the average (
i.e., longer-range) atomic-scale structure, manifested in the Bragg-like peaks, and local structural distortions, manifested in the diffuse component of the XRD pattern, are reflected in the PDF.
In this PDF method, the reduced atomic PDF is defined as G(r) = 4πr[(ρ(r) − ρ0)], where ρ(r) and ρ0 are the local and average atomic number densities, respectively, and r is the radial distance in real-space. G(r) peaks at characteristic distances separating pairs of atoms and thus reflects the atomic-scale structure of the materials studied. The PDF G(r) is the Fourier transform of the experimentally observable total structure function, S(Q), that is,
|
 | (2) |
To obtain real-space information with sufficient resolution, it is necessary to measure S(Q) up to the higher-Q region since higher resolution in real space is achieved with larger Qmax.
2.4. EXAFS measurement and data analysis
Extended X-ray absorption fine structure (EXAFS) experiments were conducted at ambient temperature in a transmission mode with the detector of ionization chambers in different beamlines. The measurements at the Pt L3-edge (11
562.0 eV) of Pt nanoparticles and their reference samples were carried out at beamline BL-9C in Photon Factory (PF), High Energy Accelerator Research Organization (KEK) in Japan. This beamline was operated at 2.5 GeV in a top-up mode set to 450 mA. The measurements of Pd K-edge (24
348.0 eV) for Pd nanoparticles and Rh K-edge (23
219.8 eV) for Rh nanoparticles and their references were collected at beamline NW10A of Photon Factory Advanced Ring (PF-AR) in KEK. This source operates at 6.0 GeV with the maximum beam current of 55 mA. The powder samples of these nanoparticles were prepared by mixing with BN powder and pressed into pellets with the diameter of 10 mm, or they were uniformly sandwiched between Kapton films. The spectra were collected between 150 eV below and 1200 eV above the corresponding edges to ensure reproducibility and the analysis was performed on the averaged data. Metal foils of Pt, Pd, and Rh were used as energy calibration standards. The typical experimental setups were described in previous publications.10,56 The raw data was processed using Athena program and modeled using the Artemis program from the IFEFFIT XAFS analysis software package using reasonable theoretical contributions.57,58 The construction of theoretical contributions for Pt–Pt, Pd–Pd, and Rh–Rh bond was obtained from the known crystal structure of bulk Pt, Pd, and Rh, respectively. These contributions were combined in Artemis into a total theoretical signal and used for the modeling of all nanoparticles. For the r-space fits, an amplitude reduction factor of S02 = 0.932 (for Pt), 0.876 (for Pd), and 0.954 (for Rh) were first obtained from the transmission EXAFS data of the corresponding foil.
2.5. RMC modeling
RMC simulations were used to obtain structure information, including the atomic pair distribution, coordination number, and bond angle distribution, directly from RMC-generated 3D configuration models using RMC_POT software59 furnished in the case of non-periodic boundary conditions for experimental structure factor data. As a typical example to describe the practical implementation of the RMC simulations, the spherical shaped structure of Pt nanoparticles was chosen. Two types of the initial configurations are considered: (1) the initial configurations including 1100 Pt atoms were generated from the known crystal structure of fcc bulk Pt and extended in a radial fashion to a Pt nanoparticle diameter equivalent to those (3.5 nm in diameter) found from HRTEM experiments. (2) The initial configurations were a computer-generated random atomic configuration consisting of 1100 Pt atoms in a spherical shape with 3.5 nm in diameter. The atomic number density of 0.04900058 Å−3 corresponding to 21.45 g cm−3, which is equivalent to the atomic packing factor (0.74) of fcc bulk Pt, was used in both models. During the RMC simulation, the 3D atomic configuration was generated by random atomic move. In a similar manner, data from literature sources for the crystal structures of bulk Pd and Rh were used as starting values in the fitting. The initial configurations of Pd nanoparticles were containing 1687 Pd atoms in a Pd nanoparticle with 4.0 nm in diameter, while those of Rh nanoparticles containing 1206 Rh atoms in a Rh nanoparticle with 3.5 nm in diameter. The atomic number density of 0.05034664 Å−3 and 0.053742278 Å−3 was applied for the Pd and Rh nanoparticles, respectively. The RMC simulation was carried out only by the experimental data and some user inputted restrictions. The average coordination constraint enforcing 12 first atomic neighbors between 0.23 and 0.33 nm was applied to take into account the close-packed fcc nature of these Pt, Pd, and Rh nanoparticles. The simulation was repeated until the computed data converges to the experimental data in very good detail. That is, it was done as to minimize a goodness-of-fit indicator, Rw, defined as |
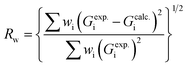 | (3) |
where Gexp. and Gcalc. are the experimental and calculated data, respectively, and wi are weighting factors reflecting the statistical quality of the individual data points.
For the refinement of RMC simulations using experimental HEXRD S(Q) data, the analysis of experimental EXAFS k3χ(k) data using RMC simulations was successively performed by means of RMC_POT software.59 The simulations did not contain any free parameters and were based on HEXRD results for the atom configuration of the Pt, Pd, and Rh nanoparticles. Filtered (by Fourier back transformation from r-space between 1.7 and 3.0 Å) data were investigated since the RMC analysis of the filtered data showed a better convergence behavior. Theoretical EXAFS amplitude and phase functions for RMC analysis were obtained by FEFF 8 simulations.60,61 In the present work the RMC simulations were applied successively to the HEXRD and EXAFS data for these nanoparticles. The initial configurations were generated from refined results of the RMC simulations of PDFs of the corresponding samples and also contain an appropriate number of metal atoms. Here we present only the models that quantitatively fit the EXAFS data best as determined from the fitting residual Rw-value. Here we demonstrate that combined RMC simulations using HEXRD and EXAFS data, while confirming the principal conclusions of the traditional EXAFS analysis,57,61 provide more comprehensive and reliable information on the local structure characteristics than the individual techniques alone.
3. Results and discussion
3.1. High-energy synchrotron X-ray diffraction of Pt, Pd, and Rh nanoparticles
Experimental atomic PDFs, G(r), of the Pt, Pd, and Rh nanoparticles synthesized by the photoreduction are shown in Fig. 1. All show a series of broad peaks reflecting the presence of well-defined atomic coordination spheres in the particle size less than 4 nm, and they decay to nearly zero at distances corresponding to the nanoparticles sizes (e.g., at 3.5 nm for the Pt nanoparticles, at 4.0 nm for the Pd nanoparticles, and at 3.5 nm for the Rh nanoparticles), indicating the presence of substantial local structural disorder. The first G(r) peak of the Pt, Pd, and Rh nanoparticles is positioned at 2.77, 2.77, and 2.70 Å, respectively, as shown in the inset of Fig. 1. For the ideal fcc metallic bulk phase,62 the first atomic neighbor distance in bulk Pt, Pd, and Rh is 2.78, 2.76, and 2.70 Å, respectively. This implies that the bond distance of Pt–Pt, Pd–Pd, and Rh–Rh in the respective nanoparticles is almost identical to those in the bulk phase. Although the width of the first G(r) peaks might depend on the degree of structural distortions due to the interactions between nanoparticles and PVP chains, the atomic PDFs do not exhibit any peaks at less than 2 Å. This result strongly indicates that the use of PVP capping reagents did not result in the formation of a substantial number of atomic correlations on the surface of nanoparticle. Thus, we were able to analyze the atomic-scale structure of the fcc-type nanoparticles by employing the RMC modeling technique.
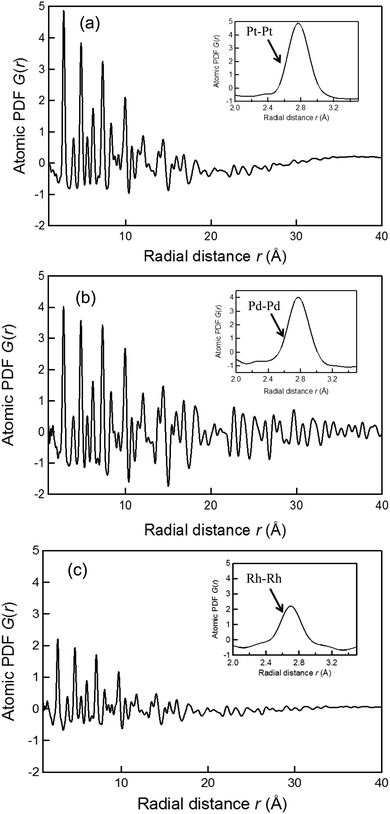 |
| Fig. 1 Experimental atomic PDFs G(r), obtained from the Fourier transformation of the reduced structure function Q[S(Q) − 1], of the (a) Pt, (b) Pd, and (c) Rh nanoparticles prepared by the photoreduction in the presence of PVP and benzophenone. | |
3.2. EXAFS analysis of Pt, Pd, and Rh nanoparticles
Fourier transform (FT) magnitudes of the k3χ(k) spectra at the Pt L3-edge, Pd K-edge, and Rh K-edge for the Pt, Pd, and Rh nanoparticles, together with the corresponding foils, are shown in Fig. S2 (see the ESI†). In all the edges, the main peak is observed between 2.0 and 3.0 Å (phase shift uncorrected) which is assigned to a metallic Pt–Pt (for Pt nanoparticles), Pd–Pd (for Pd nanoparticles), and Rh–Rh (for Rh nanoparticles) bond. For the curve fitting, the high-frequency noise was removed by a Fourier filtering technique, and the inverse Fourier transformation to the k space was employed. Curve fitting analysis was performed to obtain quantitative coordination number (CN) and interatomic bond distance (r) values. A structural model based on the fcc bulk metal foil was used for these samples in performing the Artemis program. Table S1 of the ESI† shows the structural parameters, such as CN, r, energy shift (ΔE), Debye–Waller factor (σ) and R factor. Based on these results presented in Fig. S2 and Table S1,† no peak is observed around 1.8 Å (phase shift uncorrected) assigned to metal–oxygen and/or metal–nitrogen atomic correlations which originate from the PVP stabilizer as a capping reagent. Here it should be noted that the bond distance of Pt–Pt, Pd–Pd, and Rh–Rh in the respective nanoparticles obtained from the EXAFS analysis is consistent with that obtained from the PDF analysis as shown in Fig. 1.
3.3. RMC modeling using HEXRD data
In general, there is no direct route for obtaining CNs that described the local atomic environment of the nanoparticles from PDF analysis, while the PDFs can provide long-range structural information. The RMC-generated 3D models were constructed to obtain real-space structure details, including the atomic pair distribution, coordination number, and bond angle distribution, for all the nanoparticles. The RMC simulated and experimental total structure factor S(Q) data sets for the Pt, Pd, and Rh nanoparticles, starting from different initial configurations, are shown in Fig. 2.
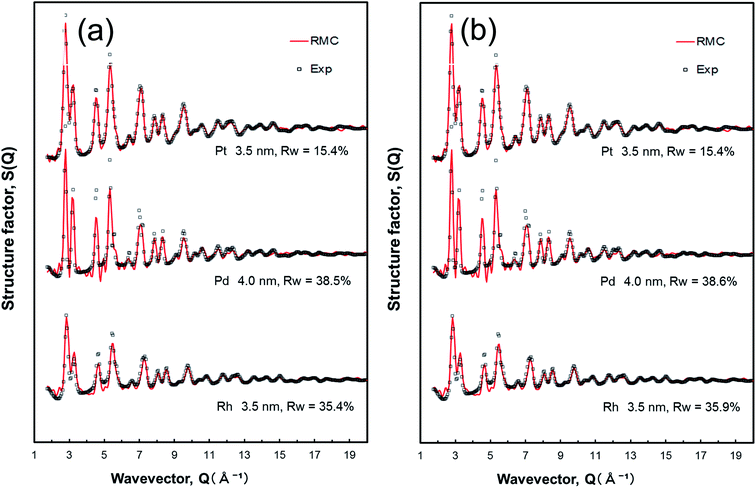 |
| Fig. 2 Experimental and RMC-simulated total structure factor S(Q) profiles for the Pt, Pd, and Rh nanoparticles with different particle sizes, prepared by the photoreduction in the presence of PVP and benzophenone. The initial configuration for the RMC simulations is based on (a) the fcc bulk crystal structure and (b) the computer-generated random atomic configuration. The black squares represent the experimental total structure factor S(Q) profiles, and the RMC-simulated total structure factor S(Q) profiles are shown as red solid lines. | |
For the Pt nanoparticles studied here, the initial configurations were spherical in shape and were varied with the size of 2.5, 3.0, 3.5, 4.0, and 4.5 nm, as shown in Fig. S3 (see the ESI†). As may be expected, the RMC-generated Pt nanoparticle model with 3.5 nm in size converged on the experimental structure factor S(Q) data very well (also see Fig. 2). In the case of the initial configuration coming from the crystal structure of fcc bulk Pt, as shown in Fig. S3(a),† finite size atomic configurations cut out from a perfect fcc Pt lattice were used to start the RMC simulations. In the simulations, the position of each atom from 401, 693, 1100, 1642, and 2338 atom configurations, respectfully, was adjusted so as to minimize the difference Rw (see eqn (3)) between the model and experimental S(Q) data. Atoms were constrained (i) not to come closer than preselected distances of closest approach and (ii) to maintain as maximal (i.e., as close to 12) as possible coordination numbers. That is, the first constraint reflects the fact that two neighboring Pt atoms in the nanoparticles do not approach each other closer than 2.4 Å as the experimental G(r) shows. The second constraint takes into account the close-packing fcc nature of the atomic ordering in bulk Pt metals. At the same time, the energy of the configurations was optimized by minimizing pairwise (Lennard-Jones type) potentials for Pt taken from literature sources. The combined utilization of constraints and potential minimization ensures a smooth convergence of the RMC simulations. The observed minimum Rw of 2.5, 3.0, 3.5, 4.0, and 4.5 nm size fcc Pt nanoparticles are 32.0%, 20.7%, 15.4%, 14.4%, and 14.5%, respectively. While the fits to the 2.5–3.0 nm Pt nanoparticle samples are insufficient due to the deficiency of atom number employed in the simulations, the fits to the 3.5–4.5 nm Pt nanoparticle samples is quite good, with Rw values equal to ca.15% that is generally considered an acceptable fit of fcc models to S(Q) data of nanoparticle in the literature.63,64
On the other hand, in the case of the initial configuration coming from the computer-generated random atomic configuration, as shown in Fig. S3(b),† two constraints (i.e., a cut off distance of 2.4 Å for the first coordination sphere, and CN of 12 for the first coordination neighbors in fcc bulk Pt) described above were applied in the RMC simulations. The similar fitting results to Pt nanoparticles in size ranging from 2.5 to 4.5 nm were observed, and the minimum Rw of 2.5, 3.0, 3.5, 4.0, and 4.5 nm size fcc Pt nanoparticles are 31.5%, 20.8%, 15.4%, 14.9%, and 14.7%, respectively. Not surprisingly, they fit the experimental atomic PDFs as good as the periodic, fcc lattice constrained models (Fig. S3(a)†). On the basis of the RMC simulations and HRTEM image (Fig. S1(a)†), it is strongly suggested that the atomic-scale 3D configuration of Pt nanoparticles exhibits fcc-type structure with an average particle diameter of 3.5 nm with some distinct disorders.
The RMC simulated and experimental total structure factor S(Q) data sets for the Pd and Rh nanoparticles are also shown in Fig. 2 as well as Fig. S4 and S5 of the ESI.† In a similar procedure of the Pt nanoparticles, the initial configurations were spherical in shape and with the particle size ranging from 2.5 to 4.5 nm for the Pd nanoparticles (Fig. S4†). The analyzed particle sizes of Rh nanoparticles range between 2.0 and 4.0 nm (Fig. S5†), which is slightly less than those of Pt and Pd nanoparticles, according to the HRTEM images (Fig. S1†). In the RMC simulations for the Pd nanoparticles, the position of each atom from 412, 712, 1130, 1687, and 2402 atom configurations was adjusted to minimize the difference Rw between the model and experimental Pd S(Q) data. Here the atomic number density of 0.05034664 Å−3 in the Pd nanoparticles. Similarly, the position of each atom from 225, 440, 760, 1206, and 1801 atom configurations (the atomic number density of 0.053742278 Å−3) was adjusted to minimize the difference Rw between the model and experimental Rh S(Q) data. The initial configuration derived from the fcc bulk Pd leads to good fit to the 4.0 nm Pd nanoparticle model. The similar feature is also observed in the case of Rh nanoparticle samples, indicating that the initial configuration derived from the fcc bulk Rh exhibits best fit to the 3.5 nm Rh nanoparticle model.
Exemplary structure models refined by the RMC simulations for 3.5 nm Pt nanoparticles, 4.0 nm Pd nanoparticles, and 3.5 nm Rh nanoparticles are shown in Fig. 3. Atoms at the inner part of the nanoparticles show fewer mean-square displacements than atoms closer to the nanoparticles surface. From the RMC-generated models for 2.0–4.5 nm metallic nanoparticles, we computed not only two-atom correlation functions such as the PDFs but also three-atom correlation functions such as distributions of bond angles as well. The bond angles are a very sensitive indicator of the type of local atomic ordering. It is noted that despite the presence of a substantial structural disorder, all nanoparticles studied here have a well-defined local atomic ordering seen as a sequence of well-defined PDF peaks (see Fig. S3 to S5 of the ESI†). Firstly, we shall now discuss the average CN for describing the atomic arrangement in the metal nanoparticles. It is noted that the first main PDF peak shown in Fig. 2 for the bulk metals reflected the presence of 12 first atomic neighbors. Since the first minimum after the first main PDF peak is about 0.33 nm, the CNs in the first coordination-shell were evaluated by counting the number of metal atoms in the spherical shell with a radius between 0.23 and 0.33 nm around each central atom. The CN in the second coordination-shell was obtained by counting the number of metal atoms in the spherical shell with a radius between 0.34 and 0.43 nm. The distributions of CNs in the first and second coordination-shell of the Pt, Pd, and Rh nanoparticles are shown in Fig. S6–S8 (see the ESI†). Table 1 lists the values of CNs in the first and second coordination-shell of these nanoparticles. Such CNs are characteristic in a sense that they lie between those of bulk (CN = 12) and those of atoms locating on surfaces of metal nanoparticles.41 The CNs in the first coordination-shell obtained from the RMC-generated models are consistently lower than those obtained by EXAFS data (Table S1†). This has most likely to do with the inefficiency of the EXAFS technique for materials with substantial local structural disorder.
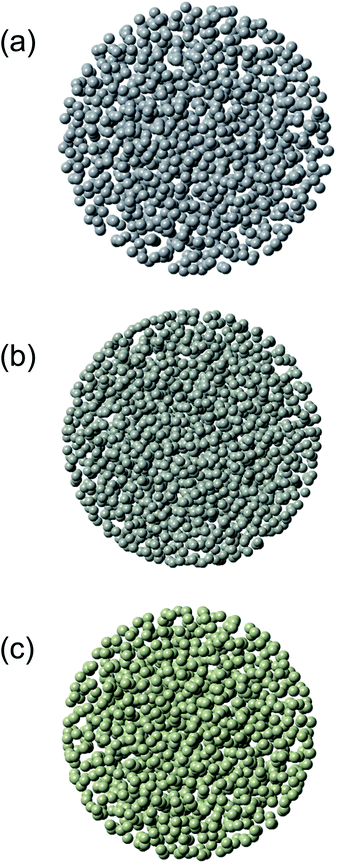 |
| Fig. 3 RMC-generated 3D structure models for the (a) 3.5 nm Pt, (b) 4.0 nm Pd, and (c) 3.5 nm Rh nanoparticles prepared by the photoreduction in the aqueous PVP solutions. The initial configuration for the RMC simulations is based on the computer-generated random atomic configuration for the respective nanoparticles. | |
Table 1 Coordination numbers obtained from RMC simulations for the HEXRD and EXAFS data of the metal nanoparticles
Sample |
Initial structure |
RMC-HEXRD |
RMC-EXAFS |
First shell |
Second shell |
First shell |
Second shell |
Pt nanoparticles (3.5 nm) |
fcc |
5.7 |
4.4 |
5.5 |
5.2 |
Random |
5.7 |
4.3 |
5.5 |
5.2 |
Pd nanoparticles (4 nm) |
fcc |
5.6 |
5.8 |
5.7 |
6.2 |
Random |
5.6 |
5.9 |
5.7 |
6.2 |
Rh nanoparticles (3.5 nm) |
fcc |
5.7 |
4.9 |
5.9 |
5.0 |
Random |
5.7 |
4.8 |
5.9 |
4.9 |
In addition, as shown in Fig. 4, the bond angle distributions of Pt nanoparticles (3.5 nm), Pd nanoparticles (4.0 nm), and Rh nanoparticles (3.5 nm) have been evaluated from the atomic configurations obtained by the RMC procedure. In a perfect bulk fcc metal, bond angles spike at 60, 90, and 120°. The bond angles show a broad distribution that is spread around the bond angles of a perfect fcc lattice, clearly confirming the distinct disorders in the atomic-scale structure of these nanoparticles. This is to be expected since the RMC configuration models feature an fcc-type structure that is intrinsic to the fcc-type Pt, Pd, and Rh bulk counterpart.
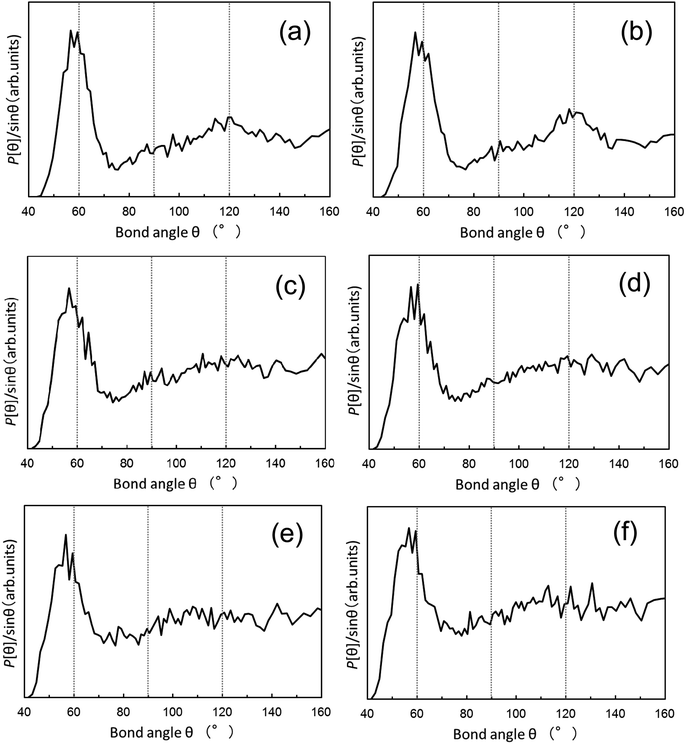 |
| Fig. 4 Distribution of bond angles derived from the RMC simulations using PDFs data for the 3.5 nm Pt nanoparticles, 4.0 nm Pd nanoparticles, and 3.5 nm Rh nanoparticles. The distributions are extracted from the RMC-generated structure models as shown in Fig. 3. The initial configuration for the RMC simulations is based on (a, c, and e) the fcc bulk crystal structure and (b, d, and f) the computer-generated random atomic configuration for the respective nanoparticles. (a and b): Pt nanoparticles, (c and d): Pd nanoparticles, and (e and f): Rh nanoparticles. | |
3.4. RMC modeling using EXAFS data
For the purpose of EXAFS refinement of Pt, Pd, and Rh nanoparticles, we have performed a successful method for structural refinement, based on the RMC simulations, which can be applied to experimental S(Q) data and subsequently to experimental EXAFS data. In Fig. 5, we compare their experimental k3χ(k) EXAFS spectra with the k3χ(k) spectra calculated in the RMC simulation for the refined models obtained from the RMC simulations of S(Q) data. Here the experimental k3χ(k) spectra are derived by a Fourier filtering from r-space between 1.7 and 3.0 Å (see Fig. S2 of the ESI†), and the inverse Fourier transformation to the k space (the range 4–15 Å−1) was employed. The backscattering factors such as amplitudes and phases are calculated by FEFF 8 program.60,61 It is obvious that the minimum Rw values are 14.9 and 14.6% (for the 3.5 nm Pt nanoparticles), 8.3 and 8.3% (for the 4.0 nm Pd nanoparticles), as well as 28.5 and 28.9% (for the 3.5 nm Rh nanoparticles). In particular, it appears that best fitting results are obtained in the case of Pd nanoparticles, compared with those obtained from the RMC simulations using S(Q) data. This can be a consequence of the possibility of reproducing the EXAFS signal with the RMC simulations. In addition, the distributions of CNs in the first and second coordination-shell of the Pt, Pd, and Rh nanoparticles are shown in Fig. S9–S11 (see the ESI†) in addition to Table 1. The bond angle distributions are also demonstrated in Fig. S12 of the ESI.† It is noted that the trends concerning to the CNs and their bond angle distributions in the analysis of RMC-EXAFS is very similar to those in the analysis of RMC-HEXRD (Fig. 4). In addition to more accurate and detailed structure determination of monometallic and/or bimetallic nanoparticles, simultaneous fitting of the total scattering PDF and EXAFS data will provide an effective method for benchmarking the EXAFS theory/approximations against total scattering PDF.
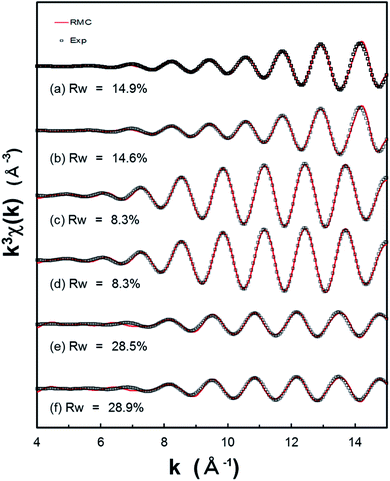 |
| Fig. 5 Experimental and RMC-simulated k3-weighted EXAFS spectra (k3χ(k)) for the 3.5 nm Pt nanoparticles, 4.0 nm Pd nanoparticles, and 3.5 nm Rh nanoparticles. The initial configurations are generated from refined results of the RMC simulations of S(Q) for the corresponding nanoparticles. The initial configuration for the RMC simulations is based on (a, c, and e) the fcc bulk crystal structure and (b, d, and f) the computer-generated random atomic configuration for the respective nanoparticles. (a and b): Pt nanoparticles, (c and d): Pd nanoparticles, and (e and f): Rh nanoparticles. | |
4. Conclusion
The 3D atomic structure of Pt, Pd, and Rh nanoparticles in spherical shape with average diameters less than 5 nm were explored by means of the RMC-based simulations, without applying periodic boundary conditions, using total scattering experimental structure factor S(Q) obtained from HEXRD. For the purpose of refinement of model structures for these nanoparticles, we performed subsequent RMC-based simulations using experimental k3χ(k) EXAFS data with the initial configurations calculated from the refined results of the X-ray structure factor based RMC model. The reduced PDF G(r) showed that the first-neighbor peak is in accordance with that obtained from conventional EXAFS analysis. These nanoparticles were disordered at short- to intermediate-range atomic distances, but unable to access particle–particle correlations over long-range orders. The first-shell coordination numbers and bond angle distributions for the RMC-simulated metal nanoparticles indicated face-centered cubic (fcc) structure with appropriate number density. The combined method of HEXRD (or EXAFS) and RMC modeling that we have used here is a promising tool for elucidating the details of atomic-scale structures of many other nanoparticles. The present work will encourage researchers to larger extent to determine the averaged structural parameters of the nanoparticles obtained experimentally or by RMC-based simulations.
Conflicts of interest
The authors declare no competing financial interest.
Acknowledgements
We appreciate the approval of the Japan Synchrotron Radiation Research Institute (JASRI) (Proposal No. 2015A1261 and 2016A1093) for the high-energy XRD measurements performed at the BL04B2 in the SPring-8. We are grateful to the approval of Photon Factory Advisory Committee (PAC) (Proposal No. 2017G028) at High Energy Accelerator Research Organization (KEK) for the EXAFS measurements. This study was partially supported by the Cooperative Research Program (Program No. 16B1015) of Institute for Catalysis, Hokkaido University. We also thank Prof. K. Asakura at Institute for Catalysis, Hokkaido University, for the discussion of RMC-EXAFS simulations.
References
- C. B. Murray, C. R. Kagan and M. G. Bawendi, Synthesis and Characterization of Monodisperse Nanocrystals and Close-Packed Nanocrystal Assemblies, Annu. Rev. Mater. Sci., 2000, 30, 545–610 CrossRef CAS.
- Y. Xia, Y. J. Xiong, B. Lim and S. E. Skrabalak, Shape-Controlled Synthesis of Metal Nanocrystals: Simple Chemistry Meets Complex Physics?, Angew. Chem., Int. Ed., 2009, 48, 60–103 CrossRef CAS PubMed.
- Y. Tao, M. Li, J. Ren and X. Qu, Metal Nanoclusters: Novel Probes for Diagnostic and Therapeutic Applications, Chem. Soc. Rev., 2015, 44, 8636–8663 RSC.
- D. R. Clary, M. Nabil, M. M. Sedeh, Y. El-Hasadi and G. Mills, Photochemical Generation of Ag, Pd, and Pt Particles in Octane, J. Phys. Chem. C, 2012, 116, 9243–9250 CrossRef CAS.
- M. Harada and S. Kizaki, Formation Mechanism of Gold Nanoparticles Synthesized by Photoreduction in Aqueous Ethanol Solutions of Polymers Using In Situ Quick Scanning X-ray Absorption Fine Structure and Small-Angle X-ray Scattering, Cryst. Growth Des., 2016, 16, 1200–1212 CrossRef CAS.
- Y. Shiraishi, H. Tanaka, H. Sakamoto, N. Hayashi, Y. Kofuji, S. Ichikawa and T. Hirai, Synthesis of Au Nanoparticles with Benzoic Acid as Reductant and Surface Stabilizer Promoted Solely by UV Light, Langmuir, 2017, 33, 13797–13804 CrossRef CAS PubMed.
- J. Miao, P. Ercius and S. J. L. Billinge, Atomic electron tomography: 3D structures without crystals, Science, 2016, 353, aaf2157 CrossRef PubMed.
- X. Fu, B. Chen, C. Li, H. Li, Z.-M. Liao, D. Yu and A. H. Zewail, Direct Visualization of Photomorphic Reaction Dynamics of Plasmonic Nanoparticles in Liquid by Four-Dimensional Electron Microscopy, J. Phys. Chem. Lett., 2018, 9, 4045–4052 CrossRef CAS PubMed.
- B. Roldan Cuenya, J. R. Croy, S. Mostafa, F. Behafarid, L. Li, Z. Zhang, J. C. Yang, Q. Wang and A. I. Frenkel, Solving the Structure of Size-Selected Pt Nanocatalysts Synthesized by Inverse Micelle Encapsulation, J. Am. Chem. Soc., 2010, 132, 8747–8756 CrossRef CAS PubMed.
- M. Harada and Y. Kamigaito, Nucleation and Aggregative Growth Process of Platinum Nanoparticles Studied by in Situ Quick XAFS Spectroscopy, Langmuir, 2012, 28, 2415–2428 CrossRef CAS PubMed.
- J. Polte, T. T. Ahner, F. Delissen, S. Sokolov, F. Emmerling, A. F. Thünemann and R. Kraehnert, Mechanism of Gold Nanoparticle Formation in the Classical Citrate Synthesis Method Derived from Coupled In Situ XANES and SAXS Evaluation, J. Am. Chem. Soc., 2010, 132, 1296–1301 CrossRef CAS PubMed.
- M. Harada, N. Tamura and M. Tekenaka, Nucleation and Growth of Metal Nanoparticles during Photoreduction Using In Situ Time-Resolved SAXS Analysis, J. Phys. Chem. C, 2011, 115, 14081–14092 CrossRef CAS.
- G. Renaud, R. Lazzari and F. Leroy, Probing Surface and Interface Morphology with Grazing Incidence Small Angle X-Ray Scattering, Surf. Sci. Rep., 2009, 64, 255–380 CrossRef CAS.
- E. Metwalli, V. Körstgens, K. Schlage, R. Meier, G. Kaune, A. Buffet, S. Couet, S. V. Roth, R. Röhlsberger and P. Müller-Buschbaum, Cobalt Nanoparticles Growth on a Block Copolymer Thin Film: A Time-Resolved GISAXS Study, Langmuir, 2013, 29, 6331–6340 CrossRef CAS PubMed.
- T. T. Bao, Y. Kim, J. Lee and J.-G. Lee, Preparation and Thermal Analysis of Sn-Ag Nano Solders, Mater. Trans., 2010, 51, 2145–2149 CrossRef CAS.
- T. Muthukumaran and J. Philip, Effect of phosphate and oleic acid capping on structure, magnetic properties and thermal stability of iron oxide nanoparticles, J. Alloys Compd., 2016, 689, 959–968 CrossRef CAS.
- V. Petkov, Nanostructure by high-energy X-ray Diffraction, Mater. Today, 2008, 11, 28–38 CrossRef CAS.
- N. Bedford, C. Dablemont, G. Viau, P. Chupas and V. Petkov, 3-D Structure of Nanosized Catalysts by High-Energy X-ray Diffraction and Reverse Monte Carlo Simulations: Study of Ru, J. Phys. Chem. C, 2007, 111, 18214–18219 CrossRef CAS.
- O. Gereben and V. Petkov, Reverse Monte Carlo study of spherical sample under non-periodic boundary conditions: the structure of Ru nanoparticles based on x-ray diffraction data, J. Phys.: Condens. Matter, 2013, 25, 454211 CrossRef PubMed.
- X.-Y. Quek, I. A. W. Filot, R. Pestman, R. A. van Santen, V. Petkov and E. J. M. Hensen, Correlating Fischer-Tropsch activity to Ru nanoparticle surface structure as probed by high-energy X-ray diffraction, Chem. Commun., 2014, 50, 6005–6008 RSC.
- V. Petkov, N. Benford, M. R. Knecht, M. G. Weir, R. M. Crooks, W. Tang, G. Henkelman and A. Frenkel, Periodicity and Atomic Ordering in Nanosized Particles of Crystals, J. Phys. Chem. C, 2008, 112, 8907–8911 CrossRef CAS.
- V. Petkov, Y. Lee, S. Sun and Y. Ren, Noncrystallographic Atomic Arrangement Driven Enhancement of the Catalytic Activity of Au Nanoparticles, J. Phys. Chem. C, 2012, 116, 26668–26673 CrossRef CAS.
- V. Petkov, Y. Ren, S. Shan, J. Luo and C.-J. Zhong, A distinct atomic structure-catalytic activity relationship in 3-10 nm supported Au particles, Nanoscale, 2014, 6, 532–538 RSC.
- V. Petkov, S. Shastri, S. Shan, P. Joseph, J. Luo, C.-J. Zhong, T. Nakamura, Y. Herbani and S. Sato, Resolving Atomic Ordering Differences in Group 11 Nanosized Metals and Binary Alloy Catalysts by Resonant High-Energy X-ray Diffraction and Computer Simulations, J. Phys. Chem. C, 2013, 117, 22131–22141 CrossRef CAS.
- V. Petkov, B. Prasai, Y. Ren, S. Shan, J. Luo, P. Joseph and C.-J. Zhong, Solving the nanostructure problem: exemplified on metallic alloy nanoparticles, Nanoscale, 2014, 6, 10048–10061 RSC.
- V. Petkov, B. Prasai, S. Shastri and T.-Y. Chen, 3D Atomic Arrangement at Functional Interfaces Inside Nanoparticles by Resonant High-Energy X-ray Diffraction, ACS Appl. Mater. Interfaces, 2015, 7, 23265–23277 CrossRef CAS.
- P. J. Chupas, K. W. Chapman, G. Jennings, P. L. Lee and C. P. Grey, Watching Nanoparticles Grow: The Mechanism and Kinetics for the Formation of TiO2-Supported Platinum Nanoparticles, J. Am. Chem. Soc., 2007, 129, 13822–13824 CrossRef CAS PubMed.
- M. A. Newton, K. W. Chapman, D. Thompsett and P. J. Chupas, Chasing Changing Nanoparticles with Time-Resolved Pair Distribution Function Methods, J. Am. Chem. Soc., 2012, 134, 5036–5039 CrossRef CAS PubMed.
- Y. Lei, H. Zhao, R. D. Rivas, S. Lee, B. Liu, J. Lu, E. Stach, R. E. Winans, K. W. Chapman, J. P. Greeley, J. T. Miller and P. J. Chupas, Adsorbate-Induced Structural Changes in 1–3 nm Platinum Nanoparticles, J. Am. Chem. Soc., 2014, 136, 9320–9326 CrossRef CAS PubMed.
- A. S. Masadeh, E. S. Božin, C. L. Farrow, G. Paglia, P. Juhas, S. J. L. Billinge, A. Karkamkar and M. G. Kanatzidis, Quantitative size-dependent structure and strain determination of CdSe nanoparticles using atomic pair distribution function analysis, Phys. Rev. B: Condens. Matter Mater. Phys., 2007, 76, 115413 CrossRef.
- V. V. T. Doan-Nguyen, S. A. J. Kimber, D. Pontoni, D. R. Hickey, B. T. Diroll, X. Yang, M. Miglierini, C. B. Murray and S. J. L. Billinge, Bulk Metallic Glass-like Scattering Signal in Small Metallic Nanoparticles, ACS Nano, 2014, 8, 6163–6170 CrossRef CAS PubMed.
- X. Tuaev, S. Rudi, V. Petkov, A. Hoell and P. Strasser, In Situ Study of Atomic Structure Transformations
of Pt-Ni Nanoparticle Catalysts during Electrochemical Potential Cycling, ACS Nano, 2013, 7, 5666–5674 CrossRef CAS PubMed.
- K. Page, T. C. Hood, T. Proffen and R. B. Neder, Building and refining complete nanoparticle structures with total scattering data, J. Appl. Crystallogr., 2011, 44, 327–336 CrossRef CAS.
- S. I. Sanchez, M. W. Small, E. S. Bozin, J.-G. Wen, J.-M. Zuo and R. G. Nuzzo, Metastability and Structural Polymorphism in Noble Metals: The Role of Composition and Metal Atom Coordination in Mono- and Bimetallic Nanoclusters, ACS Nano, 2013, 7, 1542–1557 CrossRef CAS PubMed.
- S. Banerjee, C.-H. Liu, J. D. Lee, A. Kovyakh, V. Grasmik, O. Prymak, C. Koenigsmann, H. Liu, L. Wang, A. M. M. Abeykoon, S. S. Wong, M. Epple, C. B. Murray and S. J. L. Billinge, Improved Models for Metallic Nanoparticle Cores from Atomic Pair Distribution Function (PDF) Analysis, J. Phys. Chem. C, 2018, 122, 29498–29506 CrossRef CAS.
- R. L. McGreevy and L. Pusztai, Reverse monte carlo simulation: a new technique for the determination of disordered structures, Mol. Simul., 1988, 1, 359–367 CrossRef.
- R. L. McGreevy, Reverse Monte Carlo modelling, J. Phys.: Condens. Matter, 2001, 13, R877–R913 CrossRef CAS.
- V. Petkov, B. N. Wanjala, R. Loukrakpam, J. Luo, L. Yang, C.-J. Zhong and S. Shastri, Pt-Au Alloying at the Nanoscale, Nano Lett., 2012, 12, 4289–4299 CrossRef CAS PubMed.
- N. A. Merrill, E. M. McKee, K. C. Merino, L. F. Drummy, S. Lee, B. Reinhart, Y. Ren, A. I. Frenkel, R. R. Naik, N. M. Bedford and M. R. Knecht, Identifying the Atomic-Level Effects of Metal Composition on the Structure and Catalytic Activity of Peptide-Templated Materials, ACS Nano, 2015, 9, 11968–11979 CrossRef CAS PubMed.
- K. M. Ø. Jensen, P. Juhas, M. A. Tofanelli, C. L. Heinecke, G. Vaughan, C. J. Ackerson and S. J. L. Billinge, Polymorphism in magic-sized Au144(SR)60 clusters, Nat. Commun., 2016, 7, 11859 CrossRef CAS PubMed.
- L. S. R. Kumara, O. Sakata, S. Kohara, A. Yang, C. Song, K. Kusada, H. Kobayashi and H. Kitagawa, Origin of the catalytic activity of face-centered-cubic ruthenium nanoparticles determined from an atomic-scale structure, Phys. Chem. Chem. Phys., 2016, 18, 30622–30629 RSC.
- S. J. Gurman and R. L. McGreevy, Reverse Monte Carlo simulation for the analysis of EXAFS data, J. Phys.: Condens. Matter, 1990, 2, 9463–9473 CrossRef.
- M. Winterer, Reverse Monte Carlo analysis of extended x-ray absorption fine structure spectra of monoclinic and amorphous zirconia, J. Appl. Phys., 2000, 88, 5635–5644 CrossRef CAS.
- A. Di Cicco and A. Trapananti, Reverse Monte Carlo refinement of molecular and condensed systems by x-ray absorption spectroscopy, J. Phys.: Condens. Matter, 2005, 17, S135–S144 CrossRef CAS.
- K. Németh, K. W. Chapman, M. Balasubramanian, B. Shyam, P. J. Chupas, S. M. Heald, M. Newville, R. J. Klingler, R. E. Winans, J. D. Almer, G. Sandi and G. Srajer, Efficient simultaneous reverse Monte Carlo modeling of pair-distribution functions and extended x-ray-absorption fine structure spectra of crystalline disordered materials, J. Chem. Phys., 2012, 136, 074105 CrossRef PubMed.
- A. Di Cicco, A. Trapananti, S. Faggioni and A. Filipponi, Is There Icosahedral Ordering in Liquid and Undercooled Metals?, Phys. Rev. Lett., 2003, 91, 135505 CrossRef PubMed.
- A. Di Cicco, F. Iesari, S. De Panfilis, M. Celino, S. Giusepponi and A. Filipponi, Local fivefold symmetry in liquid and undercooled Ni probed by x-ray absorption spectroscopy and computer simulations, Phys. Rev.
B: Condens. Matter Mater. Phys., 2014, 89, 060102 CrossRef.
- M. Matsuura, W. Zhang, S. Yamaura, T. Fujita, K. Ohara, S. Kohara and J. Mizuno, Structural Features of the Extraordinary Low Glass Transition Temperature for Au65Cu18Si17 Bulk Metallic Glass, Mater. Trans., 2013, 54, 1351–1355 CrossRef CAS.
- J. Akola, S. Kohara, K. Ohara, A. Fujiwara, Y. Watanabe, A. Masuno, T. Usuki, T. Kubo, A. Nakahira, K. Nitta, T. Uruga, J. K. R. Weber and C. J. Benmore, Network topology for the formation of solvated electrons in binary CaO-Al2O3 composition glasses, Proc. Natl. Acad. Sci. U. S. A., 2013, 110, 10129–10134 CrossRef CAS.
- D. Wakabayashi, N. Funamori, T. Kikegawa, K. Watanabe, S. Kohara, H. Nitani, Y. Niwa, Y. Takeichi, H. Abe and M. Kimura, Nature of the transformation in liquid iodine at 4 GPa, Phys. Rev. B, 2017, 96, 024105 CrossRef.
- A. Di Cicco, F. Iesari, A. Trapananti, P. D'Angelo and A. Filipponi, Structure and atomic correlations in molecular systems probed by XAS reverse Monte Carlo refinement, J. Chem. Phys., 2018, 148, 094307 CrossRef.
- V. Krayzman, I. Levin, J. C. Woicik, T. Proffen, T. A. Vanderah and M. G. Tucker, A combined fit of total scattering and extended X-ray absorption fine structure data for local-structure determination in crystalline materials, J. Appl. Crystallogr., 2009, 42, 867–877 CrossRef CAS.
- V. Krayzman, I. Levin and M. G. Tucker, Simultaneous reverse Monte Carlo refinements of local structures in perovskite solid solutions using EXAFS and the total scattering pair-distribution function, J. Appl. Crystallogr., 2008, 41, 705–714 CrossRef CAS.
- S. Kohara, M. Itou, K. Suzuya, Y. Inamura, Y. Sakurai, Y. Ohishi and M. Takata, Structural studies of disordered materials using high-energy x-ray diffraction from ambient to extreme conditions, J. Phys.: Condens. Matter, 2007, 19, 506101–506115 CrossRef.
- S. J. L. Billinge and M. G. Kanatzidis, Beyond crystallography: the study of disorder, nanocrystallinity and crystallographically challenged materials with pair distribution functions, Chem. Commun., 2004, 749–760 RSC.
- M. Harada and Y. Inada, In Situ Time-Resolved XAFS Studies of Metal Particle Formation by Photoreduction in Polymer Solutions, Langmuir, 2009, 25, 6049–6061 CrossRef CAS PubMed.
- M. Newville, IFEFFIT: interactive XAFS analysis and FEFF fitting, J. Synchrotron Radiat., 2001, 8, 322–324 CrossRef CAS PubMed.
- B. Ravel and M. Newville, ATHENA, ARTEMIS, HEPHAESTUS: data analysis for X-ray absorption spectroscopy using IFEFFIT, J. Synchrotron Radiat., 2005, 12, 537–541 CrossRef CAS PubMed.
- O. Gereben and L. Pusztai, RMC_POT: A Computer Code for Reverse Monte Carlo Modeling the Structure of Disordered Systems Containing Molecules of Arbitrary Complexity, J. Comput. Chem., 2012, 33, 2285–2291 CrossRef CAS PubMed.
- A. L. Ankudinov, B. Ravel, J. J. Rehr and S. D. Conradson, Real-space multiple-scattering calculation and interpretation of x-ray-absorption near-edge structure, Phys. Rev. B: Condens. Matter Mater. Phys., 1998, 58, 7565–7576 CrossRef CAS.
- J. J. Rehr and R. C. Albers, Theoretical approaches to x-ray absorption fine structure, Rev. Mod. Phys., 2000, 72, 621–654 CrossRef CAS.
- R. W. G. Wyckoff, Crystal Structures, Interscience Publishers, New York, 2nd edn, 1963, vol. 1 Search PubMed.
- Q. Li, H. Zhu, L. Zheng, L. Fan, N. Wang, Y. Rong, Y. Ren, J. Chen, J. Deng and X. Xing, Local Chemical Ordering and Negative Thermal Expansion in PtNi Alloy Nanoparticles, Nano Lett., 2017, 17, 7892–7896 CrossRef CAS PubMed.
- L. S. R. Kumara, O. Sakata, H. Kobayashi, C. Song, S. Kohara, T. Ina, T. Yoshimoto, S. Yoshioka, S. Matsumura and H. Kitagawa, Hydrogen storage and stability properties of Pd-Pt solid-solution nanoparticles revealed via atomic and electronic structure, Sci. Rep., 2017, 7, 14606 CrossRef PubMed.
Footnote |
† Electronic supplementary information (ESI) available: TEM images and particle size distributions of the diameter for Pt, Pd, and Rh nanoparticles prepared by the photoreduction; Fourier transforms of k3χ(k) EXAFS spectra of the Pt, Pd, and Rh nanoparticles; distributions of the coordination numbers (CNs) in the first and second coordination shell for the nanoparticles; distributions of bond angles derived from the RMC simulations in the analysis of EXAFS data. See DOI: 10.1039/c9ra06519a |
|
This journal is © The Royal Society of Chemistry 2019 |
Click here to see how this site uses Cookies. View our privacy policy here.