DOI:
10.1039/C9RA03740C
(Paper)
RSC Adv., 2019,
9, 23373-23381
Chemical and genetic discrimination of commercial Guangchenpi (Citrus reticulata ‘Chachi’) by using UPLC-QTOF-MS/MS based metabolomics and DNA barcoding approaches†
Received
18th May 2019
, Accepted 2nd July 2019
First published on 29th July 2019
Abstract
CRP (Citri Reticulatae Pericarpium), a famous traditional Chinese medicine, has also been extensively used in foods and condiments in dietary practice for centuries. According to the Chinese Pharmacopeia (2015 edition) it contains two subtypes, Guangchenpi (GCP) and Chenpi (CP). GCP exclusively originates from the pericarp of Citrus reticulata ‘Chachi’ cultivar and it's generally believed that GCP has superior qualities compared with the other main cultivars (CP). In the present study, an integrated approach combining LC-QTOF MS-based untargeted metabolomics analysis and DNA barcoding molecular identification was conducted to study the genetic diversity and chemical differences between GCP and CP. A validated UPLC-QTOF MS metabolomics method was established to identify markers by using PCA and OPLS-DA models. 34 identified metabolites could be used as chemical markers to distinguish effectively between the two subtypes. Among them polymethoxyflavones (PMF) such as hexamethoxyflavone (nobiletin and natsudaidain), pentamethoxyflavone (tangeretin and sinensetin), and tetramethoxyflavone are the most influential markers. Support vector machines were employed to classify all the samples and these markers showed good prediction accuracy (100%). The results of DNA barcoding showed that the secondary structure of the ITS2 sequences were significantly different among GCP and other three cultivars. The study indicated the integrated method could be a powerful and reliable analytical tool for differentiating GCP from CP.
1. Introduction
Citri Reticulate Pericarpium (CRP), the dried matured tangerine pericarp, is one of the most well-known Chinese medicinal materials which has long been extensively used for health purposes. According to traditional Chinese Medicine theory, CRP has been used as a Qi-regulating herb to treat nausea, vomiting, indigestion, anepithymia, diarrhea, coughs, expectoration, and so on.1 Besides a medical purpose, CRP has been frequently used in foods, drinks, snacks, health-care products and condiments in China and Southeast Asia.2 In recent years, many researchers have focused on the pharmacological actions of CRP, such as beneficial effects on the gastrointestinal and respiratory systems, antioxidant, anti-inflammatory, and anti-cancer activities, as well as protective effects on the liver and nervous system.3–6
CRP is divided into two subtypes, Guangchenpi (GCP) and Chenpi (CP), as stated unambiguously in the current version of Chinese Pharmacopeia.7 Generally, GCP is defined as pericarp of a specific cultivar of Citrus reticulata, namely Citrus reticulata ‘Chachi’, while other main cultivars refer to CP, including Citrus reticulata ‘Dahongpao’, Citrus reticulata ‘Unshiu’, Citrus reticulata ‘Tangerina’, and so on. As a rule, Citrus reticulata ‘Chachi’, cultivated and harvested in a limited area, Xinhui county of Guangdong Province in China, is regarded as the genuine source of GCP,8 while CP is widely distributed cultivated within China. In spite of moderate differences in morphological traits between CP and GCP, their commercial products are frequently confused as some morphological traits have been lost after processing. There is no systemic method to distinguish GCP and CP, however, the current method relies on visual and morphological evaluation by artificial experience. According to recent studies, chemical composition of GCP and CP often vary among varieties, which could affect their quality and pharmacological effects.9,10 Thus, it is imperative to investigate their genetic and chemical differences for discrimination and quality control of these similar subtypes.
DNA barcoding methods have been used to overcome the limitations of morphological identification in plant discrimination based on the DNA sequences, such as the ITS region of nrDNA, and chloroplast DNA (cpDNA) rbcL, psbA-trnH, and matK.11,12 Previous studies have demonstrated the ability of DNA barcoding to identify Citrus plants.13 The internal transcribed spacer 2 (ITS2) which is part of the eukaryotic nuclear rDNA cistron and lies between the 5.8S and 28S rDNA, as a novel DNA barcode for identifying medicinal plant species.14 Compared with other sequences, the advantage of ITS2 is the standard sequence that can be obtained through the website annotation (http://its2.bioapps.biozentrum.uni-wuerzburg.de/)15 and predicted secondary structure of ITS2 from the database helps us to learn more about the genetic information of species for identification. A previous study has shown that there are differences in the secondary structures of ITS2 sequence between Citrus reticulata ‘Chachi’ and other two cultivars.16
Recently developed metabolomics analysis method is regarded as a powerful technology that could systematically and comprehensively investigate the metabolic profiles in different samples, which enable us to reveal the differences of chemical features among the closely related species of traditional Chinese Medicines.9,10 Previously studies have successfully applied GC-MS and/or LC-MS based metabolomics analysis for discriminating the Citrus genus products such as the Citrus peel,9 fruit,17 and/or fruit juice.18 However, basing on a synthetic strategy combing metabolomics analysis and DNA barcoding methods could be a more practical solution to make integrated evaluation on their cultivar differentiation.
In present study, 51 batches of commercial CRP samples, including four cultivars originated from Hunan, Jiangxi, Zhejiang, Hubei, Sichuan and Guangdong province in China were collected. We aim to clarify the genetic and chemical diversities between GCP and CP by combining deoxyribonucleic acid (DNA) barcoding method using mainly sequences of ITS2 and psbA-trnH, with a metabolomics approach using ultra-performance liquid chromatography coupled with quadrupole time-of-flight mass spectrometry (UPLC-QTOF-MS/MS).
2. Experimental
2.1 Plant materials
In present study, a total of 51 batches of CRP samples were purchased from herbal medicine market in southern and eastern China, including 20 batches of GCP samples from Xinhui county (Guangdong province), the genuine GCP producing area and 31 CP collected from local herb market in Sichuan, Zhejiang, Jiangxi and Hunan, Hubei province, respectively (Table S1†). The samples were authenticated by Professor Guangxiong Zhou (Pharmacy College, Jinan University, Guangzhou, China) and the voucher specimens were saved in China Academy of Chinese Medical sciences Guangdong Branch, Guangzhou.
2.2 Chemicals and materials
2-Mercaptoethanol, LC grade acetonitrile and methanol were purchased from Merck (Darmstadt, Germany). EDTA, NaCl, HCl were of analytical reagent and manufactured by Tianjin Damao Chemical Reagent Factory (Tianjin, China). Tris–base and Agarose were purchased from Sigma Chemical Co. (MO, Missouri, USA). 2 × Taq PCR Mix was obtained from Aidlab Biotechnologies Co., Ltd (Beijing, China). LC-MS grade formic acid was obtained from Thermo Fisher Scientific Company China Branch (Shanghai, China). Analytical grade methanol was obtained from Guangzhou Chemical Reagent (Guangzhou, China). Deionized water (18 MΩ) was purified by a Mill-Q ultrapure water system (Millipore, USA). Reference compounds nobiletin, tangeretin, 3,5,6,7,8,3′,4′-heptamethoxyflavone, rutin, eriocitrin and hesperetin were obtained from Shanghai Yuanye Co, Ltd. (Shanghai, China).
2.3 LC-MS analysis
2.3.1 Sample preparation for LC-MS analysis. Each CRP sample was powdered by a mill, and passed through 60 mesh sieve. 0.2 g of each powdered sample was accurately weighed and extracted with 15 mL methanol in an ultrasonic bath (40 kHz, 250 W) for 30 min. After cooling at room temperature, the extracted solution was added with methanol to the original weight, and followed by centrifugation at 5000 r min−1 for 10 min. Then the supernatant was filtered through a 0.22 μm membrane prior to use.The quality control (QC) sample was used for validating the reproducibility and stability of the UPLC-QTOF-MS/MS methodology. It was made up by 20 μL of each CRP sample (prepared as described previously) and the mixed solution was vortexed for 1 min, and then filtered through a 0.22 μm membrane prior to use.
2.3.2 LC-MS conditions. The LC analysis was carried out on an ACQUITY™ UPLC™ H-CLASS instrument (Waters Corp., Milford, MA, USA) equipped with an automatic degasser, a quaternary pump, a column oven and an autosampler. Separation was performed on a Waters ACQUITY™ UPLC™ HSS T3 column (100 × 2.1 mm, 1.7 μm) at 30 °C. The mobile phase consisted of solvent A (water containing 0.1% formic acid) and solvent B (acetonitrile), and the elution program was optimized as follows: 0–3.5 min, 5–15% B; 3.5–6 min, 15–25% B; 6–15 min, 25–40% B; 15–18 min, 40–55% B; 18–20 min, 55–85% B; 20–22 min, 85–95% B. The flow rate was set to 0.2 mL min−1, with an injection volume set to 2 μL.The MS analysis was performed on a TripleTOF™ 5600+ mass spectrometer (AB SCIEX, Foster City, CA, USA) equipped with a DuoSpray™ Electron Spray Ionization (ESI) source in both positive and negative modes. The ESI condition was applied with the following parameters: ion spray voltage, 4500 V in positive mode (−4500 V in negative mode); ion source temperature, 500 °C; curtain gas, 25 psi; nebulizer gas (GS1) 50 psi; heater gas (GS2), 50 psi; and declustering potential (DP), 80 V (−80 V in negative mode). The mass range was set between m/z 120–1200 in both positive and negative modes. The MS scanning program consisted of a TOF MS survey scan and MS/MS scans of eight most intense ions using the information-dependent acquisition (IDA) mode, that was set for structural analysis of potential biomarkers. The IDA collision energy (CE) was set at 35 eV in positive mode (−35 eV in negative mode), and the collision energy spread (CES) was (±) 10 eV. For metabolomics analysis, MS survey scanning was only applied in an independent MS method, in which all the collected data were only used for chemometrics analysis.
2.3.3 Data processing, statistical analysis and chemical metabolite identification. The raw LC-MS data were extracted and aligned by MarkerView v1.2.1 software (AB SCIEX, Foster City, CA, USA) with default parameters setting except the binning and alignment settings were “RT window” = 0.2 min and “mass window” = 10 ppm. The data matrix were normalized by using Total Area Sums function and the normalized data were then exported into the SIMCA-P 14.1 (Umetrics, Sweden), which was used for multivariate statistical analyses including principal component analysis (PCA) and orthogonal partial least squares discriminant analysis (OPLS-DA).19 The data matrix was scaled using the mean centering and Pareto scaling algorithm for PCA and OPLS-DA analysis by SIMCA-P, respectively. First, unsupervised PCA was performed to obtain the overview of the relationships among the data matrix. Then, supervised orthogonal partial least-squares discriminate analysis (OPLS-DA) was used to distinguish the differences of metabolites between GCP and CP. The established model was assessed by calculating the R2 and Q2 values. A large R2 (close to 1) indicate the established model is a good model. A large Q2 (Q2 > 0.5) indicates how well the model predicts new data based on cross-validation and good predictability.20 Permutation test was used to validate the OPLS-DA model. Variables importance in projection (VIP) value was calculated from the OPLS-DA model. Components were selected as potential variables when the VIP value was more than 1.5. SPSS 23.0 (IBM, USA) software was used to perform Mann–Whitney U test for these variables. Metabolites with p < 0.05 and VIP value > 1.5 were considered to be statistically significant. A support vector machines (SVM) model for prediction and classification of CRP samples was conducted by the package e1071 of free R language platform.The accurate mass determination of their precursor ions and MS/MS fragments were conducted by using the high resolution UPLC-QTOF-MS/MS. The mass error between theoretical mass value and experimental mass value was less than 5 ppm. The potential biomarkers were identified or tentatively identified by matching the molecular formulas and key fragment ions and pathways with that of standards and compounds previously identified from the same plant source.
2.3.4 Validation of analytical method. In order to ensure the reliability of the analysis method, the QC sample has been used to validate reproducibility of the method and precision of UPLC-QTOF-MS/MS instrument by duplicate analysis of six injections. In addition, during the CRP detection, the QC sample was analyzed once every 10 experimental samples. Then the retention times and intensities of 10 randomly selected peaks from the chromatograms of all the QC samples were determined using PeakView software 1.2.0 and statistical analysis of relative standard deviations (RSD) was performed to validate the analytical method. The results showed that the RSD of typical ion intensities and retention time values were less than 10% and 0.2%, respectively.
2.4 DNA barcoding experiment
2.4.1 Sample pretreatment for DNA extraction. 50 mg of each dried powered CRP sample was added into a 2 mL tube and ground into fine powder by a Precellys 24 Homogenizer (Bertin Technologies Co. France). Then the powder was mixed with 800 μL pretreatment extracting buffer (containing 0.1 mol L−1 Tris–HCl, 0.02 mol L−1 EDTA, 4% (w/v) NaCl and 2% (v/v) 2-mercaptoethanol) for 5 min, further centrifuged at 10
000 rpm for 1 min, and then discarded the supernatant.
2.4.2 DNA extraction, PCR, sequencing and analysis. A DP305 Plant Genomic DNA Kit (TIANGEN Biotech Company, Beijing, China) was used for genomic DNA extraction. Extracting operation steps were carried out in accordance with the specification. The extracted total DNA samples were stored in the TE buffer at −20 °C until use. Polymerase chain reactions (PCR) were performed to amplify the internal transcribed spacer 2 (ITS2),21 and the non-coding region between the chloroplast psbA gene and the trnH gene (psbA-trnH)The universal primers used for the amplification of ITS2 region were:
S2F: 5′-ATGCGATACTTGGTGTGAAT-3′; |
S3R: 5′-GACGCTTCTCCAGACTACAAT-3′. |
The primers for psbA-trnH regions were:
fwd PA: 5′-GTTATGCATGAACGTAATGCTC-3′; |
rev TF: 5′-CGCGCATGGTGGATTCACAATCC-3′. |
Each reaction mixture (25 μL) contained 2 × Taq PCR Mix 12.50 μL, 1.0 μL of each 0.25 μM forward and reverse primer, 2.0 μL DNA template, and ddH2O 8.5 μL. The PCR protocol used to amplify ITS2 was as follows: initial denaturation at 94 °C for 5 min, 35 cycles of 94 °C for 30 s, 56 °C for 30 s, 72 °C 45 s, then final extension at 72 °C for 10 min, and hold at 4 °C. The PCR program for psbA-trnH was: 94 °C for 5 min, 30 cycles of 94 °C for 1 min, 55 °C for 1 min, 72 °C 1.5 min, and final extension at 72 °C for 7 min, before cooling down at 4 °C. PCR reactions were carried out on a ProFlexTM PCR system (Thermo fisher Scientific, USA). Amplicons were examined on 1% agarose gels electrophoresis and visualized under ultraviolet light. The PCR products with bright and single band were selected, and sent for sequencing by Majorbio Com. (Guangzhou, China). The sequences were assembled using CodonCode Aligner v6.0.2 (USA). ITS2 sequence annotations were carried out on the website (http://its2.bioapps.biozentrum.uni-wuerzburg.de/) with Hidden Markov model (HMMer) and predicted the secondary structures of ITS2. Then the sequences were analyzed using MEGA 6.0 (Molecular Evolutionary Genetics analysis v6.0). Genetic distance within interspecies was calculated with K2P model.
3. Results
3.1 Discrimination of GCP and CP based on LC-MS multivariate analysis
The LC-MS data of 51 batches of CRP samples were obtained by an optimized UPLC-QTOF-MS/MS method and the typical base peak chromatograms (BPCs) were shown in Fig. 1. The raw data preliminarily processed by MarkerView v1.2.1 software were exported into SIMCA-P (14.1) for PCA and OPLS-DA multivariate analysis. An obvious two separated trends among GCP and CP were observed in PCA score plot both of in positive and negative ion modes (Fig. 2A and C). Principal component 1 and component 2 accounted for 30.1% and 15.1% of total variance of normalized LC-MS data in the positive modes (29.0% and 15.0% in the negative modes), respectively. In order to further identify the chemotypic variation between GCP and CP and to find potential chemical markers, a supervised OPLS-DA method was adopted. The result of OPLS-DA model derived from data both of the positive modes and negative modes analysis was displayed in Fig. 2B and D showed clear separation among the two groups. In positive mode the relevant R2Y = 0.989 and Q2 = 0.974 (and in negative mode the relevant R2Y = 0.994 and Q2 = 0.982) indicated a good predictability and goodness-of-fit of the model. The 200 iterations permutation test was applied to assess the predictability of the model (Fig. S1†).
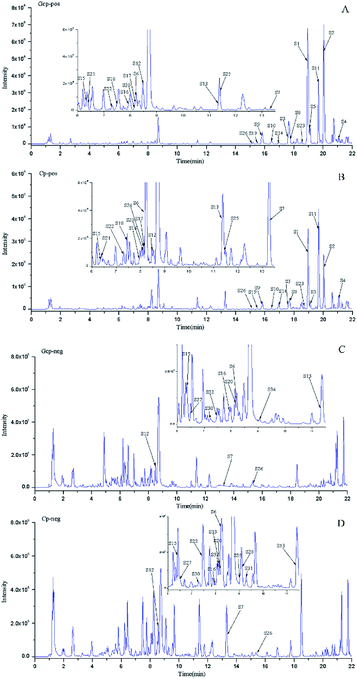 |
| Fig. 1 The typical base peak chromatograms (BPC) of GCP and CP samples in positive and negative modes. (A) BPC of GCP in positive MS mode; (B) BPC of CP in positive MS mode; (C) BPC of GCP in negative MS mode; and (D) BPC of CP in negative MS mode. | |
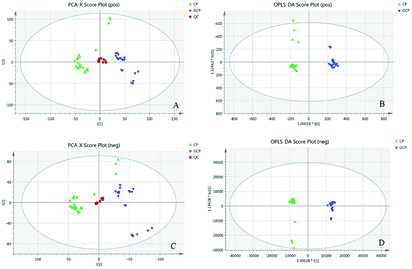 |
| Fig. 2 (A) PCA score plot in positive mode (R2X = 0.873, R2Q = 0.723). (B) OPLS-DA score plot in positive mode with statistical parameters (R2X = 0.656, R2Y = 0.989, Q2 = 0.974). (C) PCA score plot in negative mode (R2X = 0.888, R2Q = 0.741). (D) OPLS-DA score plot in negative mode with statistical parameters (R2X = 0.549, R2Y = 0.994, Q2 = 0.982). Blue triangles represent GCP samples, green triangle represent CP samples and pentacles represent QC samples. | |
3.1.1 Selection, identification of the potential chemical markers. According to variable importance of the project (VIP), the differentiated metabolites with VIP value > 1.5 from the OPLS-DA models were selected for further Mann–Whitney U-test using SPSS 23.0. The metabolites that VIP > 1.5 and p < 0.05 were selected as the potential chemical markers (S-plots see Fig. S2†). Based on the MS information of standard references and compounds from previously reported literatures, the accurate mass determination of their precursor ions and MS/MS fragments were conducted by using the high resolution UPLC-QTOF-MS/MS for identification of the chemical markers. Consequently, 34 metabolites were identified and selected as chemical markers by comparison of the differences between GCP and CP (Table 1). All of the identified compounds were marked in the represented chromatogram of GCP and CP sample in positive and negative mode (Fig. 1). The mean MS intensities of 34 metabolites between GCP and CP are shown in Fig. 3, indicating their main chemical differences. The differences of chemical markers in GCP and CP by the heatmap are shown in Fig. S3.†
Table 1 Identification of potential chemical markersa
No. |
Compounds |
MF |
Retention time |
Ion mode |
m/z |
Mass error (ppm) |
MS2 |
VIP |
The compound with “*” means it was compared with reference standard; the p-value <0.05. |
S1 |
Nobiletin* |
C21H22O8 |
18.88 |
[M + H]+ |
403.1391 |
0.9 |
388; 387; 373; 355; 330; 327; 211; 183 |
24.4378 |
S2 |
Tangeretin* |
C20H20O7 |
20.02 |
[M + H]+ |
373.1279 |
−0.7 |
358; 343; 328; 325; 300; 297; 271; 211; 183; 135 |
24.445 |
S3 |
Sinensetin |
C20H20O7 |
17.58 |
[M + H]+ |
373.1284 |
0.6 |
358; 357; 343; 329; 315; 312; 297; 153; 151 |
11.1517 |
S4 |
Natsudaidai |
C21H22O9 |
21.07 |
[M + H]+ |
419.1334 |
−0.6 |
404; 389; 371; 361; 343; 165 |
12.0638 |
S5 |
Tetramethyl-O-isoscutellarin |
C19H18O6 |
19.06 |
[M + H]+ |
343.1187 |
3.2 |
327; 299; 282; 285; 267; 253; 153 |
9.3755 |
S6 |
Narirutin |
C27H32O14 |
8.24 |
[M + H]+ |
581.1858 |
−1.2 |
435; 419; 401; 383; 339; 315; 273; 263; 195; 153; 129 |
11.4284 |
S7 |
Melitidin |
C33H40O18 |
13.23 |
[M + H]+ |
725.2277 |
−1.4 |
419; 404; 389 |
10.5253 |
S8 |
Tetramethyl-O-scutellarin |
C19H18O6 |
17.64 |
[M + H]+ |
343.1178 |
0.5 |
328; 313; 285; 181 |
8.59813 |
S9 |
Isosinensetin |
C20H20O7 |
15.82 |
[M + H]+ |
373.1282 |
1.1 |
358; 357; 343; 315; 181; 153 |
6.99732 |
S10 |
Monohydroxy-pentamethoxyflavone |
C20H20O8 |
16.38 |
[M + H]+ |
389.1232 |
0.3 |
374; 359; 313; 287 |
5.77288 |
S11 |
3,5,6,7,8,3′,4′-heptamethoxyflavone* |
C22H24O9 |
19.61 |
[M + H]+ |
433.1490 |
−0.7 |
433; 418; 417; 403; 385; 342; 165 |
4.08285 |
S12 |
Diosmin |
C28H32O15 |
8.49 |
[M + H]+ |
607.1679 |
1.7 |
299; 284 |
5.35966 |
S13 |
Didymin |
C28H34O14 |
11.34 |
[M + H]+ |
595.2010 |
−1.9 |
449; 397; 329; 287; 263; 153 |
7.48591 |
S14 |
Hexamethoxyflavone |
C21H22O8 |
16.95 |
[M + H]+ |
403.1386 |
−0.4 |
403; 388; 387; 373; 345; 327; 165 |
3.99101 |
S15 |
Diosmetin-6,8-di-C-glucoside |
C28H32O16 |
6.34 |
[M + H]+ |
625.1750 |
−2.1 |
607; 589; 571; 487; 439; 409; 367; 355; 325; 313 |
3.21375 |
S16 |
Diosmetin-6-C-glucoside |
C22H22O11 |
7.94 |
[M + H]+ |
463.1231 |
−0.8 |
445; 427; 367; 343; 325; 313; 301; 151 |
3.49067 |
S17 |
Monohydroxy-hexamethoxyflavone |
C21H22O9 |
8.22 |
[M + H]+ |
419.1331 |
−1.3 |
401; 383; 273; 263; 245; 219; 195; 165 |
3.2909 |
S18 |
Rutin* |
C27H30O16 |
7.51 |
[M + H]+ |
611.1594 |
−2.1 |
465; 303; 129 |
3.33815 |
S19 |
5-Hydroxy-6,7,8,3′-4′-pentamethoxyflavone |
C20H20O8 |
15.49 |
[M + H]+ |
389.1227 |
−1.0 |
374; 359; 341; 316; 197 |
2.52485 |
S20 |
Isorhoifolin |
C27H30O14 |
8.16 |
[M + H]+ |
579.1697 |
−2.0 |
433; 271 |
2.60807 |
S21 |
Natsudaidain-3-O-β-D-glucoside |
C27H32O14 |
6.39 |
[M + H]+ |
581.1850 |
−2.6 |
419; 383; 339; 273; 263 |
3.37778 |
S22 |
Eriocitrin* |
C27H32O15 |
7.44 |
[M + H]+ |
597.1801 |
−2.2 |
451; 435; 355; 289; 287; 195; 153 |
3.31181 |
S23 |
Pentamethoxyflavone |
C20H20O7 |
18.71 |
[M + H]+ |
373.1279 |
−0.7 |
358; 373; 343; 315; 297; 135 |
2.24149 |
S24 |
Naringenin isomer |
C15H12O5 |
8.22 |
[M + H]+ |
273.0754 |
−1.3 |
153; 147 |
2.4109 |
S25 |
Apigenin-8-C-glucoside |
C22H24O9 |
11.38 |
[M + H]+ |
433.1482 |
−2.6 |
415; 397; 379; 353; 337; 287; 263; 245; 219; 195; 161 |
1.99918 |
S26 |
Hesperetin* |
C16H14O6 |
15.36 |
[M + H]+ |
303.0861 |
−0.7 |
243; 179; 177; 153 |
1.96456 |
S27 |
3′,5,7-Trihydroxy-4′-methoxyflavanonol-7-O-rutinoside |
C28H34O16 |
6.48 |
[M − H]− |
625.1779 |
0.8 |
597; 317; 313; 289 |
4.44001 |
S28 |
5,7,4′-Trihydroxy-8,3′-dimethoxy flavonol-3-O-[2′′/6′′-(3-hydroxy-3-methylglutaryl)]-glucoside |
C29H32O17 |
9.06 |
[M − H]− |
651.1580 |
2.0 |
589; 549; 507; 345; 330; 315; 287 |
9.02038 |
S29 |
Limocitrol or isolimocitrol-3-O-[6′′-(3-hydroxy-3-methylglutaryl)]-glucoside |
C30H34O18 |
9.01 |
[M − H]− |
681.1684 |
1.7 |
619; 579; 537; 375; 360; 359 |
3.49999 |
S30 |
Apigenin-C-glucosyl-O-xyloside |
C26H28O14 |
7.29 |
[M − H]− |
563.1470 |
0.1 |
443; 413; 323; 313; 293 |
3.02874 |
S31 |
Isorhamnetin-3-O-[6′′-(3-hydroxy-3-methylglutaryl)]-glucoside |
C28H30O16 |
9.25 |
[M − H]− |
621.1470 |
1.4 |
559; 519; 477; 417; 315 |
3.51437 |
S32 |
Nicotiflorin |
C27H30O15 |
8.09 |
[M − H]− |
593.1515 |
0.5 |
285; 284; 255 |
2.30776 |
S33 |
8-Methoxyquercetin-3-O-[6′′-(3-hydroxy-3-methylglutaroyl)]-glucoside |
C28H30O17 |
8.07 |
[M − H]− |
637.1413 |
0.4 |
575; 535; 493; 331; 330; 316; 315 |
2.56254 |
S34 |
Diosmetin-7-O-glucoside |
C22H22O11 |
8.99 |
[M − H]− |
461.1087 |
−0.5 |
446; 415; 371; 341; 313; 299; 298; 297; 283; 255 |
1.94619 |
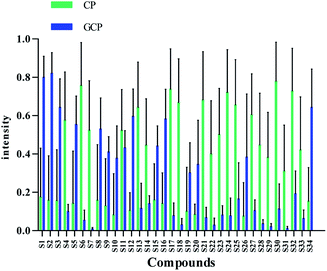 |
| Fig. 3 Differences of the 34 chemical markers between GCP and CP. | |
Flavonoids are abundant in CRP. In our present study, most of the chemical markers responsible for differentiating GCP from CP are flavonoids especially polymethoxyflavones (PMF). The typical flavonoids in CRP such as nobiletin, tangeretin, 3,5,6,7,8,3′,4′-heptamethoxyflavone, rutin, eriocitrin, hesperetin were identified by comparing the accurate mass determination and the retention time with those of reference standards. The detailed MS information of other chemical components were listed in Table 1 and they were identified based on accurate mass determination of precursor ions and their fragment peaks, which were compared with relevant literatures.22–24 Take S12 for example, it gave a [M − H]− ion at m/z 607.1679, which produced the base fragment ion at m/z 299, which refers to the aglycone residue, as well as a characteristic odd-electron ion at m/z 284 [aglycone–·CH2–H]− in the MS2 spectrum. By comparing with related literatures, it was identified as diosmin.22 The basic fragmentation pathways of this compound was shown on Fig. 4.
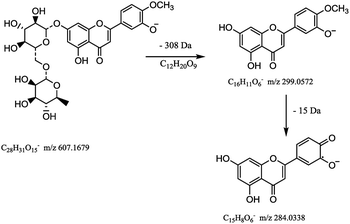 |
| Fig. 4 The MS fragmentation pathways of diosmin. | |
3.1.2 Support vector machine (SVM). Support vector machine is a supervised learning model with the idea of separating data and sequential minimal optimization algorithm, which is regards as a promising tool for data mining and classification. After training on set data, SVM can be used to predict the objects whose values are unknown. Previous studies had successfully employed it to predict and classify the species of Clematidis Radix et Rhizoma25 and for Citrus herbs.24 To demonstrate the capability of the marker compounds in classification and prediction of the CRP samples, 25 markers obtained from positive LC-MS data were selected as the inputs to build a 25-dimentional dataset for performing SVM model on the free R platform. Three vectors (GCP, CP and QC) were applied as outputs. To enhance the model accuracy and prevent data over fitting, 5-fold cross-validation was applied. 47 batches of samples (including QC samples) were randomly treated as a training set to build the model. In the SVM ( ) function, type is C-classification and kernel is radial and the two parameters is optimized as γ = 0.05 and C = 1 respectively. As showed in Table S2,† the model have an excellent predication accuracy of 100% for the training set and the otherwise test set of 13 samples. The results demonstrate that the markers show potential powerful ability of discriminating GCP from CP.
3.2 ITS2 sequences analysis
According to the optimized DNA extraction protocol, the total DNA of all CRP samples were successfully extracted and the ITS2 sequences were further amplified and sequenced. The ITS2 regions were aligned and assembled by Codoncode Aligner, and then further annotated on the website (http://its2.bioapps.biozentrum.uni-wuerzburg.de/). Differences of the ITS2 sequence properties between GCP (the pericarp of Citrus reticulata ‘Chachi’) and CP (Citrus reticulata ‘Dahongpao’, Citrus reticulata ‘Unshiu’, and Citrus reticulata ‘Tangerina’ in present study) are shown in Table 2. The standard ITS2 sequence length of both Citrus reticulata ‘Chachi’ and Citrus reticulata ‘Dahongpao’ was 232 bp and the GC percentage content was 71.55%. The interspecies distance between the two cultivars was 0.001, indicating that the two genetic information in ITS2 sequences are very close. Citrus reticulata ‘Unshiu’ and Citrus reticulata ‘Tangerina’ showed higher genetic distances and lower GC content. A total of 10 variation sites were detected in 51 GCP and CP ITS2 sequences, including C–G mutations at 11, 37 and 118 sites; C–T mutations at 83 and 197 sites; C–A mutations on 103 site; G–A mutations at 183, 199 sites; G–T mutations at 188 sites, and one insertion or deletion 149 site. Most of the variation sites are consistent with previous report.16 The characteristics of the dominant haplotype sequences were shown on the Fig. 5.
Table 2 Properties of ITS2 of Citrus reticulata
Sample |
Base number |
GC (%) |
Genetic distance |
Citrus reticulata ‘Chachi’ |
232 |
71.55 |
— |
Citrus reticulata ‘Dahongpao’ |
232 |
71.55 |
0.001 |
Citrus reticulata ‘Unshiu’ |
231 |
69.26 |
0.031 |
Citrus reticulata ‘Tangerina’ |
231 |
69.26 |
0.035 |
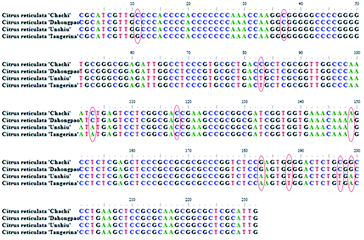 |
| Fig. 5 The characteristics of the dominant haplotype sequence of four species (the variation sites were framed). | |
The ITS2 secondary structures of these GCP and CP samples were predicted according to the database and website (http://its2.bioapps.biozentrum.uni-wuerzburg.de/) displayed on the Fig. 6. It was shown that the ITS2 secondary structure of Citrus reticulata ‘Chachi’ were significantly different. Compared with Citrus reticulata ‘Unshiu’ which were cultivated in Taizhou City, Zhejiang Province and Citrus reticulata ‘Tangerina’ from Nanchang City, Jiangxi Province, Citrus reticulata ‘Chachi’ has longer length in the Helix I. In the part of Helix II, there are obvious differences between Citrus reticulata ‘Chachi’ and the two species. As the loops structure characteristic of the Helix III, Citrus reticulata ‘Chachi’ has less loops than Citrus reticulata ‘Dahongpao’ which harvested in Zigong City of Sichuan Province, despite their rest parts of the Helix structure were similar. In conclusion, ITS2 regions and its secondary structures could provide scientific evidence for the uniqueness of GCP (the pericarp of Citrus reticulata ‘Chachi’), indicating that the ITS2 DNA barcoding method shows good discriminating power for distinguishing GCP from other cultivars.
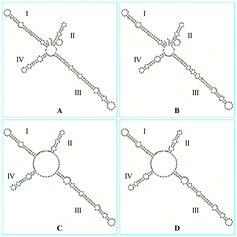 |
| Fig. 6 The secondary structures of: (A) Citrus reticulata ‘Chachi’; (B) Citrus reticulata ‘Dahongpao’; (C) Citrus reticulata ‘Unshiu’; (D) Citrus reticulata ‘Tangerina’. | |
3.3 The psbA-trnH sequences analysis
According the optimized DNA extraction protocol, the total DNA of all CRP samples were successfully extracted but samples with longer storage time (Gcp-17, Gcp-18, Gcp-19 and Gcp-20) has failed to amplify and sequence. The successfully sequenced psbA-trnH sequences were aligned and assembled by Codoncode Aligner. The psbA-trnH sequence properties of GCP (the pericarp of Citrus reticulata ‘Chachi’) contrasting with CP (the pericarp of Citrus reticulate Blanco, such as the cultivars of Citrus reticulata ‘Dahongpao’, Citrus reticulata ‘Unshiu’, and Citrus reticulata ‘Tangerina’) are shown in Fig. 7. All of the samples showed nearly same psbA-trnH sequence length and GC content, the length was approximately 517 bp and GC content was 32.11%. Although two deletion sites (44 and 487) and one deletion site (31) were observed for Citrus reticulata ‘Unshiu’ and ‘Tangerina’, respectively, the psbA-trnH sequence similarity between GCP and CP is close, indicating that the psbA-trnH sequence is not suitable for distinguishing GCP from CP.
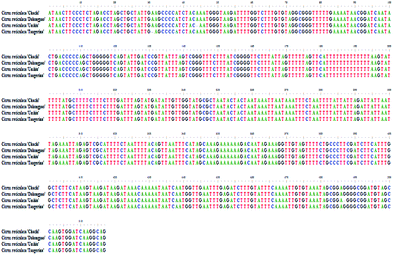 |
| Fig. 7 The psbA-trnH sequences of the four Species. | |
4. Discussion and conclusions
The ripe pericarp of Citri reticulate ‘Chachi’ originated from Xinhui county of Guangdong province in China, exclusively listed in Chinese Pharmacopeia (2015 edition) as name Guangchenpi (GCP), is regarded as the most genuine medicinal material. Thus a valid authentication method is crucial for discriminating GCP from other CP. Recent studies had been focused on chemical profiling of different varieties of CRP based on metabolomics.9,18,26 It's found that both volatile constituents and flavonoids compounds could be potential biomarkers for discriminating GCP from CP. However, these metabolomics methodologies were not validated by using QC samples. In present study, a validated metabolomics method using UPLC-QTOF MS was developed to identify potential markers that could discriminate GCP from CP. Our results suggested that polymethoxyflavones (PMF) such as hexamethoxyflavone (nobiletin and natsudaidain), pentamethoxyflavone (tangeretin and sinensetin), and tetramethoxyflavone are the most influential markers (Table 1). Some studies was also found that GCP has a distinct secondary metabolite composition. Duan27 found that the composition of PMFs in GCP was distinct and the content of PMFs in GCP was far higher than that in other Citrus cultivars. Furthermore, the GCP presents unique aroma, which is attributed to the distinct compositions of volatile components.28 These distinctions of secondary metabolite composition might be originated from genetic nuances.
External factors such as maturity stage, storage period, processing method and storage conditions could also affect secondary metabolites profile of individual CRP sample. Studies showed that the chemical constituents of GCP in different storage periods were changed remarkably.29,30 Contents of flavonoids in Citrus reticulata ‘Chachi’ were found varied from different habitats and collecting periods.31 The DNA sequences and some of intrinsic genetic information therein are believed to be more stable and would not influenced by these factors. Thus a DNA barcoding molecular identification method was incorporated with LC-MS based secondary metabolites profiling to explore the genetic characteristics and chemical diversity among 51 batches of commercial CRP samples. Results showed that the ITS2 secondary structure (Fig. 5) of Citri reticulate ‘Chachi’ (GCP) is different with other three cultivars (‘Unshiu’, ‘Dahongpao’ and ‘Tangerina’), indicating that ITS2 is a desirable DNA barcoding for discriminating (GCP) from other CP. Previous studies also proved that ITS region is suitable for authentication of Pericarpium Citri Reticulatae and Citri Unshius Pericarpium,32 and ITS2 region is suitable for authentication Citri reticulate ‘Chachi’, Citri reticulate ‘Dahongpao’ and Citri reticulate ‘Unshiu’.16
In the DNA barcoding experiment, it is easy to obtain high-quality DNA sequences from fresh CRP materials, however it's more complicated for long-term stored commercial CRP samples, especially when “CRP, the older, the better” was recognized in China. Thus the operation of DNA extracting by Plant Genomic DNA kit was improved in our experiment. The pretreatment of nuclear separation buffers was beneficial to improve the DNA extraction rate for commercial GCP specimens. Our study also studied the DNA barcording of the main adulterants of CRP, such as Citrus maxima (Burm.) Merr and Citrus limon (L.) Burm, and compared their ITS2 secondary structures with GCP. It was clearly showed that the differences between GCP and related species in the ITS2 secondary spiral structures (Fig. S4†).
In conclusion, the results presented in this study showed that the chemical analysis by UPLC-QTOF-MS/MS based metabolomics to discriminate GCP and CP was accurate. Through chemical pattern recognition, the 34 chemical markers were identified for discrimination of commercial GCP and other CP. The DNA barcoding method provides the variation sites and secondary structural diversity of ITS2 to identify GCP and CP in terms of genetic feature. The advantages of the two methods are exerted in this study. Combining the chemical composition analysis based metabolomics and the ITS2 secondary structure to establish a comprehensive and accurate method for distinguishing GCP from other CP.
Conflicts of interest
There are no conflicts to declare.
Acknowledgements
The authors would like to acknowledge the financial support from National Natural Science Foundation of China (81803482), Natural Science Foundation of Guangdong province (2017A030313709), Science and Technology Planning Project of Guangdong Province, China (2016A020226037, 2016A020226045), Technology Development Project of Guangdong Institute for Drug Control (2016KT1014) and 2018 Guangdong Forestry Department Lingnan Chinese Medicinal Materials Protection Project (2017KT1835), and Pearl River S&T Nova Program of Guangzhou (201806010048).
References
- X. Yu, S. Sun, Y. Guo, Y. Liu, D. Yang, G. Li and S. Lü, Citri Reticulatae Pericarpium (Chenpi): botany, ethnopharmacology, phytochemistry, and pharmacology of a frequently used traditional Chinese medicine, J. Ethnopharmacol., 2018, 220, 265–282, DOI:10.1016/j.jep.2018.03.031.
- J. Xu, X. Wu, M. Li, G. Li, Y. Yang, H. Luo, W. Huang, H. Y. Chung, W. Ye, G. Wang and Y. Li, Antiviral Activity of Polymethoxylated Flavones from “Guangchenpi”, the Edible and Medicinal Pericarps of Citrus reticulata ‘Chachi’, J. Agric. Food Chem., 2014, 62, 2182–2189, DOI:10.1021/jf404310y.
- O. Benavente-García and J. Castillo, Update on Uses and Properties of Citrus Flavonoids: New Findings in Anticancer, Cardiovascular, and Anti-inflammatory Activity, J. Agric. Food Chem., 2008, 56, 6185–6205, DOI:10.1021/jf8006568.
- S. Ho and C. Lin, Investigation of Heat Treating Conditions for Enhancing the Anti-Inflammatory Activity of Citrus Fruit (Citrus reticulata) Peels, J. Agric. Food Chem., 2008, 56, 7976–7982, DOI:10.1021/jf801434c.
- M. J. Kim, H. J. Park, M. S. Hong, H. J. Park, M. S. Kim, K. H. Leem, J. B. Kim, Y. J. Kim and H. K. Kim, Citrus Reticulata blanco induces apoptosis in human gastric cancer cells SNU-668, Nutr. Cancer, 2005, 51, 78–82, DOI:10.1207/s15327914nc5101_11.
- E. Tripoli, M. Giammanco, G. Tabacchi, D. Di Majo, S. Giammanco and M. La Guardia, The phenolic compounds of olive oil: structure, biological activity and beneficial effects on human health, Nutr. Res. Rev., 2005, 18, 98, DOI:10.1079/NRR200495.
- Chinese Pharmacopoeia Commission, Pharmacopoeia of People's Republic of China, Version 2015, China Medical Science Publisher, Beijing, 2015, vol. 1, p. 191 Search PubMed.
- Y. Sun, J. Wang, S. Gu, Z. Liu, Y. Zhang and X. Zhang, Simultaneous Determination of Flavonoids in Different Parts of Citrus reticulata ‘Chachi’ Fruit by High Performance Liquid Chromatography—Photodiode Array Detection, Molecules, 2010, 15, 5378–5388, DOI:10.3390/molecules15085378.
- L. Duan, L. Guo, L. Dou, C. Zhou, F. Xu, G. Zheng, P. Li and E. Liu, Discrimination of Citrus reticulata Blanco and Citrus reticulata ‘Chachi’ by gas chromatograph-mass spectrometry based metabolomics approach, Food Chem., 2016, 212, 123–127, DOI:10.1016/j.foodchem.2016.05.141.
- L. Yi, N. Dong, S. Liu, Z. Yi and Y. Zhang, Chemical features of Pericarpium Citri Reticulatae and Pericarpium Citri Reticulatae Viride revealed by GC–MS metabolomics analysis, Food Chem., 2015, 186, 192–199, DOI:10.1016/j.foodchem.2014.07.067.
- M. Agarwal, N. Shrivastava and H. Padh, Advances in molecular marker techniques and their applications in plant sciences, Plant Cell Rep., 2008, 27, 617–631, DOI:10.1007/s00299-008-0507-z.
- N. Techen, I. Parveen, Z. Pan and I. A. Khan, DNA barcoding of medicinal plant material for identification, Curr. Opin. Biotechnol., 2014, 25, 103–110, DOI:10.1016/j.copbio.2013.09.010.
- Y. L. Sun, H. M. Kang, S. H. Han, Y. C. Park and S. K. Hong, Taxonomy and phylogeny of the genus Citrus based on the nuclear ribosomal DNA its region sequence, Pak. J. Bot., 2015, 47(1), 95–101 CAS.
- S. Chen, H. Yao, J. Han, C. Liu, J. Song, L. Shi, Y. Zhu, X. Ma, T. Gao, X. Pang, K. Luo, Y. Li, X. Li, X. Jia, Y. Lin and C. Leon, Validation of the ITS2 region as a novel DNA barcode for identifying medicinal plant
species, PLoS One, 2010, 5, e8613, DOI:10.1371/journal.pone.0008613.
- A. Keller, T. Schleicher, J. Schultz, T. Müller, T. Dandekar and M. Wolf, 5.8S-28S rRNA interaction and HMM-based ITS2 annotation, Gene, 2009, 430, 50–57, DOI:10.1016/j.gene.2008.10.012.
- X. Yu, Y. Zhang, D. Wang, L. Jiang and X. Xu, Identification of Three Kinds of Citri Reticulatae Pericarpium Based on Deoxyribonucleic Acid Barcoding and High-performance Liquid Chromatography-diode Array Detection-electrospray Ionization/Mass Spectrometry/Mass Spectrometry Combined with Chemometric Analysis, Pharmacogn. Mag., 2018, 14, 64–69, DOI:10.4103/pm.pm_581_16.
- Q. Xue-Mei, D. Yun-Tao, Z. Li-Zeng, G. Xiao-Qing and S. Hong-Xia, Discrimination of three medicinal materials from the Citrus genus by HPLC fingerprint coupled with two complementary software, Phytochem. Anal., 2009, 4, 307–313, DOI:10.1002/pca.1128.
- Z. Jandrić, M. Islam, D. K. Singh and A. Cannavan, Authentication of Indian Citrus fruit/fruit juices by untargeted and targeted metabolomics, Food Control, 2017, 72, 181–188, DOI:10.1016/j.foodcont.2015.10.044.
- C. Zhang, M. Niu, R. Li, W. Feng, X. Ma, Q. Dong, Z. Ma, G. Li, Y. Meng, Y. Wang, P. Yin, L. He, Y. Li, P. Tan, Y. Zhao, J. Wang, X. Dong and X. Xiao, Untargeted Metabolomics Reveals Dose-Response Characteristics for Effect of Rhubarb in a Rat Model of Cholestasis, Front. Pharmacol., 2016, 7, 85, DOI:10.3389/fphar.2016.00085.
- X. Zhang, J. Li, B. Xie, B. Wu, S. Lei, Y. Yao, M. He, H. Ouyang, Y. Feng, W. Xu and S. Yang, Comparative Metabolomics Analysis of Cervicitis in Human Patients and a Phenol Mucilage-Induced Rat Model Using Liquid Chromatography Tandem Mass Spectrometry, Front. Pharmacol., 2018, 9, 282, DOI:10.3389/fphar.2018.00282.
- K. Luo, S. Chen, K. Chen, J. Song, H. Yao, X. Ma, Y. Zhu, X. Pang, H. Yu, X. Li and Z. Liu, Assessment of candidate plant DNA barcodes using the Rutaceae family, Sci. China Life Sci., 2010, 53, 701–708, DOI:10.1007/s11427-010-4009-1.
- G. Cao, Q. Fu, C. Zhang, H. Wang and S. Chen, Rapid characterization of 96 chemical constituents in Citri Reticulatae Folium (leaves of ‘Fuju’) using HPLC-DAD-ESI-MSn, J. Chin. Pharm. Sci., 2016, 25, 91–110, DOI:10.5246/jcps.2016.02.010.
- L. Guo, R. Li, K. Liu, J. Yang, H. Li, S. Li, J. Liu, L. Liua and G. Xin, Structural characterization and discrimination of Chinese medicinal materials with multiple botanical origins based on metabolite profiling and chemometrics analysis: Clematidis radix et Rhizoma as a case study, J. Chromatogr. A, 2015, 1425, 129–140, DOI:10.1016/j.chroma.2015.11.013.
- L. Duan, L. Guo, K. Liu, E. H. Liu and P. Li, Characterization and classification of seven Citrus herbs by liquid chromatography–quadrupole time-of-flight mass spectrometry and genetic algorithm optimized support vector machines, J. Chromatogr. A, 2014, 1339, 118–127, DOI:10.1016/j.chroma.2014.02.091.
- X. Ye, D. Cao, X. Zhao, F. Song, Q. Huang, G. Fan and F. Wu, Chemical fingerprint and metabolic profile analysis of Citrus reticulate ‘Chachi’ decoction by HPLC-PDA-IT-MSn and HPLC-Quadrupole-Orbitrap-MS method, J. Chromatogr. B: Anal. Technol. Biomed. Life Sci., 2014, 970, 108–120, DOI:10.1016/j.jchromb.2014.06.035.
- Y. Zheng, X. Zeng, W. Peng, Z. Wu and W. Su, Characterisation and classification of Citri Reticulatae
Pericarpium varieties based on UHPLC-Q-TOF-MS/MS combined with multivariate statistical analyses, Phytochem. Anal., 2019, 30, 278–291, DOI:10.1002/pca.2812.
- L. Duan, L. Dou, K. Yu, L. Guo, C. Bai-Zhong, P. Li and E. Liu, Polymethoxyflavones in peel of, Citrus reticulata, ‘Chachi’ and their biological activities, Food Chem., 2017, 234, 254–261, DOI:10.1016/j.foodchem.2017.05.018.
- M. Luo, H. Luo, P. Hu, Y. Yang, B. Wu and G. Zheng, Evaluation of chemical components in Citri Reticulatae Pericarpium of different cultivars collected from different regions by GC-MS and HPLC, Nutr. Food Sci., 2018, 6, 400–416, DOI:10.1002/fsn3.569.
- M. Fu, Y. Xu, Y. Chen, J. Wu, Y. Yu, B. Zou, K. An and G. Xiao, Evaluation of bioactive flavonoids and antioxidant activity in Pericarpium Citri Reticulatae (Citrus reticulata ‘Chachi’) during storage, Food Chem., 2017, 230, 649–656, DOI:10.1016/j.foodchem.2017.03.098.
- Y. Luo, W. Zeng, K. Huang, W. Chen, X. Yu and X. Ke, Discrimination of Citrus reticulata Blanco and Citrus reticulata ‘Chachi’ as well as the Citrus reticulata ‘Chachi’ within different storage years using ultra high performance liquid chromatography quadrupole/time-of-flight mass spectrometry based metabolomics approach, J. Pharm. Biomed. Anal., 2019, 171, 218–231, DOI:10.1016/j.jpba.2019.03.056.
- L. Lin, J. Lin and G. Zheng, Study on the contents of flavonoids in Citrus reticulata ‘Chachi’ from various habitats and different collecting periods, Zhongyaocai, 2010, 33, 173–176 CAS.
- H. Wang, M. Kim, Y. Kim, H. Lee, H. Jin, J. Chen and D. Yang, Molecular authentication of the Oriental medicines Pericarpium Citri Reticulatae and Citri Unshius Pericarpium using SNP markers, Gene, 2012, 494, 92–95, DOI:10.1016/j.gene.2011.11.026.
Footnotes |
† Electronic supplementary information (ESI) available. See DOI: 10.1039/c9ra03740c |
‡ These authors have contributed equally to this work. |
|
This journal is © The Royal Society of Chemistry 2019 |
Click here to see how this site uses Cookies. View our privacy policy here.