DOI:
10.1039/C8MO00239H
(Research Article)
Mol. Omics, 2019,
15, 67-76
Degradation of the extracellular matrix is part of the pathology of ulcerative colitis†
Received
16th October 2018
, Accepted 2nd January 2019
First published on 31st January 2019
Abstract
The scientific value of re-analyzing existing datasets is often proportional to the complexity of the data. Proteomics data are inherently complex and can be analyzed at many levels, including proteins, peptides, and post-translational modifications to verify and/or develop new hypotheses. In this paper, we present our re-analysis of a previously published study comparing colon biopsy samples from ulcerative colitis (UC) patients to non-affected controls. We used a different statistical approach, employing a linear mixed-effects regression model and analyzed the data both on the protein and peptide level. In addition to confirming and reinforcing the original finding of upregulation of neutrophil extracellular traps (NETs), we report novel findings, including that Extracellular Matrix (ECM) degradation and neutrophil maturation are involved in the pathology of UC. The pharmaceutically most relevant differential protein expressions were confirmed using immunohistochemistry as an orthogonal method. As part of this study, we also compared proteomics data to previously published mRNA expression data. These comparisons indicated compensatory regulation at transcription levels of the ECM proteins we identified and open possible new avenues for drug discovery.
Introduction
Ulcerative colitis (UC) is a chronic relapsing inflammatory disorder of the gastrointestinal tract.1–3 The prevalence of UC is increasing worldwide. In Europe, for example, the incidence has reached almost 24 cases per 100
000 person-years.4,5 The disease manifests itself at all ages and it severely affects the quality of life of the patients and their families. In addition, UC has a large impact on society, due to interrupted education, sick leave, and the significant strain it places on the healthcare system.
The underlying cause of UC is not completely understood, but both genetics and the environment have been found to be factors in the disease etiology. More than 230 genetic loci have been identified as being associated with the inflammatory bowel diseases (IBD) which, in addition to UC, include Crohn's disease (CD).6 Identified genes have been found to be involved in the epithelial barrier function and both the innate and adaptive immune response.7 Additionally, a westernized lifestyle and environmental impact is associated with increased risk of IBD, which may be linked to changes in the gut microbiome.8 Accordingly, the prevalence in the West is increasing.9 Cigarette smoking also affects the risk of IBD and gene-smoking interactions have been identified.10
An early diagnosis and individualized management of the disease are critical in avoiding irreversible damage to the intestinal tract. However, finding good treatments remains a challenge due to the heterogeneous nature of the disease.11–16 Hence, despite the introduction of biological treatments for UC, colectomy still is required in 25–30% of the patients at some point during their lifetime due to the development of colitis refractory to other treatments.17,18 The intestinal mucosa is critical to inflammatory processes and regulates immune responses at the interface between the host and the environment.19–22 It has been previously hypothesized that initial damage to the intestinal mucosal barrier results in a misdirected immune response which ultimately leads to the manifestation of IBD.7 An in-depth characterization of the intestinal mucosa via molecular profiling technologies would therefore help to understand the molecular pathophysiology.23 Using a comparative analysis of the proteome in IBD and healthy colon mucosa, dysregulated molecular analytes and pathways, infiltrating myeloid or lymphocytic markers, chemokines, and inflammatory molecules could be identified. Dysregulated molecular analytes and pathways could be biomarker candidates (prognostic or predictive), diagnostics or even potential targets for drug development.
To investigate this hypothesis, in a previous study the proteomes of intestinal biopsies from UC patients in remission and gastrointestinally healthy controls was mapped.23 In this study a biobank with colon biopsies from ten UC-patients and ten gastrointestinally healthy controls was established. The UC patients were in remission and the biopsies were taken with sigmoidoscopy from macroscopically healthy-looking (non-inflamed) tissue. The samples were analyzed by high-throughput bottom-up proteomics using a label-free quantitation strategy. This study lead to the identification and quantification of 5711 different proteins and found an increased abundance of neutrophils and neutrophil extracellular traps (NETs) in the biopsy samples from UC patients.23
Proteomics data are inherently complex and can be analyzed at many levels, and statistical analysis of proteomics datasets can be confounded by a number of factors. We present here our re-examination of our proteomics dataset comparing UC samples to gastrointestinally healthy controls, using a different statistical approach compared to the original study. Employing a linear mixed-effects regression model, rather than t-tests, and analyzing the data on the protein and peptide level, we obtained a higher statistical sensitivity. In this study we confirmed the findings of the initial publication. In addition, the expanded findings allowed us to hypothesize that the degradation of the colon mucosa extracellular matrix (ECM) may play a critical role in the pathology of UC, in addition to neutrophil extracellular traps (NETs).
Materials and methods
Proteomics data acquisition
For the original study,23 colon biopsies were taken from ten UC patients and 10 gastrointestinally healthy controls with sigmoidoscopy from macroscopically healthy-looking (non-inflamed) tissue at the Regional Hospital Silkeborg in Denmark. The samples were immediately snap-frozen in liquid nitrogen and stored at −80 °C until time of processing within one year. The samples were prepared for bottom-up proteomics analysis using a label-free quantitation strategy. Briefly explained, the biopsies were homogenized using steel beads and digested using a filter-aided sample preparation protocol with the protease trypsin. The samples were analyzed by high-throughput bottom-up proteomics on a UltiMate 3000 UPLC system (Thermo Scientific, Waltham, MA) coupled online to a Q Exactive Plus mass spectrometer (Thermo Scientific), operated in a data-dependent TOP12 mode. The proteomics raw data were deposited by Bennike et al.24 to the ProteomeXchange Consortium via the PRIDE ProteomXchange repository with the dataset identifier PXD001608. The study material was composed of colon mucosa biopsies from ten UC patients and ten gastrointestinally healthy controls. These data were retrieved from the PRIDE EBI archive using wget.
Proteomics search
The sequence database for proteomics searches was assembled from a combination of Ensembl, Refseq and Uniprot databases. In addition, Refseq annotation was used to add fragments unique to mature proteins. We used MaxQuant 1.5.3.825 to accomplish all protein identification searches. MaxQuant configurations associated with each search are provided in the (available in the downloadable Supplementary Materials file, ESI†) section.
The proteinGroups.txt file produced by the MaxQuant search was used for quality control (QC) of the dataset. The QC report was generated using Rstudio26 running R version 3.3.1.27 From these initial plots of raw Intensity and LFQ (DP = 2), none of the samples was deemed to be of significant concern and thus all samples were included in the subsequent analysis.
The results presented throughout this paper are based on our analysis of protein groups as defined by MaxQuant; for simplicity we are going to refer to those as proteins.
Statistical models
Filtering.
We removed all proteins where too many data points were missing, using both sample-frequency (66% per group) and donor-frequency (70%) thresholds. Since we had 30 samples (3 replicates in 10 donors) per group, a protein that had no readout in 10 or more samples in each group would be considered non-quantifiable and would be eliminated from further analysis. Similarly, proteins considered for further analysis had to be present in at least 7 donors in either group.
Imputation.
We imputed missing values for quantifiable proteins using the minimum LFQ value in the sample where the missing data point was present. This represents a conservative approach in most cases, as such imputation of values missing at random would likely increase the variability within a group and therefore increase the p-value.
Differential expression analysis.
We fit a linear mixed-effects regression model of Intensity, with a fixed effect of DiseaseState (UC versus controls) and a random Donor effect, using function lme from the R package “nlme”.28 We controlled for false discovery by applying the Benjamini–Hochberg correction method. Proteins were considered to be significantly differentially regulated at 5% FDR and ±0.5
log2-fold-change. Peptides were considered to be significantly differentially regulated at 5% FDR and ±1
log2-fold-change.
Differential modification analysis.
To test for the presence of global shifts in modifications (hydroxyproline oxidation, ubiquitination), we averaged the raw intensity from all peptides carrying the specific modification for each donor within the control and UC group. We then applied a two tailed t-test to those averaged values for each group.
Microarray data and correlation analysis.
Microarray data were retrieved from GEO. Three datasets were reanalyzed: GSE3871329 (referred to as Plannell), GSE1061630 (referred to as Kugathasan), and GSE945231 (referred to as Olsen). Each dataset was RMA normalized. Only probesets with an expression level more than log2 RMA of 3.5 in at least 10 samples were considered. Fold change values were determined for the control subjects vs. the UC patients (Table 1).
Table 1 Number of participants in the three microarray studies
Study |
Controls |
Ulcerative colitis |
Olsen31 |
5 |
21 |
Planell29 |
13 |
30 |
Kugathasan30 |
16 |
10 |
Fold change values for probesets were collapsed to a gene level by taking the median. Pearson pairwise correlations were then calculated for all microarray sets and the proteomics data.
The list of proteins with significant disagreement between RNA expression and protein levels was compiled by thresholding for mass spec fold change and microarray median fold change and sorting by the mass spec fold change. The directionality and downregulation of proteins was stored as a Boolean vector.
Pathway analysis.
Differentially regulated peptides were converted to protein identifiers using the peptides.txt file. Protein identifiers were converted to EntrezGene identifiers using a combination of conversion techniques according to the source of the originating protein ID (Refseq, Ensembl and Uniprot).
We split the lists into up- and down-regulated sublists and examined each list separately with WebGestalt and GSEA. Webgestalt32 was used to explore GO enrichment and GSEA was used to explore other ontologies and gene lists, such as MSIGDB, Reactome (http://www.reactome.org) and Pubmed (http://www.ncbi.nlm.nih.gov/pubmed/).
Both tools allowed lists of genes to be uploaded and to serve as background models and we used all identified proteins to define the background reference.
Reproducibility
Most results from this analysis (hits lists, graphs, etc.) can be reproduced on a Linux system with R 3.3.1, perl and several R packages (listed available in the downloadable Supplementary Materials file, ESI†). Once all input datasets are downloaded along with the scripts, executing reproduce.sh would generate identical results to those we communicate in this paper. All datasets and scripts necessary to reproduce these findings are downloadable from http://pubd.web.bms.com/.
Immunohistochemistry
Formalin-fixated colon biopsies24 were paraffin embedded, cut in 10 μm sections, mounted on Superfrost Plus slides (Thermo Scientific) and deparaffinized. Sections were blocked with 0.1% bovine serum albumin (BSA) in phosphate-buffered saline (PBS) for 1 hour. Thereafter, the sections were incubated for one hour with the primary antibody in 0.1% BSA in PBS using either 2 μg ml−1 rabbit anti-Protein S100-A9 (Abcam, Cambridge, UK), 2 μg ml−1 mouse IgG1 anti-Protein S100-A12 (Abcam), 2 μg ml−1 rabbit anti-myeloperoxidase (ab9535, Abcam), 2 μg ml−1 isotype control mouse monoclonal IgG1, or 2 μg ml−1 rabbit IgG. Sections were washed 3 times with PBS. The samples were then incubated for 1 hour in PBS with the secondary antibody using either TRITC conjugated goat anti-rabbit IgG or TRITC conjugated goat anti-mouse IgG (Jackson ImmunoResearch, Bar Harbor, ME). The samples were then diluted 1
:
200 in PBS with 0.1% BSA. To stain for DNA, the sections were washed in PBS and incubated for 30 minutes with 1 μM ToPro-3 (Thermo Scientific) in PBS. The slides were analyzed with an SP5-confocal microscope (Leica, Wetzlar, Germany) using an HC PL APO 63×/1.40 Oil objective (Leica) and the data was analyzed in ImageJ.33
To provide a semi-quantitative addition to the proteomics data, the stain intensity surrounding the 10 cells with the highest staining intensity was averaged for each confocal microscopy image using ImageJ 1.48v. The intensities between the samples was compared by two sample t-tests in R.
Results
Quality control
We have established an R-based pipeline to comprehensively evaluate the quality of proteomics data (manuscript in preparation). A full quality report is available in the (available in the downloadable Supplementary Materials file, ESI†).
We used the quantro R package34 to evaluate global effects and observed no statistically significant change in protein intensities between the UC and control groups (p = 0.11, 10
000 simulations). We therefore applied median centering as a global normalization method.
Protein level analysis
This study aimed to identify proteins differing in abundance on average between the UC and control groups. For this purpose we performed linear regression analysis based on the protein groups generated by MaxQuant.
We identified a similar number of protein groups – 6399 – compared to the original study where 6268 were identified. After rigorous filtering, 5093 proteins were considered to be quantifiable, versus 5711 in the original publication. However, our statistical model allowed us to identify substantially more differentially regulated proteins: 251 versus 49 in the previous study. This difference reflects two methodological differences: different filtering and imputation strategies, and the linear mixed effects model we used that allowed us to take advantage of the technical replicates, which had been averaged in the original study. Including a random donor effect to allow for intra-donor correlations between technical replicates allowed the assessment of technical variability and increased statistical power of hypothesis tests.
As with the original study we were able to establish statistically significant overlap with NETs (hypergeometric test, p < 0.001), most significantly with NET granules.35 We were able to confirm the presence of a neutrophil immune cell signature also based on MSIGDB.36
Of the differentially regulated proteins, 90 had increased and 161 had decreased abundance in the UC samples. However, 9 of the top 10 proteins with the largest absolute expression difference (Table 2) were upregulated, signifying the larger effects observed in diseased tissue. The only downregulated protein in the top 10 is elastin, an indication of changes in the extracellular matrix. This observation is in line with previous research implicating bacterial or host proteases in the degradation of the ECM in IBD.37,38
Table 2 The top 10 differentially regulated proteins when comparing the UC and control groups. The list is filtered by FDR adjusted p-value (p < 0.05) and sorted by effect size (log2 ratio). Complete table of the differentially regulated proteins is available as Supplementary File 1 and the non-filtered data is available in Supplementary File 2 (ESI)
Protein acc. |
p-value |
FDR |
Entrez gene |
log2 ratio |
Description |
Gene |
NETs protein |
NP_002334 |
8.57 × 10−12 |
4.37 × 10−8 |
4057 |
7.45 |
Lactotransferrin |
LTF |
Yes |
XP_011523124 |
2.02 × 10−13 |
1.03 × 10−9 |
4353 |
6.76 |
Myeloperoxidase |
MPO |
Yes |
NP_004985 |
1.87 × 10−12 |
9.52 × 10−9 |
4318 |
6.06 |
Matrix metallopeptidase 9 |
MMP9 |
Yes |
XP_011545190 |
2.93 × 10−7 |
1.49 × 10−3 |
1991 |
5.02 |
Elastase, neutrophil expressed |
ELA2 |
Yes |
NP_001691 |
1.28 × 10−8 |
6.50 × 10−5 |
566 |
4.92 |
Azurocidin 1 |
AZU1 |
Yes |
XP_011514177 |
5.16 × 10−13 |
2.63 × 10−9 |
2006 |
−4.88 |
Elastin |
ELN |
No |
NP_002956 |
2.13 × 10−9 |
1.09 × 10−5 |
6280 |
4.67 |
S100 calcium binding protein A9 |
S100A9 |
Yes |
NP_000623 |
2.02 × 10−10 |
1.03 × 10−6 |
3684 |
4.53 |
Integrin subunit alpha M |
ITGAM |
No |
NP_002768 |
6.37 × 10−7 |
3.24 × 10−3 |
5657 |
4.49 |
Proteinase 3 |
PRTN3 |
Yes |
XP_005260579 |
8.75 × 10−10 |
4.46 × 10−6 |
671 |
4.45 |
Bactericidal/permeability-increasing protein |
BPI |
No |
We compared our results to previous research of differential gene expression in transcriptomics datasets, comparing colon biopsies from UC-patients to controls. For that purpose we selected 3 publicly available transcriptional profiling datasets.29–31 We extracted the lists of differentially regulated genes from the microarray data and then calculated the log2 ratio for all sets, including the present proteome dataset. All further comparisons were based on this metric. Merging between datasets was done using a translation table available in the (available in the downloadable Supplementary Materials file, ESI†). We were able to match 219 of the 251 hits in all datasets. Overall the correlation between the transcriptional profiling studies is high. In contrast, the correlation to our proteomics dataset was moderate with R ≤ 0.38 (Fig. 1 and Fig. S1, ESI†). Unlike mRNA-protein level correlation, for which we anticipated low correlation based on established research,39 we expected differentially expressed proteins to exhibit greater correlation when fold changes were used for comparison.40
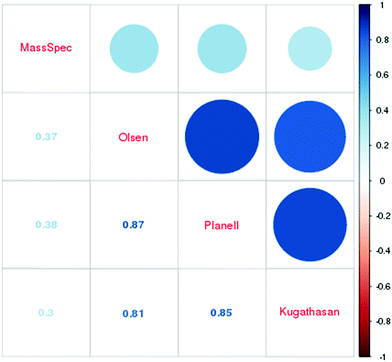 |
| Fig. 1 The Pearson correlation matrix based log2 ratios in microarray and protein expression data. | |
Surprisingly, genes that were highly expressed in neutrophils and are considered granulocyte markers showed different direction changes in the proteomics data and even between different transcriptional studies. Myeloperoxidase (MPO), for example, is well studied and known to be highly induced in active UC.41 The reverse observation may be the result of a suboptimal design of microarray probesets. The lack of high correlation may be due to post-translational events, differences in general technologies, or sample selection. Another possible explanation would be that transcription is halted when secretory granules are full, resulting in high protein levels, but low transcript abundance.
There also were significant differences between the transcriptional and protein fold changes when examining the downregulated proteins (Supplementary File 1, ESI†). A substantial number49 of the 149 downregulated proteins were either upregulated (log2 ratio > 0.5, n = 16) or unchanged with log2 ratio between 0 and 0.5 (n = 33). Most of these proteins (Table 3) are part of the ECM.
Table 3 Top 10 of the proteins where significant disagreement between protein log2 ratios and transcriptional profiling ratios were observed, ranked by mass spec log2 fold change
Protein |
MassSpec |
Olsen |
Planell |
Kugathasan |
Mean of all arrays |
Symbol |
In bold-bona fide components of the extracellular matrix (ECM). |
NP_005469 |
−3.25 |
0.55 |
0.19 |
1.06 |
0.60 |
INSL5 |
XP_005252935
|
−2.42
|
0.26
|
0.02
|
1.53
|
0.60
|
OLFML1
|
XP_011508876
|
−2.37
|
1.57
|
1.94
|
2.41
|
1.97
|
COL6A3
|
NP_001034438
|
−2.15
|
−0.24
|
0.39
|
1.92
|
0.69
|
EFEMP1
|
NP_060150
|
−1.71
|
0.26
|
0.27
|
1.29
|
0.60
|
ASPN
|
NP_001129408
|
−1.41
|
0.92
|
0.64
|
1.08
|
0.88
|
POSTN
|
XP_011519350
|
−1.34
|
1.41
|
1.67
|
1.86
|
1.65
|
COL4A1
|
NP_002014
|
−1.13
|
0.36
|
0.44
|
1.48
|
0.76
|
FMOD
|
NP_001275908 |
−1.09 |
1.70 |
0.48 |
1.69 |
1.29 |
LIPA |
NP_000129
|
−1.02
|
0.95
|
0.60
|
2.01
|
1.19
|
FBN1
|
NP_000080
|
−1.00
|
1.44
|
1.43
|
1.86
|
1.58
|
COL1A2
|
NP_001837
|
−0.95
|
0.94
|
0.64
|
1.26
|
0.94
|
COL4A2
|
NP_004376
|
−0.84
|
1.20
|
0.77
|
2.18
|
1.38
|
VCAN
|
XP_006719333
|
−0.66
|
1.14
|
0.71
|
2.23
|
1.36
|
DCN
|
NP_001702
|
−0.64
|
0.75
|
0.72
|
0.52
|
0.66
|
BGN
|
XP_011527754
|
−0.61
|
0.38
|
0.77
|
0.58
|
0.58
|
COL6A2
|
Anecdotal reports of similar phenomena have been recorded in the past. For example, collagen protein is known to be lost due to degradation in models of IBD, and PGP fragments are subsequently increased.42 This degradation of collagen could trigger compensation at a transcriptional regulation level and would explain the upregulation seen in the microarray studies29–31 we examined. In a recently published RNA-Seq dataset,43 3 of the genes we found to be upregulated as transcripts, but at decreased protein levels were confirmed to be increased as transcripts as well (Table S2, ESI†).
Chromogranin A
In addition to the protein level analysis, we also looked at differential expression at the peptide level (available in the downloadable Supplementary Materials file, ESI†). This analysis may occasionally highlight differences in protein processing and secretion. We decided to look specifically at chromogranin A, as the log2 ratio for the identified peptides originating from this protein showed substantial dispersion. The variability of most peptides is higher in the UC group because of more imputed values.
Chromogranin A (CHGA) is a protein that is post-translationally processed to multiple hormones and functional peptides.44,45 While chromogranin A was one of the differentially regulated proteins, it was not a top hit. CHGA ranked in the top 28.17% of all hits (71/252) in terms of fold change (log2 FC = −1.77, FDR = 0.0015). At the peptide level, differential regulation was more pronounced with fold changes ranging from −3.7 to −5.7, and the top peptide ranked at the top 6.54% of all hits (42/642). The reason for this discrepancy is that some of the peptides were significantly downregulated in UC (Fig. 2), while others were only marginally higher in controls. For all peptides, the characteristics and fold change differences between tissues from healthy controls and UC patients are given in the (Supplementary File 3, ESI†).
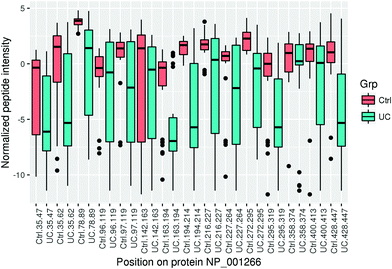 |
| Fig. 2 Mean normalized intensity of chromogranin A peptides in ulcerative colitis (UC) and controls (ctrl) according to the position of the individual peptides in the full protein sequence. | |
Identification of CHGA sub-regions as some of the most significant peptide hits (available in the downloadable Supplementary Materials file, ESI†) allowed us to describe EA-92, chomofungin, serpinin, and pancreastatin as potential biomarkers and perhaps targets in the context of UC. In addition, we can now speculate on the involvement of EA-92 in inflammation.
Significant changes occurred in four peptide regions. These regions overlap with the biologically active peptides serpinin, vasostatin and chromofungin that are known to have anti-microbial activity.46,47
As we did not intend to explore bioactive peptide expression, it is hard to define exactly which functional regions are differentially regulated. We can speculate, for example, that the CHGA downregulated peptide probably originates from chromofungin, since changes in other vasostatin I and vasostatin II peptides were not statistically significant. We also observed significant changes in EA-92, but some peptides originating from the same region were not consistent with this change. It is possible that EA-92 is further processed into a shorter mature product.
Decreased hydroxyproline in ulcerative colitis
Collagen is a central ECM protein and degradation of it has been associated with IBD.42 Hydroxyproline modifications correlate well with collagen content, collagen protein abundances, and the degree of fibrosis48 which can be found in >30% of CD patients and about 5% of UC patients.49,50 We therefore decided to include a quantitative analysis of the hydroxyproline PTM content. In addition, ubiquitination has been implicated in IBD and was for that reason also included in the analysis.51
We did not observe differential regulation of any of the individual amino acid sites, either for hydroxyproline or ubiquitinated PTMs. Additionally, we did not observe a statistically significant difference between total or peptide specific ubiquitination (p = 0.146). However, the overall abundance of peptides with a hydroxyproline modification was significantly lower in the UC colon tissue compared to control (Fig. 3), which is consistent with the reduced abundance of collagen proteins determined by proteomics and previously published research of collagen degradation.52
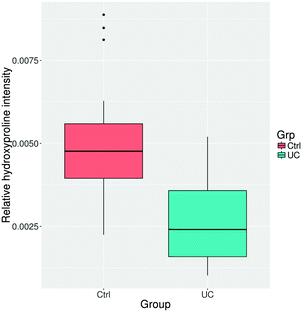 |
| Fig. 3 Overall change in hydroxyproline modified peptide abundance in ulcerative colitis (UC) compared to controls (Ctrl), demonstrates a reduced abundance in UC (p-value = 1.12 × 10−7, Welch two sample t-test). p-Value = 1.12 × 10−7, Welch two sample t-test. | |
Pathway analysis
We explored the presence of over-represented categories from several different ontologies using lists of upregulated and downregulated features at the peptide and protein levels. When using the GO Biological process ontology and comparing to our protein-level list (available in the downloadable Supplementary Materials file, ESI†), most categories were related to the immune system. The most significant category we observed was “Response to bacteria”. We also observed the enrichment of leukocyte migration, which is a hallmark feature of IBD.53 At the same time, downregulated proteins significantly overlapped with extracellular structure organization as well as mitochondrial respiratory complex assembly and glycosylation (available in the downloadable Supplementary Materials file, ESI†). While mitochondrial changes have been previously implicated in the pathogenesis of IBD,54 the association of ECM categories with downregulated proteins was largely unexpected. This observation was consistent with the decreased hydroxyproline levels and the data we obtained by comparing transcriptional profiling data with the list of differentially regulated proteins.
Next, we explored additional ontologies and gene lists, including MSigDB and Pubmed (available in the downloadable Supplementary Materials file, ESI†). Most lists we identified as significantly overlapping with our list of upregulated proteins were related to immune function, and further reinforce the neutrophil association. One category also of interest is Reactome's “Degradation of extracellular matrix”, which is consistent with decreased hydroxyproline levels and collagen protein, supporting our hypothesis. In addition, it appears that lists associated with cell line proliferation (stem cells, cancer) overlap significantly with our downregulated list of proteins.
In the GO Component ontology, only one category was enriched in the list of upregulated peptides: “Blood microparticles” (Fig. S6, ESI†). Blood microparticles (MPs) have been associated previously with inflammation and infection, including Crohn's disease.55 Secreted MPs or secreted Microvesicles (sEV) are a diverse range of lipid vesicles containing a cargo of protein and RNA/DNA originating from a range of cell types including neutrofils.56
IHC confirmation
Colon biopsy sections from 4 UC patients and 2 healthy controls were stained for the proteins S100-A9 and S100-A12, or for myeloperoxidase, all of which are present in granulocytes. In Fig. 4, results from one patient with ulcerative colitis and one healthy control are shown. No reaction with antibodies to S100-A9 and S100-A12 was observed in sections of the healthy control (Fig. 4, A and D) or in immunoglobulin controls (Fig. 4F and I), showing that granulocytes are not present in healthy intestinal mucosa (mucous epithelium and lamina propria). A strong staining was observed for S100-A9 in the UC patients, and, in addition, more cells were observed in lamina propria than in controls (Fig. 4B). By enlarging the image, it can be seen that cells stained for S100-A 9 are polymorphonuclear (Segmented nuclei), in agreement with granulocytes, and S100-A9 is located in the cytoplasm of the cells (Fig. 4C). It can also be seen that the S100-A9 contained in polymorphonuclear cells are located between the mucosal epithelial cells (Fig. 4B and C). S100-A12 are also present in the cytoplasm of polymorphonuclear cells in the UC patient (Fig. 4). Myeloperoxidase shows a similar staining pattern as S100-A9 and S100-A12, in agreement with its localization in granulocytes. An analysis of the staining intensity confirmed the statistically significant (t-test p-value <0.001) increase of S100-A9, S100-A12, and MPO in the UC colonic biopsies compared to controls (Fig. S4, ESI†). The main function of MPO is remodeling of the ECM during degradation, hence the increased abundance of MPO as determined by proteomics and validated by immunofluorescence underlines the degradation of the colonic ECM during UC.
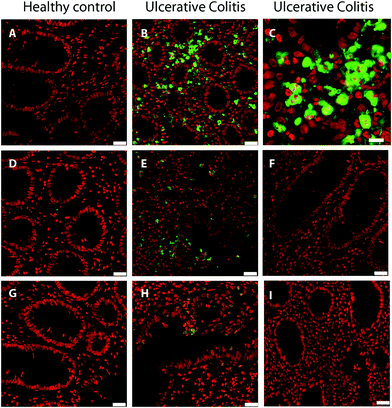 |
| Fig. 4 Confocal microscopy of colon biopsies from a healthy control and an ulcerative colitis patient. (A) Healthy control, (B and C) ulcerative colitis patient stained with anti-protein S100-A9. Healthy control (D and E) Ulcerative colitis patient stained with anti-protein S100-A12. (F) Isotype control with mouse IgG1 monoclonal antibody. (G) Healthy control stained with anti-myeloperoxidase and (H) ulcerative colitis patient. (I) IgG control of ulcerative colitis patient section stained with normal rabbit IgG. Nuclei are stained red with To-Pro3. The bar indicates 25 μm in the picture (A), (B), (D–I) and 10 μm in (C). | |
Discussion
One of the most unexpected results we report here, supported by several separate findings, is the presence of significantly lower levels of multiple ECM proteins in intestinal biopsies from UC patients. Such downregulation can occur as a result of either modulation of transcriptional and translational activity or degradation and displacement (with the second explanation being more likely based on microscopy findings). The notion that degradation drives the changes we observe is further supported by the increased detection of ECM matrix fragments, reported in the serum of UC patients vs. controls or CD patients.57,58 Of note, the current study is not powered to make definitive conclusion regarding the general population, and the hypotheses we raise require extensive confirmatory studies.
Fibrosis is a well described complication of IBD,59 especially in CD.49,50 Therefore, we expected to observe upregulation of collagen proteins and hydroxyproline-containing peptides. Indeed, increases in collagen transcripts were found with all 3 previously published transcriptomics datasets.29,31,60 However, among current proteomics datasets several collagen proteins were found to have lower abundances in the UC group. The discovery of decreased collagen protein abundance in UC was supported by a lower abundance of hydroxyproline-containing peptides, and was in agreement with previous reports of UC colon mucosa.61 The UC patients in our proteomics study were all medicated and no visible inflammation could be observed on the surface of the colon from which the biopsies were taken. Additionally, the histology analysis of the colon biopsies found that the crypt architecture was preserved and without distinct fibrosis, which is in agreement with the proteomics findings. However, an increased presence of neutrophils, NETs, and proinflammatory proteins could be detected with proteomics, and validated by immunofluorescence. We speculate that these seemingly contradictory findings between the collagen protein abundances and the transcript levels are two sides of the same complex process. It is possible that an active process caused by or causing the degradation of the ECM would lead to a compensatory yet insufficient transcriptional response. The comparisons between microarray, RNA-seq, and proteomics data underline the notion that mRNA abundances cannot be directly translated to protein abundances, but rather are a proxy for the protein synthesis-rates. Taken together, the findings indicate the presence of a low-grade inflammation in the tissue, which could trigger the degradation of collagen. It would be interesting to learn the turnover of ECM proteins in the active sites of the disease. Increased rates would support our hypothesis, and increased ECM protein turnover has already been observed in the bleomycin model of pulmonary fibrosis.62
Consistent with ECM degradation, we detected increased abundances of ECM degrading enzymes, including myeloblastin, neutrophil collagenase, MMP9, and MMP10. However, our data somewhat surprisingly cannot confirm that MMP9 and MMP10 upregulation is the cause for the ECM protein degradation as we could not detect increased abundance of the activated MMP fragments, suggesting a lack of MMP activation. This observation requires further validation as we are basing this hypothesis on known MMP substrates which may not represent the full diversity, thus potentially skewing the results. In addition, it is possible the current proteomics workflow may not be able to assess the presence of MMP fragments fully. However, the increase in MMP proteins is consistent with the reduced abundance of collagen proteins and hydroxyproline, and by extension the reduction in ECM protein content, thus adding credibility to the potential of ECM degradation as a major event in IBD pathology. We can further speculate that ECM degradation is an initial trigger for both neutrophil infiltration and ECM transcriptional activation which may overcompensate and eventually result in fibrosis. Purely speculatively, ECM degradation could be triggered partially by leaky epithelial tight junctions in intestinal inflammation. Tight junctions are multi-protein complexes that form a seal between adjacent epithelial cells, and thus act as barriers that normally regulate the transport of macromolecules. UC genetic studies have identified susceptibility loci related to defects of the epithelial barrier,63,64 and IBD patients often demonstrate a loss of tight junction functions. While not sufficient to cause IBD, the loss of the tight junction function causes an immune activation.65 Accordingly, epithelial leaks can be detected early in UC,66 and it has been suggested that these barrier defects allow bacterial antigens to enter the mucosa from the lumen, causing inflammation of the mucosa which could lead to ECM degradation.67 Degradation of ECM proteins could potentially have an impact on IBD pathology. It has been reported that proteolytic fragments (PGP) of ECM proteins can serve as neutrophil chemoattractants through CXCR1.68 PGP has also been identified as a potential biomarker in COPD, another disease where inflammation and neutrophil infiltration play a major role.69 Taken together, emerging evidence of ECM protein degradation and peptidase involvement in the pathology of UC may open new opportunities for biomarker discovery and novel therapeutic options.
We also observed another interesting example of disconnect between protein and mRNA regulation of Chromogranin A (CHGA). CHGA is a great example of a situation where the mRNA and its role in the generation of multiple hormones was characterized decades ago,44,70,71 yet we continue to find novel proteoforms originating from this locus.46 Chromogranin A upregulated peptides also diverge significantly in terms of differential regulation, potentially due to the different regulation and/or secretion of the many proteoforms that are encoded by this locus. While chromogranin A itself is differentially regulated, specific regions of the protein exhibited much larger differences between UC patients and the control population.
An elevated level of NETs is closely associated with general inflammation by the release of a extracellular lattice, composed of DNA associated with proteins citrullinated by protein-arginine deiminase 4 (PAD4) from neutrophils.72 The NETosis or NETlysis processes are associated with a range of autoimmune diseases or triggered by inflammatory activation including UC and reumatroid arthritis.24,73 Berthelot et al. have found that NETosis increases the possibility of association between autoantigens and infectious antigens in mucosal biofilms, impairing the clearance of pathogens and possibly triggering autoimmune reactions such as autoantibody formation (ANCAs). These autoantibodies against NET components have been suggested as possible explanations for the breakdown of tolerance to NET autoantigens, such as hypercitrullination. Of the NETs granule proteins identified in this study, LTF, MPO, and PRTN3 have been associated with autoantibody formation in relation to IBD and ECM-related processes.74 The release of active PAD2 and PAD4 isoforms into synovial fluid by neutrophil cell death is a plausible explanation for the generation of extracellular autoantigens in RA.75 The inhibition of PADs by either small molecule drugs or biologics has been implicated in treatment strategies for autoimmune diseases such as RA but no evidence for such potential has been provided so far.76
Conclusion
One could argue that proteomics data are more complicated than other OMICs data. RNASeq- and DNASeq-data have a single output: variants and/or transcript expression. In contrast, proteomics data can be analyzed in different contexts-expression levels as well as post-translational modifications, and variations. In addition, the field has a much better understanding of splicing isoforms than of proteoforms. All this presents a significant challenge to the community. Therefore, in this work, we re-investigated an existing proteomics dataset, and confirmed and extended the original findings. Some of the differences in the data analysis method included variations in the proteomics identification, filtering, and imputation procedures. However, the largest contributor to the new observations and results can be ascribed to the different statistical test that was applied. In the original dataset, statistically significant proteins were identified mainly using t-tests. In comparison, in this reanalysis, we used tests based on linear mixed effects models, which allowed us to include the replicates in the statistical analysis rather than combining them, thereby increasing the number of significant proteins identified substantially. Combining these findings with an existing microarray and RNAseq-dataset, allowed us to draw novel conclusions, including ECM degradation in UC. In addition to the increased understanding of the pathophysiology of UC, our work demonstrates the significant value in sharing raw data with the community through resources such as PRIDE to enable such cross-OMIC analyses.
Conflicts of interest
There are no conflicts to declare.
Acknowledgements
We would like to acknowledge Thomas Gelsing Carlsen, Torkell Ellingsen, Ole Kristian Bonderup, Henning Glerup, Martin Bøgsted and Gunna Christiansen for their help generating the data that this manuscript is based on and Joseph Szustakowski for helpful comments. We also wish to thank the anonymous reviewers for their help in significantly improving this manuscript.
References
- D. C. Baumgart and S. R. Carding, Inflammatory bowel disease: cause and immunobiology, Lancet, 2007, 369(9573), 1627–1640 CrossRef CAS.
- D. C. Baumgart and W. J. Sandborn, Inflammatory bowel disease: clinical aspects and established and evolving therapies, Lancet, 2007, 369(9573), 1641–1657 CrossRef CAS.
- W. Strober, I. Fuss and P. Mannon, The fundamental basis of inflammatory bowel disease, J. Clin. Invest., 2007, 117(3), 514–521 CrossRef CAS PubMed.
- N. A. Molodecky, I. S. Soon, D. M. Rabi, W. A. Ghali, M. Ferris and G. Chernoff,
et al., Increasing incidence and prevalence of the inflammatory bowel diseases with time, based on systematic review, Gastroenterology, 2012, 142(1), 46–54 CrossRef PubMed.
- F. T. Moller, V. Andersen, J. Wohlfahrt and T. Jess, Familial risk of inflammatory bowel disease: a population-based cohort study 1977–2011, Am. J. Gastroenterol., 2015, 110(4), 564–571 CrossRef PubMed.
- J. Z. Liu, S. van Sommeren, H. Huang, S. C. Ng, R. Alberts and A. Takahashi,
et al., Association analyses identify 38 susceptibility loci for inflammatory bowel disease and highlight shared genetic risk across populations, Nat. Genet., 2015, 47(9), 979–986 CrossRef CAS PubMed.
- L. Jostins, S. Ripke, R. K. Weersma, R. H. Duerr, D. P. McGovern and K. Y. Hui,
et al., Host-microbe interactions have shaped the genetic architecture of inflammatory bowel disease, Nature, 2012, 491(7422), 119–124 CrossRef CAS PubMed.
- V. Andersen, A. K. Hansen and A. B. Heitmann, Potential Impact of Diet on Treatment Effect from Anti-TNF Drugs in Inflammatory Bowel Disease, Nutrients, 2017, 9(3), E286 CrossRef PubMed.
- G. G. Kaplan, The global burden of IBD: from 2015 to 2025, Nat. Rev. Gastroenterol. Hepatol., 2015, 12(12), 720–727 CrossRef PubMed.
- P. Yadav, D. Ellinghaus, G. Remy, S. Freitag-Wolf, A. Cesaro and F. Degenhardt,
et al., Genetic Factors Interact With Tobacco Smoke to Modify Risk for Inflammatory Bowel Disease in Humans and Mice, Gastroenterology, 2017, 153(2), 550–565 CrossRef CAS PubMed.
- A. Dignass, J. O. Lindsay, A. Sturm, A. Windsor, J. F. Colombel and M. Allez,
et al., Second European evidence-based consensus on the diagnosis and management of ulcerative colitis part 2: current management, J. Crohns Colitis, 2012, 6(10), 991–1030 CrossRef PubMed.
- A. Dignass, R. Eliakim, F. Magro, C. Maaser, Y. Chowers and K. Geboes,
et al., Second European evidence-based consensus on the diagnosis and management of ulcerative colitis part 1: definitions and diagnosis, J. Crohns Colitis, 2012, 6(10), 965–990 CrossRef PubMed.
- A. Dignass, G. Van Assche, J. O. Lindsay, M. Lemann, J. Soderholm and J. F. Colombel,
et al., The second European evidence-based Consensus on the diagnosis and management of Crohn's disease: Current management, J. Crohns Colitis, 2010, 4(1), 28–62 CrossRef CAS PubMed.
- G. Van Assche, A. Dignass, B. Bokemeyer, S. Danese, P. Gionchetti and G. Moser,
et al., Second European evidence-based consensus on the diagnosis and management of ulcerative colitis part 3: special situations, J. Crohns Colitis, 2013, 7(1), 1–33 CrossRef PubMed.
- G. Van Assche, A. Dignass, J. Panes, L. Beaugerie, J. Karagiannis and M. Allez,
et al., The second European evidence-based Consensus on the diagnosis and management of Crohn's disease: Definitions and diagnosis, J. Crohns Colitis, 2010, 4(1), 7–27 CrossRef PubMed.
- G. Van Assche, A. Dignass, W. Reinisch, C. J. van der Woude, A. Sturm and M. De Vos,
et al., The second European evidence-based Consensus on the diagnosis and management of Crohn's disease: Special situations, J. Crohns Colitis, 2010, 4(1), 63–101 CrossRef PubMed.
- G. Bouguen and L. Peyrin-Biroulet, Surgery for adult Crohn's disease: what is the actual risk?, Gut, 2011, 60(9), 1178–1181 CrossRef PubMed.
- L. Hancock and N. J. Mortensen, How often do IBD patients require resection of their intestine?, Inflammatory Bowel Dis., 2008, 14(Suppl 2), S68–S69 CrossRef PubMed.
- H. Hammad and B. N. Lambrecht, Barrier Epithelial Cells and the Control of Type 2 Immunity, Immunity, 2015, 43(1), 29–40 CrossRef CAS PubMed.
- A. Kaser, S. Zeissig and R. S. Blumberg, Inflammatory bowel disease, Annu. Rev. Immunol., 2010, 28, 573–621 CrossRef CAS PubMed.
- J. A. Whitsett and T. Alenghat, Respiratory epithelial cells orchestrate pulmonary innate immunity, Nat. Immunol., 2015, 16(1), 27–35 CrossRef CAS PubMed.
- L. W. Peterson and D. Artis, Intestinal epithelial cells: regulators of barrier function and immune homeostasis, Nat. Rev. Immunol., 2014, 14(3), 141–153 CrossRef CAS PubMed.
- T. Bennike, S. Birkelund, A. Stensballe and V. Andersen, Biomarkers in inflammatory bowel diseases: current status and proteomics identification strategies, World J. Gastroenterol., 2014, 20(12), 3231–3244 CrossRef CAS PubMed.
- T. B. Bennike, T. G. Carlsen, T. Ellingsen, O. K. Bonderup, H. Glerup and M. Bøgsted,
et al., Neutrophil Extracellular Traps in Ulcerative Colitis: A Proteome Analysis of Intestinal Biopsies, Inflammatory Bowel Dis., 2015, 21(9), 2052–2067 CrossRef PubMed.
- J. Cox and M. Mann, MaxQuant enables high peptide identification rates, individualized p.p.b.-range mass accuracies and proteome-wide protein quantification, Nat. Biotechnol., 2008, 26(12), 1367–1372 CrossRef CAS PubMed.
- Rstudio Team. RStudio: Integrated Development for R. 2016, available from: http://www.rstudio.com.
- R Core Team. R: A language and environment for statistical computing. R Foundation for Statistical Computing. 2017, available from: https://www.R-project.org/.
- R Core Team, J. Pinheiro, D. Bates, S. DebRoy and D. Sarkar, nlme: Linear and Nonlinear Mixed Effects Models. R package version 3.1-137, 2018, available from: https://CRAN.R-project.org/package=nlme.
- N. Planell, J. J. Lozano, R. Mora-Buch, M. C. Masamunt, M. Jimeno and I. Ordás,
et al., Transcriptional analysis of the intestinal mucosa of patients with ulcerative colitis in remission reveals lasting epithelial cell alterations, Gut, 2013, 62(7), 967–976 CrossRef CAS PubMed.
- S. Kugathasan, R. N. Baldassano, J. P. Bradfield, P. M. A. Sleiman, M. Imielinski and S. L. Guthery,
et al., Loci on 20q13 and 21q22 are associated with pediatric-onset inflammatory bowel disease, Nat. Genet., 2008, 40(10), 1211–1215 CrossRef CAS PubMed.
- J. Olsen, T. A. Gerds, J. B. Seidelin, C. Csillag, J. T. Bjerrum and J. T. Troelsen,
et al., Diagnosis of ulcerative colitis before onset of inflammation by multivariate modeling of genome-wide gene expression data, Inflammatory Bowel Dis., 2009, 15(7), 1032–1038 CrossRef PubMed.
- B. Zhang, S. Kirov and J. Snoddy, WebGestalt: an integrated system for exploring gene sets in various biological contexts, Nucleic Acids Res., 2005, 33(Web Server issue), W741–W748 CrossRef CAS PubMed.
- C. A. Schneider, W. S. Rasband and K. W. Eliceiri, NIH Image to ImageJ: 25 years of image analysis, Nat. Methods, 2012, 9(7), 671–675 CrossRef CAS PubMed.
- S. C. Hicks and R. A. Irizarry, quantro: a data-driven approach to guide the choice of an appropriate normalization method, Genome Biol., 2015, 16(1), 117 CrossRef PubMed.
- C. F. Urban, D. Ermert, M. Schmid, U. Abu-Abed, C. Goosmann and W. Nacken,
et al., Neutrophil extracellular traps contain calprotectin, a cytosolic protein complex involved in host defense against Candida albicans, PLoS Pathog., 2009, 5(10), e1000639 CrossRef PubMed.
- A. Subramanian, P. Tamayo, V. K. Mootha, S. Mukherjee, B. L. Ebert and M. A. Gillette,
et al., Gene set enrichment analysis: A knowledge-based approach for interpreting genome-wide expression profiles, Proc. Natl. Acad. Sci. U. S. A., 2005, 102(43), 15545–15550 CrossRef CAS PubMed.
- M. Pruteanu, N. P. Hyland, D. J. Clarke, B. Kiely and F. Shanahan, Degradation of the extracellular matrix components by bacterial-derived metalloproteases: implications for inflammatory bowel diseases, Inflammatory Bowel Dis., 2011, 17(5), 1189–1200 CrossRef PubMed.
- E. Shimshoni, D. Yablecovitch, L. Baram, I. Dotan and I. Sagi, ECM remodelling in IBD: innocent bystander or partner in crime? The emerging role of extracellular molecular events in sustaining intestinal inflammation, Gut, 2015, 64(3), 367–372 CrossRef CAS PubMed.
- G. Kustatscher, P. Grabowski and J. Rappsilber, Pervasive coexpression of spatially proximal genes is buffered at the protein level, Mol. Syst. Biol., 2017, 13(8), 937 CrossRef PubMed.
- A. Koussounadis, S. P. Langdon, I. H. Um, D. J. Harrison and V. A. Smith, Relationship between differentially expressed mRNA and mRNA-protein correlations in a xenograft model system, Sci. Rep., 2015, 5, 10775 CrossRef PubMed.
- M. Carlson, Y. Raab, L. Sevéus, S. Xu, R. Hällgren and P. Venge, Human neutrophil lipocalin is a unique marker of neutrophil inflammation in ulcerative colitis and proctitis, Gut, 2002, 50(4), 501–506 CrossRef CAS PubMed.
- P. J. Koelink, S. A. Overbeek, S. Braber, M. E. Morgan, P. A. J. Henricks and M. A. Roda,
et al., Collagen degradation and neutrophilic infiltration: a vicious circle in inflammatory bowel disease, Gut, 2014, 63(4), 578–587 CrossRef CAS PubMed.
- H. Taman, C. G. Fenton, I. V. Hensel, E. Anderssen, J. Florholmen and R. H. Paulssen, Transcriptomic Landscape of Treatment—Naïve Ulcerative Colitis, J. Crohns Colitis, 2018, 12(3), 327–336 CrossRef PubMed.
- D. S. Konecki, U. M. Benedum, H. H. Gerdes and W. B. Huttner, The primary structure of human chromogranin A and pancreastatin, J. Biol. Chem., 1987, 262(35), 17026–17030 CAS.
- D. F. Orr, T. Chen, A. H. Johnsen, R. Chalk, K. D. Buchanan, J. M. Sloan, P. Rao and C. Shaw, The spectrum of endogenous human chromogranin A-derived peptides identified using a modified proteomic strategy, Proteomics, 2002, 2(11), 1586–1600 CrossRef.
- P. Shooshtarizadeh, D. Zhang, J.-F. Chich, C. Gasnier, F. Schneider and Y. Haïkel,
et al., The antimicrobial peptides derived from chromogranin/secretogranin family, new actors of innate immunity, Regul. Pept., 2010, 165(1), 102–110 CrossRef CAS PubMed.
- D. Zhang, P. Shooshtarizadeh, B. J. Laventie, D. A. Colin, J. F. Chich, J. Vidic, J. de Barry, S. Chasserot-Golaz, F. Delalande, A. Van Dorsselaer, F. Schneider, K. Helle, D. Aunis, G. Prévost and M. H. Metz-Boutigue, Two Chromogranin A-Derived Peptides Induce Calcium Entry in Human Neutrophils by Calmodulin-Regulated Calcium Independent Phospholipase A2, PLoS One, 2009, 4(2), e4501 CrossRef PubMed.
- Y. Toyoki, M. Sasaki, S. Narumi, S. Yoshihara, T. Morita and M. Konn, Semiquantitative evaluation of hepatic fibrosis by measuring tissue hydroxyproline, Hepatogastroenterology, 1998, 45(24), 2261–2264 CAS.
- F. Rieder and C. Fiocchi, Mechanisms of tissue remodeling in inflammatory bowel disease, Dig. Dis., 2013, 31(2), 186–193 CrossRef PubMed.
- C. Fiocchi and P. K. Lund, Themes in fibrosis and gastrointestinal inflammation, Am. J. Physiol.: Gastrointest. Liver Physiol., 2011, 300(5), G677–G683 CrossRef CAS PubMed.
- I. Cleynen, E. Vazeille, M. Artieda, H. W. Verspaget, M. Szczypiorska and M.-A. Bringer,
et al., Genetic and microbial factors modulating the ubiquitin
proteasome system in inflammatory bowel disease, Gut, 2014, 63(8), 1265–1274 CrossRef CAS PubMed.
- M. Rojkind and E. González, An improved method for determining specific radioactivities of proline-14C and hydroxyproline-14C in collagen and in noncollagenous proteins, Anal. Biochem., 1974, 57(1), 1–7 CrossRef CAS PubMed.
- J. Brazil, N. Louis and C. Parkos, The role of polymorphonuclear leukocyte trafficking in the perpetuation of inflammation during inflammatory bowel disease, Inflammatory Bowel Dis., 2013, 19(7), 1556–1565 CrossRef PubMed.
- K. G. Sifroni, C. R. Damiani, C. Stoffel, M. R. Cardoso, G. K. Ferreira and I. C. Jeremias,
et al., Mitochondrial respiratory chain in the colonic mucosal of patients with ulcerative colitis, Mol. Cell. Biochem., 2010, 342(1–2), 111–115 CrossRef CAS PubMed.
- R. Suades, T. Padró and L. Badimon, The Role of Blood-Borne Microparticles in Inflammation and Hemostasis, Semin Thromb Hemostasis., 2015, 41(6), 590–606 CrossRef CAS PubMed.
- A. S. Shet, Characterizing blood microparticles: Technical aspects and challenges, Vasc. Health Risk Manag., 2008, 4(4), 769–774 CrossRef PubMed.
- J. H. Mortensen, T. Manon-Jensen, M. D. Jensen, P. Hägglund, L. G. Klinge and J. Kjeldsen,
et al., Ulcerative colitis, Crohn's disease, and irritable bowel syndrome have different profiles of extracellular matrix turnover, which also reflects disease activity in Crohn's disease, PLoS One, 2017, 12(10), e0185855 CrossRef PubMed.
- J. H. Mortensen, L. E. Godskesen, M. D. Jensen, W. T. Van Haaften, L. G. Klinge and P. Olinga,
et al., Fragments of Citrullinated and MMP-degraded Vimentin and MMP-degraded Type III Collagen Are Novel Serological Biomarkers to Differentiate Crohn's Disease from Ulcerative Colitis, J. Crohns Colitis, 2015, 9(10), 863–872 CrossRef PubMed.
- F. Rieder and C. Fiocchi, Intestinal fibrosis in IBD--a dynamic, multifactorial process, Nat. Rev. Gastroenterol. Hepatol., 2009, 6(4), 228–235 CrossRef CAS PubMed.
- K. Holgersen, B. Kutlu, B. Fox, K. Serikawa, J. Lord and A. K. Hansen,
et al., High-Resolution Gene Expression Profiling Using RNA Sequencing in Patients With Inflammatory Bowel Disease and in Mouse Models of Colitis, J. Crohns Colitis, 2015, 9(6), 492–506 CrossRef PubMed.
- M. F. Graham, R. F. Diegelmann, C. O. Elson, W. J. Lindblad, N. Gotschalk and S. Gay,
et al., Collagen content and types in the intestinal strictures of Crohn's disease, Gastroenterology, 1988, 94(2), 257–265 CrossRef CAS.
- M. L. Decaris, M. Gatmaitan, S. FlorCruz, F. Luo, K. Li and W. E. Holmes,
et al., Proteomic Analysis of Altered Extracellular Matrix Turnover in Bleomycin-induced Pulmonary Fibrosis, Mol. Cell. Proteomics, 2014, 13(7), 1741–1752 CrossRef CAS PubMed.
- C. A. Anderson, G. Boucher, C. W. Lees, A. Franke, M. D’Amato and K. D. Taylor,
et al., Meta-analysis identifies 29 additional ulcerative colitis risk loci, increasing the number of confirmed associations to 47, Nat. Genet., 2011, 43(3), 246–252 CrossRef CAS PubMed.
- UK IBD Genetics Consortium, J. C. Barrett, J. C. Lee, C. W. Lees, N. J. Prescott and C. A. Anderson,
et al., Genome-wide association study of ulcerative colitis identifies three new susceptibility loci, including the HNF4A region, Nat. Genet., 2009, 41(12), 1330–1334 CrossRef PubMed.
- K. L. Edelblum and J. R. Turner, The tight junction in inflammatory disease: communication breakdown, Curr. Opin. Pharmacol., 2009, 9(6), 715–720 CrossRef CAS PubMed.
- A. H. Gitter, K. Bendfeldt, J. D. Schulzke and M. Fromm, Leaks in the epithelial barrier caused by spontaneous and TNF-alpha-induced single-cell apoptosis, FASEB J., 2000, 14(12), 1749–1753 CrossRef CAS PubMed.
- J. D. Schulzke, S. Ploeger, M. Amasheh, A. Fromm, S. Zeissig and H. Troeger,
et al., Epithelial tight junctions in intestinal inflammation, Ann. N. Y. Acad. Sci., 2009, 1165, 294–300 CrossRef PubMed.
- A. Gaggar, P. L. Jackson, B. D. Noerager, P. J. O’Reilly, D. B. McQuaid and S. M. Rowe,
et al., A Novel Proteolytic Cascade Generates an Extracellular Matrix-Derived Chemoattractant in Chronic Neutrophilic Inflammation, J. Immunol., 2008, 180(8), 5662–5669 CrossRef CAS.
- M. A. Roda, A. M. Fernstrand, F. A. Redegeld, J. E. Blalock, A. Gaggar and G. Folkerts, The matrikine PGP as a potential biomarker in COPD, Am. J. Physiol.: Lung Cell. Mol. Physiol., 2015, 308(11), L1095–L1101 CrossRef PubMed.
- U. M. Benedum, P. A. Baeuerle, D. S. Konecki, R. Frank, J. Powell and J. Mallet,
et al., The primary structure of bovine chromogranin A: a representative of a class of acidic secretory proteins common to a variety of peptidergic cells, EMBO J., 1986, 5(7), 1495–1502 CrossRef CAS.
- A. L. Iacangelo, R. Fischer-Colbrie, K. J. Koller, M. J. Brownstein and L. E. Eiden, The Sequence Of Porcine Chromogranin A Messenger Rna Demonstrates Chromogranin A Can Serve As The Precursor For The Biologically Active Hormone, Pancreastatin, Endocrinology, 1988, 122(5), 2339–2341 CrossRef CAS PubMed.
- D. N. Nguyen, A. Stensballe, J. C. Lai, P. Jiang, A. Brunse and Y. Li,
et al., Elevated levels of circulating cell-free DNA and neutrophil proteins are associated with neonatal sepsis and necrotizing enterocolitis in immature mice, pigs and infants, Innate Immun., 2017, 23(6), 524–536 CrossRef CAS PubMed.
- J. M. Berthelot, B. Le Goff, A. Neel, Y. Maugars and M. Hamidou, NETosis: At the crossroads of rheumatoid arthritis, lupus, and vasculitis, Jt., Bone, Spine, 2017, 84(3), 255–262 CrossRef PubMed.
- D. Wang, L. Yang, P. Zhang, J. LaBaer, H. Hermjakob and D. Li,
et al., AAgAtlas 1.0: a human autoantigen database, Nucleic Acids Res., 2017, 45(D1), D769–D776 CrossRef CAS PubMed.
- J. Spengler, B. Lugonja, A. J. Ytterberg, R. A. Zubarev, A. J. Creese and M. J. Pearson,
et al., Release of Active Peptidyl Arginine Deiminases by Neutrophils Can Explain Production of Extracellular Citrullinated Autoantigens in Rheumatoid Arthritis Synovial Fluid, Arthritis Rheumatol., 2015, 67(12), 3135–3145 CrossRef CAS PubMed.
- V. C. Willis, N. K. Banda, K. N. Cordova, P. E. Chandra, W. H. Robinson and D. C. Cooper,
et al., Protein arginine deiminase 4 inhibition is sufficient for the amelioration of collagen-induced arthritis, Clin. Exp. Immunol., 2017, 188(2), 263–274 CrossRef CAS PubMed.
Footnote |
† Electronic supplementary information (ESI) available. See DOI: 10.1039/c8mo00239h |
|
This journal is © The Royal Society of Chemistry 2019 |
Click here to see how this site uses Cookies. View our privacy policy here.