DOI:
10.1039/C8MO00137E
(Research Article)
Mol. Omics, 2019,
15, 59-66
Integrative data fusion for comprehensive assessment of a novel CHEK2 variant using combined genomics, imaging, and functional–structural assessments via protein informatics†
Received
17th June 2018
, Accepted 17th December 2018
First published on 18th December 2018
Abstract
The CHEK2 gene and its encoded protein Chk2 have a well-known role in cancers, especially those related to breast cancer mediated through the BRCA1 gene. Additionally Chk2 has a crucial role in DNA repair, apoptosis and the cell cycle, which is why classification of variants of uncertain significance (VUS) is an area highly sought for a better elucidation of the “genomic effect” that results. Because it can often take years before enough clinical data is accumulated, and the costly and expensive functional analysis for individual variants presents a significant hurdle, it is important to identify other tools to help aid in clarifying the impact of specific variants on a protein's function and eventually the patient's health outcome. Here we describe a newly identified CHEK2 variant and analyze with an integrated approach combining genomics (whole exome analysis), clinical study, radiographic imaging, and protein informatics to identify and predict the functional impact of the VUS on the protein's behavior and predicted impact on the related pathways. The observed and analyzed defects in the protein were consistent with the expected clinical effect. Here, we support the use of personalized protein modeling and informatics and further our goal of developing a large-scale protein deposition archive for all protein-level VUS.
Introduction
Identification of a hereditary cancer syndrome in a patient can have a meaningful impact on that individual's medical management. Depending on the result, increased surveillance to improve detection or prophylactic surgery to lower the risk may be recommended.1 Genetic testing, however, may reveal the presence of a variant that is not well characterized and thus does not always provide a clear answer regarding whether or not a patient has a strong genetic predisposition to cancer. Genetic variants identified can be classified as pathogenic, likely pathogenic, uncertain significance, likely benign or benign.2 Guidelines have been created to help standardize the variant classification process,2 but discrepancies still exist between laboratories.3,4
The Prospective Registry of Multiplex Testing (PROMPT) analyzed how many of the variants reported by their participants had conflicting classifications by different commercial laboratories.4 Of the 1191 individuals who self-enrolled into the study, 603 genetic variants in cancer susceptibility genes were reported. Around 25% (155/603) of the variants had not been classified identically by all laboratories. Conflicting classifications were discovered most frequently within the CHEK2 and ATM genes. There were specifically seven variants within CHEK2 that were classified as pathogenic or likely pathogenic by one lab and as a variant of uncertain significance by another lab. CHEK2 is shown in comparison with other relevant genes (Table 1). Medical management recommendations generally would not be based on a variant of uncertain significance,5 but the discovery of a (likely) pathogenic variant in CHEK2 could impact care. Depending on which laboratory completed the testing, a genetic test report may or may not support altering care.
Table 1 Breast cancer high/moderate risk panel
Gene |
Lifetime cancer and/or tumor risks |
BRCA1
|
Female breast (57–87%), ovarian (24–54%), prostate, male breast, pancreatic, fallopian tube, primary peritoneal, endometria |
BRCA2
|
Female breast (41–84%), prostate (20–34%), ovarian (11–27%), pancreatic (5–7%), male breast (4–7%), melanoma, fallopian tube, primary peritoneal, endometrial |
TP53
|
Female breast, sarcoma-bone and soft tissue, brain, hematologic malignancies, adrenocortical carcinoma, among others. Overall risk for cancer: nearly 100% in females, 73% in males |
PTEN
|
Female breast (25–85%), thyroid (3–38%), endometrial (5–28%), colon, renal, melanoma, gastrointestinal polyps |
CDH1
|
Gastric cancer (40–83%), female breast (39–52%), colon |
PALB2
|
Female breast (25–58%), male breast, pancreatic, ovarian |
ATM
|
Female breast, colon, pancreatic |
CHEK2
|
Female breast, male breast, colon, prostate, thyroid, endometrial, ovarian |
Pathogenic variants in CHEK2 may be associated with increased risk for multiple cancers, including breast, prostate, colon, thyroid and kidney cancer (Table 2).6 Breast cancer may be the most well defined cancer risk, but risk estimates can be influenced by genotype and family history.7,8 For example, the missense I157T variant in CHEK2 is suspected to be associated with lower cancer risks than the frameshift 1100delC variant.7 Breast management recommendations by the National Comprehensive Cancer Network are based on evidence regarding frameshift mutations in CHEK2.1 Women with pathogenic frameshift mutations are recommended to consider completing annual mammogram with tomosynthesis and breast MRI with contrast.1 Colorectal surveillance recommendations suggest colonoscopy at age 40 or 10 years prior to the youngest diagnosis of colorectal cancer in a first degree relative.9
Table 2 Cancer risk estimates associated with CHEK2 variants
Organ |
Cancer risk (95% CI)* |
Comment |
Ref. |
Comments: *95% confidence interval presented if available. CHEK2p.I157T variant. CHEK2 truncating mutation.^Values based on odds ratio multiplied by general population risk for breast cancer based on the SEER Cancer Statistics Review (Noone, A. M., et al., 2018). |
Breast |
20% (18–22)^a |
|
Han et al. 2013 |
Colon |
7% (5–10)^a |
|
Breast |
20%b |
No affected 1st or 2nd degree relative |
Cybulski et al. 2011 |
28%b |
Affected 2nd degree relative |
34%b |
Affected 1st degree relative |
44%b |
Affected 1st and 2nd degree relative |
Breast |
37% (26–56)b |
|
Weisher et al. 2008 |
Breast |
17%^a |
|
Cybulski et al. 2004 |
Colon |
8%^a |
Prostate |
25%^a |
Thyroid |
2%^a |
Kidney |
3%^a |
Functional studies have been completed on one of these conflicting CHEK2 variants, p.Glu161del, in an attempt to more confidently classify it as benign or pathogenic.10,11 The p.Glu161del variant protein product was found to be no longer stable and kinase activity was apparently null.10,11 These two studies supported pathogenicity of the CHEK2 Glu161del variant, but some laboratories still have not felt comfortable upgrading the classification to pathogenic or likely pathogenic in Clinvar. The role of CHEK2 in cancer, the cell cycle and DNA repair is well known (Fig. 1).4,7–11
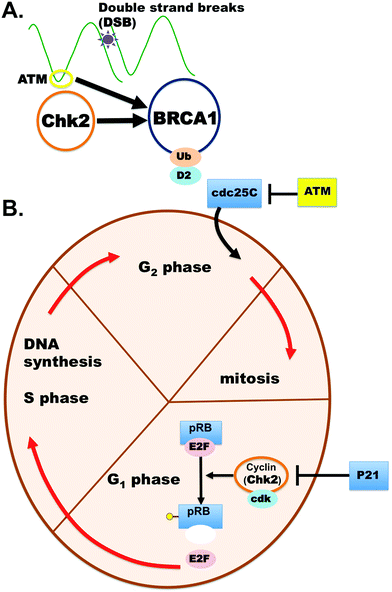 |
| Fig. 1
CHEK2 role in cancers. (A) CHEK2 protein plays a crucial role in cancers and has a direct interaction with other important cancer genes like BRCA1. (B) CHEK2 is a cyclin-dependent kinase that has a role in the cell cycle, DNA repair and apoptosis. | |
This variant was identified in a patient at the Mayo Clinic FL Hereditary Cancer Clinic. The clinical presentation of the proband is described below. Protein molecular modeling was completed to better evaluate the likelihood of pathogenicity.
Case presentation
A 44 year-old woman was referred to the genetic clinic following her diagnosis of stage IIA invasive ductal carcinoma in the left breast. After palpation of a left breast lump, a diagnostic mammogram was performed that detected an irregular mass. A subsequent ultrasound showed a 2.5 × 3.2 × 2.5 cm heterogeneous, mixed density mass; biopsy of this mass revealed the presence of invasive ductal carcinoma, grade 3, estrogen receptor positive ER [+]/progesterone receptor negative PR [−]/Her2-Neu positive HER-2 [+] (Fig. 2). She underwent a left total mastectomy with axillary sentinel lymph node biopsy, right prophylactic mastectomy and immediate reconstruction with tissue expanders. The patient had an extensive family history of cancer, which raised suspicion for a familial cancer syndrome. Her mother was diagnosed with breast cancer at 59 years of age, and her father was diagnosed with melanoma in his 50s and prostate cancer in his 60s. Her brother was diagnosed with bladder cancer at age 33. Other family members with breast cancer included a paternal grandmother, paternal aunt and two paternal cousins (Fig. 3). She completed an 8 gene panel, which included the following genes: ATM, BRCA1, BRCA2, CDH1, CHEK2, PALB2, PTEN, PTEN, and TP53. She was found to be heterozygous for a likely pathogenic variant [c.483_485delAGA (p.Glu161del)] in CHEK2.
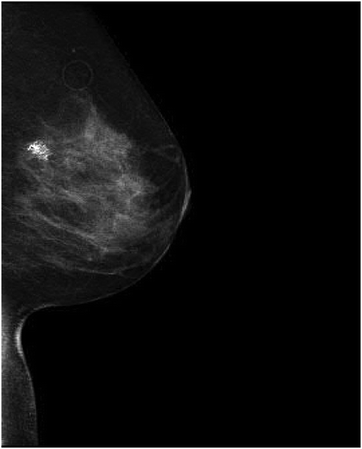 |
| Fig. 2 CHK2-dependent tumor formation. An irregular mass with associated dystrophic calcifications and radiopaque marker in the upper-outer quadrant of the left breast. | |
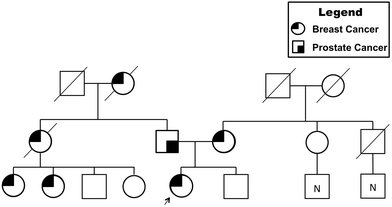 |
| Fig. 3 Familial pedigree for cancer genetics. Presentation of prostate and breast cancer prevalence within the patient's family is indicated. | |
Results
For WT versus the truncation variant p.E161del, we found the stability of the object from energetic calculations for ΔG to be quite informative on the change in object stability (folding state) and ability to partner (form dimers). Stability of the object for CHEK2 (all 543 amino acids), as measured by ΔG, is 140.95 kcal mol−1 Å−2 and per residue is 0.26 kcal aa−1 mol−1 Å−2. The p.E161del variant has a total stability ΔG of 59.05 kcal mol−1 Å−2 and per residue of 0.37 kcal aa−1 mol−1 Å−2, which indicates decreased stability for the VUS that would account for lower dimerization likelihood. If we look instead at direct interaction energy (favorable) for the dimer complex instead of the individual object stability, we also see an interesting decrease in dimerization favorability, where the full-length dimer has a ΔG (interaction) of −48.05 kcal mol−1 Å−2 that is quite favorable, versus the p.E161del VUS, which has only −1.62 kcal mol−1 Å−2 indicating a loss of dimerization. We have used this method in the past hypothesis generation and mutagenesis experiments.12–18
This object stability did indicate changes in structure that were deleterious to formation of the complex from immediate inspection; additionally the loss of the kinase domain is detrimental to the protein having any function (Fig. 4). Additionally, we examined the local residues that may be useful to explain any change in function. The molecular models for the full structure and its truncated form were analyzed using our established techniques.12–15,18–26
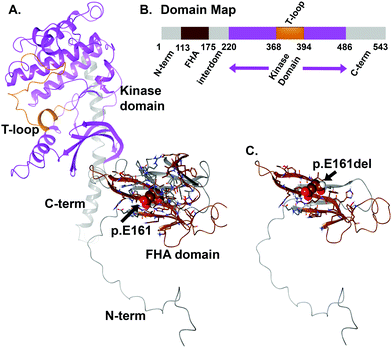 |
| Fig. 4
CHEK2 molecular model for the full-length human sequence consisting of 543 amino acids and the truncation variant p.E161del. (A) Full-length model for the entire CHEK2 structure that shows two clear domains separated around amino acids 175–220, where E171 plays a role in the FHA domain that precedes the kinase domain (220–486). (B) Domain map for CHEK2 is shown. The 543 amino acid protein has the domains labeled by the corresponding color shown on the ribbons in panels A and C. (C) Truncation structure for p.E161del of CHEK2 protein. The labeling for both models is indicated. All protein ribbons are colored by the domain map color key and residues shown are rendered as either “licorice sticks” or van der Waals (vdW) spheres and using standard element coloring (O – red, N – blue, H – white, S – yellow) except for the carbon atoms that are colored to match the domain key color map. | |
Local residues within the 12 Å cutoff near the “cleavage” site are quite distal from the domain critical to function (kinase domain), so we can focus on the loss-of-function as a result of loss-of-domain rather than due to individual amino acids in or about the region of the truncation site (Fig. 4 and 5A, B). Additionally, we can see from the mapping of the surface (blue and brown) for the interaction interface of the predicted dimer of CHEK2 that there is substantial loss of the interface from the wild type to the p.E161del variant. In addition, the electrostatic surface shown in the zoomed-in insets in the figure panel reveals a loss of attractive potential that holds the complex together (Fig. 5A–D).
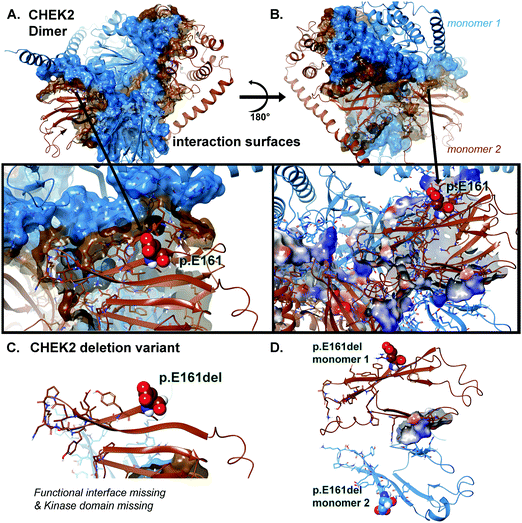 |
| Fig. 5
CHEK2 surface and electrostatic mapping for interaction potentials. (A) Full-length model for the entire CHEK2 dimeric structure colored as either blue or brown ribbons and surface to distinguish the two protein monomer chains. And for the inset panels indicated directly below, electrostatics as calculated from Poisson–Boltzmann (PB) is shown for the important region around p.E161 using an overlay onto the structure. The PB is colored from positive to negative using a blue-white-red scheme. Interacting residues between the two proteins are shown along with electrostatics mapped onto. (B) The dimeric structure is rotated 180° to allow a different view of the interaction surface and the corresponding inset below matches. (C) Deletion construct for the truncated p.E161del for CHEK2 is given with only ribbons shown. (D) Rotation by the X–Y plane at 180° is done to show the birds-eye-view of the remaining interaction interface of the truncation VUS with the minimal PB surface shown overlaid on that region. | |
We mapped the electrostatic charges upon the protein–protein interface of the dimer complex using the Poisson–Boltzmann calculation for both the 543 amino acid structure and the 161 amino acid structure. The effects of the changes were quite obvious (Fig. 5), where we used a distribution cutoff of +3 KT/E for both maps. The WT particle (all 543 aa) has a balanced distribution for electrostatic charge at the interaction zones between the two protein chains, which is >90% lost in the p.E161del VUS (Fig. 5D). The p.E161del particle is nearly dissociated into separate proteins (Fig. 5C), which accounts for lowering of its interaction potential with partner proteins and the loss of attractive ΔG.12–18
Discussion and conclusions
While it is interesting that the energetics measurements from the calculations for the variants and the wild-type clearly indicate defects in protein behavior and the electrostatic calculations show interaction deficits that would negatively impact partner protein interactions, it is also interesting to note the local conformational changes that have a correlated motion effect on distal regions of the protein that occur from application of simulations to determine the conformational changes of the structure that would likely happen as a consequence of these variants’ changes.
The results of this analysis appear to further support the previous findings of pathogenicity of the CHEK2 p.Glu161del variant.10,11 The application of these modeling techniques for the expansion of protein-level knowledge may provide a diagnostic tool for clinicians (to use in conjunction with clinical and family history); this has been ongoing and moving towards development of a database.27,28
Methods
Clinical genomics
Our clinical genomics team has multiple resources at disposal for these studies, including the hematology/oncology clinic, which is located on the 3rd floor of the Mangurian Building on the Mayo Clinic Florida campus. This is the primary site for all clinical research activity with offices of all the faculty, allied health professionals, treatment infusion center and the administrative staff. The hematology/oncology clinic provides comprehensive support to all patients with hematologic diseases as well as solid tumor cancers. All patients are evaluated and treated on this floor by expert hematologists/oncologists. The hematology clinic also has extensive collaborations with the department of medicine faculty for referrals within the Mayo Clinic system as well as regional cancer centers and primary care providers of the greater Jacksonville and the “Panhandle” area. Mayo Clinic is the only NCI designated cancer center in the northern Florida. Additionally, the BAP/PRC Laboratory resource provides biospecimen procurement (blood, body fluids and tissue), processing, storage, DNA extractions on whole blood and buffy coat samples and a full-service research histology laboratory which includes immunohistochemistry, digital slide imaging and tissue micro array services to all Mayo investigators. We have 6 full-time Biospecimen Collection Technologists and 1 full-time supervisor. The lab provides reports to investigators, real-time Research Laboratory Information Management System (RLIMS) biospecimen tracking on collected samples and support services to Cancer Clinical Studies and General Clinical Studies Units for budget information, collection services, study set-up and any protocol supply purchasing. We support approximately 30 clinical and basic science principal investigators and their collections. We will be using the facility for storage and processing of the animal tumor tissues for further immunohistochemical studies. The BAP/PRC lab in MN has pathology core and gene expression, cytogenetics and genotyping facilities, which are accessible and can be used during the grant period as and when necessary. Additionally, we have the Biostatistics Core, a part of the Division of Biomedical Statistics and Informatics which provides comprehensive biostatistics consulting and analysis services to Mayo Clinic investigators at all stages of the research process. The core, including genetic analyses, clinical trials, outcomes research and clinical studies with individual Mayo Clinic departments, supports large ranges of project types. As needed, the core works to develop and refine statistical methods and software. Core members work within a team structure that includes—depending on the needs of the project—PhD statisticians, bioinformatics specialists and programmers. At any given time, the core is collaborating on thousands of projects. Personnel include more than 60 master's-level statisticians who consult on protocol development, study design and statistical methodologies. There are also more than 90 statistical programmer analysts who support data management and analysis. The core is closely involved in studies connected to Mayo Clinic centers, including the Center for Individualized Medicine, Center for Regenerative Medicine and Center for the Science of Health Care Delivery. Importantly, this core will support our bioinformatics and genomics analysis needs. Additionally, our Next Generation Sequencing division has computer resources available on the general HPC cluster, RCS supports dedicated hardware and software workflows dedicated for whole genome and exome sequencing analysis. The HPC cluster has 60 Nehalem nodes with 8 cores and 32 Gb of RAM and 2 TB of temporary storage. Also, it has six Advanced HPC nodes with 48 cores and 512 Gb of RAM. The storage available is 1.2 PB of Isilon X, 0.5 PB of Isilon NL in Rochester and 1.0 PB of Isilon NL in Arizona for long-term storage. From these resources we have amassed a growing set of data using this integrative process.28–30
Whole exome sequencing
Using genomic DNA extracted from the patient and family members, the Agilent Clinical Research Exome kit was used to target exonic regions and flanking splice junctions of the genome. These regions were sequenced by massively parallel sequencing on an Illumina HiSeq with 100
bp paired-end reads (mean depth of coverage: 119×; quality threshold: 94.9%). The bidirectional sequence was assembled, aligned to reference gene sequences based on human genome builds, and analyzed for sequence variants using a custom-developed analysis tool (Xome Analyzer, GeneDx). Capillary sequencing was used to confirm the presence or absence of all potentially pathogenic variants identified in all samples.
Experimental radiography
Our description of data collection for radiographic samples and patient imaging was collected. Radiographic imaging was obtained for members of the family, and involved multiple scans. We reviewed all scans available that had been obtained for members for clinical purposes. The primary radiographic image is given (Fig. 2).
Molecular modeling
The CHEK2 (or CHK2) gene encodes for the protein Checkpoint Kinase 2 (Chk2), a serine/threonine-protein kinase which is required for checkpoint-mediated cell cycle arrest and apoptosis. The CHK2 protein is also implicated in DNA repair and cellular apoptosis in response to DNA double-strand breaks. The cellular arrest occurs through multiple phosphorylation events with Cell Division Cycle (CDC) proteins and leads to increased Cyclin-Dependent Kinase (CDK)-cyclin inhibition and prevention of continuation of the cell cycle (Fig. 4). The CHEK2 gene sequence was taken from the NCBI Reference Accession Sequence: NP_009125: version NP_009125.1, which encodes for the amino acid sequence: MSRESDVEAQQSHGSSACSQPHGSVTQSQGSSSQSQGISSSSTSTMPNSSQSSHSSSGTLSSLETVSTQELYSIPEDQEPEDQEPEEPTPAPWARLWALQDGFANLECVNDNYWFGRDKSCEYCFDEPLLKRTDKYRTYSKKHFRIFREVGPKNSYIAYIEDHSGNGTFVNTELVGKGKRRPLNNNSEIALSLSRNKVFVFFDLTVDDQSVYPKALRDEYIMSKTLGSGACGEVKLAFERKTCKKVAIKIISKRKFAIGSAREADPALNVETEIEILKKLNHPCIIKIKNFFDAEDYYIVLELMEGGELFDKVVGNKRLKEATCKLYFYQMLLAVQYLHENGIIHRDLKPENVLLSSQEEDCLIKITDFGHSKILGETSLMRTLCGTPTYLAPEVLVSVGTAGYNRAVDCWSLGVILFICLSGYPPFSEHRTQVSLKDQITSGKYNFIPEVWAEVSEKALDLVKKLLVVDPKARFTTEEALRHPWLQDEDMKRKFQDLLSEENESTALPQVLAQPSTSRKRPREGEAEGAETTKRPAVCAAVL. The NP_009125.1 sequence was used for computer assisted modeling to evaluate the differences between the various X-ray structures for the composite full-length CHK2 protein (no omissions in structure) versus the variant. Monte Carlo simulations were performed on the mutant to allow local regional changes for full-length 543 amino acids and when the p.E161del variant was introduced.
Using Monte Carlo simulations, we were able to refine the multitude of X-ray structures (all partially missing fragments, side chains, loops or rotamers) to generate a composite full-length model for studies with simulations using our previous work with the Yasara SSP/PSSM method.31–36 The structure was relaxed to the YASARA/Amber force field using knowledge-based potentials within Yasara. The side chains and rotamers were adjusted with knowledge-based potentials, simulated annealing with explicit solvent, and small equilibration simulations using multiple parallel Yasara refinement protocols.37 Both the entire full-length structure and VUS structure were modeled, filling in any gaps or unresolved portions from the X-ray.
Refinement of the finalized model was completed using either Schrodinger's LC-MOD Monte Carlo-based module or NAMD2 protocols. All refinements started with YASARA generated initial refinement and VUS p.E161del or the wild-type.31–33,35 The superposition and subsequent refinement of the overlapping regions yields a complete model for VUS p.E161del or the wild type. The final structures were subjected to energy optimization with the PR conjugate gradient with an R-dependent dielectric to ensure that the stereochemistry was in strict adherence to the force field.
Atom consistency was checked for all 543 amino acids (7757 atoms), or for the dimeric complex (15
514 atoms), of the p.E161del model and 161 amino acids (1670 atoms) for the truncation variant (or 3340 atoms for the dimeric fragment), verifying correctness of chain name, dihedrals, angles, torsions, non-bonds, electrostatics, atom-typing, and parameters (ESI†). Each model was exported into user-ready formats: Maestro (MAE) and YASARA (PDB) for easy viewing. Model manipulation was completed with Maestro (Macromodel, version 9.8, Schrodinger, LLC, New York, NY, 2017), or Visual Molecular Dynamics (VMD1.92).38
Monte Carlo dynamics searching was completed on each model for conformational sampling, using methods previously described in the literature.12–14,19 Briefly, each CHEK2 or CHEK2 VUS was minimized with relaxed restraints using either Steepest Descent or Conjugate Gradient PR, and then allowed to undergo the MC search criteria, as previously demonstrated.12–14,19 The primary purpose of MC, in this scenario, is examining any conformational variability that may occur with different mutations, structural change, or truncations, in or about the region near the site and the possible effect on partner proteins’ complementary binding disruption with CHK2.
Disclosures
None.
Contribution statement
All authors made substantial contributions to the conception or design of the work; or the acquisition, analysis, or interpretation of data for the work AND drafting of the work or revising it critically for important intellectual consent AND gave final approval of the version to be published AND agreed to be accountable for all aspects of the work in ensuring that questions related to the accuracy or integrity of any part of the work are appropriately investigated and resolved.
Funding
Funding was provided by the Center for Individualized Medicine, Mayo Clinic, which provided funds to conduct the molecular modeling.
Consent for publication and informed consent
All procedures followed were in accordance with the ethical standards of the responsible committee on human experimentation (institutional and national) and with the Helsinki Declaration of 1975, as revised in 2000 (5). Informed consent was obtained from all patients for being included in the study.
Availability of data and materials
Datasets and materials are detailed in the manuscript.
Conflicts of interest
The authors report that there are no conflicts of interest in the manuscript.
References
- M. B. Daly, R. Pilarski, M. Berry, S. S. Buys, M. Farmer, S. Friedman, J. E. Garber, N. D. Kauff, S. Khan, C. Klein, W. Kohlmann, A. Kurian, J. K. Litton, L. Madlensky, S. D. Merajver, K. Offit, T. Pal, G. Reiser, K. M. Shannon, E. Swisher, S. Vinayak, N. C. Voian, J. N. Weitzel, M. J. Wick, G. L. Wiesner, M. Dwyer and S. Darlow, J. Natl. Compr. Cancer Network, 2017, 15, 9–20 CrossRef CAS
.
- S. Richards, N. Aziz, S. Bale, D. Bick, S. Das, J. Gastier-Foster, W. W. Grody, M. Hegde, E. Lyon, E. Spector, K. Voelkerding, H. L. Rehm and A. L. Q. A. Committee, Genet. Med., 2015, 17, 405–424 CrossRef
.
- L. M. Amendola, G. P. Jarvik, M. C. Leo, H. M. McLaughlin, Y. Akkari, M. D. Amaral, J. S. Berg, S. Biswas, K. M. Bowling, L. K. Conlin, G. M. Cooper, M. O. Dorschner, M. C. Dulik, A. A. Ghazani, R. Ghosh, R. C. Green, R. Hart, C. Horton, J. J. Johnston, M. S. Lebo, A. Milosavljevic, J. Ou, C. M. Pak, R. Y. Patel, S. Punj, C. S. Richards, J. Salama, N. T. Strande, Y. Yang, S. E. Plon, L. G. Biesecker and H. L. Rehm, Am. J. Hum. Genet., 2016, 98, 1067–1076 CrossRef CAS PubMed
.
- J. Balmana, L. Digiovanni, P. Gaddam, M. F. Walsh, V. Joseph, Z. K. Stadler, K. L. Nathanson, J. E. Garber, F. J. Couch, K. Offit, M. E. Robson and S. M. Domchek, J. Clin. Oncol., 2016, 34, 4071–4078 CrossRef CAS PubMed
.
- D. M. Eccles, G. Mitchell, A. N. Monteiro, R. Schmutzler, F. J. Couch, A. B. Spurdle, E. B. Gomez-Garcia and E. C. W. Group, Ann. Oncol., 2015, 26, 2057–2065 CrossRef CAS PubMed
.
- K. A. Cronin, A. J. Lake, S. Scott, R. L. Sherman, A. M. Noone, N. Howlader, S. J. Henley, R. N. Anderson, A. U. Firth, J. Ma, B. A. Kohler and A. Jemal, Cancer, 2018 DOI:10.1002/cncr.31551
.
- P. Apostolou and I. Papasotiriou, Breast Cancer, 2017, 9, 331–335 CAS
.
- C. Cybulski, D. Wokołorczyk, A. Jakubowska, T. Huzarski, T. Byrski, J. Gronwald, B. Masojć, T. Deebniak, B. Górski, P. Blecharz, S. A. Narod and J. Lubiński, J. Clin. Oncol., 2011, 29(28), 3747–3752 CrossRef CAS
.
- D. Provenzale, S. Gupta, D. J. Ahnen, T. Bray, J. A. Cannon, G. Cooper, D. S. David, D. S. Early, D. Erwin, J. M. Ford, F. M. Giardiello, W. Grady, A. L. Halverson, S. R. Hamilton, H. Hampel, M. K. Ismail, J. B. Klapman, D. W. Larson, A. J. Lazenby, P. M. Lynch, R. J. Mayer, R. M. Ness, S. E. Regenbogen, N. J. Samadder, M. Shike, G. Steinbach, D. Weinberg, M. Dwyer and S. Darlow, J. Natl. Compr. Cancer Network, 2016, 14(8), 1010–1030 CrossRef CAS
.
- A. Desrichard, Y. Bidet, N. Uhrhammer and Y. J. Bignon, Breast Cancer Res., 2011, 13, R119 CrossRef CAS PubMed
.
- N. Sodha, T. S. Mantoni, S. V. Tavtigian, R. Eeles and M. D. Garrett, Cancer Res., 2006, 66, 8966–8970 CrossRef CAS PubMed
.
- T. Caulfield and B. Devkota, Proteins, 2012, 80, 2489–2500 CrossRef CAS PubMed
.
- T. Caulfield and J. L. Medina-Franco, J. Struct. Biol., 2011, 176, 185–191 CrossRef CAS PubMed
.
- T. R. Caulfield, J. Mol. Graphics Modell., 2011, 29, 1006–1014 CrossRef CAS PubMed
.
- F. Lopez-Vallejo, T. Caulfield, K. Martinez-Mayorga, M. A. Giulianotti, A. Nefzi, R. A. Houghten and J. L. Medina-Franco, Comb. Chem. High Throughput Screening, 2011, 14, 475–487 CrossRef CAS PubMed
.
- J. Reumers, J. Schymkowitz, J. Ferkinghoff-Borg, F. Stricher, L. Serrano and F. Rousseau, Nucleic Acids Res., 2005, 33, D527–532 CrossRef CAS
.
- J. W. Schymkowitz, F. Rousseau, I. C. Martins, J. Ferkinghoff-Borg, F. Stricher and L. Serrano, Proc. Natl. Acad. Sci. U. S. A., 2005, 102, 10147–10152 CrossRef CAS PubMed
.
- Y. J. Zhang, T. Caulfield, Y. F. Xu, T. F. Gendron, J. Hubbard, C. Stetler, H. Sasaguri, E. C. Whitelaw, S. Cai, W. C. Lee and L. Petrucelli, Hum. Mol. Genet., 2013, 22, 3112–3122 CrossRef CAS PubMed
.
- T. R. Caulfield, B. Devkota and G. C. Rollins, J. Biophys., 2011, 219515 Search PubMed
.
- S. O. Abdul-Hay, A. L. Lane, T. R. Caulfield, C. Claussin, J. Bertrand, A. Masson, S. Choudhry, A. H. Fauq, G. M. Maharvi and M. A. Leissring, J. Med. Chem., 2013, 56, 2246–2255 CrossRef CAS
.
- M. Ando, F. C. Fiesel, R. Hudec, T. R. Caulfield, K. Ogaki, P. Gorka-Skoczylas, D. Koziorowski, A. Friedman, L. Chen, V. L. Dawson, T. M. Dawson, G. Bu, O. A. Ross, Z. K. Wszolek and W. Springer, Mol. Neurodegener., 2017, 12, 32 CrossRef PubMed
.
- T. R. Caulfield, F. C. Fiesel, E. L. Moussaud-Lamodiere, D. F. Dourado, S. C. Flores and W. Springer, PLoS Comput. Biol., 2014, 10, e1003935 CrossRef PubMed
.
- T. R. Caulfield, F. C. Fiesel and W. Springer, Biochem. Soc. Trans., 2015, 43, 269–274 CrossRef CAS PubMed
.
- F. C. Fiesel, M. Ando, R. Hudec, A. R. Hill, M. Castanedes-Casey, T. R. Caulfield, E. L. Moussaud-Lamodiere, J. N. Stankowski, P. O. Bauer, O. Lorenzo-Betancor, I. Ferrer, J. M. Arbelo, J. Siuda, L. Chen, V. L. Dawson, T. M. Dawson, Z. K. Wszolek, O. A. Ross, D. W. Dickson and W. Springer, EMBO Rep., 2015, 16, 1114–1130 CrossRef CAS
.
- F. C. Fiesel, T. R. Caulfield, E. L. Moussaud-Lamodiere, K. Ogaki, D. F. Dourado, S. C. Flores, O. A. Ross and W. Springer, Hum. Mutat., 2015, 36, 774–786 CrossRef CAS PubMed
.
- A. Puschmann, F. C. Fiesel, T. R. Caulfield, R. Hudec, M. Ando, D. Truban, X. Hou, K. Ogaki, M. G. Heckman, E. D. James, M. Swanberg, I. Jimenez-Ferrer, O. Hansson, G. Opala, J. Siuda, M. Boczarska-Jedynak, A. Friedman, D. Koziorowski, J. O. Aasly, T. Lynch, G. D. Mellick, M. Mohan, P. A. Silburn, Y. Sanotsky, C. Vilarino-Guell, M. J. Farrer, L. Chen, V. L. Dawson, T. M. Dawson, Z. K. Wszolek, O. A. Ross and W. Springer, Brain, 2017, 140, 98–117 CrossRef PubMed
.
- C. A. von Roemeling, T. R. Caulfield, L. Marlow, I. Bok, J. Wen, J. L. Miller, R. Hughes, L. Hazlehurst, A. B. Pinkerton, D. C. Radisky, H. W. Tun, Y. S. B. Kim, A. L. Lane and J. A. Copland, Oncotarget, 2018, 9, 3–20 CrossRef
.
- A. L. Harris, P. R. Blackburn, J. E. Richter, Jr., J. M. Gass, T. R. Caulfield, A. N. Mohammad and P. S. Atwal, Case Rep. Genet., 2018, 6968395 Search PubMed
.
- T. R. Caulfield, J. E. Richter, Jr., E. E. Brown, A. N. Mohammad, D. P. Judge and P. S. Atwal, Mol. Genet. Genomic Med., 2018 DOI:10.1002/mgg3.401
.
- J. E. Richter, H. G. Robles, E. Mauricio, A. Mohammad, P. S. Atwal and T. R. Caulfield, Hum. Genome Var., 2018, 5, 18016 CrossRef CAS PubMed
.
- S. F. Altschul, T. L. Madden, A. A. Schaffer, J. Zhang, Z. Zhang, W. Miller and D. J. Lipman, Nucleic Acids Res., 1997, 25, 3389–3402 CrossRef CAS
.
- R. W. Hooft, C. Sander, M. Scharf and G. Vriend, CABIOS, Comput. Appl. Biosci., 1996, 12, 525–529 CAS
.
- R. W. Hooft, G. Vriend, C. Sander and E. E. Abola, Nature, 1996, 381, 272 CrossRef CAS PubMed
.
- R. D. King and M. J. Sternberg, Protein science: a publication of the Protein Society, 1996, 5, 2298–2310 CrossRef CAS PubMed
.
- E. Krieger, K. Joo, J. Lee, J. Lee, S. Raman, J. Thompson, M. Tyka, D. Baker and K. Karplus, Proteins, 2009, 77(Suppl. 9), 114–122 CrossRef CAS
.
- J. Qiu and R. Elber, Proteins, 2006, 62, 881–891 CrossRef CAS PubMed
.
- M. M. Albá, R. A. Laskowski, D. S. Moss and J. M. Thornton, J. Appl. Crystallogr., 1993, 283–291 Search PubMed
.
- W. Humphrey, A. Dalke and K. Schulten, J. Mol. Graphics, 1996, 14(33–38), 27–38 Search PubMed
.
Footnote |
† Electronic supplementary information (ESI) available from the author on request: Structural modelling information provided for generation of structures for full-length (no omission of missing side chains, amino acids, or loops) complete human sequence for the wild type and variant. X-ray structures have various missing segments contained, whilst this composite structure is complete. |
|
This journal is © The Royal Society of Chemistry 2019 |