DOI:
10.1039/C8MO00139A
(Research Article)
Mol. Omics, 2019,
15, 77-87
Investigating the intracellular effects of hyperbranched polycation–DNA complexes on lung cancer cells using LC-MS-based metabolite profiling†
Received
20th June 2018
, Accepted 3rd December 2018
First published on 1st February 2019
Abstract
Cationic polymers have emerged as a promising alternative to viral vectors in gene therapy. They are cheap to scale up, easy to functionalise and are potentially safer than viral vectors, however many are cytotoxic. The large number of polycations, designed to address the toxicity problem, raises a practical need to develop a fast and reliable method for assessing the safety of these materials. In this regard, metabolomics provides a detailed and comprehensive method that can assess the potential toxicity at the cellular and molecular level. Here, we applied metabolomics to investigate the impact of hyperbranched polylysine, hyperbranched polylysine-co-histidine and branched polyethyleneimine polyplexes at sub-toxic concentrations on the metabolic pathways of A459 and H1299 lung carcinoma cell lines. The study revealed that the polyplexes downregulated metabolites associated with glycolysis and the TCA cycle, and induced oxidative stress in both cell lines. The relative changes of the metabolites indicated that the polyplexes of polyethyleneimine and hyperbranched polylysine affected the metabolism much more than the polyplexes of hyperbranched polylysine-co-histidine. This was in line with transfection results, suggesting a correlation between the toxicity and transfection efficiency of these polyplexes. Our work highlights the importance of the metabolomics approach not just to assess the potential toxicity of polyplexes but also to understand the molecular mechanisms underlying any adverse effects, which could help in designing more efficient vectors.
Introduction
Gene therapy is a promising approach for addressing both hereditary and acquired diseases. However, the clinical applications of this approach are still limited because of the difficulties in delivery of therapeutic nucleic acids.1–4 Viral vectors, although they are efficient, have serious safety issues and technical drawbacks, which have led to more attention being focused on non-viral carriers.2,5–7 In this context, cationic polymers have emerged as attractive gene delivery systems; they are easy to functionalise and cheap to scale up, however many of them are cytotoxic.8–12
Over the last two decades, a large number of cationic polymers have been designed and investigated to develop efficient gene vectors with especial emphasis on toxicity issues.13–15 However, the toxicity of these polymers has been routinely evaluated in in vitro cell culture based on simple cell viability assays, such as MTT and LDH.16,17 These methods, although they are fast and appropriate for routine toxicity assessment, have several limitations and do not provide sufficient information on potential mechanisms of cell toxicity to inform the design of new polymeric materials.18 Also, these assays give indications of the crude toxicity of materials but not the safety of these materials at sub toxic concentrations. In some cases, mechanistic studies have been applied to understand the toxic effects of cationic polymers and their complexes with nucleic acids,19–21 however, these studies have generally relied on limited conventional toxicity assays which are expensive and time consuming. Hence, there is a practical incentive to establish comprehensive and reliable toxicity assessment methods that can be applied to evaluate the safety of drug delivery materials in general.22 In this regard, metabolomics can be considered as a promising tool that could fulfil the requirements for in depth nanotoxicology investigations. This approach traces the changes in metabolite levels present in biological samples in response to external stimuli and can afford deep understanding of the molecular mechanisms of toxicity.22,23 Metabolomics provides qualitative and quantitative measurements of small biomolecules like sugars, amino acids, nucleotides, etc., which are the end products of genetic translation processes. The very large number of detected metabolites in these types of analysis provides a comprehensive view about the intracellular functions and can create a chemical map of the affected pathways in the cells, which reflects the impact of a xenobiotic, such as an applied cationic polymer, and its potential toxicity.24,25
Polylysine, PEI and their derivatives are among the most studied cationic polymers for gene delivery. Apart from the variation in their transfection efficiency, several studies indicated that they share some similar manifestations of cytotoxicity.19,20 They interact with, and induce plasma membrane damage in, the early stage of exposure, and can provoke programed cellular death (apoptosis) through destabilisation of mitochondrial membranes and release of cytochrome C, as a late stage of toxicity.26 Furthermore, it has been reported that the cytotoxicity of these polycations is highly related to their architectures.21,27 Considering that the branched forms of polylysine and PEI showed a better transfection efficiency in comparison with the linear forms, they would be interesting candidates for metabolomics studies. Also, thermally polymerised hyperbranched polymers have attracted our interest due to their advantages over dendritic polymers in terms of applicability and feasibility for manufacturing and scale up, and because the impact of these polymers and their polyplexes at the cellular and molecular level is not fully understood.27,28 In the present work, a global metabolic profiling approach was employed to study the effect of hyperbranched polylysine (hb-polyK), hyperbranched polylysine-co-histidine (hb-polyKH2) and branched polyethyleneimine (PEI) polyplexes with plasmid DNA on two lung carcinoma cell lines (A549 and H1299). These two cell lines were chosen as they are initial targets for local administration and inhalation in cancer therapy. In addition, these cell lines showed unexpected variations in their responses to gene delivery complexes in terms of uptake and transfection,28 thus an evaluation of their metabolomics profiles was expected to enhance our understanding of the intracellular behaviour of the polyplexes and their potential toxicity at sub-toxic concentrations.
Materials and methods
Materials
Hyperbranched polylysine, hyperbranched polylysine-co-histidine, and their FITC-labelled forms were prepared and characterised as described previously.28 Branched polyethyleneimine (25 kDa), methylthiazolyldiphenyl-tetrazolium bromide (MTT), the annexin V-FITC/PI apoptosis detection kit, N-acetylcysteine (NAC) and all buffers were purchased from Sigma-Aldrich. The Cytotoxicity Detection KitPLUS (for LDH) was obtained from Roche. Luciferase reporter plasmid (gWiz-Luc) from Aldevron and the Luciferase assay kit were purchased from Promega Corporation. The Label IT® Cy™3 Labeling Kit was from Mirus. All routine cell culture materials were purchased from Sigma-Aldrich. Fisher Scientific provided Trypan blue 0.4% and all HPLC/LC-MS grade solvents.
Preparation of polyplexes
The complexes of the polymers with plasmid DNA were prepared in 10 mM HEPES buffer of pH 7.4. Aliquots of similar volumes and required concentrations, to prepare the desired N/P ratios, of polymers and plasmid DNA solutions were mixed and vortexed for 10 s. Then, the polyplexes were left for 30 min before being used in the experiments. The N/P ratios, the charge ratio, were calculated based on the results of a fluorescamine assay and all the physicochemical characterisations were as reported in our previous work.28
Cell culture
A549 (human adenocarcinomic alveolar basal epithelial, ATCC® CCL-185™) and H1299 (human non-small cell lung carcinoma, ATCC® CRL-5803™) cells were cultured in DMEM and RPMI media respectively, supplemented with 10% FBS and 1% L-glutamic acid, in a humidified atmosphere containing 5% CO2. The cells were used at a passage number between 30 and 40.
Metabolic activity (MTT) assay
In 100 μl of fully supplemented medium, A549 and H1299 cells were seeded on 96-well plates at a density of 25 × 103 cells per cm2. After 24 hours, the cells were washed with PBS and treated with polyplexes (at different N/P ratios) suspended in 100 μl of FBS free medium to a concentration of plasmid of 2 μg ml−1 for 4 hours. Then, the culture media were replaced with fresh fully supplemented media and the cells were incubated for an additional 24 hours. 25 μl per well of MTT reagent (5 mg ml−1 in PBS) was directly added to the cells and incubated for 3 hours. Thereafter, the cells were washed with PBS, 150 μl of DMSO was added and the metabolic activity was determined by measuring the absorbance at 570 nm using cells treated with FBS free media and Triton 0.2% as a positive and negative control respectively. The following equation was used to calculate the percentage of metabolic activity:
Metabolic activity (%) = S–T/C–T × 100 |
where S is the absorbance obtained with the tested samples, T is the absorbance observed with Triton, and C is the absorbance observed with the FBS free media treated cells.
Lactate dehydrogenase (LDH) detection assay
On 96-well plates, A549 and H1299 cells were seeded at a density of 25 × 103 cell per cm2 and cultured in 100 μl of media for 24 hours. Then, the cells were washed, treated with polyplexes at different N/P ratios (final concentration of plasmid DNA was 2 μg ml−1 in FBS-free media) and incubated for 4 hours. After that, 50 μl aliquots of the treatment media were taken, mixed with 50 μl LDH reagent and allowed to react at room temperature for 20–30 minutes before adding the stop solution, 5 μl. The released LDH was detected by measuring the absorbance at 490 nm using cells treated with Triton 0.2% and FBS free medium as controls. The percentage of released LDH was calculated using the following equation:
Released LDH (%) = S–C/T–C × 100 |
where S is the absorbance obtained with the tested samples, T is the absorbance observed with Triton, and C is the absorbance observed with the FBS free media treated cells.
Annexin-V/PI apoptosis assay
On 24-well plates, A549 and H1299 cells were seeded at a density of 25 × 103 cell per cm2 and cultured in 500 μl of the fully supplemented medium for 24 hours. Then, the cells were washed, and incubated for 4 hours in FBS-free medium with the polyplexes at N/P ratios of 10 and concentration equivalent to 2 μg ml−1 of plasmid DNA. After the incubation period, the treatment medium was replaced with fully supplemented medium and the cells were incubated for a further 24 hours. Thereafter, the culture medium was collected into labelled FACS tubes on ice (4 °C), and the cells were detached (using trypsin/EDTA solution for 5 min), and collected together with their corresponding culture medium. The cells were centrifuged, re-suspended in 100 μl of 1× annexin V binding buffer, treated with 2.5 μl annexin-V FITC and 1 μl of 100 μg ml−1 PI solutions (from ThermoFisher), and incubated in the dark for 15 min. Then, the samples were diluted with 1× binding buffer to 500 μl and analysed using a Beckman Coulter FC-500 flow cytometer. The data were processed using Kaluza 1.5 software.
In vitro transfection study
Both cell lines were seeded on 24-well plates at a density of 25 × 103 cell per cm2 and incubated in 500 μl of fully supplemented medium for 24 hours. Then, the cells were washed with PBS, covered with 500 μl of FBS-free media, and treated with gWiz-Luc polyplexes of N/P ratio of 10 at a concentration of 2 μg ml−1 of plasmid. After 4 hours, the transfection medium was replaced with fully supplemented medium and the cells incubated for a further 24 hours. After that, the cells were washed with PBS and the expression of luciferase was detected by a luciferase assay kit. The effect of NAC on the transfection efficiency was studied by adding NAC at a concentration of 5 mM into the culture medium before for 1 hour and after the treatment with the polyplexes for 24 hour, while keeping the polyplexes free from NAC during the treatment period.
Cellular uptake study
Polyplexes were prepared using FITC-labelled polymers and Cy3-labelled plasmid DNA to study the cellular uptake and internalisation. For confocal microscopy, A549 and H1299 cells were seeded into 8-well chamber slides (obtain from Ibidi) at a seeding density of 1 × 104 cell per cm2 in 300 μl of fully supplemented medium. After 24 hours, the cells were washed with PBS, covered with 300 μl of FBS-free media, and treated with labelled polyplexes with an N/P ratio of 10 at a concentration of 2 μg ml−1 of DNA for 4 hours. Then, the cells were washed with PBS three times, fixed with 4% paraformaldehyde for 15 minutes, stained with DRAQ5 (20 μM in PBS for 5 minutes), and covered with mounting medium. In the case of flow cytometry, the cells were prepared under conditions similar to the transfection experiment. After incubation of the cells with labelled polyplexes for 4 hours, the cells were washed with PBS three times, and detached from the plates using trypsin solution for 5 minutes at 37 °C. Then, the cells were collected, centrifuged, and re-suspended in 0.3 ml of PBS in FACS tubes. Thereafter, the samples were mixed with 150 μl of 0.4% trypan blue and measured using an Astrios flow cytometer from Beckman Coulter Life Sciences.
Metabolomic analysis
Incubation of polyplexes with cells and metabolite extraction.
The assay times and cell treatments with the polyplexes were based on those shown to be efficient for transgene expression in our previous experiments with polymer/DNA complexes in A54 and H1299 cells. Both cell lines were seeded on T25 flasks (6 replicate for each condition) at a density of 25 × 103 cell per cm2 and incubated in 5 ml of fully supplemented medium for 24 hours. Then, the cells were washed with PBS, covered with 5 ml of serum free medium, and treated with polyplexes of N/P ratio of 10 at a concentration of 2 μg ml−1 of plasmid DNA. After 4 hours, aliquots were taken from the transfection medium and the medium replaced with fully supplemented medium. The cells were incubated for a further 24 hours and aliquots from the medium were taken. Then, after removing the medium, the cells were washed briefly once with pre-warmed PBS at 37 °C. The cellular metabolism was quenched and the metabolites were extracted simultaneously by adding 0.5 ml of methanol (−48 °C), and cell handling after quenching was performed on ice. The cells were then scraped and transferred to precooled fresh tubes at 4 °C. The cell solution was vortexed vigorously for 1 h at 4 °C and centrifuged at 17
000 × g for 10 min at 4 °C. After the centrifugation, the supernatant was removed and dried under vacuum at room temperature. The metabolite extract was reconstituted using 70 μl of pre-cooled methanol (4 °C). 10 μl aliquots were taken from each sample to make a pooled QC in order to assess instrument performance.29
Liquid chromatography-mass spectrometry (LC-MS).
LC was performed on a ZIC-pHILIC 5 μm, 4.6 × 150 mm column from Merck Sequant (Watford, UK), using a Accela LC system with a mobile phase consisting of A: 20 mM ammonium carbonate and B: 100% acetonitrile as previously described in ref. 30 and 31. Compounds were separated by the following linear gradient: 20% A (0 min) to 95% A at 15 min to 20% A at 17 min and held to 24 min. The flow rate was 300 μl min−1 and the injection volume was 10 μl. Samples were maintained at 4 °C, and the column was maintained at 45 °C.
MS was performed on an Orbitrap Exactive MS (Thermo Fisher Scientific, Hemel Hempstead, UK) with ESI running in positive and negative ionisation modes. Briefly, spectra were acquired in full MS scan in the range of m/z 70–1400 and the resolution was 50
000 in both modes. The capillary and probe temperatures were maintained at 275 and 150 °C, respectively. The instrument calibration was performed by modified Thermo calibration mixture masses with inclusion of C2H6NO2 (m/z 76.0393) for positive ion electrospray ionisation and C3H5O3 (m/z 89.0244) for negative ion electrospray ionisation in order to extend the calibration mass range to small metabolites.
Data analysis and metabolite identification.
Raw LC-MS data from the control group (untreated cells), the treatment groups (cells exposed to the different NPs), and reagent blanks were acquired using Xcalibur v2.1 software (Thermo Scientific, Hemel Hemstead UK), and processed with XCMS for untargeted peak-picking.32 Peak matching and related peak annotation were performed using mzMatch33 and noise filtering and putative metabolite identification were then carried out using IDEOM with the default parameters.34 Metabolites that were matched with accurate masses and retention times of authentic standards were identified with Level 1 metabolite identification according to the metabolomics standards initiative,35,36 but when standards were not available, metabolites were identified by employing predicted retention times considered as putative (Level 2 identification). Pooled QC samples were injected randomly in between every 5–6 samples to validate system suitability and stability.29 Multivariate data analysis was employed to assess changes in the cell metabolome between the control and each treatment group using orthogonal partial least squares discriminant analysis (OPLS-DA) using SIMCA-P v13.0.2 (Umetrics, Umea, Sweden).37 In addition to the multivariate analysis, univariate one-way ANOVA was carried out using Metaboanalyst 3.0.38 Mass ions with false discovery rate (FDR) less than 5% and variable importance in projection scores (VIP) greater than one were selected as potential biomarkers. The lists of significantly altered metabolites were imported to Metaboanalyst 3.0 to visualise the affected metabolic pathways.39
Results and discussion
In this study, a metabolomics approach was adopted as a comprehensive and reliable method to assess the impact of the polyplexes of hb-polyK, hb-polyKH2 and PEI, and their potential toxicity at sub-toxic concentration, on A549 and H1299 cell lines. We chose chromatographic separation of metabolites followed by mass spectrometric analysis, as this is the most frequently used approach in metabolomics, especially via GC-MS and LC MS systems. NMR spectroscopic analysis has also been widely used in metabolomics as it is quantitative, reproducible, selective and more importantly does not need complicated sample preparation.40,41 However, current levels of sensitivity remain a limitation in comparison to mass spectrometric techniques. In addition, MS metabolic approaches have the advantage of high throughput and wide metabolite coverage.42 For example, GS-MS has high resolution and is able to retain small molecules that elute with the solvent front in LC approaches; nevertheless, sample derivatisation is needed for GS-MS and it is only applicable for thermally stable stationary phases and samples. Although the LC-MS approach has been reported to be less reproducible than GS-MS and NMR,43 its high sensitivity and the wide range of compounds that can be analysed by LC-MS, in addition to the non-destructive soft ionisation techniques in both polarities, has made LC-MS one of the most attractive approaches for metabolic profiling in recent years, hence our adoption of this method.
The polyplexes were prepared at the N/P ratio of 10, where they showed the best transfection efficiency as reported in our previous work.28 The size and zeta potential measurements indicated that the polymers efficiently condensed plasmid DNA into positively charged nanoparticles of around 150 nm in diameter and the AFM images confirmed the results (Fig. S1, ESI†). Regarding the uptake, confocal microscopy images showed that the polyplexes were efficiently internalised in both cell lines. Flow cytometry revealed that there was no statistically significant difference between the uptakes of all polyplexes in H1299 cells. Also, the extent of internalisation of hb-polyK and PEI polyplexes in A549 cells was similar, but the histidine-containing polymer hb-polyKH2 complexes with DNA were present in significantly fewer A549 cells. However, the median of florescence intensity was comparable for all the polyplexes in A549 cells (Fig. 1A and B). Conversely, the transfection experiments revealed that PEI achieved the best transfection efficiency in both cell lines in comparison with hb-polyK and hb-polyKH2. Also, in A549 cells, hb-polyK showed greater transfection efficiency than hb-polyKH2, while in H1299 the difference between them was not statistically significant (Fig. 1C). Considering the results of the uptake studies, it can be suggested that the variation of transfection, at least in the case of PEI, may have been related to the different intracellular processing of these polymers and their polyplexes. Accordingly, we aimed to investigate the metabolomics profiles for these polyplexes in the selected cell lines, as a means to understand better the pathways by which the cells processed the polyplexes during the transfection experiments.
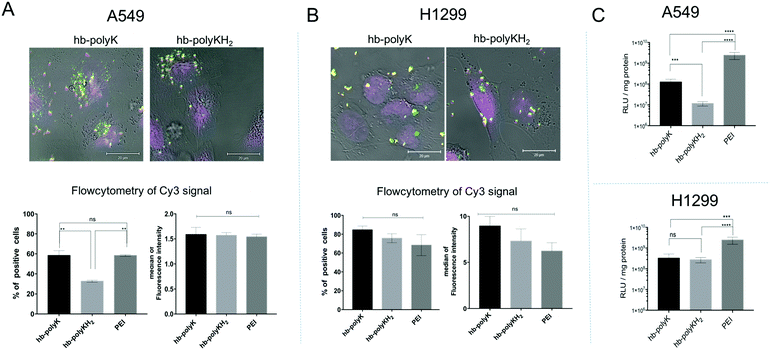 |
| Fig. 1 The uptake data in A549 cells (A) and in H1299 cells (B); presented by confocal images (more images in Fig. S2, ESI†) and flow cytometry data, where the flow cytometry data are reported as a percentage of positive cells and the median of the florescence intensity of the signal of the Cy3 labelled plasmid. (C) The transfection efficiency of the polyplexes with gWiz-Luc in A549 cells and in H1299 cells. The results represent mean and SD values (n = 4). The significance represents the difference in comparison with the controls, where ns = insignificant, * = significant (p < 0.05), ** = very significant (p < 0.01), *** = extremely significant (p < 0.001) and **** = extremely significant (p < 0.0001). | |
In metabolomics studies it is important to work at the sub-toxic level as the differences in viability between the control and treated cells would affect the reliability of the results. Therefore, MTT and LDH assays were used to investigate any toxicity resulting from the polyplexes (Fig. 2). These data show that the polyplexes of all the polymers were well tolerated by both cell lines at the selected concentration and N/P ratio. Signs of toxicity were seen, especially for PEI, at high N/P ratios (>20). It was previously reported that these polymers could provoke apoptosis as a late stage of toxicity;19,44 therefore the cells were further assessed using annexin-V/PI apoptosis assays to check if there were any apoptotic features on treatment with the polyplexes at the concentrations used in this study. The results (Fig. S3, ESI†) did not show any significant shift in the cells toward apoptosis and/or necrosis and confirmed that the selected concentrations of polyplexes were suitable for metabolomics study. We emphasise that the N/P ratio used in this study is the charge ratio and reflects the effective concentration of each polymer which achieved the best DNA condensation and delivery in our prior work.28 Accordingly, the 2 μg ml−1 of plasmid DNA, used to treat the cells at the N/P ratio of 10, corresponded to 0.012, 0.016 and 0.002 mg ml−1 of hb-polyK, hb-polyKH2 and PEI respectively. As expected, PEI was a more potent transfection agent than hb-polyK and hb-polyKH2, but it was also more cytotoxic.
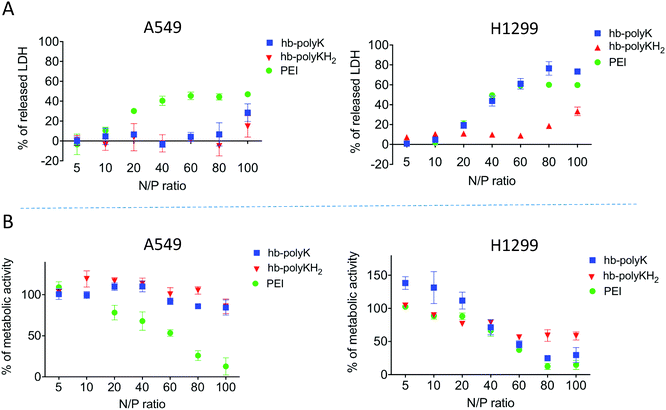 |
| Fig. 2 (A) LDH and (B) MTT assay of hb-polyK, hb-polyKH2 and PEI polyplexes at different N/P ratios in A549 and H1299 cell lines. The polyplexes were prepared in HEPES (10 mM, pH 7.4), diluted with FBS-free medium, and applied on the cells at a final concentration of 2 μg ml−1 of siRNA for 4 hours. The results are shown as mean and SE values of triplicate experiments (n = 4). | |
Multivariate analysis of LC-MS profiling data
A total of 482 and 421 metabolites were putatively identified in A459 and H1299 cells respectively, including amino acids, carbohydrates, nucleotides and lipids (Fig. 3A). In order to study the metabolic changes in A459 and H1299 cells after treatment with polyplexes of hb-polyK, hb-polyKH2 and PEI, extracts from treated and control cells from both cell lines were analysed by LC-MS. 3D data sets (m/z, retention time and peak intensity) were transformed into 2D XY output data (peak tables) by mzMatch to assign each mass ion with the corresponding exact mass and retention time. The CV was calculated for all the mass ions in the repeatedly injected QC pooled samples and it was less than 30% for more than 70% of the mass ions, indicating that the chromatography systems and mass spectroscopic analyses were stable throughout the whole run. OPLS-DA was implemented to visualise metabolic changes caused by the different polyplexes and to select potential biomarkers based on VIP values calculated based on the model. The six biological replicates for each sample group were clustered clearly in the OPLS-DA score plots (Fig. 3B), indicating reproducible data sets. OPLS-DA score plots also showed distinct separations between the different sample groups revealing that LC-MS-based metabolic profiling was capable of investigating different molecular effects of each group of polyplexes on the cell metabolomes. Mass ions of VIP score greater than 1 were selected as potential biomarkers. In addition to the multivariate analysis results, univariate ANOVA was performed to identify significantly changed compounds; mass ions with the false discovery rate ≤0.05 were selected as significant variables.45 Combining multi and univariate analyses revealed that 98 and 86 metabolites were altered significantly in A549 and H1299 cells respectively after the exposure to the different polyplexes, Tables S1 and S2, ESI.†
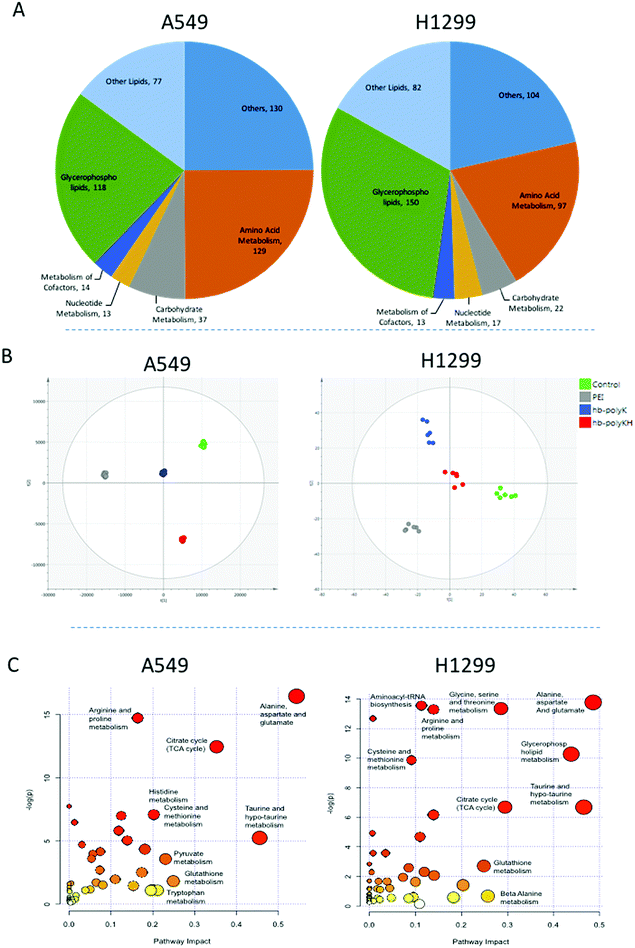 |
| Fig. 3 (A) Classification of the identified metabolites according to their biological role. (B) OPLS-DA score plots of A549 cell samples (R2X = 0.89, R2Y = 0.99, Q2 = 0.72, CV ANOVA p value = 3.1 × 10−4) and H1299 cell samples (R2X = 0.71, R2Y = 0.83 Q2 = 0.56, p value = 9.4 × 10−3) after four hours of treatment with polyplexes of hb-polyK, hb-polyKH2 and PEI (the control group with FBS-free medium), and then 20 hour of incubation without fully supplemented medium. (C) Summary of pathway analysis with MetaboAnalyst 4.0. The node colour is based on its p value and the node radius is determined based on their pathway impact values. | |
Disturbed metabolic pathways
The metabolomics approach revealed that the polyplexes affected several pathways in A459 and H1299 cells, including amino acid and nucleotide metabolism, citric acid cycle, and redox state; as shown in Fig. 3C. Although we cannot rule out effects of the delivered plasmid on all the metabolic changes detected, we specifically focused on metabolites previously linked to toxicity mechanisms of polymers as reported in the literature.19,26 Accordingly, we show the significantly changed and biologically relevant metabolites summarised in Table 1.
Table 1 The most biologically relevant metabolites that were changed significantly in A549 and H1299 cells after the treatment with polyplexes, IDC: metabolite identification level according to the metabolomics standards initiative: L1 – Level 1, L2 – Level 2. Colours in the table represent changes in metabolite levels with blue indicating a decrease and red denoting an increase
Mass |
RT |
Formula |
Putative metabolite |
ID confidence |
A549 |
H1299 |
PEI |
hb-polyK |
hb-polyKH2 |
PEI |
hb-polyK |
hb-polyKH2 |
TCA cycle |
146.0215 |
10.29 |
C5H6O5 |
2-Oxoglutarate |
L1 |
|
192.0270 |
11.56 |
C6H8O7 |
Citrate |
L2 |
134.0216 |
10.44 |
C4H6O5 |
(S)-Malate |
L1 |
174.0164 |
11.55 |
C6H6O6 |
cis-Aconitate |
L2 |
118.0267 |
10.35 |
C4H6O4 |
Succinate |
L1 |
|
Glycolysis |
88.0161 |
7.30 |
C3H4O3 |
Pyruvate |
L1 |
|
167.9823 |
11.28 |
C3H5O6P |
Phosphoenolpyruvate |
L1 |
185.9928 |
11.01 |
C3H7O7P |
3-Phospho-D-glycerate |
L2 |
|
Carnitine shuttle |
425.3505 |
5.23 |
C25H47NO4 |
Elaidiccarnitine |
L2 |
|
427.3662 |
5.18 |
C25H49NO4 |
Stearoylcarnitine |
L2 |
399.3349 |
5.3 |
C23H45NO4 |
Palmitoylcarnitine |
L2 |
161.1052 |
9.84 |
C7H15NO3 |
L-Carnitine |
L1 |
|
Redox homeostasis and apoptosis |
147.0532 |
7.44 |
C5H9NO4 |
O-Acetyl-L-serine |
L2 |
|
161.0688 |
7.41 |
C6H11NO4 |
O-Acetyl-L-homoserine |
L2 |
219.0742 |
9.4 |
C8H13NO6 |
O-Succinyl-L-homoserine |
L2 |
183.0661 |
10.09 |
C5H14NO4P |
Choline phosphate |
L1 |
103.0997 |
10.23 |
C5H13NO |
Choline |
L2 |
125.0147 |
10.70 |
C2H7NO3S |
Taurine |
L1 |
307.0838 |
9.82 |
C10H17N3O6S |
Glutathione |
L1 |
147.0532 |
5.54 |
C11H12N2O2 |
L-Glutamate |
L1 |
|
Kynurenine metabolism |
204.0899 |
9.56 |
C10H12N2O3 |
L-Tryptophan |
L1 |
|
208.0848 |
9.05 |
C11H12N2O4 |
L-Kynurenine |
L1 |
236.0797 |
8.38 |
C8H7NO3 |
L-Formylkynurenine |
L2 |
165.0426 |
4.89 |
C7H7NO2 |
Formylanthranilate |
L2 |
137.0476 |
7.35 |
C7H7NO2 |
Anthranilate |
L2 |
First of all, the fold changes in metabolite levels (as presented in the ‘heat map’ of Table 1) show that hb-polyKH2 had the least impact on cellular metabolism, which in turn may indicate reduced toxicity of this polymer in comparison to PEI and hb-polyK. Although the metabolic changes could, in principle, be connected to the lower uptake of hb-polyKH2 in A549 cells, they were more likely attributed to the different intracellular behaviour of this polymer in H1299 cells, as the cellular uptake results were comparable for all polyplexes. This is possibly due to the low ability of hb-polyKH2 to interact and permeabilise the cellular membrane as it is less flexible and has lower charge density in comparison with hb-polyK and PEI, an effect which is more pronounced in the H1299 cell line, as reported in our previous study.28 The reduced membrane disruption activity of the hb-polyKH2 can be noted in the LDH assay as well (Fig. 2). In this regard, several studies have suggested that there is a correlation between the toxicity of cationic polymers and their transfection efficiency, particularly PEI. These studies have considered the ability of cationic polymers to interact, destabilise, and permeabilise the biological membrane as a mechanism for transfection and toxicity as well. It has been suggested that this ability can enhance the uptake, endosomal escape and nuclear entry of polyplexes but also induce apoptotic events; mainly by interacting with the mitochondrial membrane.46–48 The early findings of metabolomics are in agreement with this suggestion, in that PEI, which achieved the best transfection, had the highest impact on the cellular metabolism. Furthermore, the metabolomics data suggest that the PEI and hb-polyK polyplexes altered the cellular energy metabolism of A459 and H1299 cells through glycolysis and the tricarboxylic acid (TCA) cycle. Usually, cancer cells predominantly use glycolysis for energy production rather than the TCA cycle, a phenomenon known as the Warburg effect.49 The metabolic changes, observed in this study, indicate that PEI and hb-polyK polyplexes inhibited glycolysis; the levels of three main glycolysis metabolites (pyruvate, phosphoenolpyruvate and 3-phospho-D-glycerate in A549 cells and only pyruvate and 3-phospho-D-glycerate in H1299 cells) were decreased. Similarly, PEI and hb-polyK polyplexes showed a down-regulated TCA cycle activity in both cell lines, which was manifested as a decrease in four main TCA cycle intermediates: 2-oxoglutarate, citrate, malate and cis-aconitate as shown in Table 1. PEI and hb-polyK have been reported previously to cause mitochondrial impairment by either directly interacting with the mitochondrial membrane or by inducing oxidative stress;44,50 in addition, it is known that mitochondrial impairment can result in TCA cycle dysfunction.51 The inhibition of glycolysis could have been a consequence of ATP decreases resulting from TCA dysfunction, because the early steps of glycolysis are ATP dependent. PEI and hb-polyK have been reported previously26 to interfere with cell membrane permeability/integrity and to induce LDH release outside the cell. This might be a detrimental factor because LDH is essential for glycolysis,50 however in this study, the concentrations of the polyplexes applied were below those causing a significant release of LDH (Fig. 2).
Interestingly, the energy metabolism of H1299 cells was shifted towards fatty acid oxidation, whereas a decrease in carnitine and increase in several acyl carnitines were noticed,52 as shown in Table 1. Upregulated fatty acid oxidation results in the accumulation of acetyl CoA, especially when the TCA cycle is malfunctioning, because there is not enough oxaloacetate to convert acetyl CoA to citrate. This will lead to increased production of acetoacetate and decreased intracellular pH, until ketoacidosis is reached,53 which might explain the higher susceptibility of H1299 cells towards the polyplexes in our study in comparison to A549 cells.
Cellular redox homeostasis is directly linked to energy metabolism, where glycolysis and the TCA cycle are two of the main sources of nicotinamide adenine dinucleotide reduced form (NADH). NADH is utilised inside the mitochondria to produce nicotinamide adenine dinucleotide phosphate (NADPH),54 which can restore any oxidized glutathione (GSSG) to its reduced state (GSH) through the action of glutathione reductase as the electron donor,55 thus affecting the redox homeostasis directly.56 The observed changes in metabolites also indicated that the amino acid metabolism and redox state of A459 and H1299 cells were altered. In particular, cysteine metabolism was one of the perturbed pathways reported by these experiments. Cysteine, alongside glutamic acid and glycine, is used to form GSH, which plays a key role in cellular redox balance. PEI and hb-polyK polyplexes resulted in a decrease in GSH and at least two of the three cysteine precursors in both cell lines (Table 1). These changes might indicate that the cells altered their synthesis pathways for GSH from amino acids due to a defect in GSH recycling from its oxidised form.57 A summarised metabolic pathway diagram of disturbed pathways in A549 and H1299 cells after the treatment with the different polyplexes is shown in Fig. 4, revealing alterations in energy metabolism and their consequences on redox homeostasis.
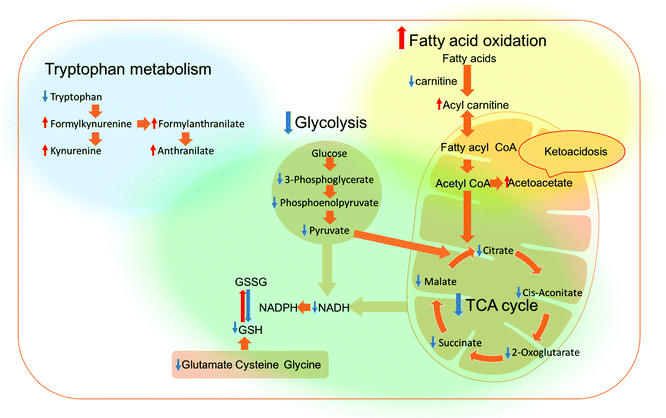 |
| Fig. 4 Overview of the affected pathways in the A549 and H1299 cells after treatment with the polyplexes. Red arrow = increased, blue arrow = decreased, blue region = altered pathways in A549 cells only, yellow region = altered pathways in H1299 cells only, and the green region = altered pathways in both cell lines. | |
Several studies have reported oxidative stress in cells following treatment with similar materials.50,58,59 For example, Moghimi et al. found that intracellular ROS were increased on treatment with branched PEI, suggesting that the mitochondrial destruction and glycolytic collapse caused by PEI treatment led to the impaired ability of cells to maintain the level of GSH and in turn the oxidative haemostasis. This study also reported that the presence of N-acetylcysteine (NAC), a precursor of GSH, improved the transfection of PEI polyplexes significantly.21
Therefore, in order to investigate the effect of oxidative stress on the transfection efficiency of the polyplexes, further transfection experiments were performed in the presence of NAC. However, as shown in Fig. 5, the transfection efficiency for all the polyplexes and in both cell lines was significantly decreased in the presence of NAC, These data implied that oxidative stress improved the transfection efficiency, in contrast with the results reported by Moghimi et al. However, it should be noted that in the prior study, the enhancement of transfection was in part due to an increase in cell viability in the presence of NAC due to a decrease in the toxic effect of ROS.21 In our work, sub-toxic concentrations of polyplexes/polymers were used and thus no improvement in cell viability would be expected in the presence of NAC. In addition, the transfection data in our case were normalised based on total protein measurement of the cells, which cancelled any differences related to the number of cells. Although a high level of ROS is toxic for cells, a sub-toxic level can act as a key modulator for the expression of several transcription factors, growth factors and cytokines,60 which in turn can either directly or indirectly affect transfection and transgene expression. Collectively our results alongside those from Moghimi et al. suggest that NAC either (a) improves the transfection when toxic levels of polyplexes (i.e. ROS) are used by improving cell viability or (b) decreases the transfection efficiency when sub-toxic concentrations of polyplexes are used, probably by counteracting the effects of sub-toxic levels of ROS.
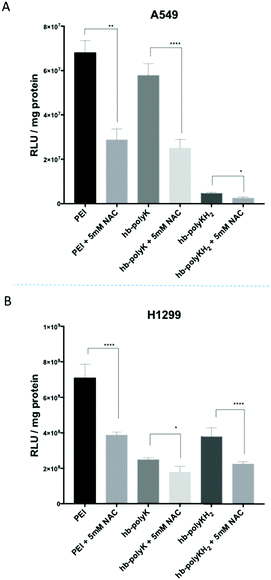 |
| Fig. 5 The transfection efficiency of polyplexes in the presence of 5 mM NAC in A549 and H1299 cells. The results represent mean and SD value (n = 4). The significance represents the difference in comparison with the controls, where * = significant (p < 0.05), ** = very significant (p < 0.01), and **** = extremely significant (p < 0.0001). | |
It has been reported also that the reduction of choline, taurine and GSH metabolites is associated with apoptosis.61,62 In this study, the levels of choline, taurine and GSH were significantly decreased in A549 cells upon treatment with PEI polyplexes, whereas all the other polyplexes caused decreases in GSH and taurine in H1299 cells. Choline increased in H1299 cells, due most probably to the increase in lipid metabolism in this cell line. These metabolites suggest that the polyplexes may promote apoptosis, which is in line with the reported toxicity for PEI and hb-polyK polyplexes.19,44 Interestingly, the changes in levels of choline, taurine and GSH metabolites were the least in A459 cells for hb-polyK and hb-polyKH2 polyplexes, indicating that this effect was more tolerated in A549 cells. This agrees with the results of metabolic activity assays of the cells (MTT), which showed that increasing the N/P ratio increased the toxicity of PEI polyplexes only in A549 but increased the toxicity of all the polyplexes in H1299 cells (Fig. 2).
Tryptophan metabolism was affected in A549 cells by the polyplexes. The level of tryptophan decreased overall, while four intermediates of the kynurenine pathway, L-formylkynurenine, formylanthranilate, L-kynurenine and anthranilate were increased. This implied that the tryptophan metabolism via indoleamine 2,3 dioxygenase (IDO), a first and rate-limiting step in the tryptophan catabolism along the kynurenine pathway, had been upregulated, most likely by pro-inflammatory cytokines including TNF α and interferon γ.63 This pathway is involved in different immune/inflammatory activities that are associated with several pathological conditions such as infection,64,65 which may have been an indicator that this effect had been caused in part by the delivered plasmid. However, Matz et al. reported a similar inflammatory response upon transfection with PEI polyplexes and found that this effect was unrelated to the origin of the promoter in the plasmid, whether cytomegalovirus or elongation factor-1 alpha.66 Interestingly, this inflammatory response was not detected in H1299 cells, an effect which can be correlated with the greater transgene expression observed in this cell line for all polyplexes in comparison with A549 cells (Fig. 1C).
Conclusions
In this study, branched polycationic polymers (hyperbranched polylysine (hb-polyK), hyperbranched polylysine-co-histidine (hb-polyKH2) and branched polyethyleneimine (PEI)), were used as polyplexes with DNA and were administered to A549 and H1299 cells and global metabolic profiling was performed. Sub toxic concentrations of the polyplexes were used, as determined by MTT and LDH assays. Metabolic profiling revealed that PEI and hb-polyK polyplexes down regulated metabolites associated with glycolysis and the TCA cycle, and induced oxidative stress in A549 cells. The suggested markers for apoptosis were reported only with PEI polyplexes in this cell line. On the other hand, analogous metabolic profiling in H1299 cells showed that PEI and hb-polyK polyplexes down regulated metabolites associated with glycolysis and the TCA cycle, and all the polyplexes shifted the energy metabolism toward fatty acid oxidation, and induced markers of oxidative stress and apoptosis. The lower extent of changes in metabolites suggests that the hb-polyKH2 polyplexes were the most tolerated polyplexes in this study, which might be due to the reduced ability of hb-polyKH2 to interact with and destabilise the cellular membrane, particularly in H1299 cells. The results also indicated that there was a correlation between the toxicity of these polyplexes and their transfection efficiency.
Finally, this study suggests that the metabolomics approach is a powerful safety assessment tool, as these assays were able to detect potential toxicity markers at sub-toxic concentrations where the traditional methods are insensitive. Also, this method provided insight into the molecular mechanism of toxicity and therapeutic effect alike, which might accelerate the design of more efficient and applicable delivery systems. Although metabolomics studies of delivery systems are still in their infancy, we believe that this approach is highly promising and in the future can enhance our understanding of the efficacy and safety of drug delivery materials.
Data access statement
All raw data created during this research are openly available from the corresponding author (http://cameron.alexander@nottingham.ac.uk) and at the University of Nottingham Research Data Management Repository (https://rdmc.nottingham.ac.uk/) and all analysed data supporting this study are provided in the ESI† accompanying this paper.
Conflicts of interest
There are no conflicts to declare.
Acknowledgements
This work was supported by the Engineering and Physical Sciences Research Council [grant numbers EP/H005625/1, EP/N03371X/1]; and the Royal Society [Wolfson Research Merit Award WM150086] (to CA) and the HCED-Iraq for funding (scholarship to AA). We also thank Tom Booth, Paul Cooling, Esme Ireson and Christine Grainger-Boultby for excellent technical support.
References
- Z. Meng, J. O'Keeffe-Ahern, J. Lyu, L. Pierucci, D. Zhou and W. Wang, Biomater. Sci., 2017, 5, 2381–2392 RSC.
- E. Keles, Y. Song, D. Du, W.-J. Dong and Y. Lin, Biomater. Sci., 2016, 4, 1291–1309 RSC.
- A. N. Zelikin, C. Ehrhardt and A. M. Healy, Nat. Chem., 2016, 8, 997–1007 CrossRef CAS PubMed.
- C. Scholz and E. Wagner, J. Controlled Release, 2012, 554–565 CrossRef CAS PubMed.
- H. Yin, R. L. Kanasty, A. A. Eltoukhy, A. J. Vegas, J. R. Dorkin and D. G. Anderson, Nat. Rev. Genet., 2014, 15, 541–555 CrossRef CAS PubMed.
- M. A. Islam, E. K. G. Reesor, Y. J. Xu, H. R. Zope, B. R. Zetter and J. J. Shi, Biomater. Sci., 2015, 3, 1519–1533 RSC.
- Y. Zhang, H. F. Chan and K. W. Leong, Adv. Drug Delivery Rev., 2013, 65, 104–120 CrossRef CAS PubMed.
- J. Chen, Z. Guo, H. Tian and X. Chen, Mol. Ther.–Methods Clin. Dev., 2016, 3, 16023 CrossRef PubMed.
- S. K. Samal, M. Dash, S. Van Vlierberghe, D. L. Kaplan, E. Chiellini, C. van Blitterswijk, L. Moroni and P. Dubruel, Chem. Soc. Rev., 2012, 41, 7147–7194 RSC.
- L. Kudsiova, K. Welser, F. Campbell, A. Mohammadi, N. Dawson, L. L. Cui, H. C. Hailes, M. J. Lawrence and A. B. Tabor, Mol. BioSyst., 2016, 12, 934–951 RSC.
- S. C. De Smedt, J. Demeester and W. E. Hennink, Pharm. Res., 2000, 17, 113–126 CrossRef CAS.
- D. Oupicky, C. Konak, K. Ulbrich, M. A. Wolfert and L. W. Seymour, J. Controlled Release, 2000, 65, 149–171 CrossRef CAS PubMed.
- R. Bansal, S. Tayal, K. C. Gupta and P. Kumar, Org. Biomol. Chem., 2015, 13, 3128–3135 RSC.
- T. Wang, J. R. Upponi and V. P. Torchilin, Int. J. Pharm., 2012, 427, 3–20 CrossRef CAS PubMed.
- M. Morille, C. Passirani, A. Vonarbourg, A. Clavreul and J. P. Benoit, Biomaterials, 2008, 29, 3477–3496 CrossRef CAS PubMed.
- W. W. Wang, M. Balk, Z. J. Deng, C. Wischke, M. Gossen, M. Behl, N. Ma and A. Lendlein, J. Controlled Release, 2016, 242, 71–79 CrossRef CAS PubMed.
- M. Breunig, U. Lungwitz, R. Liebl and A. Goepferich, Proc. Natl. Acad. Sci. U. S. A., 2007, 104, 14454–14459 CrossRef CAS PubMed.
- A. Dhawan and V. Sharma, Anal. Bioanal. Chem., 2010, 398, 589–605 CrossRef CAS PubMed.
- S. M. Moghimi, P. Symonds, J. C. Murray, A. C. Hunter, G. Debska and A. Szewczyk, Mol. Ther., 2005, 11, 990–995 CrossRef CAS PubMed.
- P. Symonds, J. C. Murray, A. C. Hunter, G. Debska, A. Szewczyk and S. M. Moghimi, FEBS Lett., 2005, 579, 6191–6198 CrossRef CAS PubMed.
- A. Hall, L. Parhamifar, M. K. Lange, K. D. Meyle, M. Sanderhoff, H. Andersen, M. Roursgaard, A. K. Larsen, P. B. Jensen, C. Christensen, J. Bartek and S. M. Moghimi, Biochim. Biophys. Acta, 2015, 1847, 328–342 CrossRef CAS PubMed.
- E. Caballero-Díaz and M. Valcárcel Cases, TrAC, Trends Anal. Chem., 2016, 84, 160–171 CrossRef.
- U. A. Meyer, U. M. Zanger and M. Schwab, Annu. Rev. Pharmacol. Toxicol., 2013, 53, 475–502 CrossRef CAS PubMed.
- D.-H. K. D. J. Creek, Bioanalysis, 2015, 7, 629–631 CrossRef PubMed.
- S. M. Huang, X. B. Zuo, J. J. Li, S. F. Y. Li, B. H. Bay and C. N. Ong, Adv. Healthcare Mater., 2012, 1, 779–784 CrossRef CAS PubMed.
- A. C. Hunter and S. M. Moghimi, Biochim. Biophys. Acta, 2010, 1797, 1203–1209 CrossRef CAS PubMed.
- Z. Kadlecova, Y. Rajendra, M. Matasci, L. Baldi, D. L. Hacker, F. M. Wurm and H. A. Klok, J. Controlled Release, 2013, 169, 276–288 CrossRef CAS PubMed.
- A. Alazzo, T. Lovato, H. Collins, V. Taresco, S. Stolnik, M. Soliman, K. Spriggs and C. Alexander, J. Interdiscip. Nanomed., 2018, 3, 38–54 CrossRef CAS.
- E. J. Want, I. D. Wilson, H. Gika, G. Theodoridis, R. S. Plumb, J. Shockcor, E. Holmes and J. K. Nicholson, Nat. Protoc., 2010, 5, 1005–1018 CrossRef CAS.
- D. J. Creek, A. Jankevics, R. Breitling, D. G. Watson, M. P. Barrett and K. E. Burgess, Anal. Chem., 2011, 83, 8703–8710 CrossRef CAS PubMed.
- A. Surrati, R. Linforth, I. D. Fisk, V. Sottile and D. H. Kim, Analyst, 2016, 141, 3776–3787 RSC.
- R. Tautenhahn, C. Böttcher and S. Neumann, BMC Bioinf., 2008, 9, 504 CrossRef PubMed.
- R. A. Scheltema, A. Jankevics, R. C. Jansen, M. A. Swertz and R. Breitling, Anal. Chem., 2011, 83, 2786–2793 CrossRef CAS PubMed.
- D. J. Creek, A. Jankevics, K. E. Burgess, R. Breitling and M. P. Barrett, Bioinformatics, 2012, 28, 1048–1049 CrossRef CAS PubMed.
- L. W. Sumner, A. Amberg, D. Barrett, M. H. Beale, R. Beger, C. A. Daykin, T. W. M. Fan, O. Fiehn, R. Goodacre, J. L. Griffin, T. Hankemeier, N. Hardy, J. Harnly, R. Higashi, J. Kopka, A. N. Lane, J. C. Lindon, P. Marriott, A. W. Nicholls, M. D. Reily, J. J. Thaden and M. R. Viant, Metabolomics, 2007, 3, 211–221 CrossRef CAS PubMed.
- L. W. Sumner, Z. Lei, B. J. Nikolau, K. Saito, U. Roessner and R. Trengove, Metabolomics, 2014, 10, 212–229 CrossRef.
- J. Boccard and D. N. Rutledge, Anal. Chim. Acta, 2013, 769, 30–39 CrossRef CAS PubMed.
- J. Xia, I. V. Sinelnikov, B. Han and D. S. Wishart, Nucleic Acids Res., 2015, 43, W251–W257 CrossRef CAS PubMed.
- M. Kanehisa, S. Goto, Y. Sato, M. Kawashima, M. Furumichi and M. Tanabe, Nucleic Acids Res., 2014, 42, D199–D205 CrossRef CAS PubMed.
- K. Contrepois, L. Jiang and M. Snyder, Mol. Cell. Proteomics, 2015, 14, 1684–1695 CrossRef CAS PubMed.
- A. Armiñán, M. Palomino-Schätzlein, C. Deladriere, J. J. Arroyo-Crespo, S. Vicente-Ruiz, M. J. Vicent and A. Pineda-Lucena, Biomaterials, 2018, 162, 144–153 CrossRef PubMed.
- Y. Wang, S. Liu, Y. Hu, P. Li and J.-B. Wan, RSC Adv., 2015, 5, 78728–78737 RSC.
- G. A. Nagana Gowda and D. Raftery, J. Magn. Reson., 2015, 260, 144–160 CrossRef CAS PubMed.
- Z. Kadlecova, L. Baldi, D. Hacker, F. M. Wurm and H. A. Klok, Biomacromolecules, 2012, 13, 3127–3137 CrossRef CAS PubMed.
- J. Xu, F.-L. Hu, W. Wang, X.-C. Wan and G.-H. Bao, Food Chem., 2015, 186, 176–184 CrossRef CAS PubMed.
- G. Grandinetti, A. E. Smith and T. M. Reineke, Mol. Pharmaceutics, 2012, 9, 523–538 CrossRef CAS PubMed.
- S. Vaidyanathan, B. G. Orr and M. M. Banaszak Holl, Acc. Chem. Res., 2016, 49, 1486–1493 CrossRef CAS PubMed.
- A. A. Y. Almulathanon, E. Ranucci, P. Ferruti, M. C. Garnett and C. Bosquillon, Pharm. Res., 2018, 35, 86 CrossRef PubMed.
- E. Panieri and M. M. Santoro, Cell Death Dis., 2016, 7, e2253 CrossRef CAS PubMed.
- A. Hall, U. Lachelt, J. Bartek, E. Wagner and S. M. Moghimi, Mol. Ther., 2017, 25, 1476–1490 CrossRef CAS PubMed.
- Y. M. Choi, H. K. Kim, W. Shim, M. A. Anwar, J. W. Kwon, H. K. Kwon, H. J. Kim, H. Jeong, H. M. Kim, D. Hwang, H. S. Kim and S. Choi, PLoS One, 2015, 10, e0135083 CrossRef PubMed.
- C. Hoppel, Am. J. Kidney Dis., 2003, 41, S4–S12 CrossRef CAS PubMed.
- T. Fukao, G. Mitchell, J. O. Sass, T. Hori, K. Orii and Y. Aoyama, J. Inherited Metab. Dis., 2014, 37, 541–551 CrossRef CAS PubMed.
- F. Ciccarese and V. Ciminale, Front. Oncol., 2017, 7, 117 CrossRef PubMed.
- B. S. Winkler, N. DeSantis and F. Solomon, Exp. Eye Res., 1986, 43, 829–847 CrossRef CAS PubMed.
- R. J. Mailloux, R. Bériault, J. Lemire, R. Singh, D. R. Chénier, R. D. Hamel and V. D. Appanna, PLoS One, 2007, 2, e690 CrossRef PubMed.
-
M. Ramen T, Depletion of Glutathione during Oxidative Stress and Efficacy of N-Acetyl Cysteine: An Old Drug with New Approaches, 2015 Search PubMed.
- M. S. Lee, N. W. Kim, K. Lee, H. Kim and J. H. Jeong, Pharm. Res., 2013, 30, 1642–1651 CrossRef CAS PubMed.
- A. Hall, L. P. Wu, L. Parhamifar and S. M. Moghimi, Biomacromolecules, 2015, 16, 2119–2126 CrossRef CAS PubMed.
- Y. Morel and R. Barouki, Biochem. J., 1999, 342(Pt 3), 481–496 CrossRef CAS PubMed.
- A. Halama, N. Riesen, G. Moller, M. Hrabe de Angelis and J. Adamski, J. Intern. Med., 2013, 274, 425–439 CrossRef CAS PubMed.
- G. Rainaldi, R. Romano, P. Indovina, A. Ferrante, A. Motta, P. L. Indovina and M. T. Santini, Radiat. Res., 2008, 169, 170–180 CrossRef CAS PubMed.
- O. Takikawa, Biochem. Biophys. Res. Commun., 2005, 338, 12–19 CrossRef CAS PubMed.
- S. R. Thomas and R. Stocker, Redox Rep., 1999, 4, 199–220 CrossRef CAS PubMed.
- T. W. Stone and L. G. Darlington, Nat. Rev. Drug Discovery, 2002, 1, 609 CrossRef CAS PubMed.
- R. L. Matz, B. Erickson, S. Vaidyanathan, J. F. Kukowska-Latallo, J. R. Baker, Jr., B. G. Orr and M. M. Banaszak Holl, Mol. Pharmaceutics, 2013, 10, 1306–1317 CrossRef CAS PubMed.
Footnotes |
† Electronic supplementary information (ESI) available. See DOI: 10.1039/c8mo00139a |
‡ Both authors have equally contributed to this work. |
|
This journal is © The Royal Society of Chemistry 2019 |
Click here to see how this site uses Cookies. View our privacy policy here.