DOI:
10.1039/C8MO00218E
(Research Article)
Mol. Omics, 2019,
15, 88-100
Comprehensive bioinformation analysis of methylated and differentially expressed genes in esophageal squamous cell carcinoma†
Received
20th September 2018
, Accepted 4th January 2019
First published on 24th January 2019
Abstract
Differentially methylated genes (DMGs) play a crucial role in the etiology and pathogenesis of esophageal squamous cell carcinoma (ESCC). This study aimed to ascertain aberrant DMGs and pathways by comprehensive bioinformatics analysis. We downloaded the gene expression microarray of GSE51287 from the Gene Expression Omnibus (GEO). Aberrant DMGs were obtained using the GEO2R tool. Gene ontology (GO) analysis and Kyoto Encyclopedia of Gene and Genome pathway enrichment analyses were performed on selected genes by using the Database for Annotation Visualization and Integrated Discovery. A protein–protein interaction (PPI) network was constructed with the Retrieval of Interacting Genes (STRING) and visualized in Cytoscape. Then, the modules in the PPI networks were analyzed with Molecular Complex Detection, and the hub genes derived from the PPI networks were verified by using the Cancer Genome Atlas. A total of 271 DMGs, including 173 hypermethylated genes, were enriched in the biological processes of peptidyl-tyrosine phosphorylation, positive regulation of transcription from RNA polymerase II promoters, and autophosphorylation. Pathway analysis enrichment revealed cancer, PI3K-Akt, and Ras signaling pathways, and 98 hypomethylated genes were enriched in the biological processes of the immune response, extracellular matrix disassembly, and macrophage differentiation. Pathway enrichment showed rheumatoid arthritis, cytokine–cytokine receptor interaction, and toxoplasmosis. Furthermore, bioinformatics analysis indicated feasible aberrant DMGs and pathways in ESCC. The results provided valuable information on the pathogenesis of ESCC. The significant DMGs may provide novel insights into their potential predictive and prognostic value as methylation-based biomarkers for the precise diagnosis and treatment of ESCC.
1. Introduction
Esophageal squamous cell carcinoma (ESCC) is one of the most common malignancies, ranking eighth in terms of incidence and sixth among the leading causes of cancer-related deaths worldwide.1 Currently, great progress has been made in the investigation of ESCC etiology and prevention. Multiple factors may contribute to ESCC susceptibility, including tobacco smoking, alcohol drinking, dietary carcinogens, and insufficient micronutrients, which are synergistic dietary parameters.2 Most patients with ESCC undergo first advanced metastatic tumors diagnosis.3 Although ESCC treatment methods have considerably improved, especially in surgery, radiation, chemotherapy, or combined treatments, cancer metastasis and invasion remain prevalent. After surgery, overall 5-year survival remains low (14–22%).4,5 The accumulation of miscellaneous genetic and epigenetic alternations in esophageal epithelial cells is an essential process that drives the initiation and progression of ESCC,6 but the accurate molecular mechanism underlying the occurrence and progression of the disease has not been evaluated yet. Thus, new insights into the diagnosis and treatment of ESCC can be obtained by increasing the level of understanding of ESCC pathogenesis.
Methylated aberrant DNA is a primary epigenetically modified form of a DNA sequence, and aberrant DNA methylation is an important means of regulating genomic function.7,8 The process influences the functions of key genes by modifying gene expression particularly through hypermethylation of tumor suppressor genes or hypomethylation of oncogenes.9,10 Differentially methylated genes may play a significant role in the development of various cancers.11,12 Numerous studies have reported that some genes undergo different methylation processes in ESCC, and some methods for discriminating prognostic genes on the basis of DMG data are currently available.13 Therefore, novel cancer-related genes can be sifted by comparing the DNA methylation status of a cancer with that of a normal tissue.14
Bioinformatics is a subject that interprets life characteristics and gene functions by analyzing microarray data, reading thousands of pieces of gene hybridization information on a chip, and linking the data to biological processes.15 As promising and efficient tools, microarrays are based on high-throughput platforms for visualizing screened virtual genetic or epigenetic alternations during carcinogenesis and identifying biomarkers for the diagnosis and prognosis of cancers.16 In the present research, we explored DMGs in ESCC by using online bioinformatics resources. We downloaded the expression profile of GSE51287 and used it to identify the DMGs between tumor tissues and normal tissues from an ESCC. The DMGs were then subjected to hierarchical clustering, and functional enrichment analyses of GO and KEGG pathways were performed using the Database for Annotation Visualization and Integrated Discovery (DAVID). Furthermore, we explored the main hub nodes in PPI networks containing DMGs by using STRING and validated the results using the Cancer Genome Atlas (TCGA) data to identify DMGs involved in the pathogenesis of ESCC. The flowcharts of the bioinformatics analysis methods are illustrated, and different bioinformatics tools are described in the ESI,† Fig. 1. Our results provide comprehensive biological information about DMGs and enhance the level of understanding regarding the development and progression of ESCC.
2. Materials and methods
2.1. Microarray data
The gene expression microarray of GSE51287 was obtained from the Gene Expression Omnibus (GEO) database (https://www.ncbi.nlm.nih.gov/gds/) of the National Center for Biotechnology Information (NCBI). GSE51287 is a methylation profile of patients with ESCC and cancer-free individuals in Taiwan. A total of 98 tumor tissues and 93 normal tissues from ESCC were examined in GSE51287 (Platform GPL9183 Illumina GoldenGate Methylation Cancer Panel I).
2.2. Data processing of DMGs
GEO2R was used in the analysis of GSE51287 and detected DMGs between the tumor tissues and normal tissues. GEO2R (http://www.ncbi.nlm.nih.gov/geo/geo2r/) is an interactive web tool that facilitates the comparison of two or more groups of samples in a GEO series and identification of differentially expressed genes under specific experimental conditions. P of <0.05 and |t| of >2 were set as the cut-off criteria for the identification of DMGs. Subsequently, 271 DMGs were obtained, including 173 hypermethylated and 98 hypomethylated genes.
2.3. Go and KEGG pathway analysis of DMGs
Gene ontology (GO) analysis is a useful bioinformatics tool for annotating genes and gene products and identifying characteristic biological attributes for high-throughput genome or transcriptome data,17 which include cellular components, molecular functions, and biological processes.18 Kyoto Encyclopedia of Genes and Genomes (KEGG) pathway enrichment analysis was executed by using DAVID (https://david.ncifcrf.gov/) for the selected DMGs.19P < 0.05 was set as the cut-off criterion and regarded as statistically significant.20
2.4. Hub genes and PPI network construction, MCODE, and BiNGO analysis
Hub genes that generally represent some important genes involved in diseases can be identified by comprehensive bioinformation analysis. Based on the information obtained through the STRING protein query performed in public databases and on a critical workflow component for executing network visualization, analysis, and publishing tasks in Cytoscape, we made the PPI network of the top 20 hub genes with high degrees of connectivity. PPI analysis is vital to the illustration of the molecular mechanisms of key cellular processes during carcinogenesis. The purpose of the STRING database (http://string-db.org) is to provide bases for critical assessment and integrate PPIs through direct (physical) and indirect (functional) associations.21 Cytoscape is a critical workflow component for executing network visualization and analysis and publishing tasks.22 Molecular complex detection (MCODE) is a method used to detect densely connected regions in PPI networks.23 The Biological Networks Gene Ontology (BiNGO) tool as a plug-in for Cytoscape permitted Cytoscape to link to the GO database.24 MCODE and BINGO are available in the Cytoscape app store (http://apps.cytoscape.org). A clustering tool was used to screen modules and GO terms of the PPI network in Cytoscape.
For our study, we used the Cytoscape software to construct PPI networks based on the STRING results. Interactions with a combined score of >0.9 were considered significant. Subsequently, MCODE was utilized to screen modules of the PPI network with a score of >4 and number of nodes >4 in Cytoscape. Moreover, the pathway analysis of genes in each module was implemented by DAVID. Using a P-value cut-off of 0.05, we determined the GO terms of the BINGO analysis.
2.5. TCGA data validation of the Hub DMGs
The cBioPortal for Cancer Genomics (http://cbioportal.org) provides a Web resource for exploring, visualizing, and analyzing multidimensional cancer genomics data. The portal provides the graphical summaries of gene-level data from multiple platforms, network visualization and analysis, survival analysis, patient-centric queries, and software programmatic access including TCGA data. Through TCGA data validation, we selected the top 20 genes with high DMGs of connectivity as hub genes. Given the small sample size of our study, we performed validation analysis to verify our results for gene methylation and expression analysis by cBioPortal.
2.6. Expression level and survival analysis of the Hub DMGs
UALCAN (http://ualcan.path.uab.edu/index.html) is an easy-to-use and open source interactive web portal for the in-depth analysis of TCGA gene expression data. UALCAN uses TCGA level 3 RNA-Seq and clinical data from 31 cancer types. The portal has user-friendly features25 and allows the customization of functions, such as tumor and normal differential expression analysis. By using this tool, we were able to demonstrate the expression levels of hub genes in normal tissues, adenocarcinomas, and squamous cell carcinomas of ESCA. Eventually, a boxplot graph was produced for the visualization of relationships. The association of IL6, IL8, EPO, FLT1, CCL3, and IHH expression levels with overall survival (OS) or recurrence-free survival (RFS) was assessed by using Kaplan–Meier survival curves, and the hazard ratio (HR) with 95% confidence intervals and log rank P-values were calculated and displayed on the plot.
3. Results
3.1. DMG identification in ESCC
For the gene methylation microarray, 271 DMGs were obtained between ESCC and normal samples, including 173 hypermethylated and 98 hypomethylated genes. The remarkable heat map of the top 50 hypermethylated and hypomethylated genes in GSE51287 is shown in Fig. 1.
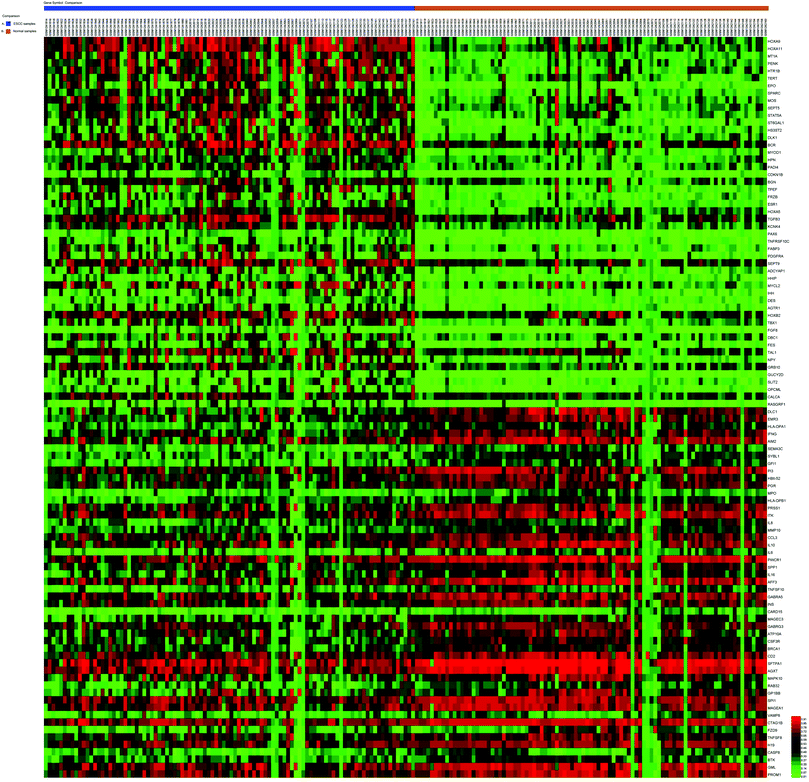 |
| Fig. 1 Representative heat map of the top 100 differentially methylated genes in dataset GSE51287 (50 up-regulated and 50 down-regulated genes). The horizontal axis represents sample names. The blue part represents ESCC samples, and the brown part represents normal samples. The right vertical axis shows clusters of DMGs, and the above horizontal axis shows clusters of samples. Red color represents up-regulated genes, whereas green color represents down-regulated genes. | |
3.2. GO functional enrichment analysis
The top 20 remarkable terms obtained by GO enrichment analysis were illustrated in our study. We imported all DMGs to DAVID, and the GO analysis results showed that hypermethylated DMGs and hypomethylated DMGs were particularly enriched in biological processes, including peptidyl-tyrosine phosphorylation, positive regulation of transcription by RNA polymerase II promoters, autophosphorylation, and positive regulation of cell proliferation. In the cell component, the DMGs showed extracellular spaces and regions, plasma membranes, and neuron projections. In addition, molecular functions displayed enrichment predominantly during protein tyrosine kinase activity, transmembrane receptor protein tyrosine kinase activity, vascular endothelial growth factor-activated receptor activity, and the binding of sequence-specific DNA and proteins.
In the hypomethylated DMGs, the enriched biological processes included the immune response, the extracellular matrix disassembly, macrophage differentiation, the cellular response to lipopolysaccharides, and cell–cell signaling. The GO cell component displayed extracellular spaces and regions, integral components of plasma membranes, proteinaceous extracellular matrixes and membrane rafts. In addition, molecular function enrichment indicated cytokine activity, receptor binding, metalloendopeptidase activity, protein binding, and growth factor activity. These screened pathways demonstrated that DMGs perform a crucial function in the tumor microenvironment in ESCC (Table 1 and the ESI,† Fig. 2).
Table 1 Gene ontology analysis of methylated-differentially expressed genes associated with ESCC
Category |
GO analysis |
Term |
Gene count |
% |
P value |
If there were more than five terms enriched in this category, the top five terms were selected according to the P value. Count: the number of enriched genes in each term. |
Hypermethylation |
|
|
31 |
11.44 |
2.00 × 10−24 |
GOTERM_BP_DIRECT |
GO:0018108 ∼ peptidyl-tyrosine phosphorylation |
19 |
11.65644172 |
7.63 × 10−15 |
GOTERM_BP_DIRECT |
GO:0045944 ∼ positive regulation of transcription from RNA polymerase II promoter |
38 |
23.31288344 |
4.93 × 10−13 |
GOTERM_BP_DIRECT |
GO:0046777 ∼ protein autophosphorylation |
17 |
10.42944785 |
9.95 × 10−12 |
GOTERM_BP_DIRECT |
GO:0008284 ∼ positive regulation of cell proliferation |
25 |
15.33742331 |
2.01 × 10−11 |
GOTERM_BP_DIRECT |
GO:0043065 ∼ positive regulation of the apoptotic process |
18 |
11.04294479 |
5.11 × 10−9 |
GOTERM_CC_DIRECT |
GO:0005576 ∼ extracellular region |
42 |
25.76687117 |
4.22 × 10−10 |
GOTERM_CC_DIRECT |
GO:0005615 ∼ extracellular space |
37 |
22.6993865 |
1.79 × 10−9 |
GOTERM_CC_DIRECT |
GO:0005887 ∼ integral component of plasma membrane |
35 |
21.47239264 |
7.69 × 10−8 |
GOTERM_CC_DIRECT |
GO:0043025 ∼ neuronal cell body |
15 |
9.202453988 |
8.84 × 10−7 |
GOTERM_CC_DIRECT |
GO:0009986 ∼ cell surface |
19 |
11.65644172 |
1.64 × 10−6 |
GOTERM_MF_DIRECT |
GO:0004713 ∼ protein tyrosine kinase activity |
12 |
7.36196319 |
3.95 × 10−8 |
GOTERM_MF_DIRECT |
GO:0004714 ∼ transmembrane receptor protein tyrosine kinase activity |
8 |
4.90797546 |
4.77 × 10−8 |
GOTERM_MF_DIRECT |
GO:0005021 ∼ vascular endothelial growth factor-activated receptor activity |
5 |
3.067484663 |
2.34 × 10−7 |
GOTERM_MF_DIRECT |
GO:0043565 ∼ sequence-specific DNA binding |
19 |
11.65644172 |
1.32 × 10−6 |
GOTERM_MF_DIRECT |
GO:0005515 ∼ protein binding |
109 |
66.87116564 |
5.46 × 10−6 |
Hypomethylation |
GOTERM_BP_DIRECT |
GO:0006955 ∼ immune response |
16 |
17.97752809 |
2.32 × 10−9 |
GOTERM_BP_DIRECT |
GO:0022617 ∼ extracellular matrix disassembly |
8 |
8.988764045 |
1.01 × 10−7 |
GOTERM_BP_DIRECT |
GO:0030225 ∼ macrophage differentiation |
4 |
4.494382022 |
9.33 × 10−5 |
GOTERM_BP_DIRECT |
GO:0071222 ∼ cellular response to lipopolysaccharides |
6 |
6.741573034 |
2.55 × 10−4 |
GOTERM_BP_DIRECT |
GO:0007267 ∼ cell–cell signaling |
8 |
8.988764045 |
2.80 × 10−4 |
GOTERM_CC_DIRECT |
GO:0005615 ∼ extracellular space |
26 |
29.21348315 |
6.95 × 10−10 |
GOTERM_CC_DIRECT |
GO:0005576 ∼ extracellular region |
25 |
28.08988764 |
1.18 × 10−7 |
GOTERM_CC_DIRECT |
GO:0005887 ∼ integral component of plasma membrane |
19 |
21.34831461 |
5.44 × 10−5 |
GOTERM_CC_DIRECT |
GO:0005578 ∼ proteinaceous extracellular matrix |
8 |
8.988764045 |
2.20 × 10−4 |
GOTERM_CC_DIRECT |
GO:0045121 ∼ membrane raft |
7 |
7.865168539 |
3.55 × 10−4 |
GOTERM_MF_DIRECT |
GO:0005125 ∼ cytokine activity |
8 |
8.988764045 |
2.53 × 10−5 |
GOTERM_MF_DIRECT |
GO:0005102 ∼ receptor binding |
9 |
10.11235955 |
3.40 × 10−4 |
GOTERM_MF_DIRECT |
GO:0004222 ∼ metalloendopeptidase activity |
5 |
5.617977528 |
0.00232978 |
GOTERM_MF_DIRECT |
GO:0005515 ∼ protein binding |
57 |
64.04494382 |
0.003089369 |
GOTERM_MF_DIRECT |
GO:0008083 ∼ growth factor activity |
5 |
5.617977528 |
0.00834797 |
3.3. Pathway functional enrichment analysis
Using the KEGG pathway enrichment analysis, hypermethylated DMGs were momentously enriched in several pathways, such as cancer, PI3K-Akt, Ras and Rap1 signaling, and transcriptional misregulation in cancer. Meanwhile, hypomethylated DMGs indicated enrichment in the pathways of rheumatoid arthritis, cytokine–cytokine receptor interaction, toxoplasmosis, and transcriptional misregulation in cancer and tuberculosis. These screened pathways suggested that DMGs have essential functions in the etiology and pathogenesis of ESCC (Table 2).
Table 2 KEGG pathway analysis of methylated differentially expressed genes associated with ESCC
Category |
GO analysis |
Term |
Gene count |
% |
P value |
If there were more than five terms enriched in this category, the top five terms were selected according to the P value. Count: the number of enriched genes in each term. |
Hypermethylation |
KEGG_PATHWAY |
hsa05200:Pathways in cancer |
26 |
15.95092025 |
4.07 × 10−10 |
KEGG_PATHWAY |
hsa04151:PI3K-Akt signaling pathway |
18 |
11.04294479 |
1.29 × 10−5 |
KEGG_PATHWAY |
hsa04014:Ras signaling pathway |
14 |
8.588957055 |
3.03 × 10−5 |
KEGG_PATHWAY |
hsa05202:Transcriptional misregulation in cancer |
12 |
7.36196319 |
3.99 × 10−5 |
KEGG_PATHWAY |
hsa04015:Rap1 signaling pathway |
13 |
7.975460123 |
6.69 × 10−5 |
|
Hypomethylation |
KEGG_PATHWAY |
hsa05323:Rheumatoid arthritis |
7 |
7.865168539 |
6.31 × 10−5 |
KEGG_PATHWAY |
hsa04060:Cytokine–cytokine receptor interaction |
10 |
11.23595506 |
7.17 × 10−5 |
KEGG_PATHWAY |
hsa05145:Toxoplasmosis |
7 |
7.865168539 |
3.20 × 10−4 |
KEGG_PATHWAY |
hsa05202:Transcriptional misregulation in cancer |
8 |
8.988764045 |
3.36 × 10−4 |
KEGG_PATHWAY |
hsa05152:Tuberculosis |
8 |
8.988764045 |
4.62 × 10−4 |
3.4. PPI network construction and module analysis
On the basis of date in the STRING protein query and downloaded data from public databases, the PPI DMG network was constructed. The network contained 223 nodes and 1289 edges, including 173 hypermethylated genes and 98 hypomethylated genes, as shown in Fig. 2. We used MCODE to explore meaningful modules for DMGs in this network. The top six modules in the network were triaged to be statistically significant (Table 3). The four DMG modules selected are shown in Fig. 3. KEGG pathway enrichment analysis showed that the four core modules manifested the functions of PI3K-Akt signaling and cancer pathway, cytokine–cytokine receptor interaction, transcriptional misregulation and proteoglycans in cancer, the p53 signaling pathway, and focal adhesion.
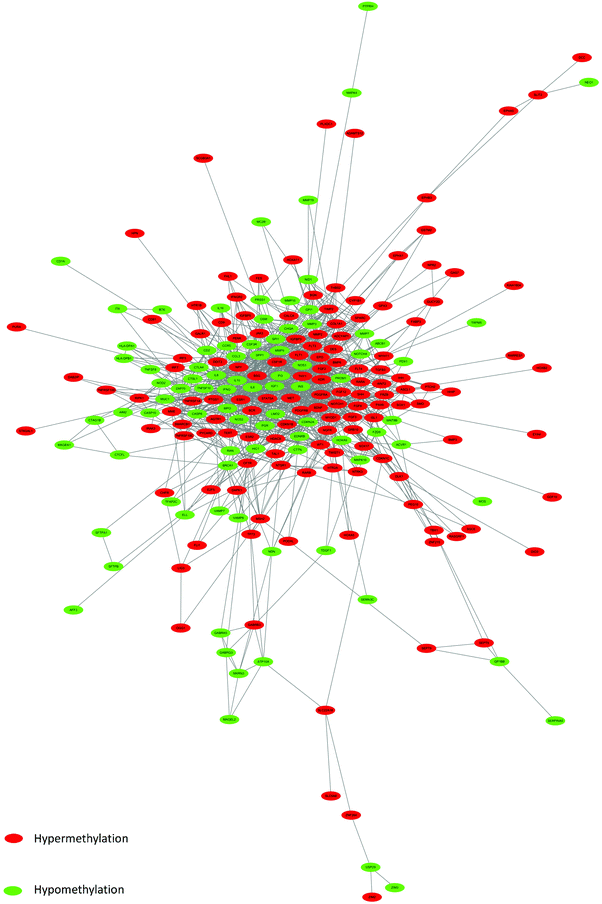 |
| Fig. 2 PPI network of DMGs. Red color represents up-regulated genes, and green color represents down-regulated genes. | |
Table 3 Modules of the protein–protein interaction networks
Category |
Score |
Nodes |
Edges |
Gene |
1 |
10.696 |
24 |
123 |
CDKN2A, IL6, MMP7, CSF1R, TERT, CASP8, TNFSF10, ESR1, INS, WT1, TNFRSF1A, PDGFRB, IGF1, CFTR, IGFBP3, FLT4, NOTCH1, BDNF, MYOD1, EPO, MMP9, OSM, THY1, PROM1 |
2 |
8.833 |
13 |
53 |
IL8, FLT1, MMP3, SPP1, KDR, FGF2, PGR, PI3, MET, IL10, MMP2, TIMP2, NOS3 |
3 |
4 |
4 |
6 |
MKRN3, GABRB3, GABRG3, GABRA5 |
4 |
3.6 |
6 |
9 |
CCR5, CD2, CTLA4, HTR1B, PENK, GALR1 |
5 |
3.333 |
4 |
5 |
HLA-DPA1, HLA-DPB1, CTSL1, ZAP70 |
6 |
3.25 |
9 |
13 |
CDKN1B, ISL1, IFNG, STAT5A, SPI1, PAX6, BCR, SHH, CSF3R |
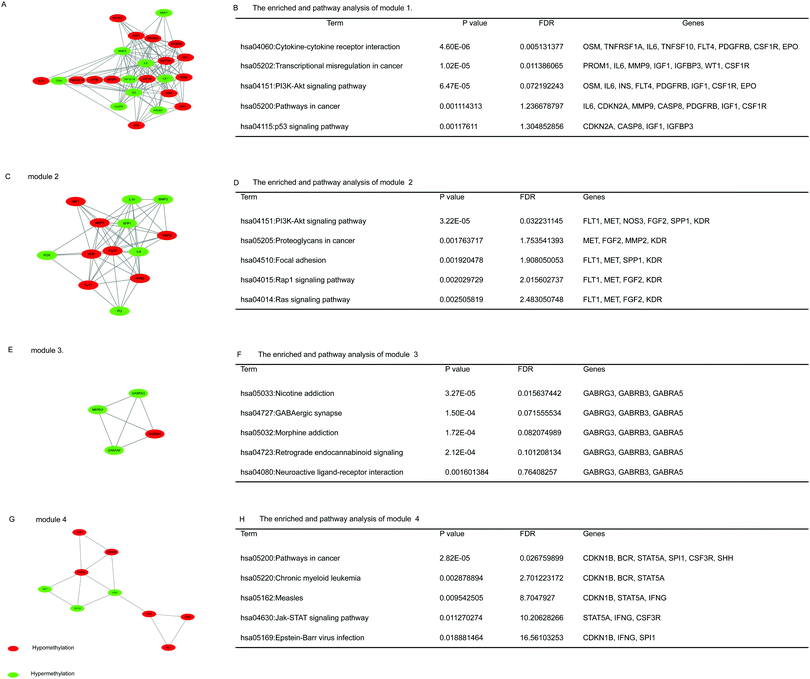 |
| Fig. 3 The top four modules for DMGs were selected. (A) Module 1, (B) the enriched pathways of module 1, (C) module 2, (D) the enriched pathways of module 2, (E) module 3, (F) the enriched pathways of module 3, (G) module 4, and (H) the enriched pathways of modules 4. Red color represents up-regulated genes, and green color represents down-regulated genes. | |
3.5. Validation of the hub genes in the TCGA database
We selected the top 20 genes with high DMGs of connectivity as hub genes by using the PPI network. The genes were annotated as INS, IL6, FGF2, IGF1, NOTCH1, MMP9, IL8, IL10, ESR1, CDKN2A, KDR, MMP2, IFNG, NOS3, SHH, BCR, STAT5A, SPP1, EPO, and BDNF and then validated in another TCGA database. The results showed that eight hub genes, namely, NOTCH1, MMP9, IL8, CDKN2A, IFNG, BCR, SPP1, and EPO, had significant differences between the tumor and normal tissues of ESCAs. Finally, six hypomethylated and up-regulation genes for NOTCH1, MMP9, IL8, IFNG, BCR, and SPP1 were identified. Meanwhile, one hypomethylated and down-regulation gene was designated CDKN2A (Table 4 and Fig. 3, ESI†). The status of methylation and expression of the hub genes was considerably altered. Our study showed this alteration, thereby indicating the stability and reliability of our results.
Table 4 Top 20 hub genes with higher degree of connectivity and validation of the hub genes in the TCGA database
Category |
Hub gene |
Degree of connectivity |
Methylation status |
P value |
Expression status |
P value |
Hypomethylation |
INS |
78 |
— |
— |
No differential |
>0.05 |
IL6 |
71 |
Hypermethylation |
0.0475 |
No differential |
>0.05 |
MMP9 |
53 |
Hypomethylation |
1.674 × 10−4 |
Up-regulation
|
5.53 × 10−5 |
IL8 |
52 |
Hypomethylation |
0.0542 |
Up-regulation
|
1.68 × 10−5 |
IL10 |
50 |
Hypermethylation |
0.0140 |
No differential |
>0.05 |
IFNG |
41 |
Hypomethylation |
0.0222 |
Up-regulation
|
7.31 × 10−8 |
NOS3 |
38 |
Hypomethylation |
0.0172 |
No differential |
>0.05 |
SPP1 |
30 |
Hypomethylation |
9.94 × 10−7 |
Up-regulation
|
1.33 × 10−8 |
EPO |
30 |
— |
— |
Up-regulation
|
3.03 × 10−4 |
|
Hypermethylation |
FGF2 |
62 |
Hypermethylation |
1.54 × 10−13 |
No differential |
>0.05 |
IGF1 |
60 |
Hypermethylation |
1.575 × 10−3 |
No differential |
>0.05 |
NOTCH1 |
58 |
Hypomethylation |
1.767 × 10−6 |
Up-regulation
|
3.36 × 10−8 |
ESR1 |
45 |
Hypermethylation |
1.35 × 10−10 |
No differential |
>0.05 |
CDKN2A |
43 |
Hypomethylation |
4.64 × 10−16 |
Down-regulation
|
1.42 × 10−4 |
KDR |
42 |
Hypermethylation |
0.0295 |
No differential |
>0.05 |
MMP2 |
42 |
Hypomethylation |
8.556 × 10−6 |
No differential |
>0.05 |
SHH |
33 |
Hypermethylation |
1.37 × 10−7 |
No differential |
>0.05 |
BCR |
31 |
Hypomethylation |
4.672 × 10−4 |
Up-regulation
|
4.28 × 10−3 |
STAT5A |
30 |
Hypomethylation |
5.09 × 10−31 |
No differential |
>0.05 |
BDNF |
28 |
Hypermethylation |
5.08 × 10−8 |
No differential |
>0.05 |
3.6. Expression level and survival analysis of hub DMGs in ESCA
The expression of the eight hub DMGs, namely, NOTCH1, MMP9, IL8, CDKN2A, IFNG, BCR, SPP1, and EPO was demonstrated in normal tissues and in the adenocarcinomas and squamous cell carcinomas of ESCA. Further, boxplots were made for the visualization of the relationships among the expression levels of the eight pivotal DMGs. In the hub DMGs, MMP9, IL8, IFNG, SPP1, and EPO were overexpressed in ESCA relative to those in normal esophageal tissues. Similarly, CDKN2A had lower expression levels. In TCGA, the cases with adenocarcinoma (N = 89) and squamous cell carcinoma of ESCA (N = 95) had significantly higher GENES expression levels than those with normal esophageal tissues (N = 11; P > 0.05; Fig. 4). Six DMGs with high degrees of connectivity were selected (gene count > 10). High IL6, IL8, FLT1, CCL3, and IHH expression was consistently associated with worse overall survival (OS). However, EPO expression is reversed according to our results. We inferred that the high expression levels of the six DMGs are independent predictors for ESCC according to UALCAN (Fig. 5).
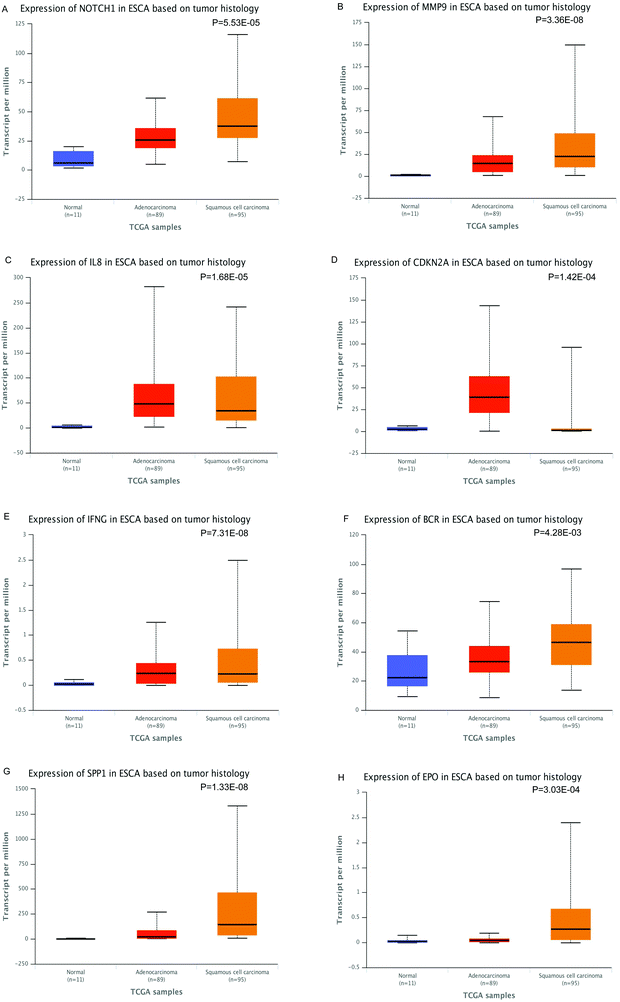 |
| Fig. 4 Expression level of the hub genes in ESCA based on tumor histology (normal, adenocarcinomas and squamous cell carcinomas), P-values < 0.05 (*) have statistical significance. | |
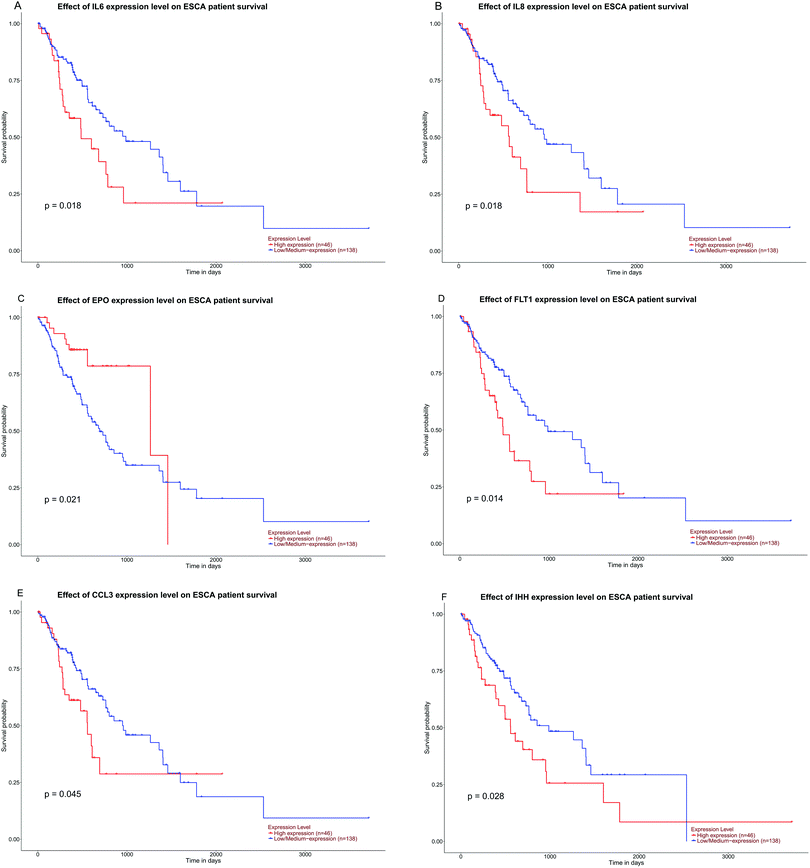 |
| Fig. 5 The hub DMGs of IL6, IL8, FLT1, CCL3, and IHH low/medium and EPO high expression level effects on ESCA patients’ survival were associated with better prognosis; P-values < 0.05 (*) have statistical significance. Six DMGs with a high degree of connectivity were selected (gene count > 10). | |
4. Discussion
ESCC is one of the most general types of malignant tumors in China, and it is usually detected in the late stage. When no positive result arises from intervention treatments for ESCC patients, leading recurrence is present, and a very low 5-year survival rate and high mortality are achieved.26 DNA methylation can shift the expression level of genes, which can contribute to cancer development.27 Hypomethylation commonly arises early and has been linked to chromosome instability (CIN) and loss of imprinting, whereas hypermethylation is associated with promoters and can arise secondary to gene (oncogene suppressor) silencing.28,29 The underlying mechanisms indicated that the initiation and progression of ESCC would greatly contribute to the diagnosis, treatment, and prognosis assessment. For this study, we identified 173 hypermethylated genes and 98 hypomethylated genes that may be involved in the molecular regulation of essential pathways associated with ESCC development through analyzing available data of GSE51287 in ESCC by using numerous bioinformatics tools.
As revealed by GO term analysis, hypermethylated genes in ESCC were enriched in the biological processes of peptidyl-tyrosine phosphorylation, protein autophosphorylation, and positive regulation of transcription from RNA polymerase II promoters, cell proliferation, and apoptotic processes. Remarkably, about 25 hypermethylated genes enriched positive regulation of cell proliferation in biological processes. This result is inconsistent with the previous conclusion that hypermethylation should correlate with gene down-regulation or silencing, and hypomethylation should fit with gene overexpression. What is the accurate relationship between the degree of methylation and expression for genes? We need to conduct in-depth studies warranted to determine their role in ESCC development and progression.
Molecular function indicated enrichment in the kinase activity of protein tyrosine and transmembrane receptor protein tyrosine, vascular endothelial growth factor-activated receptor activity, sequence-specific DNA binding, and protein binding. For hypomethylated genes in ESCC, GO analysis suggested that the enriched biological processes were the immune response, extracellular matrix disassembly, macrophage differentiation, the cellular response to lipopolysaccharides, and cell–cell signaling. Molecular function enrichment revealed the cytokine activity, receptor binding, metalloendopeptidase activity, protein binding, and growth factor activity. The results of these analyses are reasonable, because frequent cell proliferation and loss of cell adhesion are signs of many cancers, including ESCC.30–32 As the main inflammatory mediator, cytokines strictly determine the tumor-promoting or anti-tumor signals within the ESCC environment.33 Moreover, methylation could affect development and progression of ESCC by the phosphorylation of a multitude of enzymes and proteins.34 In addition, nerve ablation delays the development of precancerous lesions and inhibits tumor growth and metastasis;35 nevertheless, the relevant research in ESCC has not been performed. Therefore, the regulation of methylation for nerve relevant genes might become a promising research point for ESCC development in the future.
KEGG analysis displayed significant enrichment in pathways including cancer, PI3K-Akt, Ras and Rap1 signaling, transcriptional misregulation, and cytokine–cytokine receptor interaction in cancer of DMGs. The phosphatidylinositol-3-kinase (PI3K) signaling pathway has multiple cellular functions, such as cell metabolism, proliferation, cell-cycle progression, and survival. Several studies indicated that numerous components of the PI3K/AKT pathway were targeted by amplification, mutation, and translocation more frequently than any other pathway in cancer patients, thereby leading to pathway activation.36–38 Studies have shown that inhibition of Ras and Rap1 signaling pathway methylation can suppress proliferation, metastasis, and invasion in ESCC.39,40 This finding is consistent with the regulatory mechanisms in ESCC. These studies outlined the crucial functions of transcription factors and cytokine–cytokine receptors in ESCC, which were candidate study targets because of their underlying mechanisms of methylation and dysregulation.
Core modules within the PPI network of DMGs have functions that include PI3K-Akt signaling and cancer pathways, cytokine–cytokine receptor interaction, transcriptional misregulations and proteoglycans in cancer, the p53 signaling pathway, and focal adhesion. The top two modules were related to the PI3K-Akt signaling pathway, thereby hinting that methylation mainly affected the expression of genes in that pathway. At present, many related studies on the PI3K-Akt pathway exist. However, studies on its pathways associated with the methylation of genes in ESCC are rare. Thus, further research on the methylation of genes involved in the PI3K-Akt pathway is needed. Furthermore, focal adhesion kinase inhibition could substantially limit cancer progression and extend the survival time of cancer patients.41
The PPI network for DMGs clarified the overview of their functional connections, of which the top 20 hub genes were also selected: INS, IL6, FGF2, IGF1, NOTCH1, MMP9, IL8, IL10, ESR1, CDKN2A, KDR, MMP2, IFNG, NOS3, SHH, BCR, STAT5A, SPP1, EPO, and BDNF. Moreover, we used the TCGA database analysis to verify the top 20 hub genes. NOTCH1, MMP9, IL8, CDKN2A, IFNG, BCR, SPP1, and EPO were the DMGs in gene expression and DNA methylation status. Six DMGs with a high degree of connectivity were selected, namely, IL6, IL8, EPO, FLT1, CCL3, and IHH (gene count > 10).
In a previous study on these selected DMGs, the expression levels of INS and IGF1 were determined in ESCC; researchers investigated their detailed function and screened the potential applications of INS in clinical development.42,43 The characteristics and functions of interleukins (ILs) including IL6, IL8, and IL10 were gradually clarified. They play an important role in tumorigenesis, development, and cancer treatment. For instance, IL is highly expressed in tumor tissues of ESCC patients and may play an important role in invasion and metastasis of ESCC.44,45 Fibroblast growth factors (FGFs) are involved in a variety of cellular processes, such as stemness, proliferation, anti-apoptosis, drug resistance, and angiogenesis.46,47 NOTCH1 is a tumor suppressor gene in head and neck squamous cell carcinoma (HNSCC),48 but little is known about its role in ESCC. The higher levels of MMP-9 protein in ESCC compared with normal esophageal tissues suggest their association with esophageal oncogenesis.49 The application of EPO in the treatment of patients with ESCC might increase the survival rate. The difference in the expression level and survival analysis indicates that the relationship between EPO expression in ESCC and patient prognosis may be affected by many factors.50 The abovementioned molecules have a very crucial feature for the entire process of ESCC development. FLT1, an “fms-like tyrosine kinase” receptor, has been suggested to play an active role in vascular endothelial growth factor (VEGF)-mediated autocrine signaling of tumor growth and angiogenesis.51 CCL3 and IHH genes have not been included in an ESCC study and are worthy of research. In conclusion, the DMGs may have potential predictive and prognostic value and could be used as methylation-based biomarkers for precise ESCC diagnosis and treatment. Therefore, in-depth studies are warranted to determine their role in the development and progression of esophageal cancer.
5. Conclusions
In short, by using the multilayer and multi-angle of data mining and business-like bioinformatics analysis of ESCC microarray gene methylation data, we discovered that the occurrence and development of ESCC involve multi-gene abnormal methylation caused by a plurality of signaling pathways, which leads to esophagus malignant transformation. The progress of ESCC can be better controlled with new research ideas and methods for cancer prevention, diagnosis, and treatment. Further molecular biological experiments are required to verify the function of the identified candidate genes in ESCC.
Abbreviations
DMGs | Differentially methylated genes |
ESCC | Esophageal squamous cell carcinoma |
GEO | Gene expression omnibus |
GO | Gene ontology |
KEGG | Kyoto encyclopedia of gene and genome |
DAVID | Database for Annotation Visualization and Integrated Discovery |
PPI | Protein–protein interaction |
STRING | Constructed by the retrieval of interacting genes |
MCODE | Molecular complex detection |
TCGA | The cancer genome atlas |
ESCA | Esophageal carcinoma |
OS | Overall survival |
RFS | Recurrence-free survival |
HR | Hazard ratio |
BP | Biological processes |
CC | Cell component |
MF | Molecular function |
PI3K | Phosphatidylinositol 3-kinase |
ILs | Interleukins |
FGFs | Fibroblast growth factors |
HNSCC | Head and neck squamous cell carcinoma |
VEGF | Vascular endothelial growth factor |
Author contribution
All authors participated in the preparation of the manuscript. XC and YC performed the study conception and design. HP and SW conceptualised the research. LY standardised the procedure for sequence search. SW applied them on all the data and wrote the first draft of the manuscript.
Conflicts of interest
The authors declare no conflicts of interest.
Acknowledgements
This study was supported by Grants from the National Natural Science Foundation of China (No. 81460362, 81773116, 81760436, 81560399, 81860518, and 81360358), the medical and health science and technology project of Suzhou high tech Zone (No. 2017Z006), the international science and technology cooperation project of Shihezi University (No. GJHZ201702), the Applied Basic Research Projects of Xinjiang Production and Construction Corps (No. 2016AG020), the Youth Science and Technology Innovation Leading Talents Project of Corps (2017CB004), the Major science and technology projects of Shihezi University (No. gxjs2014-zdgg06), the high-level talent project of Shihezi University (No. RCZX201533), and the Foundation for Distinguished Young Scholars of Shihezi University (No. 2015ZRKXJQ02). The funders were not involved in the study design, data collection and analysis, decision to publish, or preparation of the manuscript.
References
- J. Ferlay, H. R. Shin, F. Bray, D. Forman, C. Mathers and D. M. Parkin, Estimates of worldwide burden of cancer in 2008: GLOBOCAN 2008, Int. J. Cancer, 2010, 127(12), 2893–2917 CrossRef CAS PubMed.
- H. Kuwano, K. Sumiyoshi and K. Sonoda,
et al., Pathogenesis of esophageal squamous cell carcinoma with lymphoid stroma, Hepatogastroenterology, 2001, 48(38), 458–461 CAS.
- X. B. Lv, G. Y. Lian, H. R. Wang, E. Song, H. Yao and M. H. Wang, Long Noncoding RNA HOTAIR Is a Prognostic Marker for Esophageal Squamous Cell Carcinoma Progression and Survival, PLoS One, 2013, 8(5), e63516 CrossRef CAS PubMed.
- P. Sun, F. Zhang and C. Chen,
et al., Prognostic impact of body mass index stratified by smoking status in patients with esophageal squamous cell carcinoma, OncoTargets Ther., 2016, 9, 6389–6397 CrossRef PubMed.
- Z. Gamliel and M. J. Krasna, Multimodality treatment of esophageal cancer, Surg. Clin. North Am., 2005, 85(3), 621–630 CrossRef PubMed.
- A. M. Kaz and W. M. Grady, Epigenetic biomarkers in esophageal cancer, Cancer Lett., 2014, 342(2), 193–199 CrossRef CAS PubMed.
- D. Aran and A. Hellman, DNA methylation of transcriptional enhancers and cancer predisposition, Cell, 2013, 154(1), 11 CrossRef CAS PubMed.
- S. B. Baylin and P. A. Jones, A decade of exploring the cancer epigenome – biological and translational implications, Nat. Rev. Cancer, 2011, 11(10), 726–734 CrossRef CAS PubMed.
- E. Manel, CpG island hypermethylation and tumor suppressor genes: a booming present, a brighter future, Oncogene, 2002, 21(35), 5427 CrossRef PubMed.
- A. P. Feinberg and B. Vogelstein, Hypomethylation of ras oncogenes in primary human cancers, Biochem. Biophys. Res. Commun., 1983, 111(1), 47–54 CrossRef CAS PubMed.
- A. Razin and A. D. Riggs, DNA methylation and gene function, Science, 1980, 210(4470), 604–610 CrossRef CAS PubMed.
- R. J. Klose and A. P. Bird, Genomic DNA methylation: the mark and its mediators, Trends Biochem. Sci., 2006, 31(2), 89–97 CrossRef CAS PubMed.
- J. Sandoval, J. Mendezgonzalez and E. Nadal,
et al., A Prognostic DNA Methylation Signature for Stage I Non-Small-Cell Lung Cancer, J. Clin. Oncol., 2013, 31(32), 4140–4147 CrossRef PubMed.
- F. Coppedè, Epigenetic biomarkers of colorectal cancer: Focus on DNA methylation, Cancer Lett., 2014, 342(2), 238–247 CrossRef PubMed.
- J. Quackenbush, Computational analysis of microarray data, Nat. Rev. Genet., 2001, 2(6), 418 CrossRef CAS PubMed.
- V. Kulasingam and E. P. Diamandis, Strategies for discovering novel cancer biomarkers through utilization of emerging technologies, Nat. Clin. Pract. Oncol., 2008, 5(10), 588–599 CrossRef CAS PubMed.
- M. Ashburner, C. Ball and J. Blake,
et al., Gene ontology: tool for the unification of biology. The Gene Ontology Consortium, Nat. Genet., 2000, 25(1), 25–29 CrossRef CAS PubMed.
- The Gene Ontology (GO) project in 2006, Nucleic Acids Res., 2006, 34, D322–D326 CrossRef PubMed.
- M. Kanehisa and S. Goto, KEGG: kyoto encyclopedia of genes and genomes, Nucleic Acids Res., 2000, 28(1), 27–30 CrossRef CAS PubMed.
- d. W. Huang, B. Sherman and R. Lempicki, Systematic and integrative analysis of large gene lists using DAVID bioinformatics resources, Nat. Protoc., 2009, 4(1), 44–57 CrossRef CAS PubMed.
- D. Szklarczyk, A. Franceschini and S. Wyder,
et al., STRINGv10: protein-protein interaction networks, integrated over the tree of life, Nucleic Acids Res., 2015, 43, D447–D452 CrossRef CAS PubMed.
- K. Ono, T. Muetze, G. Kolishovski, P. Shannon and B. Demchak, CyREST: Turbocharging Cytoscape Access for External Tools via a RESTful API, F1000Research, 2015, 4, 478 Search PubMed.
- G. D. Bader and C. W. Hogue, An automated method for finding molecular
complexes in large protein interaction networks, BMC Bioinf., 2003, 4(1), 2 CrossRef.
- S. Maere, K. Heymans and M. Kuiper, BiNGO: a Cytoscape plugin to assess overrepresentation of gene ontology categories in biological networks, Bioinformatics., 2005, 21(16), 3448–3449 CrossRef CAS PubMed.
- D. S. Chandrashekar, B. Bashel and B. Sah,
et al., UALCAN: A Portal for Facilitating Tumor Subgroup Gene Expression and Survival Analyses, Neoplasia, 2017, 19(8), 649–658 CrossRef CAS PubMed.
- J. J. González-Plaza, N. Hulak, E. García-Fuentes, L. Garrido-Sánchez, Z. Zhumadilov and A. Akilzhanova, Oesophageal squamous cell carcinoma (ESCC): advances through omics technologies, towards ESCC salivaomics, Drug Discoveries Ther., 2015, 9(4), 247 CrossRef PubMed.
- B. Győrffy, G. Bottai and T. Fleischer,
et al., Aberrant DNA methylation impacts gene expression and prognosis in breast cancer subtypes, Int. J. Cancer, 2016, 138(1), 87–97 CrossRef PubMed.
- J. J. Lee, J. Geli and C. Larsson,
et al., Gene-specific promoter hypermethylation without global hypomethylation in follicular thyroid cancer, Int. J. Oncol., 2008, 33(4), 861 CAS.
- S. Pavanello, V. Bollati and A. C. Pesatori,
et al., Global and gene – specific promoter methylation changes are related to anti-B[a]PDE-DNA adduct levels and influence micronuclei levels in polycyclic aromatic hydrocarbon-exposed individuals, Int. J. Cancer, 2009, 125(7), 1692–1697 CrossRef CAS PubMed.
- M. F. Roizen, Hallmarks of Cancer: The Next Generation, Yearbook of Anesthesiology & Pain Management, 2012, 2012, 13 Search PubMed.
- D. Hanahan and R. Weinberg, Hallmarks of cancer: the next generation, Cell, 2011, 144(5), 646–674 CrossRef CAS PubMed.
- D. Hanahan and R. A. Weinberg, The hallmark of cancer, Cell, 2000, 100, 57–71 CrossRef CAS PubMed.
- M. W. Epperly, J. A. Gretton and S. J. Defilippi,
et al., Modulation of Radiation-Induced Cytokine Elevation Associated with Esophagitis and Esophageal Stricture by Manganese Superoxide Dismutase-Plasmid/Liposome (SOD2-PL) Gene Therapy, Radiat. Res., 2001, 155(1), 2–14 CrossRef CAS PubMed.
- T. Hunter, Protein kinases and phosphatases: the yin and yang of protein phosphorylation and signaling, Cell, 1995, 80(2), 225 CrossRef CAS PubMed.
- J. L. Saloman, K. M. Albers, A. D. Rhim and B. M. Davis, Can Stopping Nerves, Stop Cancer?, Trends Neurosci., 2016, 39(12), 880 CrossRef CAS PubMed.
- I. Vivanco and C. L. Sawyers, The phosphatidylinositol 3-Kinase AKT pathway in human cancer, Nat. Rev. Cancer, 2002, 2(7), 489–501 CrossRef CAS PubMed.
- I. Vivanco and C. L. Sawyers, The phosphatidylinositol 3-Kinase|[ndash]|AKT pathway in human cancer, Nat. Rev. Cancer, 2002, 2(7), 489–501 CrossRef CAS PubMed.
- B. H. Jiang and L. Z. Liu, PI3K/PTEN Signaling in Angiogenesis and Tumorigenesis, Adv. Cancer Res., 2009, 102(1), 19–65 CrossRef CAS PubMed.
- F. J. Zwartkruis and J. L. Bos, Ras and Rap1: two highly related small GTPases with distinct function, Exp. Cell Res., 1999, 253(1), 157–165 CrossRef CAS PubMed.
- J. Bai, X. Zhang and K. Hu,
et al., Silencing DNA methyltransferase 1 (DNMT1) inhibits proliferation, metastasis and invasion in ESCC by suppressing methylation of RASSF1A and DAPK, Oncotarget, 2016, 7(28), 44129–44141 CrossRef PubMed.
- L. Xiang, G. Xie, J. Ou, X. Wei, F. Pan and H. Liang, The Extra Domain A of Fibronectin Increases VEGF-C Expression in Colorectal Carcinoma Involving the PI3K/AKT Signaling Pathway, PLoS One, 2012, 7(4), e35378 CrossRef CAS PubMed.
- W. Ma, T. Zhang and J. Pan,
et al., Assessment of insulin-like growth factor 1 receptor as an oncogene in esophageal squamous cell carcinoma and its potential implication in chemotherapy, Oncol. Rep., 2014, 32(4), 1601–1609 CrossRef CAS PubMed.
- K. L. Kong, D. L. Kwong and T. H. Chan,
et al., MicroRNA-375 inhibits tumour growth and metastasis in oesophageal squamous cell carcinoma through repressing insulin-like growth factor 1 receptor, Gut, 2012, 61(1), 33–42 CrossRef CAS PubMed.
- H. Dong, J. Xu and W. Li,
et al., Reciprocal androgen receptor/interleukin-6 crosstalk drives oesophageal carcinoma progression and contributes to patient prognosis, J. Pathol., 2016, 241, 4 Search PubMed.
- Y. Guo, F. Xu, T. Lu, Z. Duan and Z. Zhang, Interleukin-6 signaling pathway in targeted therapy for cancer, Cancer Treat. Rev., 2012, 38(7), 904–910 CrossRef CAS PubMed.
- M. Katoh and H. Nakagama, FGF receptors: cancer biology and therapeutics, Med. Res. Rev., 2014, 34(2), 280–300 CrossRef CAS.
- A. Beenken and M. Mohammadi, The FGF family: biology, pathophysiology and therapy, Nat. Rev. Drug Discovery, 2009, 8(3), 235–253 CrossRef CAS PubMed.
- N. Agrawal and J. N. Myers, Exome Sequencing of Head and Neck Squamous Cell Carcinoma Reveals Inactivating Mutations in NOTCH1, Science, 2016, 333(6046), 1154 CrossRef PubMed.
- S. Samantaray, R. Sharma, T. K. Chattopadhyaya, S. D. Gupta and R. Ralhan, Increased expression of MMP-2 and MMP-9 in esophageal squamous cell carcinoma, J. Cancer Res. Clin. Oncol., 2004, 130(1), 37–44 CrossRef CAS.
- G. Acs, P. Acs and S. M. Beckwith,
et al., Erythropoietin and erythropoietin receptor expression in human cancer, Cancer Res., 2001, 61(9), 3561–3565 CAS.
- B. Das, H. Yeger and R. Tsuchida,
et al., A hypoxia-driven vascular endothelial growth factor/Flt1 autocrine loop interacts with hypoxia-inducible factor-1alpha through mitogen-activated protein kinase/extracellular signal-regulated kinase 1/2 pathway in neuroblastoma, Cancer Res., 2005, 65(16), 7267–7275 CrossRef CAS PubMed.
Footnotes |
† Electronic supplementary information (ESI) available. Supplementary Fig. 1: Flowchart of bioinformatics analysis methods and the different bioinformatics tools are described. DMGs: differentially methylated genes. Supplementary Fig. 2: GO protein network of the hub DMGs. The round show the hub genes. GO terms are labeled by their GO IDs. The degree of color saturation of each round is positively associated with the enrichment significance of the corresponding GO term and the hub DMGs, respectively. (A) GO terms from biological processes. (B) GO terms from cellular components. (C) GO terms from molecular functions. Supplementary Fig. 3: The correlations between mRNA methylation and gene expression of the hub DMGs, P-values < 0.05 (*) have statistical significance. See DOI: 10.1039/c8mo00218e |
‡ These authors contributed equally to this work. |
|
This journal is © The Royal Society of Chemistry 2019 |
Click here to see how this site uses Cookies. View our privacy policy here.