Simulating graphene oxide nanomaterial phototransformation and transport in surface water†
Received
27th September 2018
, Accepted 15th November 2018
First published on 23rd November 2018
Abstract
The production of graphene-family nanomaterials (GFNs) has increased appreciably in recent years. Graphene oxide (GO) has been found to be the most toxic nanomaterial among GFNs and, to our knowledge, no studies have been conducted to model its fate and transport in the environment. Lab studies show that GO undergoes phototransformation in surface waters under sunlight radiation resulting in formation of photoreduced GO (rGO). In this study, the recently updated Water Quality Analysis Simulation Program (WASP8) is used to simulate time-dependent environmental exposure concentrations of GO and its major phototransformation product, rGO, for Brier Creek, GA, USA at two flow scenarios under a constant loading of GO to the river for a period of 20 years. Analysis shows that the degree of phototransformation is closely associated with river flow condition: up to of 40% of GO undergoes phototransformation at low flow condition, whereas only 2.5% of GO phototransformation occurs at mean flow condition. River flow and heteroaggregation exhibit a ‘competing’ effect in determining the formation of rGO heteroagglomerates. Mass fraction analysis indicates that the vast majority of rGO heteroagglomerates settle to the sediment layers due to the settling of suspended solids. Simulation of natural recovery after removal of the GO source suggests that free GO and rGO are the immediate contaminants of concern in the studied surface water system, while rGO heteroaggregated with suspended solids can have a long-term ecological impact on both the water column and sediments.
Environmental significance
There has been growing interest in simulating the fate and transport of engineered nanomaterials in the environment. The Water Quality Analysis Simulation Program (WASP) is one of the most widely used water quality models and has recently been upgraded to WASP8. WASP8 incorporates the algorithms to simulate the fate and transport of nanoparticles in surface waters. This study simulates the fate and transport of graphene oxide (GO) nanomaterials and their major phototransformation product, photoreduced graphene oxide (rGO), for Brier Creek, GA, USA. We specifically explored the influences of three important processes on the fate and transport of GO: (1) light attenuation, (2) phototransformation, and (3) heteroaggregation, and simulated their distributions in the river, including the water column and sediment.
|
1. Introduction
Engineered nanomaterials (ENMs) have been used in a wide range of applications in recent years.1–8 Among these ENMs, graphene-family nanomaterials (GFNs) – including pristine graphene, graphene oxide (GO), and reduced graphene oxide (rGO) – have become some of the fastest growing in the nanomaterials industry. Production of GFNs was estimated to be approximately 16t in 2009 and increased to 200t in 2011.9 Surfaces of graphene and rGO are mainly composed of a hydrophobic graphite lattice, and thus tend to undergo homoaggregation due to hydrophobic interactions, while GO's surface is enriched with carboxyl, hydroxyl, and epoxy groups, enabling GO nanomaterial to form stable aqueous suspensions.10,11 Among GFNs, GO has broad applications in the electronic, medical, energy, and environmental remediation industries.11–15 Rapid expansion of GO production increases the likelihood of its release into the environment. Several studies also report that GO was the most toxic species among GFNs.16–20 Therefore, to further assess potential GO impacts on the environment, it is imperative to understand and provide predicted exposure concentrations (PECs) of GO and its primary transformation product (i.e., rGO).21 Having field measurements of ENMs would be a powerful tool for evaluating PECs and distribution of ENMs in the environment. Owing to limitations of sampling and analytical technologies, however, it is still difficult to conduct in situ measurement of ENMs in the environment.22,23 Consequently, estimation of PECs distribution in the environment still relies heavily on mathematical models. Most models currently applied to predict ENM concentrations in the environment focus on metal ENMs.24–28 To our knowledge, few studies have simulated the environmental fate of carbon-based ENMs (e.g., carbon nanotubes and GFNs), and no study has modeled fate and transport of GO in the environment.
ENM environmental models fall into two classes: material flow analysis (MFA) and environmental fate and transport (F&T) models. The MFA approach developed by Nowack's research group tracks life-cycles of ENMs from their production in manufacturing to the compartments where nanomaterials are finally disposed (e.g., air, water, and soil).28–31 MFA approaches treat model parameters as probability distributions and use a probabilistic material flow analysis to account for the large uncertainties and variabilities in ENM production amount and behavior. MFA is a powerful model to analyze environmental flows of ENMs at a regional scale and has been applied to predict ENM concentration distributions in Europe and the U.S.30,32 MFA cannot sufficiently predict PECs of ENMs in the environment, however, because it does not incorporate specific governing processes. Environmental F&T models can successfully simulate organic and inorganic contaminants in surface waters, groundwater, sediments, and air. Environmental F&T models for predicting fate of ENMs in aquatic environments have been developed in recent years,24,27,33–35 yet there are still challenging issues regarding environmental F&T modeling of ENMs which must be addressed. One issue is the appropriate incorporation of ENM-specific fate descriptors into environmental F&T models. Specific ENM fate processes include oxidation, sulfidation, dissolution, phototransformation, homoaggregation, and heteroaggregation; ENMs' reaction products may need to be modeled if they have effects on ecosystem organisms (e.g., rGO, metal ions, metal sulfides). Recent models have incorporated approaches from colloid science to model heteroaggregation of ENMs with solids,24,36,37 but have not tracked the fate of ENMs' reaction products in the environment.
The Water Quality Analysis Simulation Program (WASP) is a dynamic, mass-balance, spatially resolved differential fate and transport modeling framework for environmental contaminants in surface waters and sediments. The recently updated WASP (WASP8) has a redesigned architecture and incorporates algorithms to simulate the fate of nanoscale and microscale emerging contaminants in surface waters (e.g., nanomaterial's reactions, heteroaggregation).38 WASP8 can also be linked to spatial analysis and modeling tools (e.g., BASINS, ArcGIS), which can greatly facilitate spatiotemporally explicit modeling and conducting large-scale watershed modeling studies. Previous laboratory studies demonstrated that GO undergoes phototransformation in surface waters, resulting in the production of rGO, carbon dioxide, and other less concentrated low-molecular weight products.21 Here, we develop a fate and transport model to predict environmental exposure concentrations of GO and its major reaction phototransformation product rGO for Brier Creek, GA, using WASP8. We investigate the influence of sunlight radiation on distributions of GO and rGO in Brier Creek over a 20-year period and track the fate of these two species in the water column and sediments. Owing to the rapid production of GFNs, as well as lack of environmental models to predict GO fate in surface waters, this work aims to develop critical insights into the environmental fate of released GO and rGO in surface waters and provide valuable information for risk assessment for GO in aquatic environments.
2. Methods and model descriptions
Site description and model design
Brier Creek, GA, located in the eastern US coastal plain, consisting of a mix of urban, agricultural cropland, grassland, forest, and wetland, was selected to investigate GO fate and transport. The river model was established from Bouchard et al.38 using WASP8 and adjusted to this study. Geometry parameters for Brier Creek were parameterized into WASP8 using the BASINS GIS Tool (BASINS 4.1, US EPA), which was used for river segmentation. The river of interest is divided into 12 segments along the main stream, plus two tributaries (Reedy and Brushy Creeks), as shown in Fig. 1. Each segment contains three compartments vertically, including the water column, the surface sediment layer, and subsurface sediment layer. The 12 stream segments have a mean length of 10 km. In order to fully assess the effect of hydrologic conditions on the GO phototransformation process, two flow conditions, the mean flow condition and the low flow condition, were simulated. Daily flowrate data were obtained from USGS gage 02197830 over 7 years from January 1st 1992 to December 31st 1999 because daily flowrate is only available during this period. HSPF (Hydrological Simulation Program-Fortran, WinHSPF 3.0 US EPA) was used to calibrate the flowrate to the observed flowrate data from USGS gage 02197830. The mean flow condition is the mean flowrate in each segment that represents the general hydrologic condition, and the low flow condition is the lowest flowrate in each segment that reflects droughts and typical low flow summer conditions, all based on HSPF simulation. Detailed procedures about HSPF calibration and flowrate calculation for both flow conditions are available in ESI 1.1.† Suspended solids concentration and sediment concentration data were obtained from USGS gage 02197830. Before running nanomaterial simulations, the Brier Creek WASP8 model was run for the sediment concentration to reach steady state. Dissolved organic carbon (DOC) and other water chemistry parameters were measured as in Bouchard et al.38 River stream segmentation information, river channel geometry data, calibration of sediment transport and flow rate, water quality and chemistry data, and hydraulic residence time of Brier Creek in each segment are available in ESI 1.1–1.3.† Model output interval is set at 1 day. In this study, we assume that GO released into Brier Creek is from a wastewater treatment plant (WWTP) effluent. The mass flow method is usually used to estimate the release of ENMs into environmental media;23,28,30,39 however, sufficiently detailed GO mass flow data are not readily available. Particularly, the mass flow data from GO production to application are scarce. Bouchard et al.38 simulated the fate and transport of multi-walled carbon nanotubes (MWCNTs) in surface waters, using a mass loading of 0.1 kg d−1 estimated based on the mass flow method. Because MWCNTs and GO have similar applications, we assume a similar release of 0.1 kg d−1 for GO.
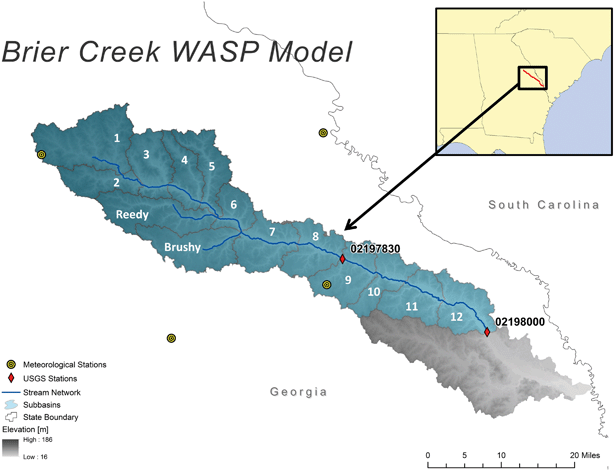 |
| Fig. 1 Model segmentation for Brier Creek, GA, USA. The river of interest was divided into 12 segments along the main stem with two tributaries (Reedy and Brushy Creeks).38 | |
Light attenuation model
WASP8 incorporates light attenuation and phototransformation modules. The GO phototransformation rate constant in surface waters is calculated using the average light intensity in the water body, which is affected by light attenuation. WASP8 divides the sunlight spectrum into 11 specific wavelength bands, including ultraviolet, visible, and infrared, mainly owing to the fact that these wavelengths can drive specific environmental processes.40–45 The partitioning of the sunlight spectrum into wavelength bands and the default attenuation coefficient values for each specific band implemented in WASP8 are shown in Table 1. The default attenuation coefficient values for each specific band are based on those used in U.S. EPA's exposure analysis modeling system (EXAMS) model.46 In our study, we only consider the impacts of ultraviolet and visible light on the GO phototransformation because infrared light is not photochemically reactive.
Table 1 Sunlight spectrum partitioning, water attenuation background coefficient, DOC attenuation coefficient, suspended solids attenuation coefficient and energy fraction of each wavelength band
Sunlight spectrum group |
Wavelength bands specification |
Water background attenuation coefficient (m−1) |
DOC attenuation coefficient (m−1 mg−1 L) |
Solids attenuation coefficient (m−1 mg−1 L) |
Energy fraction of each band of sunlight radiation at 30° N |
Ultraviolet |
UVb medium (295–304 nm) |
0.1510 |
6.220 |
0.34 |
0.00009 |
UVb high (305–314 nm) |
0.1090 |
5.400 |
0.34 |
0.00110 |
UVa low (315–334 nm) |
0.0805 |
4.590 |
0.34 |
0.00768 |
UVa medium (335–354 nm) |
0.0512 |
3.400 |
0.34 |
0.01074 |
UVa high (355–379 nm) |
0.0340 |
2.540 |
0.34 |
0.01639 |
Visible |
Violet (380–449 nm) |
0.0169 |
0.300 |
0.34 |
0.07527 |
Blue (450–494 nm) |
0.0166 |
0.300 |
0.34 |
0.06656 |
Green (495–569 nm) |
0.0475 |
0.300 |
0.34 |
0.10420 |
Yellow-orange (570–619 nm) |
0.2170 |
0.300 |
0.34 |
0.06581 |
Red (620–749 nm) |
1.0070 |
0.300 |
0.34 |
0.15217 |
Infrated light |
Infrared (750–2500 nm) |
2.0700 |
0.000 |
0.34 |
0.50000 |
The attenuation of sunlight radiation through the water column is influenced by the light absorption of the water medium and relative optical path in the water body. The optical path parameter depends on the angle of incidence of the light source, and we assume an optical path parameter of 1. The attenuation in the water column is quantified as the diffuse attenuation coefficient (Ke,λ in m−1),47–49 which results from background attenuation from water, dissolved organic carbon (DOC), and suspended solids (SS). The diffuse attenuation coefficient is expressed as
| Ke,λ = Kw,λ + KDOC,λ[DOC] + KSS,λ[SS] | (1) |
where
Kw,λ is the water background attenuation coefficient,
KDOC,λ is the DOC attenuation coefficient, and
Ksolid,λ is the SS attenuation coefficient. The subscript
λ emphasizes that each wavelength has a specific value for the different absorption coefficients, as presented in
Table 1, and each wavelength band has a specific diffuse attenuation coefficient at a given time. [DOC] represents total DOC concentration (as carbon mg L
−1) in the water column at a given time; and [SS] is SS concentration (mg L
−1) in the water column at a given time. [DOC] and [SS] at a given time can be calculated by WASP8, based on their initial conditions and boundary conditions, which are WASP8 inputs and available in ESI 1.
† We sampled Brier Creek water samples and measured DOC concentration. Mean DOC concentration in Brier Creek was measured as 5.6 mg L
−1, which is the initial condition and boundary condition set in WASP8 in this study.
Light attenuation is modeled according to the Beer–Lambert equation,50 where light intensity at a given time of each wavelength at the bottom of the water column after transmission through a homogeneous layer of depth z is expressed as
| Iz,λ = (I0kband,λ) exp (−Ke,λz) | (2) |
where
I0 is the solar radiation intensity (W m
−2) at the water surface at a given time,
Ke,λ is the diffuse attenuation coefficient (m
−1), and
z is water column depth (m). The data for
I0 were obtained from the North American Land Data Assimilation System (NLDAS) website by inputting the coordinates of Brier Creek and the period of simulation. Hourly sunlight solar radiation intensity on the Brier Creek surface (
I0) for 20 years, from 1997 to 2016, was used. The method to retrieve sunlight radiation intensity at the Brier Creek surface is described in ESI 2.1.
† The
kband,λ represents the energy fraction of each specific wavelength of total sunlight solar radiation (
Table 1). The values of
kband,λ are different at different latitudes, and the method to determine
kband,λ is available in ESI 2.2.
†
The average light intensity of each wavelength in a completely-mixed water column of depth z is calculated as
| 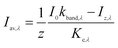 | (3) |
The sunlight spectrum is divided into 10 specific wavelength bands, so there are 10 average light intensity bands at a given time for a specific stream segment. A sample calculation of Iav,λ is provided in ESI 2.3.†
Total average light intensity in the water column can be calculated by summing the average light intensity of each wavelength band together, which is expressed by
| 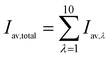 | (4) |
WASP8 internally calculates Ke,λ, Iav,λ and Iav,total; solar intensity at the water surface (I0) is a WASP8 input parameter.
GO phototransformation module
In WASP8, phototransformation is driven by direct photolysis, and OECD 316 guidelines51 were followed to develop WASP8's phototransformation module. The GO phototransformation rate constant in surface waters is calculated using the average light intensity in the water body, as described by Zepp and Cline.52 Considering the heterogeneity of GO structures, the phototransformation rate constant is quantified by measuring the change in DOC concentration in simulated sunlight irradiation, as has been previously reported.21 The measured DOC was as assumed to be a combination of the DOC from two constituents: GO and rGO. By assuming that the change in measured DOC at 19 h is due to the loss of carbon dioxide during the formation of rGO, the reaction yield of GO to rGO was calculated to be 0.55 gram-Carbon rGO/g-Carbon GO based on the initial and final DOC data. Under the assumption that 1 unit of GO is reduced to 1 unit of rGO, along with the loss of carbon as CO2, a pseudo-first-order photoreduction rate constant kobs of 0.062 h−1 was obtained by minimizing the sum of squared errors between measured and predicted data (Fig. S3†).
To separate the contribution of different wavelengths, the quantum yield for phototransformation of GO is held constant over 295–750 nm. Combining the absorbed fraction of light by the GO suspension and the spectral light intensity of the solar simulator (Fig. S4†), the total photon flux (Pa) applied to the 40 mg L−1 GO solution is 2.54 × 1016 quanta (cm−2 s−1), calculated by
| 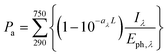 | (5) |
where
Iλ is the flux of light that enters the system from the solar simulator (W cm
−2 nm
−1);
Eph,λ is the energy of a photon of wavelength
λ (kg m
2 s
−2); and
aλ is the absorption coefficient of GO solution at wavelength
λ (cm
−1), and
L is the average light path length of quartz tubes (∼1 cm).
The average contribution of a single photon to the observed photoreduction rate constant, kph, is 6.83 × 10−22 cm2 quanta−1, as calculated by dividing kobs by Pa. The wavelength-dependent reaction rate constant as a function of light intensity per specific wavelength band can then be determined by
| 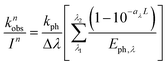 | (6) |
where
knobs is the resulted reaction rate constant (h
−1) due to the irradiation of
In, which is the total light intensity in the bandwidth from
λ1 to
λ2. Δ
λ is the width of band in nm. The calculated wavelength-dependent reaction rate

for each wavelength is listed in Table S10;
† these are used as WASP8 input parameters.
The phototransformation rate constant for each wavelength band is calculated as
|  | (7) |
and the overall phototransformation rate constant is expressed as
| 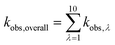 | (8) |
GO phototransformation and rGO production are expressed as follows
| 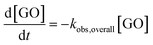 | (9) |
| 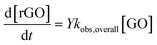 | (10) |
where [GO] and [rGO] are GO and rGO concentrations in the water column (ng L
−1), and
Y is the reaction yield (0.55 g-Carbon rGO/g-Carbon GO). WASP8 calculates
kobs,λ,
kobs,overall, [GO], and [rGO] internally based on
and
Y, which are WASP8 inputs.
GO and rGO fate and transport modeling in surface waters
Phototransformation and heteroaggregation are important processes in determining the fate of GO in surface waters.21,53 Phototransformation of GO can result in formation of rGO, and heteroaggregation can result in formation of new heteroaggregates. Important GO and rGO physicochemical parameters are available in Table S11.† Batch experiments were conducted in Brier Creek water samples to evaluate GO/rGO's heteroaggregation attachment efficiencies with Brier Creek sediment. Detailed experimental procedures and results are available in ESI 3.† Heteroaggregation between ENMs and natural suspended solids is acknowledged as the predominant process governing the fate of ENMs in aquatic environments.54,55 Homoaggregation between ENMs in natural surface waters is negligible however,55–58 and therefore is not implemented in WASP8. The modeling approach for simulating heteroaggregation in WASP8 follows the approach described by Praetorius et al.,24 and utilized to simulate heteroaggregration of multi-walled carbon nanotubes in Brier Creek.38 In this approach, ENMs collision with suspended solids is based on particle number rather than mass. Information on the heteroaggregation modeling methods are thoroughly described in ESI 6.† This modeling approach is based on three key assumptions: (1) heteroaggregation is a kinetically controlled process, (2) heteroaggregation between ENMs and suspended solids (SS) is an irreversible process, and (3) free (unaggregated) nanomaterial does not settle from surface waters. Three suspended solids (SS), including silt, clay, and particulate organic matter (POM), were simulated by WASP8. Sand has a fast settling velocity due to its large size, so we assume that sand settles to the bottom of water column immediately as soon as it enters Brier Creek. Given this assumption, sand is not presented in the water column, nor does it resuspend back to the water column from the sediment. Since sand is not present in the water column, the transport of sand was not simulated. Table S3–S5† show the parameters of solids in the water column and sediments implemented in WASP8. GO or rGO heteroaggregated with SS is denoted as GO-SS or rGO-SS. We assume that the transport of GO- or rGO-SS is governed by the suspended solids that GO or rGO are associated with, because the size of SS is at least five orders of magnitude larger than GO or rGO. Besides heteroaggregation, advective flow, deposition of SS and ENMs (e.g., GO, rGO, GO- and rGO-SS) from the water column to sediment, resuspension from sediment to the water column, and sediment burial are all important processes that govern GO and rGO fate. The algorithms to represent each of the processes governing GO and rGO fate have been incorporated into WASP8. Detailed WASP8 modeling methods are described in a recent published paper.59
3. Results and discussion
Average light intensity in the water column and GO phototransformation rate constants under different flow conditions
The average light radiation in the water column after attenuation was simulated for 20 years (1997–2016) at two flow conditions. The change of flow conditions can cause changes of [SS], [DOC] and river depth, which determine the diffuse attenuation coeffcient and phototransformation rate based on eqn (1) to (10). The year 2016 is used to illustrate the influence of flow conditions upon average light intensity in the water column and GO phototransformation rate.
Since WASP divides the sunlight spectrum into ten specific wavelength bands, the average light intensity and the phototransformation rate constant in the water column are simulated for each specific wavelength. The diffuse attenuation coefficient values of each wavelength in the 12 stream segments in Brier Creek at the two flow conditions are shown in Tables S15 and S16.† Generally, the values of diffuse attenuation coefficients at the low condition are lower than as those at the mean flow condition. This difference is attributed to the fact that river depth is shallower (Tables S1 and S2†) and [SS] also is lower for the low flow condition (Fig. S15†). For both flow conditions, the values of diffuse attenuation coefficients in the ultraviolet light group are higher than those in the visible light group. Values of diffuse attenuation coefficients in different stream segments under the same wavelength are similar because [DOC] and [SS] (Table S3†) in all stream segments are similar. Fig. S16† shows contributions of water, DOC, and SS attenuation to the diffuse attenuation coefficient for each wavelength in the water column of stream segment 1. The ultraviolet light group and visible light group show contrasting results. In general, contributions from background water absorption are negligible from uVb med to visible green wavelengths. For yellow-orange and red bands, the contributions of water absorption background are 3% and 11%, respectively. For the five wavelength bands in the ultraviolet light group, the contribution from DOC to the diffuse attenuation coefficient is dominant, accounting for at least 80% for five specific wavelength bands. For the violet light group, SS is the primary factor attenuating the light in the water column. Another finding is that average light intensity in the water column gradually decreases from upstream to downstream (Fig. S2†) because river depth increases from upstream to downstream.
Stacked area charts present the average light intensity in the first segment for each wavelength band in the water column after attenuation (Fig. 3a and c), illustrating how each wavelength band contributes to total average light intensity. For total average light intensity, visible light contributes more than 99% of total average light intensity, whereas ultraviolet light accounts for less than 1% of total average light intensity in the water column (Fig. 3a and c). Phototransformation rate constants of GO in 2016 in the first segment, calculated based on eqn (7), nevertheless indicate an opposite trend: results show that the contribution of ultraviolet light to the total phototransformation rate constant is more than 99%, whereas the visible light contribution accounts for less than 1% (Fig. 3b and d). Analysis of the phototransformation rate constants reveals that ultraviolet light is the predominant photochemically active wavelength and ultraviolet light is therefore the primary driving force initiating GO photodegradation, which is consistent with previous studies on photochemical transformation.41,43,60,61 The values of average daily light intensity and phototransformation rate constant are almost twice as high under low flow conditions as those at mean flow due to the more shallow stream depth and lower total [SS] at the lower flow conditions.
[GO] and [rGO] in the water column
Laboratory results for GO and rGO stability in Brier Creek water are available in ESI 3.2.† Results indicate that neither homoaggregation nor heteroaggregation is significant for GO. Homoaggregation between rGO was not observed, while heteroaggregation between rGO and SS is observed to occur. Experimental results yielded an rGO heteroaggregation attachment efficiency (αhetagg) of 2 × 10−7 in Brier Creek water samples, and this value of αhetagg was used as an input parameter for the WASP8 simulations. The processes controlling the fate of GO and rGO in Brier Creek are summarized in Fig. 2. The rGO-SS parameters used in the simulations are available in Table S12.†
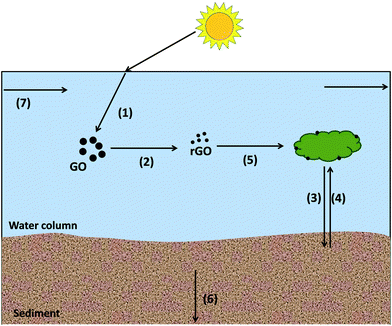 |
| Fig. 2 Conceptual model for the fate and transport of GO and rGO in Brier Creek. The governing processes for GO and rGO are: (1) sunlight attenuation, (2) phototransformation of GO to rGO, (3) deposition, (4) resuspension, (5) heteroaggregation between rGO and SS, (6) sediment burial, and (7) river flow. | |
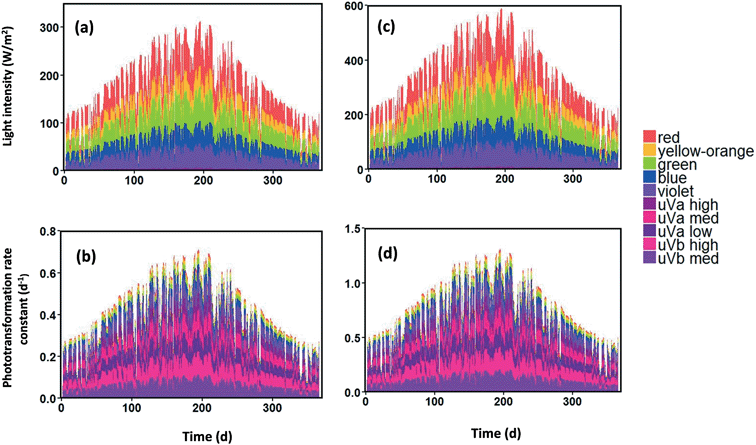 |
| Fig. 3 (a) The daily average light intensity (W m−2) in the water column of the upstream segment 1 in 2016 at the mean flow condition; (b) daily phototransformation rate constant (d−1) in the water column of the upstream segment 1 in 2016 at the mean flow condition; (c) the daily average light intensity (W m−2) in the water column of the upstream segment 1 in 2016 at the low flow condition; (d) daily phototransformation rate constant (d−1) in the water column of the upstream segment 1 in 2016 at the low flow condition. | |
[GO] and [rGO] in Brier Creek were simulated over 20 years at two flow conditions. To illustrate trends of [GO] and [rGO] in the water column for 20 years, concentrations in stream segments 1, 6, and 12, corresponding to upstream, midstream and downstream segments of Brier Creek are plotted versus time in Fig. 4a and b, corresponding to the mean flow and low flow conditions, respectively. In Fig. 4a and b, GO and rGO particle concentrations calculated from eqn (S7)† as well as their corresponding mass concentrations are presented. In general, [GO] in the water column exhibits a periodic variability throughout the year across the 20-year simulation period: [GO] gradually decreases from January, reaches a minimum concentration during the summer, and then gradually recovers (Fig. S10†). This result is consistent with the average light intensity trend in the water column over the year, as the phototransformation rate gradually increases and then declines as sunlight radiation intensity increases from the beginning of the year to summer, then decreases. [rGO] shows the opposite trend (Fig. S10†), which is expected because rGO is the direct product of GO phototransformation.
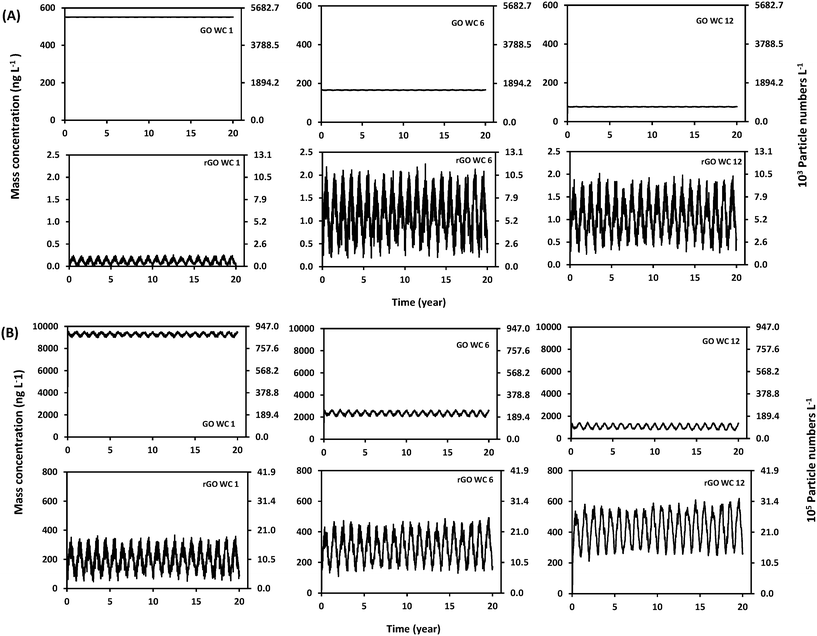 |
| Fig. 4 Free [GO] and [rGO] in the water column in stream segments 1, 6, and 12 over 20 years at two flow conditions. (A) Is mean flow condition, and (B) is low flow condition. WC represents the water column. Left y-axis is ENM mass concentration, and right y-axis is the ENM particle concentration corresponding to the mass concentration. | |
Comparing [GO] and [rGO] in Brier Creek at two different flow conditions, simulation results show that more GO photochemically transforms into rGO at the low flow condition (Fig. 4b). GO mass flowrate analyses in each river segment at two flow conditions indicates that phototransformation has a substantial effect on the decrease of [GO] in Brier Creek at the low flow condition: up to 40% of GO phototransforms in the 12th creek segment while only 2.5% of GO phototransformation occurs at mean flow condition (Fig. S9† and GO mass flowrate principle is presented ESI 5†). Compared to the mean flow condition, the high [rGO] in the water column at the low flow condition can be attributed to the decrease in stream depth (Tables S1 and S2†) and [SS] (Fig. S15†) and the resultant increase the phototransformation rate constant, and also to the increase in GO residence time in the stream segment that increases the amount of time for the GO to rGO reaction to occur. Specifically, the phototransformation rate constant at the low flow condition is twice as high as at the mean flow condition (Fig. 3(b) and (d)), and residence time at the low flow condition increases at least 7 times (Tables S1 and S2†).
Mean [GO] and [rGO] were calculated and plotted with 85% and 15% quantiles as a function of river distance for the 20-year period in Fig. 5. In general, [GO] gradually decreases from the ENMs' release point to the end of the river. At the mean flow condition, [rGO] increases from segment 1 to 5, decreases from segment 5 to 6, and slightly increases from segment 7 to 12. The drop of [GO] from segment 5 to 6 is likely due to three reasons: (1) Reedy Creek, a tributary of Brier Creek, flows into segment 6 that might significantly dilute [rGO], (2) residence time in segment 6 increases (Table S1†), and (3) the phototransformation rate in segment 6 also decreases compared to segment 5. At the low flow condition more rGO is photochemically produced, and [rGO] keeps increasing in each sequential river segment.
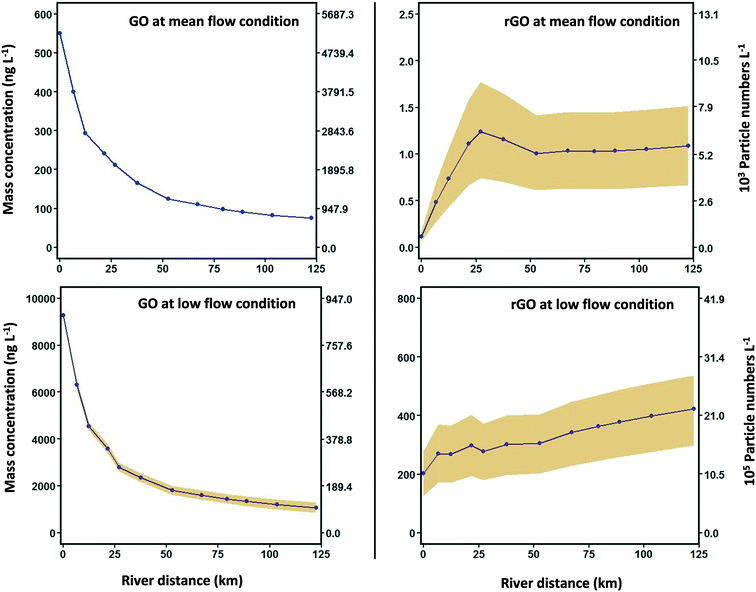 |
| Fig. 5 Free mean [GO] and [rGO] concentrations in the water column of Brier Creek as a function of river distance when the system reaches stationarity at the mean flow and low flow conditions. Lines indicate modeled mean [GO] and [rGO] in the water column over 20 years. Corresponding colored bands express the 15% and 85% quantiles of [GO] and [rGO] in the water column over 20 years, respectively. Left y-axis is ENM mass concentration, and right y-axis is the ENM particle concentration corresponding to the mass concentration. | |
Sensitivity analysis of heteroaggregation attachment efficiency (αhetagg) on rGO fate
GO is stable in surface waters due to its physicochemical properties, while rGO is likely to be destabilized in natural surface waters, as discussed in ESI 3.2.† In addition to hydrologic effects, the fate of rGO in Brier Creek is influenced by the heteroaggregation process. Therefore, the effects of varied rGO heteroaggregation attachment efficiency (αhetagg) values from 2 × 10−7 to 1 on [rGO] were investigated at the mean flow condition. An increase in the value of αhetagg from 2 × 10−7 to 2 × 10−3 for rGO has a negligible effect on [rGO] because no noticeable decrease of [rGO] is observed compared to the condition where no heteroaggregation occurs between rGO and SS (αhetagg is 0). A slight decline of [rGO] is found when the αhetagg value is equal to 2 × 10−1, and a large drop of [rGO] occurs when αhetagg is equal to 1, as shown in Fig. 6. When simulating [TiO2] in the Rhine River with αhetagg ranging from 0.001 to 1, a significant decline of [TiO2] in the river was observed as αhetagg increased.24 The simulation in Brier Creek did not exhibit this trend due to the different environmental and hydrologic conditions of Brier Creek compared to the Rhine River. From eqn (S4) and (S5) in ESI 6,† the heteroaggregation rate constant is not only determined by αhetagg but also is influenced by the size and settling velocity of the ENMs and suspended solids, and suspended solids concentration. Because sizes of different ENMs are usually similar, the heteroaggregation rate constant is more sensitive to variations of suspended solids concentration in surface waters than the size and settling velocity of ENMs, which has been demonstrated.56 Compared to Brier Creek, the Rhine River has a much higher suspended solids concentration (50 mg L−1), which can result in a much higher heteroaggregation rate constant, according to eqn S4 and S5 in ESI 6.†
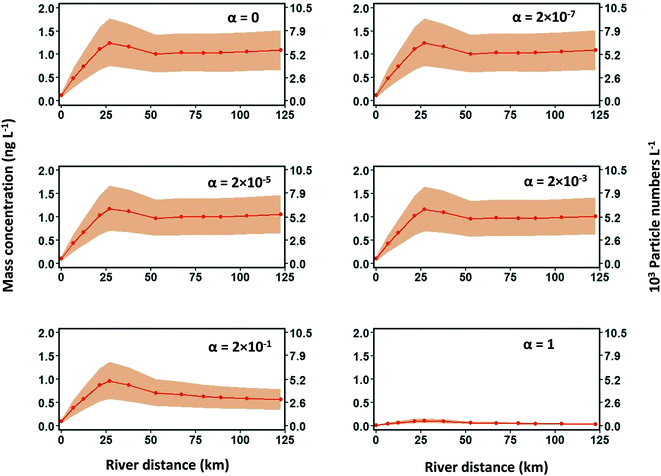 |
| Fig. 6 Free [rGO] in the water column as a function of river distance when system has reached stationarity with different values of heteroaggregation attachment efficiency (α) in the water column. Lines represent mean [rGO] in the water column over 20 years. Colored bands express the range between 15% and 85% quantiles of [rGO] in the water column over 20 years. Left y-axis is ENM mass concentration, and right y-axis is the ENM particle concentration corresponding to the mass concentration. | |
rGO can be removed from the water column either by river flow or by forming settleable heteroaggregates. Values of flowrate constant (eqn (S6)†) and heteroaggregation rate constant in each segment in Brier Creek at mean flow condition are presented in Table 2. It is clear that even though αhetagg increases from 2 × 10−7 to 2 × 10−3, the heteroaggregation rate constant is always at least two orders of magnitude lower than the flow rate constant, implying that rGO is primarily removed by river flow and rGO is flushed out of each segment before it can aggregate with SS. When αhetagg is 2 × 10−1, values of the heteroaggregation rate constant and flowrate constant are of the same order of magnitude while flowrate constants are still slightly higher than heteroaggregation rate constants, suggesting that both river flow and heteroaggregation contribute to the removal of rGO. When αhetagg increases to 1, [rGO] declines significantly (Fig. 6), and the heteroaggregation rate constant is higher than the river flow constant, indicating that heteroaggregation is the dominant process for removing rGO under this condition. To summarize, the formation of ENM heteroaggregates is determined by specific surface water parameters, including αhetagg, suspended solids concentration, and river flow rate.
Table 2 Values of river flow constant and heteroaggregation rate constant with varied heteroaggregation attachment efficiency in Brier Creek at mean flow condition
Segment no. |
River flow constant, s−1 |
Heteroaggregation rate constant when α is at 2.1 × 10−7, s−1 |
Heteroaggregation rate constant when α is at 2.1 × 10−5, s−1 |
Heteroaggregation rate constant when α is at 2.1 × 10−3, s−1 |
Heteroaggregation rate constant when α is at 2.1 × 10−1, s−1 |
Heteroaggregation rate constant when α is at 1, s−1 |
1 |
1.03 × 10−4 |
2.00 × 10−11 |
2.00 × 10−9 |
2.00 × 10−7 |
2.00 × 10−5 |
9.54 × 10−5 |
2 |
8.74 × 10−5 |
1.99 × 10−11 |
1.99 × 10−9 |
1.99 × 10−7 |
1.99 × 10−5 |
9.48 × 10−5 |
3 |
1.08 × 10−4 |
1.98 × 10−11 |
1.98 × 10−9 |
1.98 × 10−7 |
1.98 × 10−5 |
9.45 × 10−5 |
4 |
7.08 × 10−5 |
1.97 × 10−11 |
1.97 × 10−9 |
1.97 × 10−7 |
1.97 × 10−5 |
9.40 × 10−5 |
5 |
9.25 × 10−5 |
1.97 × 10−11 |
1.97 × 10−9 |
1.97 × 10−7 |
1.97 × 10−5 |
9.37 × 10−5 |
6 |
5.38 × 10−5 |
1.96 × 10−11 |
1.96 × 10−9 |
1.96 × 10−7 |
1.96 × 10−5 |
9.33 × 10−5 |
7 |
4.11 × 10−5 |
1.96 × 10−11 |
1.96 × 10−9 |
1.96 × 10−7 |
1.96 × 10−5 |
9.33 × 10−5 |
8 |
4.15 × 10−5 |
1.96 × 10−11 |
1.96 × 10−9 |
1.96 × 10−7 |
1.96 × 10−5 |
9.32 × 10−5 |
9 |
5.31 × 10−5 |
1.96 × 10−11 |
1.96 × 10−9 |
1.96 × 10−7 |
1.96 × 10−5 |
9.32 × 10−5 |
10 |
7.02 × 10−5 |
1.95 × 10−11 |
1.95 × 10−9 |
1.95 × 10−7 |
1.95 × 10−5 |
9.30 × 10−5 |
11 |
4.61 × 10−5 |
1.95 × 10−11 |
1.95 × 10−9 |
1.95 × 10−7 |
1.95 × 10−5 |
9.30 × 10−5 |
12 |
3.55 × 10−5 |
1.95 × 10−11 |
1.95 × 10−9 |
1.95 × 10−7 |
1.95 × 10−5 |
9.29 × 10−5 |
[rGO-SS] in sediments
Due to their physicochemical properties and subsequent colloidal stability, free GO and rGO are present in the water column. While no heteroaggregation was observed for GO at the mean flow condition, approximately 1% of rGO heteroaggregates with suspended solids in the water column and is removed from the water column due to settling. Three types of [rGO-SS] profiles in the surface and subsurface layers in stream segment 1 (upstream), 6 (midstream), and 12 (downstream) at the mean flow condition over 20 years, respectively, are shown in Fig. S14.† The general trend of [rGO-SS] in the sediment shows an increase as a function of time in each segment. rGO-silt has the highest concentration among the three types of rGO-SS particles, and [rGO-silt], [rGO-clay], and [rGO-POM] in sediments gradually increase from the point of release to the end of the river. The mass fraction of rGO-POM, rGO-clay and rGO-silt in the surface sediment layer and subsurface sediment layer as a function of time are shown in Fig. S11.† Mass fractions of these three types of rGO-SS do not vary from upstream to downstream in the two sediment layers: rGO-silt has the highest mass fraction among the three types of rGO-SS species in sediment layer, accounting for about 50% in the whole river, while mass fractions of rGO-clay and rGO-POM are approximately 30% and 20%, respectively.
A 100-year recovery simulation, in which the GO source is removed after 20 years of continuous GO loading, also suggests that rGO-SS sediment residence times can be long: rGO-SS in the surface sediment layer has a residence time around 20 years due to the burial process, and resident time of rGO-SS in the subsurface layers is even longer, i.e., the resident time of rGO-SS in segment 6 and 12 is more than 80 years (Fig. S14†). Again, due to the resuspension of solids from sediment to the water column, the three types of rGO-SS are still present in the water column after GO loading into the river stops, although their concentrations are extremely low (Fig. S13†).
GO, rGO, and rGO-SS mass distribution in Brier Creek
The simulated mass fraction distribution of GO, rGO and rGO-SS as a function of time in stream segments 1, 6, and 12 over 20 years is illustrated in Fig. 7. At the mean flow condition, GO is predominant among all modeled species throughout the river, including the water column and sediments, accounting for more than 99% of total ENM mass. GO is entirely in the water column due to its colloidal stability, i.e., it doesn't heteroaggregate. rGO species, including free rGO and three types of rGO-SS, account for a maximum of 1% of total ENM mass. Free rGO is the predominant rGO species and accounts for 99% of rGO ENM mass, including free rGO and rGO-SS. All free rGO is also present in the water column, whereas rGO-SS species are found in both the water column and in the sediments, but predominantly in the two sediment layers. Three kinds of rGO-SS initially accumulate in the surface sediment, but the mass fraction of rGO-SS in the subsurface sediment gradually increases; in segment 1, mass fraction of rGO-SS in the subsurface sediment eventually becomes more than that in the surface sediment, owing primarily to the burial process from surface sediment to subsurface sediment.
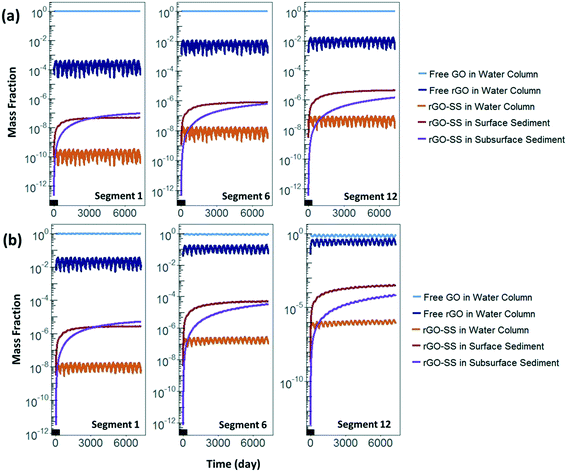 |
| Fig. 7 Daily mass fractions of GO, rGO and rGO-SS in different compartments in stream segments 1, 6, and 12 over 20 years. (a) Mean flow condition, and (b) low flow condition. rGO-SS is the sum of mass of rGO-silt, rGO-clay and rGO-POM, respectively. | |
At the low flow condition, GO is the dominant species in the 1st segment, while the rGO mass fraction increases to approximately 0.1 in the 6th segment, and approximately 0.4 in the 12th segment. Similar to the mean flow condition, rGO-SS species are predominantly present in the sediments. The values of rGO-SS mass fraction in two sediment layers and in the water column increase two orders of magnitude compared to those at the mean flow condition, as presented in Fig. 7.
Environmental implications
An ENM environmental fate and transport model is fundamentally different from an organic solute fate and transport model in describing contaminant-solids interactions. Organic solutes in the environment can reach thermodynamic equilibrium, and equilibrium partitioning coefficients, such as the octanol–water partition coefficient (Kow) or the soil–water distribution coefficient (Kd), are usually applied to simulate solute-solids interactions.62–65 ENMs environmental fate and transport modeling is based on colloid transport theory, and the key tenant of that theory is that ENMs in the environment are thermodynamically unstable suspensions and their behavior is governed by a kinetic attachment process.24,66–69 As a result, the ENM fate model in WASP8 is programmed following the particle balance principle based on colloid science, whereas solute contaminant fate modeling is implemented following the mass balance approach.
Due to the large-scale commercial production of GO and the lack of available analytical methods to quantify [GO] and [rGO] in the environment, it is important to develop mathematical models to predict the fate and transport of GO and its reaction products in the environment. Lab studies have demonstrated that GO undergoes phototransformation under sunlight resulting in the formation of rGO. rGO production from GO is not only related to the sunlight solar radiation intensity at the water surface, but also is determined by water body hydrology. For our hypothetical scenario with a 20-year steady loading to the river, it is not possible for GO and rGO concentrations to reach steady-state in the water column due to the variation in seasonal and hourly sunlight radiation; rather, GO and rGO concentrations in the water column are shown to continually fluctuate around a mean value.
The degree of GO phototransformation depends on light intensity in the water column and flow conditions because light intensity in the water column directly initiates phototransformation, and river flow conditions affect suspended solids and DOC concentrations, river depth, and residence time in the stream. At the mean flow condition, the degree of GO phototransformation is low, yet it increases notably under low flow conditions because light attenuation decreases as the river depth decreases, total suspended solids concentration drops, and hydrology residence time increases. Formation of rGO-SS is affected by the heteroaggregation process and river flow, and our analysis suggests that river flow is the primary factor to remove free rGO from the water column in Brier Creek. A small fraction of rGO is associated with SS due to the heteroaggregation process under both flow conditions, but the vast majority of rGO-SS settles to the bottom sediments. Recovery simulation shows that if the loading of GO to the river is stopped, the three types of rGO-SS can be present at low concentrations in the water column and in sediments for decades.
Compared to metal NPs (e.g., ZnO NPs, Ag NPs),25,26,35,70–74 GO does not undergo dissolution, oxidation, and sulfidation reactions, and the fate of GO and its reaction product is appreciably different. Based on our modeling study, free GO should be the primary species of concern in surface waters with limited potential for heteroaggregation between GO and suspended solids. For water bodies with high photoreaction potential (shallow, low suspended solids, long residence times) significant phototransformation of GO to rGO can occur. The fate of the [rGO] photoproduct and formation of rGO-SS heteroaggregates in the water column are in turn affected by heteroaggregation and river flow conditions. rGO-SS initially accumulates in surface sediment, with gradual transport into the deep sediment layer.
Other processes such as indirect photoreactions involving reactive transients produced by sunlight irradiation of water constituents are also likely to be involved with degradation of GO over longer time periods.75 Simulation of natural attenuation (Fig. S12–S14†) indicates that free GO and rGO can be completely removed from the water column shortly after the cessation of GO loading into the river, however, three types of rGO-SS remain present in the water column and sediments for a long period of time. In this study the environmental fate of GO in Brier Creek is simulated. To fully understand and assess GO fate, other surface waters systems should be explored. Previous studies indicate that GO tends to undergo homoaggregation at high ionic strengths, so marine bays and estuaries with spatially variable ionic strengths are possible choices for further study.
Disclaimer
This paper has been reviewed in accordance with the U.S. Environmental Protection Agency's peer and administrative review policies and approved for publication. Mention of trade names or commercial products does not constitute endorsement or recommendation for use. The views expressed in this article are those of the authors and do not necessarily represent the views or policies of the USEPA.
Conflicts of interest
There are no conflicts to declare.
References
- T. M. Tolaymat, A. M. El Badawy, A. Genaidy, K. G. Scheckel, T. P. Luxton and M. Suidan, An evidence-based environmental perspective of manufactured silver nanoparticle in syntheses and applications: a systematic review and critical appraisal of peer-reviewed scientific papers, Sci. Total Environ., 2010, 408, 999–1006 CrossRef CAS PubMed.
- C.-N. Lok, C.-M. Ho, R. Chen, Q.-Y. He, W.-Y. Yu, H. Sun, P. K.-H. Tam, J.-F. Chiu and C.-M. Che, Silver nanoparticles: partial oxidation and antibacterial activities, JBIC, J. Biol. Inorg. Chem., 2007, 12, 527–534 CrossRef CAS PubMed.
- A. Weir, P. Westerhoff, L. Fabricius, K. Hristovski and N. Von Goetz, Titanium dioxide nanoparticles in food and personal care products, Environ. Sci. Technol., 2012, 46, 2242–2250 CrossRef CAS PubMed.
- Y. Han and W. Yan, Reductive Dechlorination of Trichloroethene by Zero-valent Iron Nanoparticles: Reactivity Enhancement through Sulfidation Treatment, Environ. Sci. Technol., 2016, 50, 12992–13001 CrossRef CAS PubMed.
- D. Zhang, K. Ryu, X. Liu, E. Polikarpov, J. Ly, M. E. Tompson and C. Zhou, Transparent, conductive, and flexible carbon nanotube films and their application in organic light-emitting diodes, Nano Lett., 2006, 6, 1880–1886 CrossRef CAS PubMed.
- S. Das, J. M. Dowding, K. E. Klump, J. F. McGinnis, W. Self and S. Seal, Cerium oxide nanoparticles: applications and prospects in nanomedicine, Nanomedicine, 2013, 8, 1483–1508 CrossRef CAS PubMed.
- J. W. Rasmussen, E. Martinez, P. Louka and D. G. Wingett, Zinc oxide nanoparticles for selective destruction of tumor cells and potential for drug delivery applications, Expert Opin. Drug Delivery, 2010, 7, 1063–1077 CrossRef CAS PubMed.
- A. K. Gupta and M. Gupta, Synthesis and surface engineering of iron oxide nanoparticles for biomedical applications, Biomaterials, 2005, 26, 3995–4021 CrossRef CAS PubMed.
- M. Segal, Selling graphene by the ton, Nat. Nanotechnol., 2009, 4, 612–614 CrossRef CAS PubMed.
- I. Chowdhury, M. C. Duch, N. D. Mansukhani, M. C. Hersam and D. Bouchard, Colloidal properties and stability of graphene oxide nanomaterials in the aquatic environment, Environ. Sci. Technol., 2013, 47, 6288–6296 CrossRef CAS PubMed.
- Y. Zhu, S. Murali, W. Cai, X. Li, J. W. Suk, J. R. Potts and R. S. Ruoff, Graphene and graphene oxide: synthesis, properties, and applications, Adv. Mater., 2010, 22, 3906–3924 CrossRef CAS PubMed.
- D. Chen, H. Feng and J. Li, Graphene oxide: preparation, functionalization, and electrochemical applications, Chem. Rev., 2012, 112, 6027–6053 CrossRef CAS PubMed.
- K. P. Loh, Q. Bao, G. Eda and M. Chhowalla, Graphene oxide as a chemically tunable platform for optical applications, Nat. Chem., 2010, 2, 1015–1024 CrossRef CAS PubMed.
- M. Hu and B. Mi, Enabling graphene oxide nanosheets as water separation membranes, Environ. Sci. Technol., 2013, 47, 3715–3723 CrossRef CAS PubMed.
- S. Pavagadhi, A. L. L. Tang, M. Sathishkumar, K. P. Loh and R. Balasubramanian, Removal of microcystin-LR and microcystin-RR by graphene oxide: adsorption and kinetic experiments, Water Res., 2013, 47, 4621–4629 CrossRef CAS PubMed.
- O. Akhavan and E. Ghaderi, Toxicity of graphene and graphene oxide nanowalls against bacteria, ACS Nano, 2010, 4, 5731–5736 CrossRef CAS PubMed.
- Y. Chang, S.-T. Yang, J.-H. Liu, E. Dong, Y. Wang, A. Cao, Y. Liu and H. Wang, In vitro toxicity evaluation of graphene oxide on A549 cells, Toxicol. Lett., 2011, 200, 201–210 CrossRef CAS PubMed.
- A. Sasidharan, L. S. Panchakarla, P. Chandran, D. Menon, S. Nair, C. N. R. Rao and M. Koyakutty, Differential nano-bio interactions and toxicity effects of pristine versus functionalized graphene, Nanoscale, 2011, 3, 2461–2464 RSC.
- N. V. Vallabani, S. Mittal, R. K. Shukla, A. K. Pandey, S. R. Dhakate, R. Pasricha and A. Dhawan, Toxicity of graphene in normal human lung cells (BEAS-2B), J. Biomed. Nanotechnol., 2011, 7, 106–107 CrossRef CAS PubMed.
- I. E. M. Carpio, C. M. Santos, X. Wei and D. F. Rodrigues, Toxicity of a polymer–graphene oxide composite against bacterial planktonic cells, biofilms, and mammalian cells, Nanoscale, 2012, 4, 4746–4756 RSC.
- W.-C. Hou, I. Chowdhury, D. G. Goodwin Jr, W. M. Henderson, D. H. Fairbrother, D. Bouchard and R. G. Zepp, Photochemical transformation of graphene oxide in sunlight, Environ. Sci. Technol., 2015, 49, 3435–3443 CrossRef CAS PubMed.
- G. Cornelis, K. Hund-Rinke, T. Kuhlbusch, N. Van den Brink and C. Nickel, Fate and bioavailability of engineered nanoparticles in soils: a review, Crit. Rev. Environ. Sci. Technol., 2014, 44, 2720–2764 CrossRef CAS.
- F. Gottschalk, T. Sun and B. Nowack, Environmental concentrations of engineered nanomaterials: review of modeling and analytical studies, Environ. Pollut., 2013, 181, 287–300 CrossRef CAS PubMed.
- A. Praetorius, M. Scheringer and K. Hungerbühler, Development of Environmental Fate Models for Engineered Nanoparticles, A Case Study of TiO2 Nanoparticles in the Rhine River, Environ. Sci. Technol., 2012, 46, 6705–6713 CrossRef CAS PubMed.
- A. L. Dale, G. V. Lowry and E. A. Casman, Stream dynamics and chemical transformations control the environmental fate of silver and zinc oxide nanoparticles in a watershed-scale model, Environ. Sci. Technol., 2015, 49, 7285–7293 CrossRef CAS PubMed.
- K. L. Garner, S. Suh and A. A. Keller, Assessing the Risk of Engineered Nanomaterials in the Environment: Development and Application of the nanoFate Model, Environ. Sci. Technol., 2017, 51, 5541–5551 CrossRef CAS PubMed.
- A. A. Markus, J. R. Parsons, E. W. M. Roex, P. De Voogt and R. Laane, Modelling the transport of engineered metallic nanoparticles in the river Rhine, Water Res., 2016, 91, 214–224 CrossRef CAS PubMed.
- T. Y. Sun, D. M. Mitrano, N. A. Bornhöft, M. Scheringer, K. Hungerbühler and B. Nowack, Envisioning Nano Release Dynamics in a Changing World: Using Dynamic Probabilistic Modeling to Assess Future Environmental Emissions of Engineered Nanomaterials, Environ. Sci. Technol., 2017, 51, 2854–2863 CrossRef CAS PubMed.
- N. C. Mueller and B. Nowack, Exposure modeling of engineered nanoparticles in the environment, Environ. Sci. Technol., 2008, 42, 4447–4453 CrossRef CAS PubMed.
- F. Gottschalk, T. Sonderer, R. W. Scholz and B. Nowack, Modeled environmental concentrations of engineered nanomaterials (TiO2, ZnO, Ag, CNT, fullerenes) for different regions, Environ. Sci. Technol., 2009, 43, 9216–9222 CrossRef CAS PubMed.
- F. Gottschalk, R. W. Scholz and B. Nowack, Probabilistic material flow modeling for assessing the environmental exposure to compounds: methodology and an application to engineered nano-TiO 2 particles, Environmental Modelling & Software, 2010, 25, 320–332 Search PubMed.
- A. A. Keller and A. Lazareva, Predicted releases of engineered nanomaterials: from global to regional to local, Environ. Sci. Technol. Lett., 2013, 1, 65–70 CrossRef.
- A. A. Markus, J. R. Parsons, E. W. M. Roex, G. C. M. Kenter and R. W. P. M. Laane, Predicting the contribution of nanoparticles (Zn, Ti, Ag) to the annual metal load in the Dutch reaches of the Rhine and Meuse, Sci. Total Environ., 2013, 456, 154–160 CrossRef PubMed.
- H. H. Liu and Y. Cohen, Multimedia environmental distribution of engineered nanomaterials, Environ. Sci. Technol., 2014, 48, 3281–3292 CrossRef CAS PubMed.
- E. Dumont, A. C. Johnson, V. D. J. Keller and R. J. Williams, Nano silver and nano zinc-oxide in surface waters – Exposure estimation for Europe at high spatial and temporal resolution, Environ. Pollut., 2015, 196, 341–349 CrossRef CAS PubMed.
- J. Hammes, J. A. Gallego-Urrea and M. Hassellöv, Geographically distributed classification of surface water chemical parameters influencing fate and behavior of nanoparticles and colloid facilitated contaminant transport, Water Res., 2013, 47, 5350–5361 CrossRef CAS PubMed.
- R. Arvidsson, S. Molander, B. A. Sandén and M. Hassellöv, Challenges in exposure modeling of nanoparticles in aquatic environments, Hum. Ecol. Risk Assess., 2011, 17, 245–262 CrossRef CAS.
- D. Bouchard, C. Knightes, X. Chang and B. Avant, Simulating Multiwalled Carbon Nanotube Transport in Surface Water Systems Using the Water Quality Analysis Simulation Program (WASP), Environ. Sci. Technol., 2017, 51, 11174–11184 CrossRef CAS PubMed.
- N. C. Mueller, J. Braun, J. Bruns, M. Cernik, P. Rissing, D. Rickerby and B. Nowack, Application of nanoscale zero valent iron (NZVI) for groundwater remediation in Europe, Environ. Sci. Pollut. Res., 2012, 19, 550–558 CrossRef CAS PubMed.
- G. L. Stephens, J. Li, M. Wild, C. A. Clayson, N. Loeb, S. Kato, T. L'Ecuyer, P. W. Stackhouse Jr, M. Lebsock and T. Andrews, An update on Earth's energy balance in light of the latest global observations, Nat. Geosci., 2012, 5, 691–696 CrossRef CAS.
- S. Canonica, L. Meunier and U. Von Gunten, Phototransformation of selected pharmaceuticals during UV treatment of drinking water, Water Res., 2008, 42, 121–128 CrossRef CAS PubMed.
- D. Fatta-Kassinos, M. I. Vasquez and K. Kümmerer, Transformation products of pharmaceuticals in surface waters and wastewater formed during photolysis and advanced oxidation processes–degradation, elucidation of byproducts and assessment of their biological potency, Chemosphere, 2011, 85, 693–709 CrossRef CAS PubMed.
- H. Yu, Environmental carcinogenic polycyclic aromatic hydrocarbons: photochemistry and phototoxicity, J. Environ. Sci. Health, Part C: Environ. Carcinog. Ecotoxicol. Rev., 2002, 20, 149–183 CrossRef PubMed.
- A. N. Glazer, Light guides. Directional energy transfer in a photosynthetic antenna, J. Biol. Chem., 1989, 264, 1–4 CAS.
- Y. Hautier, P. A. Niklaus and A. Hector, Competition for light causes plant biodiversity loss after eutrophication, Science, 2009, 324, 636–638 CrossRef CAS PubMed.
-
L. A. Burns, D. M. Cline and R. R. Lassiter, Exposure analysis modeling system (EXAMS): User manual and system documentation, Environmental Research Laboratory, Office of Research and Development, US Environmental Protection Agency, 1982 Search PubMed.
-
J. T. O. Kirk, Light and photosynthesis in aquatic ecosystems, Cambridge university press, 1994 Search PubMed.
- H. R. Gordon, Can the Lambert-Beer law be applied to the diffuse attenuation coefficient of ocean water?, Limnol. Oceanogr., 1989, 34, 1389–1409 CrossRef.
-
B. R. Hargreaves, Water column optics and penetration of UVR, UV effects in aquatic organisms and ecosystems, 2003, vol. 1, pp. 59–108 Search PubMed.
- D. F. Swinehart, The beer-lambert law, J. Chem. Educ., 1962, 39, 333 CrossRef CAS.
-
D. Organisation for Economic Co-operation and Test No. 316: Phototransformation of Chemicals in Water–Direct Photolysis, OECD Publishing, 2008 Search PubMed.
- R. G. Zepp and D. M. Cline, Rates of direct photolysis in aquatic environment, Environ. Sci. Technol., 1977, 11, 359–366 CrossRef CAS.
- I. Chowdhury, W.-C. Hou, D. Goodwin, M. Henderson, R. G. Zepp and D. Bouchard, Sunlight affects aggregation and deposition of graphene oxide in the aquatic environment, Water Res., 2015, 78, 37–46 CrossRef CAS PubMed.
- A. A. Keller, H. Wang, D. Zhou, H. S. Lenihan, G. Cherr, B. J. Cardinale, R. Miller and Z. Ji, Stability and aggregation of metal oxide nanoparticles in natural aqueous matrices, Environ. Sci. Technol., 2010, 44, 1962–1967 CrossRef CAS PubMed.
- J. T. K. Quik, I. Velzeboer, M. Wouterse, A. A. Koelmans and D. Van de Meent, Heteroaggregation and sedimentation rates for nanomaterials in natural waters, Water Res., 2014, 48, 269–279 CrossRef CAS PubMed.
- J. T. K. Quik, D. van De Meent and A. A. Koelmans, Simplifying modeling of nanoparticle aggregation–sedimentation behavior in environmental systems: A theoretical analysis, Water Res., 2014, 62, 193–201 CrossRef CAS PubMed.
- J. T. K. Quik, M. C. Stuart, M. Wouterse, W. Peijnenburg, A. J. Hendriks and D. van de Meent, Natural colloids are the dominant factor in the sedimentation of nanoparticles, Environ. Toxicol. Chem., 2012, 31, 1019–1022 CrossRef CAS PubMed.
- D. Zhou, A. I. Abdel-Fattah and A. A. Keller, Clay particles destabilize engineered nanoparticles in aqueous environments, Environ. Sci. Technol., 2012, 46, 7520–7526 CrossRef CAS PubMed.
- C. D. Knightes, R. B. Ambrose Jr., B. Avant, Y. Han, B. Acrey, D. C. Bouchard, R. Zepp and T. Wool, Modeling framework for simulating concentrations of solute chemicals, nanoparticles, and solids in surface waters and sediments: WASP8 Advanced Toxicant Module, Environ. Model. Softw., 2018 DOI:10.1016/j.envsoft.2018.10.012 , in press.
- R. Salgado, V. J. Pereira, G. Carvalho, R. Soeiro, V. Gaffney, C. Almeida, V. V. Cardoso, E. Ferreira, M. J. Benoliel, T. A. Ternes, A. Oehmen, M. A. M. Reis and J. P. Noronha, Photodegradation kinetics and transformation products of ketoprofen, diclofenac and atenolol in pure water and treated wastewater, J. Hazard. Mater., 2013, 244-245, 516–527 CrossRef CAS PubMed.
- B. A. Wols and C. H. M. Hofman-Caris, Review of photochemical reaction constants of organic micropollutants required for UV advanced oxidation processes in water, Water Res., 2012, 46, 2815–2827 CrossRef CAS PubMed.
- F. Wania and D. Mackay, A global distribution model for persistent organic chemicals, Sci. Total Environ., 1995, 160-161, 211–232 CrossRef.
- F. Wania and D. Mackay, The evolution of mass balance models of persistent organic pollutant fate in the environment, Environ. Pollut., 1999, 100, 223–240 CrossRef CAS PubMed.
- F. Wania, Assessing the potential of persistent organic chemicals for long-range transport and accumulation in polar regions, Environ. Sci. Technol., 2003, 37, 1344–1351 CrossRef CAS.
- H. Singer, S. Müller, C. Tixier and L. Pillonel, Triclosan: Occurrence and Fate of a Widely Used Biocide in the Aquatic Environment: Field Measurements in Wastewater Treatment Plants, Surface Waters, and Lake Sediments, Environ. Sci. Technol., 2002, 36, 4998–5004 CrossRef CAS PubMed.
- J. R. Lead and K. J. Wilkinson, Aquatic colloids and nanoparticles: current knowledge and future trends, Environ. Chem., 2006, 3, 159–171 CrossRef CAS.
- A. L. Dale, G. V. Lowry and E. A. Casman, Modeling nanosilver transformations in freshwater sediments, Environ. Sci. Technol., 2013, 47, 12920–12928 CrossRef CAS PubMed.
- A. L. Dale, G. V. Lowry and E. A. Casman, Much ado about α: reframing the debate over appropriate fate descriptors in nanoparticle environmental risk modeling, Environ. Sci.: Nano, 2015, 2, 27–32 RSC.
- A. Praetorius, N. Tufenkji, K.-U. Goss, M. Scheringer, F. von der Kammer and M. Elimelech, The road to nowhere: equilibrium partition coefficients for nanoparticles, Environ. Sci.: Nano, 2014, 1, 317–323 RSC.
- M. N. Martin, A. J. Allen, R. I. MacCuspie and V. A. Hackley, Dissolution, agglomerate morphology, and stability limits of protein-coated silver nanoparticles, Langmuir, 2014, 30, 11442–11452 CrossRef CAS PubMed.
- C. Levard, E. M. Hotze, G. V. Lowry and G. E. Brown Jr, Environmental transformations of silver nanoparticles: impact on stability and toxicity, Environ. Sci. Technol., 2012, 46, 6900–6914 CrossRef CAS PubMed.
- R. Ma, C. m. Levard, J. D. Judy, J. M. Unrine, M. Durenkamp, B. Martin, B. Jefferson and G. V. Lowry, Fate of zinc oxide and silver nanoparticles in a pilot wastewater treatment plant and in processed biosolids, Environ. Sci. Technol., 2013, 48, 104–112 CrossRef PubMed.
- J. Liu and R. H. Hurt, Ion release kinetics and particle persistence in aqueous nano-silver colloids, Environ. Sci. Technol., 2010, 44, 2169–2175 CrossRef CAS PubMed.
- A. J. Miao, X. Y. Zhang, Z. Luo, C. S. Chen, W. C. Chin, P. H. Santschi and A. Quigg, Zinc oxide–engineered nanoparticles: Dissolution and toxicity to marine phytoplankton, Environ. Toxicol. Chem., 2010, 29, 2814–2822 CrossRef CAS PubMed.
- W.-C. Hou, W. M. Henderson, I. Chowdhury, D. G. Goodwin, X. Chang, S. Martin, D. H. Fairbrother, D. Bouchard and R. G. Zepp, The contribution of indirect photolysis to the degradation of graphene oxide in sunlight, Carbon, 2016, 110, 426–437 CrossRef CAS.
Footnote |
† Electronic supplementary information (ESI) available. See DOI: 10.1039/c8en01088a |
|
This journal is © The Royal Society of Chemistry 2019 |
Click here to see how this site uses Cookies. View our privacy policy here.