DOI:
10.1039/C8RA08256A
(Paper)
RSC Adv., 2018,
8, 42087-42094
Temperature dependence of denitrification microbial communities and functional genes in an expanded granular sludge bed reactor treating nitrate-rich wastewater†
Received
6th October 2018
, Accepted 28th November 2018
First published on 18th December 2018
Abstract
The temperature dependence of denitrification was investigated for high nitrate nitrogen denitrification in an expanded granular sludge bed (EGSB) reactor. The optimal reaction temperatures were 15–35 °C in which nearly complete denitrification was achieved with the removal of COD maintained over 80%. Nitrite accumulation was observed at 10 °C indicating the incomplete denitrification at low temperature. However, almost complete denitrification was even accomplished as high as 52 °C. High-throughput sequencing detected a total of 84 bacterial genera and 7 phyla, and temperature variation resulted in the shift of microbial community structure and diversity. Proteobacteria thrived while Firmicutes and Bacteroidetes were inhibited by temperature stress. The predominance of Halomonas and the significant decrease of Azoarcus at low temperature indicated a more important role of these two genera in denitrification in an EGSB reactor. The results of qPCR indicated that temperature exerted effects on the abundance of denitrification function genes, nirK, nirS, narG, and nosZ, due to the shift of the bacterial community. This study provided a comprehensive understanding of temperature effects on the denitrification process in an EGSB reactor treating high concentration nitrate wastewater.
1. Introduction
Some industrial wastewaters from the fertilizer, uranium, nuclear, metal finishing, cellophane, and explosives industries usually contain nitrate in high concentration. For instance, the effluent nitrogen concentration from the fertilizer industry can be as high as 950 mg L−1.1 In the uranium industry, after a liquid–liquid extraction, uranyl nitrate raffinate (UNR) contains up to 77
000 mg L−1 of nitrate.2 Excess nitrate (NO3−) in water bodies can lead to eutrophication3 and presents a potential hazard to human health.4 Therefore, treatment of highly concentrated nitrate wastewater has become a major concern in these industries.
Biological denitrification with the reduction of nitrate to nitrogen gas by heterotrophic bacteria under anoxic conditions is effective for nitrate removal and has been achieved in fluidized bed biofilm reactors (FBBR),5 fluidized-bed reactors (FBRs),6 continuous stirred tank reactors (CSTR),2 sequencing batch reactors (SBR),7 sequencing batch biofilm reactors (SBBRs)8 and anoxic/oxic-membrane bioreactors (A/O-MBR).9 In our previous studies, expanded granular sludge bed (EGSB) reactors was successfully applied for denitrification with high concentrations of nitrate nitrogen to 14
000 mg L−1, in which the parameters including the ratio of carbon to nitrogen, up-flow liquid velocity Vup, and pH value were investigated.10–13 In spite of those researches, more efforts still needs to be made in order for the practical application of promising EGSB reactor in the treatment of nitrate-rich wastewater.
Temperature is also an important parameter in the functioning of biological reactors. Elefsiniotis and Li pointed that a temperature change from 10 °C to 20 °C exerted a greater effect on both the specific denitrification and carbon consumption rates than a further temperature increase from 20 °C to 30 °C.14 Adouani et al. found that average nitrogen abatement was slowed down when temperature decreased from 20 °C to 5 °C.15 However, the vast majority of these previous studies mainly focused on the performance and were conducted by batch tests rather than continuous experiments. Considering the involvement of different bacteria in denitrification process, reactor temperature might affect microbial community structure and function, subsequently produce an impact on denitrification performance. However, little information is available for the EGSB reactor treating wastewater containing high nitrate concentration. Moreover, compared to low and medium temperature, high temperature over 35 °C was rarely concerned. Whether denitrification could proceed is unknown. Since some wastewaters were discharged with high temperature or treated by thermophilic anaerobic digestion, additional knowledge on the denitrification process at high temperatures could offer a help in the design optimizing and practical application.
In the present study, the influence of reaction temperature transition ranging from 10 °C to 52 °C on denitrification was explored based on a continuous EGSB reactor. Apart from the performance of the reactor, the molecular ecological mechanisms behind the response of denitrifiers and their function in combination of high-throughput sequencing and quantitative polymerase chain reaction (qPCR) technology was also fully investigated. The results may help to provide a comprehensively understanding of temperature effect on the denitrification process and theoretical support to enhance the performance of EGSB reactor treating high concentration nitrate wastewater.
2. Materials and methods
2.1 Experimental procedure
A lab scale EGSB reactor in this study was described in previous study.10 The Plexiglas EGSB reactor was 60 mm in inner diameter and 910 mm high, giving a total volume of 3.02 L and a working volume of 1.74 L (Fig. S1 in ESI†). The seed sludge was from another denitrification reactor with mixed liquor suspended solids and mixed liquor volatile suspended solids were 41.68 g L−1 and 25.81 g L−1, respectively.10 The characteristics of the wastewater were derived from the composition of the synthetic wastewater (g L−1), which was composed of 2.0 NaNO3, 7.0 Na2HPO4, 1.5 K2HPO4, 0.1 MgSO4, and 0.1 NH4Cl. A trace element solution, which consisted (g L−1) of 5.55 CaCl2, 5.0 FeSO4·7H2O, 5.06 MnCl2·4H2O, 2.2 ZnSO4·7H2O, 1.51 CuSO4·5H2O, 1.61 CoCl2·H2O, 50.00 EDTA, and 1.1 (NH4)6Mo7O24·4H2O. The trace element solution was added to the synthetic wastewater at a volume ratio of 1
:
500; the C
:
N mole ratio was regulated at 2
:
1 by adding sodium nitrate and sodium acetate, which were made of the synthetic wastewater. During the start up stage, the EGSB reactor was fed with synthetic wastewater prepared following previous study10 in which influent NO3−–N concentration was elevated from 200 mg L−1 to 2000 mg L−1.
Afterwards, in order to investigate the effect of temperature on denitrification process, the reactor was further continuously operated for 86 days keeping Vup of 3.0 m h−1, a mole ratio of carbon/nitrate nitrogen (C/N) of 2.0, a hydraulic retention time (HRT) of 24 h and an influent pH of 7.1–7.3. The reaction temperature of the EGSB gradually was decreased from 35 °C through 25 °C and 15 °C to 10 °C and then gradually increased from 10 °C through 35 °C to 52 °C. Detailed operational parameters is listed in Table 1.
Table 1 Continuous operational parameters of the EGSB reactor
Stage |
Duration (days) |
Nitrate concentration (mg L−1) |
Vup(m h−1) |
Influent pH value |
HRT (h) |
Reaction temperature (°C) |
Start-up |
1–7 |
200 |
2.0 |
7.20 ± 0.05 |
24 |
35 |
8–14 |
500 |
2.0 |
7.20 ± 0.05 |
24 |
35 |
15–21 |
1000 |
2.0 |
7.20 ± 0.05 |
24 |
35 |
22–28 |
1500 |
3.0 |
7.20 ± 0.10 |
24 |
35 |
29–35 |
2000 |
3.0 |
7.20 ± 0.10 |
24 |
35 |
Effect of temperature |
36–49 |
2000 |
3.0 |
7.20 ± 0.10 |
24 |
35 |
Period 2 |
50–64 |
2000 |
3.0 |
7.20 ± 0.10 |
24 |
25 |
Period 3 |
65–78 |
2000 |
3.0 |
7.20 ± 0.10 |
24 |
15 |
Period 4 |
79–91 |
2000 |
3.0 |
7.20 ± 0.10 |
24 |
10 |
Period 5 |
92–105 |
2000 |
3.0 |
7.20 ± 0.10 |
24 |
35 |
Period 6 |
106–121 |
2000 |
3.0 |
7.20 ± 0.10 |
24 |
52 |
2.2 Chemical and statistical analysis
Concentrations of nitrite and nitrate were measured using the colorimetric method and the ultraviolet spectrophotometric method, respectively.16 The COD were determined by standard methods. SPSS 20.0 software was applied for the calculation of t test in order to assess the significant differences.
2.3 DNA extraction and polymerase chain reactions
Sludge samples were collected from the bottom of the reactor at different reaction temperatures (35 °C, 25 °C, 10 °C and 52 °C), three samples were taken at each temperature time point and mixed into one temperature sample and each temperature sample were named T35, T25, T10, and T52, respectively. The genomic DNA was extracted by FastDNA® SPIN Kit for Soil (MP Biomedicals, CA, USA). The concentration and purity of the DNA were determined by micro-spectrophotometry (ND-1000, NanoDrop, USA).
PCR were conducted to amplify the denitrifying functional genes, including the nitrate reductase gene narG, nitrite reductase genes nirK and nirS, and the nitrous oxide reductase gene nosZ. The primers of denitrifying functional genes and PCR programs was listed in Table 2. Detailed reaction system were described in literature.12
Table 2 Primers and reaction protocols used for the PCR and quantitative PCR in this study
Target |
Sequence (5′–3′) |
Fragment size (bp) |
Thermal profile for PCR (35 cycles) |
Thermal profile for qPCR (40 cycles) |
Ref. |
nirS |
F:GTSAACGTSAAGGARACSGG |
425 |
94 °C/30 s, 59 °C/30 s, 72 °C/60 s |
95 °C/60 s, 59 °C/60 s, 72 °C/60 s |
17 |
R:GASTTCGGRTGSGTCTTGA |
18 |
nirK |
F:ATCATGGTSCTGCCGCG |
473 |
94 °C/30 s, 62.5 °C/30 s, 72 °C/60 s |
95 °C/40 s, 62 °C/40 s, 72 °C/60 s |
19 |
R:GCCTCGATCAGRTTGTGGTT |
19 |
nosZ |
F:CGCRACGGCAASAAGGTSMSSGT |
267 |
95 °C/45 s, 59.5 °C/60 s, 72 °C/60 s |
95 °C/60 s, 60 °C/60 s, 72 °C/60 s |
20 |
R:CAKRTGCAKSGCRTGGCAGAA |
20 |
narG |
F:TAYGTSGGGCAGGARAAACTG |
109 |
95 °C/20 s, 59 °C/30 s, 72 °C/40 s |
95 °C/20 s, 59 °C/30 s, 72 °C/40 s |
21 |
R:CGTAGAAGAAGCTGGTGCTGTT |
21 |
Bacterial 16S rRNA |
F:AAGGAGGTGATCCRGCCGCA |
172 |
|
95 °C/30 s, 55 °C/45 s, 72 °C/50 s |
21 |
R:CGGTGAATACGTTCYCGG |
21 |
2.4 Quantitative real-time PCR (q-PCR)
The copy number for the four denitrifying functional genes (narG, nirK, nirS, and nosZ) was quantified using Rotor-Gene 6000 Series Software 1.7 (QIAGEN, the Netherlands). The system of the q-PCR mixture (20 μL) contained 1.4 μL of ddH2O, 0.3 μL of each primer set (10 μM), 8 μL of template DNA (5 ng μL−1), and 10 μL of SYBR Premix EXTaq Super Mix (TaKaRa, Japan). The reaction programs are listed in Table 2. Each sample was measured by three times for each functional genes. Five to seven-point calibration curves (Ct values versus log of initial target gene copy) were generated using a 10-fold serial dilution of the plasmid containing the target gene with a range from 109 to 103 copies. To minimize the variation in the DNA extraction efficiency of the samples, the eubacterial 16S rRNA gene was quantified simultaneously,21 and the relative abundance of the target genes was normalized to each total bacterial community. The reaction efficiency of each gene ranged from 85.6% to 104.5% with R2 values greater than 0.996 for all the calibration curves.
2.5 Pyrosequencing of the 16S rRNA gene
The bacterial DNA was amplified with a set of primers targeting the hypervariable V3–V4 region (about 526 bp) of the 16S rRNA gene. The forward primer was 341F (5′-CCTACGGGNGGCWGCAG-3′) and the reverse primer was 802R (5′-TACNVGGGTATCTAATCC-3′).22,23 High-throughput sequencing was conducted at Majorbio Bio-pharm Technology Co., Ltd (Shanghai, China) on Illumina Mi-seq platform. The generated raw sequences for all the samples were assigned by Ribosomal Database Project (RDP) (http://rdp.cme.msu.edu/) to roughly trim off the adapters and barcodes and remove sequences containing ambiguous ‘N’ nucleotides or those which were shorter than 200 bps. Then the reads were denoised using the Mothur software (http://www.mothur.org/). Downstream taxonomic assignment was performed by RDP. The richness and diversity indices including operational taxonomic units (OTUs), Chao1, Shannon index (H′), evenness index (E), were calculated by Mothur. In order for better understanding of the relationship between environmental factors and community composition, canonical correspondence analysis (CCA) was conducted by Past 3.21 software in this study.
3. Results and discussion
3.1 Effect of temperature on the denitrification process
Fig. 1 shows the effects of different reaction temperatures on the removal of COD and nitrate as well as the accumulation of nitrite. Clearly, when the temperature decreased from 35 °C to 15 °C, nearly complete denitrification was achieved with influent nitrate concentration at 2000 mg L−1 and the removal of COD maintained over 80%. While at low temperature (10 °C), the removal efficiency of the nitrate-N was lower with nitrite accumulation was observed. The peak concentration of effluent nitrite-N in the EGSB reactor was 122 mg L−1. The denitrification was obviously inhibited suggesting the incomplete denitrification in low temperature, which might due to the inhibition of the growth of some denitrifying bacteria by low temperature. After once again raising the temperature to 35 °C, the denitrification of EGSB reactor was restored since the removal of nitrate increased and the effluent nitrite-N content decreased noticeably. Further raising the temperature high to 52 °C, the denitrification was not apparently affected, and the removal efficiency of the nitrate-N maintained 99%. While the removal efficiency of COD decreased noticeably and the average removal efficiency was only about 70%. Although the removal efficiencies of nitrate nitrogen under all temperature conditions was above 97%, the t-test showed that there was an extremely significant difference (p < 0.01) between nitrate removal of T52, T35, T25 and that of T10 (Table S2†). In summary, the suitable temperatures for the denitrification were wider in the range of 15–35 °C of EGSB reactor treating wastewater with high nitrate content. Noticeably, thermophilic condition had a slightly impact on the denitrification without the nitrite accumulation. Zhang et al. also pointed that the nitrate and nitrite concentrations did not differ significantly under mesophilic (35 °C) and thermophilic (55 °C) anaerobic digestion of cattle manure.24 It can be speculated that the denitrification and methanogenesis coexisted in thermophilic anaerobic digestion of organic wastewater containing nitrate. Denitrification process will play a competitive role with methanogenesis process. This was because that nitrate was energetically more favorable electron acceptors than CO2. In addition, denitrifiers and methanogens would competed for readily biodegradable carbon source such as acetate.45 The inhibition of nitrite might also contributed the suppression of methanogenesis.46
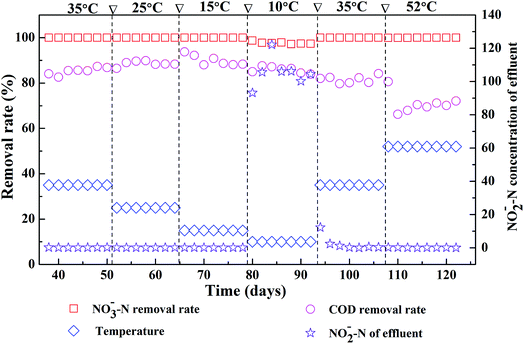 |
| Fig. 1 Effects of different reaction temperatures on denitrification in an EGSB reactor. | |
3.2 Microbial diversity by pyrosequencing
To study the response of microbial community structure on the variation of temperatures, the T10, T25, T35, and T52 samples were each sequenced using pyrosequencing. A total of 59251 16S rRNA sequence reads were generated by the pyrosequencing of duplicate samples from the EGSB reactor. After the raw reads were denoised and their chimera were removed using the process reported in,25 the number of selected bacterial sequences was 17
892, which were used in all the subsequent analyses.
Rarefaction analysis was employed to standardize and compare the observed taxon richness and to identify whether each sample was adequately sampled. In the rarefaction curves, distance values of 0.03, 0.05, 0.07, and 0.1 are generally accepted as points at which differentiation occurs at the species, genus and family/class levels, respectively.26–29 Since there is some controversy about these distinctions, particularly at the 0.03 cutoff for novel isolates, a more stringent 0.01 distance value was also calculated for the rarefaction curve. A rarefaction curve that reaches a plateau reflects a habitat that has been sampled to saturation with respect to the species diversity in an ecosystem.28
The slopes for the four samples in the rarefaction curve were all flat at the 0.03 level (Fig. S2†), thus the number of samples was reasonable. This result supports the usefulness of pyrosequencing as a tool for in-depth assessment of bacterial diversity as a function of 16S rRNA gene sequences in aquatic ecosystems.10 The microbial diversity abundance of these samples was as follows: T35 > T10 > T25 > T52 (Fig. S2†). The T35 sample has a most abundant microbial diversity. The corresponding numbers of operational taxonomic units (OTUs), Chao1, Shannon index (H′), and evenness index (E) are summarized in Table 3, which also shows that the samples from the EGSB reactor differed in their diversity.
Table 3 Values of OTUs, Chao1, Shannon index (H′), and evenness index (E) for the T10, T25, T35, and T52 samplesa
Sample |
Similarity |
OTUs |
Chao1 |
H′ |
E |
OTUs – operational taxonomic units. Chao1 – community richness. A higher number represents greater richness. Shannon index (H′) – community diversity. A higher number represents higher diversity. E – evenness index. |
T10 |
99% |
1649 |
4068 |
6.63748 ± 0.00071 |
0.896 |
97% |
255 |
485 |
3.47458 ± 0.00111 |
0.62704 |
95% |
92 |
205 |
2.3093 ± 0.00079 |
0.5107 |
93% |
58 |
145 |
1.73572 ± 0.00058 |
0.42747 |
90% |
39 |
59 |
1.41074 ± 0.00049 |
0.38507 |
T25 |
99% |
407 |
618 |
4.29167 ± 0.00071 |
0.71423 |
97% |
130 |
149 |
2.86091 ± 0.00065 |
0.58775 |
95% |
92 |
113 |
2.65453 ± 0.00052 |
0.58705 |
93% |
76 |
89 |
2.44422 ± 0.00049 |
0.56439 |
90% |
58 |
67 |
2.19908 ± 0.00044 |
0.54159 |
T35 |
99% |
1040 |
1938 |
5.25538 ± 0.0005 |
0.7565 |
97% |
335 |
466 |
3.63232 ± 0.00045 |
0.62474 |
95% |
205 |
280 |
2.80963 ± 0.00045 |
0.52783 |
93% |
151 |
188 |
2.52633 ± 0.00038 |
0.50352 |
90% |
104 |
131 |
2.0929 ± 0.00038 |
0.45063 |
T52 |
99% |
448 |
832 |
4.91442 ± 0.00225 |
0.80501 |
97% |
81 |
118 |
1.94488 ± 0.00213 |
0.44258 |
95% |
53 |
63 |
1.7279 ± 0.00175 |
0.43521 |
93% |
40 |
45 |
1.55628 ± 0.00153 |
0.42188 |
90% |
30 |
33 |
1.05974 ± 0.00156 |
0.31158 |
At the 0.03 level, the OTUs numbers for the T35, T10, T25, and T52 samples were 335, 255, 130, and 81, respectively, and corresponded to Shannon index (H′) values of 3.63, 3.47, 2.86, and 1.94. This also indicated that the microbial diversity in the T35 sample was the most abundant. Furthermore, the evenness indices (E) of the T35, T10, T25, and T52 samples were 0.625, 0.627, 0.588, and 0.443, respectively, and corresponding Chao1 index of 466, 485, 149, and 118. That indicated that the species distributions in the T35 and T10 was more abundant, and community richness was greater. However, the community diversity was less rich than that reported by Zhao et al. and Ye et al., who respectively found 644 OTUs in an anaerobic sludge and 494 OTUs in a nitrification reactor sludge at a 3% cutoff level.30,31 The samples also showed considerably less richness compared with activated sludge from sewage treatment plants,25,32 where 1183–4120 OTUs were in a sludge sample at 3% cutoff level and H′ varied from 6.3 to 7.3.
3.3 Phyletic distribution analysis by pyrosequencing
Each of the samples from the four different reaction temperatures used in the denitrification treatment system in the EGSB reactor was assessed to determine the taxonomic composition at the phylum level; the results were shown in Fig. 2. There are primarily seven phyla in the four samples including Proteobacteria, Firmicutes, Bacteroidetes, Actinobacteria, Tenericutes, Synergistetes, and Deinococcus–Thermus. Obviously, Proteobacteria was the absolutely predominant phyla accounting for 89–96% in each sample followed by Firmicutes (1.9–6.0%) and Bacteroidetes (2.2–3.3%), which indicated that the three phyla played a dominant role in denitrification in the high nitrate wastewater treatment system using an EGSB reactor. This was consistent with the report that many types of denitrifiers are included in the phyla of Proteobacteria, Firmicutes and Bacteroidetes.33,34 Interestingly, the abundance of phylum Proteobacteria increased with the temperature increased to 52 °C or decreased to 10 °C from 25 °C. Meanwhile, Firmicutes and Bacteroidetes occupied a relative lower abundance at low temperature 10 °C and high temperature 52 °C compared with those at 25 °C and 35 °C. It indicated that some bacterium in Firmicutes and Bacteroidetes were inhibited by temperature stress, while Proteobacteria thrived and enriched even in stress condition. It is noticed that phyla of Synergistetes and Deinococcus–Thermus were not be detectable in T10 sample comparing with the other three samples of T25, T35, and T52, revealing that the low temperature stress could wash out some species in Synergistetes and Deinococcus–Thermus.
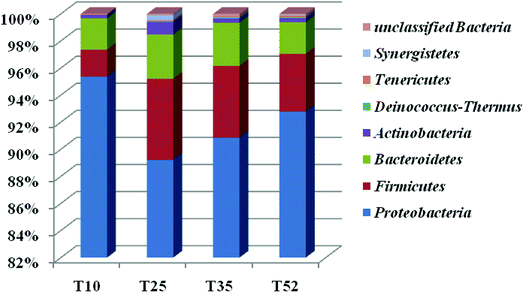 |
| Fig. 2 Bacterial phylum detected in the T10, T25, T35, and T52 samples. Comparison of the sludge bacterial phylum abundance was determined by sequencing 16S hypervariable rDNA regions. Relative abundance of the major bacterial phyla found in the sequence of the four different sludge samples is shown. Y-axis represents relative abundance. | |
The taxonomic composition of the four samples at the genus level was further determined (Table S1†) and an hierarchical cluster analysis was shown in Fig. 3. It is obvious that the microbial community structure changed across the different reaction temperatures in the EGSB reactor. Some genus disappeared while some dominant genera strengthened. T35 sample had most microbiota in genera. Furthermore, 84 different genera were detected in the four samples. Among them, Halomonas, a genus within Gammaproteobacteria, occupied predominance comparing other genus, with the abundance of 81.59%, 45.68%, 41.62%, and 76.89% in T10, T25, T35, and T52 samples, respectively. In addition, the genus of Azoarcus, Pseudomonas and Wandonia were also dominant. The high percentage abundance in T10 and T52 revealed that Halomonas played a more important role in denitrification at temperature stress. Moreover, the complete removal of nitrate might be mainly attributed to the genus Halomonas, since nitrate reductases related to Gammaproteobacteria of the genus Marinobacter and Halomonas.35 The genera Azoarcus which also play important roles in denitrification,36–41 was obviously inhibited at the low temperature. The relative abundance of Azoarcus was 29.13% at 35 °C, whereas the relative abundance of Azoarcus was only 2.6% at 10 °C, which might contribute to the nitrite accumulation and the decrease in the nitrate removal at 10 °C (Fig. 1).
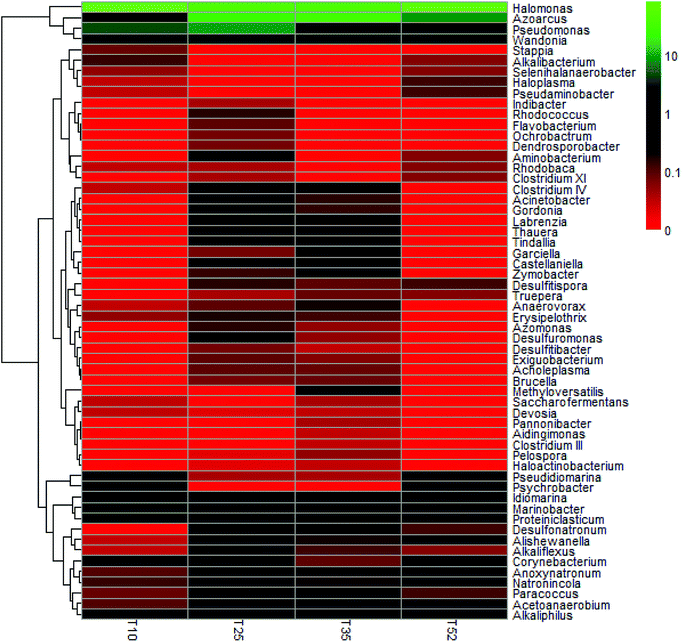 |
| Fig. 3 Hierarchical cluster analysis of the bacterial communities in the four samples. The Y-axis is the clustering of the most abundant OTUs (3% distance) in number of reads. The OTUs were ordered by genus. The sample communities were clustered based on the complete linkage method. The color intensity of the scale indicates the relative abundance of each OTU read. Relative abundance was defined as the number of sequences affiliated with that OTU divided by the total number of sequences per sample. | |
For better understanding of the relationship between environmental or operational factors and community composition at genus level, canonical correspondence analysis (CCA) was conducted in this study and the results was shown in Fig. 4. Clearly, The nitrate removal vector almost overlapped with nitrite content in effluent vector with opposite direction. The temperature were positively associated to nitrate removal. The predominance Halomonas and Azoarcus correlated with nitrate removal and nitrite content in effluent, revealing their contribution on the denitrification. Pseudomonas and Wandonia had a positively correlation with COD removal, indicating that they might play an important role in the COD degradation in EGSB reactor.
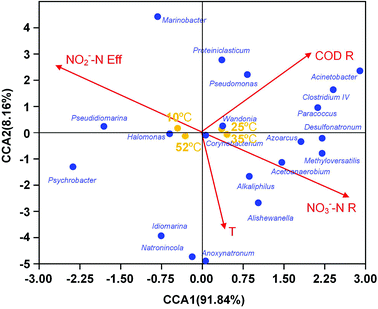 |
| Fig. 4 Ordination plots generated by canonical correspondence analysis (CCA) showing the relationship between microbial community compositions at genus level and environment parameters. Correlations between environmental variables and CCA axes were represented by the length and angle of arrows (environmental factor vectors). T temperature, NO2−–Neff effluent NO2−–N concentration, CODR COD removal efficiency, NO3−–NR NO3−–N removal efficiency. | |
3.4 Abundance of functional genes in an EGSB reactor
Denitrification functional genes nirK, nirS, narG, and nosZ, at different reaction temperatures in the EGSB reactor were investigated using qPCR technology and the results showed in Fig. 5. The variation of temperature posed an obvious impact on the abundance of denitrification genes, which might due to the shift of bacterial community. The narG gene is often used as a marker for the anaerobic conversion of NO3–N to NO2–N.21 The relative abundance of the narG gene decreased with an increase in reaction temperature, and the relative abundance of the narG gene was the highest at low temperature (10 °C), which might results from the enrichment of Halomonas and Marinobacter. Because previous studies showed that nitrate reductases related to Gammaproteobacteria of the genus Marinobacter and Halomonas by using the narG gene as a diagnostic marker for nitrate-reducing bacteria.35
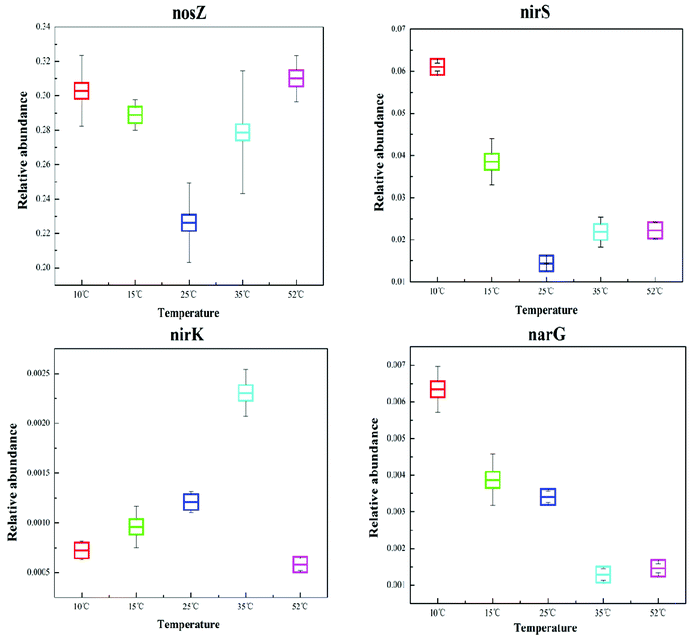 |
| Fig. 5 Comparison of the relative abundance of the corresponding dinitrification functional genes, nirK, nirS, narG, and nosZ, in the T10, T25, T35, and T52 samples. | |
Since the gas-producing process from nitrite to nitric oxide is critical in denitrification, the functional genes, nirK and nirS, are the most commonly studied when investigating denitrifying bacteria community structure. As can be seen in Fig. 5, the genes of nirK and nirS exhibit different response to the temperature variation. Furthermore, the relative abundance of the nirS gene was one order of magnitude greater than that of the nirK gene, congruent with previous studies which reported that the nirS gene was environmentally more abundant than the nirK gene.12,42 Although nirK had a lower abundance than nirS in the different reaction temperature environments, the former often plays a more crucial role in nitrite reduction.43 In this study, T35 had the highest abundance of nirK functional gene indicating the powerful reduction ability of nitrite to nitric oxide at 35 °C.
The nosZ gene (nitrous oxide reduction) often acts as a marker for complete denitrification.44 In this study, the nosZ gene was the most abundant with an average fraction of 28.1%, greatly exceeding the other target functional narG, nirK, and nirS genes. This indicated that the reduction rate of nitrous oxide to nitrogen was rapid in the EGSB reactor. Perhaps denitrifying organisms that possess the nosZ gene also played an important role in denitrification in this system.
4. Conclusions
Complete denitrification with high content of influent nitrate nitrogen was accomplished in the EGSB reactor at 15–35 °C. Accumulation of nitrite was observed at low temperature of 10 °C. High temperature of 52 °C induced a noticeably decrease of COD removal. The microbial diversity was highest in the condition of 35 °C. Seven bacterial phyla and 84 bacterial genera were detected in the EGSB reactor. Firmicutes and Bacteroidetes were inhibited while Proteobacteria thrived in temperature stress. Halomonas, Azoarcus, and Marinobacter were dominant genera. Halomonas played an important role in denitrification at temperature stress. The genera Azoarcus reduction might contribute to the nitrite accumulation at 10 °C. The denitrification functional genes, nirK, nirS, narG, and nosZ, also exhibited different dependences of temperature due to the shift of denitrifier community.
Conflicts of interest
There are no conflicts to declare.
Acknowledgements
We gratefully acknowledge generous support provided by National Natural Science Foundation of China (51468024), Jiangxi Provincial Department of Education Science and Technology Research Project (GJJ160887), Dr Start-up funding of Jingdezhen Ceramic Institute, P. R. China. Special Fund for Young and Middle-aged Teachers Development Plan Visiting Scholar in Jiangxi Province General Undergraduate College.
Notes and references
- L. Z. Swati, A. Jayalexmy and J. D. Anjana, World J. Microbiol. Biotechnol., 2004, 20, 661–665 CrossRef.
- P. M. Biradar, P. B. Dhamole, R. R. Nair, S. B. Roy, S. K. Satpati, S. F. D'Souza, S. S. Lele and A. B. Pandit, AIChE, 2008, 27(3), 365–372 CAS.
- G. F. McIsaac, M. B. David, G. Z. Gertner and D. A. Goolsby, Nature, 2001, 414, 166–167 CrossRef CAS PubMed.
- L. Knobeloch, B. Salna, A. Hogan, J. Postle and H. Anderson, Environ. Health Perspect., 2000, 108, 675–678 CrossRef CAS PubMed.
- K. J. R. Fahid and F. D. Mohamed, Water Res., 2004, 38, 3719–3728 CrossRef PubMed.
- F. D. Capua, I. Milone, A. M. Lakaniemi, P. N. L. Lens and G. Esposito, Chem. Eng. J., 2017, 313, 591–598 CrossRef.
- Y. Fernández-Nava, E. Marañón, J. Soons and L. Castrillón, J. Hazard. Mater., 2010, 173, 682–688 CrossRef PubMed.
- Y. Wang, B. Gong, Z. Lin, J. Wang, J. Zhang and J. Zhou, Biochem. Eng. J., 2018, 132, 112–121 CrossRef CAS.
- J. Shen, R. He, W. Han, X. Sun, J. Li and L. Wang, J. Hazard. Mater., 2009, 172, 595–600 CrossRef CAS PubMed.
- R. Liao, K. Shen, A. M. Li, P. Shi, Y. Li, Q. Shi and Z. Wang, Bioresour. Technol., 2013, 134, 190–197 CrossRef CAS PubMed.
- R. Liao, Y. Li, J. Du, A. Li, H. Song, Z. Shen and Y. Li, Desalin. Water Treat., 2016, 57, 4357–4364 CAS.
- Y. Miao, R. Liao, X. Zhang, Y. Wang, Z. Wang, P. Shi, B. Liu and A. Li, Water Res., 2015, 76, 43–52 CrossRef CAS PubMed.
- Y. Miao, Z. Wang, R. Liao, P. Shi and A. Li, Chem. Eng. J., 2017, 330, 757–763 CrossRef CAS.
- P. Elefsiniotis and D. Li, Biochem. Eng. J., 2006, 28, 148–155 CrossRef CAS.
- N. Adouani, L. Limousy, T. Lendormi and O. Sire, C. R. Chimie, 2015, 18, 15–22 CrossRef CAS.
- E. Sahinkaya, A. Kilic, B. Calimlioglu and Y. Toker, J. Hazard. Mater., 2013, 262, 234–239 CrossRef CAS PubMed.
- V. Michotey, V. Mejean and P. Bonin, Appl. Environ. Microbiol., 2000, 66, 1564–1571 CrossRef CAS PubMed.
- I. N. Throback, K. Enwall, A. Jarvis and S. Hallin, FEMS Microbiol. Ecol., 2004, 49(3), 401–417 CrossRef CAS PubMed.
- S. Hallin and P. E. Lindgren, Appl. Environ. Microbiol., 1999, 65, 1652–1657 CAS.
- S. Henry, D. Bru, B. Stres, S. Hallet and L. Philippot, Appl. Environ. Microbiol., 2006, 72, 5181–5189 CrossRef CAS PubMed.
- J. C. Lopez-Gutierrez, S. Henry, S. Hallet, F. Martin-Laurent, G. Catroux and L. Philippot, J. Microbiol. Methods, 2004, 57, 399–407 CrossRef CAS PubMed.
- M. J. Claesson, O. O'Sullivan, Q. Wang, J. Nikkila, J. R. Marchesi, H. Smidt, W. M. de Vos, R. P. Ross and P. W. O'Toole, PLoS One, 2009, 4, e6669 CrossRef PubMed.
- Y. Liang, D. Li, X. Zhang, H. Zeng, Y. Yang and J. Zhang, Bioresour. Technol., 2015, 193, 408–414 CrossRef CAS PubMed.
- K. Zhang, J. Gu, X. Wang, Y. Yin, X. Zhang, R. Zhang, X. Tuo and L. Zhang, Sci. Total Environ., 2018, 634, 501–508 CrossRef CAS PubMed.
- T. Zhang, M. F. Shao and L. Ye, ISME J., 2011, 6(6), 1–11 Search PubMed.
- E. Stackebrandt and B. M. Goebel, Int. J. Syst. Bacteriol., 1994, 44, 846–849 CrossRef CAS.
- P. Hugenholtz, B. M. Goebel and N. R. Pace, J. Bacteriol., 1998, 180, 4765–4774 CAS.
- G. F. Matcher, R. A. Dorrington, T. O. Henninger and P. W. Froneman, Water SA, 2011, 381–390 Search PubMed.
- J. S. Bowman, S. Rasmussen, N. Blom, J. W. Deming, S. Rysgaard and T. Sicheritz-Ponten, ISME J., 2012, 6, 11–20 CrossRef CAS PubMed.
- G. Zhao, F. Ma, L. Wei and H. Chua, Bioresour. Technol., 2012, 113, 83–88 CrossRef CAS PubMed.
- L. Ye, M. F. Shao, T. Zhang, A. H. Y. Tong and S. Lok, Water Res., 2011, 45(15), 4390–4398 CrossRef CAS PubMed.
- M. Hu, X. H. Wang, X. H. Wen and Y. Xia, Bioresour. Technol., 2012, 117, 72–79 CrossRef CAS PubMed.
- S. H. Lee, S. Kondaveeti, B. Min and H. D. Park, Process Biochem., 2013, 48, 306–311 CrossRef CAS.
- M. Zielinska, P. Rusanowska, J. Jarzabek and J. L. Nielsen, Environ. Technol., 2016, 7, 1–10 Search PubMed.
- I. Pérez-Rodríguez, K. A. Bohnert, M. Cuebas, R. Keddis and C. Vetriani, FEMS Microbiol. Ecol., 2013, 86, 256–267 CrossRef PubMed.
- C. Chen, X. J. Xu, P. Xie, Y. Yuan, X. Zhou, A. J. Wang, D. J. Lee and N. Q. Ren, Chemosphere, 2017, 171, 294–301 CrossRef CAS PubMed.
- L. Christianson, C. Lepine, S. Tsukuda, K. Saito and S. Summerfelt, Aquacult. Eng., 2015, 68, 10–18 CrossRef.
- M. F. Shao, T. Zhang and H. H. Fang, Appl. Microbiol. Biotechnol., 2010, 88, 1027–1042 CrossRef CAS PubMed.
- J. Pang, M. Matsuda, M. Kuroda, D. Inoue, K. Sei, K. Nishida and M. Ike, Front. Environ. Sci. Eng., 2016, 10(4), 07 CrossRef.
- T. R. Thomsen, Y. Kong and P. H. Nielsen, FEMS Microbiol. Ecol., 2007, 60, 370–382 CrossRef CAS PubMed.
- Y. Xia, X. Wen, B. Zhang and Y. Yang, Biotechnol. Adv., 2018, 36, 1038–1047 CrossRef PubMed.
- G. X. Guo, H. Deng, M. Qiao, H. Y. Yao and Y. G. Zhu, Environ. Sci. Technol., 2013, 47(7), 3105–3113 CrossRef CAS PubMed.
- E. Attard, S. Recous, A. Chabbi, C. De Berranger, N. Guillaumaud, J. Labreuche, L. Philippot, B. Schmid and X. Le Roux, Global Change Biol., 2011, 17(5), 1975–1989 CrossRef.
- B. Stres, I. Mahne, G. Avguštin and J. M. Tiedje, Appl. Environ. Microbiol., 2004, 70(1), 301–309 CrossRef CAS PubMed.
- M. Andalib, G. Nakhla, E. McIntee and J. Zhu, Desalination, 2011, 279, 1–14 CrossRef CAS.
- A. E. Tugtas and S. G. Pavlostathis, Biotechnol. Bioeng., 2007, 96–3, 444–455 CrossRef PubMed.
Footnote |
† Electronic supplementary information (ESI) available. See DOI: 10.1039/c8ra08256a |
|
This journal is © The Royal Society of Chemistry 2018 |
Click here to see how this site uses Cookies. View our privacy policy here.