DOI:
10.1039/C8RA07615D
(Paper)
RSC Adv., 2018,
8, 41705-41712
Atomistic investigation of an Iowa Amyloid-β trimer in aqueous solution†
Received
13th September 2018
, Accepted 4th December 2018
First published on 13th December 2018
Abstract
The self-assembly of Amyloid beta (Aβ) peptides are widely accepted to associate with Alzheimer's disease (AD) via several proposed mechanisms. Because Aβ oligomers exist in a complicated environment consisting of various forms of Aβ, including oligomers, protofibrils, and fibrils, their structure has not been well understood. The negatively charged residue D23 is one of the critical residues of the Aβ peptide as it is located in the central hydrophobic domain of the Aβ N-terminal and forms a salt-bridge D23-K28, which helps stabilize the loop domain. In the familial Iowa (D23N) mutant, the total net charge of Aβ oligomers decreases, resulting in the decrease of electrostatic repulsion between D23N Aβ monomers and thus the increase in their self-aggregation rate. In this work, the impact of the D23N mutation on 3Aβ11–40 trimer was characterized utilizing temperature replica exchange molecular dynamics (REMD) simulations. Our simulation reveals that D23N mutation significantly enhances the affinity between the constituting chains in the trimer, increases the β-content (especially in the sequence 21–23), and shifts the β-strand hydrophobic core from crossing arrangement to parallel arrangement, which is consistent with the increase in self-aggregation rate. Molecular docking indicates that the Aβ fibril-binding ligands bind to the D23N and WT forms at different poses. These compounds prefer to bind to the N-terminal β-strand of the D23N mutant trimer, while they mostly bind to the N-terminal loop region of the WT. It is important to take into account the difference in the binding of ligands to mutant and wild type Aβ peptides in designing efficient inhibitors for various types of AD.
Introduction
World Health Organization (WHO) reported that dementia affected approximately 50 million people worldwide in 2017 and the number of new patients could increase to ca. 10 million annually.1 Alzheimer's disease (AD) is the most common type of dementia (60–70% cases),1 which is strongly linked to the self-aggregation of the Amyloid beta (Aβ) peptides.2 Aβ peptides consist of 36–43 residues formed via the proteolysis of the transmembrane Amyloid Precursor Protein (APP). According to the Amyloid cascade hypothesis,3–5 Aβ peptides self-assemble into Aβ oligomers, which have been shown to be neurotoxic via several mechanisms, including damaging neurites and synapses2,6 or forming transmembrane pores that disrupt Ca2+ homeostasis.7,8 The most abundant form of Aβ peptides consists of 40 amino acids (Aβ40) and accounts for approximately 80–90% of the total population.9 The development of AD therapy relies on the understanding of Aβ oligomer structures,10–13 unfortunately, because Aβ oligomers exist in complicated environments comprising of numerous forms of oligomers and fibrils, their structure has not been well characterized.11,14 Therefore, molecular dynamics simulation has been one of the main tools in assessing the structure of Aβ oligomers.15–17
The self-assembly process of Aβ peptides highly depends on their amino acid sequence.18 Mutations can alter the Aβ oligomer structures and their properties. Especially, the common mutations in the central hydrophobic domain of the Aβ N-terminal, including Flemish (A21G),19 Dutch (E22Q),20 Italian (E22K),21 Arctic (E22G),22 and Iowa (D23N)23 significantly affect the dynamics and conformations of the Aβ oligomers and fibrils. It is known that the central hydrophobic domains of an Aβ chain forms rigid β-structure that in turn forms strong interaction with other Aβ chains, leading to Aβ self-aggregation. Inhibitors that target the N-terminal β-strand have been shown to successfully inhibit Aβ self-aggregation.24–26
In the N-terminal hydrophobic domain, D23 stabilizes the loop region of Aβ peptides by forming a salt-bridge to the residue K28 and polar contacts to other residues in loop domain.27–29 Substituting the negatively charged residue D23 by another residue could significantly alter the structure of Aβ peptides. In the familial Iowa mutant, D23 is replaced by the neutral residue asparagine,23 which reduces the total net charge and thus the electrostatic repulsion between constituting chains of Aβ oligomers. Consequently, the self-aggregation rate of D23N oligomers has been shown to increase.30 Therefore, studying the impact of the D23N mutation on the self-assembly has been carried out for a number of Aβ peptide systems, including Aβ21–30,31 Aβ10–35,32 Aβ1–40,33 Aβ1–42,34 2Aβ1–40,35 and 6Aβ16–35.36 However, the effect of D23N mutation on Aβ40 trimer (3Aβ40), one of the most neurotoxic Aβ oligomers,10,11 has not been revealed.
We are interested in Aβ40 as it is the most abundance form of Aβ. Although Aβ42 oligomers are more neurotoxic than Aβ40 oligomers, the population of Aβ40 peptides is approximately ten times higher than that of Aβ42.9 Thus, structural investigation of Aβ40 and Aβ42 are both important. In addition, structure–neurotoxicity relationship has been established for Aβ40 oligomers but not for Aβ42 oligomers.37 In order to gain insights into the changes in the structure and dynamics of the neurotoxic Aβ trimer in aqueous environment upon D23N mutation, we investigated the D23N 3Aβ11–40 mutant in comparison with the wild type (WT) trimer using extensive replica exchange molecular dynamic (REMD) simulation over 20
000 ns in total. It is noted that the D23N Aβ peptides are able to form both parallel and anti-parallel motifs;38,39 however, in this work, we only considered the D23N 3Aβ11–40 starting from the parallel structure. Our results indicate that the D23N mutant has higher β-structure and stronger inter-chain interaction. We also identified seven optimized conformations of solvated D23N 3Aβ11–40 using the combination of free energy landscape and clustering methods. Finally, molecular docking method was used to evaluate the binding affinity of available inhibitors to D23N and WT Aβ11–40 trimer and monomer in order to reveal the influence of D23N mutant on AD therapy.
Materials and methods
Initial conformation
The three-dimensional fibril-like conformation of WT 3Aβ11–40 peptide was taken from the two-fold 12Aβ11–40 fibril.40 Then, the residue D23 was mutated to N using the mutagenesis tool in Pymol (Fig. 1).29 The backbone of trimer was not changed, but the sidechain of D23 was slightly different to N23. The distances between N23 and neighboring atoms remained larger than the corresponding atomic van der Waals (vdW) radii. D23N 3Aβ11–40 was then inserted into a dodecahedron periodic boundary condition (PBC) box with the volume of ∼463 nm3 and solvated with 12
852 water molecules. The mutant trimer was treated with AMBER99SB-ILDN force field41 and water molecules were presented utilizing TIP3P water model42 as described in previous works.29,43
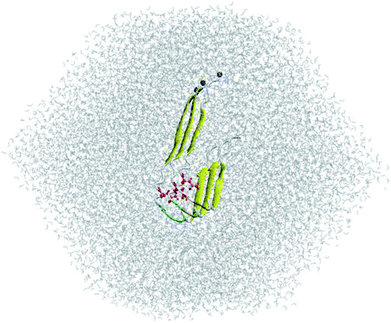 |
| Fig. 1 Input conformation of solvated D23N 3Aβ11–40. N23 residues are highlighted in red. N-termini are indicated with blue balls. | |
In addition, the D23N and WT Aβ11–40 monomers were derived from the trimer systems. Both monomers were solvated in a dodecahedron PBC box with size of ∼270 nm3 using ∼8600 water molecules. Na+ ions were added to WT Aβ11–40 monomer in order to neutralize the system.
Atomistic simulations
Atomistic simulations were carried out as previously described.29,44 GROMACS 5.0.6 (ref. 45) was employed to simulate the solvated D23N 3Aβ11–40 system. The non-covalent bond pair cut-off was set at 0.9 nm. The electrostatic potential was treated using the fast smooth particle-mesh Ewald electrostatic method.46 The vdW interaction was set at 0.9 nm. The first step of simulation was energy minimization with the steepest descent method, which was followed by a 500 ps positionally restraint simulation in NVT ensemble. REMD simulation was then applied to sample the conformations of solvated D23N 3Aβ11–40. 48 replicas at various temperatures ranging from 295 to 382 K (ESI†) were simulated as reported in our previous works.29,43 During REMD simulation, the exchanges were performed every 1 ps. Each replica was simulated over 417 ns. The solvated D23N and WT Aβ11–40 monomers were also simulated for 100 ns at 300 K in order to generate the target for molecular docking. The trimer conformations in REMD simulations were recorded every 10 ps, while monomeric conformations in MD simulations were monitored every 1 ps.
Structural analysis
The Defined Secondary Structure of Protein (DSSP) method was employed to predict the secondary structure term of the D23N 3Aβ11–40.47 The inter-/intra-molecular sidechain (inter-/intra-SC) contacts were counted when the spacing between non-hydrogen atoms are smaller than 0.45 nm. The inter-/intra-molecular hydrogen bond (HB) contacts were counted when the spacing between donor and acceptor is smaller than 0.35 nm and the angle between acceptor–hydrogen–donor is larger than 135°. The collective variable free energy landscape (FEL) was generated with two reaction coordinates, including the Cα root-mean-square deviation (RMSD) and gyration of radius Rg. The non-covalent bond interaction energy and solvent surface access area (SASA) were calculated using GROMACS tools.45 The collision cross section (CCS) was computed using the Ion Mobility Projection Approximation Calculation Tool (IMPACT).48
Binding free energy calculations
The binding free energy of an Aβ peptide chain to the other chains within D23N 3Aβ11–40 was calculated using MM/PBSA method49–51 as previously described.29
Molecular docking
The binding configuration and affinity of available inhibitors to Aβ trimers and monomers were investigated using Autodock Vina package52 as described previously.53 The input file of docking was prepared using AutodockTools 1.5.6.54 The docking grid was chosen at the center of mass of the peptide with the size of 60 × 60 × 60 nm, which is large enough to cover the entire peptides. The exhaustiveness was set as 400.
Results and discussion
REMD simulations of solvated D23N 3Aβ11–40
REMD simulation is known to be one of the most efficient sampling methods to evaluate the self-aggregation of Aβ peptides.15,16,55,56 Because simulation of the self-aggregation process of Aβ peptides is very slow and it is highly unlikely to reach the stable structure of Aβ oligomers starting from a random structure, input conformations were obtained from rom fibril-like structures,29,43,44 which was also applied in the case of Aβ11–40 trimer and its D23N mutant. REMD simulations were sufficient as the mean exchange rate of ca. 32% and the Aβ conformations entirely walked through the temperature space (Fig. S1†). The first 300 ns of REMD simulations were omitted from any analysis due to sidestepping any inclination toward the starting conformation. All computational metrics of the solvated D23N 3Aβ11–40 were only evaluated over the last 117 ns of REMD simulation at 300 K.
The simulation reached the equilibrium state at 300 K after 300 ns as indicated by the superposition of secondary structure metrics over various intervals 300–417 and 300–360 ns (Fig. S2†). All metrics were computed over all the snapshots of the simulation window 300–417 ns. As shown in Fig. 2, the β-content value diffuses in the range of 11–71% with the mean value of ∼48 ± 7%. It is significantly larger than that of WT 3Aβ11–40 (∼42 ± 6%).29 The shift of β-content distribution under the effect of D23N mutation is clearly shown in Fig. 2. Rg fluctuates in the range of 1.28–1.64 nm with an averaged value of ∼1.49 ± 0.05 nm, which is larger than the corresponding value of the WT trimer (∼1.43 ± 0.05 nm).29 SASA of D23N 3Aβ11–40 (∼62.84 ± 2.66 nm2) is slightly smaller than that of WT 3Aβ11–40 (∼63.51 ± 2.59 nm2).29
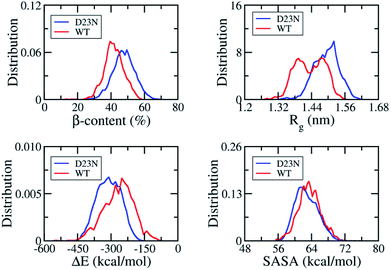 |
| Fig. 2 Distributions of D23N 3Aβ11–40 (red) and WT 3Aβ11–40 (blue) in REMD simulation time interval of 300–417 ns at 300 K. The values of WT 3Aβ11–40 were reproduced with permission of Royal Chemistry Society.29 | |
The non-covalent bond interaction energy between peptide chains of D23N 3Aβ11–40 was obtained using the GROMACS tool 4.3, which falls in the range from −515.7 to −160.5 kcal mol−1 (Fig. 2). The average interaction energy is −310.2 ± 53.3 kcal mol−1, which is significantly larger than that of WT 3Aβ11–40 (−267.0 ± 67.2 kcal mol−1).29 The stronger interaction energy between establishing chains is observed when the electrostatic repulsion is reduced. The obtained results indicated that the sidechain charge–charge interactions contribute to the instability of the WT trimer.57 This result implies that D23N 3Aβ11–40 is more stable and forms at higher rate in comparison to the WT trimer.
Effects of D23N mutation on the distribution of secondary structure parameters per residue
Fig. 3 shows the distribution of secondary structure terms per residues of the D23N 3Aβ11–40 including β-, α-, turn-, and coil-structures. Obtained results are consistent with the available solid state NMR data that showed a high neurotoxic Aβ40 oligomer possessing stable N-terminal β-strands.58 D23N 3Aβ11–40 adopts parallel U-shape structure consisting of five domains, which is in good agreement with a recent study.38 The sequences 11–14, 24–30, and 36–40 mostly form coil structure, while β-structure is dominant in the sequences 15–23 and 31–35. Residues 21–23 in the D23N mutant possess remarkably higher β-structure content compared to those in the WT trimer. Moreover, β-structure content is also observed to increase in other residues, including the sequence 12–20, while it only decreases in several residues, including the sequence 31–34. The loop region (sequence 24–28) forms more coil-structure and less α-/turn-structure in comparison with the WT form. Overall, the significant decrease in α-structure, an intermediate structure in the Aβ folding progress,59,60 together with the significant increase in β-structure could speed up the self-aggregation of D23N 3Aβ in comparison with that of the WT trimer.
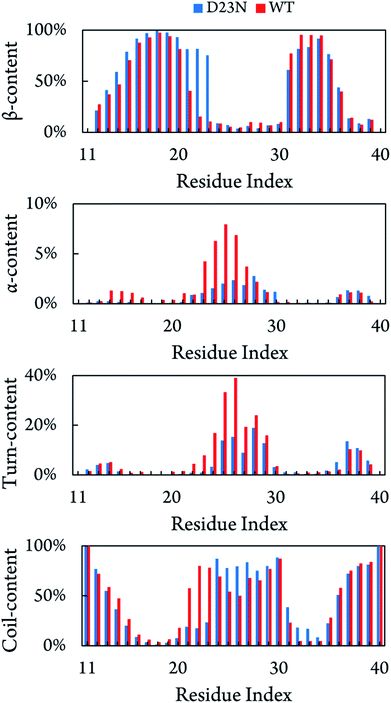 |
| Fig. 3 Secondary structure terms per residues averaged for all three chains of D23N 3Aβ11–40 and WT 3Aβ11–40.29 The values of WT 3Aβ11–40 were reproduced with permission of Royal Chemistry Society.29 | |
Non-covalent bond contacts
The stability of Aβ oligomer depends on the intermolecular non-covalent bonds between constituting chains, involving inter-SC and inter-HB contacts. The distributions of these values are shown in Fig. 4. As mentioned above, D23N Aβ11–40 forms more inter-SC contact (66.0 ± 4.5) than the WT system (61.8 ± 5.2).29 Similarly, D23N Aβ11–40 adopts more inter-HB contact (12.1 ± 5.0) than WT trimer (10.2 ± 1.7).29 Moreover, the number of intramolecular contacts of the D23N 3Aβ11–40 (135.7 ± 7.8) is significantly smaller than those of WT 3Aβ11–40 (171.2 ± 8.3).29 Likewise, the intra-HB of D23N 3Aβ11–40 (6.8 ± 2.0) is lower than that of the WT trimer (7.6 ± 2.9).29 This result is consistent with the significant increase in interaction energy between the peptide chains of the trimers obtained using the GROMACS tools described above, as well as with the increase in Gibbs binding free energy between peptide chains derived from MM/PBSA method (vide infra).
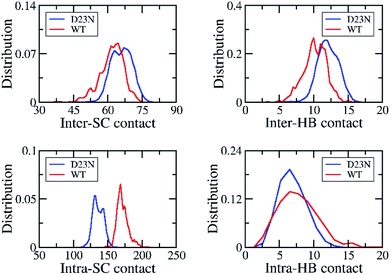 |
| Fig. 4 The distribution of inter/intra-molecular SC and HB contacts of the solvated D23 and WT 3Aβ11–40. The results of WT system was reproduced with permission of Royal of Chemistry Society.29 | |
Free energy landscape
The collective variable free energy landscape (FEL) of D23N 3Aβ11–40 was generated using the GROMACS tool named “sham”.45 Cα RMSD and Rg were chosen as the coordinates as they were successfully used to obtain the FEL for other Aβ peptide systems.61–63 The FEL of solvated D23N 3Aβ11–40 is displayed in Fig. 5, which contains four minima centered at (RMSD; Rg) coordinates of (0.48; 1.52), (1.50; 0.61), (0.68; 1.46), (0.66; 1.48). Applying clustering method on the refined MD snapshots located in the free energy holes, we found seven representative conformations of D23N 3Aβ11–40. These conformations are noted as A, B, C, D, E, F, and G as shown in Fig. 5.
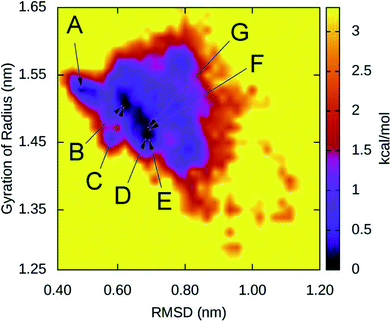 |
| Fig. 5 Collective variable free energy landscape of D23N 3Aβ11–40 generated with the reaction coordinates of Cα RMSD and Rg. Seven minima, namely A, B, C, D, E, F, and G, are found in four free energy holes. The corresponding (RMSD; Rg) coordinates of minima are (0.48; 1.52) for A, (0.61; 1.50) for B and C, (0.68; 0.68) for D and E, and (0.66; 1.48) for F and G. | |
The selected structural parameters of these conformations are provided in Table 1. The dimensions of these conformations, which are indicated by Rg, SASA, and CCS, are roughly comparable to those found for the representative structures of the WT trimers. The β-content of these structures is greater than 42%, which is significantly higher than that found for the representative structures of WT 3Aβ, namely MA (37%), MB (40%), and MC (32%).29 These results are consistent with the analysis of all equilibrated snapshots described above.
Table 1 Structural details of the solvated D23N 3Aβ11–40 peptide
|
Rg (nm) |
SASA (nm2) |
CCS (nm2) |
β (%) |
A |
1.52 |
61.70 |
13.19 |
51 |
B |
1.51 |
61.79 |
13.07 |
54 |
C |
1.50 |
63.08 |
13.38 |
47 |
D |
1.46 |
60.46 |
13.14 |
48 |
E |
1.46 |
58.97 |
12.83 |
46 |
F |
1.48 |
63.13 |
13.54 |
42 |
G |
1.48 |
58.80 |
12.91 |
58 |
The 3-D structures of A–G and their populations are shown in Fig. 6. All conformations exhibit the U-shaped parallel structures. As mentioned above, the β-strand of the N-terminal in the D23N mutant forms more β-structure as previously observed by solid state NMR.58 It is worthy to note that the central hydrophobic domain is solvent accessible, to which other Aβ peptides could dock, resulting in the self-aggregation.64 Unlike the β-strands in the WT trimer that form crossing structure,29 the β-strands in D23N 3Aβ11–40 are parallel to each other. Docking of additional monomer to the parallel structure of D23N 3Aβ11–40 is much more efficient than that to the crossing structure of the WT trimer.64
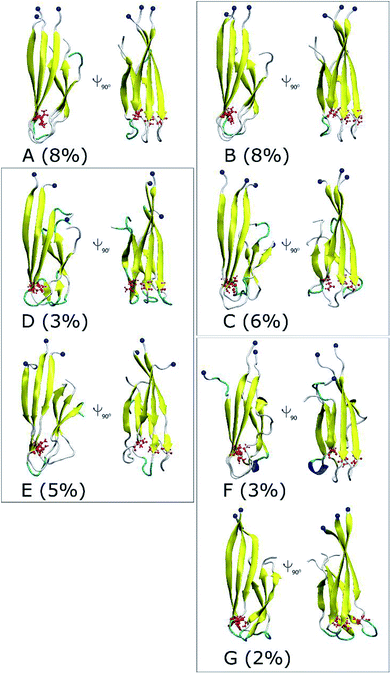 |
| Fig. 6 Representative structures of the solvated D23N 3Aβ11–40 obtained from the combination of FEL and clustering methods. The blue balls note the N-terminus of D23N trimer. | |
Binding affinity between peptide chains in Aβ11–40 trimers
The increase in the interaction energy and non-covalent bond contacts upon D23N mutation of 3Aβ11–40 described above suggest that the peptide chains in the mutant trimer bind more strongly to each other than in the WT trimer. To further access this result, we performed MM/PBSA calculation to evaluate the Gibbs free binding energy (ΔGbind) of a peptide chain to the other chains in D23N 3Aβ11–40, using the same protocol previously described for WT 3Aβ11–40.29 Evaluating binding affinity between peptide chains of the trimer using ΔGbind obtained with MM/PBSA method is more accurate than using the interaction energy obtained with GROMACS tool. The parameters obtained with MM/PBSA method are provided in Table 2. Replacing the negatively-charged D23 residue by a neutral N residue results in remarkably stronger electrostatic interaction (ΔEelec) and slightly stronger vdW interaction (ΔEvdW) as a result of weaker repulsion force between the peptide chains. The polar interaction is significantly stronger in the mutant, which off-set the increase in affinity between the chains. Nevertheless, the magnitude of ΔGbind increases significantly (by ∼22%) when D23 is mutated to N, which is consistent with the increase in the magnitude of interaction energy obtained with GROMACS tool.
Table 2 MMPBSA calculation parameters (kcal mol−1)a
|
ΔEelec |
ΔEvdW |
ΔGsur |
ΔGpolar |
−TΔS |
ΔGbind |
ΔEelec = electrostatic interaction energy; ΔEvdW = van der Waals interaction energy; ΔGsur = non-polar free energy of binding; ΔGpolar = polar free energy of binding; −TΔS = contribution of entropy to Gibbs free energy; the results of the WT system were reproduced with permission of Royal of Chemistry Society.29 |
WT29 |
−90.08 |
−144.92 |
−21.26 |
153.62 |
66.82 |
−35.82 ± 7.89 |
D23N |
−180.72 |
−126.39 |
−24.6 |
217.78 |
70.29 |
−43.67 ± 15.90 |
Binding of inhibitors to Aβ trimers and monomers
In order to gain more insights into how D23N mutation affects the structure of Aβ trimers, we compared the binding of twelve experimentally characterized amyloid plaque-binding compounds65–71 to D23N 3Aβ11–40 and WT 3Aβ11–40 using Autodock Vina.52 This tool has been used to study the binding of ligands to amyloid peptides and provided good correlation between predicted and experimental binding affinities.72,73 Molecular docking of these ligands was carried out for all representative structures of D23N and WT 3Aβ11–40. For each system, the binding affinity was averaged from the values obtained for all representative conformations to compensate for the lack of receptor-inhibitor dynamics in Autodock Vina. The exhaustiveness was chosen as 400 to enhance the docking accuracy.
The docking results are presented in Tables 3 and S1†. Except for one ligand, the estimated binding affinity of the ligands to D23N 3Aβ11–40 is slightly higher than that to WT 3Aβ11–40, however, the difference is negligible. Fig. 7 shows the binding poses of the ligands to the representative conformations of D23N 3Aβ11–40 (A–G) and WT 3Aβ11–40 (MA–MC). Overall, the ligands bind to D23N 3Aβ11–40 in different positions compared to WT 3Aβ11–40. The binding poses of the ligands to D23N 3Aβ11–40 are relatively wide spread, but in most cases they bind to the N-terminal β-strands. While the ligands also bind to the N-terminal domain of WT 3Aβ11–40, they mostly dock to the loop regions in similar poses in each conformation. This result suggests that these ligands could inhibit the aggregation of D23N and WT Aβ by different mechanisms. Binding of the ligands to the β-strands may inhibit the docking of new Aβ chain to the existing oligomers, while binding of the ligand to the loop regions could lead to the destabilization of the oligomers.
Table 3 Binding affinity (kcal mol−1) of selected inhibitors to D23N and WT 3Aβ11–40
No. |
PubChem ID |
Ref. |
D23N 3Aβ11–40 |
WT 3Aβ11–40 |
1 |
156 391 |
65 |
−5.8 |
−5.4 |
2 |
234 475 |
66 |
−5.4 |
−5.2 |
3 |
1 358 096 |
67 |
−6.0 |
−5.7 |
4 |
9 835 167 |
66 |
−5.7 |
−5.5 |
5 |
9 837 643 |
66 |
−5.6 |
−5.4 |
6 |
9 839 907 |
68 |
−5.7 |
−5.5 |
7 |
10 133 297 |
69 |
−5.7 |
−5.5 |
8 |
10 335 228 |
66 |
−5.5 |
−5.2 |
9 |
10 422 457 |
66 |
−5.7 |
−5.5 |
10 |
10 444 413 |
66 |
−5.7 |
−5.5 |
11 |
10 844 298 |
70 |
−6.2 |
−6.1 |
12 |
16 087 303 |
71 |
−4.8 |
−4.9 |
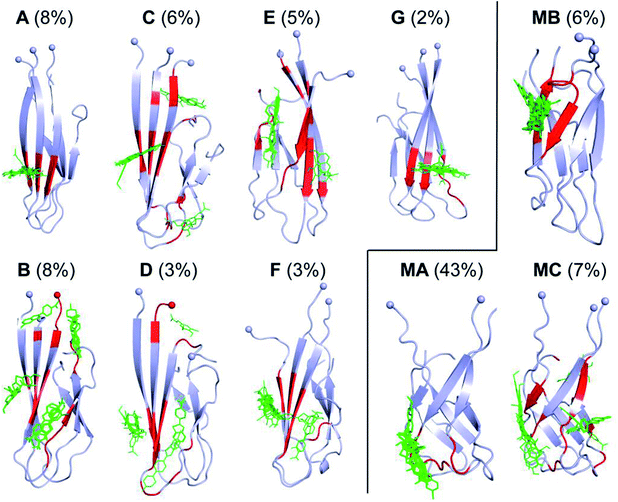 |
| Fig. 7 Binding poses of twelve ligands to the representative conformations of D23N 3Aβ11–40 (A–G) (this work) and WT 3Aβ11–40 (MA–MC) (obtained from our previous study)29 generated using Autodock Vina protocol.52 The residues highlighted by red color form non-bonded contacts to the ligands. Spheres denote the Cα atoms of the N-terminal. | |
In addition, the inhibitors were also docked to the D23N and WT Aβ11–40 the representative structures of the monomers obtained from FEL and clustering methods (Fig. S4†). D23N mutation cause significant changes in the structure of Aβ11–40 monomer (Fig. S4†). The binding poses of the inhibitors to D23N monomer are different from those to WT Aβ11–40 monomer (Fig. S5†). The average ligand binding affinity of D23N monomer (−5.0 ± 0.2 kcal mol−1) is 0.7 kcal mol−1 smaller than that of D23N trimer (−5.7 ± 0.3 kcal mol−1) (Table S2†). Similarly, the average ligand binding affinity of WT Aβ11–40 (−4.8 ± 0.2) is 0.7 kcal mol−1 smaller than that of WT 3Aβ11–40 (−5.5 ± 0.3 kcal mol−1). This result indicates that the inhibitors have higher affinity for the trimers than for the monomers. It also shows that D23N mutation also causes changes in ligand binding poses of the monomers, further corroborating the results obtained for the trimers.
Conclusions
Atomistic structural and dynamic information of the (11–40) truncated Iowa Aβ mutant trimer (D23N 3Aβ11–40) were extensively simulated using REMD with 48 replicas over simulation time of 417 ns per replica (∼20
000 ns of total simulations time). Replacing the negatively-charged D23 residue by the neutral residue N increases β-structure content from ∼42% to ∼48%, which occurs concomitantly with a shift in the hydrophobic β-strand core from crossing to parallel arrangement (Fig. 7). Subsequent docking of other Aβ chains via dock-lock mechanism64,74 to the existing oligomer with parallel hydrophobic core could occur much more efficiently than to the oligomer with crossing core. The number of non-covalent contacts increases sizably in the D23N mutant trimer, which is consistent with the increase in the magnitude of both the interaction energy (from −267.0 ± 67.2 kcal mol−1 to −310.2 ± 53.3 kcal mol−1) derived with the GROMACS tool and the Gibbs free binding energy (from −35.82 ± 7.89 to −43.67 ± 15.90) obtained with MM/PBSA method. Altogether, our results show that D23N mutation induces significant changes in structure and dynamics of 3Aβ11–40 that allow the mutant peptide to aggregate at faster rate, consistent with previous experimental results on the formation of Iowa Aβ fibrils.30
Molecular docking shows that D23N mutation causes significant changes of Aβ11–40 structure. These changes lead to differences in ligand binding poses of D23N and WT trimers despite having similar binding affinity. The ligands mostly bind to the N-terminal β-strand of the D23N mutant trimer, while they prefer to bind to the N-terminal loop region of the WT trimer. Likewise, these compounds bind to D23N and WT Aβ11–40 monomers at different binding positions while having the same range in binding affinity. This result provides important information for further study in drug design for different types of AD. Rigorous MD simulations are needed to gain further insights into this aspects.
Conflicts of interest
There are no conflicts to declare.
Acknowledgements
This research is funded by Vietnam National Foundation for Science and Technology Development (NAFOSTED) under grant number 103.01-2016.48.
Notes and references
- WHO, Dimentia, http://www.who.int/en/news-room/fact-sheets/detail/dementia, (accessed July 29, 2018).
- D. J. Selkoe, Science, 2002, 298, 789–791 CrossRef CAS.
- K. Beyreuther and C. L. Masters, Brain Pathol., 1991, 1, 241–251 CrossRef CAS.
- J. Hardy and D. Allsop, Trends Pharmacol. Sci., 1991, 12, 383–388 CrossRef CAS.
- D. J. Selkoe, Neuron, 1991, 6, 487–498 CrossRef CAS.
- S. Chimon, M. A. Shaibat, C. R. Jones, D. C. Calero, B. Aizezi and Y. Ishii, Nat. Struct. Mol. Biol., 2007, 14, 1157 CrossRef CAS PubMed.
- T. L. Williams and L. C. Serpell, FEBS J., 2011, 278, 3905–3917 CrossRef CAS PubMed.
- A. Quist, I. Doudevski, H. Lin, R. Azimova, D. Ng, B. Frangione, B. Kagan, J. Ghiso and R. Lal, Proc. Natl. Acad. Sci. U. S. A., 2005, 102, 10427–10432 CrossRef CAS.
- M. P. Murphy and H. LeVine, J. Alzheimers Dis., 2010, 19, 311 Search PubMed.
- S. J. C. Lee, E. Nam, H. J. Lee, M. G. Savelieff and M. H. Lim, Chem. Soc. Rev., 2017, 46, 310–323 RSC.
- M. K. Jana, R. Cappai, C. L. L. Pham and G. D. Ciccotosto, J. Neurochem., 2016, 136, 594–608 CrossRef CAS PubMed.
- S. T. Ngo, S.-T. Fang, S.-H. Huang, C.-L. Chou, P. D. Q. Huy, M. S. Li and Y.-C. Chen, J. Chem. Inf. Model., 2016, 56, 1344–1356 CrossRef CAS PubMed.
- D. J. Selkoe and J. Hardy, EMBO Mol. Med., 2016, 8, 595–608 CrossRef CAS.
- S. Banerjee, Z. Sun, E. Y. Hayden, D. B. Teplow and Y. L. Lyubchenko, ACS Nano, 2017, 11, 12202–12209 CrossRef CAS PubMed.
- P. H. Nguyen, F. Sterpone, J. M. Campanera, J. Nasica-Labouze and P. Derreumaux, ACS Chem. Neurosci., 2016, 7, 823–832 CrossRef CAS.
- S. T. Ngo, M. T. Nguyen, N. T. Nguyen and V. V. Vu, J. Phys. Chem. B, 2017, 121, 8467–8474 CrossRef CAS.
- S. T. Ngo, Commun. Phys., 2018, 28, 265–276 CrossRef.
- L. K. Thompson, Proc. Natl. Acad. Sci. U. S. A., 2003, 100, 383–385 CrossRef CAS PubMed.
- L. Hendriks, C. M. van Duijn, P. Cras, M. Cruts, W. Van Hul, F. van Harskamp, A. Warren, M. G. McInnis, S. E. Antonarakis, J.-J. Martin, A. Hofman and C. Van Broeckhoven, Nat. Genet., 1992, 1, 218–221 CrossRef CAS.
- E. Levy, M. Carman, I. Fernandez-Madrid, M. Power, I. Lieberburg, S. van Duinen, G. Bots, W. Luyendijk and B. Frangione, Science, 1990, 248, 1124–1126 CrossRef CAS.
- O. Bugiani, A. Padovani, M. Magoni, G. Andora, M. Sgarzi, M. Savoiardo, A. Bizzi, G. Giaccone, G. Rossi and F. Tagliavini, Neurobiol. Aging, 1998, 19, S238 Search PubMed.
- C. Nilsberth, A. Westlind-Danielsson, C. B. Eckman, M. M. Condron, K. Axelman, C. Forsell, C. Stenh, J. Luthman, D. B. Teplow, S. G. Younkin, J. Naslund and L. Lannfelt, Nat. Neurosci., 2001, 4, 887–893 CrossRef CAS PubMed.
- T. J. Grabowski, H. S. Cho, J. P. G. Vonsattel, G. W. Rebeck and S. M. Greenberg, Ann. Neurol., 2001, 49, 697–705 CrossRef CAS.
- D. Sehlin, H. Englund, B. Simu, M. Karlsson, M. Ingelsson, F. Nikolajeff, L. Lannfelt and F. E. Pettersson, PLoS One, 2012, 7, e32014 CrossRef CAS.
- L. Lannfelt, C. Möller, H. Basun, G. Osswald, D. Sehlin, A. Satlin, V. Logovinsky and P. Gellerfors, Alzheimer's Res. Ther., 2014, 6, 16 CrossRef.
- C. Swanson, A Study to Evaluate Safety, Tolerability, and Efficacy of BAN2401 in Subjects With Early Alzheimer's Disease, https://clinicaltrials.gov/ct2/show/NCT01767311, (accessed July 29, 2018) Search PubMed.
- B. Tarus, J. E. Straub and D. Thirumalai, J. Mol. Biol., 2005, 345, 1141–1156 CrossRef CAS PubMed.
- B. Ma and R. Nussinov, Proc. Natl. Acad. Sci. U. S. A., 2002, 99, 14126–14131 CrossRef CAS PubMed.
- S. T. Ngo, H. M. Hung, D. T. Truong and M. T. Nguyen, Phys. Chem. Chem. Phys., 2017, 19, 1909–1919 RSC.
- X. Yang, G. Meisl, B. Frohm, E. Thulin, T. P. J. Knowles and S. Linse, Proc. Natl. Acad. Sci. U. S. A., 2018, 115, E5849–E5858 CrossRef CAS PubMed.
- B. Tarus, J. E. Straub and D. Thirumalai, J. Mol. Biol., 2008, 379, 815–829 CrossRef CAS PubMed.
- W. Han and Y.-D. Wu, J. Am. Chem. Soc., 2005, 127, 15408–15416 CrossRef CAS.
- S. Côté, P. Derreumaux and N. Mousseau, J. Chem. Theory Comput., 2011, 7, 2584–2592 CrossRef.
- S.-H. Chong, J. Yim and S. Ham, Mol. BioSyst., 2013, 9, 997–1003 RSC.
- S. Côté, R. Laghaei, P. Derreumaux and N. Mousseau, J. Phys. Chem. B, 2012, 116, 4043–4055 CrossRef PubMed.
- W. Han and Y.-D. Wu, Proteins, 2007, 66, 575–587 CrossRef CAS.
- K. Ono, M. M. Condron and D. B. Teplow, Proc. Natl. Acad. Sci. U. S. A., 2009, 106, 14745–14750 CrossRef CAS.
- N. G. Sgourakis, W.-M. Yau and W. Qiang, Structure, 2015, 23, 216–227 CrossRef CAS PubMed.
- W. Qiang, W.-M. Yau, Y. Luo, M. P. Mattson and R. Tycko, Proc. Natl. Acad. Sci. U. S. A., 2012, 109, 4443–4448 CrossRef CAS PubMed.
- I. Bertini, L. Gonnelli, C. Luchinat, J. Mao and A. Nesi, J. Am. Chem. Soc., 2011, 133, 16013–16022 CrossRef CAS.
- K. Lindorff-Larsen, S. Piana, K. Palmo, P. Maragakis, J. L. Klepeis, R. O. Dror and D. E. Shaw, Proteins: Struct., Funct., Bioinf., 2010, 78, 1950–1958 CAS.
- W. L. Jorgensen, J. Chandrasekhar, J. D. Madura, R. W. Impey and M. L. Klein, J. Chem. Phys., 1983, 79, 926–935 CrossRef CAS.
- S. T. Ngo, X.-C. Luu, M. T. Nguyen, C. N. Le and V. V. Vu, RSC Adv., 2017, 7, 42379–42386 RSC.
- S. T. Ngo, H. M. Hung, N. D. Hong and N. T. Tung, J. Mol. Graphics Modell., 2018, 83, 122–128 CrossRef CAS.
- M. J. Abraham, T. Murtola, R. Schulz, S. Páll, J. C. Smith, B. Hess and E. Lindahl, SoftwareX, 2015, 1–2, 19–25 CrossRef.
- T. Darden, D. York and L. Pedersen, J. Chem. Phys., 1993, 98, 10089–10092 CrossRef CAS.
- W. G. Touw, C. Baakman, J. Black, T. A. H. te Beek, E. Krieger, R. P. Joosten and G. Vriend, Nucleic Acids Res., 2015, 43, D364–D368 CrossRef CAS.
- E. G. Marklund, M. T. Degiacomi, C. V. Robinson, A. J. Baldwin and J. L. P. Benesch, Structure, 2015, 23, 791–799 CrossRef CAS.
- J. Srinivasan, T. E. Cheatham, P. Cieplak, P. A. Kollman and D. A. Case, J. Am. Chem. Soc., 1998, 120, 9401–9409 CrossRef CAS.
- P. A. Kollman, I. Massova, C. Reyes, B. Kuhn, S. Huo, L. Chong, M. Lee, T. Lee, Y. Duan, W. Wang, O. Donini, P. Cieplak, J. Srinivasan, D. A. Case and I. T. E. Cheatham, Acc. Chem. Res., 2000, 33, 889–897 CrossRef CAS.
- H. K. Srivastava and G. N. Sastry, J. Chem. Inf. Model., 2012, 52, 3088–3098 CrossRef CAS PubMed.
- O. Trott and A. J. Olson, J. Comput. Chem., 2010, 31, 455–461 CAS.
- S. T. Ngo and M. S. Li, Mol. Simul., 2013, 39, 279–291 CrossRef CAS.
- G. M. Morris, R. Huey, W. Lindstrom, M. F. Sanner, R. K. Belew, D. S. Goodsell and A. J. Olson, J. Comput. Chem., 2009, 30, 2785–2791 CrossRef CAS PubMed.
- V. Knecht, J. Phys. Chem. B, 2010, 114, 12701–12707 CrossRef CAS PubMed.
- S. T. Ngo, H. M. Hung, K. N. Tran and M. T. Nguyen, RSC Adv., 2017, 7, 7346–7357 RSC.
- J. A. Lemkul, J. Huang and A. D. MacKerell, J. Phys. Chem. B, 2015, 119, 15574–15582 CrossRef CAS PubMed.
- C. Haupt, J. Leppert, R. Rönicke, J. Meinhardt, J. K. Yadav, R. Ramachandran, O. Ohlenschläger, K. G. Reymann, M. Görlach and M. Fändrich, Angew. Chem., Int. Ed., 2012, 51, 1576–1579 CrossRef CAS.
- M. D. Kirkitadze, M. M. Condron and D. B. Teplow, J. Mol. Biol., 2001, 312, 1103–1119 CrossRef CAS.
- Y. Fezoui and D. B. Teplow, J. Biol. Chem., 2002, 277, 36948–36954 CrossRef CAS PubMed.
- L. Tran, N. Basdevant, C. Prévost and T. Ha-Duong, Sci. Rep., 2016, 6, 21429 CrossRef CAS.
- L. Tran, S. T. Ngo and M. T. Nguyen, Chem. Phys. Lett., 2018, 696, 55–60 CrossRef CAS.
- S. T. Ngo, X.-C. Luu, N. T. Nguyen, V. V. Vu and H. T. T. Phung, PLoS One, 2018, 13, e0204026 CrossRef PubMed.
- A. Baumketner, M. G. Krone and J.-E. Shea, Proc. Natl. Acad. Sci. U. S. A., 2008, 105, 6027–6032 CrossRef CAS PubMed.
- E. D. Agdeppa, V. Kepe, A. Petric, N. Satyamurthy, J. Liu, S. C. Huang, G. W. Small, G. M. Coled and J. R. Barrio, Neuroscience, 2003, 117, 723–730 CrossRef CAS.
- C. A. Mathis, Y. M. Wang, D. P. Holt, G. F. Huang, M. L. Debnath and W. E. Klunk, J. Med. Chem., 2003, 46, 2740–2754 CrossRef CAS PubMed.
- D. Alagille, H. DaCosta, R. M. Baldwin and G. D. Tamagnan, Bioorg. Med. Chem. Lett., 2011, 21, 2966–2968 CrossRef CAS PubMed.
- Z. P. Zhuang, M. P. Kung, A. Wilson, C. W. Lee, K. Plossl, C. Hou, D. M. Holtzman and H. F. Kung, J. Med. Chem., 2003, 46, 237–243 CrossRef CAS PubMed.
- C.-W. Lee, Z.-P. Zhuang, M.-P. Kung, K. Plössl, D. Skovronsky, T. Gur, C. Hou, J. Q. Trojanowski, V. M. Y. Lee and H. F. Kung, J. Med. Chem., 2001, 44, 2270–2275 CrossRef CAS PubMed.
- Z. P. Zhuang, M. P. Kung, C. Hou, D. M. Skovronsky, T. L. Gur, K. Plossl, J. Q. Trojanowski, V. M. Y. Lee and H. F. Kung, J. Med. Chem., 2001, 44, 1905–1914 CrossRef CAS.
- E. K. Ryu, Y. S. Choe, K. H. Lee, Y. Choi and B. T. Kim, J. Med. Chem., 2006, 49, 6111–6119 CrossRef CAS PubMed.
- S. T. Ngo and M. S. Li, J. Phys. Chem. B, 2012, 116, 10165–10175 CrossRef CAS PubMed.
- P. D. Huy, Y. C. Yu, S. T. Ngo, T. V. Thao, C. P. Chen, M. S. Li and Y. C. Chen, Biochim. Biophys. Acta, Gen. Subj., 2013, 1830, 2960–2969 CrossRef CAS PubMed.
- R. A. Rodriguez, L. Y. Chen, G. Plascencia-Villa and G. Perry, ACS Chem. Neurosci., 2018, 9, 783–789 CrossRef CAS PubMed.
Footnote |
† Electronic supplementary information (ESI) available: List of temperatures in REMD simulations. Additional figures displaying the diffusion of the first replica in the entire temperature space; the secondary structure distribution per residue of D23N 3Aβ11–40 in different simulation intervals; the RMSD and FEL of D23N and WT Aβ11–40 monomers; and docked conformations of ligands to monomers. See DOI: 10.1039/c8ra07615d |
|
This journal is © The Royal Society of Chemistry 2018 |
Click here to see how this site uses Cookies. View our privacy policy here.