DOI:
10.1039/C8RA00888D
(Review Article)
RSC Adv., 2018,
8, 17334-17345
The unequivocal preponderance of biocomputation in clinical virology
Received
29th January 2018
, Accepted 14th March 2018
First published on 18th May 2018
Abstract
Bioinformatics and computer based data simulation and modeling are captivating biological research, delivering great results already and promising to deliver more. As biological research is a complex, intricate, diverse field, any available support is gladly taken. With recent outbreaks and epidemics, pathogens are a constant threat to the global economy and security. Virus related plagues are somehow the most difficult to handle. Biocomputation has provided appreciable help in resolving clinical virology related issues. This review, for the first time, surveys the current status of the role of computation in virus related research. Advances made in the fields of clinical virology, antiviral drug design, viral immunology and viral oncology, through input from biocomputation, have been discussed. The amount of progress made and the software platforms available are consolidated in this review. The limitations of computation based methods are presented. Finally, the challenges facing the future of biocomputation in clinical virology are speculated upon.
1. Introduction
Generally, viral outbreaks are pandemic and mostly transmitted via oral and nasal passages, the gastrointestinal tract, the skin and the urogenital tract/vagina. Viral diseases are not only a health threat, but they also result in outbreaks that have huge impacts on the global economy. Viral infections are a tremendous disease burden on humanity and combating them is an increasing challenge, since new viruses are continuously found. According to a recent World Health Organization (WHO) report, viral diseases which have recently caused outbreaks include: Ebola virus, influenza, human immunodeficiency virus (HIV), Middle East respiratory syndrome coronavirus (MERS-CoV), severe acute respiratory syndrome (SARS) and Zika viral infection.1 These diseases are major threats to health and global security. From 2013 to 2016, Ebola virus was most predominant in Guinea, Sierra Leone and Liberia. Since May 2016, 28
616 suspected cases have been found, 11
310 deaths reported and the fatality rate was approximately 70.8%.2,3 Outbreaks of Zika virus have been traced back to October 2015 in Brazil. The outbreak of Zika virus was evidenced by a rapid increase in cases among pregnant women, whose infants are born with extremely underdeveloped brains and will grow up to be adults with limited cognitive abilities and motor skills. The World Bank estimated that this outbreak caused a loss to the global economy to the magnitude of a total of $8.9 billion USD.4 The identification and characterization of the causative agents and prophylaxis to limit the spread of a virus require the successful isolation of viral isolates via ‘wet-lab’ experimentation. This is necessary for curbing these outbreaks, in order to facilitate restoration.
1.1 Importance of clinical virology
With morbidity and mortality rates being significantly high with respect to virus related infections, clinical virology is at the forefront of research highlights. Clinical virology is a field of medicine which consists of the identification of viral pathogens responsible for human diseases like polio, chikungunya, severe acute respiratory disease (SARS), influenza, acquired immune deficiency syndrome (AIDS), Ebola hemorrhagic fever, hepatitis etc.5 Clinical virology is an interdisciplinary field, integrating virology/medical virology and healthcare sciences that characterize the safety and efficacy of medication, devices, diagnostic products and treatment regimes intended for mankind, which can be utilized for the prevention, treatment and diagnosis of infectious viral diseases. The effective prevention and clinical management of infectious diseases are intimately linked to the early and accurate screening of pathogens. This includes detecting the infectious particles in the organism and elucidating the aspects that confer resistance to therapy, mutations and genotype disparity. In this aspect, the accurate interpretation of laboratory results warrants the effective clinical management and control of a disease,6 however on the other hand, erroneous diagnosis could lead to financial and human loss. EM and culture-based methods work together and were one of the early traditional methods for the diagnosis of viral infections, along with serology testing for the detection of antibodies targeted against viruses. These conventional methods are still fundamental practices in many medical labs.7 Cell culturing is yet another popular method for isolating viruses using cell lines.8 The complement fixation test (CFT) is one of the oldest methods in the history of clinical virology.9 The haemagglutination inhibition test is generally used for detecting arboviruses and influenza and parainfluenza virus subtypes and is capable of yielding relative quantitation of the virus particles.10,11 More recent, new generation diagnostic methods include: immunoassay methods,12,13 amplification based assays,14,15 mass spectrometric methods16,17 and next generation sequencing.18,19
Biocomputational tools and database resources provide a wealth of valuable information about viral genomic sequences, molecular structures and viral–host pathogenesis. This information on infectious agents can lead to better diagnostics, therapeutics and vaccine development. Bioinformatics addresses these specific needs during data acquisition, storage and analysis and for the integration of this research with major therapeutic research areas such as viral oncology, viral immunology and antiviral research. In this review, the importance of exploring the role of biocomputation for additional knowledge in the realms of clinical virology is discussed. Advances made in the fields of clinical virology, antiviral drug design, viral immunology and viral oncology, through input from biocomputation, are recorded. Computation based approaches, their effects on clinical virology and their therapeutic usefulness are presented. Finally, the challenges facing the future of biocomputation in clinical virology are speculated upon.
2. Current status of biocomputational approaches in clinical virology
In virology, computational approaches have played crucial roles in various aspects of viral genome sequence analysis, therapeutic protein identification, anti-viral drug design/discovery, in silico vaccine design, differentially expressed gene identification, microRNA based signature identification and therapeutic design. Through in silico analysis, researchers gain a profound understanding of viral–host pathogenesis, which leads to better diagnostics, therapeutics and vaccines. Viruses have always been a major cause of a large number of infectious diseases. Molecular knowledge on viral proteins is thus seen to play an important role in the development of improved peptide-based vaccines, the design of novel anti-viral agents and the understanding of the entry mechanisms of viruses. Ongoing major research areas where biocomputation has played a positive role in clinical virology are represented in Fig. 1.
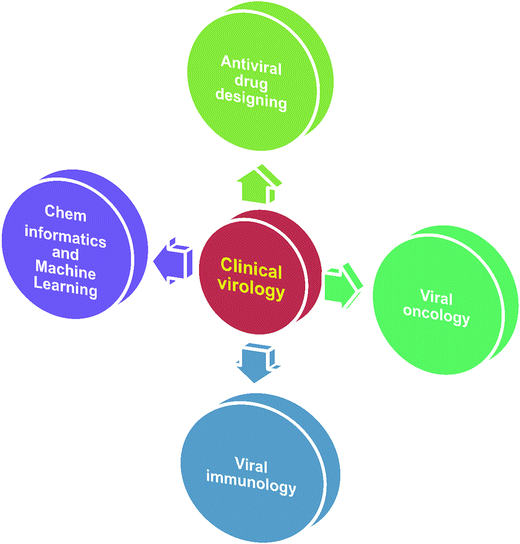 |
| Fig. 1 Scheme depicting the ongoing major research areas in clinical virology. | |
2.1 Biocomputation for antiviral drug design
Antiviral drugs are aimed at targeting selective viral infections. In particular, antiviral drugs have been designed against herpes, HIV, human cytomegalovirus (HCMV), varicella-zoster virus (VZV), hepatitis B and C, and influenza A and B viral infections which have caused chronic infection in millions all over the world.20 There are many difficulties facing the accurate design of an antiviral drug against pathogenic viruses. Since viruses are parasites, they are unable to replicate on their own. They reproduce within the cells of an infected host and disturb the functions of the host cells. Many clinically important viruses don’t have model systems due to their dangerous ability to mutate. Cultures are also hard to maintain and expensive. Viruses which show early infectious symptoms like influenza, common cold etc., are treated with antiviral drugs, but for viruses with symptoms that appear during the later stages of infection, the population is put at risk.21
A very early stage of viral infection is viral entry; a second approach is to target the processes that synthesize viral components after a virus invades a cell and establishes a critical infection. Based on the level of infection, the receptor protein and its therapeutic compounds are different.22 So before designing a drug, we need to ascertain the level of infection. To date, however, many viruses remain devoid of effective immunization and only a few antiviral drugs are licensed for clinical practice. Hence, there is an urgent need to discover novel antivirals that are highly efficacious and cost-effective for the management and control of viral infections at times when vaccines and standard therapies are lacking.23
Previously identified antiviral drugs do not destroy the target pathogen of the host, but instead they are able to reduce their growth and development. Modern in silico antiviral drug design aims to identify viral proteins (receptor proteins) that induce disease in humans or the survival of a microbial pathogen. Target proteins are common among many strains of viruses. Once the potent targets are identified, the appropriate drugs can be designed and administered.24 Target protein sequences were retrieved from sequence databases like viral genome databases (see Table 1) and structures will be retrieved from viral protein databases (Table 1). The binding site of the receptor protein is called the active site and this will be identified using in silico binding site prediction tools, such as active site prediction servers, CASTp,36 PDBeMotif,37 metaPocket,38 3DLigandSite,39 Pocketome,40 PocketDepth,41 Pocket-Finder,42 FINDSITE43 etc. Drugs can be designed which bind to the active region and inhibit receptor molecules. The structure of a drug molecule that can specifically interact with the biomolecules can be modeled using molecular modeling techniques via computational tools like I-TASSER,44 Robetta,45 HHpred,46 MODELLER,47 MODBASE,48 RaptorX,49 SWISS-MODEL50 etc.
Table 1 A consolidated list of various biocomputational resources used in clinical virology
Feature |
Computational resource |
Website |
Reference |
Viral sequence database |
Viral genomes resource |
https://www.ncbi.nlm.nih.gov/genome/viruses/ |
25 |
Virus pathogen database and analysis resource (ViPR) |
https://www.viprbrc.org/ |
26 |
Viral genome databases (VGDB) – Oxford academic |
https://www.oxfordjournals.org/nar/database/subcat/5/18 |
27 |
Viral reference sequences – ViralZone |
http://www.viralzone.expasy.org/6096 |
28 |
Influenza research database |
https://www.fludb.org/ |
29 |
viruSITE |
http://www.virusite.org/ |
30 |
RNAVirusDB |
http://virus.zoo.ox.ac.uk/rnavirusdb |
31 |
http://hivweb.sanbi.ac.za/rnavirusdb |
http://bioinf.cs.auckland.ac.nz/rnavirusdb |
http://tree.bio.ed.ac.uk/rnavirusdb |
Viral protein structure database |
VPDB |
http://vpdb.bicpu.edu.in/ |
32 |
VIDA |
http://www.biochem.ucl.ac.uk/bsm/virus_database |
33 |
VIPERdb |
http://viperdb.scripps.edu/ |
34 |
PhEVER |
http://pbil.univ-lyon1.fr/databases/phever |
35 |
Antiviral drug database |
Antiviral library |
http://www.enamine.net/ |
101 and 102 |
Drug office – oral antiviral drugs |
https://www.drugoffice.gov.hk/eps/do/en/consumer/news_informations/dm_17.html |
103 |
Virus pathogen database and analysis resource (ViPR) |
https://www.viprbrc.org/ |
26 |
Database of anticancer peptides and proteins (CancerPPD) |
http://crdd.osdd.net/raghava/cancerppd/ |
104 |
Cancer drug resistance database (CancerDR) |
http://crdd.osdd.net/raghava/cancerdr/ |
105 |
CancerHSP |
http://lsp.nwsuaf.edu.cn/CancerHSP.php |
106 |
http://www.inpacdb.org |
107 |
Microarray data |
Stanford microarray database (SMD) |
http://genome-www.stanford.edu/microarray/ |
108 |
GEO datasets |
https://www.ncbi.nlm.nih.gov/geo/info/datasets.html |
109 |
The first antiviral drug 5-iodo-2′-deoxyuridine (idoxuridine, IDU) was designed for the herpes virus infection in 1960.51 Computer aided drug design (CADD) played an important role in designing a suitable antiviral drug against the deadly viral infection. There are two types of CADD, structure based and ligand based.52 The available drugs were retrieved from antiviral drug databases (see Table 1). The successful design of antiviral drugs like Saquinavir, Relenza, and Tamiflu has validated the application of these techniques and indicates a bright future for drug discovery protocols.53 The creation of a structure based pharmacophore model from the 3D structure of a target protein provides helpful information for investigating protein–ligand interactions and upgrading the knowledge of ligand binding affinities. The structure based pharmacophore regions are used for screening chemical databases for potential lead structures. CATALYST,54 PharmDock,55 PHASE,56 and ROCS57 are used for pharmacophore modeling. Molecular docking is used to study the interactions between a target protein and a ligand molecule. Tools and software used for molecular docking include: AutoDock,58 DOCK,59 Glide,60 GOLD,61 PatchDock62 etc. Molecular dynamics (MD) simulations can be used to provide dynamic insight into the structures of viruses and their components.63 These simulations can be performed using CHARMM,64 Desmond,65 GROMACS66 etc. A flowchart for a typical antiviral drug design process is shown in Fig. 2.
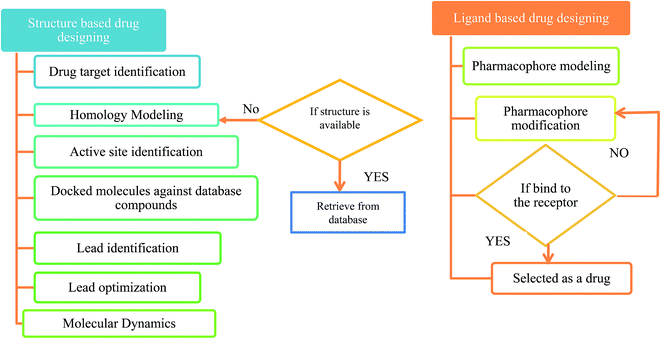 |
| Fig. 2 A flowchart for antiviral drug design. | |
2.2 Biocomputation in viral immunology and subsequent in silico vaccine design
Viral immunology is the most challenging and rapidly growing field in biology. During computational approaches, it relates to computational immunology or immunoinformatics, which deal with immunological problems using computational methods.67 Generally, the immune system connects thousands of molecules which become closely and intricately linked with each other; based on individuals the structure and the function of these molecules are different. Experimentally, immunologists have generated a vast amount of functional, clinical and epidemiological data. Using in silico techniques, these data have been stored and analyzed. In silico methods are affordable, because they reduce the time and costs involved in the laboratory analysis of pathogenic proteins. In vivo methods require the cultivation of the pathogenic genome in order to identify antigenic proteins. Although pathogens grow quickly, the extraction of their proteins and then the testing of those proteins on a large scale is expensive and time consuming.68 The use of immunoinformatics solves these problems and can identify virulence genes, potential target proteins and binding sites; this will lead to the development of novel vaccines and immunotherapeutics. This in silico method for vaccine design is called reverse vaccinology.
In 1796, Edward Jenner first identified the smallpox vaccine and introduced the term vaccine.69 A century later, Louis Pasteur introduced a rational method for vaccine development.70 Subsequently, researchers discovered many in vivo vaccines like the polio vaccine (Albert Sabin),71 measles, mumps and rubella (Hilleman),72 and so on. In later years, researchers faced the challenge of cultivating pathogenic viruses. It was found that in the case of hepatitis B viral infection, the pathogenic virus could not be cultured in vitro. Thus, they collected the viral antigen from chronically infected patients, the antigens were inactivated and the vaccine was developed.73 At the end of the 20th century, researchers developed vaccines based on the genomes of microorganisms. The first pathogenic organism identified by the reverse vaccinology approach was meningococcus B.74 Recently, Reza Taherkhani and Fatemeh Farshadpour developed an epitope based vaccine for the hepatitis E viral infection75 and vaccines have been designed for other recent outbreaks including for the Zika virus76 and the Ebola virus.77
An adaptive immune response occurs against a pathogen. There are two types of adaptive immune response, cellular and humoral, mediated by T cells and B cells. The antigenic determinant region is called the epitope, which is recognized by the corresponding receptor present on B or T cells.78 In bioinformatics, epitope based vaccine design relies on machine learning techniques, such as support vector machines (SVMs), hidden Markov models (HMMs) and neural networks (NNs).79–81 The in silico vaccine design process requires the identification of a key molecule on the receptor protein, which is predicted using the Epitope Database (IEDB).82 Then, the immunogenetic peptides will be identified using Kolaskar and Tongaonkar’s predicted antigenic peptide tools, based on applied semi empirical methods,83 and the peptide-epitope will be mapped. Subsequently, peptide and immunodominant epitope docking will be performed for vaccine design. Molecular dynamics based simulations will be carried out for conformational analysis. Finally, the peptide will be synthesized for practical application. The steps involved in in silico vaccine design are shown in Fig. 3.
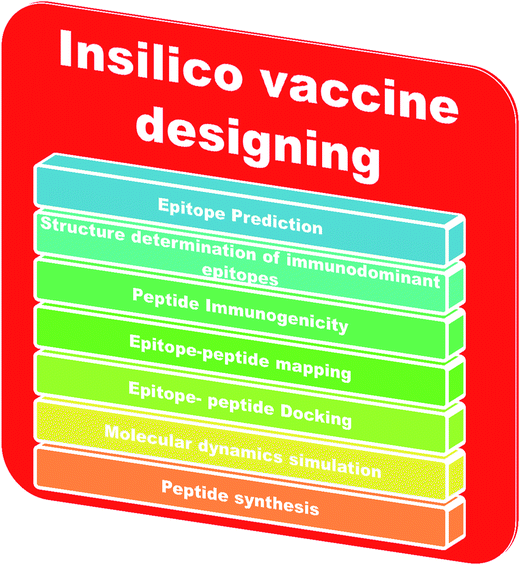 |
| Fig. 3 Steps in in silico vaccine design. | |
2.3 Biocomputational inputs in viral oncology
Viral oncology is an ongoing and essential research field for biologists. According to a report in 2017 by the American Cancer Society, 1
688
780 cancer cases were expected to be treated in 2017.84 Certain tumors are reported to be caused by viruses like the human papilloma virus (HPV), Epstein–Barr virus (EBV), Kaposi’s sarcoma-associated herpesvirus (KSHV), hepatitis C virus (HCV), human immunodeficiency virus (HIV) and hepatitis B virus (HBV). More than 90% of anal cancers have been caused by 500
000 HPV infections per year worldwide.85 Traditionally, viral oncology research has relied on biological techniques, but recently, computational techniques have enabled the diagnosis of malignancies.
In recent years, high throughput technologies have generated vast amounts of data, but on the other hand only limited experimental information is available for most genes. Next Generation Sequencing (NGS) plays an especially important role in cancer therapeutics. Computers can analyze vast amounts of data using remarkable techniques based on supercomputers. Computational oncology techniques have created in silico biological system models. To understand normal vs. malignant patient sequence characteristics, bioinformaticians first retrieved enormous sequences from various databases.86 Tumor suppressor genes (TSG), sequence annotation, their relation to diseases and gene ontology (GO) processes are identified using these databases. Cellular pathway databases have played an important role in identifying the essential proteins that induce disease, constructing biological networks and predicting models.87 Using traditional methods, only single genotype–phenotype relationships can be identified at a time. In contrast, high-throughput technology examines the phenotypic outcomes of multiple mutations simultaneously.88 Fig. 4 presents the typical process steps of the NGS of clinical data.
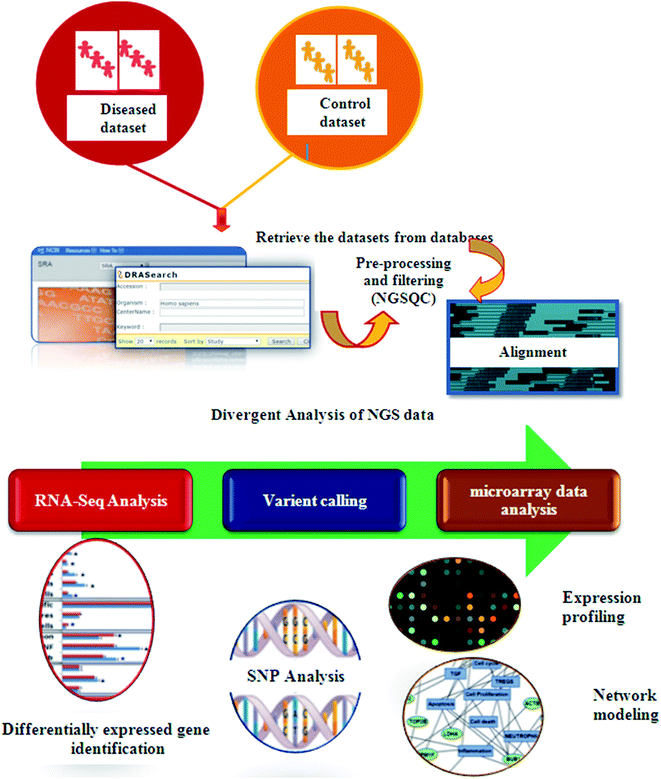 |
| Fig. 4 Schematic representation of the process steps of the NGS of clinical data. | |
Microarrays contain thousands of gene expressions and transcriptome data. Using a computer, we can analyse microarray data. From transcriptome data, differentially expressed genes can be identified using NGS techniques. The differentially expressed genes lead to the identification of cellular target genes that identify the therapeutic compound.89,90 Computer based drug design techniques use the pharmacokinetics and pharmacodynamic relationships of the available anticancer agents to improve treatment and drug development. These techniques include virtual screening, QSAR (quantitative structure activity relationship) models and molecular docking.91 Pharmacophore-mapping algorithms are employed for the inverse screening of some representative compounds for a large set of pharmacophore models constructed from human target proteins. Molecular docking studies were carried out to assess the binding affinity of these compounds to proteins responsible for mediating tumor growth. Furthermore, the important structural features of compounds for anticancer activity were assessed using Monte Carlo-based SMILES and hydrogen graph-based QSAR studies.92
2.4 Cheminformatics and machine learning models for anti-viral drug discovery
Structure activity relationships (SARs) with molecular systems, quantitative structure activity relationships (QSARs) and quantitative structure–property relationships (QSPRs) with molecules play a major role in computational drug discovery. In this context, machine learning approaches for viral drug discovery are pioneering as an emerging and ongoing research technique in clinical virology. Machine learning approaches can help make complex network models for confirming the effectiveness of combinations of drugs for epidemiological outbreaks in large populations. In 2015, Herrera and his coworkers collected datasets from ChEMBL (anti-HIV chemical compounds), the AIDSVu database (HIV surveillance reports) and the Census Bureau (socioeconomic data) and proposed the first artificial neural network (ANN) model for the prediction of HAART cocktails, to halt AIDS in the epidemic networks of the U.S.93 The first multitasking model for quantitative structure–biological effect relationships (mtk-QSBERs) was predicted, in silico fragment based drug design for drug–molecule interaction study was performed and its molecular entities were screened by virtual screening for the Hepatitis C viral infection. This was then experimentally validated for anti-HCV activity and ADMET (Absorption, Distribution, Metabolism, Excretion and Toxicity) properties.94 Very recently, the first multitasking model was developed for anti-HIV agents from 29
682 HIV cases using quantitative structure–biological effect relationships (mtk-QSBER) based on fragment based drug design and virtual screening approaches. More than 96% of fragments contributed towards the multiple biological effects.95
ANNs have been used to link data related to AIDS in the U.S. to ChEMBL data. ANNs are network prediction models that are mainly used in medicinal chemistry and drug development.96 Moreover, in 2008 Francisco and coworkers constructed drug–drug complex networks against different species of virus.97 González-Díaz et al., used the MARkovian CHemicals IN SIlico DEsign (MARCH-INSIDE) approach and predicted novel antimicrobial drugs and targets using drug–drug similarity complex networks.98 Computational studies of structural stability relationships produce novel stochastic moments. In 2005, González-Díaz et al. constructed a new Markov model, which makes use of novel stochastic moments such as molecular descriptors for viral protein surfaces in quantitative structure–activity relationship (QSAR) studies for small molecules for human rhinoviruses (HRVs).99 Markovian Backbone Negentropies (MBNs) have been introduced in order to model their effects on protein structure stability relationships. An MBN based on a Markov chain model of electron delocalization throughout the protein backbone for the computational study of structure/stability relationships has also been reported.100 These approaches mainly focus on cheminformatic approaches towards drug design, drug development and drug–target interactions in in silico clinical fields, especially with respect to viral diseases.
2.5 Challenges facing biocomputation in clinical virology
Antiviral drug discovery and design processes are not exceptionally without fault; they do have certain limitations and face a few challenges. Firstly, therapeutic target selection is difficult in certain conditions, for instance in the disordered physiological processes (pathophysiology) of nervous system related disorders. Thus, the integration of large experimental data with machine learning approaches requires the development of new brain-inspired computational algorithms.110 If the molecular mechanisms of the disease are unknown, it is difficult to find the specific receptor and with the function of the protein not determined, it is difficult to make much progress.111 No single medicine is a common solution for all diseased patients; it may vary based on the patient’s symptoms, disease conditions and their history. In such a case, we have to follow a personalized medicinal approach. Based on this approach we need gene expression data for individuals in order to identify the potent drug target and design a drug and in such situations we need a knowledge base. We need to investigate many drug targets based on the drug candidate, which is a trial and error process.112 The use of molecular signatures like peptides in epitope based vaccine design hits a limitation here due to a lack of delivery systems or disease models, rendering it incapable of reaching its set goals in therapeutics.113 The mtk-QSBER model was able to integrate multiple chemical compounds with multiple biological target molecules and develop drug like compounds.93,94,114–116
Emerging diseases, especially viral diseases including cancer related viruses, threaten human life with mortality and morbidity. Emerging viral diseases caused by RNA viruses are increasing due to increasing mutation rates. In clinical virology, emerging techniques, such as microarrays (expression analysis), metagenomic biosynthetic gene cluster identification, host–pathogen interaction analysis, unknown disease-associated viruses, the discovery of novel human viruses and NGS technologies (differential gene expression, de novo sequencing, epigenetics, variant calling, SNPs etc.), are enormously supported.117,118 Using these technologies, it is possible to analyze millions of data simultaneously at a low cost.
Microarrays generate a vast amount of gene expression datasets for healthy and control samples. NGS also produces gigabytes of transcriptome data. Here, the major challenge is storage of this voluminous data. This requires multiprocessors and multicore computers with hundreds of gigabytes of RAM and terabytes of hard drive space in Linux operating systems. Even with the use of internet based supercomputing, the delivery of results is greatly delayed and results become queued up. It is also impossible to submit whole datasets as these have to be divided up to adhere to the accessible memory size and then submitted. In this way, the entire process is greatly affected and becomes time consuming because of the data storage issue.119 Fig. 5 summarizes the advantages and disadvantages of computation in clinical virology.
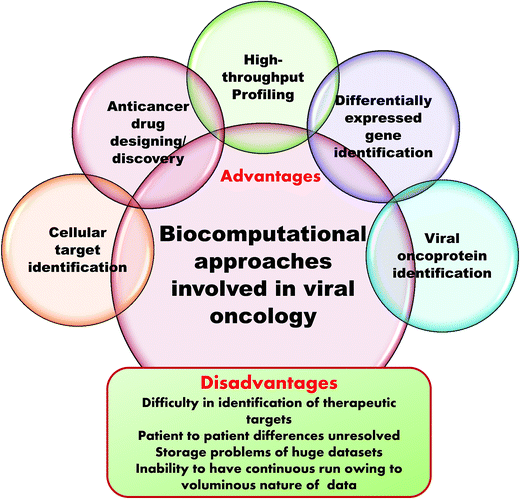 |
| Fig. 5 Advantages and disadvantages of biocomputation in clinical virology. | |
3. Summary
Computer-assisted clinical virology research areas are without doubt very essential, although many aspects of improvement are still in progress. Every year, lots of web-assisted tools, software and algorithms are continuously being developed for various aspects of antiviral drug design, viral immunology and viral oncology. High throughput technologies generate data for thousands of patients as well as their corresponding expression data. Thus, computer-assisted approaches do overcome cumbersome wet lab procedures to a large extent and provide valuable insights into clinical virology and a positive direction for antiviral drug design, epitope-based vaccine design, differentially expressed gene identification and potential drug target identification strategies.
Conflicts of interest
The authors have no conflicts of interest whatsoever.
References
- Emergencies preparedness, response, WHO report 2017,http://www.who.int/csr/don/archive/year/2017/en/.
- B. Jeremy, H. Jonas and M. Timothy, et al., The economics of Ebola, LSE Connect I, summer, 2015, pp. 11–13 Search PubMed.
- WHO Ebola Response Team, Ebola Virus Disease in West Africa — The First 9 Months of the Epidemic and Forward Projections, N. Engl. J. Med., 2014, 371(16), 1481–1495 CrossRef PubMed.
- P. Aparaajita, Economic Cost of Zika Virus ICWA View Point, 2016 Search PubMed.
- W. James L. Duc and N. Nathanson, Emerging Viral Diseases. Why We Need to Worry about Bats, Camels, and Airplanes. Emergence and Control of Viral Infections, 2016 Search PubMed.
- S. M. Lemon, M. A. Hamburg and P. F. Sparling, et al., Global infectious disease surveillance and detection: assessing the challenges—finding solutions, Workshop Summary, Institute of Medicine (US) Forum on Microbial Threats, National Academies Press, US, 2007 Search PubMed.
- C. S. Goldsmith and S. E. Miller, Modern uses of electron microscopy for detection of viruses, Clin. Microbiol. Rev., 2009, 22(4), 552–563 CrossRef PubMed.
- F. C. Robbins, J. F. Enders and T. H. Weller, Cytopathogenic effect of poliomyelitis viruses in vitro on human embryonic tissues, Exp. Biol. Med., 1950, 75(2), 370–374 CrossRef.
- J. Casals and R. Palacios, The complement fixation test in the diagnosis of virus infections of the central nervous system, J. Exp. Med., 1941, 74(5), 409–426 CrossRef PubMed.
- M. Grandien, C. A. Pettersson and L. Svensson, et al., Latex agglutination test for adenovirus diagnosis in diarrheal disease, J. Med. Virol., 1987, 23(4), 311–316 CrossRef PubMed.
- R. Sandeep, K. Rajesh and R. A. Antony, et al., Rapid particle agglutination test for human immunodeficiency virus: hospital-based evaluation, J. Clin. Microbiol., 2002, 40(4), 1553–1554 CrossRef.
- M. Deguchi, N. Yamashita and M. Kagita, et al., Quantitation of Hepatitis B surface antigen by an automated chemiluminescent microparticle immunoassay, J. Virol. Methods, 2004, 15, 217–222 CrossRef.
- N. Shinkai, K. Matsuura and F. Sugauchi, et al., Application of a newly developed high-sensitivity HBsAg chemiluminescent enzyme immunoassay for Hepatitis B patients with HBsAg seroclearance, J. Clin. Microbiol., 2013, 51(11), 3484–3491 CrossRef PubMed.
- K. B. Mullis and F. A. Faloona, Specific synthesis of DNA in vitro via a polymerase-catalysed chain reaction, Methods Enzymol., 1987, 155, 335–350 Search PubMed.
- R. J. Hall, M. Peacey and Q. S. Huang, et al., Rapid method to support diagnosis of swine-origin influenza virus infection by sequencing of real-time PCR amplicons from diagnostic assays, J. Clin. Microbiol., 2009, 47, 3053–3054 CrossRef PubMed.
- J. K. Lewis, M. Bendahmane, T. J. Smith, R. N. Beachy and G. Siuzdak, Identification of viral mutants by mass spectrometry, Proc. Natl. Acad. Sci. U. S. A., 1998, 95(15), 8596–8601 CrossRef.
- G. Siuzdak, Probing viruses with mass spectrometry, J. Mass Spectrom., 1998, 33, 203–211 CrossRef.
- F. Sanger, S. Nicklen and A. R. Coulson, DNA sequencing with chain-terminating inhibitors, Proceedings of the National Academy of Science USA, 1977, 74, 5463–5467 CrossRef.
- S. N. Naccache, S. Federman, N. Veeraraghavan, M. Zaharia, D. Lee, E. Samayoa, J. Bouquet, A. L. Greninger, K. C. Luk, B. Enge, D. A. Wadford, S. L. Messenger, G. L. Genrich, K. Pellegrino, G. Grard, E. Leroy, B. S. Schneider, J. N. Fair, M. A. Martínez, P. Isa, J. A. Crump, J. L. DeRisi, T. Sittler, J. Hackett Jr, S. Miller and C. Chiu, A cloud-compatible bioinformatics pipeline for ultra-rapid pathogen identification from next-generation sequencing of clinical samples, Genome Res., 2014, 24(7), 1180–1192 CrossRef PubMed.
- E. De Clercq, Antivirals and antiviral strategies, Nat. Rev. Microbiol., 2004, 2, 704–720 CrossRef PubMed.
- A. L. Menéndez and F. Gago, Antiviral Agents: Structural Basis of Action and Rational Design, Subcell. Biochem., 2013, 68, 599–630 Search PubMed.
- E. M. Damm and L. Pelkmans, Systems biology of virus entry in mammalian cells, Cell. Microbiol., 2006, 8, 1219–1227 CrossRef PubMed.
- L. T. Lin, W. C. Hsu and C. C. Lin, et al., Antiviral Natural Products and Herbal Medicines, Journal of Traditional and Complementary Medicine, 2014, 4(1), 24–35 CrossRef PubMed.
- A. D. Welch, P. Calabresi and W. H. Prusoff, et al., Initial Clinical Studies with 5-Iodo-2′-deoxyuridine, Experimental Cell Research, 1963, 24, 479 CrossRef.
- B. J. Rodney, A. Danso and B. Yiming, et al., NCBI Viral Genomes Resource, Nucleic Acids Res., 2014, 3, 1, DOI:10.1093/nar/gku1207.
- E. P. Brett, S. Douglas and Y. Z. Greer, et al., Virus Pathogen Database and Analysis Resource (ViPR): A Comprehensive Bioinformatics Database and Analysis Resource for the Coronavirus Research Community, Viruses, 2012, 4, 3209–3226 CrossRef PubMed.
- H. C. U. David, Viral Genome DataBase: storing and analyzing genes and proteins from complete viral genomes, Bioinformatics, 2000, 16(5), 484–485 CrossRef.
- C. Hulo, E. Castro and P. Masson, et al., ViralZone: a knowledge resource to understand virus diversity, Nucleic Acids Res., 2011, 39, D576–D582 CrossRef PubMed.
- Z. Yun and B. D. Aevermann, Influenza Research Database: An integrated bioinformatics resource for influenza virus research, Nucleic Acids Res., 2017, 45, D466–D474 CrossRef PubMed.
- S. Matej, B. Gabor and K. Lubos, et al., viruSITE—integrated database for viral genomics, Database, 2016, baw162 Search PubMed.
- B. Robert, O. Tulio and M. Sidney, et al., The RNA Virus Database, Nucleic Acids Research, 2009, 37(1), D431–D435 Search PubMed.
- O. P. Sharma, A. Jadhav and A. Hussain, et al., VPDB: Viral Protein Structural Database, Bioinformation, 2011, 6(8), 324–326 CrossRef PubMed.
- M. M. Alba, D. Lee and F. M. Pearl, VIDA: a virus database system for the organization of animal virus genome open reading frames, Nucleic Acids Res., 2001, 29(1), 133–136 CrossRef PubMed.
- C. M. Shepherd, I. A. Borelli and G. Lander, et al., VIPERdb: a relational database for structural virology, Nucleic Acids Res., 2006, 34, D386–D389 CrossRef PubMed.
- L. Palmeira, S. Penel and V. Lotteau, et al., PhEVER: a database for the global exploration of virus-host evolutionary relationships, Nucleic Acids Res., 2011, 39, D569–D575 CrossRef PubMed.
- L. Jie, E. Herbert and W. Clare, Anatomy of Protein Pockets and Cavities: Measurement of Binding Site Geometry and Implications for Ligand Design, Protein Sciene, 1998, 7, 1884–1897 CrossRef PubMed.
- PD. BeMotif Tutorial, Protein Data Bank in Europe, http://pdbe.org/motif/.
- A. Golovin and K. Henrick, MSDmotif: exploring protein sites and motifs, BMC Bioinf., 2008, 9, 312 CrossRef PubMed.
- B. Huang, MetaPocket: a meta approach to improve protein ligand binding site prediction., OMICS, 2009, 13(4), 325–330 CrossRef PubMed.
- M. N. Wass and L. A. Kelley, Sternberg MJ. 3DLigandSite: predicting ligand-binding sites using similar structures, Nucleic Acids Res., 2010, 38, W469–W473 CrossRef PubMed.
- J. An, M. Totrov and R. Abagyan, et al., Pocketome via comprehensive identification and classification of ligand binding envelopes, Mol. Cell. Proteomics, 2005, 4, 752–761 Search PubMed.
- Y. Kalidas and N. Chandra, PocketDepth: a new depth based algorithm for identification of ligand binding sites in proteins, J. Struct. Biol., 2008, 161(1), 31–42 CrossRef PubMed.
- K. Theodora, A. Georgios and G. Spyroulias, et al., Computational approaches in target identification and drug discovery, Comput. Struct. Biotechnol. J., 2016, 14, 177–184 CrossRef PubMed.
- J. Yang, R. Yan and A. Roy, The I-TASSER Suite: Protein structure and function prediction, Nat. Methods, 2015, 12, 7–8 CrossRef PubMed.
- E. K. David, C. Dylan and B. David, Protein structure prediction and analysis using the Robetta server, Nucleic Acids Res., 2004, 32, W526–W531 CrossRef PubMed.
- S. Johannes, B. Andreas and N. Andrei, et al., The HHpred interactive server for protein homology detection and structure prediction, Nucleic Acids Res., 2015, 33(1), W244–W248 Search PubMed.
- A. Sali and T. L. Blundell, Comparative protein modelling by satisfaction of spatial restraints, J. Mol. Biol., 1993, 234(3), 779–815 CrossRef PubMed.
- U. Pieper, N. Eswar and A. C. Stuart, et al., MODBASE, a database of annotated comparative protein structure models, Nucleic Acids Res., 2002, 30(1), 255–259 CrossRef PubMed.
- K. Morten, W. Haipeng and W. Sheng, et al., Template-based protein structure modeling using the RaptorX web server, Nat. Protoc., 2012, 7, 1511–1522 CrossRef PubMed.
- M. Biasini, S. Bienert and A. Waterhouse, et al., SWISS-MODEL: modelling protein tertiary and quaternary structure using evolutionary information, Nucleic Acids Res., 2014, 42(W1), W252–W258 CrossRef PubMed.
- H. Reuveni, C. O. Bull and M. L. Landry, et al., Antiviral activity of 5-iodo-2′-deoxyuridine and related drugs in human keratinocytes infected in vitro with herpes simplex virus type 1, Skin Pharmacol., 1991, 4(4), 291–297 CrossRef PubMed.
- K. Vikash, C. Sharat and I. Mohammad, et al., Recent Advances in the Development of Antiviral Agents Using Computer-aided Structure Based Approaches, Curr. Pharm. Des., 2014, 20(21), 3488–3499 CrossRef.
- L. Backert and O. Kohlbacher, Immunoinformatics and epitope prediction in the age of genomic medicine, Genome Med., 2015, 7(119) DOI:10.1186/s13073-015-0245-0.
- A. H. Evan and D. Chaya, et al., Use of Catalyst Pharmacophore Models for Screening of Large Combinatorial Libraries, J. Chem. Inf. Comput. Sci., 2002, 0042(5), 1204–1211 CrossRef.
- H. Bingjie and M. A. Lill, PharmDock: a pharmacophore-based docking program, J. Cheminf., 2014, 6, 14 Search PubMed.
- S. L. Dixon, A. M. Smondyrev and E. H. Knoll, PHASE: a new engine for pharmacophore perception, 3D QSAR model development, and 3D database screening: Methodology and preliminary results, J. Comput.-Aided Mol. Des., 2006, 20(10–11), 647–671 CrossRef PubMed.
- T. S. Rush, J. A. Grant and L. Mosyak, et al., A shape-based 3-D scaffold hopping method and its application to a bacterial proteinprotein interaction, J. Med. Chem., 2005, 48(5), 1489–1495 CrossRef PubMed.
- G. M. Morris, R. Huey and A. J. Olson, Using AutoDock for ligandreceptor docking, Curr. Protoc. Bioinformatics, 2008, 8(14) Search PubMed.
- M. Shingo and D. Irwin, Automated flexible ligand docking method and its application for database search, J. Comput. Chem., 1997, 18(14), 1812–1825 CrossRef.
- R. A. Friesner, J. L. Banks and R. B. Murphy, et al., Glide: a new approach for rapid, accurate docking and scoring Method and assessment of docking accuracy, J. Med. Chem., 2004, 47(7), 1739–1749 CrossRef PubMed.
- G. Jones, P. Willett and R. C. Glen, Molecular recognition of receptor sites using agenetic algorithm with a description of desolvation, J. Mol. Biol., 1995, 245(1), 43–53 CrossRef PubMed.
- S. D. Dina, I. R. Yuval and J. Haim, et al., PatchDock and SymmDock: servers for rigid and symmetric docking, Nucleic Acids Res., 2005, 33(2), W363–W367 Search PubMed.
- R. Tyler and S. P. S. Mark, Computational virology: From the inside out, Biochim. Biophys. Acta, 2016, 7, 1610–1618 Search PubMed.
- B. R. Brooks, C. L. Brooks and A. D. Mackerell, et al., CHARMM: The biomolecular simulationprogram, J. Comput. Chem., 2009, 30(10), 1545–1614 CrossRef PubMed.
- J. B. Kevin and C. Edmond, Scalable Algorithms for Molecular Dynamics Simulations on Commodity Clusters (PDF), Proceedings of the ACM/IEEE Conference on Supercomputing (SC06), Tampa, Florida, November 11–17, 2006 Search PubMed.
- N. Schmid, A. Eichenberger and A. Choutko, et al. Definition and testing of the GROMOS force-field, Eur. Biophys. J., 2011, 40(7), 843–856 CrossRef PubMed.
- L. Backert and O. Kohlbacher, Immunoinformatics and epitope prediction in the age of genomic medicine, Genome Med., 2015, 7, 119, DOI:10.1186/s13073-015-0245-0.
- N. Rapin, O. Lund and M. Bernaschi, et al. Computational Immunology Meets Bioinformatics: The Use of Prediction Tools for Molecular Binding in the Simulation of the Immune System, PLoS One, 2010, 5(4), e9862 Search PubMed.
- M. N. Davies and D. R. Flower, Harnessing bioinformatics to discover new vaccines, Drug Discovery Today, 2007, 12(9–10), 389–395 CrossRef PubMed.
- L. Pasteur, De l’attenuation du virus du Choléra des poules, C. R. Seances Acad. Sci., 1880, 91, 673–680 Search PubMed.
- M. M. Levine, R. Lagos and J. Esparza, Vaccines and Vaccination in Historical Perspective, New Generation Vaccines, Informa Healthcare Inc., New York, USA, 4th edn, 2009, pp. 1–11 Search PubMed.
- P. A. Offit. Vaccinated: One Man’s Quest to Defeat the World’s Deadliest Diseases, HarperCollins, New York, 2007 Search PubMed.
- E. B. Buynak, R. R. Roehm and A. A. Tytell, et al., Vaccine Against Human Hepatitis B, JAMA, J. Am. Med. Assoc., 1996, 276(22), 1793–1795 CrossRef PubMed.
- M. M. Giuliani, J. Adu-Bobie and M. Comanducci, et al., A universal vaccine for serogroup B meningococcus, Proc. Natl. Acad. Sci., 2006, 103(29), 10834–10839 CrossRef PubMed.
- T. Reza and F. Fatemeh, A new strategy for development of hepatitis E vaccine: Epitope-based vaccines, Pathog. Infect. Dis., 2015, 1, e933, DOI:10.14800/pid.933.
- A. Alam, S. Ali and S. Ahamad, From ZikV genome to vaccine: in silico approach for the epitope-based peptide vaccine against Zika virus envelope glycoprotein, Immunology, 2016, 149(4), 386–399, DOI:10.1111/imm.12656.
- R. Dash, R. Das and M. Junaid, In silico-based vaccine design against Ebola virus glycoprotein, Adv. Appl. Bioinf. Chem., 2017, 21(10), 11–28, DOI:10.2147/AABC.S115859.
- K. Thomas, J. Goldsby and J. Kuby et al., Kuby Immunology, WH Freeman and Co, New York, 6th edn, 2006 Search PubMed.
- M. Bhasin and G. P. Raghava, Analysis and prediction of affinity of TAP binding peptides using cascade SVM, Protein Sci., 2004, 13(3), 596–607 CrossRef PubMed.
- J. E. P. Larsen, O. Lund and M. Nielsen, Improved method for predicting linear B-cell epitopes, Immunome Res., 2006, 2(2) DOI:10.1186/1745-7580-2-2.
- S. Saha and G. P. Raghava, Prediction of continuous B-cell epitopes in an antigen using recurrent neural network, Proteins, 2006, 65(1), 40–48 CrossRef PubMed.
- J. Greenbaum, The Immune Epitope Database and Analysis Resource (IEDB), EMBRACE Bioinformatics of Immunology Workshop, La Jolla Institute for Allergy and Immunology, Lyngby, Denmark, January 24, 2007 Search PubMed.
- A. S. Kolaskar and P. C. Tongaonkar, A semi-empirical method for prediction of antigenic determinants on protein antigens, FEBS Lett., 1990 Dec 10, 276(1–2), 172–174 Search PubMed.
- Cancer facts and figures 2017, American Cancer society, https://www.cancer.org/content/dam/cancer-org/research/cancer-facts-and-statistics/annual-cancer-facts-and-figures/2017/cancer-facts-and-figures-2017.pdf.
- I. H. Frazer, D. R. Lowy and J. T. Schiller, Prevention of cancer through immunization: Prospects and challenges for the 21st century, Eur. J. Immunol., 2007, 37(1), S148–S155 CrossRef PubMed.
- A. W. C. Daskalaki and R. P. T. Herwig, Computational tools and resources for systems biology approaches to cancer, Comput. Biol., 2009, 227–242 Search PubMed.
- D. Janusz, K. Michael and A. Michal, A gene ontology inferred from molecular networks, Nat. Biotechnol., 2013, 31(1), 37–48 CrossRef.
- C. Nicholas and P. Y. Arthur, High-throughput profiling of influenza A virus hemagglutinin gene at single-nucleotide resolution, Sci. Rep., 2015, 4, 4942 CrossRef PubMed.
- D. V. Nguyen and D. M. Rocke, On partial least squares dimension reduction for microarray based classification, Comput. Stat. Data Anal., 2004, 46, 407–425 CrossRef.
- Y. Chen, D. Nguyen and T. Pham, Identification of relevant genes from microarray experiments based on partial least squares weights: application to cancer genomics, Computational Biology: Issues and Applications in Oncology, New York, Springer, 2009, pp. 1–18 Search PubMed.
- A. V. Denisenko, T. Druzhenko and Y. Skalenko, Photochemical synthesis of 3 azabicyclo[3.2.0] heptanes: advanced building blocks for drug discovery, J. Org. Chem., 2017, 82(18), 9627–9636 CrossRef PubMed.
- B. Chalyk, M. Butko and O. Yanshyna, Synthesis of spirocyclic pyrrolidines: advanced building blocks for drug discovery, Chem.–Eur. J, 2017, 23(66), 16782–16786 CrossRef PubMed.
- D. M. Herrera-Ibatá, A. Pazos and R. A. Orbegozo-Medina, et al. Mapping chemical structure-activity information of HAART-drug cocktails over complex networks of AIDS epidemiology and socioeconomic data of U.S. counties, Biosystems, 2015, 132–133, 20–34 CrossRef PubMed.
- A. Speck-Planche, Dias Soeiro Cordeiro MN. Speeding up Early Drug Discovery in Antiviral Research: A Fragment-Based in Silico Approach for the Design of Virtual Anti-Hepatitis C Leads, ACS Comb. Sci., 2017, 19(8), 501–512 CrossRef PubMed.
- V. V. Kleandrova and A. Speck Planche, Multitasking model for computer-aided design and virtual screening of compounds with high anti-HIV activity and desirable ADMET properties, in. Multi-Scale Approaches in Drug Discovery: From Empirical Knowledge to In Silico Experiments and Back, ed. A. Speck-Planche, Elsevier, Oxford, UK, 2017, pp. 55–81 Search PubMed.
- H. González-Díaz, D. M. Herrera-Ibatá and A. Duardo-Sánchez, et al. ANN multiscale model of anti-HIV drugs activity vs. AIDS prevalence in the US at county level based on information indices of molecular graphs and social networks, J. Chem. Inf. Model., 2014, 54(3), 744–755 CrossRef PubMed.
- F. J. Prado-Prado, O. Martinez de la Vega and E. Uriarte, et al. Unified QSAR approach to antimicrobials. 4. Multi-target QSAR modeling and comparative multi-distance study of the giant components of antiviral drug-drug complex networks, Bioorg. Med. Chem., 2009, 17(2), 569–575 CrossRef PubMed.
- H. González-Díaz, F. Prado-Prado and F. M. Ubeira, Predicting antimicrobial drugs and targets with the MARCH-INSIDE approach, Curr. Top. Med. Chem., 2008, 8(18), 1676–1690 CrossRef.
- H. González-Díaz and E. Uriarte, Biopolymer stochastic moments. I. Modeling human rhinovirus cellular recognition with protein surface electrostatic moments, Biopolymers, 2005, 77(5), 296–303 CrossRef PubMed.
- R. Ramos de Armas, H. González Díaz and R. Molina, et al. Markovian Backbone Negentropies: Molecular descriptors for protein research. I. Predicting protein stability in Arc repressor mutants, Proteins, 2004, 56(4), 715–723 CrossRef PubMed.
- Department of health drug office drug registration and import/export control division guidance notes on registration of pharmaceutical products/substances, 2017, 1–12.
- B. Dominique, C. Joseph and L. Bruno, Computational oncology — mathematical modelling of drug regimens for precision medicine, Nat. Rev. Clin. Oncol., 2016, 13, 242–254 CrossRef PubMed.
- L. Simon, A. Imane, K. K. Srinivasan, L. Pathak and I. Daoud, In Silico Drug-Designing Studies on Flavanoids as Anticolon Cancer Agents: Pharmacophore Mapping, Molecular Docking, and Monte Carlo Method-Based QSAR Modeling, Interdiscip. Sci.: Comput. Life Sci., 2017, 9(3), 445–458 CrossRef PubMed.
- T. Atul, T. Abhishek and A. Priya, CancerPPD: a database of anticancer peptides and proteins, Nucleic Acids Res., 2015, 43, D837–D843 CrossRef PubMed.
- R. Kumar, et al. CancerDR: Cancer Drug Resistance Database, Sci. Rep., 2013, 1445 Search PubMed.
- T. Weiyang, L. Bohui and G. Shuo, et al. CancerHSP: anticancer herbs database of systems pharmacology, Sci. Rep., 2015, 5, 11481 CrossRef PubMed.
- V. Umashankar, S. Nandhitha and P. Kalabharath, InPACdb – Indian plant anticancer compounds database, Bioinformation, 2009, 4(2), 71–74 CrossRef.
- J Gollub, C. A. Ball, G. Binkley, J. Demeter, D. B. Finkelstein, J. M. Hebert, T. Hernandez-Boussard, H. Jin, M. Kaloper, J. C. Matese, M. Schroeder, P. O. Brown, D. Botstein and G. Sherlock, The Stanford Microarray Database: data access and quality assessment toolsOxford Journals Life Sciences, Nucleic Acids Res., 2003, 31(1), 94–96 CrossRef PubMed.
- Y. Bernard, C. Konstantina and R. Sophie, Statistical Analysis of GEO Datasets, Gene Technol., 2014, 4, 1 Search PubMed.
- F. J. Romero-Duran, N. Alonso, M. Yanez, O. Caamano, X. Garcia-Mera and H. Gonzalez-Diaz, Brain-inspired cheminformatics of drug-target brain interactome, synthesis, and assay of TVP1022 derivatives, Neuropharmacology, 2016, 103, 270–278 CrossRef PubMed.
- Forum on Neuroscience and Nervous System Disorders, Board on Health Sciences Policy; Institute of Medicine, Improving and Accelerating Therapeutic Development for Nervous System Disorders: Workshop Summary, National Academies Press (US), Washington (DC), 2014, Drug Development Challenges Search PubMed.
- Personalized Medicine 101: The Challenges, Personalized Medicine Coalition, Retrieved April 26, 2014 Search PubMed.
- H. E. K. Stefan, M. E. MJuliana and J. M. L. David, Challenges and responses in human vaccine development, Curr. Opin. Immunol., 2014, 28, 18–26 CrossRef PubMed.
- A. Speck-Planche and M. N. D. S. Cordeiro, De novo computational design of compounds virtually displaying potent antibacterial activity and desirable in vitro ADMET profiles, Med. Chem. Res., 2017, 26(10), 2345–2356 CrossRef.
- V. V. Kleandrova, J. M. Ruso, A. Speck-Planche and M. N. Dias Soeiro Cordeiro, Enabling the Discovery and Virtual Screening of Potent and Safe Antimicrobial Peptides. Simultaneous Prediction of Antibacterial Activity and Cytotoxicity, ACS Comb. Sci., 2016, 18(8), 490–498 CrossRef PubMed.
- A. Speck-Planche and M. N. D. S. Cordeiro, Simultaneous virtual prediction of anti-Escherichia coli activities and ADMET profiles: A chemoinformatic complementary approach for high-throughput screening, ACS Comb. Sci., 2014, 16(2), 78–84 CrossRef PubMed.
- S. T. Park and J. Kim, Trends in Next-Generation Sequencing and a New Era for Whole Genome Sequencing, Int. Neurourol. J., 2016, 20, 76–83 CrossRef PubMed.
- L. Barzon, E. Lavezzo, V. Militello, S. Toppo and G. Palù, Applications of next-generation sequencing technologies to diagnostic virology, Int. J. Mol. Sci., 2011, 12(11), 7861–7884 CrossRef PubMed.
- S. Tony, Immunology of Bats and Their Viruses: Challenges and Opportunities, Viruses, 2014, 6, 4880–4901 CrossRef PubMed.
|
This journal is © The Royal Society of Chemistry 2018 |
Click here to see how this site uses Cookies. View our privacy policy here.