DOI:
10.1039/C7RA12266G
(Paper)
RSC Adv., 2018,
8, 222-229
Recognition of osmotolerant yeast spoilage in kiwi juices by near-infrared spectroscopy coupled with chemometrics and wavelength selection
Received
9th November 2017
, Accepted 13th December 2017
First published on 2nd January 2018
Abstract
The recognition of food spoilage by osmotolerant yeast is important in food safety surveillance. In the study, Fourier transform near-infrared spectroscopy was employed for acquisition of five osmotolerant yeast (Hanseniaspora uvarum, Candida tropicalis, Candida intermedia, Meyerozyma guilliermondii and Saccharomyces cerevisiae) contaminated kiwi juice spectra. Support Vector Machine (SVM) recognition models were built with Direct Orthogonal Signal Correction processed full range wavelength spectra or Competitive Adaptive Reweighted Sampling (CARS) selected wavelength spectra. Grid search, Particle Swarm Optimization and Genetic Algorithm were employed for SVM parameter optimization to improve model performance. The overall correct rate was 100% for single strain recognition and 98.8% for yeast cocktail recognition both by employing the full and CARS selected wavelengths. Analysis on CARS selected wavelengths inferred that amide bond containing compounds (5068, 5064, 5207, 5076 and 5072 cm−1) and water (5277 and 5412 cm−1) may be relevant in discriminating kiwi juice spoiled by different yeasts. The method provides a fast, simple and high-throughput method for yeast spoilage recognition, which can be applied in monitoring osmotolerant yeast spoilage.
Introduction
Osmotolerant yeast is a collection of yeast species that can tolerate high osmotic stresses, such as the tolerance to 50–65% (w/v) sugar concentration in sugary food. These yeasts are frequently recovered from high sugar foods, such as fruit concentrates, confectionery, juices and honey.1–3 One of the most notorious osmotolerant yeasts Zygosacchromyces rouxii (Z. rouxii) is repeatedly isolated from apple juice and grape juice concentrates4,5 and is an extremely osmotolerant yeast which can tolerate up to 90% w/v glucose concentration. However, we have reported that moderate osmotolerant yeasts, Hanseniaspora uvarum, Candida tropicalis, Saccharomyces cerevisiae, Meyerozyma guilliermondii and Candida intermedia etc., were capable of contaminating kiwi fruit concentrate.6 These yeasts can lead to blown packages, off-odor and off-flavor of food and potential economic loss to food manufacturers. The detection and classification of osmotolerant yeasts is important in food spoilage surveillance. Although detection of some yeasts by real-time PCR methods has been proposed,7 there is still an urgent need to build a high-throughput and easily operated method such as Near Infrared Spectroscopy based methods to this end.
Near infrared spectroscopy (NIRS) in the wavelength range of 780–2500 nm is a high throughput, cost-effective and a rapid analytical method, the feasibility of which has been verified with various food related classification and quantification applications such as discrimination of Chinese liquors8 and quantification of cholesterol in dairy powders.9 In the field of microbiology, NIR in conjugation with Partial Least Squares-Discriminate Analysis achieved 99.5% correct classification of Salmonella enterica and 100% correct classification of Bacillus subtilis, Escherichia coli, Pseudomonas fluorescens in sterile saline solutions.10 Similar successful classification of E. coli and Listeria on the species and strain levels in phosphate buffered saline was also provided.11 However, the recognition of spoilage osmotolerant yeasts in food matrix by NIRS has not yet been reported.
Among the many multivariate algorithms, Support Vector Machine (SVM) is a popular multivariate classifier that achieves classification by searching the maximum margin between classes in the higher dimensional feature space generated by mapping input vectors.12 With its superior performance, SVM has been coupled with VIS/NIR spectroscopy for measurement of soluble solid contents (SSC) and pH of White Vinegar,13 classification of swill cooked oil with terahertz spectroscopy14 and detection of pork adulteration in veal product in conjugation with principal component analysis.15
Due to the multivariate nature of NIR spectra, the effort in reducing collinearity and improve model interpretation has been put. Competitive Adaptive Reweighted Sampling (CARS) is a wavelength selection method based on ‘survival of the fittest’ from Darwin's Evolution Theory aiming at reducing redundant information in the full wavelengths models.16 Compared with other existent wavelength selection algorithms, CARS is effective, simple and can obtain key wavelengths that are meaningful in chemistry, therefore it is contributive to data interpretation. CARS involve several procedures: (1) Monte Carlo sampling; (2) computation of kept variables; (3) exponentially decreasing function for enforced removal of wavelengths with lower regression coefficients; (4) competitive wavelength reduction by adaptively reweighted sampling; (5) root mean squared error calculation for each sampling subset. CARS can be used in not only PLS modelling as well as other types of pattern recognition methods such as SVM.
The objective of this study was to recognize kiwi juice contamination by osmotolerant yeasts and detect yeast strains that are responsible for that spoilage. The aim was completed by (1) recognize a single yeast strain or yeast cocktails incurred kiwi juice spoilage and determine the responsible yeast; (2) find key variables in the NIRS models by CARS and offer possible chemical explanations. To our knowledge, this is the first attempt on recognition of osmotolerant yeasts relevant to kiwi juice spoilage by NIRS.
Results and discussion
NIR spectra overview
Fig. 1 showed the acquired raw spectra for the tested yeasts (Table 1). The kiwi juice NIR absorbance spectra presented an identical profile with those for pure water and sugar solutions.17 It was worth mentioning that spectra of the used two varieties of kiwi juices shared the same profile. All the kiwi juice spectra with or without yeast spoilage had similar prominent peaks at 4559–5381 cm−1 and 6233–7228 cm−1, corresponding to NIR combinations, first overtone and second overtone regions. The two main peaks were attributed to O–H combinations stretching and first overtone region of O–H. Besides, band at 4559–5381 cm−1 is believed to include CH3 group C–H stretch and C–H deformation combination stretching of ethanol (2270 nm) as well as CH2 group stretching at 2300 nm. The range of 6233–7228 cm−1 cover the deformation of O–H group in ethanol.18,19 Overall, sample spectra overlapped to a great extent, and the profile resemblance largely impeded the discrimination of kiwi juices with regard to yeasts spoilage.
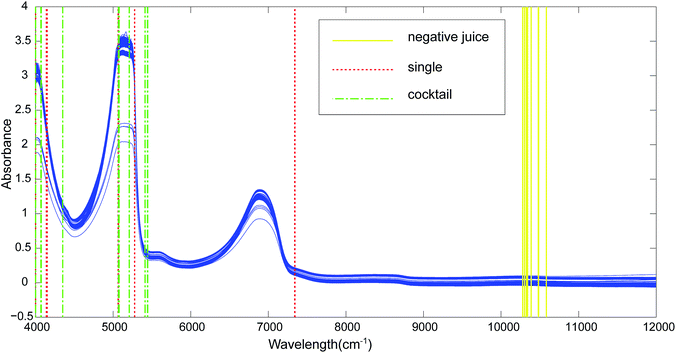 |
| Fig. 1 Raw NIR spectra of kiwi juice samples. The selected NIR variables for kiwi juice variety discrimination (yellow solid line), single strain recognition (red dotted line) and yeast cocktail recognition (green dash-dotted line) are marked with vertical lines. | |
Table 1 Strains used in the study
Species |
Strain number |
Origin |
Hanseniaspora uvarum |
B-NC-12-F10 |
Quick frozen kiwifruit |
Hanseniaspora uvarum |
B-NC-12-F13 |
Water after cleaning kiwi fruit |
Candida tropicalis |
B-NC-12-F09 |
Kiwi fruit after cleaning |
Candida tropicalis |
B-NC-12-F17 |
Air in the peeling section |
Saccharomyces cerevisiae |
B-NC-12-OZ03 |
Fallen kiwi fruit |
Saccharomyces cerevisiae |
B-NC-12-OZ18 |
Kiwi fruit tree leaves |
Meyerozyma guilliermondii |
B-NC-12-OZ01 |
Kiwi fruit |
Meyerozyma guilliermondii |
B-NC-12-OM16 |
Kiwi fruit |
Candida intermedia |
B-NC-12-F16 |
Air in the peeling section |
Candida intermedia |
B-NC-12-OM10 |
Kiwi fruit |
Principal component analysis
Principal component analysis (PCA) was conducted on the single strain and yeast cocktail sets for an overview of the spectra and detection of outliers. For single strain spectra set, the first two principal components cumulatively accounted for 98.3% variance (PC-1 for 87.0% and PC-2 for 11.3% variance). For the yeast cocktail spectra set, principal component analysis revealed that the first two principal components cumulatively accounted for 98.6% of the total variance, with PC-1 accounted for 87.6% and PC-2 accounted for 11.0%. One and three outliers were detected in the two sets since these samples exceeded the residual limit and Hotelling's T2 limit at 5% significant level and were thus removed. After the removal of outliers, the contribution of the first two PCs slightly increased to 98.9% and 98.7% for the two sets, respectively. The major improvement in contributed variance was from PC-1, whose contribution experienced an increase from 87.0% to 92.3% for the single strain sample set and from 87.6% to 93.2% for the yeast cocktail set, respectively. Score plots (Fig. 2(a and b)) of the two sample sets showed four obvious clusters. The partition of Qinmei and Hayward kiwi juice suggested a component difference in the two kiwi fruit cultivars. Spectra samples from 24 h or 72 h yeast spoilage were not well resolved given the close vicinity of Hayward-24 h and Hayward-72 h. Further, yeast negative kiwi juice (kiwi juice without yeast inoculation) samples were distinguished from spoilage samples in single strain set and yeast cocktail set (discrete points not in circles). One exception was the negative Hayward juice samples that were grouped into Hayward-72 h cluster (Fig. 2a). Simultaneously, when the sample spectra spoiled by different yeast strains or yeast cocktails were examined, they were not clustered according to the identities, it was suggested that pre-processing treatment was necessary so as to achieve recognition of responsible yeast species.
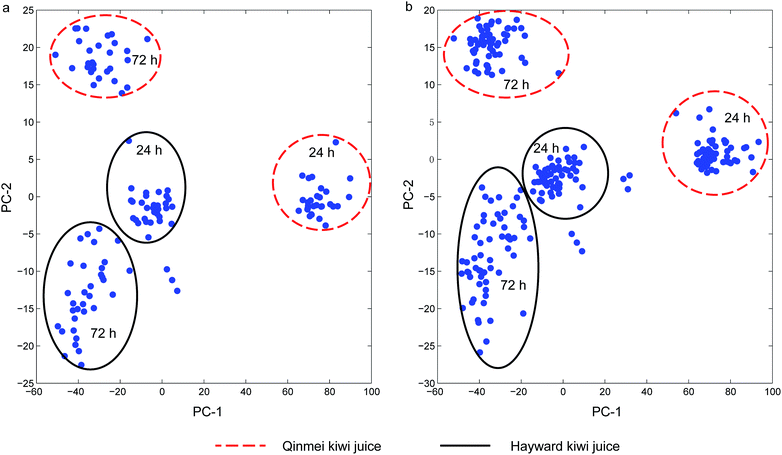 |
| Fig. 2 Principal component analysis score plot: (a) single strain sample set; (b) cocktail sample set. | |
Single yeast strain recognition
Direct orthogonal signal correction was applied to both sets of spectra to expose and enlarge important wavelength ranges so as to achieve recognition of tested yeast strains and cocktails in SVM models. Models were established using the full wavelength NIR spectra and CARS selected NIR wavelengths and the performance was compared. In addition, parameter optimization aimed at searching for a pair of C and γ parameters was conducted to enhance model performance. The full wavelength spectra (contain 2074 variables) model with a total of 84 support vectors only achieved an overall correct rate of 26.4% (Table 2). Only the strain S. cerevisiae OZ18 (Fig. 3a, x = 10) was correctly recognized with a sensitivity and PPV (positive predictive value) equalled to 1.00. The other S. cerevisiae strain OZ03 (Fig. 3a, x = 9) was classified with sensitivity of 0.50 (Fig. 3a) and PPV of 0.67 (Fig. 3b). Although C. tropicalis F17 (Fig. 3a, x = 2) was correctly classified and the sensitivity was 1.00, there were additional spectra that were misclassified as this strain thus the PPV was only 0.20 (Fig. 3b). The model failed to recognize the other C. tropicalis strain F09 (x = 1), whose sensitivity and PPV were both 0. Except for the two correctly classified M. guilliermondii OZ01 (x = 7) spectra, the overall correct rate of the species H. uvarum (x = 3 and 4), C. intermedia (x = 5 and 6) and the strain M. guilliermondii OM16 (x = 8) were 0. However, the performance of full wavelength SVM model was greatly enhanced by using the optimized parameter C and γ. Although the three parameter optimization methods, grid search (GS), particle swarm optimization (PSO) and genetic algorithm (GA) produced different optimized C and γ values, all optimized models achieved correct rate (CR) of 100.0%. Accordingly, the sensitivity and PPV for all tested strains (x = 1 to 10) were 1.00. The negative juice samples were also correctly discriminated from others, which was indicative of a clear separation of yeast negative samples from yeast spoilage samples. The variable selection method CARS reduced the wavelength variables from 2074 to 8. With only 8 selected wavelengths, SVM model produced an overall correct rate of 60.8% without using optimized C and γ. Albeit not accurate enough to be useful, the CARS selected 8-variable based model outperformed the 2074-variable full wavelength model (CR = 26.4%). With this simplified model, the sensitivity of the species C. intermedia (Fig. 3a x = 5, 6), M. guilliermondii OZ01 (x = 7) and S. cerevisiae OZ03 (x = 9) reached 1.00, and the PPV for these strains were increased to 1.00, 0.60, and 0.86, respectively (Fig. 3b). It was thus denoted that variables carrying important information were extracted from the numerous NIR variables. When the CARS simplified model was subjected to parameter optimization with GS, PSO and GA methods, the overall correct rate were again increased to 100%. The sensitivity and PPV for all strains were also enhanced to 1.00. The total number of support vectors was decreased from 69–77 for the full spectra models to 42–67 for the optimized models. With only 8 variables, the model was still capable of correctly recognize the strain or species responsible for the kiwi juice spoilage, in spite of the spoilage time (24 h or 72 h) and kiwi juice varieties (Qinmei or Hayward).
Table 2 Model performance for single strain recognition and yeast cocktail recognition
Model |
Single strain |
Cocktail |
nSV |
C |
γ |
CR |
nSV |
C |
γ |
CR |
Full spectra |
84 |
1.00 |
0.00 |
26.4% |
161 |
1.00 |
0.00 |
30.0% |
Full spectra-GS |
77 |
1.00 |
0.09 |
100.0% |
128 |
724.1 |
0.00 |
98.8% |
Full spectra-PSO |
76 |
5.35 |
0.01 |
100.0% |
155 |
27.7 |
0.01 |
98.4% |
Full spectra-GA |
69 |
3.31 |
0.07 |
100.0% |
160 |
86.6 |
0.00 |
98.8% |
CARS |
84 |
1.00 |
0.13 |
60.8% |
161 |
1.00 |
0.09 |
67.9% |
CARS-GS |
67 |
0.35 |
32.0 |
100.0% |
115 |
1.00 |
128.0 |
98.8% |
CARS-PSO |
61 |
3.98 |
2.05 |
100.0% |
115 |
1.14 |
117.3 |
98.8% |
CARS-GA |
42 |
12.4 |
2.62 |
100.0% |
113 |
1.38 |
111.9 |
98.8% |
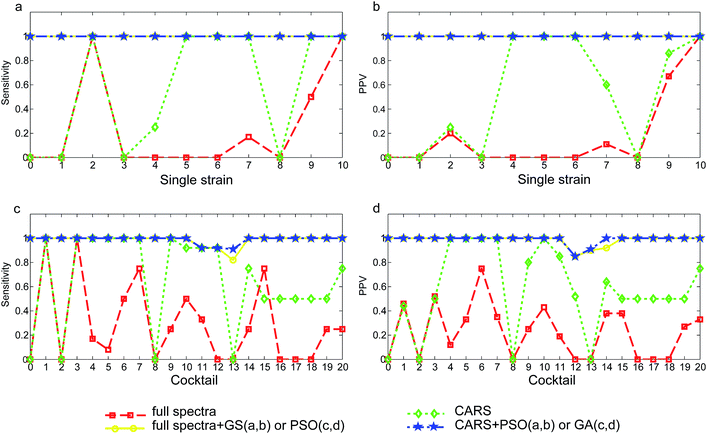 |
| Fig. 3 Sensitivity and PPV of developed SVM models for single strain (a, b) and yeast cocktail set (c, d). In single strain set, 0: negative kiwi juice, 1: C. tropicalis-F09, 2: C. tropicalis-F17, 3: H. uvarum-F10, 4: H. uvarum-F13, 5: C. intermediaF16, 6: C. intermedia-OM10, 7: M. guilliermondii-OZ01, 8: M. guilliermondii-OM16, 9: S. cerevisiae-OZ03, 10: S. cerevisiae-OZ18. In yeast cocktail set, 1: H. uvarum + S. cerevisiae, 2: H. uvarum + C. tropicalis, 3: H. uvarum + C. intermedia, 4: H. uvarum + M. guilliermondii, 5: C. tropicalis + S. cerevisiae, 6: C. intermedia + S. cerevisiae,7: M. guililermondii + S. cerevisiae, 8:C. tropicalis + M. guilliermondii, 9:C. tropicalis + C. intermedia,10:C. intermedia + M. guilliermondii, 11:C. tropicalis + H. uvarum + C. intermedia, 12: C. tropicalis + H. uvarum + M. guilliermondii, 13: H. uvarum + C. intermedia + M. guilliermondii, 14:C. tropicalis + C. intermedia + S. cerevisiae, 15:C. tropicalis + M. guilliermondii + S. cerevisiae, 16:C. intermedia + M. guilliermondii + S. cerevisiae, 17: C. tropicalis + C. intermedia + M. guilliermondii, 18: C. tropicalis + H. uvarum + S. cerevisiae, 19: H. uvarum + C. intermedia + S. cerevisiae, 20: H. uvarum + M. guilliermondii + S. cerevisiae. | |
Yeast cocktail recognition
Similar to the single strain model, full spectra yeast cocktail model gave the poorest performance since only 73 out of 243 juice samples were accurately recognized (CR = 30.0%), as shown in Table 2. Correctly classified yeast cocktails were H. uvarum + S. cerevisiae (Fig. 3c, x = 1) and H. uvarum + C. intermedia (Fig. 3c, x = 3) (sensitivity = 1.00), but the corresponding PPV were only 0.46 and 0.52, suggesting many samples were misidentified as the two yeast cocktails. Identical to single strain model, parameter optimization greatly enhanced model performance. With the optimized C and γ, SVM models not only had higher correct rates but also offering high sensitivity and PPV for each yeast cocktail. The correct rate for full spectra-GS model and full spectra-GA model were 98.8%. The poorly classified yeast cocktails of C. tropicalis + H. uvarum + C. intermedia (Fig. 3c, x = 11), C. tropicalis + H. uvarum + M. guilliermondii (x = 12) and H. uvarum + C. intermedia + M. guilliermondii (x = 13) had sensitivity of 0.92, 0.92 and 0.91 and PPV of 1.00, 0.85 and 0.91. Three spectra were mistakenly identified: one Hayward juice sample harvested at 24 h and spoiled by C. tropicalis + H. uvarum + C. intermedia (x = 11) cocktail was mistakenly predicted as C. tropicalis + H. uvarum + M. guilliermondii (x = 12) cocktail. Two Qinmei juice samples harvested at 72 h and spoiled by C. tropicalis + H. uvarum + M. guilliermondii (x = 12) and H. uvarum + C. intermedia + M. guilliermondii (x = 13) cocktails were confused with each other.
The application of CARS was also effective for the yeast cocktail spectra set. The primitive 2074 variables were decreased to 11 and the CR in the non-optimized model was increased from 30.0% to 67.9%. For example, the H. uvarum + M. guilliermondii (Fig. 3c and d, x = 4) cocktail previously had a sensitivity of 0.17 and PPV of 0.12 in the full wavelength model, it was more accurately classified by the CARS-model offering increased sensitivity and PPV of 1.00 and 1.00, respectively.
On the whole, the performance of parameter optimized CARS models (CARS-GS/PSO/GA) was as good as full wavelength model. No deterioration of model performance was observed. The result was slightly different from prior research showing that model accuracy can be enhanced by CARS. The high accuracies that single and yeast cocktail model obtained were outstanding as the spectra samples comprising each category were of dual kiwi juice sources and spoilage time, as indicated in PCA score plot. The CARS-(GS/PSO/GA) models correctly recognized single strains or yeast cocktails spoilage regardless of the kiwi juice component discrepancy and spoilage time, which is an indication of the robustness of the developed model. The successful recognition of yeast negative samples (x = 0) in single strain or yeast cocktail spectra set proved that NIRS can achieve discrimination of yeast spoilage kiwi juice samples from non-spoilage ones. In addition, the model could recognize yeast contaminator with high accuracies, no matter the spoiler was single yeast or were multiple yeasts.
Contributive compound analysis
The NIR technique is a black-box method and only chemical bonds can be assigned and the correct correspondence to exact chemical compounds has not been achieved so far. The employment of CARS reduced variables substantially, offering a possibility to narrow the range of the responsible chemical bonding. In an attempt to discriminate the employed two varieties of kiwi juice (yeast negative samples), CARS found 8 wavelengths: 10
584, 10
484, 10
480, 10
387, 10
341, 10
326, 10
302, 10
279 cm−1 (Fig. 1, negative juice-soild line). The wavelength 10
584, 10
484, 10
480 cm−1 correspond to the third overtone of phenolic compounds. Thus the difference in phenol type and quantity of Qinmei and Hayward cultivar was probably contributive to the classification. The finding is meaningful as it was reported that the content of quercetin-3-O-glucoside, ferulic acid glucoside, dimethyl caffeic acid hexoside, coumaric acid derivative, 2,4-dihydroxybenzoic acid, (+)-catechin and procyanidin B2 were different in the two cultivars.20 Selected wavelengths of 10
387, 10
341, 10
326, 10
302, 10
279 cm−1 corresponded to the third overtone of H2O, which is the main component of kiwi juice.21 The difference in juice water may be caused by the prism effect of water22 on the solubilized kiwi fruit constituents and yeast metabolites.
By using CARS for selecting important variables, 8 wavelengths including 7344, 7340, 5277, 5068, 5064, 4150, 4139 and 4000 cm−1 (Fig. 1, single-dotted line) were selected for single strain model and 11 wavelengths including 5446, 5442, 5412, 5207, 5076, 5072, 5068, 4351, 4073, 4069 and 4000 cm−1 (Fig. 1, cocktail-dash dotted line) were obtained. In both CARS selections (Fig. 4a and d), the number of kept variable reduced fast firstly and then gradually levelled off. As sampling runs increased, RMSECV (root mean square error in cross validation) remained fundamentally unchanged until the elimination of some variables (Fig. 4b and e). It can be seen in regression coefficients path plots that when the coefficient of key variable drop to 0 (Fig. 4c and f), the RMSECV increase tremendously (Fig. 4b and e). The common chemical bonding in both models were the first overtone of H2O (5277 and 5412 cm−1), first overtone of COHN2 (5068, 5064, 5207, 5076 and 5072 cm−1), combinations of CH, CH2 and CH3 (4150, 4139 and 4351 cm−1). The wavelength range of 7340–7344 cm−1 representing second overtone of CH3 and first overtone of RCO2H at 5277 cm−1 were unique for single strain model.
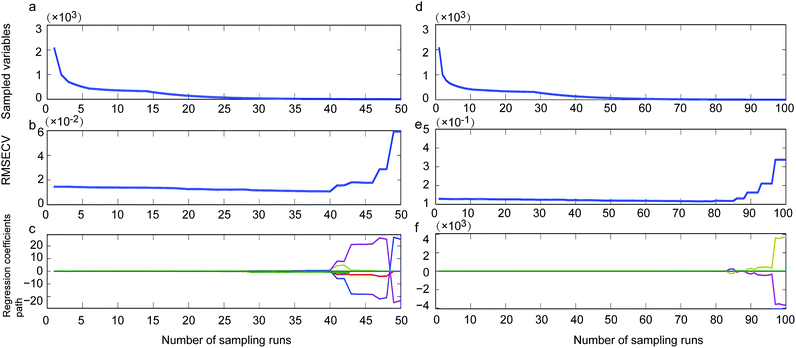 |
| Fig. 4 Changing trend of the sampled wavelength variables (a and d), root mean squared error of cross validation (RMSECV) (b and e) and the regression coefficient path of each variable (c and f) for the single strain recognition model (a–c) and yeast cocktail recognition model (d–f). | |
Consistent with juice variety discrimination, water bands played a role in both single strain and yeast cocktail model development. The difference was that in the single strain and yeast cocktail models, yeast metabolites as well as juice components both could alter the water structure and brought about water vibrational change.
As the first overtone of amido bond (COHN2) occupied certain seats of the selected variables, it was an important wavelength contributive to the performance of both models. The natural origin of amido bond might be from kiwi fruit proteinaceous compounds such as glutamine, asparagine, aspartic acid, arginine and glutamic acid.23 Additionally, amido bond can be synthesized from the reaction between carbonyl containing substances and amino compounds. Previous study suggested that H. uvarum species and C. tropicalis (F09) could decrease aldehyde (carbonyl containing) concentration in kiwi juice concentrate, whereas their content remained unchanged during M. guilliermondii (OZ01) and S. cerevisiae (OZ03) spoilage. The donors of amino may be the kiwi fruit amino acid and biogenic amines generated by yeast. It was reported that S. cerevisiae and H. uvarum can produce biogenic amines such as methylamine, agmatine and 2-phenylethylamine in different quantities.24 The carbonyl and amino group may be consumed differently leading to difference in amido bond vibration. Extracellular enzymes or killer toxins (extracellular protein or glycoprotein) excreted by yeast also provides CONH2 bond. As an evidence, H. uvarum produces protease, β-glucosidase25 and cellulase.26 M. guilliermondii was reported to secrete cellulose, xylanase and protease.27 C. intermedia was reported to exhibit protease activity. S. cerevisiae is an abundant extracellular enzyme producer, those enzymes include proteases, β-glucosidase and even acidic proteolytic thaumatin-like proteins and chitinases.28 Also, the yeast species that usually produce killer toxins involve S. cerevisiae, Candida and the Hanseniaspora genus. S. cerevisiae may produce killer toxin akin to O-glycosylated protein KT28 toxin to inhibit growth of other yeasts.29 These killer toxins might play a role in the yeast cocktail recognition model where more than one yeast species existed.
The compounds containing methyl (CH3), methylene (CH2), methyne (CH) cover a wide range of chemical categories and the probable sources were the kiwi juice composition and yeast metabolites. The RCO2H bonding may be from kiwi juice organic acid (such as ascorbic acid) as well as yeast metabolic synthesis (acetic acid, et al.). Although the NIR spectra pointing to a series of chemicals present in the spoiled kiwi juice, with the scope narrowed by CARS selection, possible contributing chemicals gradually exposed. The finding may lay the foundation for chemical analysis of yeasts recognition by NIRS.
Experimental
Kiwi juices
Two varieties of kiwi juices made from Qinmei or Hayward cultivars were obtained. Qinmei kiwi fruits were purchased from a local farmer and the clarified juices were prepared following peeling, squeezing, pectinase treatment for 2 h at 40 °C, 2 min of inactivation of pectinase through microwave oven and centrifugation at 7000×g for 5 min to precipitate pomace. Finally, seeds flocculating in the supernatant were removed by filtration through 0.45 μm filter and the clarified juices were stored under refrigeration at −20 °C. The pH of Qinmei kiwi juice was 3.26 and soluble solid content (SSC) was 12 °Brix. Hayward kiwifruit purees were obtained from GlobalHort Co., Ltd. and were further clarified following similar protocols. The pH of Hayward kiwi juice was 3.21 and soluble solid content (SSC) was 13 °Brix.
Spoilage sample preparation
The yeast strains with glucose tolerance higher than 50% (w/v) were previously isolated from kiwi fruit orchards and manufacturing environment in Shaanxi, China. The detailed species and isolate source information are listed in Table 1. Single strain spoilage samples were made by independently inoculating the listed ten strains, and yeast cocktail spoilage samples were prepared by employing all combinations of two or three strains from F09 (Candida tropicalis), F10 (Hanseniaspora uvarum), F16 (Candida intermedia), OZ01 (Meyerozyma guilliermondii) and OZ03 (Saccharomyces cerevisiae) representing each species to mimic real spoilage incidences where multiple yeast species participated in spoilage.
Glycerol preserved yeasts were grown in YPD (glucose 20g L−1, peptone 20g L−1 and yeast extract 10g L−1) for two successive recoveries to obtain the optimal growth state. Then the recovered culture was diluted and coated on YPD plate (glucose 20g L−1, peptone 20g L−1, yeast extract 10g L−1 and agar 20g L−1), and a single colony was picked and solubilized with the respective kiwi juice to adjust the seed culture to the concentration of 5 × 105 cells per mL. For single yeast spoilage kiwi juice, 100 μL of seed culture was added to 50 mL of kiwi juice sample, which was aseptically dispensed in 60 mL screw glass bottle. For the yeast cocktail spoilage kiwi juice, 50 μL or 33.3 μL of each seed culture was added to juice to keep consistent initial yeast inoculation level at 103 cells per mL for single and yeast cocktail spoilage juices.
Three replicate samples were conducted, including the yeast negative kiwi juice. The total sample number of single strain spoilage juice was 126 (3 replicates × 10 strains × 2 cultivars × 2 harvest time points + 6 blank juices). The 20 yeast cocktails combinations generate 246 samples (3 replicates × 20 yeast cocktail combinations × 2 cultivars × 2 harvest time point + 6 blank juices) for yeast cocktail spoilage juice.
NIR spectra acquisition
The juices were harvested at 24 and 72 h after inoculation, followed by centrifugation (7000×g, 10 min) to remove cell pellets so as to get rid of the interference from cell flocculation, and the obtained supernatants were kept at 4 °C and equilibrated in a rotary shaker at 25 °C for 20 min (120 rpm) right before NIR spectra acquisition. Upon spectra acquisition, juices were added into quartz cuvette with 1 mm light path. Fourier Transform Near-infrared Spectrometer (MPA, Bruker Optics, Ettlingen, Germany) was employed for spectra scanning at wavelengths range of 4000–12
000 cm−1 with a resolution of 8 cm−1 and 64 scans.
Principal component analysis
Principal component analysis was used to check clustering features of the raw spectra and detect outliers by evaluating sample residuals against the limit of F-distribution and Hotelling's T2 statistic at 5% significance level.
Spectra preprocessing
Juice samples inoculated with different yeast strains were encoded as integers from 1 to 10. Different yeast cocktails were also encoded as 1 to 20 for model establishment, and yeast negative kiwi juice samples were labelled as 0 in both cases. Raw spectra were pre-processed with Direct Orthogonal Signal Correction (DOSC) before the establishment of the classification model. DOSC loosen the complete orthogonality constraint and calculates components that describe the largest variation in NIR spectra variable (X) and are orthogonal to labels (Y). In the study, two components were used and tolerance value was set at 1 × 10−3 according to ref. 30.
SVM models and optimization
Support vector machine (SVM, Libsvm 3.20 (ref. 31)) was employed on the NIR spectra to recognize the responsible yeast strain or yeast cocktail. C-SVC and radial basis function kernel (RBF) function was used for SVM model development. A time-saving 10-fold cross validation was conducted on the training sets which was consisted of two-thirds of the spectra and external validation were performed on prediction sets which contained one-third of the spectra. Optimization including grid-search optimization (GS), particle swarm optimization (PSO) and genetic algorithm (GA) from FarutoUltimate 3.14 (ref. 32) were carried out for searching for penalty parameter C and kernel parameter γ relevant to boundary complexity to enhance model performance. CARS16 was employed to extract key variables for single strain and yeast cocktail models, as well as key variables responsible for discriminating the two kiwi juices. In all cases, extraction of 5 maximal principal components, 10-fold cross validation and 50 (single yeast) or 100 (yeast cocktail) Monte Carlo sampling runs were used in CARS. All the treatment and modeling were realized with Matlab 2012a (The MathWorks, Inc.).
Model performance
The performance of developed SVM models was assessed with overall correct rate (CR). In addition, sensitivity and positive predictive value (PPV) were also used. Sensitivity is denoted as the ratio of juice samples whose spoilers are correctly identified to the number of samples in each spoilage category. Positive predictive value (PPV) is termed as the ratio of truly accurately identified strain or yeast cocktail to the sample numbers in each predicted category. Sensitivity is a measurement of true-spoiler accuracy and PPV is a measurement of the predicted-spoiler accuracy. For both parameters, good model will have sensitivity and PPV values at 1.00 while extremely poor models will have value of 0. They provide a comprehensive description of model capability.
Conclusions
This study developed NIRS-SVM models that can accurately recognize kiwi juice spoilage and find the responsible tested yeast contaminants. A preliminary inference of possible chemicals (water and amino bond) that are contributive to the developed NIR-SVM models had been obtained. Further verification research on linking the exact chemicals are still necessary. The ease of preparation, high throughput and good performance of the developed method would be contributive to food quality and security control of osmotolerant yeasts spoilage in the food industry.
Conflicts of interest
There are no conflicts to declare.
Acknowledgements
This work was financed by major projects of strategic emerging industries in Shaanxi province of China [2016KTCQ03-12]; and the National Natural Science Foundation of China [31371814].
Notes and references
- T. C. Dakal, L. Solieri and P. Giudici, Int. J. Food Microbiol., 2014, 185, 140–157 CrossRef CAS PubMed.
- C. Kurtzman, J. W. Fell and T. Boekhout, The yeasts: a taxonomic study, Elsevier, 2011 Search PubMed.
- J. I. H. Pitt and A. Diane, Fungi and food spoilage, Springer, New York, 2009 Search PubMed.
- M. C. Rojo, C. Torres Palazzolo, R. Cuello, M. Gonzalez, F. Guevara, M. L. Ponsone, L. A. Mercado, C. Martinez and M. Combina, Food Microbiol., 2017, 64, 7–14 CrossRef CAS PubMed.
- H. X. Wang, Z. Q. Ru, F. Y. Long, C. F. Guo, Y. H. Yuan and T. L. Yue, J. Food Prot., 2015, 78, 2052–2063 CrossRef PubMed.
- C. Niu, Y. Yuan, Z. Hu, Z. Wang, B. Liu, H. Wang and T. Yue, Int. J. Food Microbiol., 2016, 232, 126–133 CrossRef PubMed.
- G. D. Casey and A. D. W. Dobson, Int. J. Food Microbiol., 2004, 91, 327–335 CrossRef CAS PubMed.
- Z. Li, P.-P. Wang, C.-C. Huang, H. Shang, S.-Y. Pan and X.-J. Li, Food Analytical Methods, 2014, 7, 1337–1344 CrossRef.
- J. Chitra, M. Ghosh and H. N. Mishra, Food Control, 2017, 78, 342–349 CrossRef CAS.
- C. Quintelas, D. P. Mesquita, J. A. Lopes, E. C. Ferreira and C. Sousa, Int. J. Pharm., 2015, 492, 199–206 CrossRef CAS PubMed.
- Y.-Z. Feng, G. Downey, D.-W. Sun, D. Walsh and J.-L. Xu, J. Food Eng., 2015, 149, 87–96 CrossRef.
- C. Cortes and V. Vapnik, Mach. Learn., 1995, 20, 273–297 Search PubMed.
- Y. Bao, F. Liu, W. Kong, D. W. Sun, Y. He and Z. Qiu, Food Bioprocess Technol., 2014, 7, 54–61 CrossRef CAS.
- H. Zhan, J. Xi, K. Zhao, R. Bao and L. Xiao, Food Control, 2016, 67, 114–118 CrossRef CAS.
- M. Schmutzler, A. Beganovic, G. Böhler and C. W. Huck, Food Control, 2015, 57, 258–267 CrossRef CAS.
- H. Li, Y. Liang, Q. Xu and D. Cao, Anal. Chim. Acta, 2009, 648, 77–84 CrossRef CAS PubMed.
- E. Lanza and B. Li, J. Food Sci., 1984, 49, 995–998 CrossRef CAS.
- D. Cozzolino, M. Parker, R. G. Dambergs, M. Herderich and M. Gishen, Biotechnol. Bioeng., 2006, 95, 1101–1107 CrossRef CAS PubMed.
- M. Gishen, R. G. Dambergs and D. Cozzolino, Aust. J. Grape Wine Res., 2005, 11, 296–305 CrossRef CAS.
- J. Guo, Y. Yuan, P. Dou and T. Yue, Food Chem., 2017, 232, 552–559 CrossRef CAS PubMed.
- H. M. Dawes and J. B. Keene, J. Agric. Food Chem., 1999, 47, 2398–2403 CrossRef CAS PubMed.
- R. Tsenkova, Spectrosc. Eur., 2010, 22, 6 Search PubMed.
- M. S. Wong and D. W. Stanton, J. Food Sci., 1989, 54, 669–673 CrossRef CAS.
- M. Caruso, C. Fiore, M. Contursi, G. Salzano, A. Paparella and P. Romano, World J. Microbiol. Biotechnol., 2002, 18, 159–163 CrossRef CAS.
- C. Charoenchai, G. Fleet, P. Henschke and B. Todd, Aust. J. Grape Wine Res., 1997, 3, 2–8 CrossRef CAS.
- M. Strauss, N. Jolly, M. Lambrechts and P. Van Rensburg, J. Appl. Microbiol., 2001, 91, 182–190 CrossRef CAS PubMed.
- F. C. Gomes, S. V. Safar, A. R. Marques, A. O. Medeiros, A. R. O. Santos, C. Carvalho, M.-A. Lachance, J. P. Sampaio and C. A. Rosa, Antonie van Leeuwenhoek, 2015, 107, 597–611 CrossRef CAS PubMed.
- B. Younes, C. Cilindre, P. Jeandet and Y. Vasserot, Food Res. Int., 2013, 54, 1298–1301 CrossRef CAS.
- H. Bussey, Mol. Microbiol., 1991, 5, 2339–2343 CrossRef CAS PubMed.
- S. Wold, H. Antti, F. Lindgren and J. Öhman, Chemom. Intell. Lab. Syst., 1998, 44, 175–185 CrossRef CAS.
- C.-C. Chang and C.-J. Lin, ACM Transactions on Intelligent Systems and Technology (TIST), 2011, 2, 27 Search PubMed.
- Y. Li, LIBSVM-farutoUltimate Version:a Toolbox with Implements for Support Vector Machines based on Libsvm, 2009 Search PubMed.
|
This journal is © The Royal Society of Chemistry 2018 |
Click here to see how this site uses Cookies. View our privacy policy here.