DOI:
10.1039/C7RA12067B
(Paper)
RSC Adv., 2018,
8, 5042-5057
Metabonomic strategy for the detection of metabolic effects of probiotics combined with prebiotic supplementation in weaned rats†
Received
2nd November 2017
, Accepted 15th January 2018
First published on 30th January 2018
Abstract
The purpose of this study is to investigate the effects of probiotics combined with prebiotics (PP) supplementation on weaned rat metabolism. A metabonomic strategy employing 1H-NMR spectroscopy and multivariate data analysis was used to examine weaned rat biological responses to PP supplementation. Male Sprague-Dawley rats (post-natal day 21, PD 21) received probiotics (Lactobacillus acidophilus NCFM (L-NCFM) and Bifidobacterium lactis Bi-07 (B-LBi07), 1
:
1, 1.0 × 1011 cfu kg−1) and prebiotics (Lycium barbarum polysaccharides (LBP), Poria cocos polysaccharides (PCPs) and Lentinan, 1
:
1
:
1, 24 g kg−1) via intragastric administration for 28 consecutive days. Urine and feces were collected for analysis. Significant topographical metabolic variations were present in urine and feces. Urinary metabolites upregulated by PP treatment included alanine, N-acetylglycine, glutamine, dimethylamine, phosphorylcholine, ethylene glycol, mannitol, phenylacetylglycine and glycoate, which were related to alanine, aspartate and glutamate metabolism, and choline metabolism. Feces-derived metabolites, including caproate, valerate, butyrate, propionate, lactate, acetate, succinate, methanol, threonine and methionine, were significantly increased, which were related to short-chain fatty acid (SCFA) metabolism and TCA cycle metabolism. These results indicate that dietary PP supplementation can regulate common systemic metabolic processes, including energy metabolism, amino acid metabolism, lipid metabolism, nucleic acid metabolism, and gut microbiota-related metabolism. This study also illuminates the vital role of PP supplementation in regulating the metabolism of weaned rats.
Introduction
A multiplicity of factors and forces, such as genetic, environmental and stochastic ones, affect how organisms grow and develop. Weaning, which is an essential and critical event for human and rodent animals, may influence nutritional metabolism, and growth and immunity during adulthood.1,2 The period that an infant grows into a child is also a crucial phase illustrated by raised nutritional stress and mortality in humans.3 Weaned animals are subjected to their diet changing from breastfeeding to solid foods. Changes in nutritional status during early periods of life may lead to a decrease in food intake and impair immune function, both of which contribute to an increasing susceptibility to disease.4 In addition, weaned animals are also affected by the separation from their mother and littermates, communication with unfamiliar compatriots, and establishment of a new social hierarchy.5 The above combined stresses could result in metabolic disorder, gastrointestinal dysfunction, impaired mucosal barrier function, and imbalance of intestinal microbiota, which are responsible for the impaired growth and health of an animal.6–8 Therefore, finding methods to solve the problems associated with weaned stress is of vital importance. Previous studies have shown that problems related to weaned stress in animals can be alleviated by nutritional modulation.9,10
Probiotics are living microorganisms that is beneficial to the health of the host organism when administered in appropriate amounts.11 Probiotics can improve the digestibility of starch and protein in vitro.12 Additionally, several studies propose that probiotics can stimulate the immune system, to prevent or even treat bowel infection.13 Moreover, other studies have shown that probiotics might contribute to the integrity of the intestinal barrier and that they are effective in treating inflammatory bowel diseases.14 Lactobacilli and Bifidobacteria, the most commonly used species, are incorporated into food, and then survive in the host digestive tract where they modulate the gut microbiota and bring host beneficial effects.
Prebiotics, as a kind of indigestible component in food, can enter the lower gastrointestinal tract and selectively stimulate the growth of health-promoting bacteria.15,16 Dietary prebiotics treatment, as one of the representative strategies, selectively change the substrates that can be utilized by gut microbiota, and have a great impact on host metabolism and physiology.17 Lycium barbarum polysaccharides (LBP), Poria cocos polysaccharides (PCPs) and Lentinan, the three main types of polysaccharides came from Chinese herbal medicine, have many biological activities, including immunomodulation, anti-tumour, antioxidant, anti-diabetes,18 anti-virus, and anti-fatigue functions.19
The combination of probiotics and prebiotics is termed ‘synbiotics’. Synbiotic refers to a nutritional supplementation which combines probiotics and prebiotics in a form of synergism.20 However, few studies have assessed the effects of synbiotics regarding the changes of metabolism in weaned animals. In nutritional research, metabonomic is the dynamic complement of the endogenous metabolites within the integrated biological systems and is a powerful top-down systems biological tool in nutritional research that can provide the end point of consequences of nutritional intervention, which can help us understand how did metabolic balances disturbed by interventions.9 In addition, metabonomic is useful in exploring the complex relationship between nutritional intervention and metabolism, and figuring out the effect of dietary components on health maintenance and disease development.
In the present study, we investigated the metabolic profiles of biofluids and feces in weaned rats fed with probiotics (L-NCFM and B-LBi07) and prebiotics (LBP, PCPs and Lentinan) by using 1H-NMR integrated with multivariate data analysis. The correlation of metabolic alteration in multiple biological matrices including urine and feces was systematically analyzed. The purpose of this study was to discover the systematic metabolites changes and supply a molecular mechanism of PP regulating the metabolism in weaned rats.
Materials and methods
Animals
21 days old male specific-pathogen-free Sprague-Dawley rats (body weight = 35–45 g, n = 24) were randomly separated into two different treatments (CON, n = 12; PP, n = 12) which lasted 4 weeks. All animals were obtained from the Center of Laboratory Animal Science of Guangdong (Guangdong, China) and raised in a specific sterile colony under a complete controlled condition (temperature 23 ± 2 °C; humidity 50–70%; 12 h-light-dark cycle and lighting at 8:00 am) at the Laboratory Animal Center of Guangzhou University of Chinese Medicine (Guangzhou, China). All rats were free access to standard rat chow and tap water as they pleasured. In this study, the animal experiment was approved by the Institutional Animal Care and Use Committee of Guangzhou University of Chinese Medicine, and strictly in accordance with the National Institute of Health guidelines on the ethical use of animals.
Probiotics and prebiotics treatment
Rats in the CON group were administered with physiological saline, while probiotics (L-NCFM and B-LBi07) was administered at a final concentration of 1.0 × 1011 cfu kg−1 (1
:
1), LBP, PCPs and Lentinan (1
:
1
:
1) were used as prebiotics at a concentration of 24 g kg−1. Each treatment was administered for 28 days. Probiotics and prebiotics were provided by Infinitus (China) Company Ltd.
Sample collection and extraction
On post-dose day 14 and 28, rats were randomly individually placed in a metabolic cage after 12 h of symbiotic treatment. Then urine samples and feces samples were collected in Eppendorf tubes on ice from 20:00 pm to 8:00 am. The samples from each group were stored at −80 °C for NMR analyses.
Urine samples were thawed at room temperature. A volume of 600 μL urine samples and 60 μL phosphate buffer solution (1.5 M, K2HPO4/NaH2PO4 = 4
:
1, pH 7.4, 0.1% NaN3, 0.05% TSP, 99.9% D2O) were mixed and then centrifuged at 16
000g at 4 °C for 10 min to remove precipitates. A volume of 600 urine supernatants were transferred to 5 mm NMR tube. Feces samples were thawed at room temperature and extracted according to an optimized sample preparation procedure described before.21
NMR measurement and preprocessing
1H-NMR spectra were obtained using a Bruker AVIII 600 MHz spectrometer (Bruker Biospin, Germany) at 600.13 MHz and 298k. The acquisition parameters were essentially mentioned in previous study.22 A one-dimensional pulse sequence was used [recycle delay (RD)–G1–90°–t1–90°–tm–G2–90°–acquisition]. The water signal was suppressed by irradiation during RD of 2 s, and mixing time (tm) of 80 ms. For each sample, the 90° pulse length was adjusted to about 10 μs and a total of 64 transients were accumulated into 32k data points over spectral width of 20 ppm. Two-dimensional (2D) NMR experiments (1H–1H correlation spectroscopy (COSY), total correlation spectroscopy (TOCSY), total correlation spectroscopy (TOCSY), 1H–13C heteronuclear single quantum correlation spectroscopy (HSQC) and 1H–13C heteronuclear multiple bond correlation spectroscopy (HMBC)) were performed to assign NMR signal as previously reported.23,24 The metabolites were simultaneously identified based on the Human Metabolome Database (http://www.hmdb.ca/) and metabonomic toolbox (Chenomx NMR Suit 7.6, Chenomx, Canada) as well as published work.25,26
The phase and baseline of 1H-NMR spectra were manually corrected, and calibrated to TSP at 0.00 pm with Topspin 2.1 software (Bruker Biospin, Germany). To exclude the interference of water signal and urea signal, the urinary spectral region (δ4.70–4.95 and δ5.50–6.25) and the feces spectral region (δ4.68–4.95) were carefully discarded. The spectra in the ranges of 0.5–9.5 ppm for urine samples and 0.5–8.5 ppm for feces samples were segmented into 0.004 ppm using the AMIX (V3.9.14, Bruker Biospin). Urinary spectra were normalized to the intensity of the TSP signal and feces spectra were normalized to total area.
Multivariate data analysis
Normalized data were imported into the software package SIMCA-P+ (V. 13.0; Umetrics, Sweden) for multivariate data analysis. Principal component analysis (PCA) was carried out on the unit variance (UV)-scaled data with the scores plots showing intergroup differences and the possible presence of outliers. Partial least squares-discriminant analysis (PLS-DA) was applied to find the discriminations between groups on the basis of their different metabolic responses. A permutation tests with 200 randomizations was used to evaluate the performance of the model and the relevance of supposedly important metabolites,27,28 which were identified on the basis of the values of PLS-DA regression coefficients and variable importance in projection (VIP) scores.29 VIP indicates the contribution of the individual variable in the definition of the model. Because the mean of squared VIP scores is equal to 1, values >1 are considered to be significant variables. And orthogonal projection to latent structure-discriminant analysis (OPLS-DA) was conducted with the unit variance (UV)-scaled data and the qualities of models were assessed with CV-ANOVA30 with p < 0.05 as significant difference. In order to stay the spectral features for the ease of interpretation, back-transformation31 of the loadings generated from the OPLS-DA was performed prior to generating the loadings plots, which were color-coded with the Pearson linear correlation coefficients of variables (or metabolites) using an in-house developed script in MATLAB (Version 7.1, Mathworks Inc, USA). In these loadings plots, the warm-colored variables contributes more to intergroup differentiations than cool-colored ones.
Correlation analyses
Correlation analyses were performed in R using the “cor” function. Relationships with a Pearson's correlation coefficient greater than 0.9, or smaller than −0.9, were used to build correlation networks. Meanwhile MSEA,32 an approach to value whether significant metabolites found in known metabolic pathway maps or databases coincide with the metabolic signature at a certain level in the biochemical pathway or not, was applied to reduce the massive number of insignificant metabolic pathways.
The concentration changes of metabolites were calculated individually against their concentration in CON group, expressing as (CPP − CCON)/CCON, where CPP and CCON stood for the average concentrations of a metabolite in PP and CON groups respectively.
Whole-metagenome shotgun sequence analysis
The extracted DNA from the CON group and PP group (n = 6, average 3 per group) were sequenced on the Hiseq 4000 Sequencer (Hiseq 4000 SBS Kit, Illumina) with the read lengths 150 bp and insert size of the DNA fragments 350 bp according to the manufacturer's directions by Huada Gene Institute. To determine the abundance of genes, the high quality reads from each sample were aligned against the gene catalogue by SOAP2.33 Only genes with ≥2 mapped reads were remained in a sample.34 The abundance of genes was calculated by counting the number of reads and normalizing by gene length.35 BLASTP36 was used to search the protein sequences of the predicted genes within the KEGG database37 with E ≤ 1 × 10−5. The genes were annotated using the KEGG homologs with the lowest e-value. Each protein was allocated to KO (KEGG orthologue group) based on the highest scoring hits with at least one HSP >60 bits.38 The abundance of KO was calculated by summing the abundance of genes annotated to the same feature.
Statistical analysis
In this study, all data acquisition was obtained by concealing the group of the animals. Differences in metabolite levels in different groups were analyzed with independent-samples T-tests using SPSS software (version 13.0, SPSS). A statistically significant difference was confirmed when P value <0.05. Other statistical tests for significant difference were performed in GraphPad Prism version 5. Pearson's correlation between different metabolites and the corresponding P value were calculated using the MATLAB function. Furthermore, correlation heatmap was visualized by the ‘heat map’ module of R software (version 3.4.1). Network was constructed by cytoscape (version 3.5.1).
Results
1H-NMR spectra of urine and feces extracts
The representative 1H-NMR spectra for extracts from urine and feces on day 28 were shown (Fig. 1), respectively, from a randomly selected rat. The endogenous metabolites involved in the spectra were assigned based on the literature.39,40 A total of 129 metabolites (94 urine-derived and 55 feces-derived metabolites) were assigned and listed in Tables S1 and S2.†
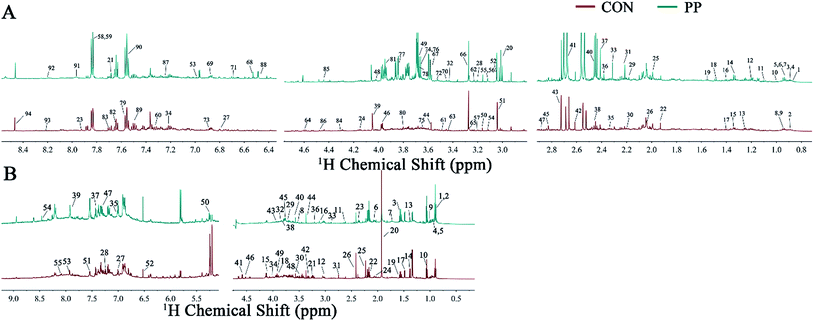 |
| Fig. 1 Representative 600 MHz 1H-NMR spectra obtained from urine extracts (A) and feces extracts (B) at day 28 post treatment. Metabolite keys are shown in Tables S1 and S2.† | |
We identified a series of urine metabolites including the metabolites in amino acid metabolism (isoleucine, leucine, ketoleucine, threonine, lysine, alanine, citrulline, ornithine, glutamate, glutamine, proline, β-alanine, asparagine, sarcosine, Nτ-methylhistidine, N6,N6,N6-trimethyllysine, histidine, N-acetyllysine, N-acetyltyrosine and phenylalanine), energy metabolism (lactate, acetate, acetone, pyruvate, succinate, 2-oxoglutarate, citrate, creatine, creatine phosphate, creatinine, β-glucose, glycogen, tartrate, 1-methylnicotinamide and fumarate), choline metabolism (choline, o-acetylcholine, methylamine (MA), dimethylamine (DMA), N,N-dimethylglycine (DMG), trimethylamine (TMA), N-nitrosodimethylamine, phosphorylcholine (PC) and trimethylamine-N-oxide (TMAO)), ketone body metabolism (acetoacetate, 2-hydroxybutyrate and acetone), microbial-derived metabolism (hippurate, isobutyrate, 4-hydroxyhippurate, N-phenylacetylglycine (N-PAG), phenylacetylglycine (PAG), indole-3-acetate (IAA), N-acetylglycine, p-cresol glucuronide (p-CG), p-cresol sulfate (p-CS), p-cresol, 4-hydroxyphenylacetate, p-hydroxyphenylacetate and isovalerylglycine), purine metabolism (xanthine, hypoxanthine and oxypurinol), and some other metabolites like taurine, trigonelline, allantion, 4-pyridoxine and betaine.
In addition, the main metabolites of feces spectra consisted of amino acids (leucine, isoleucine, valine, threonine, lysine, alanine, proline, glutamate, glutamine, methionine, desaminotyrosine, aspartate, sarcosine, asparagine, tyrosine, phenylalanine, histidine, glycine and serine), carbohydrates (β-glucose, α-glucose, β-arabinose and α-arabinose), TCA cycle intermediates (pyruvate, succinate and fumarate), microbial-related metabolites (butyrate, propionate, acetate, 3-phenylpropionate and phenylacetate), methylamine metabolites (DMA, TMA, ethanolamine and choline), ketone bodies (α-keto-β-methyl-valerate, α-ketoisocaproate and α-ketoisovalerate), purine and pyrimidine (uracil and adenine), lactate, cadaverine, creatine, malonate, taurine and betaine.
Multivariate analysis of 1H-NMR spectroscopic data of urine extracts
The PCA score plot has shown that there were obvious differences between CON and PP groups and no outliers in urinary metabonomic on day 14 and 28 (Fig. S1A† and 2A). The results of PLS-DA have shown that CON group and PP group can separate well on day 14 (Fig. S1B†) and day 28 (Fig. 2B), respectively, validating by a permutation test with 200 iterations (Fig. S1C† and 2C). OPLS-DA was further carried out on CON and PP groups at various time points. The R2 and Q2 values confirmed good qualities of all models (Fig. S1D† and 2D). Further evaluation with CV-ANOVA suggested that the p-values of these models for validity were considerably smaller than 0.05. The corresponding loading plots (Fig. 2E) further showed that about 32 urine metabolites had significant differences between PP and CON groups throughout 28 day treatment (Table 1).
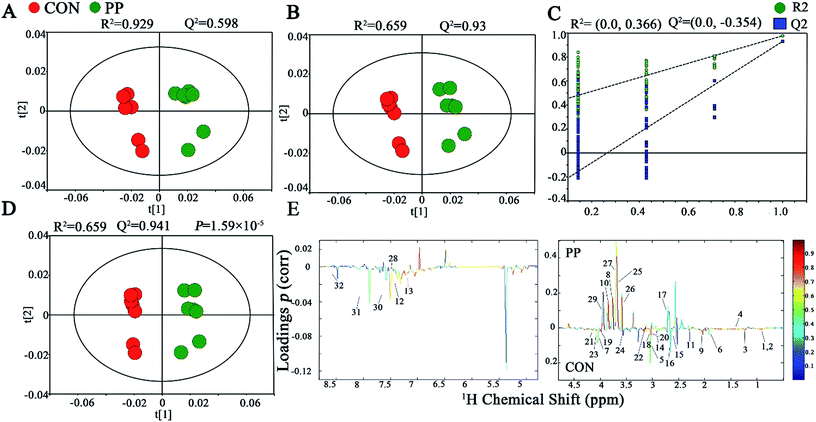 |
| Fig. 2 1H-NMR-based metabonomic analysis of urine samples. PCA (A) and PLS-DA (B) score plots derived from the 1H-NMR spectra of urine extracts obtained from CON and PP groups, and cross validation (C) by permutation test at day 28. OPLS-DA (D) score plot and coefficient plot (E) derived from the 1H-NMR spectra of urine extracts showing the discrimination between CON and PP groups at day 28. Keys to metabolites assignment are given in Table 1. | |
Table 1 Statistical analysis results of the main metabolite change in urine at days 14 and 28a
Key |
Metabolites |
Chemical shift |
Variations |
Day 14 |
Day 28 |
*: indicates significant changes compared with control *p < 0.05, **p < 0.01. |
1 |
Leucine |
0.925(d), 0.936(s), 1.703(m), 3.725(d) |
— |
↓** |
2 |
Ketoleucine |
0.944(d), 2.10(m), 2.617(d) |
↓* |
↓** |
3 |
3-Hydroxyisovalerate |
1.275(s), 2.357(s) |
↓** |
↓** |
4 |
Alanine |
1.489(d), 3.780(q) |
↑* |
↑* |
5 |
Ornithine |
1.775(m), 1.828(m), 1.929(m), 3.044(t), 3.782(d) |
— |
↓** |
6 |
Acetate |
1.927(s) |
↓* |
↓** |
7 |
Proline |
2.023(m), 2.334(m), 3.320(m), 3.392(m), 4.147(t) |
↓** |
↓** |
8 |
N-Acetylglycine |
2.044(s), 3.763(d), 7.997(s) |
↑** |
↑** |
9 |
Glutamate |
2.056(m), 2.334(m), 3.741(q) |
↓** |
↓** |
10 |
Glutamine |
2.109(m), 2.141(m), 2.425(m), 2.462(m), 3.766(t), 6.872(s), 7.589(s) |
↑* |
↑* |
11 |
Acetone |
2.229(s) |
↓** |
↓* |
12 |
p-Cresol sulfate |
2.347(s), 7.217(d), 7.293(d) |
↓** |
↓** |
13 |
p-Cresol glucuronide |
2.299(s), 7.055(m), 7.237(m) |
↓* |
↓* |
14 |
2-Oxoglutarate |
2.429(t), 2.995(t) |
— |
↓** |
15 |
Citrate |
2.552(d), 2.658(d) |
↓* |
↓** |
16 |
Methylamine |
2.613(s) |
— |
↓** |
17 |
Dimethylamine |
2.719(s) |
↑* |
↑* |
18 |
Methylguanidine |
2.828(s), 3.356(s) |
— |
↓** |
19 |
Asparagine |
2.862(dd), 2.962(m), 3.995(dd) |
↓** |
↓** |
20 |
Trimethylamine |
2.885(s) |
— |
↓* |
21 |
Creatinine |
3.043(s), 4.052(s) |
— |
↓** |
22 |
N6,N6,N6-Trimethyllysine |
3.109(s) |
↓** |
↓** |
23 |
N-Methylhydantoin |
2.917(s), 4.078(s) |
↓* |
↓* |
24 |
Choline |
3.191(s), 3.504(t), 4.085(m) |
↓** |
↓** |
25 |
Phosphorylcholine |
3.203(s), 3.671(t), 4.190(dd) |
↑** |
↑** |
26 |
Ethylene glycol |
3.662(s) |
↑** |
↑** |
27 |
Mannitol |
3.677(dd), 3.770(m), 3.805(d), 3.873(dd) |
↑** |
↑** |
28 |
Phenylacetylglycine |
3.680(s), 3.746(d), 7.365(t), 7.425(t) |
↑** |
↑** |
29 |
Glycolate |
3.932(t) |
↑** |
↑** |
30 |
Benzoate |
7.474(t), 7.546(t), 7.863(d) |
— |
↓* |
31 |
Xanthine |
7.909(s) |
↓** |
↓* |
32 |
Formate |
8.467(s) |
— |
↓* |
The results revealed that PP treatment for 28 day induced significant elevations of alanine, N-acetylglycine (N-AG), glutamine, DMA, PC, ethylene glycol, mannitol, PAG and glycoate, while conspicuously decreased levels of leucine, ketoleucine, hydroxyisovalerate, ornithine, acetate, proline, glutamate, acetone, p-CS, p-CG, α-oxoglutarate, citrate, MA, methylguanidine, asparagine, TMA, creatinine, N,N,N-trimethyllysine, N-methylhydantoin, choline, benzoate, xanthine and formate (Fig. 3).
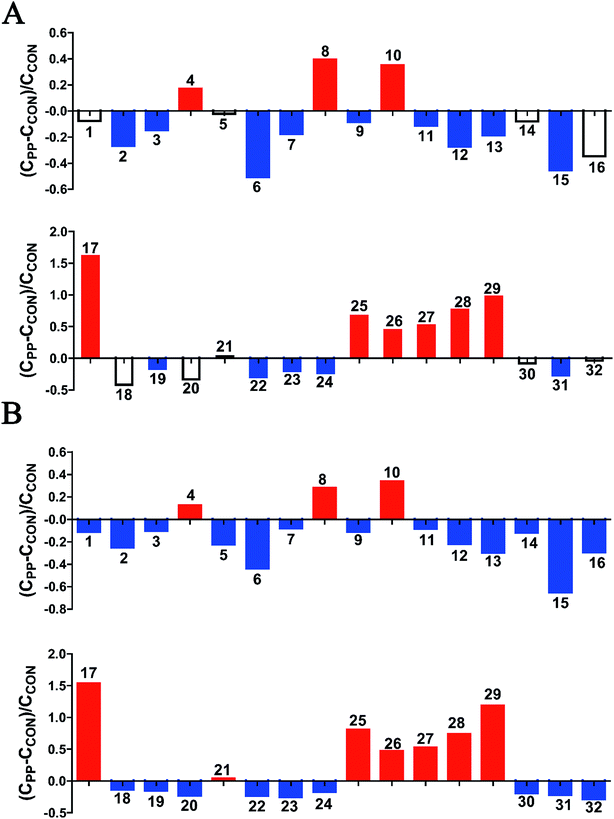 |
| Fig. 3 Relative changes for metabolite concentrations in urine induced by PP supplementation at day 14 (A) and day 28 (B). Solid bars indicate significant changes with red for increase and blue for decrease, whereas hollow bars mean no significant changes. Keys to metabolites assignment are given in Table 1. | |
Multivariate analysis of 1H-NMR spectroscopic data of feces extracts
In PCA score plot, PP and CON groups were satisfactory separated on day 28 (Fig. 4A), whereas the score plot displayed two moderate outliers but did not show clear and obvious classifications on the samples on day 14 (Fig. S2A†). PLS-DA models were established to classify samples from CON and PP groups (Fig. 4B and S2B†). The results of permutation tests had shown good qualities of all models (Fig. 4C and S2C†). To obtain the metabolic variations associated with different groups, OPLS-DA were performed on the comparison of PP and CON groups on day 14 (Fig. S2D†) and day 28 (Fig. 4D), respectively, revealing changing and distinctive metabolite compositions in different time point. The model quality indicators (R2 and Q2) clearly showed that the extracts obtained from feces were distinctive in their metabolite compositions. The above results were further supported by the consequences from the model evaluation with CV-ANOVA (P < 0.05). The corresponding loading plots (Fig. 4E) further showed that about 22 feces metabolites had significant level differences between PP and CON groups throughout 28 days treatment (Table 2).
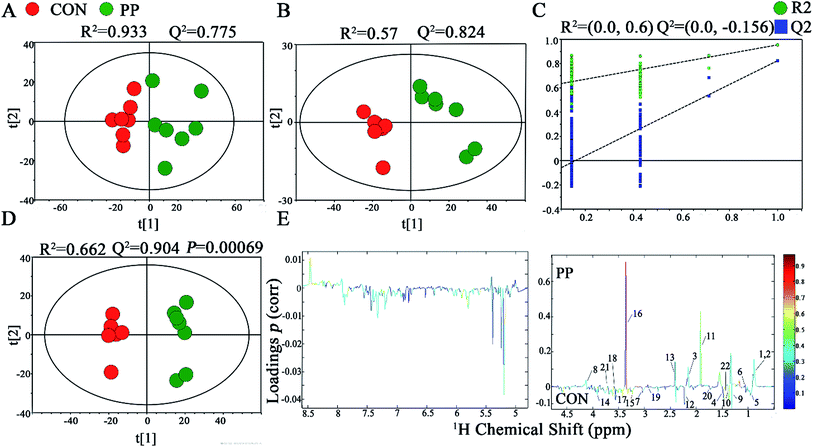 |
| Fig. 4 1H-NMR-based metabonomic analysis of feces samples. PCA (A) and PLS-DA (B) score plots derived from the 1H-NMR spectra of feces extracts obtained from CON and PP groups, and cross validation (C) by permutation test at day 28. OPLS-DA (D) score plot and coefficient plot (E) derived from the 1H-NMR spectra of feces extracts showing the discrimination between CON and PP groups at day 28. Keys to metabolites assignment are given in Table 2. | |
Table 2 Statistical analysis results of the main metabolite change in feces at days 14 and 28a
Key |
Metabolites |
Chemical shift |
Variations |
Day 14 |
Day 28 |
*: indicates significant changes compared with control *p < 0.05, **p < 0.01. |
1 |
Caproate |
0.887(t), 1.292(m), 1.309(m), 1.556(m), 2.178(t) |
↑** |
↑** |
2 |
Valerate |
0.899(t), 1.309(m), 2.178(d) |
↑** |
↑** |
3 |
Butyrate |
0.899(t), 1.562(m), 2.150(t) |
↑** |
↑** |
4 |
Isoleucine |
0.936(t), 0.995(d), 1.249(m), 1.452(m), 1.971(m), 3.655(d) |
↓* |
↓* |
5 |
Valine |
0.979(d), 1.013(d), 2.275(m), 3.617(d) |
↓** |
↓** |
6 |
Propionate |
1.061(t), 2.191(d) |
↑* |
↑* |
7 |
α-Ketoisovalerate |
1.127(d), 3.022(m) |
↓* |
↓* |
8 |
Lactate |
1.331(d), 4.115(q) |
↓* |
↑** |
9 |
Cadaverine |
1.483(d), 1.724(m), 3.022(t) |
↓* |
↓* |
10 |
Alanine |
1.489(d), 3.780(q) |
↓* |
↓* |
11 |
Acetate |
1.927(s) |
↑** |
↑** |
12 |
Pyruvate |
2.373(s) |
↓* |
↓* |
13 |
Succinate |
2.413(s) |
↑** |
↑** |
14 |
Creatine |
3.028(s), 3.926(s) |
— |
↓* |
15 |
Taurine |
3.243(t), 3.435(t) |
— |
↓* |
16 |
Methanol |
3.366(s) |
↑** |
↑** |
17 |
Glycine |
3.566(s) |
↓** |
↓** |
18 |
Threonine |
1.330(d), 3.579(d), 4.269(m) |
↓* |
↑** |
19 |
Trimethylamine |
2.871(s) |
↓* |
↓* |
20 |
5-Aminovalerate |
1.624(t), 1.650(t), 2.237(m), 3.022(m) |
↓* |
↓* |
21 |
Methionine |
2.141(s), 2.169(m), 2.648(t), 3.853(m) |
↑** |
↑** |
22 |
Lysine |
1.415(m), 1.719(m), 1.869(m), 3.018(t), 3.741(t) |
↓* |
↓* |
The results illuminated that PP supplementation for 28 day caused obvious level enrichment of caproate, valerate, butyrate, propionate, lactate, acetate, succinate, methanol, threonine and methionine, while noticeably reduced levels of isoleucine, valine, α-ketoisovalerate, cadaverine, alanine, pyruvate, creatine, taurine, glycine, TMA, aminovalerate and cadaverine (Fig. 5).
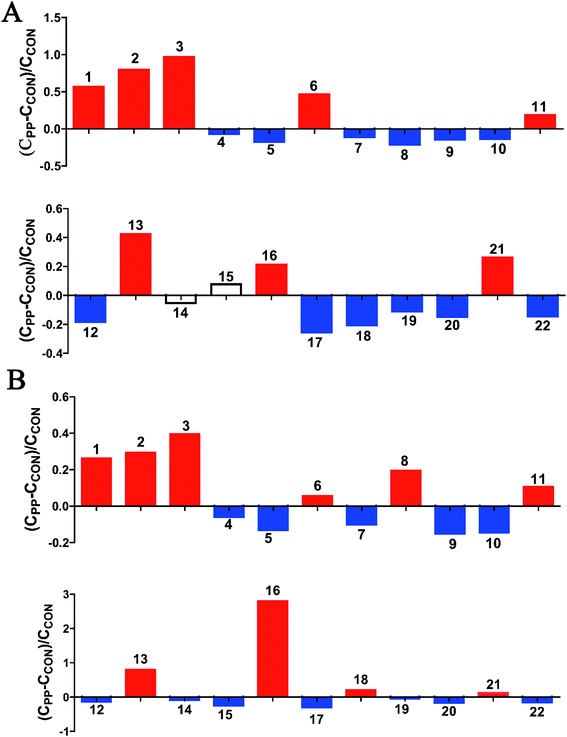 |
| Fig. 5 Relative changes for metabolite concentrations in feces induced by PP supplementation at day 14 (A) and day 28 (B). Solid bars indicate significant changes with red for increase and blue for decrease, whereas hollow bars mean no significant changes. Keys to metabolites assignment are given in Table 2. | |
Metabolite correlation network analysis
A popular way of exploring metabonomic data is correlation network analysis, which can capture the potential biomarker to illustrate the links in the potential biomarker metabolic pathway. Correlations of urinary significant metabolites NMR peak areas and feces significant metabolites NMR peak areas were performed, respectively (Fig. 6A and B). The heatmap showed that urinary metabolites have closely linked with each other, while feces metabolites were in the same situation. The Pearson correlation coefficient (r) ≥0.9 or ≤−0.9 was used to indicate a significant correlation. According to the |r| ≥ 0.9, the network, including urine network and feces network, were constructed (Fig. 6C and D). In the urine network, most of the amino acid metabolism, choline metabolism and microbiota-related metabolism were simultaneously changed in urine samples. In feces network, some obvious differences were observed between CON and PP groups. SFCAs-related metabolites significantly increase in PP group, whereas branched-chain amino acids obvious enriched in CON group.
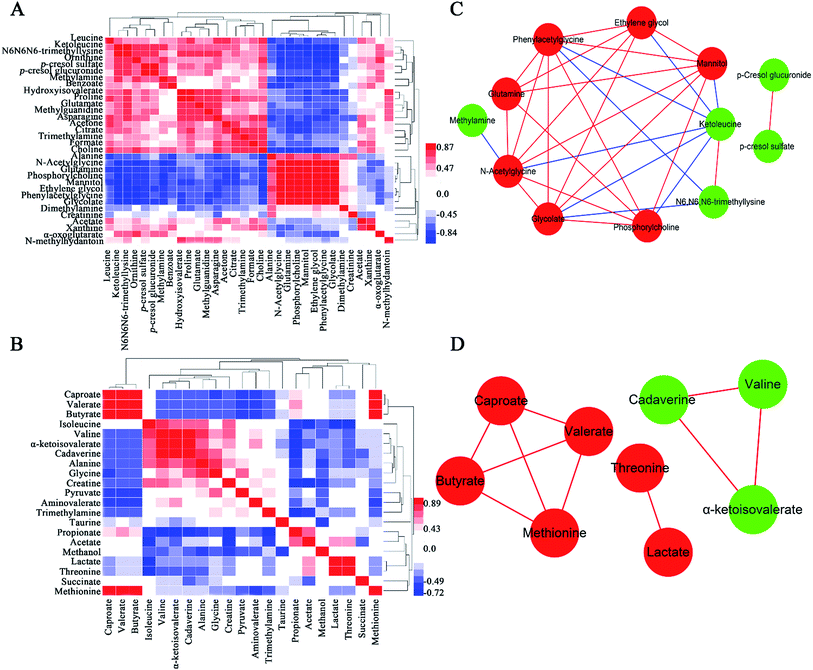 |
| Fig. 6 Correlation of urinary metabolite NMR peak areas (A) and feces metabolite NMR peak areas (B). Red denotes a positive correlation and blue a negative correlation. Network analysis of urinary metabolites (C) and feces metabolites (D) with |r| ≥ 0.9. Nodes colored red for up-regulation, green for down-regulation. Red lines correspond to positive correlations, whereas bluelines correspond to negative correlations. | |
MSEA focused on meaningful signaling pathways that regulate metabolism
MSEA is often applied to quantitative metabonomic for identifying and interpreting changes in human or others mammalian pathway-associated metabolite concentrations.41 According to previous studies,32,42 we aimed to determine the dramatically affected pathways based on the detected metabolites. From metabolite sets enrichment profiles and pathway impact illustrations, we focused on 10 significant pathways in urine and feces samples. Notably, they shared 5 pathways in some extent, although metabolites involved in the shared pathways were not absolutely uniform (Fig. 7A and 8A, Tables 3 and 4). Additionally, 3 significant metabolic pathways (alanine, aspartate and glutamate metabolism, valine, leucine and isoleucine biosynthesis and glyoxylate and dicarboxylate metabolism) were found to be the most important metabolic pathways for PP treatment in urine samples (Fig. 7B–D and Table 3). Meanwhile, in feces samples collected after treating PP, 3 significant metabolic pathways (glycine, serine and threonine metabolism, pyruvate metabolism and valine, leucine and isoleucine biosynthesis) were also discovered to be the most significant metabolic pathways (Fig. 8B–D and Table 4).
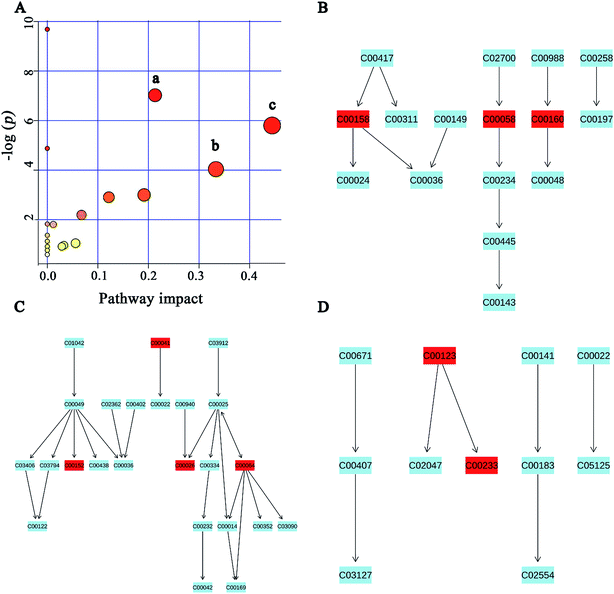 |
| Fig. 7 Potential pathways for PP supplementation in urine extracts identified by using MSEA pathway analysis. (A) (a) Alanine, aspartate and glutamate metabolism, (b) valine, leucine and isoleucine biosynthesis, (c) glyoxylate and dicarboxylate metabolism. (B) Alanine, aspartate and glutamate metabolism. (C) Valine, leucine and isoleucine biosynthesis. (D) Glyoxylate and dicarboxylate metabolism. | |
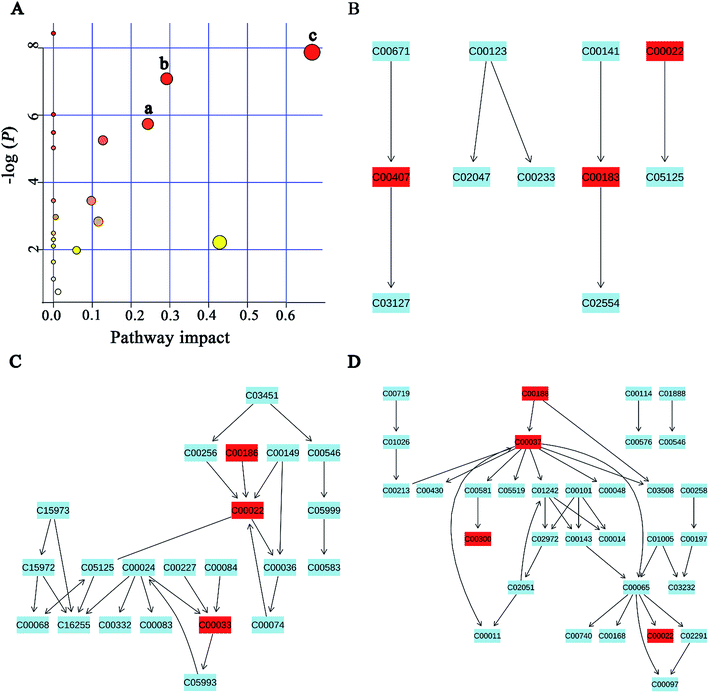 |
| Fig. 8 Potential pathways for PP supplementation in feces extracts identified by using MSEA pathway analysis. (A) (a) Glycine, serine and threonine metabolism, (b) pyruvate metabolism, (c) valine, leucine and isoleucine biosynthesis. (B) Glycine, serine and threonine metabolism. (C) Pyruvate metabolism. (D) Valine, leucine and isoleucine biosynthesis. | |
Table 3 Meaningful metabolic pathways of urine from MSEA
Pathway name |
Match status |
P |
FDR |
Impact |
Glyoxylate and dicarboxylate metabolism |
3/16 |
0.0030212 |
0.081572 |
0.44445 |
Alanine, aspartate and glutamate metabolism |
4/24 |
8.93 × 10−4 |
0.036147 |
0.21308 |
Valine, leucine and isoleucine biosynthesis |
2/11 |
0.017656 |
0.28603 |
0.33333 |
Arginine and proline metabolism |
3/44 |
0.049895 |
0.63457 |
0.19136 |
Citrate cycle (TCA cycle) |
2/20 |
0.054839 |
0.63457 |
0.12155 |
Glycerophospholipid metabolism |
2/30 |
0.1117 |
1 |
0.06759 |
Pyruvate metabolism |
1/22 |
1.0493 |
1 |
0.05583 |
Purine metabolism |
2/68 |
0.96713 |
1 |
0.0329 |
Glycolysis or gluconeogenesis |
1/26 |
0.91722 |
1 |
0.02862 |
Valine, leucine and isoleucine degradation |
2/38 |
1.8045 |
1 |
0.0119 |
Table 4 Meaningful metabolic pathways of feces from MSEA
Pathway name |
Match status |
P |
FDR |
Impact |
Valine, leucine and isoleucine biosynthesis |
3/11 |
3.81 × 10−4 |
0.015448 |
0.66666 |
Pyruvate metabolism |
3/22 |
0.0032192 |
0.052152 |
0.42857 |
Glycine, serine and threonine metabolism |
4/32 |
8.40 × 10−4 |
0.022667 |
0.29197 |
Taurine and hypotaurine metabolism |
1/8 |
0.0032192 |
0.58775 |
0.24337 |
Glycolysis or gluconeogenesis |
3/26 |
0.0052399 |
0.060633 |
0.12753 |
Cysteine and methionine metabolism |
3/28 |
0.058559 |
0.39527 |
0.11567 |
Citrate cycle (TCA cycle) |
2/20 |
0.031505 |
0.25519 |
0.0975 |
Primary bile acid biosynthesis |
2/46 |
0.13778 |
0.65648 |
0.05952 |
Arginine and proline metabolism |
1/44 |
0.47385 |
1 |
0.01198 |
Glutathione metabolism |
2/26 |
0.05121 |
0.37709 |
0.00573 |
Microbial metabolic functions associated with PP treatment in weaned rats
We validated the inferred functions using shotgun metagenomic sequencing based on a subset of 6 feces samples from different groups (Fig. 9). 14 metabolic modules retained the same predicted from 1H-NMR. Briefly, genes involved in the basic metabolism (TCA cycle, amino acid metabolism, SCFAs metabolism, nucleic acid metabolism and lipid metabolism).
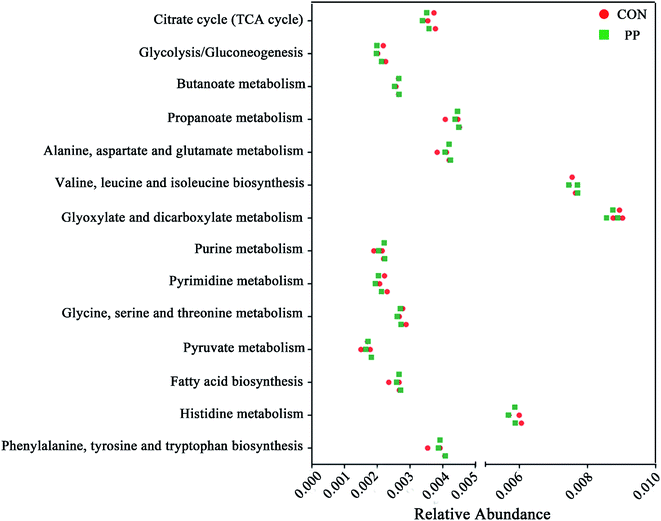 |
| Fig. 9 Shotgun sequencing validates the predicted microbial metabolic trends in a small subset of feces samples from the different two groups (CON group and PP group). | |
Discussion
This study provided an integrated comprehensive metabonomic approach to evaluate the treating effects of PP and explore the underlying mechanisms. To investigate the variation of endogenous metabolites in PP treated rats, 1H-NMR-based metabolomics combined with multivariate statistical analysis was performed to explore potential biomarkers and the affected metabolic pathways. The results showed a series of metabolite changes in urine and feces and revealed that supplementation PP could influence the metabolic pathways (Fig. 10).
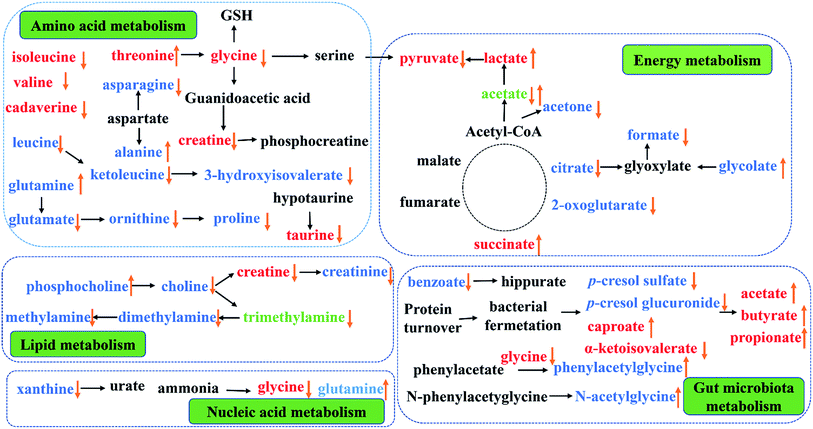 |
| Fig. 10 Metabolic pathways altered by PP supplementation. (↑) Up-regulated; (↓) down-regulated; red colour, feces; blue colour, urine; green, feces and urine. | |
Energy metabolism
In TCA cycle, a most effective and primary source of energy supply in mammals, succinate is one of the key intermediates.43 Compared with CON group, significant alterations of TCA cycle intermediates in PP group and the increase of succinate in feces, mean an activation of succinate thiokinase that can improve the efficiency of energy production. As critical intermediates in the TCA cycle, urinary citrate and 2-oxoglutarate continuously decreased in PP rats and further resulted in an increased of the energy-related metabolites in serum, indicating an increase energy metabolism. Meanwhile, glycolate, as a vital helper involved in TCA cycle,44,45 elevated while formate reduced in urine, suggesting that the glyoxylate/dicarboxylate metabolism was promoted by PP and TCA cycle was enhanced from an indirect way.
Glycolysis, another energy supplying pathway that can convert pyruvate into lactate and alanine,46,47 were significantly reduced in CON group. PP treatment could decrease pyruvate and increase lactate, indicating that glycolysis might be well activated in the weaned stage. Furthermore, as a metabolite involved in energy supply, acetone significantly increased in the urine extracts of CON group when it sharply decreased in PP group. The metabolites related with the above disordered energy metabolism were significantly reverted by PP supplementation, suggesting that PP treatment is capable of promoting the energy metabolism. In general, our results elaborated that PP was armed with a series of weapons elevating the levels of energy metabolism: regulating TCA cycle and glycolysis or gluconeogenesis metabolism.
Amino acid metabolism
Amino acid works as crucial basic substrates and regulatory factors in many metabolic pathways.48,49 Fecal branched chain amino acids (BCAAs, leucine, isoleucine and valine) in PP group was significant lower than that in CON group. In addition, the glycine, serine and threonine metabolism had been highly activated in PP group. Glycine which is a nonessential amino acid, can be endogenous synthesized from choline in mammals.50 Glycine can be converted to serine by tetrahydrofolic acid and further metabolized into pyruvate, and glycine is also degraded into creatine through betaine and N,N-dimethylglycine.51 The decreased level of glycine and creatine decreased were probably because of the increased lactate. Additionally, glycine and serine can be converted into a carbon unit, which is essential for the synthesis of purine and pyridine. The results have proved that the glycine, serine and threonine metabolism could provide indispensable substrates for basic metabolism of growth.
Taurine, a multifunctional metabolite, plays an important role in neuronal development and modulation, anti-oxidative stress and cell infiltration.52 The significant decline of taurine in feces may be associated with the effective reabsorption in small intestine53 and/or the taurine-to-sulfate transformation by gut microbiota.54 It is essential to note that taurine in small intestine can be used as an absorption promoter for some medications and can prevent mucosal damage in the gastrointestinal tract.55 It is also reported that poor intestinal absorption of taurine is linked to diabetes and results in retinal, neural, cardiac and immune dysfunctions,56 reminding us that the decreased taurine level in PP treatment group may be associated with the growth effect. The bioavailability of arginine can be used as a biomarker to assess the risk of metabolic syndrome.57 Arginine can transform into urea and ornithine, and ornithine can be further metabolized to proline by ornithine aminotransferase.58,59 The levels of ornithine and proline in urine decreased, meaning an improving bioavailability of arginine. The results suggest that PP treatment promoted the arginine and proline metabolism and prevented the metabolic syndrome. Glutamine, another nonessential amino acid and a precursor of glutamate, has been shown to promote beta-cells to secret insulin under the stimulation of glucose.60 Glutamate, which can be converted into gamma amino butyric acid (GABA), is of vital importance in the decomposition of metabolites. In addition, glutamate can also be converted into ornithine. On the other hand, aspartate can be transformed into asparagine and alanine. A study has reported that the level of alanine has a positive association with obesity, and the level of asparagine has a negative association with obesity.61 In our study, the level of alanine significant increased and the level of asparagine obviously decreased in urine. Therefore, we speculate that alanine, aspartate and glutamate metabolism could prevent the metabolic syndrome.
Choline metabolism
It is common knowledge that choline is an essential nutrient, but the choline from de novo biosynthesis is insufficient to meet physiological demands. Mammals can use dietary choline to biosynthesize PC, and choline can also be metabolized into TMA by gut microbiota and then further degraded to TMAO or DMA. On the other hand, host metabolize choline to glycine via betaine, and then decomposes it into MA or creatine, which is ultimately excreted as creatinine.51 Decreased excretion of creatinine may be caused by the enhancement of bacterial metabolism, with bacteria isolated in human feces possessing the metabolic capacity to degrade creatinine.62,63 In fact, as a primary metabolite of dietary choline degraded by the microorganisms, TMA has been reabsorbed into blood by intestinal villi and then enters systemic circulation via the hepatic portal vein.64 Such TMA accumulation is toxic to mammalian liver, causing hepatic steatosis as reported previously in mice.65 Our result showed that the level of choline, TMA, DMA, MA and creatinine significant decreased in urine extract, indicating the activation of choline metabolism and no damage to the liver of rats.
Gut microbiota metabolism
It is well known that short chain fatty acids (SCFAs), such as acetate, butyrate and propionate, are produced from fermentation of carbohydrates, including fiber, cellulose and starches, by the gut microbiota. These SCFAs are critical energy sources for the colonic epithelium in the animal intestines and regulating agents for various digestive functions. SCFAs also have immunomodulatory effects, inhibiting inflammatory cytokine secretion in culture epithelial cells, and serve as fuel for active ion transportation in the large intestines.66 Here, compared with CON group, we observed obvious increased levels of SCFAs in feces extracts in PP group. The elevated levels of SCFAs in the feces of rats fed with PP demonstrated that PP could modulate gut microbiota which can ferment dietary fiber, and bring immunomodulatory effects. We observed a higher urinary level of 2-ketoisovalerat, which is a main metabolite involved in biochemical pathway of glycolysis or gluconeogenesis. Glycolysis is the process of converting glucose into pyruvate and producing small amounts of ATP and NADH. 2-Ketoisovalerate is likely to increase anaerobic glycolysis, associating with gut microbiota in PP treatment group.
We further observed that the levels of urinary microbial-mammalian co-metabolites had significantly change, including urinary PAG, N-AG, p-CG and p-CS. PAG is typically produced from hepatic glycine-conjugation of phenylacetate, which is a catabolic product of phenylalanine and dietary polyphenols from gut microbiota.61 The p-cresol is a metabolite of 4-cresol glucuronide and undergoes glucuronidation with UDP-glucuronosyltransferase in the mammalian liver prior to urinary excretion.67,68 p-CG is a glucuronide derivative of p-cresol, a metabolic product of Clostridium difficile in the large intestine.69 The previous studies suggested that the upregulation of two gut microbial metabolites in the urine, PAG and p-CG, were associated with the dysbiosis of the intestinal microbiota.70 In line with the previous studies, the observed decrease in the output of p-CG indicates a balanced intestinal microbiota. However, the level of PAG significant increases in urine when treated with PP, and further research is needed to the specific situation. The bacterial fermentation of tyrosine and phenylalanine can improve the synthesis of p-cresol and finally p-CS.71 Evidences have proved that p-CS is a kind of uremic toxin, which not only involved in the etiology of uremic syndrome, but also have the potential to cause cardiovascular disease and renal osteodystrophy.72 These results suggested that PP treatment could regulate the host metabolism, which may be related with gut microbiota.
Nucleic acid metabolism
Xanthine is a product of purine catabolism, catalyzed by xanthine oxidase. Previous studies had reported the activity of xanthine oxidase raised in many diseases condition, but this study showed a decrease level of xanthine in urine. Thus, the activity of xanthine oxidase was changed by PP treatment. Glycine is produced from purine metabolism by degradation of hypoxanthine.73 The primary functions of glutamine are to store nitrogen in the muscle and transport it between organs. Glutamine is an essential nitrogen donor in de novo synthesis of both purine and pyrimidines.74 In our study, the level of glycine in feces and the level of glutamine in urine were both changes in PP treatment group when compared with CON group. The results suggested that PP supplementation could regulate the nucleic acid metabolism.
Conclusions
The study has shown that probiotics and prebiotics (PP) intake regulated metabolite compositional signatures in multiple biological matrices including urine and feces. To our knowledge, this is the first work to investigate the metabolic effects of probiotics combined with prebiotics in weaned rats. 1H-NMR-based metabonomic has been proven to be an effective technique to investigate the biochemical effects of the dietary PP. Studies demonstrated that metabolic responses of weaned rats to the dietary PP supplementation involved several metabolic pathways, such as energy metabolism, amino acid metabolism, choline metabolism, gut microbiota metabolism as well as nucleic acid metabolism. In conclusion, 1H-NMR-based metabonomic provided a direct viewing and useful method for monitoring the metabolic changes. These findings not only offered important information on the functions of metabolites in the development of weaned rats, but also suggested necessity for further exploration of the detailed metabolisms related to weaned rat development and growth. This work also suggests that the contributions of dietary PP towards metabolism changes in weaned rats are also an interesting topic to be explored much further and as a result, PP could be used as a functional food component.
Conflicts of interest
The authors declare no competing financial interests.
Acknowledgements
We thank the participants for their collaboration in the study. This work was supported by the National Natural Science Foundation of China (No. 81473319 and No. 81473540), the Key projects of Guangdong Natural Science Foundation (No. 2017A030311022), the Guangdong Science and Technology Program (No. 2015A030401031, 2014A020209026), the Guangdong Natural Science Foundation (No. 2015A030313123), and the Zhongshan Science and Technology Program (No. 2016C1015).
References
- G. Wu, F. W. Bazer, T. A. Davis, S. W. Kim, P. Li, R. J. Marc, S. M. Carey, S. B. Smith, T. E. Spencer and Y. Yin, Amino Acids, 2009, 37, 153–168 CrossRef CAS PubMed.
- B. Tan, X. G. Li, X. Kong, R. Huang, Z. Ruan, K. Yao, Z. Deng, M. Xie, I. Shinzato, Y. Yin and G. Wu, Amino Acids, 2009, 37, 323–331 CrossRef CAS PubMed.
- Y. Crispel, O. Katz, D. Ben-Yosef and Z. Hochberg, BMC Med., 2013, 11, 114 CrossRef PubMed.
- X. F. Kong, Y. L. Yin, Q. H. He, F. G. Yin, H. J. Liu, T. J. Li, R. L. Huang, M. M. Geng, Z. Ruan, Z. Y. Deng, M. Y. Xie and G. Wu, Amino Acids, 2009, 37, 573–582 CrossRef CAS PubMed.
- A. J. Moeser, K. A. Ryan, P. K. Niqhot and A. T. Blikslager, Am. J. Physiol.: Gastrointest. Liver Physiol., 2007, 293, G413–G421 CrossRef CAS PubMed.
- F. Smith, J. E. Clark, B. L. Overman, C. C. Tozel, J. H. Huang, J. E. Rivier, A. T. Blikslager and A. J. Moeser, Am. J. Physiol.: Gastrointest. Liver Physiol., 2010, 298, G352–G363 CrossRef CAS PubMed.
- J. P. Lalles, P. Bosi, H. Smidt and C. R. Stokes, Livest. Sci., 2007, 108, 82–93 CrossRef.
- J. R. Pluske, D. J. Hampson and I. H. Williams, Livest. Prod. Sci., 1997, 51, 215–236 CrossRef.
- Q. He, H. Tang, P. Ren, X. Kong, G. Wu, Y. Yin and Y. Wang, J. Proteome Res., 2011, 10, 5214–5221 CrossRef CAS PubMed.
- J. Wang, L. Chen, P. Li, X. Li, H. Zhou, F. Wang, D. Li, Y. Yin and G. Wu, J. Nutr., 2008, 138, 1025–1032 CrossRef CAS PubMed.
- M. A. O'Sullivan and C. A. O'Morain, Dig. Liver Dis., 2000, 32, 294–301 CrossRef.
- S. C. Sindhu and N. Khetarpaul, Nutr. Health, 2002, 16, 173–181 CrossRef CAS PubMed.
- E. Pitouni, V. Alexiou, V. Saridakis, G. Peppas and M. E. Falagas, Eur. J. Clin. Pharmacol., 2009, 65, 561–570 CrossRef PubMed.
- R. Menningen and M. Bruewer, Ann. N. Y. Acad. Sci., 2009, 1165, 183–189 CrossRef PubMed.
- L. B. Bindels, N. M. Delzenne, P. D. Cani and J. Walter, Nat. Rev. Gastroenterol. Hepatol., 2015, 12, 303–310 CrossRef CAS PubMed.
- M. R. Bomhof, D. C. Saha, D. T. Reid, H. A. Paul and R. A. Reimer, Obesity, 2014, 22, 763–771 CrossRef CAS PubMed.
- G. D. Wu, C. Compher, E. Z. Chen, S. A. Smith, R. D. Shah, K. Bittinger, C. Chehoud, L. G. Albenberg, L. Nessel, E. Gilroy, J. Star, A. M. Weljie, H. J. Flint, D. C. Metz, M. J. Bennett, H. Li, F. D. Bushman and J. D. Lewis, Gut, 2016, 65, 63–72 CrossRef CAS PubMed.
- O. A. Eseyin, M. A. Scattar, H. A. Rathore, A. Ahmad, S. Afzal, M. Lazhari, F. Ahmad and S. Akhtar, Annu. Res. Rev. Biol., 2014, 4, 1813–1826 CrossRef.
- S. Q. Zheng, F. Jiang, H. Y. Gao and J. G. Zheng, Phytother. Res., 2010, 24, 622–624 CAS.
- G. R. Gibson and M. B. Roberfroid, J. Nutr., 1995, 125, 1401–1412 CAS.
- J. Wu, Y. An, J. Yao, Y. Wang and H. Tang, Analyst, 2010, 135, 1023–1030 RSC.
- F. Dong, L. Zhang, F. Hao, H. Tang and Y. Wang, J. Proteome Res., 2013, 12, 2958–2966 CrossRef CAS PubMed.
- O. Beckonert, H. C. Keun, T. M. Ebbels, J. Bundy, E. Holmes, J. C. Lindon and J. K. Nicholson, Nat. Protoc., 2007, 2, 2692–2703 CrossRef CAS PubMed.
- J. F. Wu, E. Holmes, J. Xue, S. H. Xiao, B. H. Singer, H. R. Tang, J. Utzinger and Y. L. Wang, Int. J. Parasitol., 2010, 40, 695–703 CrossRef CAS PubMed.
- C. A. Merrield, M. Lewis, S. P. Claus, O. P. Beckonert, M. E. Dumas, S. Duncker, S. Kochhar, S. Rezzi, J. C. Lindon, M. Bailey, E. Holmes and J. K. Nicholson, Mol. BioSyst., 2011, 7, 2577–2588 RSC.
- G. le Gall, S. O. Noor, K. Ridgway, L. Scovell, C. Jamieson, I. T. Johnson, I. J. Colquhoun, E. K. Kemsley and A. Narbad, J. Proteome Res., 2011, 10, 4208–4218 CrossRef CAS PubMed.
- F. Westad, N. K. Afseth and R. Bro, Anal. Chim. Acta, 2007, 595, 323–327 CrossRef CAS PubMed.
- J. A. Westerhuis, H. C. J. Hoefsloot, S. Smit, D. J. Vis, A. K. Smilde, E. J. J. van Velzen, J. P. M. van Duijinhoven and F. A. van Dorsten, Metabolomics, 2008, 4, 81–89 CrossRef CAS.
- S. Wold, E. Johansson and M. Cocchi, PLS-partial least squares to latent structures, in 3D QSAR in drug design, ed. H. Kubinyi, ESCOM Science Publishers, Leiden, The Netherlands, 1993, pp. 523–550 Search PubMed.
- L. Eriksson, J. Trygg and S. Wold, J. Chemom., 2008, 22, 594–600 CrossRef CAS.
- O. Cloarec, M. E. Dumas, A. Craiq, R. H. Barton, J. Trygg, J. Hudson, C. Blancher, D. Gauquier, J. C. Lindon, E. Holmes and J. Nicholson, Anal. Chem., 2005, 77, 1282–1289 CrossRef CAS PubMed.
- M. E. Dumas, J. Kinross and J. K. Nicholson, Gastroenterology, 2014, 146, 46–62 CrossRef PubMed.
- N. Qin, F. Yang, A. Li, E. Prifti, Y. Chen, L. Shao, J. Guo, E. Le Chatelier, J. Yao, L. Wu, J. Zhou, S. Ni, L. Liu, N. Pons, J. M. Batto, S. P. Kennedy, P. Leonard, C. Yuan, W. Ding, Y. Chen, X. Hu, B. Zheng, G. Qian, W. Xu, S. D. Ehrlich, S. Zheng and L. Li, Nature, 2014, 513, 59–64 CrossRef CAS PubMed.
- J. Qin, R. Li, J. Raes, M. Arumugam, K. S. Burgdorf, C. Manichanh, T. Nielsen, N. Pons, F. Levenez, T. Yamada, D. R. Mende, J. Li, J. Xu, S. Li, D. Li, J. Cao, B. Wang, H. Liang, H. Zheng, Y. Xie, J. Tao, P. Lepage, M. Bertalan, J. M. Batto, T. Hansen, D. Le Paslier, A. Linneberg, H. B. Nielsen, E. Pelletier, P. Renault, T. Sicheritz-Ponten, K. Turner, H. Zhu, C. Yu, S. Li, M. Jian, Y. Zhou, Y. Li, X. Zhang, S. Li, N. Qin, H. Yang, J. Wang, S. Brunak, J. Dore, F. Guarner, K. Kristiansen, O. Pedersen, J. Parkhill, J. Weissenbach, C. MetaHIT, P. Bork, S. D. Ehrlich and J. Wang, Nature, 2010, 464, 59–65 CrossRef CAS PubMed.
- J. Qin, Y. Li, Z. Cai, S. Li, J. Zhu, F. Zhang, S. Liang, W. Zhang, Y. Yuan, D. Shen, Y. Peng, D. Zhang, Z. Jie, W. Wu, Y. Qin, W. Xue, J. Li, L. Han, D. Lu, P. Wu, Y. Dai, X. Sun, Z. Li, A. Tang, S. Zhong, X. Li, W. Chen, R. Xu, M. Wang, Q. Feng, M. Gong, J. Yu, Y. Zhang, T. Hansen, G. Sanchez, J. Raes, G. Falony, S. Okuda, M. Almeida, E. LeChatelier, P. Renault, N. Pons, J. M. Batto, Z. Zhang, H. Chen, R. Yang, W. Zheng, S. Li, H. Yang, J. Wang, S. D. Ehrlich, R. Nielsen, O. Pedersen, K. Kristiansen and J. Wang, Nature, 2012, 490, 55–60 CrossRef CAS PubMed.
- S. F. Altschul, W. Gish, W. Miller, E. W. Myers and D. J. Lipman, J. Mol. Biol., 1990, 215, 403–410 CrossRef CAS PubMed.
- M. Kanehisa, M. Araki, S. Goto, M. Hattori, M. Hirakawa, M. Itoh, T. Katayama, S. Tawashima, S. Okuda, T. Tokimatsu and Y. Yamanishi, Nucleic Acids Res., 2008, 36, D480–D484 CrossRef CAS PubMed.
- F. Bäckhed, J. Roswall, Y. Peng, Q. Feng, H. Jia, P. Kovatcheva-Datchary, Y. Li, Y. Xia, H. Xie, H. Zhong, M. T. Khan, J. Zhang, J. Li, L. Xiao, J. Al-Aama, D. Zhang, Y. S. Lee, D. Kotowska, C. Colding, V. Tremaroli, Y. Yin, S. Berqman, X. Xu, X. Xu, L. Madsen, K. Kristiansen, J. Dahlqren and J. Wang, Cell Host Microbe, 2015, 17, 852 Search PubMed.
- Y. Zhao, J. Wu, J. V. Li, N. Y. Zhou, H. Tang and Y. Wang, J. Proteome Res., 2013, 12, 2987–2999 CrossRef CAS PubMed.
- X. Shi, C. Xiao, Y. Wang and H. Tang, J. Proteome Res., 2013, 12, 991–1006 CrossRef CAS PubMed.
- J. Xia and D. S. Wishart, Nucleic Acids Res., 2010, 38(suppl), W71–W77 CrossRef CAS PubMed.
- J. Xia and D. S. Wishart, Nat. Protoc., 2011, 6, 743–760 CrossRef CAS PubMed.
- A. Pechlivanis, K. G. Papaioannou, G. Tsalis, P. Saraslanidis, V. Mouqios and G. A. Theodoridis, J. Proteome Res., 2015, 14, 4610–4622 CrossRef CAS PubMed.
- S. Zhang and D. A. Bryant, J. Biol. Chem., 2015, 290, 14019–14030 CrossRef CAS PubMed.
- N. O. van Gylswyk, Int. J. Syst. Bacteriol., 1995, 45, 297–300 CrossRef CAS PubMed.
- B. Casserly, G. S. Phillips, C. Schorr, R. P. Dellinger, S. R. Townsend, T. M. Osborn, K. Reinhart, N. Selvakumar and M. M. Levy, Crit. Care Med., 2015, 43, 567–573 CrossRef CAS PubMed.
- M. Karbowski, C. Kurono, M. Wozniak, M. Ostrowski, M. Teranishi, Y. Nishizawa, J. Usukura, T. Soji and T. Wakabayashi, Free Radical Biol. Med, 1999, 26, 396–409 CrossRef CAS PubMed.
- J. T. Brosnan, J. Nutr., 2003, 133(6, suppl 1), 2068S–2072S CrossRef CAS PubMed.
- H. N. Christensen, Physiol. Rev., 1982, 62, 1193–1233 CrossRef CAS PubMed.
- W. Wang, Z. Wu, Z. Dai, Y. Yang, J. Wang and G. Wu, Amino Acids, 2013, 45, 463–477 CrossRef CAS PubMed.
- S. S. Heinzmann, C. A. Merrield, S. Rezzi, S. Kochhar, J. C. Lindon, E. Holmes and J. K. Nicholson, J. Proteome Res., 2012, 11, 643–655 CrossRef CAS PubMed.
- R. J. Huxtable, Physiol. Rev., 1992, 72, 101–163 CrossRef CAS PubMed.
- K. S. Kim, Arch. Pharmacal Res., 1983, 6, 109–114 CrossRef CAS.
- E. Schram and R. Crokaert, Biochim. Biophys. Acta, 1957, 26, 300–308 CrossRef CAS.
- T. Kimura, K. S. Kim and H. Sezaki, J. Pharmacobio-Dyn., 1981, 4, 35–41 CrossRef CAS PubMed.
- M. Merheb, R. T. Daher, M. Nasrallah, R. Sabra, F. N. Ziyadeh and K. Barada, Diabetes Care, 2007, 30, 2652–2654 CrossRef PubMed.
- J. Moon, O. Y. Kim, G. Jo and M. J. Shin, Nutrients, 2017, 9, E740 CrossRef PubMed.
- R. B. Caldwell, H. A. Toque, S. P. Narayanan and R. W. Caldwell, Trends Pharmacol. Sci., 2015, 36, 395–405 CrossRef CAS PubMed.
- G. M. Buqa, R. Singh, S. Pervin, N. E. Roqers, D. A. Schmitz, C. P. Jenkinson, S. D. Cederbaum and L. J. Iqnarro, Am. J. Physiol., 1996, 271, H1988–H1998 CrossRef PubMed.
- Y. Tanizawa, K. Nakai, T. Sasaki, T. Anno, Y. Ohta, H. Inoue, K. Matsuo, M. Koga, S. Furukawa and Y. Oka, Diabetes, 2002, 51, 712–717 CrossRef CAS PubMed.
- N. Geidenstam, M. Maqnusson, A. P. H. Danielsson, R. E. Gerszten, T. J. Wang, L. E. Reinius, H. Mulder, O. Melander and M. Ridderstrale, Int. J. Endocrinol., 2017, 6490473 Search PubMed.
- S. R. Dunn, G. M. Gabuzda, K. P. Superdock, R. S. Kolecki, R. W. Schaedler and M. L. Simenhoff, Am. J. Kidney Dis., 1997, 29, 72–77 CrossRef CAS PubMed.
- M. Wyss and R. Kaddurah-Daouk, Physiol. Rev., 2000, 80, 1107–1213 CrossRef CAS PubMed.
- D. H. Lang, C. K. Yeung, R. M. Peter, C. Ibarra, R. Gasser, K. Itaqaki, R. M. Philpot and A. E. Rettie, Biochem. Pharmacol., 1998, 56, 1005–1012 CrossRef CAS PubMed.
- M. E. Dumas, R. H. Barton, A. Toye, O. Cloarec, C. Blancher, A. Rothwell, J. Fearnside, R. Tatoud, V. Blanc, J. C. Lindon, S. C. Mitchell, E. Holmes, M. I. McCarthy, J. Scott, D. Gauquier and J. K. Nicholson, Proc. Natl. Acad. Sci. U. S. A., 2006, 103, 12511–12516 CrossRef CAS PubMed.
- M. Asarat, T. Vasiljevic, V. Apostolopoulos and O. Donkor, Immunol. Invest., 2015, 44, 678–693 CrossRef CAS PubMed.
- J. R. Swann, K. M. Tuohy, P. Lindfors, D. T. Brown, G. R. Gibson, I. D. Wilson, J. Sidaway, J. K. Nicholson and E. Holmes, J. Proteome Res., 2011, 10, 3590–3603 CrossRef CAS PubMed.
- J. F. Wu, E. Holmes, J. Xue, S. H. Xiao, B. H. Singer, H. R. Tang, J. Utzinger and Y. L. Wang, Int. J. Parasitol., 2010, 40, 695–703 CrossRef CAS PubMed.
- M. C. Walsh, L. Brennan, E. Pujos-Guillot, J. L. Sebedio, A. Scalbert, A. Faqan, D. G. Hiqqins and M. J. Gibney, Am. J. Clin. Nutr., 2007, 86, 1687–1693 CAS.
- A. Kazuki, M. Shigenori, I. Misako, M. Hidemichi and H. Takao, Hypertens. Res., 2012, 35, 404–412 CrossRef PubMed.
- R. Poesen, K. Windey, E. Neven, D. Kuypers, V. De Preter, P. D'Haese, P. Evenepoel, K. Verbeke and B. Meijers, J. Am. Soc. Nephrol., 2015, 27, 1389–1399 CrossRef PubMed.
- J. A. Coladonato, J. Am. Soc. Nephrol., 2005,(suppl 2), S107–S114 CrossRef CAS PubMed.
- R. Abrams, E. Hammarsten and D. Shemin, J. Biol. Chem., 1948, 173, 429 CAS.
- R. J. DeBerardinis and T. Cheng, Oncogene, 2010, 29, 313–324 CrossRef CAS PubMed.
Footnote |
† Electronic supplementary information (ESI) available. See DOI: 10.1039/c7ra12067b |
|
This journal is © The Royal Society of Chemistry 2018 |
Click here to see how this site uses Cookies. View our privacy policy here.