DOI:
10.1039/C8MO00162F
(Research Article)
Mol. Omics, 2019,
15, 30-38
Lipidomics reveals insights on the biological effects of copper oxide nanoparticles in a human colon carcinoma cell line†
Received
17th July 2018
, Accepted 4th December 2018
First published on 5th December 2018
Abstract
Engineered nanomaterials have unique properties compared to their bulk counterparts. Copper oxide nanoparticles (CuO NPs) are one example of nanomaterials used in a wide range of consumer products due to their conductivity and biocidal properties. While CuO NPs can induce toxicity in various organisms, their interactions with different organisms and how they affect cellular homeostasis is yet to be fully understood. In this work, the toxicity of CuO NPs was evaluated in different human cell lines (colorectal carcinoma, cervical cancer, embryonic kidney, and lung fibroblast), showing a dose-dependent toxicity. An untargeted lipidomics approach using liquid chromatography-quadrupole time of flight mass spectrometry was employed in a human colon carcinoma cell line to investigate the impact of CuO NP exposure at the cellular level. A 24 h CuO NP exposure at 2.5 and 5 μg mL−1 resulted in upregulation of different metabolites: triacylglycerols, phosphatidylcholines, and ceramides accumulated. The most profound increase in a dose-dependent manner was observed in ceramides, specifically in C18:0, C18:1, and C22:0 species, with up to ∼10 fold accumulations. Further experiments suggested that activation of autophagy and oxidative stress could be responsible for the toxicity observed in these cell lines. Increases in the level of glutathione oxide (∼7 fold) also supported the activation of oxidative stress upon CuO NP treatment. Based on the changes in different metabolites induced by CuO NP exposure and previous studies from our laboratory, we propose that autophagy and oxidative stress could play a role in CuO NP-induced toxicity.
Introduction
Engineered nanomaterials are used in a wide range of applications in agriculture, therapeutics, environmental remediation, and consumer products, shaping concerns of possible negative impacts on the environment and human health during unintentional exposure.1–3 The effects of exposure to different nanoparticles (NPs) have been investigated in plants,4,5 bacteria,6 human cells,7 and animals,6 showing possible adverse effects in these organisms. Metal oxide NPs specifically, have been manufactured on a large scale for industrial and household applications.8 Copper oxide nanoparticles (CuO NPs) for instance, have been applied to different consumer products as conductors, antimicrobials, as well as in lubricants, plastics, cosmetics, and electronics.9 These NPs have also been used for the removal of pathogenic microorganisms in the tertiary stage of wastewater treatment plants,10 showing the versatility and novel properties of CuO NPs and their potential for a myriad of applications. CuO NPs are attractive to many industries, not only because of their physical properties but also due to the abundance and low cost of the metal and the synthetic ease in their preparation.11
Although copper is essential for living organisms, there is concern that the CuO NPs form can have adverse effects, and cause an influx of copper ions which could disrupt organismal homeostasis. Previous studies in mammalian cells have shown a dose-dependent CuO NP toxicity. However, the exposure time, concentration, and size of CuO NPs can also affect their toxicity. For example, the toxicity of rod-like 50–70 nm CuO NPs showed that the EC50 in different liver carcinoma cells varied from 25 to 85 μg mL−1 after a 24 h exposure.12 Another study in a lung carcinoma cell line showed the size-dependent toxicity of CuO NPs.13 These results suggest cell type- and size-dependent effects of CuO NPs, thus warrant the need to further study CuO NPs and their effects on mammalian cell lines.
Nanoparticles can have different physicochemical properties compared to their counterpart bulk materials.14 Bulk material toxicity assessments are based on chemical composition, dose, and exposure route, however for NPs additional factors need to be taken into consideration such as size, surface, self-assembly, quantum effect, and aggregation.15 Metabolomics could be instrumental in understanding the biochemical pathways that different NPs affect and, as such, present a new toxicological assessment tool in different cell lines.16 In this study, we aimed to leverage a metabolomics-based approach, focusing on the lipid components (i.e., lipidomics) to investigate the effects of CuO NPs in mammalian cells. In the past, we have identified several lipid markers of different cellular stress conditions, which provided key information on the biochemical pathways involved in cellular processes.4,17,18 Thus, we envisioned that the changes in lipid composition can shed light onto the mechanism of toxicity of these NPs and provide metabolite markers for CuO NP-induced toxicity, closing the knowledge gap on how these NPs interact with cells.
We first obtained dose–cell viability curves using different cancerous and non-cancerous cell lines and observed strong cell line-dependent effects. We then focused on human colon carcinoma cell lines (HCT-116) and conducted untargeted lipidomics to identify lipid species that were altered by NP treatment. We found changes in a number of lipid families, which associated with cell death and increased oxidative stress. Further investigations suggested the activation of autophagy upon CuO NP-treatment. Overall our results demonstrate that CuO NPs induce toxicity in mammalian cells most likely via the activation of autophagy and oxidative stress and that lipidomics can provide novel insights on their mechanism of toxicity.
Results and discussion
Solution behavior of CuO NPs
In this study, commercially available CuO NPs (<50 nm in size) were used. Previous studies suggested that the chemical environment of the metal oxide NPs has a large impact on their aggregation states.19–22 Specifically, previous studies with copper oxide nanoparticles have shown agglomerates of 172 and 214 nm in cell culture media.21 Thus, we first characterized the size of the particles in an aqueous medium, since in the environment nanoparticles will be present in aqueous solution. We reason that their behavior in aqueous environment will determine their interaction with cellular membranes and their uptake. CuO NPs (<50 nm) were analyzed using dynamic light scattering (DLS) as well as transmission electron microscopy (TEM) in cell culture medium. Fig. S1 (ESI†) shows the size distribution where NPs formed agglomerates with a mean size of ∼220 nm (within the most abundant peak >100 nm in size) by DLS (Fig. S1A, ESI†), which was further confirmed with TEM (Fig. S1B, ESI†). These results suggest that CuO NPs agglomerates in cell culture medium. Based on this size, it is possible that these NPs are up taken by active transport as opposed to passive diffusion.
CuO NPs induce toxicity in different cell lines
In order to assess the toxicity of CuO NPs, cell viability measurements were performed in tissue culture cell lines which included different cancerous and non-cancerous mammalian cell lines: HCT-116 (human colorectal carcinoma), HeLa (human cervical cancer), 293T HEK (human embryonic kidney), and MRC5 (human lung fibroblast). After 24 h of CuO NP exposure, all cell lines exhibited a dose-dependent response to CuO NPs at concentrations ranging from 0.125 to 25 μg mL−1 (Fig. 1A). LC50's obtained from these curves exhibit a strong dependence on the cell line used. MRC-5 and HeLa cell lines exhibited higher sensitivity to CuO NPs (LC50 ∼1.25 μg mL−1) compared to other cells lines, while HCT-116 exhibited the least sensitivity (LC50 ∼5 μg mL−1) among the cell lines tested followed by 293T HEK (LC50 ∼2.5 μg mL−1). CuO NP-exposed HCT-116 cells exhibited cell shrinkage and increased cellular blebbing, consistent with the decreased viability observed (Fig. 1B). In order to investigate the potential contribution of the metal ion released from NP to the toxicity of the nanomaterial, CuSO4 was used as a source of ionic copper and its toxicity was compared to that of CuO NPs. Notably, CuSO4 treatment did not show any toxicity up to a concentration of 25 μg mL−1 (Fig. S2, ESI†), strongly indicating that ionic copper released from the NPs did not cause the toxicity observed after 24 h treatment.
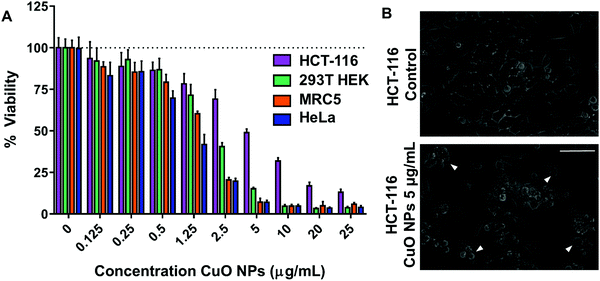 |
| Fig. 1 Effect of CuO NPs on cell viability. (A) Viability of HCT-116 (human colon carcinoma), 293T HEK (kidney), MRC-5 (lung), HeLa (human cervical cancer) cell lines that are exposed to CuO NPs. (B) Representative images from HCT-116 cells control (top) and exposed to 5 μg mL−1 CuO NPs (bottom) for 24 h. White arrowheads show cellular blebbing. The scale bar represents 75 μm. | |
Copper concentration in HCT-116 cells exposed to CuO NPs
Viability studies suggested that HCT-116 cells have the least sensitivity towards CuO NP treatment as compared to other cell lines tested in this study. Based on this, HCT-116 cell lines were further studied in order to investigate the NP uptake as determined from copper levels in cells. Two concentrations were chosen: 5 μg mL−1 (∼LC50 for HCT-116) and 2.5 μg mL−1 to determine the sub-LC50 effects of CuO NPs. After 24 h of exposure, 65Cu concentration was quantified in the control, CuO NP treated cells, and the culture growth medium via inductively coupled plasma mass spectrometry (ICP-MS). We use total copper levels in negative control and treated cells to estimate the copper oxide nanoparticle concentration in the cellular environment due to the challenges in quantifying the intact nanoparticles directly.23,24 Copper levels in the cellular growth media were 0.003 ± 0.001 μg mL−1 in the control medium, 2.353 ± 0.051 μg mL−1 in 2.5 μg mL−1 CuO NPs treatment media, and 4.882 ± 0.065 μg mL−1 in 5 μg mL−1 treatment media consistent with the concentrations that were introduced (Fig. 2A). In untreated cell lysates, copper was at 0.04 ± 0.004 ng mg−1 consistent with the estimated low cellular concentrations.25 All treatments showed increased copper levels as compared to the untreated cells with copper concentrations of 6.549 ± 1.212 ng mg−1 in 2.5 μg mL−1 CuO NP treated cells and 14.837 ± 0.544 ng mg−1 in 5 μg mL−1 CuO NP treated cells (Fig. 2B). Our results in HCT-116 cells and their growth medium show the low initial concentration of copper in control cells and growth medium and the increased levels after CuO NP treatment, showing a dose-dependent accumulation of total copper in cells.
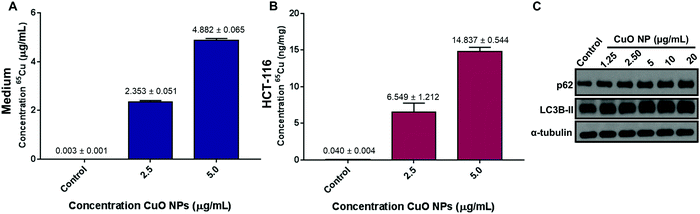 |
| Fig. 2 Total copper concentration in HCT-116 cells and growth medium as well as dose-dependent upregulation of p62 and LC3B-II. (A) Total copper concentration (65Cu) in growth cell medium (DMEM) in μg of Cu per mL of growth medium (mean ± std dev.). (B) Total copper concentration (65Cu) in HCT-116 cells in ng of Cu per mg of protein after 24 h treatment (mean ± std dev.). (C) p62, LC3B-II, and α-tubulin levels in control and 1.25, 2.5, 5, 10, and 20 μg mL−1 of CuO NP treatment after 24 h. | |
CuO NPs exposure upregulates LC3B-II and p62
Our results show that CuO NPs induce toxicity at low μg mL−1 concentrations in HCT-116 cells and the other cell lines tested with varying LC50's. As such, we aimed to investigate the biochemical pathways that are affected by CuO NP-treatment and could be responsible for the decreased viability we observed. Previous studies have linked increased autophagic activity to CuO NP-induced toxicity.26,27 Autophagy plays an important role as it is a pro-survival catabolic process and is also responsible for the modulation of cell death.28,29 A study in A549 (lung epithelial cells), showed that autophagy increased in cells after CuO NPs treatments as well as the increase in cell survival after the use of autophagy inhibitors.26 Another study in a breast cancer cell line showed that 30 nm CuO NPs induce autophagy showing upregulation of autophagy markers.27 Based on these studies, we first tested autophagy, as determined by the increase in p62 and LC3B-II levels, in CuO NP-treated HCT-116 cells. We observed a concentration-dependent increase in the levels of p62 and LC3B-II: 2.5 and 5 μg mL−1 treatments induced 89% and 116% increase in p62, respectively (Fig. S3A, ESI†). LC3B-II levels increased modestly, showing significant accumulations starting at 10 μg mL−1 (Fig. S3B, ESI†). Combined, these results suggest that autophagy was activated in HCT-116 cells upon CuO NP treatment.
Next, based on the cell shrinkage and increased cellular blebbing that was observed upon treatment (Fig. 1B), we investigated the apoptotic pathway upon CuO NP treatment. The levels of PARP, cleaved PARP, p21, and p16 were investigated (Fig. S4A, ESI†). We tested a wide range of concentrations of nanoparticles (1.25–20 μg mL−1) that induced 10–90% cell death (Fig. 1B). We did not observe cleaved PARP and p21 levels remained the same, strongly supporting the lack of apoptotic activity in CuO NP-treated cells. Finally, we used a pan caspase inhibitor zVAD-fmk in order to investigate whether the cell death observed was caspase dependent. Cell viability was not affected after the addition of zVAD-fmk, suggesting that the cell death observed upon CuO NP treatment was caspase-independent (Fig. S4B, ESI†). Overall, we infer from these results that apoptotic pathways are not the major contributors of cell death that CuO NPs induce.
Significant changes in lipid composition are observed after CuO NPs treatment
Lipids are a diverse class of metabolites with diverse structural and signaling functions.30,31 In the past, our laboratory has leveraged the changes in lipid composition to study the mechanism of toxicity of different small molecules. Building on this expertise, we first carried out untargeted lipidomics to determine the effects of CuO NPs in HCT-116 cells.
The overall workflow for the untargeted lipidomics analysis after CuO NPs exposure is shown in Fig. S5A (ESI†). Cells were treated with 2.5 and 5 μg mL−1 CuO NPs for 24 h. After normalizing different samples based on protein concentration, a biphasic extraction was performed to separate polar and nonpolar metabolites and lipidomics data was acquired using liquid chromatography quadrupole time of flight mass spectrometry (LC-QToF/MS). Fig. 3A shows the fold changes and p-values of the lipids detected. The x-axis is the log2 fold change of the relative abundance of each species in the CuO NP-treated cells versus the untreated control cells. The y-axis shows the −log10p-value. A dose-dependent response of HCT-116 to CuO NPs is observed, where more species showed significant accumulation (p < 0.01), indicated by the horizontal dashed line in Fig. 3A in 5 μg mL−1 (orange) treated cells relative to the 2.5 μg mL−1 (purple) treatment. This dataset was further analyzed to identify the most profound lipid changes, using ANOVA to determine the species that showed significant alterations at the 95% confidence level with a post hoc Tukey honest significant difference test.32 We then focused on the species that had a fold change of greater than 2 upon CuO NP treatment (Fig. S5B, ESI†).
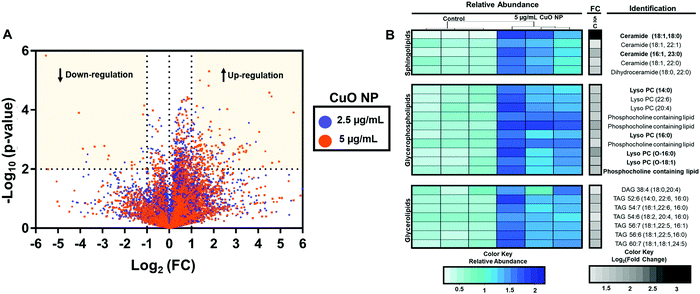 |
| Fig. 3 Untargeted lipidomics showing dose-dependent regulation of lipids after 24 h CuO NP treatment. (A) Volcano plot shows up- and down-regulation (yellow quadrants) of lipids after 24 h of 2.5 (purple) and 5 (orange) μg mL−1 CuO NP treatment. The log2 (fold change) of the relative abundance of each species in CuO NP treated and in negative control cells. The y-axis represents the −log10 (p-value) between treated and control samples. (B) The relative abundance (blue and green) and fold changes (gray and black) of 22 identified lipids via untargeted lipidomics are represented as a heatmap (n = 3). Species in bold were found to be upregulated in both treatments (2.5 and 5 μg mL−1). | |
Untargeted analysis workflow resulted in 7 and 24 species that showed significant alteration (p-value < 0.05 and fold change of greater than 2) after 2.5 and 5 μg mL−1 CuO NP treatment, respectively. Species changed in 2.5 μg L−1 treated cells were also altered in 5 μg mL−1 treatment but at a lower fold change, consistent with the dose-dependent effects of CuO NP treatment. Fig. 3B shows the relative abundance and fold change of the species upon CuO NP treatment (Table S1 and for detailed untargeted results including abundances from individual samples, m/z's and retention times, see Table S2, ESI†). We were able to positively identify 18 out of the 24 species. We predict that 4 of the 6 unidentified metabolites belong to the phosphatidylcholines and one belonged to the acylcarnitine family based on their fragmentation patterns (Table S2, ESI†). Modest accumulations (∼2–3 fold accumulation) of seven glycerolipids including six triacylglycerols (TAGs), and one diacylglycerol (DAG) were observed. Additionally, ten glycerophospholipids including (lyso)phosphatidylcholines ((L)PCs) were identified. Five sphingolipids accumulated, including four ceramides (CERs) and one dihydroceramide. Greater fold changes were observed for C18-Ceramide with 9-fold accumulations. Showing the overall changes with lipid species as a result of CuO NP exposure. Overall, these observations are consistent with previous targeted metabolomic studies with HepG2 cells that showed the increases in monoacylglycerols, phospholipids, sphingolipids, and lysolipids after CuO NP treatment.33
Interestingly, specific accumulation of polyunsaturated fatty acyl chains containing TAGs was observed. TAGs are mostly non-membrane associated and have been linked to increased oxidative stress observed during different cellular process31 and resulting from metal oxide nanoparticle toxicity, such as those observed in aluminum oxide,34 zinc oxide,35 and titanium oxide.36 We thus complemented our lipidomics approach with targeted analysis of various polar metabolites related to oxidative stress. The levels of glutathione (GSH) metabolites, nucleotides and amino acids are shown in Fig. 4. Most amino acids remain unchanged; however, leucine, phenylalanine, tyrosine, and tryptophan accumulated significantly after CuO NP treatment. This is consistent with a previous study that reported the accumulation of certain amino acids.21 More importantly, we detected changes in a GSH metabolite. GSH is a tripeptide and exists as a mixture of GSH and its oxidized form, glutathione disulfide. The balance of the mixture is essential for cell homeostasis and its imbalance has been associated with oxidative stress.37 In our analysis, GSH did not show significant changes after CuO NP treatment (p > 0.05). However, glutathione disulfide showed 3- and 7-fold accumulation in 2.5 and 5 μg mL−1 CuO NP treatment, respectively (p < 0.05). These results link polyunsaturated fatty acyl chain containing TAGs and glutathione disulfide metabolites to oxidative stress in a dose-dependent manner upon CuO NP exposure.
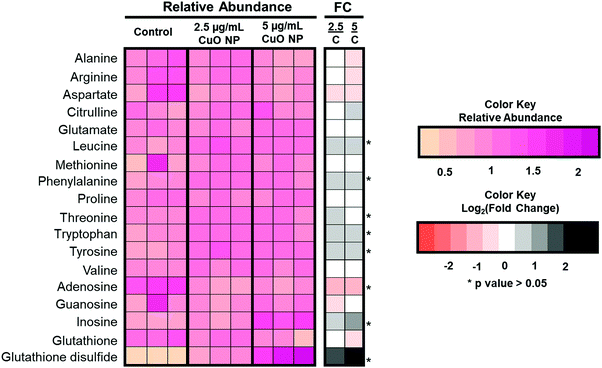 |
| Fig. 4 Targeted analysis of polar metabolites. Left heat map (pink) shows the relative abundance of targeted polar metabolites in control and 2.5 and 5 μg mL−1 CuO NP-treated HCT-116 cells (n = 3). Right heat map (orange and black) shows the log2 (fold change) comparing CuO NP-treated cells to the negative control. (*p-value < 0.05.) | |
The results of the untargeted lipidomics showed that a wide range of lipids changed after CuO NP treatment. Consequently, we performed targeted analysis of lipid families including fatty acids (FAs), CERs, phosphatidic acids (PAs), phosphatidylethanolamines (PEs), phosphatidylserines (PSs), phosphatidylglycerols (PGs), phosphatidylinositols (PIs), cholesterol esters (CEs), TAGs, DAGs, and PCs to obtain an overview of the lipidome in cells after CuO NP treatment (see Table S2, ESI†). Results showed FAs and PEs were the only lipid families which remained unchanged after CuO NP treatment (Fig. 5). The most profound increase in a dose-dependent manner in the targeted and untargeted analysis was ceramides with accumulations of up to ∼9 fold change, consistent with our observations from untargeted analysis (Fig. 5). Ceramides are sphingolipids, which mediate cell death and have been associated with autophagy induction.38,39 Our results show the accumulation of ceramides specifically C18:0, C18:1, and C22:0 species after CuO NP treatment. In parallel, targeted lipidomics shows a large increase in other membrane lipids including PCs, PSs, PIs, and PAs. These changes in membrane lipids suggest membrane remodeling that CuO NPs can cause in HCT-116 cells, which could be linked to the mechanism of their uptake.
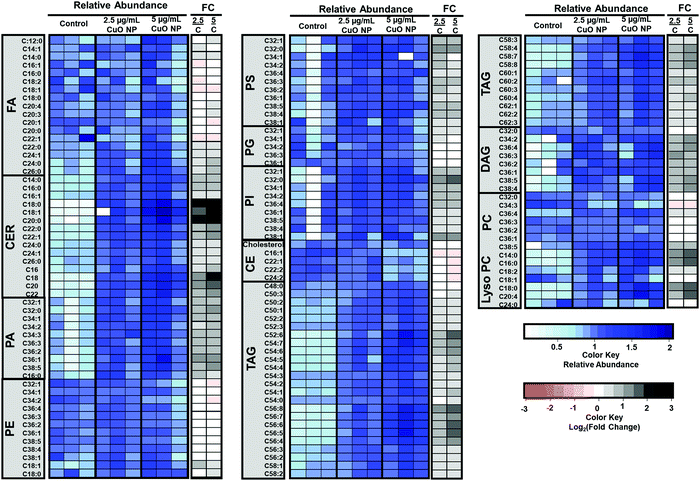 |
| Fig. 5 Targeted lipidomics shows the upregulation of several lipid classes after 24 h CuO NP treatment. The blue scale heat map shows the relative abundances of targeted lipids in the control and at 2.5 and 5 μg mL−1 CuO NP-treated cells (n = 3). Targeted lipids: fatty acids (FAs), ceramides (CERs), phosphatidic acids (PAs), phosphatidylethanolamines (PEs), phosphatidylserines (PSs), phosphatidylglycerols (PGs), phosphatidylinositols (PIs), cholesterol esters (CEs), triacylglycerols (TAGs), diacylglycerols (DAGs) and phosphatidylcholines (PCs). The white to black scale heat map shows the log2 (fold change) comparing CuO NP-treated cells to the negative control. | |
To obtain insights on how CuO NPs uptake might dictate its toxicity, we turned to 293T HEK cells, which were more sensitive to CuO NP treatment as compared to HCT-116. We measured the levels of 65Cu. We found that 293T HEK cells showed copper concentrations that are double the amount observed in HCT-116 (Fig. S6A and B, ESI†). The levels of glutathione disulfide which showed a 9- and 16-fold accumulation in 2.5 and 5 μg mL−1 CuO NP treated HEK 293T respectively, double the accumulation observed in HCT-116 cells. We also investigated the levels of LC3B-II and p62 in CuO NP-treated 293T HEK cells, which exhibited a concentration dependent increase (Fig. S6C–E, ESI†). Based on these results, we infer that the mechanism of toxicity of the NPs used in this study is cell line independent. The positive correlation between higher copper levels, oxidative stress, and cell death in 293T HEK cells when compared to HCT-116 cells indicate uptake plays a role in the differential toxicity of CuO NPs towards different cell lines.
Conclusions
In this study, we utilized a lipidomics approach to determine the effects of CuO NPs in a human colon carcinoma cell line (HCT-116) because we envisioned that the changes in lipid composition can shed light onto the mechanism of toxicity of these NPs and provide metabolite markers for CuO NP-induced toxicity. First, dose–viability curves of CuO NP in different mammalian cell lines show the cell line- and dose-dependent response to CuO NPs. We propose that this differential response could be due to the differences in CuO NP cell uptake as determined by cellular copper concentrations. In addition, untargeted lipidomics in HCT-116 exposed to two different CuO NP concentrations showed the changes in metabolite composition highlighting accumulation of polyunsaturated acyl chain containing TAGs, ceramides, and lyso PCs. Combined with our investigations at the protein level, these results strongly suggest that CuO NP treatment induced autophagy and cell death via mechanisms independent of caspase activity. These results provide a proof of concept that the mode of action of CuO NPs is complex and seems to be diverse for different cell lines used. Exciting works remain to be completed to elucidate the mechanism of toxicity and uptake of CuO NPs in mammalian cells.
Experimental
Materials
Human colon carcinoma (HCT-116), 293T HEK (kidney human embryonic), MRC5 (lung human fibroblast), HeLa was acquired from the American Type Culture Collection (ATCC). Dulbecco's Modification of Eagle's Medium (DMEM), fetal bovine serum (FBS), penicillin, streptomycin, and trypsin were obtained from Corning (Manassas, VA). 3-(4,5-Dimethylthiazol-2-yl)-2,5-diphenyltetrazolium bromide (MTT), α-tubulin antibody, dimethyl sulfoxide (DMSO) and CuO NPs (>50 nm), formic acid (88%), and ammonium hydroxide were obtained from Sigma-Aldrich (Saint Louis, MO). Poly ADP ribose polymerase (PARP) was purchased from Cell Signaling (Danvers, MA) and protease inhibitor cocktail was obtained from Roche Diagnostic (Indianapolis, IN). A Milli-Q™ water purification system was used to achieve 18.2 MΩ water. Methanol, isopropanol, and chloroform (Optima LC/MS grade) were purchased from EMD Millipore (Billerica, MA). Concentrated nitric acid was obtained from BDH (Radnor, PA), and 30% hydrogen peroxide was obtained from Fisher Scientific (Waltham, MA).
Nanoparticle characterization
CuO NPs were suspended in ethanol and sonicated for 30 min before usage. CuO NPs were characterized at 5 μg mL−1 in DMEM media by dynamic light scattering (DLS) using a Malvern Zetasizer Nano-ZS90 (Malvern Instruments Ltd, Worcestershire UK). Each DLS measurement was performed at 25 °C using automated, optimal measurement times and laser attenuation settings.
Cell culture and CuO NPs treatment (lipidomics)
All cell lines were maintained at 37 °C in a 5% CO2 atmosphere. Cells were grown in DMEM medium supplemented with 10% (v/v) FBS and 1% (v/v) penicillin/streptomycin. Approximately 3.5 × 106 cells were plated per culture dish and treated 24 h after seeding. Control cells did not receive any additional treatment and CuO NPs were used at a concentration of 2.5 or 5.0 μg mL−1 for a total treatment time of 24 h. For lipidomics analysis, we carried out two independent profiling experiments. Each profiling experiment consisted of three biological replicates per treatment. As such, a total of six biological replicates per treatment were analyzed by LC-QToF/MS and three biological replicates per treatment were analyzed by ICP/MS.
Viability assays
HCT-116, 293T HEK, HeLa and MRC5 cells were plated in 96-well plate with 15
000 cells per well and treatment was added 24 h after seeding. Five replicates were carried out per condition. After 24 h treatment, cells were centrifuged at 4 °C, 200 g for 2 minutes. Media and treatment were then removed and 200 μL of media with 0.5 mg mL−1 MTT in PBS was added and subsequently incubated for 3 h at 37 °C. A volume of 155 μL of medium was then removed and 90 μL of DMSO was added and incubated at 37 °C for 10 min, followed by pipetting to solubilize formazan product. Absorbance was measured using a Biotek Synergy HT plate reader at 550 nm. Percent viability of treated cells was calculated relative to control cells after absorbance values were blank-subtracted. Graphs were generated in GraphPad prism version 7.
Sample collection for ICP-MS and LC-QToF/MS
Following the 24 h treatment, cells were collected by scraping and centrifuged at 500g, 5 minutes, 4 °C. The media was removed and the cell pellet was washed with PBS. The cell pellets were then resuspended in 1 mL of PBS and 20 μL aliquot of the suspension was collected and added to 20 μL of lysis buffer on ice for 1 h to measure protein concentration. Remaining 980 μL aliquot was centrifuged again and PBS was removed. Cell pellets were stored at −80 °C before sample preparation.
ICP-MS analysis and ICP-MS operating conditions
Cell pellets were dried and placed in digestion vessels. The digestion was performed at 95 ± 5 °C with a mixture of 70% nitric acid (HNO3) and 30% hydrogen peroxide (H2O2). An X-Series 2 Inductively Coupled Plasma (Thermo Scientific (Waltham, MA)) was used for total copper analysis and the operational parameters are summarized in ESI† Table S3. The copper isotopes, 65Cu and 64Cu were monitored as well as 103Rh as an internal standard.
Sample preparation for lipidomics and LC-QToF/MS operating conditions
Cell pellets were thawed on ice and resuspended with 1 mL of cold deionized water. Remaining suspension was transferred to a Dounce tissue homogenizer, and 1 mL of cold methanol and 2 mL of cold chloroform were added. Samples were homogenized with 30 strokes and transferred to an 8 mL glass vial and centrifuged at 600 × g for 25 min, 4 °C. The chloroform and water/methanol layers were separated by transferring (1.3 mL) to separate glass vials. Samples were dried down and re-suspended in chloroform containing 0.5 μM TAG (13
:
0/13
:
0/13
:
0), C17-Ceramide and d9-oleic acid for lipid analysis and 50
:
50 methanol/water for polar metabolite analysis depending on the normalized volume according to the protein concentration. A 10 μL aliquot was used for LC-QToF/MS analysis.
Separation was performed using an Agilent 1260 HPLC System (Santa Clara, CA) with degasser, binary pump, autosampler, and isopump. The aqueous/polar layer was separated with a reverse phase column, with dimensions of 100 mm × 4.6 mm; the first was a Waters T3 Atlantis™ (Milford, MA) with 4 μm particle size used in positive mode Electrospray Ionization (ESI). For the non-polar layer, two reverse phase columns with dimension of 100 mm × 4.6 mm were used. A Luna C5 column with 5 μm particle size and Gemini C18 column with 5 μm particle size in positive and negative mode, respectively.
For the polar layer, samples were analyzed in positive electrospray ionization mode (+ESI) using a mobile phase A (water) and mobile phase B (methanol) supplemented with 0.1% formic acid. A 63 min method with a flow rate of 500 μL min−1 was utilized, where solvent B was held at 5% for 5 min then ramped to 90% B for over 35 min, and held at this condition for 10 min before returning to 5% B within 2 min. The column was held at 5% B for 15 min to equilibrate for the next injection. For the non-polar layer, mobile phase A with water and methanol (95
:
5) and mobile phase B isopropanol/methanol/water (65
:
35
:
5) was used and supplemented with 0.1% formic acid for positive mode analysis and 0.01% ammonium hydroxide for analysis in negative mode. For the nonpolar layer, an 80 min gradient with a flow rate of 500 μL min−1 was utilized, where solvent B was held at 5% for 5 min then ramped to 100% B over 65 min and held at this condition for 7 min before returning to 5% B within 2 min. The column was held at 5% B for 8 min to equilibrate for the next injection. All samples were analyzed in an alternate manner per layer and in positive and negative ESI. Two independent exposures were performed to provide two independent profiling experiments (3 biological replicates for each condition for each independent exposure). The data was acquired via high-resolution mass spectrometry in order to identify the reproducible changes after treatment compared to control samples.
An Agilent 6530 Accurate Mass QToF-MS (Santa Clara, CA) with a Dual JST fitted ESI was utilized for the metabolite profiling experiments. Prior to analysis, the QToF/MS was calibrated with purine and hexakis-(1H,1H,3H-tetrafluoropropoxy)phosphazine at mass-to-charge ratios (m/z's) of 118.0862, 322.0481, 622.0289, 922.0098, 1221.9906, and 1521.9715. The reference m/z’s used for mass correction were 121.0509, 922.0098 (+ESI), and 112.9856, 1033.9881 (−ESI). The MS parameters were as followed: m/z range 50–1700 at a rate of 1 spectra per second, capillary temperature 350 °C, capillary voltage 3500 V, fragmentor voltage 110 V (methanol/water layer) and 175 V (non-polar layer), drying gas temperature 350 °C, and flow rate 12 L min−1. Tandem mass spectrometry (MS/MS) data was collected at increasing collision energies of 5, 15, 35, 55 eV for each m/z.
Data analysis
For the untargeted metabolomics workflow, two sets of three biological replicates per condition were collected and analyzed independently by LC-QToF-MS, using both +ESI and −ESI modes. Mass spectral data was imported into Agilent MassHunter Profinder™ (version V.06.00) to extract peak components and chromatographic alignment based on molecular features, accurate mass, isotopic pattern, and retention times. The batch recursive feature extraction was utilized to extract and align the molecular features in all samples. The parameters for the molecular feature extraction included requirements of a peak height of ≥500 counts and extraction of possible ions inclusive of the protonated or deprotonated precursor ion, or the sodium or ammonia adduct. Furthermore, isotope-grouping restrictions comprised of a peak spacing tolerance of 0.0025 m/z and 7.0 ppm and a maximum charge state of 2. For binning and alignment, a tolerance of 0.3 minutes for retention time window and 20 ppm for mass window were set. Post-processing filters included: an absolute height filter of ≥3000 counts and the requirement for the molecular feature to be present in at least two out of three replicates in one experimental group. The aligned data were then imported into Mass Profinder Professional™ (version B12.6.1) where features were filtered according to frequency in each condition, and ANOVA was performed to compare the significance at the 95% confidence level with a post hoc Tukey honest significant difference test to determine the alterations in the global metabolome after each treatment (2.5 or 5.0 μg mL−1) with respect to the negative control group. Untargeted results species were identified by matching of feature to the online database, METLIN.40,41 As well as further MS/MS experiments using increasing collision energies for each m/z.
Agilent MassHunter™ Qualitative Analysis software (version B.06.00) was used for targeted analysis in +ESI and −ESI mode by extracting the corresponding m/z values for each hydrophilic and lipophilic compound.42 The peak areas were manually integrated for the three biological replicates in each treatment. Fold changes were determined for 2.5 μg mL−1 CuO NPs vs. negative control (untreated cells), and 5 μg mL−1 CuO NPs vs. negative control. A heat map for untargeted analysis was prepared in R (https://www.rproject.org).
Western blot analysis
Control and treated HCT-116 cell pellets were lysed on ice with lysis buffer (1 tablet of protease inhibitor dissolved in 10 mL of mammalian protein extraction) for one h. Lysates were centrifuged at 16
000 × g, 15 min, 4 °C and supernatant was used for analysis. Protein concentration was measured via Bradford protein assay, samples were diluted in a 1
:
1 with a solution of 95% Laemmli buffer and 5%, 2-mercaptoethanol. Samples were then boiled and stored at −20 °C before western blot analysis. For analysis, separation was performed with SDS-PAGE on 10% or 15% polyacrylamide gels. The proteins were transferred onto a PVDF membrane, and then washed with TBST (20 mM Tris-base, 150 mM NaCl, pH 7.5 with 0.2% v/v Tween-20). Membranes were blocked with 5% w/v nonfat dry milk in TBS-Tween [10 mM Tris-base, 100 mM NaCl, 0.1% Tween 20 (pH 7.5)] at room temperature for 1 h. PARP, LC3B-II, p62, p21, caspase 3, and α-tubulin were used for primary incubation at 4 °C for 12 h. Before and after primary incubation, membranes were washed three times with TBS-Tween for 10 minutes. Anti-rabbit (for PARP, p62, and LC3B-II) and anti-mouse (α-tubulin) secondary antibody were incubated at room temperature for 1 h, and then membranes were washed with TBS-Tween. Membranes were developed using the Super Signal West Pico kit (Thermo Scientific, Rockford, IL, USA). The intensity of the western blot bands were analyzed using ImageJ Software (National Institute of Health, Bathesda, MD). For LC3B-II (n = 3) and p62 (n = 3) quantification, the normalized ratio was determined as [relative intensityLC3B-II
or
p62]/[relative intensityα-tubulin]. The relative intensities were determined as [band intensitytreated
samples]/[band intensitycontrol]. Graphs and statistics (one-way ANOVA) were performed in GraphPad prism version 7.
Conflicts of interest
The authors declare no conflict of interest.
Acknowledgements
We acknowledge the support from the National Science Foundation grant CHE1506295 (to D. S. A.) and MCB1817468 (to G. E. A.-G.). We would like to thank the Instrument Center, specifically Dr Valerie Frerichs (UB, Department of Chemistry). This work utilized Inductively Coupled Plasma Mass Spectrometry instrument that was purchased with funding from National Science Foundation (CHE-0959565). We also would like to thank Yueling Qin (UB, Department of Chemistry) for his help with TEM.
References
- S. J. Klaine, P. J. J. Alvarez, G. E. Batley, T. F. Fernandes, R. D. Handy, D. Y. Lyon, S. Mahendra, M. J. McLaughlin and J. R. Lead, Environ. Toxicol. Chem., 2008, 27, 1825–1851 CrossRef CAS PubMed.
- B. S. Sekhon, Nanotechnol., Sci. Appl., 2014, 7, 31–53 CrossRef PubMed.
- B. Nowack and T. D. Bucheli, Environ. Pollut., 2007, 150, 5–22 CrossRef CAS PubMed.
- N. G. Chavez Soria, A. Montes, M. A. Bisson, G. E. Atilla-Gokcumen and D. S. Aga, Environ. Sci.: Nano, 2017, 4, 1944–1953 RSC.
-
M. H. Siddiqui, M. H. Al-Whaibi and F. Mohammad, Nanotechnology and Plant Sciences: Nanoparticles and Their Impact on Plants, Springer International Publishing, Cham, Switzerland, 2015 Search PubMed.
- A. Lopez-Serrano, R. M. Olivas, J. S. Landaluze and C. Camara, Anal. Methods, 2014, 6, 38–56 RSC.
- O. Bondarenko, K. Juganson, A. Ivask, K. Kasemets, M. Mortimer and A. Kahru, Arch. Toxicol., 2013, 87, 1181–1200 CrossRef CAS PubMed.
- Y. N. Chang, M. Y. Zhang, L. Xia, J. Zhang and G. M. Xing, Materials, 2012, 5, 2850–2871 CrossRef CAS.
-
A. K. Srivastava, Oxide Nanostructures: Growth, Microstructures, and Properties, Pan Stanford Publishing, Singapore, Singapore, 2014 Search PubMed.
-
A. K. Mishra, Application of Nanotechnology in Water Research, John Wiley & Sons, Incorporated, Somerset, United States, 2014 Search PubMed.
- M. B. Gawande, A. Goswami, F.-X. Felpin, T. Asefa, X. Huang, R. Silva, X. Zou, R. Zboril and R. S. Varma, Chem. Rev., 2016, 116, 3722–3811 CrossRef CAS PubMed.
- M.-L. Kung, S.-L. Hsieh, C.-C. Wu, T.-H. Chu, Y.-C. Lin, B.-W. Yeh and S. Hsieh, Nanoscale, 2015, 7, 1820–1829 RSC.
- A. Wongrakpanich, I. A. Mudunkotuwa, S. M. Geary, A. S. Morris, K. A. Mapuskar, D. R. Spitz, V. H. Grassian and A. K. Salem, Environ. Sci.: Nano, 2016, 3, 365–374 RSC.
-
M. Hosokawa, K. Nogi, M. Naito and T. Yokoyama, Nanoparticle Technology Handbook, Elsevier, Saint Louis, Netherlands, 2007 Search PubMed.
- I. Khan, K. Saeed and I. Khan, Arabian J. Chem., 2017 DOI:10.1016/j.arabjc.2017.05.011.
-
L. K. Schnackenberg, J. Sun and R. D. Beger, in Nanotoxicity: Methods and Protocols, ed. J. Reineke, Humana Press, Totowa, NJ, 2012, pp. 141–156 DOI:10.1007/978-1-62703-002-1_10.
- E. K. Matich, D. M. Butryn, M. Ghafari, V. del Solar, E. Camgoz, B. A. Pfeifer, D. S. Aga, B. Z. Haznedaroglu and G. E. Atilla-Gokcumen, Algal Res., 2016, 19, 146–154 CrossRef.
- N. Li, Y. Sancak, J. Frasor and G. E. Atilla-Gokcumen, Biochemistry, 2018, 57, 72–80 CrossRef CAS PubMed.
- S. Ortelli, A. L. Costa, M. Blosi, A. Brunelli, E. Badetti, A. Bonetto, D. Hristozov and A. Marcomini, Environ. Sci.: Nano, 2017, 4, 1264–1272 RSC.
- A. Semisch, J. Ohle, B. Witt and A. Hartwig, Part. Fibre
Toxicol., 2014, 11, 10 CrossRef PubMed.
- M. S. P. Boyles, C. Ranninger, R. Reischl, M. Rurik, R. Tessadri, O. Kohlbacher, A. Duschl and C. G. Huber, Part. Fibre Toxicol., 2016, 13, 49 CrossRef PubMed.
- A. C. Sabuncu, J. Grubbs, S. Qian, T. M. Abdel-Fattah, M. W. Stacey and A. Beskok, Colloids Surf., B, 2012, 95, 96–102 CrossRef CAS PubMed.
- B. M. Simonet and M. Valcárcel, Anal. Bioanal. Chem., 2008, 393, 17 CrossRef PubMed.
- E. J. Cho, H. Holback, K. C. Liu, S. A. Abouelmagd, J. Park and Y. Yeo, Mol. Pharmaceutics, 2013, 10, 2093–2110 CrossRef CAS PubMed.
- J. H. Kaplan and E. B. Maryon, Biophys. J., 2016, 110, 7–13 CrossRef CAS PubMed.
- T. Sun, Y. Yan, Y. Zhao, F. Guo and C. Jiang, PLoS One, 2012, 7, e43442 CrossRef CAS PubMed.
- D. Laha, A. Pramanik, J. Maity, A. Mukherjee, P. Pramanik, A. Laskar and P. Karmakar, Biochim. Biophys. Acta, Gen. Subj., 2014, 1840, 1–9 CrossRef CAS PubMed.
- S. Fulda and D. Kögel, Oncogene, 2015, 34, 5105 CrossRef CAS PubMed.
- I. Dikic and Z. Elazar, Nat. Rev. Mol. Cell Biol., 2018, 19, 349–364 CrossRef CAS PubMed.
- E. M. Storck, C. Özbalci and U. S. Eggert, Annu. Rev. Biochem., 2018, 87, 839–869 CrossRef CAS PubMed.
- D. Y. Lizardo, L. R. Parisi, N. Li and G. E. Atilla-Gokcumen, Biochemistry, 2018, 57, 22–29 CrossRef CAS PubMed.
- J. W. Tukey, Biometrics, 1949, 5, 99–114 CrossRef CAS PubMed.
- K. T. Kitchin, S. Stirdivant, B. L. Robinette, B. T. Castellon and X. Liang, Part. Fibre Toxicol., 2017, 14, 50 CrossRef PubMed.
- X.-b. Li, H. Zheng, Z.-r. Zhang, M. Li, Z.-y. Huang, H. J. Schluesener, Y.-y. Li and S.-q. Xu, Nanomedicine, 2009, 5, 473–479 CrossRef CAS PubMed.
- T. Xia, M. Kovochich, M. Liong, L. Mädler, B. Gilbert, H. Shi, J. I. Yeh, J. I. Zink and A. E. Nel, ACS Nano, 2008, 2, 2121–2134 CrossRef CAS PubMed.
- K. Bhattacharya, M. Davoren, J. Boertz, R. P. Schins, E. Hoffmann and E. Dopp, Part. Fibre Toxicol., 2009, 6, 17 CrossRef PubMed.
- M. L. Circu and T. Y. Aw, Biochim. Biophys. Acta, Mol. Cell Res., 2012, 1823, 1767–1777 CrossRef CAS PubMed.
- W. Jiang and B. Ogretmen, Biochim. Biophys. Acta, 2014, 1841, 783–792 CrossRef CAS PubMed.
- R. D. Sentelle, C. E. Senkal, W. Jiang, S. Ponnusamy, S. Gencer, S. P. Selvam, V. K. Ramshesh, Y. K. Peterson, J. J. Lemasters, Z. M. Szulc, J. Bielawski and B. Ogretmen, Nat. Chem. Biol., 2012, 8, 831–838 CrossRef CAS PubMed.
- R. Tautenhahn, K. Cho, W. Uritboonthai, Z. Zhu, G. J. Patti and G. Siuzdak, Nat. Biotechnol., 2012, 30, 826 CrossRef CAS PubMed.
- Z.-J. Zhu, A. W. Schultz, J. Wang, C. H. Johnson, S. M. Yannone, G. J. Patti and G. Siuzdak, Nat. Protoc., 2013, 8, 451 CrossRef CAS PubMed.
- N. Li, D. Y. Lizardo and G. E. Atilla-Gokcumen, ACS Chem. Biol., 2016, 11, 2583–2587 CrossRef CAS PubMed.
Footnote |
† Electronic supplementary information (ESI) available. See DOI: 10.1039/c8mo00162f |
|
This journal is © The Royal Society of Chemistry 2019 |