DOI:
10.1039/C7RA09779D
(Paper)
RSC Adv., 2017,
7, 55389-55399
Metabolomic investigation into molecular mechanisms of a clinical herb prescription against metabolic syndrome by a systematic approach†
Received
2nd September 2017
, Accepted 22nd November 2017
First published on 6th December 2017
Abstract
Wendan decoction (WDD), a classic herb prescription in China, has been extensively proved to improve metabolic syndrome (Mets) in clinics. However, till now, its pharmacological mechanisms remained vague. In this study, a systematic approach that integrated GC-TOF/MS based metabolomics, multivariate statistical techniques (PCA and PLS-DA), KEGG pathway analysis and molecular docking simulation was established to explore the pathophysiological mechanisms of Mets and elucidate the molecular mechanisms of WDD against Mets rats. Compared to the control rat group, five significantly altered and impacted pathways (P < 0.05 and IV > 1) associated with thirty-nine significantly altered metabolites related to glycolysis, the TCA cycle, the urea cycle, amino acid and lipid metabolisms, gluconeogenesis and ketogenesis were identified as biomarkers of Mets. After two weeks of treatment, seventeen and fourteen significantly altered metabolites in the Mets model group tended to be restored to normal levels by WDD and metformin, respectively. Additionally, six significantly altered pathways were involved in treatment of Mets by WDD, while no significant pathway was found for metformin (P < 0.05). Finally, the molecular docking simulation revealed that seventy compounds in WDD competed with substrates to bind with four enzymes, which led to a reduction in the serum levels of significantly altered metabolites.
Introduction
Metabolic syndrome (Mets) is a complex cluster of metabolic disorders, including abdominal obesity, insulin resistance, dyslipidemia and hyperglycemia, with an increased risk of developing type 2 diabetes and cardiovascular diseases.1 Nowadays, the prevalence of Mets is increasing significantly, which has become a serious challenge to public health worldwide.2 However, so far, there is still no single treatment to control Mets. Current therapeutic strategies for Mets still treat each component separately and its underlying mechanisms are yet to be unveiled.3
Traditional Chinese medicine (TCM) has been an important complementary and alternative medical system in China for thousands of years.4 Herbal medicine treatment of Mets has become an alternative and promising strategy in China.5 WDD, a famous six herb prescription, including Radix Glycyrrhizae Preparata, Pericarpium Citri Reticulatae, Pinellia Ternata, Poria Cocos, Citrus Aurantium, and Caulis Bambusae in Taeniam, originated in the Tang Dynasty and has been widely applied to treat syndromes related to Mets in clinics in China.5 WDD was proved to show beneficial clinical therapeutic effects on Mets patients for reducing weight and waistline, lowering glucose and cholesterol levels, blood pressure, and anti-inflammation.5 However, to date, the action mechanisms of WDD in treatment of Mets still remain vague. Considering herbal formulas are mixtures of diverse chemical ingredients and have multiple roles in the living system, it is difficult to systematically study the mechanisms of herbal formulas by using traditional analytical methods.4 Metabolomics is a novel systemic technology that can quantitatively measure the dynamic function response and metabolic changes of a living system caused by interventions in holistic context using quantitative high-throughput approaches (e.g., gas chromatography-mass spectrometry (GC-MS) and 1H-nuclear magnetic resonance (NMR)).6 Metabolomics has been successfully applied in biomarker discovery, disease diagnosis, drug efficacy and toxicity evaluation, and proved to be a powerful tool for understanding the mechanism of diseases.7 Currently, it also has been increasingly employed for assessing therapeutic mechanisms and effects of many herbal TCM prescriptions. For instance, Tao et al. found that Fu-Zhu-Jiang-Tang tablet and its optimal combination treatments could ameliorate abnormal glucose and lipid metabolism, reduce high glucose levels and reverse abnormal levels of serum metabolites in type 2 diabetes rats by using GC-MS based metabolomic approach.8 Metabolomic technique has been used to identify some potential biomarkers of Mets such as inositol and palmitoleic acid.9,10 However, to the best of our knowledge, there was no report of metabolomics studies on the metabolic pathways and herb therapeutic mechanism of Mets.
In this study, a systematic approach that integrated gas chromatography-time of flight/mass spectrometry (GC-TOF/MS)-based metabolomics, multivariate statistical techniques (principal component analysis (PCA) and partial least squares discriminant analysis (PLS-DA)),11 kyoto encyclopedia of genes and genomes (KEGG) pathway analysis12 and molecular docking simulation13 was developed to compare the metabolite changes in response to treatment with WDD and metformin, respectively, aiming to discover potential biomarkers and metabolic pathways of Mets and explore the therapeutic mechanisms of WDD in treatment of Mets. First, forty rats were randomly divided into four groups according to interventions: control group, Mets model group, herb treated group and metformin treated group. Second, GC-TOF/MS-based metabolomics was established to monitor the dynamic changes in the endogenous metabolites of serum from four rat groups. Third, multivariate analysis of pattern recognition multivariate analysis, including PCA and PLS-DA was performed to discover significantly altered metabolites in the metabolic data. Fourth, the enriched KEGG pathway analysis was utilized to reveal the obviously impacted metabolite-associated pathways between groups. Fifth, molecular docking simulation was applied to explore the molecular mechanism of WDD intervening in significantly affected pathways.
Materials and methods
Chemicals and reagents
Detection kits such as high density lipoprotein cholesterol (HDL-C) and insulin were purchased from Nanjing jiancheng bioengineering institute Inc. China; blood glucose test paper was purchased from Shanghai yuyue medical equipment Inc. China. Six herb drugs in WDD were purchased from the third affiliated hospital of Fujian university of traditional Chinese medicine. Metformin sustained release tablets were purchased from Tianfang pharmaceutical Inc. China. All metabolomics reagents such as L-2-chlorophenylalanine, trifluoroacetamide (BSTFA), trimethylchlorosilane (TMCS), methoxy amination hydrochloride, methanol, saturated fatty acid methyl esters (FAMEs: C8, C9, C10, C12, C14, C16, C18, C20, C22, C24) were chromatographic grade and purchased from Shanghai hengbai biotechnology Inc. China, Regis technologies Inc. USA, Adamas (Switzerland), and Dr Ehrenstorfer GmbH (Germany), respectively.
Animal, diets, maintenance and sample collection
All animal experiments were approved by the Animal Care and Use Committee, Fujian University of Traditional Chinese Medicine, Fuzhou, P. R. China, and all experiments were performed in accordance with the guidelines approved by the Care and Use of Laboratory Animals of the National Research Council (China). Forty Wistar male rat serum samples of normal control group, Mets model group, herb treated group and metformin treated group were taken from our recent work.14,15 Ten rats in the normal control group were fed with normal diet for 17 weeks, and thirty rats in the Mets model group were fed with fifteen-week's high-sugar-fat-diet and two-week's high-fat emulsion. The specific information of making Mets animal model was listed in ESI.†14 Rats in herb treated group were administrated with WDD by intragastric at a dose of 10 mL kg−1 d−1 for two weeks, and rats in metformin group were administrated with metformin by intragastric at a dose of 100 mg kg−1 d−1 for two weeks, respectively. The dose of WDD and metformin that we employed in the rat experiments was within the human therapeutic range according to the Guidance of FDA-CDER and Chinese pharmacopoeia.16,17 Then, all rats were sacrificed and blood samples were isolated by centrifugation at 2500 rpm at 4 °C. And, serum samples were separated into 200 μL sub-aliquots and stored at −80 °C until analysis.
Preparation of samples for GC-TOF/MS
GC-TOF/MS was performed to analyze metabolic profiling of serum samples as follows. First, 100 μL of serum sample was spiked with 0.35 mL of methanol and an internal standard (20 μL of L-2-chlorophenylalanine), and mixed by vortexing and centrifuged at 4 °C, 13
000 rpm for 20 min; 0.4 mL of supernatant was then transferred into a fresh 2 mL GC/MS glass vial, and 10 μL of each supernatant was also taken and pooled as quality control samples (QC samples),18 respectively. After dried in a vacuum concentrator, 60 μL of methoxy amination hydrochloride (20 mg mL−1 in pyridine) were added into the extracts and then incubated at 80 °C for 30 min. Subsequently, 80 μL of the BSTFA regent (1% TMCS, v/v) was added into the sample aliquots, followed by incubation at 70°Cfor 1.5 h. Finally, 8 μL of a standard mixture of fatty acid methyl esters (FAMEs, 1 mg mL−1 C8–C16 and 0.5 mg mL−1 C18–C30 in chloroform) was added.
GC-TOF/MS analysis
To test system stability, the retention time (RT) of the internal standard (L-2-chlorobenzene alanine) in all samples was monitored in GC-TOF/MS analysis.8 Besides, QC samples were used to examine the stability and repeatability of the method.19 The GC-TOF/MS analysis was performed using an Agilent 7890 gas chromatograph system coupled with a Pegasus HT time-of-flight mass spectrometer. The system utilized a DB-5MS capillary column (30 m × 250 μm i.d., 0.25 μm film thickness; J&W Scientific, Folsom, CA, USA). The optimized temperature program was set as follows. The initial temperature was kept at 50 °C for 1 min, then raised to 300 °C at a rate of 20°C min−1, then maintained at 300 °C for 6.5 min. The temperatures of injection, transfer line and ion source were set at 280 °C, 280 °C and 220 °C, respectively. The electron impact energy was fixed at −70 eV. Full-scan mass spectrometry data were obtained from the m/z range of 30 to 600 at a rate of 20 spectra per second after a solvent delay of 4.93 min.
Data analysis
The Chroma TOF 4.3X software of LECO Corporation combined with LECO-Fiehn Rtx5 database were employed for extracting raw peaks, filtering and calibrating data baselines, peak identification and integration of the peak area.20 The retention time index (RI) method was used in the peak identification, and the RI tolerance was 5000.21 Besides, metabolic features detected in less than 50% of QC samples were also removed. Further, similarity analysis based on MS spectra was followed to reduce the range of metabolites. Next, the data were normalized by the internal standard. Thereafter, multivariate analysis of pattern recognition multivariate analysis, including PCA and PLS-DA, was performed to analyze the similarity and variability of the data between groups using the SIMCA-P 12.0 software package (Umetrics, Umea, Sweden).22 Additionally, a five-fold cross-validation and a 200 permutation test were applied to evaluate the robustness, predictive ability and reliability of the derived PLS-DA model. The corresponding evaluation parameters of the models were R2Y (cum), Q2Y (cum) and Q2 intercept.6,23
Identification of significantly altered metabolites and pathways between groups
The PLS-DA model was used to identify the significantly altered metabolites between two comparison groups. Generally, the criteria for identifying significantly altered metabolites was a variable importance in the projection (VIP) value from the PLS-DA model (VIP > 1) and a student's t test (P < 0.05).24 In addition, the identified significantly altered metabolites were referenced to the pathway enrichment and topology analyses by further searching KEGG databases.25,26
Molecular docking simulation
Molecular docking was further applied to explore the molecular mechanism of WDD intervention in obviously affected pathways, which has successfully prioritized large chemical libraries to identify experimentally active compounds.27 The docking simulation was carried out by following steps. First, chemical ingredients from herbs in WDD were collected by searching the Beilstein/Gmelin CrossFire Chemical database, the Handbook of the Constituents in Chinese Herb Original Plants and Chinese Herbal Drug Database (2002 version).28,29 After removing duplicate ingredients among herbs, a total of 618 compounds were obtained30 and their two and three dimension structures were sketched and optimized using MOE2008 software (Chemical Computing Group, Montreal, Canada). Second, the three dimension crystal structures of targets involved in the regulation of significant altered metabolites in herb treated group were retrieved from the Research Collaboratory for Structural Bioinformatics Protein Data Bank. Then, these proteins were protonated using AMBER99 force field and minimized with a RMSD gradient of 0.05 kcal mol−1 Å−1. Additionally, the ligand atom mode was used as the binding site, and triangle matcher algorithm was used to search the docking placement. Finally, London dG together with a force field was adopted to calculate the interactions.31 Generally, the docking scores of the original ligands in the crystal structures of the protein–ligand complexes were the thresholds for determining strong binding forces between compounds and proteins.32 Further to illuminate the relationship between bioactive compounds in WDD and hit targets, the compound-target network was constructed by cytoscape 2.8.0.33
Results
Biochemical measurements
As shown in Fig. S1 and S2 (ESI†), compared with the control group, the abdominal perimeters, serum levels of insulin and HOMA-IR of rats in Mets model group were significantly increased, whereas the serum HDL levels were significantly decreased (P < 0.05) before drug interventions. After two weeks treatment, by comparison of Mets model group, WDD resulted in significant decreases in abdominal perimeters and serum insulin levels as well increases in the serum HDL levels (P < 0.05), and metformin treatment significantly reduced abdominal perimeters but showed no significant changes in the serum levels of insulin and HDL (P < 0.05). Additionally, both WDD and metformin have the tendency to recover the HOMA-IR to the control level compared with the normal control group (P > 0.05).
Identification and quantification of GC-TOF/MS compounds
The standard deviation of retention times of the internal standard (L-2-chlorophenylalanine) in 40 serum samples was 0.001135, demonstrating that the method was sensitive, precise, and accurate enough for metabolomic analysis. The GC-TOF/MS total ion current (TIC) chromatograms of forty samples were shown in Fig. 1. As could be seen in Fig. 1, there were various abundances and quantities of peaks among the control group, Mets model group, herb treated group and metformin treated group, and no drift was observed in any of the peaks displaying a stable retention time. In total, 303 ion peaks were identified for the four groups. Followed by searching the LECO/Fiehn metabolomics library, the majority of the peaks were identified as endogenous metabolites, and some of these peaks were attributed to the derivatives of byproducts. Besides, metabolic features detected in less than 50% of QC samples were also removed. Finally, 146 metabolites in the serum levels of four groups were in total matched and quantified.
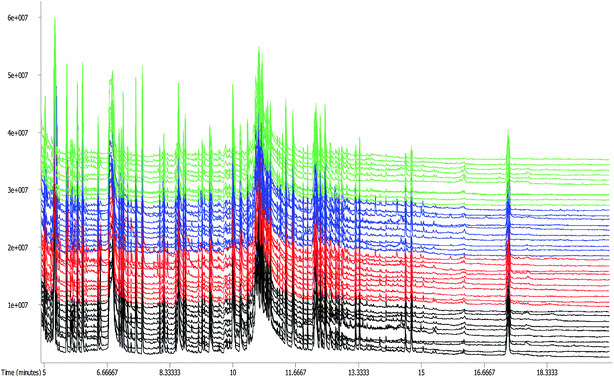 |
| Fig. 1 GC-TOF/MS TIC chromatograms of rat serum in control group (green), Mets model group (black), herb treated group (blue) and metformin treated group (red). | |
Statistical comparison of metabolites
The PCA analysis of GC-TOF/MS metabolic profiles between control group and Mets model group, Mets model and herb treated group, Mets model and metformin treated group was shown in each 3D-PCA score plot (Fig. 2A, D and G), which showed that there were differences in the metabolic patterns of the samples but could not be clearly distinguished between groups. So, the supervised PLS-DA was performed to highlight the differences between groups. The parameters for the assessment of the PLS-DA model quality in discriminating groups could be represented by the validation plots, as shown in Fig. 2B, E and H. The corresponding R2Y (cum) and Q2Y (cum) values of PLS-DA models for the control and model groups, the model and herb treated groups, the model and metformin treated groups were 0.981 and 0.964, 0.981 and 0.815, 0.894 and 0.742, respectively, indicating that the three PLS-DA models produced high predictive abilities and satisfactory effectiveness achieving distinct separations between the two comparison groups.10 The PLS-DA score scatter plots were shown in Fig. 2C, F and I. In addition, all Q2Y (cum) intercept values of the 200 permutation tests of obtained PLS-DA models were smaller than 0.05, showing no overfitting of the models.10 Therefore, the three PLS-DA models can be used to identify the differences between the two comparison groups.
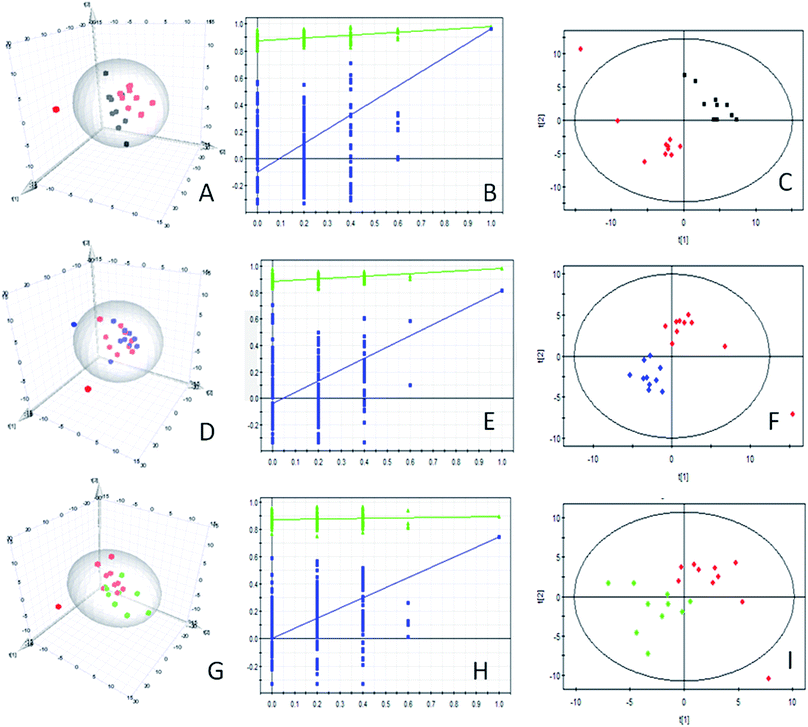 |
| Fig. 2 PCA 3D score map (A, D, and G), corresponding validation plots of PLS-DA (B, E, and H), and PLS-DA score plots (C, F, and I) derived from the metabolite profiles for Mets model group and control group (A–C), Mets model group and herb treated group (D–F), Mets model group and metformin treated group (G–I). Red represents the Mets model group, black represents the control group, blue represents the herb treated group, and green represents the metformin treated group. The validation plots were obtained by randomly permuted for 200 times with three component extracts. Green ▲ is for R2Y (cum), and blue ■ is for Q2Y (cum). | |
Statistical comparison of metabolites between groups
In total, 39, 8 and 5 significantly changed metabolites (VIP > 1 and P < 0.05) were identified in the control and Mets model groups, the Mets model and herb treated groups, and the Mets model and metformin treated groups (Table 1), respectively, which were accountable for momentous separations for PLS-DA models. Additionally, one-way ANOVA was followed to compare the relative amounts of these significantly altered metabolites between groups (see Table 1). As shown in Table 1, 31 metabolites exhibited higher concentrations in the model group than in the control group (P < 0.05), including succinate semialdehyde, phthalic acid, D-galacturonic acid, gluconic acid, L-allothreonine, uridine, 3,6-anhydro-D-galactose, 3-aminoisobutyric acid and so on. While, the concentrations of 8 metabolites in the control group were higher than that in the model group (P < 0.05), including galactose, 1-monopalmitin, palmitoleic acid, 2(alpha-D-Mannosyl)-D-glycerate, arachidonic acid, linoleic acid and 6-phosphogluconic acid. After herb treatment, obviously decreased concentrations of 3-hydroxyaspartic acid, taurine, malonamide, N-acetyl-b-D-galactosamine, xanthotoxin, glutamine and trans-4-hydroxy-L-proline and increased concentration of 3-(4-hydroxyphenyl) propionic were observed in the herb treated group (P < 0.05). Compared with Mets model group, the metformin treated group displayed the significantly decreased levels of 5 metabolites, including ribose, urea, xanthotoxin, N-acetyl-b-D-galactosamine and trehalose-6-phosphate (P < 0.05). Additionally, our results showed that the levels of seventeen and fourteen significantly altered metabolites in the Mets model group tended to be restored to normal levels by interventions of WDD and metformin by comparison with the control group (P > 0.05), respectively.
Table 1 The significantly changed metabolites between two comparison groups by one-way ANOVAa
Metabolite name |
RT (min) |
Control group (mean ± SD) |
Mets model group (mean ± SD) |
Herb treated group (mean ± SD) |
Metformin treated group (mean ± SD) |
RT represents retention time. P < 0.05 vs. control group. P > 0.05 vs. control group. P < 0.05, herb treated group vs. Mets model group. P < 0.05, metformin treated group vs. Mets model group. |
Control and model group (VIP > 1 and P < 0.05) |
1-Monopalmitin |
13.6652 |
0.00743 ± 0.00673 |
0.00001 ± 0↓b |
0.00001 ± 0 |
0.00001 ± 0 |
2,4-Diaminobutyric acid |
8.1220 |
0.0125 ± 0.00528 |
0.021 ± 0.01123↑b |
0.01349 ± 0.00623↓c |
0.01831 ± 0.01055↓c |
3,6-Anhydro-D-galactose |
9.8393 |
0.01563 ± 0.01061 |
0.02925 ± 0.01666↑b |
0.0236 ± 0.01112↓c |
0.02308 ± 0.01058↓c |
3-Aminoisobutyric acid |
8.16769 |
0.24172 ± 0.14168 |
0.42973 ± 0.21064↑b |
0.46365 ± 0.21044 |
0.27843 ± 0.16709↓c |
2-Amino-3-hydroxy-pentanoic acid |
7.59965 |
1.17736 ± 0.09862 |
1.39692 ± 0.16493↑b |
1.36661 ± 0.09382 |
1.33572 ± 0.134 |
6-Phosphogluconic acid |
13.0159 |
0.01356 ± 0.00257 |
0.01106 ± 0.00252↓b |
0.01028 ± 0.00239 |
0.01058 ± 0.00224 |
Alanine |
5.59036 |
1.27452 ± 0.16188 |
1.49534 ± 0.2117↑b |
1.44971 ± 0.22982↓c |
1.51235 ± 0.21018 |
Arachidonic acid |
12.8841 |
0.06466 ± 0.00798 |
0.04339 ± 0.00952↓b |
0.04285 ± 0.00756 |
0.03605 ± 0.00829 |
Asparagine |
8.44785 |
0.00614 ± 0.00172 |
0.00964 ± 0.0036↑b |
0.00715 ± 0.00202↓c |
0.00954 ± 0.00352 |
Aspartic acid |
8.47918 |
0.04386 ± 0.00783 |
0.07189 ± 0.0215↑b |
0.07841 ± 0.01392 |
0.07859 ± 0.02897 |
2(Alpha-D-mannosyl)-D-glycerate |
10.3875 |
0.31942 ± 0.0705 |
0.24741 ± 0.0348↓b |
0.24049 ± 0.06142 |
0.25021 ± 0.02638 |
Canavanine |
11.0516 |
0.00462 ± 0.00183 |
0.0071 ± 0.00159↑b |
0.0059 ± 0.00186↓c |
0.00618 ± 0.00282↓c |
Citrulline |
10.2511 |
0.01423 ± 0.00381 |
0.02424 ± 0.00647↑b |
0.01767 ± 0.00773↓c |
0.01756 ± 0.00922↓c |
Cytosine |
8.63388 |
0.01124 ± 0.00223 |
0.0146 ± 0.00299↑b |
0.01442 ± 0.00737↓c |
0.01584 ± 0.0059 |
D-Galacturonic acid |
10.9769 |
0.01333 ± 0.0073 |
0.02934 ± 0.00814↑b |
0.02389 ± 0.0071 |
0.0236 ± 0.00976 |
D-Glucoheptose |
11.8627 |
0.02455 ± 0.00916 |
0.04027 ± 0.01831↑b |
0.03903 ± 0.01313 |
0.0367 ± 0.00611 |
D-Glyceric acid |
7.21031 |
0.16081 ± 0.04594 |
0.24483 ± 0.06016↑b |
0.20238 ± 0.04142 |
0.19692 ± 0.10473↓c |
Galactose |
10.7749 |
0.03311 ± 0.02338 |
0.00001 ± 0↓b |
0.00001 ± 0 |
0.00001 ± 0 |
Calcium gluceptate |
12.3776 |
0.03202 ± 0.01116 |
0.0504 ± 0.01884↑b |
0.05803 ± 0.02395 |
0.03677 ± 0.00943↓c |
Gluconic acid |
11.1405 |
0.00133 ± 0.00096 |
0.00283 ± 0.00099↑b |
0.00278 ± 0.00124 |
0.00194 ± 0.00148↓c |
Glutamic acid |
9.08531 |
0.0177 ± 0.00419 |
0.02633 ± 0.00763↑b |
0.02208 ± 0.0045 |
0.02168 ± 0.00614↓c |
L-Allothreonine |
7.66133 |
0.00127 ± 0.00136 |
0.00269 ± 0.00111↑b |
0.0017 ± 0.00119↓c |
0.00181 ± 0.0013↓c |
Linoleic acid |
12.1830 |
0.05296 ± 0.01111 |
0.03875 ± 0.01093↓b |
0.03626 ± 0.01081 |
0.0354 ± 0.01106 |
Lysine |
10.8060 |
0.26707 ± 0.03525 |
0.33498 ± 0.06949↑b |
0.36098 ± 0.04785 |
0.31169 ± 0.03589 |
Lyxose |
9.29867 |
0.0188 ± 0.00836 |
0.02665 ± 0.00304↑b |
0.02653 ± 0.00663 |
0.02295 ± 0.00487↓c |
Malonamide |
8.73769 |
0.67425 ± 0.17445 |
0.8872 ± 0.17824↑b |
0.71402 ± 0.07539↓c |
0.91612 ± 0.24714 |
Myo-inositol |
11.5905 |
0.31363 ± 0.07297 |
0.39673 ± 0.07043↑b |
0.37231 ± 0.07104↓c |
0.33395 ± 0.07041↓c |
N-Methyl-DL-alanine |
6.10418 |
0.03809 ± 0.0093 |
0.05309 ± 0.01612↑b |
0.0477 ± 0.01078 |
0.06044 ± 0.02486 |
Ornithine |
10.2118 |
0.2375 ± 0.10992 |
0.33171 ± 0.08702↑b |
0.37292 ± 0.17916 |
0.41487 ± 0.21387 |
Oxalacetic acid |
8.17627 |
0.02291 ± 0.01054 |
0.03822 ± 0.01667↑b |
0.04225 ± 0.02961 |
0.02947 ± 0.01646↓c |
Pyroglutamic acid |
8.55582 |
2.25365 ± 0.19112 |
2.70373 ± 0.48728↑b |
2.37131 ± 0.18424↓c |
2.53277 ± 0.36648 |
Palmitoleic acid |
11.3180 |
0.04162 ± 0.01366 |
0.01646 ± 0.01158↓b |
0.04757 ± 0.06641↑c |
0.01202 ± 0.00894 |
Pantothenic acid |
11.1201 |
0.00239 ± 0.0012 |
0.00387 ± 0.00187↑b |
0.00302 ± 0.0013↓c |
0.00365 ± 0.00125 |
Phthalic acid |
9.5527 |
0.00219 ± 0.00218 |
0.0061 ± 0.00328↑b |
0.00443 ± 0.00477↓c |
0.00461 ± 0.00268 |
Serine |
7.42715 |
1.08122 ± 0.07831 |
1.50685 ± 0.15096↑b |
1.54283 ± 0.12191 |
1.39611 ± 0.17023 |
Succinate semialdehyde |
6.30982 |
0.00059 ± 0.00183 |
0.00358 ± 0.00269↑b |
0.00157 ± 0.00334↓c |
0.00327 ± 0.00345 |
3-Hydroxyaspartic acid |
9.02614 |
0.00572 ± 0.00198 |
0.00339 ± 0.00112↓b |
0.00183 ± 0.00112 |
0.0027 ± 0.00154 |
Threonic acid |
8.68774 |
0.0528 ± 0.00522 |
0.06479 ± 0.0152↑b |
0.06009 ± 0.01295↓c |
0.05881 ± 0.0151↓c |
Uridine |
13.2566 |
0.04225 ± 0.01358 |
0.0833 ± 0.0421↑b |
0.06747 ± 0.03631↓c |
0.07414 ± 0.01697 |
![[thin space (1/6-em)]](https://www.rsc.org/images/entities/char_2009.gif) |
Mets model group and herb treated group (VIP > 1 and P < 0.05) |
3-(4-Hydroxyphenyl)propionic acid |
9.96887 |
0.00001 ± 0 |
0.00001 ± 0 |
0.03133 ± 0.01399 |
0.00001 |
3-Hydroxyaspartic acid |
9.02614 |
0.00572 ± 0.00198 |
0.00339 ± 0.00112 |
0.00183 ± 0.00112↓d |
0.00270 ± 0.00154 |
Taurine |
9.42321 |
0.11441 ± 0.02828 |
0.14132 ± 0.03635 |
0.09798 ± 0.02959↓d |
0.14206 ± 0.06536 |
Malonamide |
8.73769 |
0.67425 ± 0.17445 |
0.88720 ± 0.17824 |
0.71402 ± 0.07539↓d |
0.91612 ± 0.24714 |
N-Acetyl-b-D-galactosamine |
11.5269 |
0.00167 ± 0.00135 |
0.00263 ± 0.00150 |
0.00110 ± 0.00113↓d |
0.00112 ± 0.0010 |
Xanthotoxin |
12.5079 |
0.00514 ± 0.00580 |
0.01709 ± 0.01932 |
0.00251 ± 0.00610↓d |
0.00120 ± 0.00373 |
Glutamine |
9.9949 |
0.00906 ± 0.00634 |
0.01296 ± 0.00540 |
0.0069 ± 0.00669↓d |
0.00888 ± 0.00669 |
Trans-4-hydroxy-L-proline |
8.52598 |
0.03102 ± 0.00819 |
0.03202 ± 0.00898 |
0.02312 ± 0.00922↓d |
0.02958 ± 0.00744 |
![[thin space (1/6-em)]](https://www.rsc.org/images/entities/char_2009.gif) |
Mets model group and metformin treated group (VIP > 1 and P < 0.05) |
Ribose |
9.38722 |
0.35362 ± 0.04564 |
0.37347 ± 0.05745 |
0.35757 ± 0.05318 |
0.30251 ± 0.04727↓e |
N-Acetyl-b-D-galactosamine |
11.5269 |
0.00167 ± 0.00135 |
0.00263 ± 0.00150 |
0.0011 ± 0.00113 |
0.00112 ± 0.001↓e |
Urea |
6.77243 |
0.26707 ± 0.10712 |
0.31285 ± 0.13825 |
0.20067 ± 0.13682 |
0.14329 ± 0.15015↓e |
Xanthotoxin |
12.5079 |
0.00514 ± 0.00580 |
0.01709 ± 0.01932 |
0.00251 ± 0.00610 |
0.00120 ± 0.00373↓e |
Trehalose-6-phosphate |
16.1281 |
0.05621 ± 0.05064 |
0.0589 ± 0.03227 |
0.05878 ± 0.04191 |
0.02915 ± 0.02145↓e |
Metabolic pathway analysis
There were 7 obviously altered KEGG enrichment pathways (P < 0.05) involved in 39 significantly changed metabolites between the control and Mets model groups (Table 2), including alanine, aspartate and glutamate metabolism, aminoacyl-tRNA biosynthesis, arginine and proline metabolism, glutathione metabolism, glycine, serine and threonine metabolism, histidine metabolism, glyoxylate and dicarboxylate metabolism. As shown in Table 2, the impact values of these pathways were 0.611, 0.138, 0.251, 0.07, 0.243, 0 and 0.149, respectively. Generally, an impact value equal to or greater than 0.1 indicates that this altered pathway is obviously affected.8 Thereby, only 5 enriched pathways were significantly perturbed in Mets model group. The enriched pathways (P < 0.05) for 8 significantly altered metabolites identified in the Mets model and herb treated groups showed that arginine and proline metabolism, alanine, aspartate and glutamate metabolism, D-glutamine and D-glutamate metabolism, taurine and hypotaurine metabolism, aminoacyl-tRNA biosynthesis and nitrogen metabolism were involved in the treatment of MS by WDD. The impact values of these pathways were also listed in Table 2. While, no enriched pathway (P < 0.05) was identified in the Mets model and metformin treated groups.
Table 2 Metabolic pathways identified from the significantly different metabolites between comparison groups
Metabolic pathway |
Compounds |
P-value |
Impact value (IV) |
Control and mets model group |
Alanine, aspartate and glutamate metabolism |
Aspartic acid; alanine; succinate semialdehyde; glutamic acid; oxalacetic acid; asparagine |
5.86 × 10−6 |
0.61076 |
Aminoacyl-tRNA biosynthesis |
Asparagine; aspartic acid; serine; alanine; lysine; glutamic acid |
0.002278 |
0.13793 |
Arginine and proline metabolism |
Ornithine; citrulline; aspartic acid; glutamic acid |
0.012754 |
0.25125 |
Glutathione metabolism |
Glutamic acid; pyroglutamic acid; ornithine |
0.016498 |
0.06965 |
Glycine, serine and threonine metabolism |
Serine; glyceric acid; allothreonine |
0.028867 |
0.2428 |
Histidine metabolism |
Glutamic acid; aspartic acid |
0.039109 |
0 |
Glyoxylate and dicarboxylate metabolism |
Glyceric acid; oxalacetic acid |
0.04411 |
0.14815 |
![[thin space (1/6-em)]](https://www.rsc.org/images/entities/char_2009.gif) |
Mets model group and herb treated group |
Arginine and proline metabolism |
Glutamine; hydroxyproline; aspartic acid |
0.000925 |
0.04414 |
Alanine, aspartate and glutamate metabolism |
Glutamine; aspartic acid |
0.005599 |
0.34283 |
D-Glutamine and D-glutamate metabolism |
Glutamine |
0.024751 |
0 |
Taurine and hypotaurine metabolism |
Taurine |
0.039348 |
0.42857 |
Aminoacyl-tRNA biosynthesis |
Glutamine; aspartic acid |
0.040445 |
0 |
Nitrogen metabolism |
Glutamine |
0.044172 |
0 |
![[thin space (1/6-em)]](https://www.rsc.org/images/entities/char_2009.gif) |
Mets model group and metformin treated group |
Pentose phosphate pathway |
Ribose |
0.06604 |
0 |
Arginine and proline metabolism |
Urea |
0.14757 |
0 |
Purine metabolism |
Urea |
0.22038 |
0 |
Molecular docking results
A total of 5 enzymes that closely associated with regulations of 8 significantly altered metabolites in obviously affected and altered pathways in herb treated group, including glutamine synthetase (PDB code: 2OJW), aspartoacylase (PDB code: 2O4H), asparaginase (PDB code: 4PVS), glutamate decarboxylase (PDB code: 2OKJ) and sulfinoalanine decarboxylase (PDB code: 2IJS), were subjected to the molecular docking simulation. The compounds with docking scores bigger than the original ligands in the crystal structures of the protein–ligand complexes were believed to have a strong affinity with the targets.32 Therefore, a total of 70 compounds in WDD were found to be well interacted with four enzymes, which were glutamate decarboxylase, asparaginase, sulfinoalanine decarboxylase and aspartoacylase.
Further to illuminate the relationship between bioactive compounds and hit targets, the compound-target network was shown in Fig. 3. It can be seen that 15 compounds had more than two links with other enzymes, indicating multi-targets of WDD. Table 3 listed a few simple parameters of the network such as degree and betweeness, which have been proposed as metrics in assessing major nodes.34
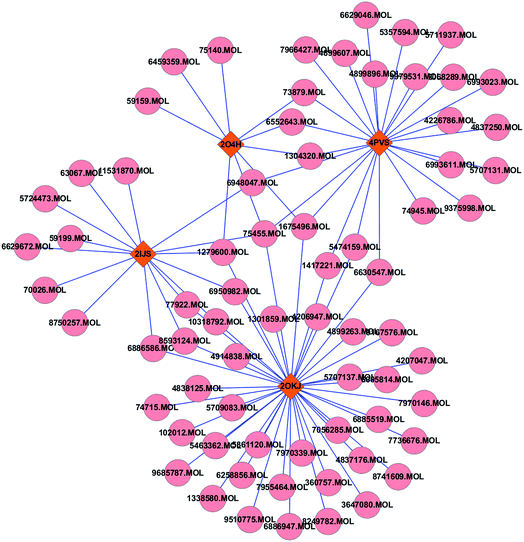 |
| Fig. 3 The compound-enzymes network analysis: the pink round nodes referred to compounds in WDD; the claybank rhombic nodes represent enzymes. | |
Table 3 Network features of targets in the compound-enzymes network
Name |
PDB code |
Degree |
Betweenness |
Glutamate decarboxylase |
2OKJ |
41 |
0.7145 |
Asparaginase |
4PVS |
24 |
0.4222 |
Sulfinoalanine decarboxylase |
2IJS |
15 |
0.2121 |
Aspartoacylase |
2O4H |
9 |
0.1077 |
Discussion
The serum biochemistry results showed that high-fat and high-glucose-fed caused significant changes in the abdominal circumferences, HOMA-IR and serum levels of HDL and insulin of rats, indicating that the Mets rat model was well established. After two weeks of treatment, WDD performed better on reducing abdominal circumferences and serum levels of insulin, and increasing serum HDL levels and than metformin. Additionally, both WDD and metformin had callback effects on HOMA-IR compared with the normal control group.
Metabolomic approach was employed to monitor the dynamic changes in the endogenous metabolites of serum from different experimental groups. Compared to control group, 79.5% of the significantly altered metabolites were elevated in serum of the Mets model group, including carbohydrates (e.g., lyxose, D-glucoheptose and 3,6-anhydro-D-galactose), lipids (e.g. D-glyceric acid, 1-monopalmitin and myo-inositol), amino acids (e.g., serine, citrulline, canavanine, asparagine and lysine). The significant reduction of metabolites was mainly unsaturated chain fatty acids (e.g., arachidonic acid, palmitoleic acid, linoleic acid). These metabolomic alterations were related to disruptions of carbohydrate metabolism, lipid metabolism and amino acid metabolism, and promotion of gluconeogenesis and ketogenesis (Fig. 4), which were consistent with the fact that the unbalance of lipid and glucose metabolisms are the main causes of Mets.
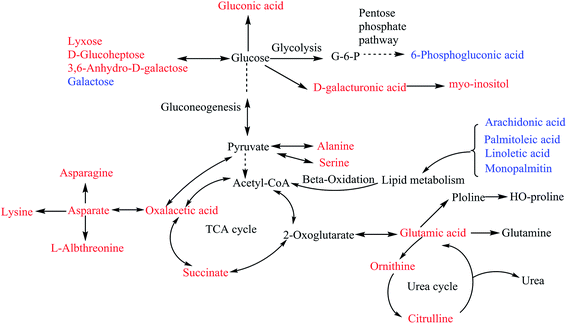 |
| Fig. 4 Perturbed metabolic network of main significantly changed metabolites in the Mets model group. Dashed line indicated a number of steps happened; red represents up-regulation, blue represents down-regulation in this metabolic process. | |
Carbohydrates are the major source of fuel for metabolism, being used as an energy source. The elevation of carbohydrates such as lyxose, D-glucoheptose and 3,6-anhydro-D-galactose in model group is associated with the high sugar-fat diet. Carbohydrrates can be oxidized to pyruvate via glycolysis to provide acetyl-CoA for TCA cycle. The levels of oxalacetic acid and succinate semialdehyde were significantly elevated in the Mets model group, which were important intermediates in the TCA cycle, a central metabolic pathway for production of ATP in animals. This implied the impaired activity of TCA cycle in the Mets model group. The significant reduction of glucose-6-phosphate indicated that glucolysis and pentose phosphate pathway were also disrupted in Mets model group (Fig. 4).
Fatty acid molecules are broken down in the mitochondria and undergo beta-oxidation to generate acetyl-CoA, which enters the TCA cycle (Fig. 4). Unsaturated fatty acids including arachidonic acid, palmitoleic acid, linoleic acid and 1-monopalmitin were significantly decreased in Mets model group. Unsaturated fatty acids can esterify and lower cholesterol, improve blood microcirculation and also were verified to be protective against insulin resistance. Thus, compared to the control group, the decreased unsaturated fatty acids level can be explained by the reduced level of HDL and elevated IR of rats in the Mets model group. These findings provide new clues for therapeutic strategies targeting fatty acid metabolic pathways. Based on the results from KEGG database analysis, arachidonic acid and linoleic acid simultaneously exist in the unsaturated fatty acid biosynthesis pathway.
The normal metabolism of amino acids is an important basis for life activities. Amino acids including serine, asparagines, L-allothreonine and alanine were important gluconeogenic precursors and played key roles in glucose–alanine cycle between tissues and liver.35 The level of these amino acids was significantly increased in the Mets model group, indicating the enhanced activity of gluconeogenesis (Fig. 4). Lysine is the ketogenic amino acid, which is degraded to acetyl-CoA and yield ketone bodies in the liver. In uncontrolled diabetes, the ability of lysine to form ketone bodies is especially pronounced. The occurrence of high levels of ketone bodies in the blood is known as ketoacidosis, which is common in patients with type 2 diabetes.36 Besides, the significantly elevated levels of ornithine and citrulline indicated that urea cycle disorders occurred in the Mets model group, which was generally characterized by the accumulation of glutamine and alanine. This can be observed by the significantly elevated levels of glutamine and alanine in the Mets model group. Most urea cycle disorders are associated with hyperammonemia, which further confirmed that the amino acid metabolism was perturbed in the Mets model group.37
After WDD treatment, 17 significantly altered metabolites in the Mets model group tended to be restored to the control levels by comparison with the normal control group (P > 0.05), including carbohydrates (e.g., 3,6-anhydro-D-galactose and threonic acid), amino acids (e.g., canavanine, citrulline, glucogenic amino acids such as alanine, L-allothreonine and asparagines, and ketogenic amino acids such as lysine), an important intermediate of TCA (succinate), and lipids (e.g., myo-inositol and palmitoleic acid). This indicated that WDD treatment tended to recover glycolysis, TCA cycle, urea cycle, amino acid and lipid metabolism, and inhibition of ketogenesis to the control level. Compared with the Mets model group, 8 metabolites were significantly changed after WDD treatment and the majority of them were decreased, including glycogenic amino acid such as glutamine, threo-beta-hyrdoxyaspartate and trans-4-hydroxy-L-proline, which contribute to decreasing gluconeogenesis. This can be observed by the reduction of HOMA-IR and serum insulin level of rats in the herb treated group.
After metformin treatment, 14 significantly altered metabolites in Mets model group tended to be restored to the normal levels by comparison with the normal control group (P > 0.05), including carbohydrates (e.g., 3,6-anhydro-D-galactose, calcium gluceptate, gluconic acid, lyxose and threonic acid), amino acids (e.g., citrulline, glutamic acid, L-allothreonine and canavanine), an important intermediate of TCA (oxalacetic acid), and lipids (e.g., D-glyceric acid). This indicated that metformin treatment also tended to adjust glycolysis, TCA cycle, urea cycle, amino acid and lipid metabolism to the control state. Compared with the model group, 5 metabolites were significantly decreased after metformin treatment.
The current study not only detected the different metabolites between groups, but also pinpointed the pathways in which these metabolites were involved. Based on both P value and impact value (IV) of metabolic pathway analysis, 5 metabolism pathways, including alanine, aspartate and glutamate metabolism, arginine and proline metabolism, glycine, serine and threonine metabolism, glyoxylate and dicarboxylate metabolism, and aminoacyl-tRNA biosynthesis, were identified as the significantly altered and strikingly perturbed pathways of Mets, which suggested occurrences of amino acid metabolism and carbohydrate metabolism disorders in Mets model rats.
After WDD treatment, alanine, aspartate and glutamate metabolism and taurine and hypotaurine metabolism were significantly altered and affected (P < 0.05 and IV > 0.1), indicating that these metabolic pathways were obviously altered and impacted by WDD. Thereby, treatment with WDD exhibited a significant benefit effect on regulating the amino acid metabolism and taurine metabolism disorders to normal state. After treated with metformin, three pathways including pentose phosphate pathway, arginine and proline metabolism and purine metabolism were involved but not obviously affected.
The docking results showed that a total of 70 compounds in WDD had high affinity with 4 enzymes, indicating that they competed with substrates to bind with these enzymes. Consequently, the serum levels of 3-hydroxyaspartic acid, trans-4-hydroxy-L-proline, glutamine and taurine were down-regulated in the herb treated group. The network analysis showed that glutamate decarboxylase had the largest degree (145) and betweeness (0.3741) among four enzymes. Generally, the larger a node's degree or betweenness is, the more important the node is in the interaction network.33 So, it can be concluded that glutamate decarboxylase was the most affected by WDD against Mets. Thereby, according to the different degrees of enzymes in the network, the degree of these enzymes affected by WDD was varied in order: glutamate decarboxylase > asparaginase > sulfinoalanine decarboxylase > aspartoacylase.
Conclusion
In this study, a systematic approach that integrated GC-TOF/MS-based metabolomics, multivariate statistical techniques (PCA and PLS-DA), KEGG pathway analysis and molecular docking simulation was successfully performed to discover the metabolic disturbances and pathways of Mets as well reveal the therapeutic mechanism of WDD against Mets. Our findings were as follows. First, 146 metabolites in the serum levels of four groups were matched and quantified based on GC-TOF/MS analysis. Second, the PLS-DA models showed the distinct separations between two comparison groups with high predictive abilities, respectively. Compared with the control group, 39 significantly altered metabolites (VIP > 1 and P < 0.05) including carbohydrates, amino acids, fatty acids and analogues were identified as potential biomarkers of Mets, associated with disruptions of glycolysis, TCA cycle, urea cycle, amino acid and lipid metabolisms, and promotion of gluconeogenesis and ketogenesis. Five metabolism pathways, including alanine, aspartate and glutamate metabolism, arginine and proline metabolism, glycine, serine and threonine metabolism, glyoxylate and dicarboxylate metabolism, and aminoacyl-tRNA biosynthesis, were identified as the significantly altered and strikingly perturbed pathways of Mets (P < 0.05 and IV > 0.1). Thirdly, compared with the normal control group, the serum levels of 17 and 14 significantly altered metabolites in the Mets model group tended to be restored to the control levels after two weeks treatment of Mets by WDD or metformin (P > 0.05), respectively, which involved in recovery of glycolysis, TCA cycle, urea cycle, amino acid and lipid metabolism, and inhibition of ketogenesis. Fourth, two significantly altered and impacted (P < 0.05 and IV > 0.1) metabolic pathways intervened by WDD against Mets were alanine, aspartate and glutamate metabolism and taurine and hypotaurine metabolism pathways. While, no significant pathway was found by treatment of metformin (P < 0.05). Fifth, the docking results demonstrated that 70 compounds in WDD competed with substrates to bind with 4 enzymes, including glutamate decarboxylase, asparaginase, sulfinoalanine decarboxylase and aspartoacylase, which led to reduction in the serum levels of significantly altered metabolites in the herb treated group. Overall, this study provided an effective and comprehensive approach for understanding the pathophysiological mechanisms of Mets and therapeutic mechanisms of WDD in treatment of Mets.
Author contributions
Meimei Chen performed experiments and wrote the manuscript; Fafu Yang designed the experiments; Jie Kang, Huijuan Gan, Xinmei Lai and Yuxing Gao helped experiments.
Conflicts of interest
The authors declare no conflicts of interest.
Acknowledgements
This work is supported by National Natural Science Foundation program of China (81503497, 21406036, and 8167151245), Fujian Provincial Natural Science fund subject of China (2015J01340 and 2017J01571), and Fujian Education Department of China: Fujian Provincial universities' incubation project for prominent young scientific researchers, and Fujian 2011 Chinese Medicine Health Management Collaboration Center of China.
References
- G. C. Moreira, J. P. Cipullo, L. A. Ciorlia, C. B. Cesarino and J. F. Vilela-Martin, PLoS One, 2013, 9, e105056 Search PubMed.
- Y. E. Wu, C. L. Zhang and Q. Zhen, Exp. Ther. Med., 2016, 12, 2390–2394 CrossRef PubMed.
- E. Martínezabundis, M. V. Méndezdel, K. G. Pérezrubio and L. Y. Zuñiga, World J. Diabetes, 2016, 7, 142–152 CrossRef PubMed.
- L. Huang, Q. Lv, D. Xie, T. Shi and C. Wen, Sci. Rep., 2016, 6, 22602 CrossRef CAS PubMed.
- Y. Huang, J. Xu, W. Ling, X. Zhang, Z. Dai, Y. Sui and H. Zhang, Altern. Ther. Health Med., 2015, 21, 54–67 Search PubMed.
- H. Sun, D. Wang, B. Wang, J. K. Wang, H. Liu, L. L. Guan and J. Liu, J. Proteome Res., 2015, 14, 1287–1298 CrossRef CAS PubMed.
- M. S. Monteiro, M. Carvalho, M. L. Bastos and D. P. P. Guedes, Curr. Med. Chem., 2013, 20, 257–271 CrossRef CAS PubMed.
- Y. Tao, X. Chen, H. Cai, W. Li, B. Cai, C. Chai, L. Di, L. Shi and L. Hu, J. Chromatogr. B: Anal. Technol. Biomed. Life Sci., 2016, 1040, 222–232 CrossRef PubMed.
- Z. Lin, C. M. Vicente Gonçalves, L. Dai, H. M. Lu, J. H. Huang, H. Ji, D. Wang, L. Yi and Y. Liang, Anal. Chim. Acta, 2014, 827, 22–27 CrossRef CAS PubMed.
- D. Ling, C. M. V. Gonçalves, L. Zhang, J. Huang, H. Lu and L. Yi, Talanta, 2015, 135, 108–114 CrossRef PubMed.
- M. Pérez-Enciso and M. Tenenhaus, Hum. Genet., 2003, 112, 581–592 Search PubMed.
- J. Du, Z. Yuan, Z. Ma, J. Song, X. Xie and Y. Chen, Mol. BioSyst., 2014, 10, 2441–2447 RSC.
- B. K. Shoichet, D. L. Bodian and I. D. Kuntz, J. Comput. Chem., 2010, 13, 380–397 CrossRef.
- Z. Chen, H. Gan, J. Kang, Y. Guo, J. Zhang and M. Chen, J. Liaoning Univ. Tradit. Chin. Med., 2017, 19, 75–77 Search PubMed.
- Z. Chen, J. Kang, Y. Guo, H. Gan and M. Chen, China J. Tradit. Chin. Med. Pharm., 2017 Search PubMed , in press.
- J. I. Felice, L. Schurman, A. D. Mccarthy, C. Sedlinsky, J. I. Aguirre and A. M. Cortizo, Diabetes Res. Clin. Pract., 2017, 126, 202–213 CrossRef CAS PubMed.
- National Pharmacopoeia Committee, Pharmacopoeia of the People's Republic of China. Part 1, Chemical Industry Press, Beijing, China, 2010 Search PubMed.
- W. B. Dunn, I. D. Wilson, A. W. Nicholls and D. Broadhurst, Bioanalysis, 2012, 4, 2249–2264 CrossRef CAS PubMed.
- M. Ugarte, M. Brown, K. A. Hollywood, G. J. Cooper, P. N. Bishop and W. B. Dunn, Genome Med., 2012, 4, 1–15 CrossRef PubMed.
- T. Kind, G. Wohlgemuth, D. Y. Lee, Y. Lu, M. Palazoglu, S. Shahbaz and O. Fiehn, Anal. Chem., 2009, 81, 10038–10048 CrossRef CAS PubMed.
- W. B. Dunn, D. Broadhurst, P. Begley, E. Zelena, S. Francis-Mcintyre, N. Anderson, M. Brown, J. Knowles, A. Halsall, J. Haselden, A. Nicholls, I. Wilson, D. Kell and R. Goodacre, Nat. Protoc., 2011, 6, 1060–1083 CrossRef CAS PubMed.
- H. Ashrafian, J. V. Li, K. Spagou, L. Harling, P. Masson, A. Darzi, J. K. Nicholson, E. Holmes and T. Athanasiou, J. Proteome Res., 2016, 13, 570–580 CrossRef PubMed.
- Z. Li, C. Lin, J. Xu, H. Wu, J. Feng and H. Huang, Anal. Biochem., 2015, 477, 105–114 CrossRef CAS PubMed.
- C. Ke, A. Li, Y. Hou, M. Sun, K. Yang, J. Cheng, J. Wang, T. Ge, F. Zhang, Q. Li, J. Li, Y. Wu, G. Lou and K. Li, Sci. Rep., 2016, 6, 23334 CrossRef CAS PubMed.
- J. Xia, N. Psychogios, N. Young and D. S. Wishart, Nucleic Acids Res., 2009, 37, 652–660 CrossRef PubMed.
- M. Kanehisa, S. Goto, Y. Sato, M. Furumichi and M. Tanabe, Nucleic Acids Res., 2012, 40, D109–D114 CrossRef CAS PubMed.
- Y. T. Tang and G. R. Marshall, Methods Mol. Biol., 2011, 716, 1–22 CAS.
- Editorial committee of State Administration of Traditional Chinese Medicine in China, Chinese MateriaMedica, Shanghai Scientific &Technical Publishers, Shanghai, China, 1st edn, 1999, pp. 30–9000 Search PubMed.
- J. Zhou, G. Xie and X. Yan, Traditional Chinese Medicines Molecular Structures, Chemical Industry Press, Beijing, China, 1st edn, 2004, pp. 10–1000 Search PubMed.
- M. Chen, F. Yang, X. Yang, X. Lai and Y. Gao, Int. J. Mol. Sci., 2016, 17, 2114 CrossRef PubMed.
- M. Chen, F. Yang, J. Kang, X. Yang, X. Lai and Y. Gao, Molecules, 2016, 21, 1639 CrossRef CAS PubMed.
- M. Chen, X. Yang, X. Lai, K. Jie, H. Gan and Y. Gao, Int. J. Mol. Sci., 2016, 17, 536 CrossRef PubMed.
- P. Shannon, A. Markiel, O. Ozier, N. S. Baliga, J. T. Wang, D. Ramage, N. Amin, B. Schwikowski and T. Ideker, Genome Res., 2003, 13, 2498–2504 CrossRef CAS PubMed.
- Y. Wang, Z. Liu, C. Li, D. Li, Y. Ouyang, J. Yu, S. Guo, F. He and W. Wang, Evid. Base Compl. Alternative Med., 2012, 2012, 69853 Search PubMed.
- Y. Nogusa, A. Mizugaki, Y. Hirabayashiosada, C. Furuta, K. Ohyama, K. Suzuki and H. Cobayashi, J. Nutr. Sci. Vitaminol., 2014, 60, 188–193 CrossRef CAS PubMed.
- M. Zhang, Y. Li, W. Cui, P. Yang, H. Li, C. Sheng, X. Cheng and S. Qu, Endocr. Pract., 2015, 21, 1364–1371 CrossRef PubMed.
- M. Mokhtarani, G. A. Diaz, W. Rhead, S. A. Berry, U. Lichter-Konecki, A. Feigenbaum, U. Lichter-Konecki, A. Feigenbaum, A. Schulze, N. Longo, J. Bartley, W. Berquist, R. Gallagher, W. Smith, S. E. McCandless, C. Harding, D. C. Rockey, J. M. Vierling, P. Mantry, M. Ghabril, R. S. Brown Jr, K. Dickinson, T. Moors, C. Norris, D. Coakley, D. A. Milikien, S. C. Nagamani, C. LeMons, B. Lee and B. F. Scharschmid, Mol. Genet. Metab., 2013, 110, 446–453 CrossRef CAS PubMed.
Footnote |
† Electronic supplementary information (ESI) available. See DOI: 10.1039/c7ra09779d |
|
This journal is © The Royal Society of Chemistry 2017 |
Click here to see how this site uses Cookies. View our privacy policy here.