DOI:
10.1039/C7RA09465E
(Paper)
RSC Adv., 2017,
7, 55044-55050
The diffusion behavior and capacitance of tetraethylammonium/tetrafluoroborate ions in acetonitrile with different molar concentrations: a molecular dynamics study
Received
26th August 2017
, Accepted 24th November 2017
First published on 4th December 2017
Abstract
A molecular dynamics (MD) simulation with the optimized potentials for liquid simulations-all atom (OPLS-AA) force field was carried out to investigate the dynamic behaviors of organic electrolyte molecules between a graphite cathode and anode. This study considered the tetraethylammonium cation (NEt4+) and tetrafluoroborate anion (BF4−) in acetonitrile (ACN) solvent. The predicted NEt4–BF4 solution density at 1 M from the MD isothermal–isobaric ensemble (NPT) is about 0.861 g cm−3, which is very close to the corresponding experimental value. This indicates that the OPLS-AA force field can accurately describe the interactions between these molecules. The detailed diffusion mechanism and the corresponding viscosity solution for different NEt4–BF4 mole fractions were explored. The charge density distribution of electrolyte molecules between the graphite cathode and anode from MD simulation was further used to obtain the potential drop by solving the Poisson equation and to obtain system capacitance. This study provides a method to determine the proper molar concentration of electrolyte NEt4–BF4 in ACN solution which can balance ionic conductivity and capacitance to enhance supercapacitor performance.
Introduction
In recent years, along with the development of portable electronic devices, energy storage has become a crucial issue for such products. Batteries and capacitors are the most commonly used power sources in commercial markets, with the Li ion battery and electric double-layer capacitor (EDLC) being the most popular products. A typical Li ion battery can generate electricity through chemical reactions, but such reactions not only result in a reduction in cycle-life but also safety concerns. For an EDLC, the charge storage is through the electrostatic attraction between the ions of the electrolyte and electrode surface, which allows the formation of oppositely charged layers at the interface, a more attractive and safer option for power sources. EDLCs are essentially maintenance-free, possessing a longer cycle-life and no memory effect due to their simple physical charging circuit. A low energy density is the weaknesses of EDLCs, but currently porous activated carbon has been used as an electrode material, as it has a high surface area at the electrode/electrolyte interface which can enhance the energy density to around 25.5 W h kg−1.1 Such a device is called a supercapacitor. Though the energy density of the supercapacitor is still lower than a Li ion battery (250–693 W h kg−1),2 its high reliability and high charging and discharging rates support many applications, especially in electric vehicles.
In an EDLC supercapacitor, energy density, or capacitance, relies on the physical adsorption of the electrolyte on the surface of the electrode to separate the oppositely polarized ions from each other. Therefore, previous research on EDLCs has mainly focused on the electrode material and enhancements of electrolyte adsorption ability. Those strategies include increasing the surface area,3 enhancing either the functionality of electrode4 or the adsorption properties of the electrolyte.5 However, the charging and discharging rate and operating voltage of EDLC are also key elements for the production of a high quality supercapacitor and are highly related to the type of electrolyte used. The typical EDLC electrolytes are classified into three categories: aqueous, organic, and ionic liquids. For aqueous electrolytes, good ion fluidity leads to a high charging rate, but an operating voltage limited to ∼1 V before water decomposes.6 However, room temperature ionic liquids with operating voltages as high as 4 V are achievable,6–9 but their slower ionic transport results in poorer power performances. The most widely used systems are comprised of salts dissolved in organic solvents (e.g., tetraethylammonium tetrafluoroborate in acetonitrile solvent, NEt4–BF4/ACN). Such organic electrolytes offer a good balance of relatively large maximum operating voltages (∼2.5 V) and high ionic conductivities (∼20–60 mS cm−1)10. However, properly adjusting ion concentrations is a tricky and crucial problem in organic electrolytes, because a high ion concentration which enhances the capacitance of EDLC will also increase the viscosity of the electrolyte and result in a low charging rate. To obtain the proper ratio of organic ions and solvent, significant trial and error testing may be executed in experiments, with results obtained at high cost. However, the numerical method and simulations have been shown to be a more efficiency pathway to solve such component mixing ratio optimization problems.10 A number of studies have been published which aim to predict fluidic behavior of electrolytes by molecular simulation. Wander et al. analyzed on a microscopic scale the diffusion and concentration of an electrolyte containing alkali metal and halide ions.11 Kalugin et al. used the molecular dynamics (MD) simulation to compare flow behavior of liquid acetonitrile and water in carbon nanotubes.12 Feng et al. performed an MD examination of the structure, capacitance and dynamics of electrolyte NEt4–BF4 ions in ACN in EDLCs.13 MD simulation was used to investigate the alternative ion layers which are a result of the overscreening effect near the charged electrode, and the obtained capacitance was also in good agreement with experiments. Vatamanu et al. combined the BF4 anion with various cations as an ionic liquid in ACN solvent with different electrode structures, investigating those structural properties by classical atomistic simulations.14 They showed that such ionic liquid electrolyte solutions can behave quite differently from their pure ionic liquid form.
Though there are many related studies of electrolyte NEt4–BF4 in ACN solution, their molar concentration range is generally narrow (usually less than 1.5 M). In addition, these studies have also lacked discussion about electric double-layer morphologies and capacitances of the electrolyte NEt4–BF4 in ACN with different concentrations. For obtaining EDLCs with high performance, some previous studies indicate that the choice of ionic liquid electrolyte should not only consider the ionic concentration but also the ionic conductivity. According to the experimental works by Bozym,15 the capacitance proportionally increase when solutions were diluted by solvents. In Burt's study,16 they measured the capacitance of ionic liquid electrolyte with different electrolyte concentrations by the experiments. The diffusion behavior of electrolytes was also carried out by molecular simulation for explaining the relationship between the ionic conductivity and capacitance. Thus, we first predict the ion diffusion ability of the organic electrolyte NEt4–BF4 ion salt dissolved in ACN for different molar concentrations by MD simulation. Furthermore, because it is difficult to directly observe the interaction between electrode and electrolyte at interface in experiments, the constant potential charge method17 and EDLC models were constructed to investigated the adsorption, ion distribution and theoretical capacitance of electrolytes in different molar concentrations. This approach can elucidate the relationship between electrolytic molar concentration and dynamic behaviors, revealing sufficient information to determine and fine tune the mixing ratios of ion salts and solvents which aid development of high quality EDLC supercapacitors.
Simulation method
The measured density of NEt4–BF4/ACN solution at 1 M is about 0.861 g cm−3.18 According to this density, the molar ratio of ion pairs over the solvent is about 1
:
15. Consequently, we can further adjust the molar ratio of ion pairs over solvent molecule to get the solution with the specific molar concentration. Four molar concentrations of NEt4–BF4 salt in ACN solvent were prepared as electrolytes in this study, the number of cations, anions and solvents are organized in Table 1. The molecular model of these four species were shown in Fig. 1(a). According the equilibrium densities of these four models, which molar concentration is 1.03 M, 1.50 M, 2.00 M and 2.99 M, respectively, and were labeled as 1 M, 1.5 M, 2 M and 3 M NEt4–BF4 solution. For each model, the optimized potentials for liquid simulations-all atom (OPLS-AA) force field was used to describe the interaction between atoms. For non-bonding interactions, the sum of site–site Lennard-Jones potential is represented as van der Waals force, and coulombic interactions are also considered. The cutoff distance of non-bonding interaction is set as 12.5 Å and long-range coulombic interaction are evaluated by the PPPM method.19 The parameters of OPLS-AA were obtained from previous studies, with Sambasivarao and Acevedo20 and Price et al.21 determining them by ab initio-derived geometries of ionic liquid and solvent as reference data. According to their studies, the OPLS-AA parameters were fitted for various ionic liquid and solvent compounds. The results show that those parameters not only represent accurate material densities, but also that the heats of vaporization and heat capacities are also in excellent agreement with experimental values. In MD simulation, the Nosé–Hoover thermostat and barostat are used to control the temperature and the pressure of the system and are carried out by the LAMMPS package.22
Table 1 Electrolyte compositions
Models |
1 M NEt4–BF4/ACN |
1.5 M NEt4–BF4/ACN |
2.M NEt4–BF4/ACN |
3 M NEt4–BF4/ACN |
NEt4 molecules |
40 |
65 |
100 |
215 |
BF4 molecules |
40 |
65 |
100 |
215 |
ACN molecules |
600 |
600 |
600 |
600 |
Equilibrium density |
0.856 (g cm−3) |
0.893 (g cm−3) |
0.927 (g cm−3) |
0.991 (g cm−3) |
Molar concentration |
1.03 M |
1.50 M |
2.00 M |
2.99 M |
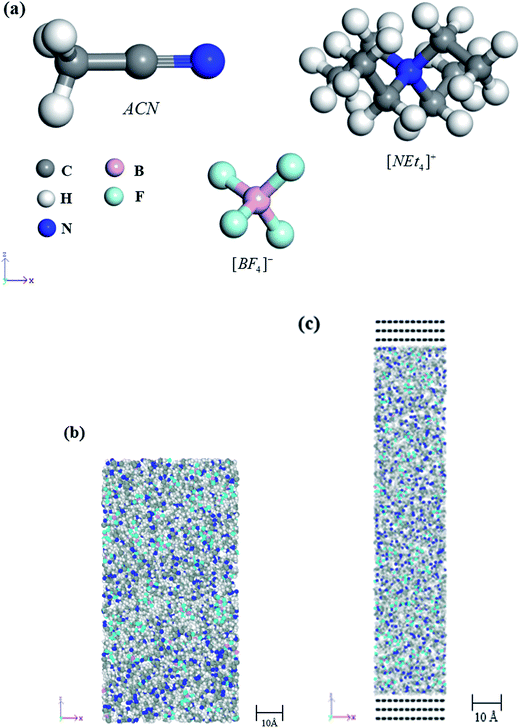 |
| Fig. 1 The all-atom model of this study: (a) the molecular structure of tetraethylammonium cation (NEt4+) and tetrafluoroborate anion (BF4−) and acetonitrile (ACN). (b) The structure of amorphous model. (c) The structure of EDLC model. | |
There are two types of simulation models which are constructed in this study. The first are amorphous models of NEt4–BF4 salt in ACN solvent with three different molar concentrations, which are used to evaluate the diffusion behavior of electrolytes, as shown in Fig. 1(b). In order to obtain the stable configuration and proper density of each amorphous model, the following steps were performed by MD simulations. (1) According to the molar concentration of the electrolyte, the specific number of NEt4–BF4 ion pairs and ACN molecules were randomly placed in a large simulation cell with 3D periodic boundary conditions. (2) The systems were compressed so that their corresponding densities approached 80% of the experimental value. This setting can ensure that there is sufficient space to follow the annealing procedure. (3) Then the system was annealed from 800 K to 400 K by MD in the NVT ensemble for 1 ns with the time step set as 1 fs. (3) Finally, 500 ps of MD simulation in the NPT ensemble was performed, with the temperature and pressure controlled at 300 K and 1 atm. The densities of equilibrium for 1 M, 1.5 M, 2 M and 3 M NEt4–BF4/ACN models were 0.856 g cm−3, 0.893 g cm−3, 0.927 g cm−3 and 0.991 g cm−3, respectively. The predicted density of 1 M NEt4–BF4/ACN model is very close to the experimental value 0.861 g cm−3,18 which confirms that the chosen force field parameters and simulation methods in this study are reasonable.
To investigate the dynamic behavior and interaction between electrode and electrolyte, the second model type, that of EDLC, were also constructed for three NEt4–BF4/ACN electrolytes of different molar concentrations. In the EDLC model, the electrolyte solution was placed between symmetric three layer graphene electrodes as shown in Fig. 1(c), which was consistent with several previous studies.23 In this model, the directions which are vertical to the electrode are periodic, and each graphene electrode contains 720 carbon atoms and has 2.45951 × 2.55599 nm2 contact area with the electrolyte. The distance between two electrodes are adjusted so as to make the volume of electrolytes consistent with equilibrium densities which have been predicted by previous amorphous models. To represent the charging environment of supercapacitors, two methods have been commonly used in previous studies, those of the fixed charge method (FCM)24 and the constant potential method (CPM).25 The principle behind these two methods are applying extra charges at individual electrode atoms and adjusting the specific charge density of the electrode in order to maintain a specific electric potential. The difference between these two methods is that the extra charges which are applied to the electrode are constant in the FCM method but dynamically adjusted in the CPM method during molecular dynamics simulations. A previous study26 has indicated that the charge distribution on the ideal conductive electrode is approximated by discrete point charges centered on the electrode itself, so a small amount of charge is found on the second layer and to a much lesser extent the third. Unlike the FCM, the electrode charges in the CPM can adjust to respond to local fluctuations in the electrolyte/ion charge density, which may lead to the results being close to actual conditions. Based on the CPM method, three molar concentrations of NEt4–BF4/ACN electrolyte were charged under 3 V of electric potential in EDLC models, which is consistent with the operating voltage range of such electrolytes. In order to reduce the MD computational time for this CPM method, a similar annealing procedure was also applied in these EDLC models to push the system more quickly to equilibrium. Finally, each EDLC model was further relaxed for 1 ns by MD with the CPM method.
Results
The self-diffusion coefficient of electrolyte ions in different molar concentrations
To investigate the self-diffusion behavior of the electrolyte ions, the mean square displacements (MSD) of the center of mass (COM) of the NEt4 cations and BF4 anions were calculated to obtain the corresponding self-diffusion coefficients of ions in ACN environment. The MSD is calculated according to eqn (1): |
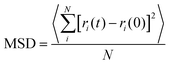 | (1) |
where ri(0) is the initial COM position vector of the ion, ri(t) represents the COM position vector of the ion after t time and N is the total number of ions in the system. The equilibrium amorphous model of three electrolyte with three molar concentrations were further equilibrated by MD in the NVT ensemble at 300 K for 1.2 ns, and the MSD values were measured over the last 200 ps. The profiles of MSD values with simulation time are shown in Fig. 2, where all the MSD curves become linear after 50 ps. It is known that the MSD profile is linear to the delay time over the long-time limit, and thus the self-diffusion coefficients D can be derived from the slopes of MSD profiles after a longer delay time by the Einstein equation (eqn (2)):27,28 |
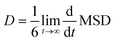 | (2) |
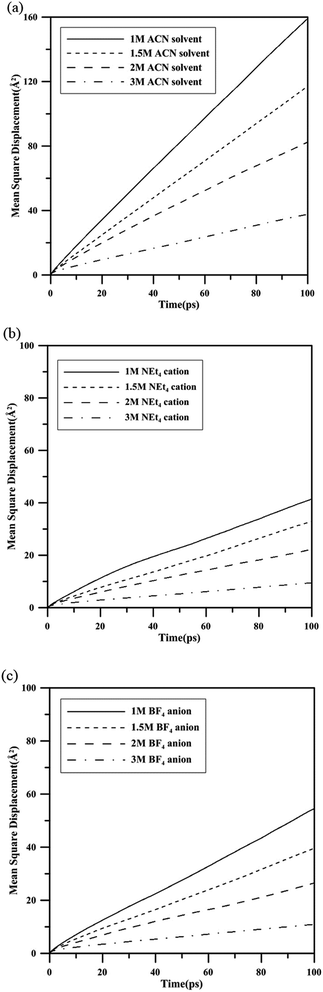 |
| Fig. 2 The mean square displacements of (a) ACN solvent, (b) NEt4 cation and (c) BF4 anion with different molar concentrations. | |
The self-diffusion coefficient of NEt4 cation and BF4 anion were organized in Table 2, which shows that the BF4 anion exhibits a higher diffusion speed than the NEt4 cation. This may be due to the larger structural size of NEt4 and strong solvation effect of ACN solvent with the cation. The diffusion coefficient of BF4 anion decreases severely when the molar concentration of electrolyte increases. This may be due to a weakening ability of the solvent to screen the electrostatic interactions with cations, causing BF4 anions to become trapped. In the 3 M NEt4–BF4/ACN solution, there is no significant difference in diffusion coefficients for these two ions. The low ion self-diffusion coefficient value in 3 M NEt4–BF4/ACN indicates that this solution is very viscous and reveals bad ion transportability in this solvation environment. This ability may be directly proportional to the formation or exchange rate of electric double layers on the electrode surface.
Table 2 The self-diffusion coefficient of BF4 anion and NEt4 cation in ACN solvent with 1 M, 2 M and 3 M molar concentrations at 300 K
Diffusion coefficients (D) D × 10−10 (m2 s−1) |
|
ACN |
BF4 |
NEt4 |
1 M |
26.000 |
8.672 |
5.950 |
1.5 M |
19.150 |
6.200 |
5.233 |
2 M |
13.150 |
3.770 |
3.350 |
3 M |
5.950 |
1.567 |
1.370 |
Investigation of electrolyte at the interface under electric potential
For investigating the dynamical behavior of the electrolyte in EDLC, 1 V, 2 V and 3 V electric potentials were applied to the EDLC model and the opposite charge were distributed at the electrode anode and cathode. The average number density distribution profiles of NEt4 cations, BF4 anions and ACN solvents are shown in Fig. 3. Before the electrodes started to be charged, the ions were distributed evenly, as in Fig. 3(a). The NEt4 has stronger adsorption than BF4 anions and shows higher distribution at the electrode interface. The 2 M and 3 M electrolyte solutions exhibit similar distribution at this stage and therefore are omitted here. Fig. 3(b)–(d) shows ionic distribution profiles of 1 M solution which were charged under 1 V, 2 V and 3 V electric potential, respectively. The electrostatic interaction inducing the ions of electrolyte move toward the electrode with opposite charge and redistribute when the system approaches equilibrium. The average number density of 1 M NEt4–BF4/ACN solution shows that the ions are mainly distributed at the interface of electrodes and were adsorbed upon the electrodes with opposite charge, as in Fig. 3(b). The solvents and first adsorption layer of NEt4 were exchanged by the BF4 anion at the anode interface. The number density of the first adsorption layer of BF4 at the anode is higher than that of the first adsorption layer of NEt4 at the cathode, due to the smaller size of anion. In addition, the alternating layers of ions with opposite charge can also be seen at the interface of electrodes in this profile, which is the so-called overscreening effect.26 This phenomenon shows that the charge of the electrode is shielded by the charge of the first adsorbed layer, with the exceeding charge inducing the opposite ions to form a second adsorbed layer, and so on. Fig. 3(c) shows the ion distribution of the 1 M solution under 2 V electric potential; the number density of cations at the cathode is similar to that under 1 V, but the number density of anions has slightly enhanced at the anode. This means that the ions do not cover the electrodes completely under such a voltage. When the EDLC had been charged to 3 V, as shown in Fig. 3(d), both cation and anion approach maximum number at the electrode interface. This phenomenon indicates that complete electric double layers may form under such a voltage, which is close to the ultimate working voltage of such an electrolyte solution. The average number density distribution profile of 1 M, 2 M and 3 M electrolyte solution under 3 V electric potential is compared in Fig. 3(d)–(f). The results show that the cations reach their maximum adsorption number of 5.0 NEt4 per Å2 in the 2 M solution, while anions do not reach 5.0 BF4 per Å2 until the concentration increases to 3 M. The results suggest that the NEt4 exhibits stronger competitive ability with the ACN solvent than does the BF4 anion. According to the distribution profile of the 3 M electrolyte solution under 3 V electric potential, as Fig. 3(f), the highly distribution of ions at the central area of the EDLC model reveal that the ions aggregate together, making it difficult to transport forward to the electrode surface. Furthermore, the overscreening effect becomes heavy in such high molar concentration solutions, and may prevent more ions approaching the electrodes with opposite charge. This may explain why a limited number of ions can be adsorbed on the electrode surface.
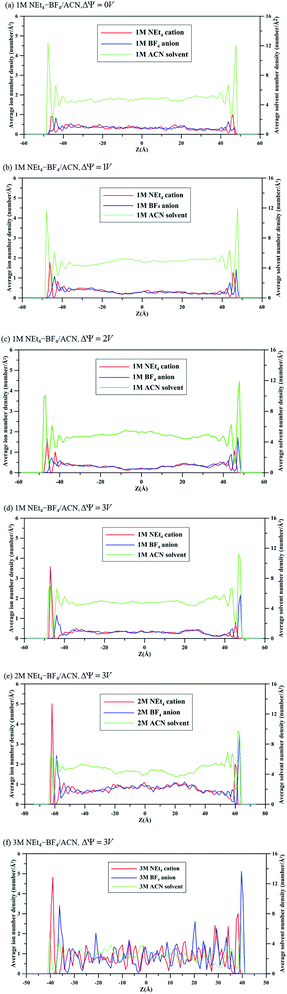 |
| Fig. 3 The average number density distribution of 1 M NEt4–BF4/ACN under (a) 0 V, (b) 1 V, (c) 2 V and (d) 3 V electric potential and (e) 2 M NEt4–BF4/ACN, and (f) 3 M NEt4–BF4/ACN solution, both under 3 V electric potential. | |
To quantitatively determine the difference in energy storage ability of electrolytes with different molar concentrations, the capacitances of 1 M, 2 M and 3 M NEt4–BF4/ACN solutions were calculated. The capacitance of EDLC can be obtained by electric potential field ψ(r) in the electrolyte between the electrodes. Calculations of ψ(r) in simulation of EDLCs are typically determined from the charge density field ρ(r) by numerically solving Poisson's equation29 as shown in eqn (3):
|
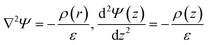 | (3) |
where
ε is the dielectric constant,
z is the direction normal to the planar electrodes and
ρ(
z) is obtained from
ρ(
r) by averaging over the plane parallel to electrode surface (
xy). In previous studies,
30 most methods to calculate the 1d electric potential profile from simulation are based on numerically solving the 1d Poisson's equation. In this work, one relatively simple and directly determine method were used, probe and average (PA) method, which was developed by Wang
et al.17 Based on the PA method, the simulation cell is overlaid with an evenly distributed mesh grid and the electric potential at each grid point, which is treated as a probe, is calculated according to
|
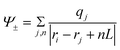 | (4) |
where
ri and
qi are the coordinate and charge of an atom,
ri is the coordinate of a grid and
nL is a displacement vector for different images. This method provides the full 3d electrostatic potential field evaluated on the discrete grid points at any time step, and the long range coulombic interactions are also considered and determined by Ewald summation.
31,32 The 3d averaged electrostatic potential function
Ψ(
r) are calculated by time averaged over the trajectory of MD simulations. The differential capacitances for anode and cathode are calculated from the differentiation of the surface charge with respect to potential drops:
|
 | (5) |
where
σS is surface charge of electrode, and Δ
Ψ± is the potential drops between the potential of electrode and the bulk screened electrolyte region.
33,34 For the symmetric EDLC model, the total capacitance
Ctotal can be obtained from the differential capacitance of anode
C+ and cathode
C− by
eqn (6):
|
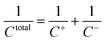 | (6) |
The total capacitance of our EDLC models and corresponding differential capacitances of anode and cathode under electric potential are organized in Table 3. At the cathode electrode, the 2 M and 3 M of NEt4–BF4 solution can store 18.62% and 31.57% more charge than the 1 M solution, respectively, due to high ion concentration. However, in 2 M and 3 M, the differential capacitance at the anode is surprisingly lower than that in 1 M because of the strong electrostatic interaction between ions and the strong overscreening effect at high molar concentrations. These results are consistent with previous average number density distribution analysis. Finally, the total capacitance increases along with the ion concentration, with results showing that the 2 M and 3 M of NEt4–BF4 solution can theoretically store about 5.92% and 9.02% more charge, respectively, than the 1 M solution.
Table 3 The differential capacitance at cathode (C−), anode (C+) and total capacitance (Ctotal) of 1 M, 2 M and 3 M NEt4–BF4/ACN under electric potential in EDLC model
Electrolyte |
C− (μF cm−2) |
C+ (μF cm−2) |
Ctotal (μF cm−2) |
1 M NEt4–BF4/ACN |
3.497 |
5.431 |
2.128 |
2 M NEt4–BF4/ACN |
4.148 |
4.938 |
2.254 |
3 M NEt4–BF4/ACN |
4.601 |
4.679 |
2.320 |
Conclusions
This study investigates the diffusion property of NEt4–BF4/ACN electrolyte solutions with different molar concentrations. In addition, different charging structures and morphologies near the electrode interface were analyzed. The BF4 anion exhibits a higher diffusion speed than the NEt4 cation due to smaller structural size and the strong solvation effect of ACN solvent with NEt4 cation. The diffusion coefficient of BF4 anion decreases severely when the molar concentration of electrolyte increases. Before the electrodes started to be charged, the NEt4 cation has stronger adsorption than BF4 anion and shows a higher distribution at the electrode interface. The 3 V EDLC charging models show that the cations reach maximum adsorption number of 5.0 NEt4 per Å2 in the 2 M solution, while anions do not reach 5.0 BF4 per Å2 until the concentration rises to 3 M. These results suggest that the NEt4 exhibits stronger competitive ability with the ACN solvent than does the BF4 anion. The NEt4–BF4 ion pairs cannot be completely separated by solvent and aggregate together in the 3 M solution, as well as suffering from a heavy overscreening effect. This phenomenon causes the electrode to be unable to adsorb more ions from the bulk region, such that its capacitance can theoretically store only 9.02% more charge than can the 1 M solution.
Conflicts of interest
There are no conflicts to declare.
References
- P. Simon and Y. Gogotsi, Acc. Chem. Res., 2012, 46, 1094–1103 CrossRef PubMed.
- T. Placke, R. Kloepsch, S. Dühnen and M. Winter, J. Solid State Electrochem., 2017, 1–26 Search PubMed.
- J. Varghese, H. Wang and L. Pilon, J. Electrochem. Soc., 2011, 158, A1106–A1114 CrossRef CAS.
- A. D. DeYoung, S.-W. Park, N. R. Dhumal, Y. Shim, Y. Jung and H. J. Kim, J. Phys. Chem. C, 2014, 118, 18472–18480 CAS.
- S. Wang, S. Li, Z. Cao and T. Yan, J. Phys. Chem. C, 2009, 114, 990–995 Search PubMed.
- C. Zhong, Y. Deng, W. Hu, J. Qiao, L. Zhang and J. Zhang, Chem. Soc. Rev., 2015, 44, 7484–7539 RSC.
- F. Béguin, V. Presser, A. Balducci and E. Frackowiak, Adv. Mater., 2014, 26, 2219–2251 CrossRef PubMed.
- A. Brandt, S. Pohlmann, A. Varzi, A. Balducci and S. Passerini, MRS Bull., 2013, 38, 554–559 CrossRef CAS.
- A. Lewandowski, A. Olejniczak, M. Galinski and I. Stepniak, J. Power Sources, 2010, 195, 5814–5819 CrossRef CAS.
- A. C. Forse, C. Merlet, J. M. Griffin and C. P. Grey, J. Am. Chem. Soc., 2016, 138, 5731–5744 CrossRef CAS PubMed.
- M. C. Wander and K. L. Shuford, J. Phys. Chem. C, 2010, 114, 20539–20546 CAS.
- O. N. Kalugin, V. V. Chaban, V. V. Loskutov and O. V. Prezhdo, Nano Lett., 2008, 8, 2126–2130 CrossRef CAS PubMed.
- G. Feng, J. Huang, B. G. Sumpter, V. Meunier and R. Qiao, Phys. Chem. Chem. Phys., 2010, 12, 5468–5479 RSC.
- J. Vatamanu, M. Vatamanu, O. Borodin and D. Bedrov, J. Phys.: Condens. Matter, 2016, 28, 464002 CrossRef PubMed.
- D. J. Bozym, B. l. Uralcan, D. T. Limmer, M. A. Pope, N. J. Szamreta, P. G. Debenedetti and I. A. Aksay, J. Phys. Chem. Lett., 2015, 6, 2644–2648 CrossRef CAS PubMed.
- R. Burt, K. Breitsprecher, B. Daffos, P.-L. Taberna, P. Simon, G. Birkett, X. Zhao, C. Holm and M. Salanne, J. Phys. Chem. Lett., 2016, 7, 4015–4021 CrossRef CAS PubMed.
- Z. Wang, D. L. Olmsted, M. Asta and B. B. Laird, J. Phys.: Condens. Matter, 2016, 28, 464006 CrossRef PubMed.
- Z.-a. Zhang, Y.-q. Lai, J. Li and Y.-x. Liu, J. Cent. South Univ. Technol., 2009, 16, 247–252 CrossRef CAS.
- U. Essmann, L. Perera, M. L. Berkowitz, T. Darden, H. Lee and L. G. Pedersen, J. Chem. Phys., 1995, 103, 8577–8593 CrossRef CAS.
- S. V. Sambasivarao and O. Acevedo, J. Chem. Theory Comput., 2009, 5, 1038–1050 CrossRef CAS PubMed.
- M. L. P. Price, D. Ostrovsky and W. L. Jorgensen, J. Comput. Chem., 2001, 22, 1340–1352 CrossRef CAS.
- S. Plimpton, J. Comput. Phys., 1995, 117, 1–19 CrossRef CAS.
- S. Li, P. Zhang, F. F. Pasquale, C. H. Patrick, G. Feng, S. Dai and T. C. Peter, J. Phys.: Condens. Matter, 2014, 26, 284105 CrossRef PubMed.
- S. K. Reed, O. J. Lanning and P. A. Madden, J. Chem. Phys., 2007, 126, 084704 CrossRef PubMed.
- Z. Wang, Y. Yang, D. L. Olmsted, M. Asta and B. B. Laird, J. Chem. Phys., 2014, 141, 184102 CrossRef PubMed.
- C. Merlet, M. Salanne, B. Rotenberg and P. A. Madden, Electrochim. Acta, 2013, 101, 262–271 CrossRef CAS.
- M. Meunier, J. Chem. Phys., 2005, 123, 134906 CrossRef CAS PubMed.
- H.-L. Chen, S.-P. Ju, T.-Y. Wu, J.-Y. Hsieh and S.-H. Liu, RSC Adv., 2015, 5, 26316–26320 RSC.
- G. Sköllermo, Math. Comput., 1975, 29, 697–711 CrossRef.
- Y. Shim, H. J. Kim and Y. Jung, Faraday Discuss., 2012, 154, 249–263 RSC.
- A.-K. Tornberg, Adv. Comput. Math., 2016, 42, 227–248 CrossRef.
- M. Kawata and M. Mikami, Chem. Phys. Lett., 2001, 340, 157–164 CrossRef CAS.
- R. Parsons, Pure Appl. Chem., 1974, 37, 499–516 CrossRef CAS.
- A. J. Bard and L. R. Faulkner, Electrochemical Methods: Fundamentals and Applications, John Wiley & Sons, Inc., New York, 2nd edn, 2001 Search PubMed.
|
This journal is © The Royal Society of Chemistry 2017 |
Click here to see how this site uses Cookies. View our privacy policy here.