DOI:
10.1039/C7RA07651G
(Paper)
RSC Adv., 2017,
7, 48136-48150
Study on alterations of physiological functions in aged constipation rats with fluid-deficiency based on metabonomic and microbiology analysis†
Received
12th July 2017
, Accepted 2nd October 2017
First published on 12th October 2017
Abstract
Constipation, a common and complex clinical symptom, is a predisposing factor for many serious diseases, such as gastrointestinal diseases, cardiovascular diseases and cancers. Some reports suggest that constipation is associated with gut microbiota, but the specific mechanism is unknown. To clarify how constipation interferes with the normal physiological function of organisms, 1H NMR profiles combined with polymerase chain reaction-denaturing gradient gel electrophoresis (PCR-DGGE) and 16s rRNA gene sequencing were used to investigate the relationship among constipation, metabolism of gut microbiota and host. 27 urinary metabolites and 22 faecal metabolites were found to be associated with constipation, which affect the metabolism of energy, butyric acid, choline, amino acid and the functions of liver and kidney. 5 biomarkers were screened to diagnose the constipation based on the receiver operating characteristic (ROC) analysis. Meanwhile, the levels of Lactobacillus, Intestinimonas, Muribaculum, Eubacterium were significantly decreased, whereas levels of Actinomyces, Culturomica, Ruminococcus, Prevotella, Ruminiclostridium and Acinetobacter were significantly increased. Moreover, the above altered microbiota showed a strong correlation with the metabolites of short chain fatty acids, amino acids, bile acids, choline, and the intermediate product of tricarboxylic acid cycle. These results implied that constipation could result in intestinal dysbacteriosis and metabolism disorder, thereby affecting some normal physiological functions in host. The relationship between gut microbiota and metabolites revealed the potential hazard of constipation from the perspectives of metabonomic and microbiology, and provided new avenues for the diagnosis of constipation and potential drug targets in preventing related diseases.
Introduction
Constipation is a highly common functional gastrointestinal disorder in the clinic with a broad spectrum of symptoms such as difficult defecation, abdominal discomfort, bloating sensation,1 pelvic pain and nausea.2 Population-based studies reported that the incidence of constipation ranged from 2% to 27%.3–6 Moreover, the prevalence of constipation is also proportional to age and sex, older people and women are more likely to suffer from constipation.7–9 Although constipation is not life-threatening, it can cause many serious diseases, such as gastrointestinal diseases, cardiovascular diseases and even cancers. Nevertheless, there are only few reports of mechanisms about how constipation affect the organism. Only understand the relationship between constipation and related diseases, people can find new avenues to cure constipation and prevent the development of other diseases.
In recent years, the existence of intestinal microecology associated with intestinal physiological and pathological processes has been gradually recognized. Intestinal microecological imbalance may lead to gastrointestinal dysfunction and changes of visceral nerves.10,11 Some studies found that the composition of gut microbiota in constipated patients was different from healthy people, suggesting that the gut microbiota might be one of the most important part in inducing constipation.12–14 The changes of intestinal flora in patients with constipation are characterized by the relative decrease of specific bacteria and the parallel increase of potential pathogenic microorganisms and fungi.15,16 The research by Kim et al.17 and Feng X. et al.18 suggested that Bifidobacterium, Lactobacillus and Methanogens significantly decreased in feces of constipated patients compared with healthy controls. The result was partially consistent with previous studies which analyzed the faecal microbiota in patients with functional constipation.19,20 The previous studies are helpful in providing valuable data on the interaction between constipation and gut microbiota. However, the mechanisms that gut microbiota affect the functions of the organism are still unknown. Some scholars speculated that the co-metabolites produced from the interaction of host and the gut microbiota might affect the potential mechanisms of intestinal microflora associated with constipation. So, figuring out the relationship among gut microbiota, host and metabolites may help to understand the potential hazard of constipation.
Nowadays, metabonomics coupled with microbiology analysis has been extensively used to investigate the correlation between the host and gut microbes.21,22 Metabonomics-based approach, which is capable of measuring the dynamic multi-parametric metabolic responses in living systems, are attractive platforms for metabolic profiling monitoring and new diagnostic biomarkers discovery.23 Numerous studies also proved that 1H NMR-based metabonomics is a promising technique for metabonomics study, which could provide rapid analysis with efficient resolution.24 Nicholson et al.25–27 applied 1H-NMR technique to demonstrate that the metabolic variations in gastrointestinal compartments, mammalian tissues from kidney and liver, and biofluids such as urine and blood are directly correlated to the activities of various microorganisms that inhabit in the gut. In addition, polymerase chain reaction (PCR) and denaturing gradient gel electrophoresis (DGGE) of the V3 region of the 16S ribosomal ribonucleic acid (16S rRNA), which were biologically common method in analyzing intestinal flora, are employed to describe the substantial diversity of the gut microbiome and quantify the complex microbial communities,28 while 16S rRNA gene sequences is utilized for identifying the microbiota.
Here, we reported a profiling study of urinary and faecal metabolites in aged constipated rat model with fluid-deficiency through 1H-NMR, and 16S rRNA V3 gene PCR-DGGE combined with sequencing technique to monitor the fluctuations and identify the species in gut microbiota. In addition, urinary and faecal samples were collected at different time points to characterize the dynamic metabolic profiles of the model. Finally, we investigated the correlation between gut microbiota and metabolites. Exploring the information is particularly important in revealing the symbiotic relationships between the host and gut microbes and helping to improve the understanding of molecular mechanisms of host–microbe interactions at multiple levels (Fig. 1).
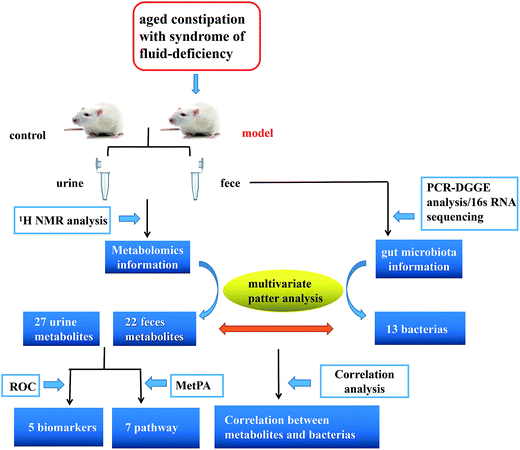 |
| Fig. 1 Scheme of the study. | |
Materials and methods
Reagents and materials
Deuterium oxide with 0.05% 3-trimethylsilyl-(2,2,3,3-2H4)-1-propionate (TSP) (D2O, 99.9%) was purchased from Sigma-Aldrich (St. Louis, MI, USA). The assay kits for superoxide dismutase (SOD) and malonyldialdehyde (MDA) were purchased from Nanjing Jiancheng Bioengineering Institute (Nanjing, P. R. China). Loperamide hydrochloride was purchased from Xian Janssen Pharmaceutical Ltd (Shanxi, P. R. China). D-Galactose was purchased from Shanghai Macklin Biochemical Co. Ltd (Shanghai, P. R. China). All other used chemicals were of analytical grade.
Animal treatment
The whole animal experiment was carried out in the sterile laminar flow environment in SPF experimental animal centre of Guangzhou University of Chinese Medicine. Animals were housed in the condition of 12/12 light–dark cycle with 24 °C and relative 50–70% relative humidity. Twenty-four male Sprague-Dawley (SD) rats (6–8 weeks old, 180–200 g) from the Laboratory Animal Centre of Guangdong (Guangzhou, China) were randomly divided into control group and model group. After one-week of adaptation, the aged fluid-insufficiency and constipated models were induced by water limitation, intragastric administration of loperamide hydrochloride and subcutaneous injection of 10% D-galactose for 5 weeks. Two groups of rats were allowed access to chow ad libitum. Body weights were measured every 4 days. Food intake and defecation were recorded weekly. The animal experiment was reviewed and approved by the Institutional Animal Care and Use Committee of Guangzhou University of Chinese Medicine, all procedures of animal treatment were in accordance with the National Guidelines for Experimental Animal Welfare (MOST, China, 2006) at the Centre for Animal Experiments. Maximum efforts were exerted to minimize animal suffering and the number of animals necessary for the attainment of reliable data.
Sample collection
The urinary and faecal samples of each animal were collected in eppendorf tube on the day – 1, 14, 21, 35 (8:00 a.m. to 16:00 p.m.). All urine samples were centrifuged (6000 rpm, 4 °C) for 10 min. Then the supernatant was carefully collected and divided into eppendorf tubes as needed. The urinary and fecal samples from each group were stored at −80 °C for NMR and microbiological analyses. Blood samples were collected, and centrifuged at 3500 rpm for 15 min at 4 °C. The resultant plasma samples were stored at −80 °C until analysis. The ink propelling rate in the small intestine was measured 30 minutes later after intragastric administration of ink. The colonic tissue was removed and washed with physiological saline and finally was put into a tube containing 10% formaldehyde solution for histopathology analysis.
Biochemistry assays and pathological examination
Biochemical indexes of plasma SOD and MDA were measured according to the instructions of enzymatic kits. The OD value was detected by ultraviolet spectrophotometer (Shimadzu UV-2450, Japan).
Colonic tissues were fixed with 10% formaldehyde solution for 48 h, embedded in paraffin, cut into 10 mm slices, and stained with hematoxylin-eosin (HE). Images were obtained and studied under Biological microscope (Leica DM5000 B, Germany).
Sample preparation for NMR spectroscopy
Each urine sample was thawed on ice and then each 600 μL of urine were mixed with 60 μL of phosphate buffer/D2O (1.5 M K2HPO4/NaH2PO4 pH 7.4). The buffer contains 0.1% NaN3 for bacteriostasis and 0.05% TSP for chemical shift reference (δ 0.00). The mixtures were centrifuged for 10 min (12
000 rpm 4 °C) to remove sediment. 600 μL supernatant was transferred into 5 mm NMR tubes for NMR analysis. Faecal samples were extracted using the optimized method described by Wu et al.29 with slight modifications. The details are as follows: take 100 mg thawed fecal sample accurately and add 800 μL buffer. After vortex mixing for about 1 min, the mixed slurry is carried on freeze–thaw treatments (3 times) and follows with ultrasonication cycles for 10 times to optimise the extracting durations. Ultrasonication is conducted in an ice bath in the form of ultrasonication (20 s)–vortex (10 s)–waiting (30 s). After 10 min centrifugation (16
000g at 4 °C). The 600 μL extractive was transferred into 5 mm NMR tubes and 1D 1H NMR spectra were recorded at 298 K on a Bruker AVIII 600 MHz spectrometer (Bruker Biospin, Germany) in 600.13 MHz. The acquisition of parameters were in accordance with the method described by Dong et al.30 The water signal was suppressed by irradiation during RD of 2 s, and mixing time (tm) of 80 ms. For each sample, the 90° pulse length was adjusted to approximately 10 μs and a total of 64 transients were accumulated into 32k data points over a spectral width of 20 ppm. For NMR signal assignment purpose, a range of two-dimensional (2D) NMR experiments (1H–1H correlation spectroscopy (COSY), total correlation spectroscopy (TOCSY), 1H–13C heteronuclear single quantum correlation spectroscopy (HSQC) and 1H–13C heteronuclear multiple bond correlation spectroscopy (HMBC)) were carried out on the selected samples as previously reported.31,32
NMR data processing
TOPSPIN (V2.1, Bruker Biospin) were used to calibrate the TSP at 0.00 ppm and manually corrected the phase and baseline distortion for all 1H NMR spectra of urine and feces. The spectral region (0.5–9.50 ppm) was integrated into regions with the bucket-width 0.004 ppm chemical shift bins by AMIX software (V3.9.14, Bruker Biospin). In urine samples, the region δ 4.70–4.95 (water signal) and δ 5.50–6.25 (urea signal) were discarded to eliminate unnecessary signal interferences. In fecal samples, the region δ 4.68–4.95 (water signal) was also discarded to exclude the efficient water suppression. Then the rest regions were normalized to the total integrated spectrum before multivariate date analysis. The metabolites were simultaneously identified based on the Human Metabolome Database (http://www.hmdb.ca/) and metabonomics toolbox (Chenomx NMRSuit 7.6, Chenomx, Canada) as well as published work.33,34
Molecular biological analysis and data processing
Total bacterial DNA was extracted from faecal samples according to the instructions of TIANamp Stool DNA Kit (Tiangen, Beijing, P. R. China) with slight modification and then stored at −20 °C for further analysis. The extracted DNA was used as template for PCR amplification and the universal primers used for amplification are referenced from Muyzer et al.35 PCR amplification system (25 μL) contained 12.5 μL Taq PCR Master Mix, 1 μL upstream primer, 1 μL downstream primer, 8.5 μL DNA template and double distilled water was added to make the total volume 25 μL. PCR reaction procedure: initial denaturation was set at 95 °C for 4 min. Then an amplification program, which included 1 min denaturation at 95 °C, 1 min annealing at 55 °C, 1 min extension at 72 °C, cyclically run 25 times and a finally extension was set at 72 °C for 10 min. The amplified product was confirmed by 2% agarose gel electrophoresis (90 V, 40 min) and then were analysed with a 40–58% gradient DGGE under constant voltage of 70 V for 12 h at 60 °C in 1× Tris-acetate-EDTA (TAE) buffer using the DCode universal mutation detection system (Junyi, Beijing, P. R. China). To observe the distribution of predominant bacteria after silver staining, digital camera (Canon, Japan) was used to record the images. DGGE images were analyzed and digitized by Quantity One (version 4.6.2). The relative band intensity was exported as a data matrix for normalization prior to pattern recognition analysis.
Multivariate pattern analysis and correlation analysis
Multivariate statistical analysis was performed to deal with the acquired NMR date and DGGE data using SIMCA-P+ (version12.0 Umetrics, Sweden). The unit variance (UV)-scaled NMR data and DGGE data were analyzed separately with principal component analysis (PCA) to acquire of the natural distribution of each matrix and identify the outliers. Then partial least squares (PLS) was utilized to find the relationship between data matrix X and Y. To eliminate the unrelated system variation of the Y matrix in the X matrix, orthogonal projection to latent structure-discriminant analysis (OPLS-DA) was applied to get a better model. R2Y and Q2 are respectively used to express the fitting degree and the predictive ability of the model to the Y variables. In addition, it is necessary to verify the validity of the multivariate model. The model was validated using a 7-fold cross-validation method and 200 permutation tests as well as further assessment using CV-ANOVA tests with a significant level of p < 0.05.36 The colour-coded loading plots were carried out in MATLAB (The Mathworks Inc.; Natwick, MA, version 7.1) based on correlation coefficient values to interpret important metabolites that contribute to the class separation. The correlations between the NMR spectra and DGGE gel data were obtained according to previous studies reported.37 Finally the correlation date was visualized by the Gephi 0.8.2 software and hemi 1.0 software.
Result
Body weight, food intake and ink propelling rate of the small intestine influenced by constipation
Body weights were recorded every four days during the whole experiment and no animals died. It was apparent that the weight growth tendency in model group was markedly slower than control rats (Fig. 2A). Significantly higher levels of body weights were observed in the model group than in control group after 8 days of modeling. The food intake and defecation were also measured weekly (Fig. 2B and C). The significant changes occurred in the first week after modeling. The food intake and defecation both decreased as time went on, whereas there was a trend of increase in the control group. The ink propelling rate of the small intestine in model groups were significantly lower than the normal group (p < 0.01) (Table 1). The results were consistent with the characteristics of constipation as previous reports.38
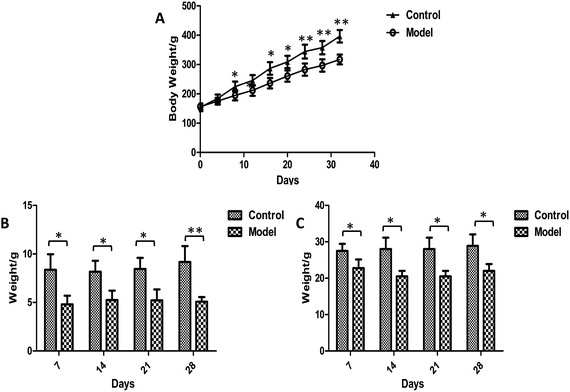 |
| Fig. 2 Plot of weight (A), food intake (B) and defecation (C) trend in control and model group. Value were expressed as mean ± S.D. (n = 10). *p < 0.05, **p < 0.01. | |
Table 1 Biochemical parameters, the ink propelling rate of the small intestine and the thickness of the colon mucosa measured in experimental animals (n = 8, mean ± S.D.)a
|
Control group |
Model group |
*P < 0.05; **P < 0.01. |
Ink propelling rate (%) |
84.8 ± 2.5** |
74.2 ± 3.2 |
Thickness of the mucosa (μm) |
290 ± 40.1* |
252.2 ± 36.3 |
SOD (U mL−1) |
356.3 ± 34.1** |
299.4 ± 51.2 |
MDA (nmol mL−1) |
3.3 ± 0.4* |
4.2 ± 0.5 |
Oxidative damages in organism induced by constipation
The biochemical parameters of experimental animals were summarized in Table 1. It has been reported that the changes of SOD and MDA were widely recognized as an indicator reflecting the oxygen free radical injury in vivo.39 The degree of oxidative injury caused by free radical was proportional to the degree of aging in rats. In the present work, the plasma SOD level in model group decreased significantly (p < 0.01), while MDA concentration was apparently higher than the control group (p < 0.01). The result was similar to previous researches.
Histological morphology examinations
The pathological results showed that the thickness of colonic mucosa in model group was significantly thinner than that in control group (p < 0.05) (Table 1). The thickness of the colon mucosa reflects the water content of the colon, and it will become thinner when the colon is dehydrated. In addition, mild lymphocytic infiltration and less goblet cells were presented in the model group (Fig. 3). Lymphocytic infiltration means inflammation, and the main function of goblet cells is to secrete mucus. The above symptoms suggested that the model had a lower water content in mucosa and mild inflammation in the colon. Although there are many metabolites that can cause inflammation and damage in the colon, this experiment has not reached a definitive conclusion for the time being and we can only show that these morphological changes are related to metabolic disturbances caused by constipation.
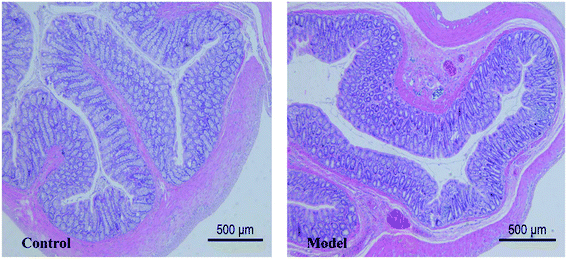 |
| Fig. 3 Histological examination of colonic mucosa. | |
Metabolites assignment with 1H NMR spectroscopy
The urinary and faecal metabolic profiles were performed by 1H NMR technology (Fig. S1 and S2†). The peaks of endogenous metabolites involved in the spectra were assigned based on the literature40,41 and were further confirmed by 2D NMR spectra. A total of 104 metabolites were figured out (70 urine-derived and 64 feces-derived metabolites) (Table S1†), mainly including choline, amino acid derivatives, carbohydrate, organic acids, amines and etc. These spectra exhibited obvious systematic differences among the groups. In order to obtain more detail information about the metabolic alterations after modelling, multivariate data analysis including PCA, PLS-DA and OPLS-DA were performed.
Dynamic metabolic profiles in urinary and fecal sample
PCA analysis was performed to generate an overview of the metabolic disturbance resulted from model intervention in urine and feces over the experimental period. Compared with day – 1, the model group and the control group showed an obvious trend of separation on day 21 through PCA model. The discrimination became more prominent on the day 35 (Fig. S3†). To evaluate the model more comprehensively, permutation test and CV-ANOVA were respectively performed under PLS-DA models and OPLS-DA models (Fig. S4 and S5†). The model validation parameters were summarized in Tables 2 and 3. R2 value and Q2 value were used as evaluation models, which represented the quality of fit and predictability of the sublevel mathematical models. The closer the value is to 1, the higher the fitting degree of the model. In the study, the R2 value and Q2 value of PCA, PLS-DA, OPLS-DA on day 35 are larger than 0.5, and the results of permutation test and CV-ANOVA were all in line with the requirements, which proved that the model was well established on day 35.
Table 2 Model validation parameters for rat fecesa
|
PCA |
PLS-DA |
OPLS-DA |
R2X |
Q2 |
R2X |
Q2 |
P test |
R2X |
Q2 |
p |
P test: permutation test, “✓” means model validation passed, “✗” means model validation failed; p value: CV-ANOVA analysis results. The same below. |
7 days |
0.455 |
0.276 |
0.609 |
0.43 |
✗ |
0.855 |
0.602 |
0.721 |
21 days |
0.493 |
0.388 |
0.674 |
0.789 |
✓ |
0.569 |
0.669 |
0.066 |
35 days |
0.671 |
0.455 |
0.624 |
0.896 |
✓ |
0.622 |
0.902 |
0.002 |
Table 3 Model validation parameters for rat urine
|
PCA |
PLS-DA |
OPLS-DA |
R2X |
Q2 |
R2X |
Q2 |
P test |
R2X |
Q2 |
p |
7 days |
0.496 |
0.334 |
0.616 |
0.792 |
✓ |
0.511 |
0.693 |
0.059 |
21 days |
0.778 |
0.585 |
0.829 |
0.962 |
✓ |
0.524 |
0.805 |
1.51 × 10−3 |
35 days |
0.697 |
0.403 |
0.583 |
0.957 |
✓ |
0.653 |
0.942 |
5.39 × 10−4 |
Urinary and faecal metabolites changes caused by constipation
After the crude screening with PCA, OPLS-DA was performed to maximize the discrimination of experimental groups and to focus on metabolic variations at the different time points in both urinary and faecal extracted spectra. The clear classifications between control groups and model groups were shown in the OPLS-DA score plots (Fig. 4), whereas the S-plot illustrated significantly altered metabolites induced by model. Consequently, all the integral data of detected peak were arranged in descending order according to VIP (Variable Importance in the Projection) values, which reflected the influence of each metabolite in the two groups. When VIP > 1, the variable can be considered as a contributor for the classification between control group and model group. Based on the VIP values (VIP > 1) and p values (p < 0.05), 27 urine metabolites and 22 fecal metabolites were finally selected as the potential biomarkers related to model, respectively (Tables S2 and S3†).
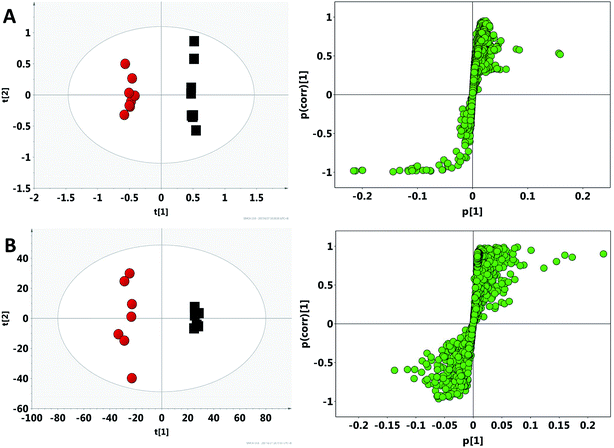 |
| Fig. 4 OPLS-DA score plots and S-plot of the control (black) and model (red) group in feces (A) and urine (B) based on 1H NMR technology. | |
Compared with control group, the contents of cholesterol (U1), taurine (U2), glycine (U3), trimethylamine N-oxide (U4), phosphorylcholine (U5), betaine (U6), butyrate (U7), trimethylamine (TMAO, U8), choline (U9), urocanate (U10) and 2-hydroxyisobutyrate (U11) significantly decreased in model group, whereas the contents of acetate (U12), pyruvate (U13), succinate (U14), methylamine (U15), dimethylamine (U16), creatinine (U17), creatine phosphate (U18), 2-oxoglutarate (U19), formate (U20), guanidoacetate (U21), glutamate (U22), creatine (U22), hippurate (U24), alanine (U25), fumarate (U26) and citrate (U27) increased significantly in urinary metabolites. There are lower levels of lactate (F1), alanine (F2), pyruvate (F3), succinate (F4), choline (F5), taurine (F6), butyrate (F7), acetate (F8), propionate (F9), glutamine (F10), tyrosine (F11), methionine (F12), leucine (F13), β-glucose (F14), α-glucose (F15), β-xylose (F16), and higher levels of methanol (F17), sarcosine (F18), proline (F19), glycine (F20), glycerol (F21), fumarate (F22) in faecal metabolites. To some extent, the above potential biomarkers altered in urine and feces, revealing the overall metabolic characteristics of the model.
Microbial profiles of faecal samples using DGGE analysis
To research the interference of the model on gut microbiota, the microbial structure on day 35 was represented by DGGE patterns of the 16S rRNA gene V3 region for predominant bacteria. Then the DGGE data was performed by multivariate pattern analysis, and the OPLS-DA score plots and corresponding coefficient loading plots showed the predictions of the difference bands between control groups and model groups (Fig. 5). 13 differential bands were identified by cloning and sequencing of 16S rRNA gene V3 regions (Table 4). The change of each band between the two groups is shown in the Fig. 6. The sequences are deposited in the GenBank with access numbers of MF409382–MF409394.‡ They mainly belonged to four phyla: six species from Firmicutes, three from Bacteroidetes, and three from Proteobacteria and one from Actinobacteria. The predominant flora in the control group was Firmicutes, while the model group was mainly composed of Bacteroidetes and Proteobacteria.
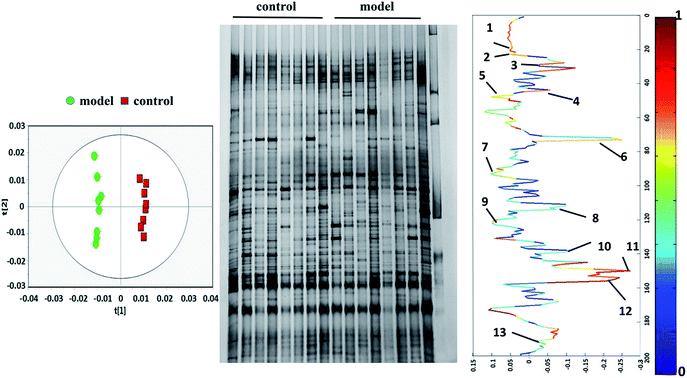 |
| Fig. 5 OPLS-DA score plots (left panel), PCR-DGGE profiles (middle panel) and correlation coefficient loading plots (right panel) derived from 16S rRNA gene V3 region. The bands of information can be found in Table 4. | |
Table 4 Closest relatives of 16s rDNA V3 regions sequences derived from DGGE bands
DGGE band |
Sample |
Domain |
Phylum |
Class |
Order |
Family |
Genus |
Species |
Ident |
Accession number |
Band 1 |
Model |
Bacteria |
Proteobacteria |
Gammaproteobacteria |
Enterobacterales |
Enterobacteriaceae |
Escherichia |
Escherichia fergusonii ATCC 35469 |
99% |
MF 409382 |
Band 2 |
Model |
Bacteria |
Actinobacteria |
Actinomycetes |
Actinomycetales |
Actinomycetaceae |
Actinomyces |
Actinomyces odontolyticus |
98% |
MF 409383 |
Band 3 |
Model |
Bacteria |
Bacteroidetes |
Bacteroidia |
Bacteroidales |
Odoribacteraceae |
Culturomica |
Culturomica massiliensis |
97% |
MF 409384 |
Band 4 |
Control |
Bacteria |
Proteobacteria |
Gammaproteobacteria |
Enterobacterales |
Enterobacteriaceae |
Escherichia |
Escherichia fergusonii |
100% |
MF 409385 |
Band 5 |
Model |
Bacteria |
Firmicutes |
Clostridia |
Clostridiales |
Ruminococcaceae |
Ruminococcus |
Ruminococcus flavefaciens |
99% |
MF 409386 |
Band 6 |
Control |
Bacteria |
Firmicutes |
Bacilli |
Lactobacillales |
Lactobacillaceae |
Lactobacillus |
Lactobacillus acidophilus |
100% |
MF 409387 |
Band 7 |
Model |
Bacteria |
Bacteroidetes |
Bacteroidia |
Bacteroidales |
Prevotellaceae |
Prevotella |
Prevotella dentalis DSM 3688 |
96% |
MF 409388 |
Band 8 |
Control |
Bacteria |
Firmicutes |
Clostridia |
Clostridiales |
Intestinimonas |
Intestinimonas butyriciproducens |
|
98% |
MF 409389 |
Band 9 |
Model |
Bacteria |
Firmicutes |
Clostridia |
Clostridiales |
Ruminococcaceae |
Ruminiclostridium |
Cellobioparum DSM 1351 = ATCC 15832 |
91% |
MF 409390 |
Band 10 |
Control |
Bacteria |
Firmicutes |
Clostridia |
Clostridiales |
Eubacteriaceae |
Eubacterium |
Eubacterium xylanophilum |
98% |
MF 409391 |
Band 11 |
Control |
Bacteria |
Bacteroidetes |
Bacteroidia |
Bacteroidales |
Porphyromonadaceae |
Muribaculum |
Muribaculum intestinale |
90% |
MF 409392 |
Band 12 |
Control |
Bacteria |
Firmicutes |
Clostridia |
Clostridiales |
Eubacteriaceae |
Eubacterium |
Eubacterium coprostanoligenes |
98% |
MF 409393 |
Band 13 |
Model |
Bacteria |
Proteobacteria |
Gammaproteobacteria |
Pseudomonadales |
Moraxellaceae |
Acinetobacter |
Acinetobacter schindleri |
99% |
MF 409394 |
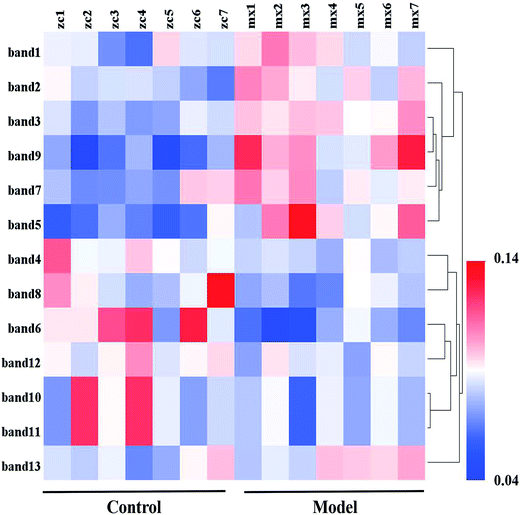 |
| Fig. 6 Heatmap analysis of 13 bands relative abundance. | |
Discussion
Constipation is a well-known symptom of multisystem diseases. Modern medicine believes that normal bowel movements are the process of pushing intestinal contents into sigmoid colon and rectum through segmental contractions and periodic peristalsis, the entire process is under the control of the primary defecation centre and the cerebral cortex. Any disorder in the above process can result in constipation. When bowel movements are inhibited, the stool stays longer in the gut, the most of water in the feces will be absorbed by the intestine, and the feces will become too dry and hard to expel. In the animal experiment, water limitation can result in fluid-deficiency and reduce moisture content in feces. Loperamide hydrochloride can inhibit the contraction of intestinal smooth muscle and reduce peristalsis. In addition, many patients with constipation are the old according to clinical practice observation. Subcutaneous injection of D-galactose is an internationally accepted method to interfere with glucose metabolism and finally obtain the aged animal model. Using the above modeling method, the characteristics of senile constipation can be well simulated.
After modeling, rats showed an abnormal condition including weight loss and poor appetite. According to the characteristics of constipation, the frequency and/or amount of defecation in model group decreased significantly, which can also explain for the significant decrease in ink propelling rate of the small intestine and thickness of colonic mucosa. The results indicate that the difficult defecation was caused by the slow bowel motility and insufficient water in intestinal tract. Therefore, the constipation model with syndrome of fluid-deficiency was successfully established. SOD, which can scavenge free radicals and protect tissue cell membranes from damage, plays a vital role in antioxidation and oxidation balance of the body. MDA can indirectly reflect the extent of cell damage. Simultaneous determination of MDA and SOD achieve more comprehensive assessment in the antioxidant capacity of the organism. In the experiment, lower level of SOD and higher level of MDA in model groups suggest that the body was heavily oxidized. As everyone knows, aging is closely related to body oxidation, so the results indicated that the aging model was successfully established.
Metabolic pathway changes caused by constipation
The metabonomics integrating feces and urine suggested that the metabolic profiles of the model rats deviated from that of the control rats. To consider the most evident metabolic disturbances, the potential biomarkers related to model was used for metabonomics pathway analysis (MetPA) with a web-tool (http://www.metaboanalyst.ca), involving in thirty-eight metabolic corresponding pathways (Fig. 7 and Table S4†). Consequently, seven metabolic pathways were screened out as the most important metabolic pathways (p < 0.05, impact > 0.1) related to faecal and urinary metabolic disturbances, including glycine, serine and threonine metabolism, alanine, aspartate and glutamate metabolism, citrate cycle (TCA cycle), glycolysis or gluconeogenesis, arginine and proline metabolism, pyruvate metabolism and valine, leucine and isoleucine biosynthesis. The metabolic alterations and the associated pathways provide insights into the mechanisms involved in the development and progression of model. The seven metabolic pathways were taken into the KEGG database (http://www.kegg.jp/) for further analysis. The key altered metabolites were widely distributed across the mammalian–microbial metabolic system involved in gut microbiome metabolism and host metabolism (Fig. 8). The Fig. 8 is a summary of all the differential metabolites found in this study, which demonstrate the association among these differential metabolites, and explain the role of these metabolites in each metabolic pathway. Finally, five meaningful metabolic pathways related to the model were summarized as below.
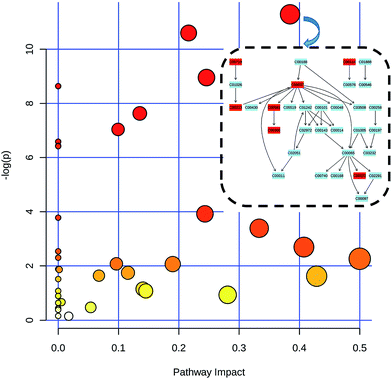 |
| Fig. 7 Metabolism pathways in urine and feces of model rats compared with control rats as visualized by bubble plots. Bubble size is proportional to the impact of each pathway and bubble color denoting the significance from highest in red to lowest in white. At the right corner, it emerged the specific pathway which detected metabolites were involved in (rectangles colored in blue are no metabolites matched, vice versa). | |
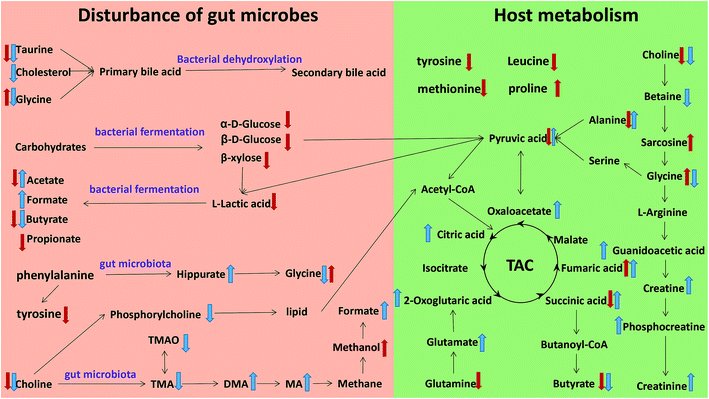 |
| Fig. 8 Metabolic pathways altered by model, ↑ up-regulated; ↓ down-regulated; red colour, faeces; blue colour, urine. | |
Energy metabolism disturbances caused by constipation
The tricarboxylic acid cycle (TCA) is the ultimate metabolic pathway of 3 major nutrient substances (carbohydrates, lipids and amino acids). Meanwhile, TCA is the hinge of metabolism of carbohydrates, lipids and amino acids. In this study, the content of fumarate, succinate, citrate and 2-oxoglutarate were up-regulated significantly after modelling, revealing that more nutrient substance were transformed into energy in the model group. The reason may be related to the decrease of food intake. Decreasing food intake is a common manifestation of poor appetite that resulted from constipation. The energy for body consuming accordingly reduce because of the lack of food. When the energy gained from food is less than the energy consumed by the body, the body has to consume the nutrients stored in the body to maintain normal physiological activity, which can further explain the loss of body weight. The above result can be demonstrated by the decreased in α-glucose and β-glucose. As constipation leads to a decrease in energy intake, more carbohydrates (such as glucose) or other nutrients need to be transformed into energy through TCA to maintain balance.
Butyric acid synthesis disturbed by constipation
Butyric acid is the final metabolite of polysaccharides that cannot be absorbed by the host. A large number of animal experiments and human clinical studies showed that butyrate plays an important role in repairing intestinal mucosa and in the treatment of enteritis. Butyrate was also considered to be a growth inducer and an inflammatory inhibitor of the colon mucosa.42 At the same time, butyric acid can reduce the risk of colorectal cancer, induce apoptosis and inhibit colon cancer.43,44 In this study, the content of butyric acid was down-regulated significantly after modelling. The result can explain the thinning of the mucosa in colon and mild inflammation in the model group.
Choline metabolism disturbed by constipation
The main function of choline is to promote brain development, improve memory and act as signalling molecules to ensure the transmission of information and to participate in some other important physiological functions.45,46 In the metabolic pathway of choline, the levels of choline were down regulated. As a metabolite of choline, amines (methylamine, dimethylamine) increased markedly. The result indicates that model intervention promotes the process of converting choline to methylamine, resulting in a decrease in choline biological activity with a series of choline deficiency symptoms. In addition, methylamine can be further decomposed into methane, which can be converted to methanol and formic acid. In the current investigation, the level of methanol and formic acid were significantly risen after modelling. In other words, the metabolic pathway of methane may have been promoted. Previous studies have shown that the content of methane is positively related to the severity of constipation because methane can weaken gastrointestinal motility.47
Constipation disturbs liver and kidney functions
The level of creatine, creatine phosphate and creatinine increased significantly in the urine samples, which indicates that the metabolism of creatinine has been promoted. Creatine is found mainly in muscle and most of it exists in the form of creatine phosphate. Then creatine phosphate, which is excreted by urine, produces creatinine after removal of phosphoric acid. Clinically, it is widely believed that elevated urinary creatinine indicates a possible renal impairment, which may be attributed to the accumulation of toxins. Traditional Chinese medicine believes that the kidney governs the water and the source of the water. In this experiment, the constipation model is induced by water limitation. Kidney damage may occur when the body lacks water. Taurine is a very important active substance in the body that participates in many physiological activities, such as promoting brain tissue and intelligence development, preventing cardiovascular disease and synthesizing bile acid and so on. Previous studies have shown that taurine has antioxidant effects, and is related to oxidative stress levels.48 In addition, the concentration of taurine in urine can be regarded as an important indicator of the extent of liver damage.49 The decrease of taurine content indicates that the antioxidant ability of the body is weakened as toxin accumulated, and the physiological activity of bile acid decreased.
Amino acid metabolism disturbed by constipation
Amino acids were significantly altered after model intervention. In this study, tyrosine, methionine and leucine significantly decrease in model groups, whereas proline increased significantly. Tyrosine, which is a non-essential amino acid and crucial component of the body proteins, not only produces neurotransmitters like levodopa, DOPA, norepinephrine, and epinephrine, but also changes melanin darkened in the skin to combat UV rays that are harmful to the body. Methionine is one of the essential amino acids in human body which is involved in protein synthesis. The lack of methionine may result in loss of appetite, slowly growing and no weight gain or even losing weight. The function of leucine is to work with isoleucine and valine to repair muscle, control blood sugar, and provide energy for body tissues. The content of the above three amino acids decreased, indicating that the physiological functions of these amino acids reduced in the model groups. Proline is a protective agent, the free radical scavenger of the inner membrane and the enzyme of the organism. In some harsh conditions, its content will rise in order to better protect the body.50 In this experiment, the elevation of proline may be due to the stimulation of the model.
Identification of related metabolic biomarkers in constipation
Taurine, glycine, choline, butyrate, acetate, pyruvate, succinate and fumarate were detected as potential biomarkers both in faeces and urine samples. Receiver operating characteristic (ROC) curve analysis was further employed to evaluate the potential utility of the eight metabolites with significant correlations (Fig. 9). The area under the curve is usually used to evaluate the results of the ROC analysis. In the case of AUC > 0.5, the closer the AUC is to 1, the better the diagnosis is. The ROC prediction has a lower accuracy when AUC ranges in 0.5–0.7, a medium accuracy in 0.7–0.9, and higher in over 0.9. As a result, taurine, glycine, choline, acetate and succinate achieved good AUC values (AUC > 0.9) in distinguishing the models and controls, which could be screened as robust predictors of model.
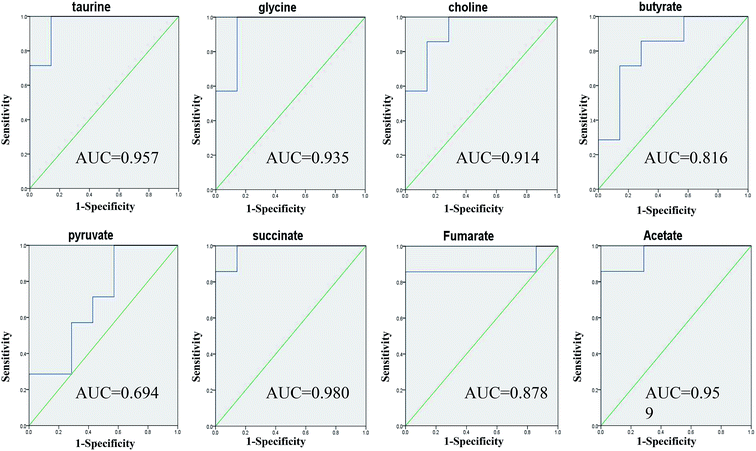 |
| Fig. 9 ROC curve analysis for the predictive power of 8 biomarkers relating to model. | |
Changes in gut microbiota influencing the host
In the genus level analysis of the 13 bands, Escherichia (band 1), Actinomyces (band 2), Culturomica (band 3), Ruminococcus (band 5), Prevotella (band 7), Ruminiclostridium (band 9) and Acinetobacter (band 13) significantly increased in model group, whereas Escherichia (band 4), Lactobacillus (band 6), Intestinimonas (band 8), Muribaculum (band 11), Eubacterium (band 10 and 12) markedly decreased. The effects of each bacterium on the organism were summarized by consulting relevant literature as follows.
Previous studies have shown that the Prevotella (band 7) thrived in proinflammatory environments and even promotes the development of inflammation. At the same time, Prevotella (band 7) was believed to be associated with rheumatic diseases.51,52 Muribaculum (band 8) belongs to the Porphyromonadaceae. Research by Kverka et al.53 showed that the other bacterium in Porphyromonadaceae could reduce the acute or chronic enteritis caused by dextran sulfate sodium (DSS), and had anti-inflammatory effect. Muribaculum (band 8) may have the same or similar function. In this study, the level of Prevotella (band 7) significantly increased and the level of Muribaculum (band 8) significantly decreased, indicating that the changes of this bacteria promotes the development of inflammation in vivo.
Ruminococcus (band 5) is a Gram positive anaerobic bacterium found in the human gut which can secrete β-glucuronidase. Through a series of reactions, β-glucuronidase can produce the active substances to damage the mucosa in colon and participate in the invasion and metastasis of tumour.54,55 Most Actinomyces (band 2) and Acinetobacter (band 13) belong to opportunistic pathogen. When their number increase, they produce toxins that harm the body health. Actinomyces has been linked to many diseases like painful abscesses in the mouth, lungs, or gastrointestinal tract.56 Acinetobacter may cause a wide range of infections.57 The three bacteria were significantly increased in the model group, indicating that harmful substances accumulated in the model group.
Eubacterium (band 10 and 12) and Intestinimonas (band 8) were the chief producer of butyric acid in intestines and faeces. Duncan et al.58,59 has isolated a variety of butyric acid bacteria from faeces samples as well as E. oxidoreducens. Research by Kläring et al.60 showed that Intestinimonas was a butyrate-producing bacterium from the mouse intestine. Their decrease may prevent the production of butyric acid which can provide nutrients for the tissue and protect the integrity of the intestinal mucosa.
Lactobacillus (band 6) is a probiotics, which can produce acetic acid, lactic acid and other short chain fatty acids to inhibit intestinal spoilage bacteria. As the most important product, lactic acid can relieve constipation by stimulating peristalsis. In the study, the decreased level of Lactobacillus (band 6) indicated that the peristalsis of the intestine slows down.
Correlation analysis of metabolites and gut microbiota
Correlation analysis between metabolites (faeces and urine) and gut microbiota was carried out with Pearson correlation coefficient (|r| > 0.5) and multivariate statistical OPLS model (p < 0.05) (Fig. 10). Then, screening of the correlation between metabolites and intestinal flora with a higher correlation of r > 0.6 (Fig. 11). According to the picture, intestinal microflora mainly participates in the metabolism of short chain fatty acids, amino acids, bile acids, choline, and tricarboxylic acid cycle in the host.
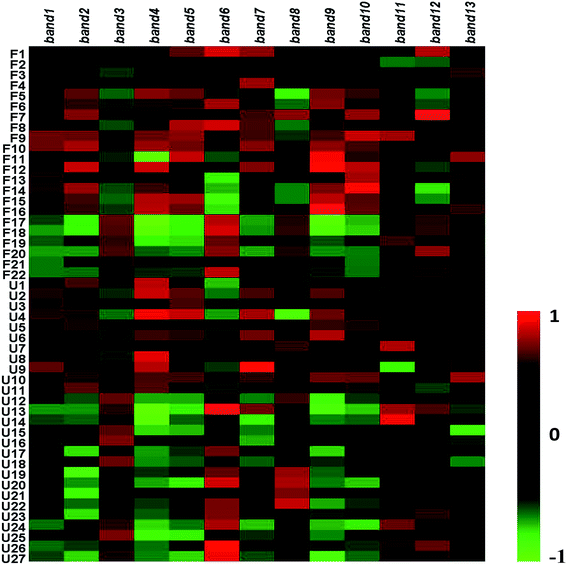 |
| Fig. 10 Pearson correlation coefficient heat map between metabolites and gut microbiota. | |
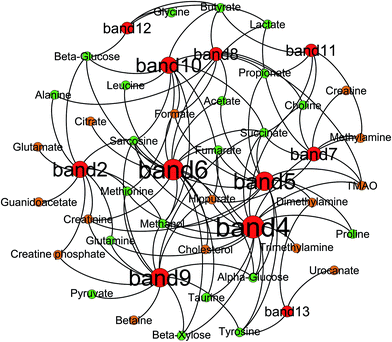 |
| Fig. 11 Correlation network between altered DGGE bands and changed metabolic components of rats' urine and faeces visualized with Gephi. Node size denotes the highest effect size for each band or metabolite comparing model group with control group. Nodes colored red for bands, green for faecal metabolites and orange for urinary ones. | |
Short chain fatty acids
The host itself is unable to digest plant polysaccharides and vitamins from the diet. The above substances are digested mostly by intestinal bacteria metabolism. The intestinal flora contains approximately 81 enzymes which are involved in the catabolism of starch, glucose, and Arabia sugars.52 Under the action of enzymes, complex carbohydrates eventually become a variety of fermentation products, such as short chain fatty acids (SCFA), other organic acids, ethanol, hydrogen and carbon dioxide. In this experiment, the short chain fatty acids detected were formic acid, acetic acid, propionic acid and butyric acid. According to correlation coefficient, R. flavefaciens (band 5), L. acidophilus (band 6), I. butyriciproducens (band 8), E. xylanophilum (band 10), M. intestinale (band 11) and E. coprostanoligenes (band 12) have a high correlation with short chain fatty acids. This result has been confirmed in the literature, which indicated that these bacteria have the function to produce short chain fatty acids through fermentation.61–63
Amino acid
Bacteria in the gut can participate in the metabolism of proteins and amino acids.64,65 After the model intervention, there were obvious changes in various amino acids, which were correlated with the change of gut microbiota. Hippurate, as a biomarker of assessing the balance of the gut microbial community,66 is positively correlated to the abundance of Clostridia in Crohn disease.22,67 In this experiment, two Firmicutes families (band 10 and 12), classified into the same order of Clostridiales, have a high correlation with hippurate. Intestinal flora can interfere the metabolism of other amino acids. For example, under the action of Clostridium and Lactobacillus, tyrosine and phenylalanine can be transformed into para-cresol.68 In this study, A. odontolyticus (band 2), E. fergusonii (band 4), R. flavefaciens (band 5), L. acidophilus (band 6), C. cellobioparum (band 9) and E. xylanophilum (band 10) showed a high correlation with various amino acids suggesting that these bacteria may be involved in the metabolism of amino acids.
Bile acids
The primary bile acids are produced by cholesterol synthesis in the liver. The main function of bile acids is to promote the metabolism of lipid and fat soluble vitamins. Then, primary bile acids were combined with taurine and glycine in the liver.69,70 90–95% bile acids complete the enterohepatic recirculation via being absorbed in the small intestine and returning to the liver. Partially, non-absorbed bile acids produce secondary bile acids under the action of hydrolases created by Bactervides, Clostridium, Eubacterium, Lactobacillus and Escherichia.71,72 In this study, E. fergusonii (band 4), L. acidophilus (band 6) and E. coprostanoligenes (band 12) have a high correlation with cholesterol, taurine and glycine. The result is consistent with that reported in the literature.
Choline
Choline, as an important active substance in the body, can be transformed into trimethylamine under the influence of intestinal flora and be further metabolized to dimethylamine or methylamine. It can also be converted to trimethylamine oxide (TMAO) by enzymes in the liver.73,74 It has been found that some functional bacteria in intestinal flora are closely related to the level of TMAO in vivo.75,76 For example, an increase in the proportion of Firmicutes in the intestinal microbiota may be associated with elevated TMAO levels in vivo, whereas Methanobacterium can reduce the level of TMAO in vivo. In this study. E. fergusonii (band 4), R. flavefaciens (band 5), P. dentalis (band 7), I. butyriciproducens (band 8) and M. intestinale (band 11) have a high correlation with choline and amines. Thus, it can be speculated that the above bacteria is related to the metabolism of choline.
Intermediate product of TCA
Succinic acid is the intermediate product of the tricarboxylic acid cycle. Previous studies found that propionate-producing bacterium such as Propionibacterium can produce succinic acid with sugar and amino acid as substrate. Some typical intestinal flora (Escherichia coli, Pectinatus sp., Bacteroides sp., and Ruminococcus sp.) can also secrete succinic acid.77 Fumaric acid and succinic acid can be converted into each other in the TCA cycle. On the one hand, succinate can produce fumaric acid through succinate dehydrogenase, and then further into malic acid. On the other hand, fumaric acid can also be reduced to succinic acid under the catalysis of fumaric acid reductase. In the research, E. fergusonii (band 4), R. flavefaciens (band 5), L. acidophilus (band 6) and P. dentalis (band 7) have a high correlation with succinic acid and fumaric acid which suggests that these bacteria might interfere the TCA cycle.
Conclusions
In summary, the study use PCR-DGGE combined with 16S rRNA gene sequencing and metabolomics profiling to study the impact of the gut microbiome and its metabolic profiles of aged constipation with fluid-deficiency. The results showed that constipation affected the body, which not only perturbed the functions of gastrointestinal tract, but also modulated the host systematic metabolism and the function of liver and kidney. In addition, strong correlations between metabolites and gut bacteria (including Escherichia, Actinomyces, Prevotella, Ruminococcus, Lactobacillus, Acinetobacter, Intestinimonas, Eubacterium and Muribaculum) were observed to guide further research. Because the change of metabolites is the result of the interaction of many bacteria and host, relation of gut microbiome and metabolomics is very complex. A specific relationship cannot be discussed here for it still needs further study to obtain more information. Taken together, these data demonstrated substantial insight into the effects regarding microbiology and metabolomics response to aged constipation with fluid-deficiency. Advances in technology in both metabolic phenotyping and microbial profiling methods have improved our ability to derive the correlations between microbial and metabolic phenotypes, which are of particularly importance to optimized therapeutic strategies and early interventions.
Authors' contributions
Deliang Liu participated in the whole experiment and writing process. Fuhai Wu, Zhiyong Xie and Qiongfeng Liao designed the scheme of experiment. Biyu Lu and Tiantian Li were in charge of animal experiments. Mingyi Chen and Yuan Li were responsible for revising the article. Ziqong Liao performed the PCR-DGGE analysis and the statistics. Haihui Zheng performed the NMR analysis and the statistics. Xiquan Yin, Minghua Hu and Zhen Luo were in charge of 16S rRNA gene sequences. All authors read and approved the final manuscript.
Conflicts of interest
The authors declare no competing financial interests.
Acknowledgements
This work was supported by the National Natural Science Foundation of China (No. 81473319 and No. 81473540), the Guangdong Science and Technology Program (No. 2014A020221027, 2013B090800011, 2015A030401031, 2014A020209026), the Natural Science Foundation of Guangdong Province (2015A030313123) and the Zhongshan Science and Technology Program (No. 2016C1015).
References
- I. J. McCallum, S. Ong and M. Mercer-Jones, BMJ, 2009, 338, b831 CrossRef PubMed.
- A. P. Manning, W. G. Thompson, K. W. Heaton and A. F. Morris, Br. Med. J., 1978, 2, 653–654 CrossRef CAS PubMed.
- M. Kanazawa, H. Miwa and A. Nakagawa, BioPsychoSoc. Med., 2016, 10(1), 19 CrossRef PubMed.
- A. D. Markland, O. Palsson, P. S. Goode, K. L. Burgio, J. Busby-Whitehead and J. E. Whitehead, Am. J. Gastroenterol., 2013, 108, 796–803 CrossRef CAS PubMed.
- P. Pare, S. Ferrazzi, W. G. Thompson, E. J. Irvine and L. Rance, Am. J. Gastroenterol., 2001, 96, 3130–3137 CrossRef CAS PubMed.
- D. W. Jun, H. Y. Park, O. Y. Lee, H. L. Lee, B. C. Yoon, H. S. Choi and C. S. Kee, Dig. Dis. Sci., 2006, 51, 1471–1477 CrossRef PubMed.
- M. Zhang, X. J. Yang, H. M. Zhu, Z. Tang, B. Y. Li and D. D. Zhao, World J. Gastroenterol., 2015, 21, 13368 CrossRef CAS PubMed.
- N. C. Suares and A. C. Ford, Am. J. Gastroenterol., 2011, 106, 1582–1591 CrossRef PubMed.
- A. Wald, C. Scarpignato, S. Mueller-Lissner, M. A. Kamm, U. Hinkel, I. Helfrich and K. G. Mandel, Aliment. Pharmacol. Ther., 2008, 28, 917–930 CAS.
- L. V. Hooper and J. L. Gordon, Science, 2001, 292(5519), 1115–1118 CrossRef CAS PubMed.
- S. Bourdu, M. Dapoigny, E. Chapuy, F. Artigue, M. P. Vasson, P. Dechelotte and D. Ardid, Gastroenterology, 2005, 128, 1996–2008 CrossRef CAS.
- D. Vandeputte, G. Falony, S. Vieira-Silva, R. Y. Tito, M. Joossens and J. Raes, Gut, 2015, 65, 57–62 CrossRef PubMed.
- L. Zhu, W. Liu, R. Alkhouri, R. D. Baker, J. E. Bard, E. M. Quigley and S. S. Baker, Physiol. Genomics, 2014, 46, 679–686 CrossRef CAS PubMed.
- G. Parthasarathy, J. Chen, X. Chen, N. Chia, H. M. O'Connor, P. G. Wolf and A. E. Bharucha, Gastroenterology, 2016, 150, 367–379 CrossRef PubMed.
- I. V. Kirgizov, A. M. Sukhorukov, V. A. Dudarev and A. A. Istomin, Clin. Appl. Thromb./Hemostasis, 2001, 7, 335–338 CrossRef CAS.
- I. L. Khalif, E. M. M. Quigley, E. A. Konovitch and I. D. Maximova, Dig. Liver Dis., 2005, 37, 838–849 CrossRef CAS PubMed.
- S. E. Kim, S. C. Choi, K. S. Park, M. I. Park, J. E. Shin, T. H. Lee and S. J. Myung, J. Neurogastroenterol. Motil., 2015, 21, 111–120 CrossRef PubMed.
- X. Feng, Y. Su, J. Jiang, N. Li, W. Ding, Z. Wang and J. Li, Am. Surg., 2015, 81, 196–204 Search PubMed.
- C. Chassard, M. Dapoigny, K. P. Scott, L. Crouzet, C. Del'Homme, P. Marquet and C. Dubray, Aliment. Pharmacol. Ther., 2012, 35, 828–838 CrossRef CAS PubMed.
- I. L. Khalif, E. M. M. Quigley, E. A. Konovitch and I. D. Maximova, Dig. Liver Dis., 2005, 37, 838–849 CrossRef CAS PubMed.
- K. Lu, R. Mahbub, P. H. Cable, H. Ru, N. M. Parry, W. M. Bodnar and S. R. Tannenbaum, Chem. Res. Toxicol., 2014, 27, 172–174 CrossRef CAS PubMed.
- M. Li, B. Wang, M. Zhang, M. Rantalainen, S. Wang, H. Zhou and H. Wei, Proc. Natl. Acad. Sci. U. S. A., 2008, 105, 2117–2122 CrossRef CAS PubMed.
- J. K. Nicholson, J. C. Lindon and E. Holmes, Xenobiotica, 1999, 29, 1181–1189 CrossRef CAS PubMed.
- C. Diao, L. Zhao, M. Guan, Y. Zheng, M. Chen, Y. Yang and H. Gao, Mol. BioSyst., 2014, 10, 686–693 RSC.
- S. P. Claus, T. M. Tsang, Y. Wang, O. Cloarec, E. Skordi, F. P. Martin and J. K. Nicholson, Mol. Syst. Biol., 2008, 4, 219 CrossRef PubMed.
- F. P. J. Martin, M. E. Dumas, Y. Wang, C. Legido-Quigley, I. K. Yap, H. Tang and N. Sprenger, Mol. Syst. Biol., 2007, 3, 112 CrossRef PubMed.
- F. P. J. Martin, Y. Wang, I. K. Yap, N. Sprenger, J. C. Lindon, S. Rezzi and J. K. Nicholson, J. Proteome Res., 2009, 8, 3464–3474 CrossRef CAS PubMed.
- L. Zhang, L. Wang, Y. Hu, Z. Liu, Y. Tian, X. Wu and Y. Wang, Biomaterials, 2013, 34(29), 7117–7126 CrossRef CAS PubMed.
- J. Wu, Y. An, J. Yao, Y. Wang and H. Tang, Analyst, 2010, 135, 1023–1030 RSC.
- F. Dong, L. Zhang, F. Hao, H. Tang and Y. Wang, J. Proteome Res., 2013, 12, 2958–2966 CrossRef CAS PubMed.
- O. Beckonert, H. C. Keun, T. M. Ebbels, J. Bundy, E. Holmes, J. C. Lindon and J. K. Nicholson, Nat. Protoc., 2007, 2, 2692–2703 CrossRef CAS PubMed.
- J. F. Wu, E. Holmes, J. Xue, S. H. Xiao, B. H. Singer, H. R. Tang and Y. Wang, Int. J. Parasitol., 2010, 40, 695–703 CrossRef CAS PubMed.
- C. A. Merrifield, M. Lewis, S. P. Claus, O. P. Beckonert, M. E. Dumas, S. Duncker and E. Holmes, Mol. BioSyst., 2011, 7, 2577–2588 RSC.
- G. Le Gall, S. O. Noor, K. Ridgway, L. Scovell, C. Jamieson, I. T. Johnson and A. Narbad, J. Proteome Res., 2011, 10, 4208–4218 CrossRef CAS PubMed.
- G. Muyzer, E. C. De Waal and A. G. Uitterlinden, Appl. Environ. Microbiol., 1993, 59, 695–700 CAS.
- T. A. Clayton, J. C. Lindon, O. Cloarec, H. Antti, C. Charuel, G. Hanton and J. R. Everett, Nature, 2006, 440, 1073–1077 CrossRef CAS PubMed.
- J. Cui, Y. Liu, Y. Hu, J. Tong, A. Li, T. Qu and G. Du, J. Pharm. Biomed. Anal., 2017, 132, 77–86 CrossRef CAS PubMed.
- G. Le Gall, S. O. Noor, K. Ridgway, L. Scovell, C. Jamieson, I. T. Johnson and A. Narbad, J. Proteome Res., 2011, 10, 4208–4218 CrossRef CAS PubMed.
- J. M. Götz, J. L. Thio, H. W. Verspaget, G. J. Offerhaus, I. Biemond, C. B. Lamers and R. A. Veenendaal, Gut, 1997, 40, 591–596 CrossRef.
- Y. Tian, L. Zhang, Y. Wang and H. Tang, J. Proteome Res., 2011, 11, 1397–1411 CrossRef PubMed.
- X. Shi, C. Xiao, Y. Wang and H. Tang, J. Proteome Res., 2012, 12, 991–1006 CrossRef PubMed.
- C. R. Cavaglieri, A. Nishiyama, L. C. Fernandes, R. Curi, E. A. Miles and P. C. Calder, Life Sci., 2003, 73(13), 1683–1690 CrossRef CAS PubMed.
- C. Neut, F. Guillemot, C. Gower-Rousseau, N. Biron, A. Cortot and J. F. Colombel, Gastroenterol. Clin. Biol., 1995, 19(11), 871–875 CAS.
- R. I. Breuer, S. K. Buto, M. L. Christ, J. Bean, P. Vernia, P. Paoluzi and R. Caprilli, Dig. Dis. Sci., 1991, 36(2), 185–187 CrossRef CAS PubMed.
- S. H. Zeisel, K. A. Da Costa, P. D. Franklin, E. A. Alexander, J. T. Lamont, N. F. Sheard and A. Beiser, FASEB J., 1991, 5, 2093–2098 CAS.
- M. Lever and S. Slow, Clin. Biochem., 2010, 43, 732–744 CrossRef CAS PubMed.
- J. Jahng, I. S. Jung, E. J. Choi, J. L. Conklin and H. Park, Neurogastroenterol. Motil., 2012, 24, 185 CrossRef CAS PubMed.
- I. G. Obrosova, L. Fathallah and M. J. Stevens, Exp. Neurol., 2001, 172, 211–219 CrossRef CAS PubMed.
- T. Miyazaki and Y. Matsuzaki, Amino Acids, 2014, 46(1), 101–110 CrossRef CAS PubMed.
- P. D. Hare, W. A. Cress and J. Van Staden, Plant, Cell Environ., 1998, 21, 535–553 CrossRef CAS.
- U. Hofer, Nat. Rev. Microbiol., 2014, 12, 5 CrossRef PubMed.
- S. R. Gill, M. Pop, R. T. DeBoy, P. B. Eckburg, P. J. Turnbaugh, B. S. Samuel and K. E. Nelson, science, 2006, 312, 1355–1359 CrossRef CAS PubMed.
- M. Kverka, Z. Zakostelska, K. Klimesova, D. Sokol, T. Hudcovic, T. Hrncir and H. Tlaskalova-Hogenova, Clin. Exp. Immunol., 2011, 163, 250–259 CrossRef CAS PubMed.
- C. Humblot, E. Lhoste, S. Knasmüller, K. Gloux, A. Bruneau, M. Bensaada and F. Kassie, J. Chromatogr. B: Anal. Technol. Biomed. Life Sci., 2004, 802, 231–237 CrossRef CAS PubMed.
- D. H. Kim and Y. H. Jin, Arch. Pharmacal Res., 2001, 24, 564 CrossRef CAS.
- J. S. McLean, Q. Liu, B. Bor, J. K. Bedree, L. Cen, M. Watling and W. Shi, Genome Announc., 2016, 4, e01685 Search PubMed.
- A. Ali, J. Botha and R. Tiruvoipati, Int. J. Surg. Case Rep., 2014, 5, 532–536 CrossRef PubMed.
- S. H. Duncan, G. L. Hold, A. Barcenilla, C. S. Stewart and H. J. Flint, Int. J. Syst. Evol. Microbiol., 2002, 52, 1615–1620 CAS.
- S. H. Duncan, K. P. Scott, A. G. Ramsay, H. J. Harmsen, G. W. Welling, C. S. Stewart and H. J. Flint, Appl. Environ. Microbiol., 2003, 69, 1136–1142 CrossRef CAS PubMed.
- K. Kläring, L. Hanske, N. Bui, C. Charrier, M. Blaut, D. Ha-ller and T. Clavel, Int. J. Syst. Evol. Microbiol., 2013, 63, 4606–4612 CrossRef PubMed.
- C. Engels, H. J. Ruscheweyh, N. Beerenwinkel, C. Lacroix and C. Schwab, Front. Microbiol., 2016, 7, 713 Search PubMed.
- T. P. N. Bui, S. A. Shetty, I. Lagkouvardos, J. Ritari, B. Chamlagain, F. P. Douillard and W. M. de Vos, Environ. Microbiol. Rep., 2016, 8, 1024–1037 CrossRef CAS PubMed.
- L. Bertuccini, R. Russo, F. Iosi and F. Superti, Int. J. Immunopathol. Pharmacol., 2017, 30, 163–167 CrossRef PubMed.
- E. A. Smith and G. T. Macfarlane, Anaerobe, 1997, 3, 327–337 CrossRef CAS PubMed.
- E. Diaz, A. Ferrandez, M. A. Prieto and J. L. Garcia, Microbiol. Mol. Biol. Rev., 2001, 65, 523–569 CrossRef CAS PubMed.
- I. K. Yap, J. V. Li, J. Saric, F. P. Martin, H. Davies, Y. Wang and E. Holmes, J. Proteome Res., 2008, 7, 3718–3728 CrossRef CAS PubMed.
- H. Sokol, C. Lay, P. Seksik and G. W. Tannock, Inflamm. Bowel. Dis., 2008, 14, 858–867 CrossRef PubMed.
- M. T. Yokoyama and J. R. Carlson, Appl. Environ. Microbiol., 1981, 41, 71–76 CAS.
- J. M. Ridlon, S. C. Harris, S. Bhowmik, D. J. Kang and P. B. Hylemon, Gut Microbes, 2016, 7, 22–39 CrossRef CAS PubMed.
- J. R. Swann, E. J. Want, F. M. Geier, K. Spagou, I. D. Wilson, J. E. Sidaway and E. Holmes, Proc. Natl. Acad. Sci. U. S. A., 2011, 108, 4523–4530 CrossRef CAS PubMed.
- K. S. Islam, S. Fukiya, M. Hagio, N. Fujii, S. Ishizuka, T. Ooka and A. Yokota, Gastroenterology, 2011, 141, 1773–1781 CrossRef CAS PubMed.
- B. V. Jones, M. Begley, C. Hill, C. G. Gahan and J. R. Marchesi, Proc. Natl. Acad. Sci. U. S. A., 2008, 105, 13580–13585 CrossRef CAS PubMed.
- Z. Wang, E. Klipfell, B. J. Bennett, R. Koeth, B. S. Levison, B. DuGar and Y. Wu, Nature, 2011, 472, 57–63 CrossRef CAS PubMed.
- W. K. Wu, S. Panyod, C. T. Ho, C. H. Kuo, M. S. Wu and L. Y. Sheen, J. Funct. Foods, 2015, 15, 408–417 CrossRef CAS.
- X. S. Li, S. Obeid, R. Klingenberg, B. Gencer, F. Mach, L. Räber and M. Miranda, Eur. Heart J., 2017, 582 CrossRef PubMed.
- J. F. Brugère, G. Borrel, N. Gaci, W. Tottey, P. W. O'Toole and C. Malpuech-Brugère, Gut Microbes, 2014, 5–10 CrossRef PubMed.
- M. P. Bryant and N. Small, J. Bacteriol., 1956, 72, 22 CAS.
Footnotes |
† Electronic supplementary information (ESI) available. See DOI: 10.1039/c7ra07651g |
‡ Accession codes: The GenBank submission accession numbers are MF409382–MF409394. |
|
This journal is © The Royal Society of Chemistry 2017 |
Click here to see how this site uses Cookies. View our privacy policy here.