DOI:
10.1039/C6RA28337C
(Paper)
RSC Adv., 2017,
7, 19894-19903
High-throughput and multi-dimensional omics approach uncovers a huaxian capsule to ameliorate the dysregulated expression profiling of severe sepsis rats†
Received
17th December 2016
, Accepted 28th March 2017
First published on 4th April 2017
Abstract
Multi-dimensional omics could be helpful to interpret the underlying mechanisms of disease. Severe sepsis (SS) is a major cause of mortality and morbidity in intensive care units and has a large burden on healthcare due to a lack of effective drugs. The underlying pathophysiology of SS is also poorly understood. A huaxian capsule (HXC) is a herbal preparation with putative effects for SS treatment. Here, we aimed to investigate the metabolic changes in SS rats by performing metabolic profiling and biomarker analysis. The phenotypic response was assessed by UPLC/MS combined with chemometrics. As a result, 12 potential metabolite biomarkers were identified and involved in multiple dysregulated metabolic pathways, such as glycerophospholipid metabolism, steroid hormone biosynthesis, and disrupted sphingolipid metabolism, etc. Then, we performed microRNA analysis to reveal that the HXC ameliorates the dysregulated expression profiling of SS. We identified 56 miRNAs that were differentially expressed in the HXC group compared with the model group, of which 20 were down-regulated and 36 were up-regulated. This study showed that microRNA and metabolic profiling is a valuable approach for exploring metabolism responses to herbal drugs, and can improve our understanding of the molecular basis of the SS treatment.
1. Introduction
Metabolomics, the global assessment of endogenous small molecule metabolites in a biological sample, provides a powerful platform for identifying biomarkers to improve the treatment of disease,1,2 identify perturbed pathways,3,4 and discover new drugs.5 By analyzing and verifying the significant difference in metabolic profiles and changes of metabolite biomarkers, metabolomics enables us to better understand metabolic pathways that can clarify the mechanism of traditional Chinese medicines (TCM).6–9 It enables us to better understand substance metabolic pathways which can explain the mechanism of action, and has been successfully used in various fields of TCM research.10–12 Ultra performance liquid chromatography coupled with quadrupole time-of-flight mass spectrometry (UPLC-Q-TOF/MS) technique has been widely used for metabolomic analysis in TCM.13
Severe sepsis (SS) has been considered a life-threatening clinical syndrome throughout the world.14–16 Also, there is an urgent need for reliable biomarkers for therapeutically evaluation of the SS disease. Despite recent advances in antibiotic therapies, sepsis remains a leading cause of morbidity and mortality in critically ill patients.17 TCM has been used as a regular treatment for many diseases in many Asian countries.18 Metabolomics has become an important tool for assessing the effects of herbs and TCM formulae.19 Huaxian capsule (HXC), TCM formulae, has been widely used for the clinical treatment of SS for many years.20 Comprehensive mechanisms of the pharmacological effects of HXC remain to be elucidated. miRNAs are endogenous non-coding single-stranded RNA molecules made of approximately 22 nucleotides. They can play an important role in the regulation of metabolic profile. Recent studies highlight the use of new miRNAs biomarkers for sepsis. The differential expression of miRNAs in the pathogenesis of sepsis including miR-150, miR-133a, miR-122, miR-223, miR-4772, miR-297 and miR-574-5p, etc.,21–25 can be used as biomarkers for the diagnosis and prognosis of sepsis. Zhou et al. had showed that the upregulation of MicroRNA-205-5b as a potential therapeutic target for the treatment of sepsis.26 Some evidence recently showed that the abnormal expression of miRNA is closely related with the occurrence and development of diseases. Therefore, the present study using high-throughput microRNA and metabolome expression profiling method was conducted to investigate the metabolic mechanisms of HXC exerting its therapeutic effect in a model of SS.
2. Materials and methods
2.1 Chemicals and reagents
Acetonitrile and methanol (HPLC grade) were purchased from Fisher Corporation (Loughborough, UK); water was produced by a Milli-Q Ultra-pure water system (Millipore, USA); formic acid was obtained from Honeywell Company (Morristown, USA); leucine enkephalin was purchased from Sigma-Aldrich (St. Louis, USA). All other reagents were HPLC grade. HXC was a clinical preparation and obtained from First Affiliated Hospital, Heilongjiang University of Chinese Medicine.
2.2 Animal
Adult male Wistar rats (weighting 220 ± 20 g) were purchased from the SLAC Laboratory Animal Co. (Shanghai, China). Rats were kept in SPF-grade Experimental Animal Houses of Heilongjiang University of Chinese Medicine (Harbin, China) with free access to food and water under standard temperature conditions at 24 ± 2 °C with 40 ± 5% humidity and a 12 h light/dark cycle. The experimental procedures were approved by the Animal Care and Ethics Committee at Heilongjiang University of Chinese Medicine (approval number: HUCM2015-0602).
2.3 Sepsis model
The cecal ligation and puncture (CLP) method was used as a SS model, as described in the literature.27 Under ether anesthesia, a midline incision (approximately 2 cm) was made and the cecum was divided carefully while avoiding all blood vessels. The distal two thirds of the cecum was punctured through twice with an 18-gauge needle and ligated tightly. Then, the cecum was placed back in the peritoneal cavity, and the abdominal cavity was closed. In sham surgical controls, the cecum was exposed but not punctured and ligated before being returned to the abdominal cavity. The animals were allowed to acclimatize and putted in the metabolism cages.
2.4 Sample collection
The rats were randomly divided into model group (n = 8, treated with 0.2 mL solution immediately and 24 hours after CLP), HXC treatment group (n = 8, orally administered at a dose of 2 g kg−1 of body weight once a day and 24 hours after CLP), and sham (control) group (n = 8, treated with shame operation and the same volume of 0.9% saline solution). Drug or vehicle was administrated between 8:00 and 9:00 am to minimize any effects of the circadian rhythm. After 3 consecutive days of drug administration, all of the animals were sacrificed by exsanguination from the abdominal aorta under isoflurane anesthesia. The blood was collected in heparinized tubes and centrifuged at 10
000 rpm for 10 min at 4 °C. Then, the plasma was obtained and stored at −80 °C for analysis.
2.5 Sample preparation and pretreatment
Prior to analysis, plasma samples were thawed at room temperature for 15 min, vortexed vigorously for 10 s, and then 200 μL of methanol was added to 100 μL of the plasma samples and vortexed vigorously for 20 s, in order to extract the metabolites. The sample mixture was allowed to stand for 10 min at 4 °C and centrifuged at 10
000 rpm for 10 min at 4 °C. The supernatant (100 μL) was transferred to afresh tube then evaporated to dryness by nitrogen blowing, then 200 μL of 80% methanol were added and vortex-mixed. The supernatant was then transferred to a auto-sampler injection vial for UPLC/MS analysis. To ensure the analytical variability and to monitor the data acquisition performance in the whole run, pooled quality control (QC) samples were prepared from 10 μL of each sample.
2.6 UPLC-QTOF/MS analysis
2.6.1 UPLC. The UPLC analysis was performed on a Waters ACQUITY UPLC system (Waters Corporation, Milford, MA). An Acquity UPLC BEH-C18 column (2.1 mm × 100 mm × 1.8 μm) was used for all analyses. The column was maintained at 40 °C, and a gradient of 0.1% formic acid in water (solvent A) and acetonitrile (solvent B) was used. The gradient used for plasma samples was as follows: 2.0–25% B over 0–4.0 min; 25–80% B over 4.0–6.0 min; and 80% for 6.0–10 min, 90% for 10–11 min, after which it was returned to 2% B for 1.0 min. The flow rate was set to 0.3 mL min−1, and a 4 μL aliquot of the sample solution was injected into the system. The samples were maintained at 4 °C during the analysis.
2.6.2 Mass spectrometry. Mass spectrometry was performed on a Q-TOF mass spectrometer (Waters, Manchester, U.K.). UHPLC-QTOF-MS was used for the relative quantification analysis of potential biomarkers. The parameters in the source were set as follows: capillary voltage, 2.0 kV; source temperature, 110 °C; desolvation temperature, 500 °C; cone gas flow, 60 L h−1; and desolvation gas flow, 400 L h−1. Centroid data were collected from 50 to 1000 m/z with a scan time of 0.03 s, and an interscan delay of 0.02 s. Data were processed further using MassLynx 4.1 (Waters, Manchester, U.K.). Leucine-enkephalin was used as the lock mass (m/z 556.2771 in ES+ and 554.2615 in ES−).
2.6.3 Data processing and multivariate data analysis. All the data for plasma was processed using MassLynx V4.1 and MarkerLynx software (Waters Corp., Milford, MA). Ion intensity was normalized with respect to the total ion count to generate a data matrix including the retention time, m/z value, and the normalized peak area. The mass data acquired were analyzed using EZinfo 2.0 software (Waters Corp, Milford, USA) for peak detection and alignment. EZinfo 2.0 software was then used to perform unsupervised principal components analysis (PCA) and partial least squares projection to supervised latent structures and discriminant analysis (PLS-DA) and orthogonal partial least-squared discriminate analysis (OPLS-DA). PCA was used to convert multiple original variable spaces into a new set of orthogonal variables, and were employed to reveal the global metabolic changes. PLS-DA and OPLS-DA were applied to determine the various metabolites responsible for the separation between the model and sham-operated groups. The corresponding variable importance in the projection (VIP values) was calculated in the OPLS-DA model as well, a weighted sum of squares, was used to select biomarkers.
2.6.4 Identification of plasma biomarkers. For the identification of potential biomarkers, some available biochemical databases, such as Chemspider, HMDB, KEGG, METLIN, and LIPIDMAPS were used by comparing the accurate mass, fragments information and MS/MS data. Moreover, the potential biomarkers among them were further identified by comparing with reference standards.
2.6.5 Construction of metabolic pathways. The metabolic pathways of endogenous metabolites could be constructed using Metaboanalyst (http://www.metaboanalyst.ca/), based on database sources, including KEGG (http://www.kegg.jp/), HMDB (http://www.hmdb.ca/), and SMPD (http://www.smpdb.ca/), to analyze and visualize the metabolic pathways and facilitate further biological interpretation. SPSS 17.0 using the t test for Windows was used for the statistical analysis.
2.7 microRNA expression analysis
Total RNA was isolated from serum by Trizol method according to the previous method. Serum RNA samples were subjected to miRNA profiling using a TaqMan® human microRNA array (Applied Biosystems Life Technologies, CA, USA). MiRNAs were analyzed with miRNA TaqMan assays (Applied Biosystems Life Technologies) accordingly to the manufacturer's instruction. Reporters on the microarray cover all mature mouse microRNA sequences as annotated in miRBase v16.0. Sequence Detection System software (version 2.2; Applied Biosystems Life Technologies) was used to read the expression signals. Each microRNA reporter was present four times on the array. After hybridization, the microarray slides were washed and scanned with a DNA microarray scanner (Agilent technologies). Feature extraction software v10 (Agilent technologies) was used to convert the scanned images into TXT files, which were imported in R (http://www.r-project.org/) for further downstream analysis. Ward's method and Manhattan distance interpretation were performed for the cluster analysis.
2.8 Ingenuity pathways analysis
To exploring the typical metabolic perturbations associated with the related moleculars, we performed using the IPA system (http://www.ingenuity.com/) which is a web-based software application that identifies biological pathways and functions relevant to bio-molecules of interest. We uploaded the related moleculars onto an IPA server. Canonical pathways and molecular interaction networks were generated based on the IPA knowledge.
3. Results and discussion
3.1 Typical footprint spectra and metabolic changes
Using the optimal chromatography conditions as described, total ion current (TIC) chromatograms of plasma samples for the model group, HXC treatment group and control group were collected in positive and negative modes are shown in Fig. S1.† TIC profiles of typical samples from each group showed in Fig. S1† displays the general information of UPLC-QTOF/MS detection. Scores plots of PCA indicate the similarity of metabolic profiles from various samples. In this study, PCA was first used to detect any inherent trends within the data. The results showed distinct clustering between the control and model group (Fig. 1A and B), and indicated that the metabolic profiling have been significantly perturbed in the model group.
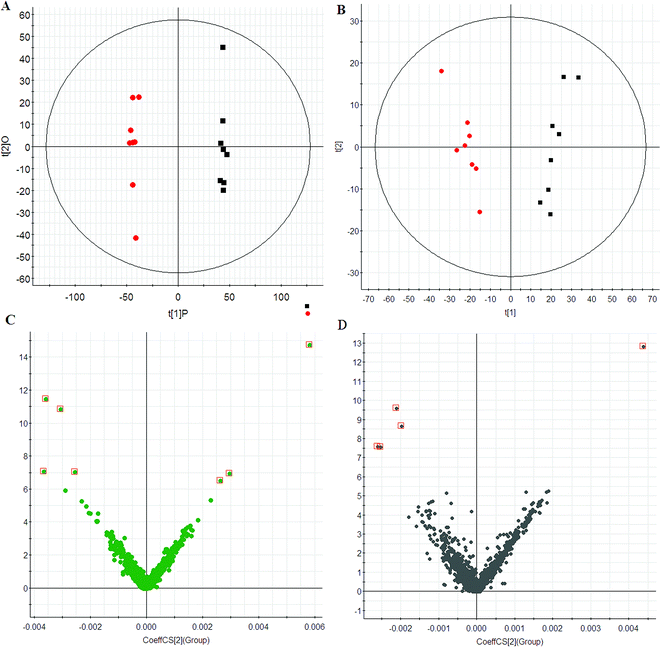 |
| Fig. 1 PCA score plots of plasma of control (red) and model group (black) in ESI+ (A) and ESI− (B) mode. VIP-plots constructed from the supervised OPLS analysis in ESI+ (C) and ESI− (D) mode. | |
3.2 Multivariate statistical analysis
To maximize this distinction, the data were analyzed by OPLS-DA to identify potential biomarkers. VIP-plots from the OPLS-DA analysis (Fig. 1C and D) were used to identify which variables account for such a significant separation. Variables were also generated based on the values of variable importance in the projection (VIP > 6) according to the orders of their contributions to the separation of clustering. Then, by combining Student's t-test with the selected variables, distinct metabolites were identified (p < 0.05) and selected for further study. Based on the VIP plot, 12 endogenous metabolites were selected as biomarkers (Table 1). Following the identification process, these metabolites were identified over a narrow ±5 ppm, including 7 identified in the positive mode and 5 identified in the negative mode. Furthermore, 7 metabolites were upregulated, and 5 metabolites were downregulated in the model group compared to the control group. Intriguingly, 7 of the metabolites detected were found to be up-regulated, while 5 were down-regulated.
Table 1 Information of SS biomarkers detected by UPLC-Q-TOF/MS
No. |
VIP |
Retention time (min) |
m/z |
Adducts |
Compound ID |
Formula |
Mass error (ppm) |
Description |
Anova (p) |
Max fold change |
1 |
11.48 |
8.61 |
734.5740 |
M + H |
HMDB00564 |
C40H80NO8P |
2.29 |
PC(16:0/16:0) |
0.0049 |
5.0330 |
2 |
10.85 |
6.44 |
552.4043 |
M + H |
HMDB10390 |
C28H58NO7P |
3.52 |
LysoPC(20:0) |
0.0843 |
5.4025 |
3 |
7.05 |
4.24 |
496.3421 |
M + H |
HMDB10382 |
C24H50NO7P |
4.77 |
LysoPC(16:0) |
0.0099 |
3.0401 |
4 |
14.74 |
3.46 |
380.2591 |
M + H |
HMDB00277 |
C18H38NO5P |
3.11 |
Sphingosine 1-phosphate |
0.0094 |
2.0049 |
5 |
6.97 |
2.83 |
347.2248 |
M + H |
HMDB00015 |
C21H30O4 |
2.07 |
Cortexolone |
0.0016 |
1.7561 |
6 |
7.08 |
1.16 |
341.1855 |
M + H |
HMDB01091 |
C20H24N2O3 |
−1.43 |
3-Hydroxyquinine |
0.0008 |
17.0374 |
7 |
6.52 |
7.12 |
331.2246 |
M + H |
HMDB00374 |
C21H30O3 |
−1.70 |
17-Hydroxyprogesterone |
0.0022 |
6.1282 |
8 |
9.59 |
3.84 |
303.1515 |
M − H |
HMDB03573 |
C17H20NO4 |
−2.34 |
Scopolamine |
0.0034 |
1.6131 |
9 |
7.58 |
8.44 |
290.2295 |
M − H |
HMDB00031 |
C19H29O2 |
−1.06 |
Androsterone |
0.0021 |
5.1217 |
10 |
12.83 |
4.05 |
257.1114 |
M − H |
HMDB00086 |
C8H19NO6P |
2.94 |
Glycerophosphocholine |
0.0016 |
1.3546 |
11 |
7.61 |
7.93 |
162.1074 |
M − H |
HMDB00450 |
C6H13N2O3 |
−2.05 |
5-Hydroxylysine |
0.0006 |
3.0063 |
12 |
8.66 |
1.51 |
131.0776 |
M − H |
HMDB00064 |
C4H8N3O2 |
2.21 |
Creatine |
0.0058 |
2.8729 |
3.3 Metabolic pathway analysis
To gain insight into the metabolic mechanism of SS and to provide accurate treatment informations of SS, altered metabolic pathway associated with SS has been further investigated. To determine possible pathways contributing to SS, MetPA analysis was performed to identify metabolic pathways and their networks using an online database. This analysis resulted in the construction of 8 metabolic pathways in the plasma (Fig. S2† and Table 2) that were important for host-responses to SS. Among the identified metabolic pathways, glycerophospholipid metabolism (impact-value: 0.21) and steroid hormone biosynthesis (impact-value: 0.09), and sphingolipid metabolism (impact-value: 0.03) in plasma were determined to be the most important. This change indicates that SS had significantly altered metabolism compared to its normal state. Previous study determined that,28 by using a targeted MS-based quantitative metabolomics approach, glycerophospholipid metabolism was shown to be disturbed in SS disease patients, and relevant endogenous metabolites were detected. These discoveries supported our results regarding metabolism and metabolites. Based on these findings, we believe that glycerophospholipid metabolism regulation plays an important role in SS disease. Early changes in the plasma levels of these metabolites associated with mortality have potential implications for early intervention.
Table 2 Result from pathway analysis with MetPAa
No. |
Pathway name |
Total |
Expected |
Hits |
Raw p |
Impact |
Total is the total number of compounds in the pathway; the hits is the actually matched number from the user uploaded data; the impact is the pathway impact value calculated from pathway topology analysis. |
1 |
Glycerophospholipid metabolism |
30 |
0.2354 |
3 |
0.0013 |
0.2065 |
2 |
Steroid hormone biosynthesis |
70 |
0.5492 |
3 |
0.0147 |
0.0906 |
3 |
Sphingolipid metabolism |
21 |
0.1648 |
1 |
0.1534 |
0.0301 |
4 |
Arginine and proline metabolism |
44 |
0.3452 |
1 |
0.2967 |
0.0120 |
5 |
Linoleic acid metabolism |
5 |
0.0392 |
1 |
0.0387 |
0 |
6 |
Alpha-linolenic acid metabolism |
9 |
0.07061 |
1 |
0.0686 |
0 |
7 |
Glycine, serine and threonine metabolism |
32 |
0.2511 |
1 |
0.2250 |
0 |
8 |
Arachidonic acid metabolism |
36 |
0.2825 |
1 |
0.2496 |
0 |
3.4 Metabolic effects of HXC treatment
The PCA score plot was used to investigate the metabolic effects of intervention with the HXC. As shown in Fig. 2A and B, the clusters representing the HXC treatment group was located between the clusters representing the model and control, which demonstrated that the disturbed metabolic states of model group was in the process of returning to the normal state after HXC treatment (Fig. 2C). Compared to the model group, the HXC treatment group showed the recovery performance from the model metabolic state. The score plot in the negative mode also showed that the HXC-treated group cluster was closer to that of the control groups than the model group cluster, which demonstrates that HXC has therapeutic effects on SS rats. Ten metabolites were reversed by HXC, and the metabolites that were reversed are primarily involved in glycerophospholipid metabolism and steroid hormone biosynthesis, and sphingolipid metabolism, which indicate that the effectiveness of HXC as an SS treatment depends on modulating metabolism pathways.
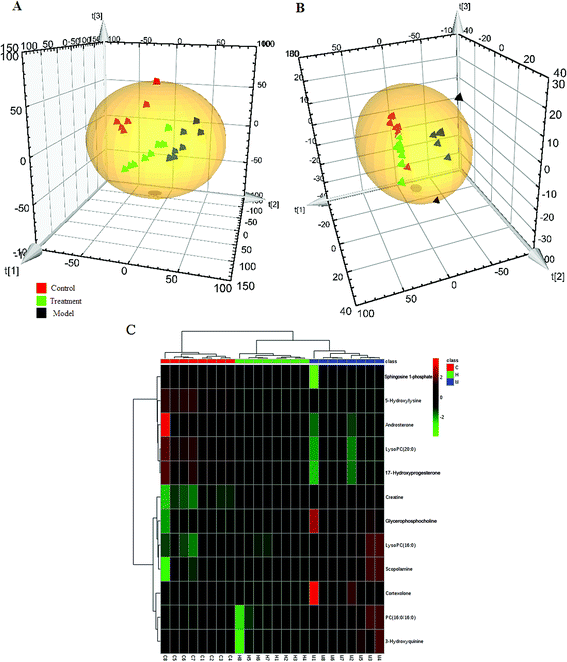 |
| Fig. 2 PCA scores plot of HXC affecting on SS rats in (A) ESI+ and ESI− (B) mode. Heat maps (C) of all the potential biomarkers in response to HXC detected by cluster analysis. The columns show the expression levels and each row represents a biomarker. The red color indicates upregulated biomarkers compared with normal control group, while the green color represents downregulated biomarkers compared with normal control group. C, control group; M, model group; H, HXC group (n = 8). | |
3.5 miRNA profiles after HXC treatment
We then investigated the HXC influence on the microRNAome in the SS. PCA scores plot of HXC affecting on SS rats showed patterns of differentially expressed microRNAs abundance among the HXC group and model group (Fig. 3A). A total of 1547 miRNAs were detected, and of which 56 miRNAs were significantly changed during HXC treatment compared with the untreated group (Fig. 3B). Our data analysis showed that 36 of the altered miRNAs were upregulated, whereas 20 miRNAs were downregulated after HXC treatment relative to the model group (Fig. 3C, Table 3). Relative expression levels (upregulation in red and downregulation in green) of the differentially expressed miRNAs were shown in Fig. 3D. Clustering analysis showed the clear differentiation patterns of differentially expressed microRNAs abundance among the HXC group and model group. In the network function analysis using IPA software, the related miRNAs and metabolites tended to gather into an integrated network (Fig. 4).
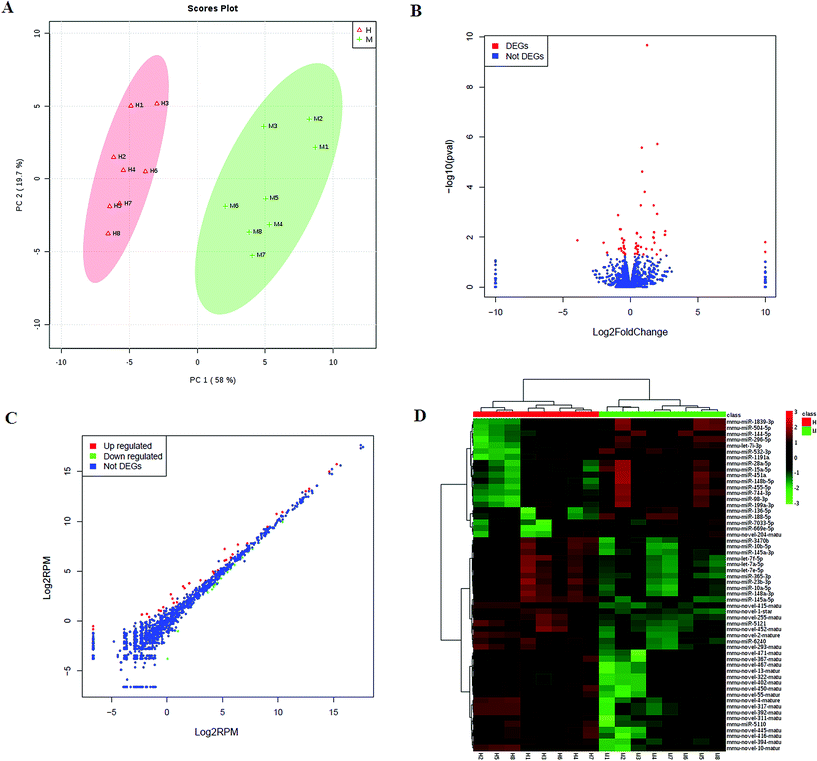 |
| Fig. 3 miRNAome analysis of HXC ameliorating the dysregulated expression profiling. (A) PCA score plot of global profiling of gene expression changes; (B) volcano plot of the differential expression of miRNA; (C) scatter plot showing expression changes of the related miRNA; (D) heat map expression levels (upregulation in red and downregulation in blue) of differentially expressed miRNAs. | |
Table 3 Summary of the differentially expressed miRNA
No. |
Type |
id |
Base mean |
Base mean A |
Base mean B |
Fold change |
log2 fold change |
p val. |
1 |
Up |
mmu-novel-1-star |
666.4979 |
396.3943 |
936.6016 |
2.3628 |
1.2405 |
0.0000 |
2 |
Up |
mmu-novel-394-mature |
32.8982 |
13.0851 |
52.7113 |
4.0283 |
2.0102 |
0.0000 |
3 |
Up |
mmu-miR-6240 |
6188.5372 |
4423.2161 |
7953.8583 |
1.7982 |
0.8466 |
0.0000 |
4 |
Up |
mmu-novel-255-mature |
245.6712 |
172.0729 |
319.2695 |
1.8554 |
0.8918 |
0.0000 |
5 |
Up |
mmu-novel-415-mature |
59.2046 |
38.2653 |
80.1440 |
2.0944 |
1.0666 |
0.0002 |
6 |
Up |
mmu-miR-10b-5p |
262.0001 |
192.5896 |
331.4106 |
1.7208 |
0.7831 |
0.0005 |
7 |
Up |
mmu-novel-445-mature |
14.2682 |
6.6009 |
21.9355 |
3.3231 |
1.7325 |
0.0005 |
8 |
Up |
mmu-novel-2-mature |
434.9094 |
174.9023 |
694.9166 |
3.9732 |
1.9903 |
0.0012 |
9 |
Up |
mmu-novel-471-mature |
4.5431 |
1.2930 |
7.7933 |
6.0271 |
2.5915 |
0.0059 |
10 |
Up |
mmu-novel-317-mature |
42.1480 |
19.1302 |
65.1659 |
3.4064 |
1.7683 |
0.0064 |
11 |
Up |
mmu-novel-392-mature |
42.1480 |
19.1302 |
65.1659 |
3.4064 |
1.7683 |
0.0064 |
12 |
Up |
mmu-miR-23b-3p |
7670.0160 |
6376.9757 |
8963.0563 |
1.4055 |
0.4911 |
0.0068 |
13 |
Up |
mmu-miR-10a-5p |
33166.1028 |
27561.8588 |
38770.3469 |
1.4067 |
0.4923 |
0.0072 |
14 |
Up |
mmu-novel-467-mature |
4.3657 |
1.2577 |
7.4737 |
5.9426 |
2.5711 |
0.0082 |
15 |
Up |
mmu-novel-13-mature |
4.3657 |
1.2577 |
7.4737 |
5.9426 |
2.5711 |
0.0082 |
16 |
Up |
mmu-miR-5121 |
379.6218 |
281.3392 |
477.9043 |
1.6987 |
0.7644 |
0.0099 |
17 |
Up |
mmu-novel-311-mature |
8.1007 |
3.8030 |
12.3984 |
3.2601 |
1.7049 |
0.0103 |
18 |
Up |
mmu-novel-4-mature |
29.8759 |
13.8661 |
45.8858 |
3.3092 |
1.7265 |
0.0107 |
19 |
Up |
mmu-novel-322-mature |
1.6491 |
0.0000 |
3.2982 |
Inf |
Inf |
0.0162 |
20 |
Up |
mmu-novel-450-mature |
6.3107 |
2.8244 |
9.7970 |
3.4687 |
1.7944 |
0.0169 |
21 |
Up |
mmu-let-7a-5p |
40121.4862 |
34264.3637 |
45978.6086 |
1.3419 |
0.4243 |
0.0181 |
22 |
Up |
mmu-novel-293-mature |
319.8828 |
254.0713 |
385.6942 |
1.5181 |
0.6022 |
0.0222 |
23 |
Up |
mmu-novel-452-mature |
136.8319 |
112.3499 |
161.3138 |
1.4358 |
0.5219 |
0.0224 |
24 |
Up |
mmu-miR-148a-3p |
221101.5993 |
189832.7475 |
252370.4511 |
1.3294 |
0.4108 |
0.0246 |
25 |
Up |
mmu-miR-145a-3p |
114.7529 |
83.8442 |
145.6616 |
1.7373 |
0.7968 |
0.0248 |
26 |
Up |
mmu-let-7e-5p |
2899.1864 |
2501.0354 |
3297.3374 |
1.3184 |
0.3988 |
0.0280 |
27 |
Up |
mmu-novel-416-mature |
11.7327 |
7.1820 |
16.2833 |
2.2672 |
1.1809 |
0.0295 |
28 |
Up |
mmu-miR-145a-5p |
108.7822 |
89.0289 |
128.5356 |
1.4438 |
0.5298 |
0.0311 |
29 |
Up |
mmu-novel-367-mature |
6.4195 |
3.1972 |
9.6418 |
3.0157 |
1.5925 |
0.0319 |
30 |
Up |
mmu-novel-10-mature |
17.7868 |
6.2564 |
29.3172 |
4.6859 |
2.2283 |
0.0333 |
31 |
Up |
mmu-miR-3470b |
64.7508 |
52.2605 |
77.2412 |
1.4780 |
0.5636 |
0.0394 |
32 |
Up |
mmu-novel-402-mature |
1.3296 |
0.0000 |
2.6591 |
Inf |
Inf |
0.0400 |
33 |
Up |
mmu-miR-365-3p |
875.7686 |
718.1130 |
1033.4242 |
1.4391 |
0.5251 |
0.0478 |
34 |
Up |
mmu-let-7f-5p |
135877.6892 |
118972.1659 |
152783.2125 |
1.2842 |
0.3609 |
0.0479 |
35 |
Up |
mmu-miR-5110 |
13.2248 |
8.9027 |
17.5470 |
1.9710 |
0.9789 |
0.0480 |
36 |
Up |
mmu-novel-55-mature |
3.8433 |
1.5650 |
6.1216 |
3.9116 |
1.9677 |
0.0499 |
37 |
Down |
mmu-miR-296-5p |
59.9804 |
78.2198 |
41.7410 |
0.5336 |
−0.9061 |
0.0013 |
38 |
Down |
mmu-miR-144-5p |
68.8227 |
86.5423 |
51.1031 |
0.5905 |
−0.7600 |
0.0048 |
39 |
Down |
mmu-let-7i-3p |
88.9647 |
110.5178 |
67.4116 |
0.6100 |
−0.7132 |
0.0050 |
40 |
Down |
mmu-miR-1839-3p |
113.5582 |
137.2077 |
89.9088 |
0.6553 |
−0.6098 |
0.0111 |
41 |
Down |
mmu-miR-451a |
842.2556 |
978.8098 |
705.7014 |
0.7210 |
−0.4720 |
0.0136 |
42 |
Down |
mmu-miR-28a-5p |
5481.6778 |
6337.6021 |
4625.7536 |
0.7299 |
−0.4542 |
0.0137 |
43 |
Down |
mmu-miR-669e-5p |
2.5718 |
4.8241 |
0.3195 |
0.0662 |
−3.9163 |
0.0138 |
44 |
Down |
mmu-miR-7033-5p |
5.7651 |
9.1906 |
2.3396 |
0.2546 |
−1.9739 |
0.0165 |
45 |
Down |
mmu-miR-532-3p |
171.1450 |
201.5868 |
140.7032 |
0.6980 |
−0.5187 |
0.0167 |
46 |
Down |
mmu-miR-455-5p |
276.8424 |
321.7984 |
231.8864 |
0.7206 |
−0.4727 |
0.0218 |
47 |
Down |
mmu-miR-98-3p |
210.4459 |
244.9304 |
175.9614 |
0.7184 |
−0.4771 |
0.0258 |
48 |
Down |
mmu-miR-504-5p |
76.7630 |
94.9908 |
58.5353 |
0.6162 |
−0.6985 |
0.0285 |
49 |
Down |
mmu-miR-136-5p |
46.3123 |
59.3736 |
33.2510 |
0.5600 |
−0.8364 |
0.0300 |
50 |
Down |
mmu-miR-15a-5p |
1262.6993 |
1441.5507 |
1083.8479 |
0.7519 |
−0.4115 |
0.0303 |
51 |
Down |
mmu-miR-188-5p |
70.7487 |
86.1537 |
55.3437 |
0.6424 |
−0.6385 |
0.0351 |
52 |
Down |
mmu-miR-1191a |
80.9246 |
95.0386 |
66.8107 |
0.7030 |
−0.5084 |
0.0416 |
53 |
Down |
mmu-miR-744-3p |
137.7009 |
159.8748 |
115.5271 |
0.7226 |
−0.4687 |
0.0417 |
54 |
Down |
mmu-novel-204-mature |
5.1190 |
7.8533 |
2.3847 |
0.3037 |
−1.7195 |
0.0427 |
55 |
Down |
mmu-miR-148b-5p |
653.3687 |
739.5400 |
567.1974 |
0.7670 |
−0.3828 |
0.0477 |
56 |
Down |
mmu-miR-199a-3p |
456.5908 |
517.4811 |
395.7005 |
0.7647 |
−0.3871 |
0.0482 |
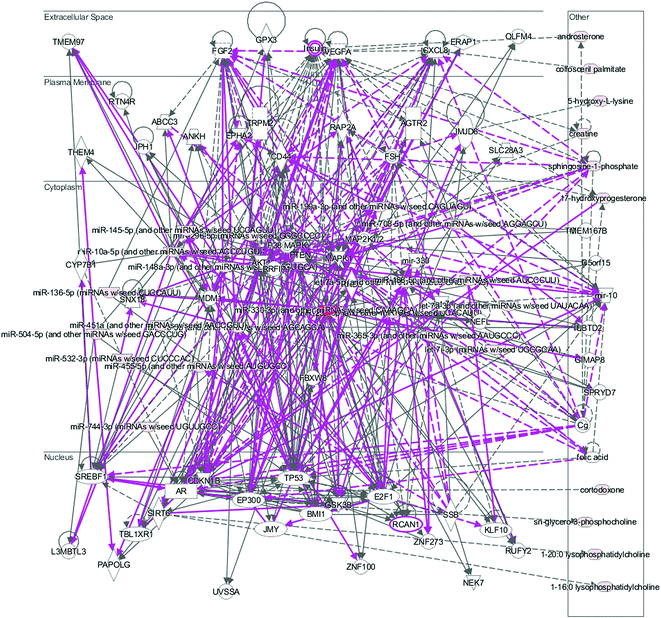 |
| Fig. 4 The merged networks of the differentially expressed moleculars. | |
Our study used plasma metabolomics technology to explore changes in the metabolic regulation in response to experimental SS rats and HXC treatment. In this study, the 12 potential metabolite biomarkers identified suggest that multiple metabolic pathways were disturbed in SS rats. These biomarkers regulated the glycerophospholipid metabolism, steroid hormone biosynthesis, and sphingolipid metabolism, in addition to playing an important role in other metabolic pathways. Based on the results of this study, it can be suggested that SS is treated by HXC via the disrupted pathways. miRNAs have recently been used as potential biomarker for the diagnosis and prognosis of sepsis, and have been validated to be potential sepsis biomarker. Recently, a report from Zheng et al. found that the silencing of miR-195 can improve the survival in sepsis, and the inhibition of miR-195 may represent a new therapeutic approach for sepsis.29 Another group showed that the upregulation of miR-205-5b is a potential therapeutic target for the treatment of sepsis.30 A variety of miRNAs such as miR-150, miR-15a/16, miR-132, miR-122, and miR-27a as biomarkers, were described in the context of sepsis.31–36 Importantly, our results demonstrated differential expression of 36 upregulated and 20 downregulated miRNAs in HXC treatment group compared with SS rats. This study would lead to a further understanding of the HXC regulating SS disease and treatment. We conclude that high-throughput microRNA and metabolome expression profiling could aid into the elucidation of the mechanism of SS and the therapeutic mechanism of HXC.
Conflict of interest
The authors declare no competing financial interests.
Abbreviations
SS | Severe sepsis |
HXC | Huaxian capsule |
TCM | Traditional Chinese medicines |
UPLC-Q-TOF/MS | Ultra performance liquid chromatography coupled with quadrupole time-of-flight mass spectrometry |
CLP | The cecal ligation and puncture |
QC | Quality control |
PCA | Principal components analysis |
PLS-DA | Partial least squares projection to supervised latent structures and discriminant analysis |
OPLS-DA | Orthogonal partial least-squared discriminate analysis |
TIC | Total ion current |
Acknowledgements
We thank BGI for excellent technical assistance. This work was supported by grants from the Key Program of Natural Science Foundation of State (Grant No. 81470196,81302905), Natural Science Foundation of Heilongjiang Province of China (H2015038), University Nursing Program for Young Scholars with Creative Talents in Heilongjiang Province (UNPYSCT-2015118).
References
- J. M. Kinross, N. Alkhamesi, R. H. Barton, D. B. Silk, I. K. Yap, A. W. Darzi, E. Holmes and J. K. Nicholson, Global metabolic phenotyping in an experimental laparotomy model of surgical trauma, J. Proteome Res., 2011, 10(1), 277–287 CrossRef CAS PubMed.
- M. E. Dumas, J. Kinross and J. K. Nicholson, Metabolic phenotyping and systems biology approaches to understanding metabolic syndrome and fatty liver disease, Gastroenterology, 2014, 146(1), 46–62 CrossRef PubMed.
- J. M. Kinross, E. Holmes, A. W. Darzi and J. K. Nicholson, Metabolic phenotyping for monitoring surgical patients, Lancet, 2011, 377(9780), 1817–1819 CrossRef.
- B. Jiménez, R. Mirnezami, J. Kinross, O. Cloarec, H. C. Keun, E. Holmes, R. D. Goldin, P. Ziprin, A. Darzi and J. K. Nicholson, 1H HR-MAS NMR spectroscopy of tumor-induced local metabolic “field-effects” enables colorectal cancer staging and prognostication, J. Proteome Res., 2013, 12(2), 959–968 CrossRef PubMed.
- J. C. Lindon, E. Holmes and J. K. Nicholson, Metabonomics in pharmaceutical R&D, FEBS J., 2007, 274(5), 1140–1151 CrossRef CAS PubMed.
- H. Chen, G. Cao, D. Q. Chen, M. Wang, N. D. Vaziri, Z. H. Zhang, J. R. Mao, X. Bai and Y. Y. Zhao, Metabolomics insights into activated redox signaling and lipid metabolism dysfunction in chronic kidney disease progression, Redox Biol., 2016, 10, 168–178 CrossRef CAS PubMed.
- Y. Li, S. Qiu and A. Zhang, High-throughput metabolomics to identify metabolites serve as diagnostic biomarkers of prostate cancer, Anal. Methods, 2016, 8, 3284–3290 RSC.
- T. Jiang, J. Qian, J. Ding, G. Wang, X. Ding, S. Liu and W. Chen, Metabolomic profiles delineate the effect of Sanmiao wan on hyperuricemia in rats, Biomed. Chromatogr., 2017, 31(2) DOI:10.1002/bmc.3792.
- J. Fang, W. Wang, S. Sun, Y. Wang, Q. Li, X. Lu, M. Qiu and Y. Zhang, Metabolomics study of renal fibrosis and intervention effects of total aglycone extracts of Scutellaria baicalensis in unilateral ureteral obstruction rats, J. Ethnopharmacol., 2016, 192, 20–29 CrossRef CAS PubMed.
- L. Zhao, A. Zhao, T. Chen, W. Chen, J. Liu, R. Wei, J. Su, X. Tang, K. Liu, R. Zhang, G. Xie, J. Panee, M. Qiu and W. Jia, Global and Targeted Metabolomics Evidence of the Protective Effect of Chinese Patent Medicine Jinkui Shenqi Pill on Adrenal Insufficiency after Acute Glucocorticoid Withdrawal in Rats, J. Proteome Res., 2016, 15(7), 2327–2336 CrossRef CAS PubMed.
- C. Lu, X. Zhao, Y. Li, Y. Li, C. Yuan, F. Xu, X. Meng, L. Hou and G. Xu, Serum metabolomics study of Traditional Chinese medicine formula intervention to polycystic ovary syndrome, J. Pharm. Biomed. Anal., 2016, 120, 127–133 CrossRef CAS PubMed.
- Y. N. Song, G. B. Zhang, Y. Y. Lu, Q. L. Chen, L. Yang, Z. T. Wang, P. Liu and S. B. Su, Huangqi decoction alleviates dimethylnitrosamine-induced liver fibrosis: An analysis of bile acids metabolic mechanism, J. Ethnopharmacol., 2016, 189, 148–156 CrossRef CAS PubMed.
- Q. Liang, T. Zhang and Y. Jiang, et al., Metabolomics-Based Screening of Salivary Biomarkers for Early Diagnosis of Alzheimer's Disease, RSC Adv., 2015, 5, 96074–96079 RSC.
- X. Shi, F. Yang and Y. N. Zheng, et al., Metabolomic approach for the identification of therapeutic targets of erythropoietin against sepsis in rat models, Eur. Rev. Med. Pharmacol. Sci., 2016, 20(3), 537–546 CAS.
- Q. Liang, H. Liu and T. Zhang, et al., High-throughput metabolic profiling for discovering metabolic biomarkers of sepsis-induced acute lung injury, RSC Adv., 2016, 6, 11008–11013 RSC.
- M. Garcia-Simon, J. M. Morales and V. Modesto-Alapont, et al., Prognosis Biomarkers of Severe Sepsis and Septic Shock by 1H NMR Urine Metabolomics in the Intensive Care Unit, PLoS One, 2015, 10(11), e0140993 Search PubMed.
- Q. Liang, H. Liu and T. Zhang, et al., Potential urine biomarkers from a high throughput metabolomics study of severe sepsis in a large Asian cohort, RSC Adv., 2015, 5, 102204–102209 RSC.
- Q. Liang, H. Liu and C. Wang, et al., Phenotypic Characterization Analysis of Human Hepatocarcinoma by Urine Metabolomics Approach, Sci. Rep., 2016, 6, 19763 CrossRef CAS PubMed.
- J. Chen, J. Zhou, S. Wei, Z. Xie, C. Wen and G. Xu, Effect of a traditional Chinese medicine prescription Quzhuotongbi decoction on hyperuricemia model rats studied by using serum metabolomics based on gas chromatography-mass spectrometry, J. Chromatogr. B, 2016, 1026, 272–278 CrossRef CAS PubMed.
- Q. Liang, H. Liu and H. Xing, et al., Urinary UPLC-MS metabolomics dissecting the underlying mechanisms of huaxian capsule protects against sepsis, RSC Adv., 2016, 6, 40436–40441 RSC.
- V. van der Heide, P. Möhnle and J. Rink, et al., Down-regulation of MicroRNA-31 in CD4+ T Cells Contributes to Immunosuppression in Human Sepsis by Promoting TH2 Skewing, Anesthesiology, 2016, 124(4), 908–922 CrossRef CAS PubMed.
- H. Lin, Z. Mo and H. Su, et al., Progress in circulating microRNAs as biomarkers of sepsis, Zhonghua Weizhongbing Jijiu Yixue, 2016, 28(8), 752–755 Search PubMed.
- H. Wang, B. Yu and J. Deng, et al., Serum miR-122 correlates with short-term mortality in sepsis patients, Crit. Care, 2014, 18(6), 704 CrossRef PubMed.
- H. J. Wang, P. J. Zhang and W. J. Chen, et al., Characterization and Identification of novel serum microRNAs in sepsis patients with different outcomes, Shock, 2013, 39(6), 480–487 CrossRef CAS PubMed.
- J. Huang, Z. Sun and W. Yan, et al., Identification of microRNA as sepsis biomarker based on miRNAs regulatory network analysis, BioMed Res. Int., 2014, 2014, 594350 Search PubMed.
- W. Zhou, J. Wang and Z. Li, et al., MicroRNA-205-5b inhibits HMGB1 expression in LPS-induced sepsis, Int. J. Mol. Med., 2016, 38(1), 312–318 CAS.
- K. A. Wichterman, A. E. Baue and I. H. Chaudry, Sepsis and septic shock–a review of laboratory models and a proposal, J. Surg. Res., 1980, 29(2), 189–201 CrossRef CAS PubMed.
- M. Ferrario, A. Cambiaghi and L. Brunelli, et al., Mortality prediction in patients with severe septic shock: a pilot study using a target metabolomics approach, Sci. Rep., 2016, 6, 20391 CrossRef CAS PubMed.
- D. Zheng, Y. Yu and M. Li, et al., Inhibition of MicroRNA 195 Prevents Apoptosis and Multiple-Organ Injury in Mouse Models of Sepsis, J. Infect. Dis., 2016, 213(10), 1661–1665 CrossRef PubMed.
- W. Zhou, J. Wang and Z. Li, et al., MicroRNA-205-5b inhibits HMGB1 expression in LPS-induced sepsis, Int. J. Mol. Med., 2016, 38(1), 312–318 CAS.
- R. Dumache, A. F. Rogobete and O. H. Bedreag, et al., Use of miRNAs as biomarkers in sepsis, Anal. Cell. Pathol., 2015, 2015, 186716 Search PubMed.
- S. Caserta, F. Kern and J. Cohen, et al., Circulating Plasma microRNAs can differentiate Human Sepsis and Systemic Inflammatory Response Syndrome (SIRS), Sci. Rep., 2016, 6, 28006 CrossRef CAS PubMed.
- X. Wang, X. Wang and X. Liu, et al., miR-15a/16 are unregulated in the serum of neonatal sepsis patients and inhibit the LPS-induced inflammatory pathway, Int. J. Clin. Exp. Med., 2015, 8(4), 5683–5690 CAS.
- F. Liu, Y. Li and R. Jiang, et al., miR-132 inhibits lipopolysaccharide-induced inflammation in alveolar
macrophages by the cholinergic anti-inflammatory pathway, Exp. Lung Res., 2015, 41(5), 261–269 CrossRef CAS PubMed.
- H. Wang, B. Yu and J. Deng, et al., Serum miR-122 correlates with short-term mortality in sepsis patients, Crit. Care, 2014, 18(6), 704 CrossRef PubMed.
- Z. Wang, Z. Ruan and Y. Mao, et al., miR-27a is up regulated and promotes inflammatory response in sepsis, Cell. Immunol., 2014, 290(2), 190–195 CrossRef CAS PubMed.
Footnote |
† Electronic supplementary information (ESI) available. See DOI: 10.1039/c6ra28337c |
|
This journal is © The Royal Society of Chemistry 2017 |
Click here to see how this site uses Cookies. View our privacy policy here.