DOI:
10.1039/C6RA28152D
(Paper)
RSC Adv., 2017,
7, 22034-22044
From chemical markers to quality markers: an integrated approach of UPLC/Q-TOF, NIRS, and chemometrics for the quality assessment of honeysuckle buds†
Received
13th December 2016
, Accepted 6th April 2017
First published on 19th April 2017
Abstract
Because of the poor discovery rate of relatively effective components and elusive component-effect correlation, formulating a quality control system for Chinese herbal medicines (CHMs) has been a great challenge for quality management. In this paper, the concept of the quality marker (Q-marker) was used, and a set of integrated strategies to improve the chemical markers of the Q-markers was introduced. Two often confused CHMs, Lonicera japonica flos (LJF) and Lonicera flos (LF), which are of the same genus but different species, are illustrated to quickly evaluate their potency. Ultra-performance liquid chromatography-quadrupole/time-of-flight (UPLC/Q-TOF) with partial least squares-discriminant analysis (PLS-DA) was used to screen the chemical markers for their herbal origin identification; then, a bioactive-guided evaluation method was performed to detect the Q-markers. As a result, four NF-κB inhibitors were proposed to be representative Q-markers for the anti-inflammatories: 3-O-caffeoylquinic acid (CA), 3,5-O-dicaffeoylquinic acid (3,5-diCQA), `iamarin, and vogeloside. After the chemometrics study, near-infrared spectroscopy (NIRS) based on the distinctive wavenumber points from the Q-markers was developed for its distinction and determination capabilities by optimum siPLS-CARS analysis (OPSC). Then, the back propagating-artificial neutral network (BP-ANN) algorithm was used to clarify the non-linear relationship between the Q-markers and their integral anti-inflammation effect. Finally, convenient and reliable fast quantitative analysis and holistic bioactivity assessment patterns were established by NIRS for the quality management of honeysuckle buds. The integrated Q-marker screen and NIRS assessment strategy was suitable for a fast quality evaluation of herbal medicines and was applied to the quality control of botanical functional foods.
1. Introduction
Because of the challenge of the increasing costs in drug research and the lack of new effective drugs to alleviate chronic illnesses, traditional Chinese medicines (TCMs) are increasingly being used with conventional medical practices in the treatment of today's complex diseases that may not be addressed by only one medical system.1 To facilitate the standard improvement of Chinese herbal medicines (CHMs), in the last few decades, many systematic studies on TCMs have centered on identifying the chemical components, pharmaceutical activities, processing methods, and quality controls.2 As we know, TCMs are commonly used as the combinations of several CHMs, which contain several hundreds of components, whereby their synergistic effects contribute to their function in the clinical application. However, in earlier years, researchers often selected one or several chemical markers to access the quality of CHMs. For a long time, the poor discovery rate of the relative effective components and their elusive component-effect correlation have been the bottleneck in TCM research.3 In 2016, Liu et al.4 introduced the new concept of a quality marker (Q-marker) of CHMs. The meaning of the Q-marker is defined as the inherent chemical compound from the herb medicine or generated compounds during the processing preparation, whose biological activity is closely related to their safety and therapeutic effects. According to the guidance, in this study, an integrated approach to transform chemical markers into Q-markers is presented. Two often confused CHMs, Lonicera japonica flos (LJF) and Lonicera flos (LF), which are of the same genus but different species, were used as a model to illustrate the confirmation process of Q-marker selection and application.
In the Chinese Pharmacopoeia (2015 edition), LJF was documented as the unique origin of Lonicera japonica Thunb. Although some results show that LJF and LF are pharmacologically similar, they differ significantly in certain aspects.5 Traditionally, the chemical profiling and comparing of LJF and LF has been performed by UPLC-UV and UPLC-QQQ-MS.6,7
Recently, some studies utilizing Fourier transform infrared spectroscopy with two-dimensional correlation analysis (2D-IR) were conducted to rapidly identify LJF and LF.8 In the 2D-IR study, a tri-step identification approach was developed to discriminate the LJF and LF samples, whereby wave peaks related to the saponins in LF were found. Quantitative methods based on near-infrared spectroscopy (NIRS) have also been established,9 whereby the Soft Independent Modeling of Class Analogy (SIMCA) model was established to identify LJF from the genuine producing area, and six organic acids were used to develop the NIRS quantitative calibration models for quantity control. Regrettably, these methods did not provide the qualitative and quantitative analysis results associated with the traditional effects, and also the chemical markers behind the satisfactory discrimination were not provided. Therefore, how to find the distinguishing chemical markers and identify them as the Q-markers for the quality control of LJF would be meaningful.
The strategy for Q-marker screening and application is presented and charted in Fig. 1. In principle, chemical markers were first selected by ultra-performance liquid chromatography-quadrupole/time-of-flight (UPLC/Q-TOF) with partial least squares-discriminant analysis (PLS-DA). Then, bioactive-based HPLC was introduced to screen the Q-markers. In detail, to identify the anti-inflammatory markers to discriminate the LJF and LF, NF-κB, which is involved in the early immune response and synthesis of cytokines and chemokines, was selected as a bioactive index.10 The variable importance parameters (VIP) plot guided us to find the potential Q-markers for the quality classification. Compared with traditional HPLC analysis methods, NIRS can record the spectra for solid and liquid samples without the need for troublesome pretreatment and this enabled the development of portable equipment to quantify multiple components in the CHMs.11 Hence, accurate NIRS methods were selected to integrate the Q-marker information and were used for the LJF identification and fast quality assessment in this paper.
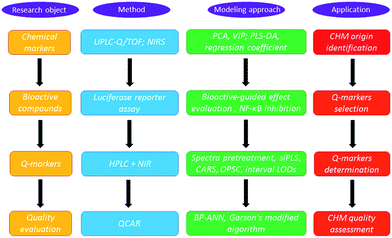 |
| Fig. 1 Flow chart of the strategy for Q-marker screening and application. | |
After systematic chemometrics optimization, a new algorithm to select the wavenumber points, named the optimum siPLS-CARS analysis (OPSC), was proposed based on the merit of synergy interval partial least squares (siPLS) and competitive adaptive reweighted sampling (CARS) to quantify those representative Q-markers.12,13 To clarify the quantitative composition-activity relationship (QCAR), a back propagating-artificial neutral network (BP-ANN) was used to build the complicated non-linear relationship between these Q-markers and their integral bioactivity.14–16 Then an integrated NIRS and Q-marker strategy was established for the fast assessment of LJF.
2. Experimental
2.1. Chemicals, reagents, and materials
Reference standards of 3-O-caffeoylquinic acid (CA), 3,5-O-dicaffeoylquinic acid (3,5-diCQA), and swertiamarin were purchased from the Chinese Institute for the Control of Pharmaceutical and Biological Products (Beijing, China). The purities of all the standards were greater than or equal to 98%. Acetonitrile, phosphoric acid, and formic acid of HPLC grade were purchased from Merck (Darmstadt, Germany). Ultrapure water was prepared with a Milli-Q purification system (Millipore, Bedford, MA, USA). All the other reagents were of analytical grade and purchased from Yifang S&T (Tianjin, China).
Five cultivars, including 98 samples of honeysuckle buds (LJF, including Lonicera japonica Thunb.; and LF, including Lonicera hypoglauca Miq., Lonicera fulvotomentosa Hsu er S. C. Cheng, Lonicera confusa DC., and Lonicera macranthoides Hand. Mazz), were collected from 11 different provinces in China. Detailed information on these samples is listed in Table S1.† Every species was authenticated by Professor Tiejun Zhang from the Tianjin Institute of Pharmaceutical Research.
2.2. Sample preparation
All the honeysuckle buds were finely pulverized and filtered through a 100-mesh sieve. The dried powders were directly used for the NIRS scans. For the sample extraction, each dried powder (1 g) was extracted with 100 mL of a methanol–water (25
:
75, v/v) solution using ultrasonic extraction apparatus (40 kHz, 500 W, Ningbo, China) for 30 min at room temperature. The same solution was used to replenish the extraction system upon solvent loss because of volatilization. The extracts were centrifuged at 12
000 rpm for 10 min, and the supernatant of 58 samples, including 29 LJF and 29 LF, was used for the UPLC/Q-TOF identification. The extraction method was identical to the above description, except the supernatant was used for the HPLC analysis and NF-kB inhibition assay. Primary stock solutions of three reference compounds (CA, 3,5-O-diCQA, and swertiamarin) at final concentrations of 598, 320, and 994 μg mL−1 were prepared by dissolving the accurately weighed reference compounds in a methanol–water (25
:
75, v/v) solution. Then, the stock solutions were serially diluted to the required concentrations to prepare the calibration curves. All the solutions were stored at 4 °C and brought to room temperature before use.
2.3. UPLC/Q-TOF-MS analysis
A Waters Acquity UPLC system (Waters Co., Milford, MA, USA) with a Photo-Diode Array detector (PDA) and a Waters Q-TOF Premier Mass Spectrometer with an electrospray ionization system (Water MS Technologies, Manchester, UK) were used for the sample analysis. Data acquisition was performed using the MassLynx V4.1 software (Waters Co., USA). Separations were performed using a Waters ACQUITY UPLC BEH C18 column (100 mm × 2.1 mm, 1.7 μm) at 25 °C. The mobile phase consisted of acetonitrile (A) and water with 1% formic acid (B) at a flow rate of 0.4 mL min−1. The gradient elution was completed as follows: 0–5 min, isocratic 2% (v/v) A; 5–9 min, 2–8% (v/v) A; 9–12 min, isocratic 8% (v/v) A; 12–15 min, 8–10% (v/v) A; 15–18 min, 10–17.5% (v/v) A; 18–22 min, 17.5–40% (v/v) A; 22–27 min, 40–100% (v/v) A; 27–28 min, isocratic 100% (v/v) A; 28–32 min, 100–40% (v/v) A; 32–35 min, 40–2% (v/v) A; 35–40 min, isocratic 2% (v/v) A. The sample injection volume was 5 μL. The ESI-MS spectra were acquired in both positive and negative ion modes. The conditions for the ESI-MS analysis were as follows: the capillary voltage was set to 3.0 kV and 2.5 kV for the positive and negative modes, respectively; the sample cone voltage was set to 30 V; the desolvation gas flow was set to 600 L h−1 at 350 °C; the cone gas was set to 50 L h−1; the source temperature was 110 °C. The Q-TOF Premier acquisition rate was 0.1 s with a 0.02 s inter-scan delay. The MS spectra were acquired from 100 to 1000 Da. Leucine enkephalinamide acetate was used as the lock mass (m/z 555.2931 in ESI+; m/z 553.2775 in the ESI−) at a concentration of 200 ng mL−1 and a flow rate of 0.2 μL min−1. The MS/MS analyses were used to obtain the mass fractions of the target ions.
2.4. NIRS collection
The spectra were collected in the diffuse reflectance mode using an integrating sphere module over the 12
000–4000 cm−1 spectral range and a Brucker TENSOR 37 FT-NIR spectrometer (Bruker Optik, Ettlingen, Germany) with an InGaAs detector. The spectra were collected with the OPUS spectral acquisition software (Bruker Optik, Ettlingen, Germany) at a resolution of 8 cm−1 per spectrum by averaging 64 scans.
2.5. Multivariate statistical analysis
2.5.1. UPLC/Q-TOF with PLS-DA analysis and NIRS with principal component analysis (PCA). To identify the distinguishing marker components from these honeysuckle buds, UPLC/Q-TOF coupled with PLS-DA was performed to screen the characteristic components and provide helpful chemical information to distinguish the two different cultivars: LJF and LF. The UPLC/Q-TOF data were imported into Markerlynx XS (V4.1 SCN884, copyright by 2012 Waters Inc.) for the PLS-DA analysis.OPUS spectral acquisition software (Bruker Optik, Ettlingen, Germany) was used for the NIR spectral data acquisition. In total, 98 samples (66 LJF samples and 32 LF samples) were used for the PCA analysis with Unscrambler software version 9.7 (CAMO Software, OSLO, Norway).
2.5.2. HotMap analysis. A semi-quantitative HotMap was used to display the clustering effect using the chemical markers from Section 2.5.1. The hierarchical cluster analysis of the quantitative HotMap was generated using Matlab 2013b in Windows 8.1. The process was conducted as follows: after the vector normalization of these marker components in the UPLC/Q-TOF data from 58 batches of honeysuckle bud samples, a hierarchical cluster tree of the peak areas was created by defining the linkage function using the Ward method, while the distance among the samples was computed using the Euclidean distance formula.
2.6. Dual-luciferase reporter assay system for the NF-κB inhibitor
The human embryonic kidney 293 (HEK 293) cell line was purchased from the American Type Culture Collection (Rockville, MD) and cultured in Dulbecco's modified Eagle's medium, which contained 10% (v/v) fetal bovine serum, for 24 h before the experiments. Then, the cells were co-transfected with the NF-κB luciferase reporter plasmid (Promega WI, USA) pGL4.32 at 100 ng per well and the Renilla luciferase reporter vector plasmid pGL-TK at 9.6 ng per well for 24 h. After the transfection, the cells were stimulated with 10 ng mL−1 TNF-α for 6 h under the protection of the herbal samples. Subsequently, the luciferase activity was assayed using a Luciferase Reporter Assay System (Promega, WI, USA). The luminescence was assessed with a Modulus luminometer from Turner Biosystems (Turner Design, CA, USA).
2.7. HPLC quantitative analysis
To analyze CA, 3,5-diCQA, and swertiamarin, HPLC analysis was performed using a Shimadzu 20A HPLC system (Shimadzu Co., Japan) with an auto sampler, column oven, and a UV detector. The chromatographic separations were conducted using an Agilent Eclipse Plus C18 column (100 mm × 4.6 mm, 3.5 μm) at 25 °C. The mobile phase system was acetonitrile (A) and water with 0.1% formic acid (B) at a flow rate of 1.0 mL min−1. The gradient elution was completed as follows: 0–10 min, 2–8% (v/v) A; 10–15 min, isocratic 8% (v/v) A; 15–20 min, 8–10% (v/v) A; 20–25 min, 10–15% (v/v) A; 25–35 min, 15–20% (v/v) A; 35–50 min, 20–100% (v/v) A; 50–55 min, isocratic 100% (v/v) A; 55–60 min, 100–2% (v/v) A; 60–67 min, isocratic 2% (v/v) A. The compounds were detected by UV at 240 nm. The sample injection volume was 10 μL.
2.8. OPSC analysis
To avoid sample selection bias, set partitioning based on the joint x–y distance (SPXY) algorithm17 was used to split the dataset into calibration and validation sets. Then, the 66 LJF samples were divided into 53 calibration samples and 13 test samples. The NIRS was pretreated to remove irrelevant information and noise before the model calibration. Preprocessing methods, such as auto-scaling (AUTO), standard normal variable transformation (SNV), de-trend (DT), DT + SNV, convolution smoothing, one-dimensional convolution (one-DC), two-dimensional convolution (two-DC), one-DC + SNV, and one-DC + DT were used to process the NIRS data. The siPLS method was used for variable selection in NIRS.12 All the possible PLS model combinations of one, two, or three intervals were used to calculate the root mean square error of cross validation (RMSECV) and the corresponding correlation coefficient R (RMSECV). The interval limit of determination (LOD) theory was used to calculate the detection limit of the siPLS method. Only the siPLS model with a suitable LOD was used to execute the next OPSC.18
After the siPLS method, some spectral variables that contained irrelevant information or noise remained. In this work, a modified CARS algorithm, namely OPSC, was used to select the key wavelengths that had large absolute regression coefficients in the siPLS model. In the OPSC, enforced wavelength reduction and adaptive reweighted sampling were used to retain informative variables. The wavelengths with higher absolute values of regression coefficients survived by mimicking the “survival of the fittest” principle. In this step, the runs of the exponentially decreasing function (EDF) were set as N = 50, which implies that to find an optimal variable subset, there are 50 runs to iteratively filter the variables with small absolute regression coefficients. In the ith run of the EDF, the number of remaining variables was calculated as follows:
where SIP is the total number of variables selected from the siPLS method, and
k is the constant parameter that controls the EDF curve, which can be computed as:
|
 | (2) |
The formula is related to the curvature of the EDF and positively correlated with the speed of the decreasing curve. From eqn (1) and (2), when i = 0, all the SIP variables are used for modeling; when i = N, only 2 variables remain. Finally, the wavelengths with higher absolute values of regression coefficients survive to calculate their R (RMSECV) with the Leave-One-Out (LOO) cross-validation method. The OPSC algorithm is different from the traditional CARS algorithm because the EDF is used to select the smallest variables whose R was above 0.9 instead of the entire variables with the highest R. With smaller variables, it is convenient to build a robust model and resolve the NIRS. All the algorithms were implemented in Matlab 2013b (MathWorks, Natick, MA, USA) under Windows 8.1.
2.9. BP-ANN for comprehensive evaluation
The feed-forward BP-ANN is a supervised ANN learning technique and can be realized using single-layer and multi-layer networks, which are particularly effective for modeling complex non-linear systems.14,19 In this paper, the weights and bias values in the BP-ANN topology were updated with the resilient back-propagation algorithm (Rprop) using Matlab 2013b. One hidden layer was introduced into the BP-ANN structure, and then the tan-sigmoid was selected as the activation function from the input layer to the hidden layer. The tan-sigmoid was selected because the dose-response relationship of the LJF anti-inflammation activity is similar to the tan-sigmoid function. A linear function was selected as the activation function from the hidden layer to the output layer. Overfitting to the training data was prevented by restricting the optimal number of nodes in the hidden layer, which was 1–10 in this experiment. A normalization procedure is necessary to train the BP-ANN. Therefore, the input and target variables were processed by mapping the minimum and maximum values from −1 to 1. Simultaneously, the 'early stopping by cross-validation' methodology was applied to prevent overfitting with the 13 test samples, which were selected as shown in Section 2.8.
3. Result and discussion
3.1. PLS-DA analysis and identification of chemical markers of honeysuckle buds
To find the distinguishing marker ingredients among the LJF and LF samples, the negative and positive ion mode data detected by UPLC/Q-TOF were simultaneously used for a global analysis. The BPI chromatograms from the honeysuckle bud samples are shown in Fig. 2A and B. The chromatograms of these samples had different peak numbers and peak intensities, which were observed through visual inspection. For further analysis, a common supervised multivariate statistical analysis PLS-DA was applied to examine the differences between the LJF and LF samples. In Fig. 2C and D, the samples were differentiated and categorized into two groups. To identify the representative chemical markers, a VIP plot was used to show the important signals that contributed to the clustering separation (Fig. 2E and F). Based on the VIP plots, variables with a VIP value > 5 in both the negative and positive modes were considered as the potential chemical markers.20
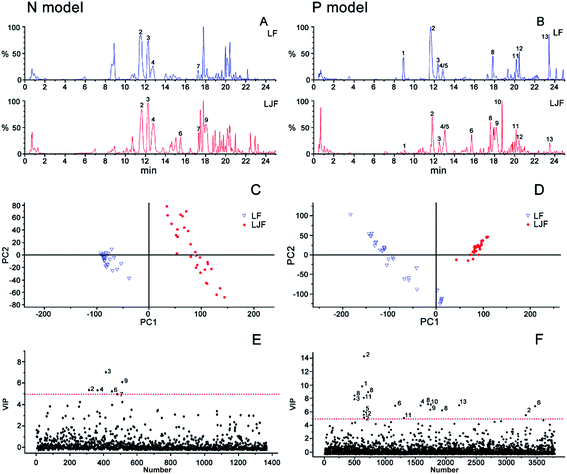 |
| Fig. 2 BPI chromatograms and PLS-DA analysis of the honeysuckle bud samples. BPI chromatograms of the honeysuckle bud samples in the negative (A) and positive modes (B). Score plot of the honeysuckle bud samples in the negative (C) and positive modes (D). Potential chemical markers in the VIP plot of the PLS-DA model among various honeysuckle bud species in the negative (E) and positive modes (F). | |
For example, the identification of CA was considered. The VIP value was 14.17 and 5.41 in the positive and negative modes, respectively. The [M + H]+ and [M − H]− ion nuclei ratios were 355.1016 and 353.0666, respectively, which resulted in the structural fragments 377 [M + Na]+, 372 [M + H + H2O]+, and 163 [caffeic acid + H − H2O]+. After searching for the presumed molecular formula using ChemSpider (http://www.chemspi-der.com), MassBank (http://www.massbank.jp/), and SMPD (http://www.smpdb.ca/), we speculated that the formula was C16H18O9, which matched the characteristics of the CA standard substance. According to the described identification method, ten other chemical markers among the thirteen distinguishing compounds were identified (Table 1): 5-O-caffeoylquinic acid, CA, loganin, swertiamarin, 4-O-caffeoylquinic acid, sweroside, 7-epi-vogeloside, secoxyloganin, vogeloside, L-phenylalaninosecologanin, 3,5-diCQA, and 3,4-O-dicaffeoylquinic acid. Interestingly, the chemical markers were consistent with published reports that honeysuckle buds mainly contain iridoid glycosides and phenolic acids.21
Table 1 MS/MS data from ESI-MS and identification of the PLS-DA results
No. |
VIP |
tR |
MS[M + H]+ |
MS/MS(m/z) |
MS[M − H]− |
MS/MS(m/z) |
Formula |
Compound |
1 |
9.85(+) |
8.98 |
355.1003 |
377, 163 |
353.0532 |
375, 191 |
C16H17O9 |
5-O-Caffeoylquinic acid |
2 |
14.17(+)/5.41(−) |
11.59 |
355.1016 |
377, 372, 355, 163 |
353.0666 |
|
C16H18O9 |
3-O-Caffeoylquinic acid |
3 |
7.99(+)/7.04(−) |
12.28 |
391.1222 |
408, 229, 211, 151 |
389.0927 |
|
C17H26O10 |
Loganin |
4 |
7.11(+)/5.40(−) |
12.84 |
375.1258 |
749, 213, 195 |
373.0914 |
747 |
C16H22O10 |
Swertiamarin |
5 |
6.28(+) |
12.88 |
355.1004 |
163 |
353.0565 |
|
C16H18O9 |
4-O-Caffeoylquinic acid |
6 |
7.05(+)/5.28(−) |
15.58 |
359.1292 |
717, 197 |
357.0896 |
403 |
C16H22O9 |
Sweroside |
7 |
5.05(−) |
17.35 |
— |
— |
419.1364 |
— |
— |
Unknown |
8 |
9.02(+) |
17.84 |
405.1364 |
243, 225, 211, 193, 165, 151 |
403.1129 |
807 |
C17H24O11 |
Secoxyloganin |
9 |
6.48(+)/6.13(−) |
18.14 |
389.1401 |
406, 227, 209, 195, 151 |
387.1046 |
433 |
C17H24O10 |
Vogeloside |
10 |
7.23(+) |
18.77 |
538.2260 |
— |
536.2078 |
— |
— |
L-Phenylalaninosecologanin |
11 |
8.18(+) |
20.20 |
517.1301 |
499, 163 |
515.1158 |
353 |
C25H24O12 |
3,5-O-Dicaffeoylquinic acid |
12 |
5.77(+) |
20.47 |
517.1302 |
499, 163 |
515.1176 |
353 |
C25H24O12 |
3,4-O-Dicaffeoylquinic acid |
13 |
7.13(+) |
23.46 |
274.2712 |
— |
— |
— |
— |
Unknown |
3.2. Semi-quantitative HotMap analysis for chemical markers
As shown in Fig. 2C and E relating to the negative model, the cluster pattern mainly resulted from iridoid compounds, especially compounds 3 (loganin) and 9 (vogeloside). Inversely, in the positive model (Fig. 2D and F), the clustering effect mainly came from the phenolic acid components, such as compound 1 (5-O-caffeoylquinic acid) and 2 (3-O-caffeoylquinic acid). To further display the difference between the LJF and LF samples, the semi-quantitative HotMap analysis was used to show the clustering effect using the normalized peak areas of the aforementioned 13 distinguishing chemical markers. As shown in Fig. 3, these different cultivar samples were obviously clustered into two categories based on the chemical markers. These marker components could also be divided into two categories by the Ward linkage with Euclidean distance. The result shows that LF is rich in phenolic acid components, but LJF is rich in iridoid glycosides, and this conclusion is consistent with the previous report.6 In detail, different types or geographical origin of the samples of LF can also be distinctive from each other.
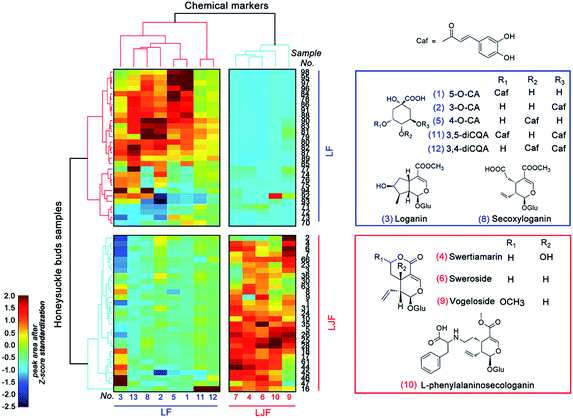 |
| Fig. 3 HotMap hierarchical clustering analysis and the chemical structures of the chemical markers in the LF (blue) and LJF (red) samples. | |
3.3. NIRS coupled with PCA for origin identification based on chemical markers
To induce a more convenient means instead of the complicated UPLC/Q-TOF cluster task, NIRS was applied for the differentiation of LJF and LF. First, the NIRS data of honeysuckle bud powders were pretreated with one-DC to remove irrelevant physical information and noise and to reveal the inherent chemical information22 (Fig. 4A). As for PLS-DA, the first two principal components enabled us to explain 87% of the total variance. Upon examination of the loading plot for the first component, we observed that the signals at wavenumbers such as 4382, 4436, 4914, 5142, 5261, 5354, 5851, 5932, 5998, 7070, 7244, 7356, 7394, and 8826 cm−1 dominated the first principal component (Fig. 4B). In Fig. 4C, PC1 accounted for 84% of the spectral variability, whereas PC2 did not show differences only for 3%.
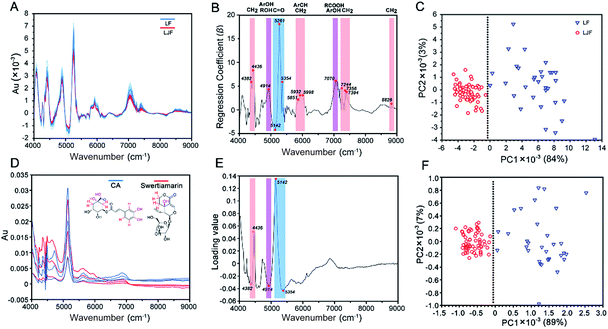 |
| Fig. 4 PLS-DA analysis by NIRS in the different honeysuckle bud samples: (A) NIRS after one-DC pretreatment; (B) regression coefficient of the PLS-DA result for the first principal component (the colors represent different functional groups); (C) score plot of the NIRS with the entire spectra; (D) NIRS of two representative chemical markers (CA and swertiamarin) in deuterated DMSO solutions; (E) loading plot of the PCA result of the two chemical markers; (F) score plot of the PCA cluster with the dominated wavenumber points. | |
To ascribe the wavenumber signals to specific functional groups in the chemical markers, CA and swertiamarin were, respectively, selected as the markers of phenolic acids and iridoid glycosides. The NIRS of these two chemical marker standards are shown in Fig. 4D, each of which had three concentration levels (1, 5, 10 mg mL−1) and were prepared with the deuterated DMSO solution. Compared with Fig. 4A, the similar prominent peaks at 4250–4300, 4500–5000, 5000–5500, 5500–6100 and 6600–7200 cm−1 can be observed by deducting the background from the deuterated DMSO. Among these wavenumber points (Fig. 4E), the five dominated wavenumbers with the highest loading values were at 4382 and 4436 cm−1 (a combination of CH stretching and CH bending) from different chemical environments of methylene on swertiamarin and CA; 4914 cm−1 (a combination of OH stretching and OH bending) from the phenolic hydroxyl in CA or different aliphatic hydroxyl chemical environments on swertiamarin and CA; 5142 and 5354 cm−1 (CO 2nd overtone region) from different carbonyl chemical environments on iridoid glycosides (lactonic ring) and phenolic acids (carboxyl). More importantly, these dominated points from two main chemical markers also appeared in the regression coefficient plot of the honeysuckle bud samples (Fig. 4B). In Fig. 4F, with these five dominated points, two clusters were also observed: one consisting of LJF samples and the other including LF samples. The spectral variability accounting by PC1 and PC2 was increased to 89% and 7%, respectively. The result illustrates that after the pretreatment, chemical information can be extracted from the complex herbal matrix, and NIRS-based identification can be achieved based on only the key spectrum characteristics from chemical markers.
3.4. Identification and quantification of NF-κB inhibitors in LJF samples
To screen and identify the anti-inflammatory Q-markers, one of the LJF extracts (no. 1) was separated by HPLC. The HPLC fractions were collected at 2 min intervals; each fraction was concentrated and tested for NF-κB inhibition activity using the luciferase reporter assay system. From the results in Fig. 5, four fractions (no. 2, 4, 9, 11) showed significant NF-κB inhibition. Compared with the reference substances or UPLC/Q-TOF-MS/MS information, peaks no. 2, 4, 11, and 9 were identified as CA, swertiamarin, 3,5-diCQA, and vogeloside, respectively. Studies have reported that CA can suppress the LPS-induced COX-2 expression by attenuating the activation of NF-κB-dependent pathways and JNK/AP-1 signaling pathways.23 3,5-diCQA inhibits the LPS-induced RAW 264.7 macrophage inflammation by suppressing the nitric oxide/inducible nitric oxide and prostaglandin E2/cyclooxygenase-2 pathways by inhibiting the nucleus translocation of p50 and p65.24
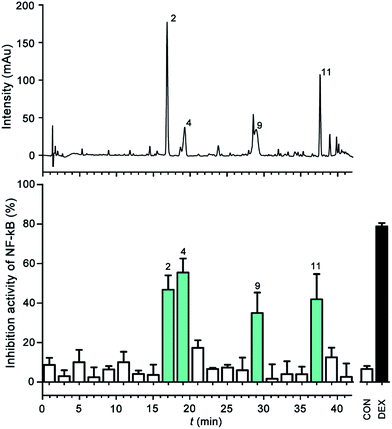 |
| Fig. 5 HPLC-UV coupled with the luciferase reporter assay system for the NF-κB inhibitor analysis. (A) UV chromatograms (240 nm); (B) bioactivity chromatogram obtained via the luciferase reporter assay system for NF-κB inhibition. The peak numbers are consistent with those in Table 1. | |
Swertiamarin treatment can decrease the release of proinflammatory cytokines (IL1, TNF, IL-6) and proangiogenic enzymes (MMPs, iNOS, PGE2, PPARγ, and COX-2) by modulating NF-κB and JAK2/STAT3 signaling.25 Vogeloside showed the inhibition of nitric oxide production in LPS-induced macrophages.26 Therefore, these four NF-κB inhibitors are responsible for the anti-inflammatory bioactivity and can be presented as the Q-markers for further tests.4,27
The accurate contents of the four Q-markers in 98 batches of the honeysuckle bud samples were detected using the HPLC method, and the details are listed in the ESI (Tables S1 and S2†).
3.5. Quantification of the Q-markers in the LJF samples with the partial least square regression (PLSR) algorithm
In this section, the joint x–y distance (SPXY) algorithm was used to split the dataset of 66 LJF samples into 53 calibration samples and 13 test samples. The LOO cross-validation method with 53 LJF calibration samples was used to calculate the RMSEC, RMSECV, Rcal, and Rval. The remaining 13 test samples were used to check the robustness of the NIRS model and calculate the RMSEP and Rpre.
3.5.1. Interval LODs with the siPLS algorithm. The inherent characteristics of multiple components and lower concentrations in the complex herbal matrices created enormous challenges in the quantitative analysis of the NIRS as this method itself has a higher detection limit and low sensitivity. In addition, a suitable variable selection algorithm could improve the model performance and detection sensitivity of the NIRS model. Thus, the siPLS algorithm was used as the variable selection method to identify the optimum subset combination of spectral frequencies, which produced the smallest RMSECV in the quantitative determinations and improved the sensitivity. Hence, the siPLS algorithm has been successfully applied to search and account for the optimal spectral frequencies in multiple herbal medicines.12,28 Table 2 lists the most suitable conditions with the siPLS algorithm (including the pretreatment methods, interval number, and LV) to quantify these Q-markers by LOO calibration with 53 LJF samples. Then, 13 LJF samples were used for the external validation to evaluate the robustness of the built NIRS model. The predictive effects of the built model are listed in Table 3. The LOD is one of the most significant values to determine which components are suitable to build the NIRS model. The interval LOD theory18 was introduced to calculate LODmin and LODmax. As shown in Fig. 6, although the LOD of HPLC was more sensitive than the NIRS method, the LODmax of NIRS for all four Q-markers was lower than their median values, which indicated that the four components were suitable to build the NIRS model for the LJF quality management (Table 4). In addition, due to the low abundance of swertiamarin and vogeloside in LF, iridoid glycosides are not suitable as Q-markers for quality control in LF.
Table 2 Most suitable conditions to calibrate and validate the Q-marker components
Compounds |
Pretreatment methods |
Interval number |
LV |
Rcal |
RMSEC |
Rval |
RMSECV |
Mean concentration (%) |
CA |
One-DC + SNV |
14 19 20 |
16 |
0.9976 |
0.0263 |
0.9000 |
0.1652 |
2.3716 |
3,5-diCQA |
One-DC + SNV |
15 18 19 |
9 |
0.9762 |
0.0313 |
0.8638 |
0.0733 |
0.8447 |
Swertiamarin |
One-DC + DT |
16 20 |
13 |
0.9863 |
0.0316 |
0.8612 |
0.0981 |
1.0987 |
Vogeloside |
None |
14 17 18 |
15 |
0.9894 |
0.1162 |
0.9019 |
0.3500 |
1.1320 |
Table 3 Predictive effect of the external validation set with the most suitable NIR model parameters
Compounds |
Rpre |
RMSEP |
RPD |
Mean concentration (%) |
CA |
0.9451 |
0.2823 |
2.9384 |
1.8895 |
3,5-diCQA |
0.8691 |
0.1264 |
2.0286 |
0.7748 |
Swertiamarin |
0.9321 |
0.1224 |
2.4933 |
1.0671 |
Vogeloside |
0.9373 |
0.6020 |
2.6025 |
1.7427 |
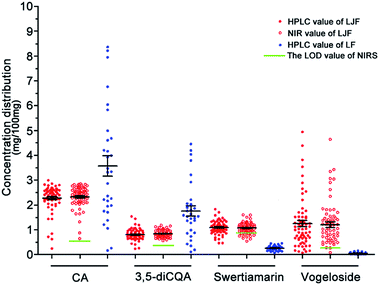 |
| Fig. 6 Concentration distribution analysis of the four Q-markers in LJF or LF. | |
Table 4 LOD values of the five main compounds in the LJFa
|
CA |
3,5-diCQA |
Swertiamarin |
Vogeloside |
LODref (%) is the LOD from the HPLC results. |
Mean con range (%) |
2.26(0.25 − 2.99) |
0.83(0.24 − 1.54) |
1.08(0.47 − 1.83) |
1.03(0.07 − 4.94) |
LV |
16 |
9 |
13 |
15 |
Var (x)1/2 |
0.0247 |
6.09 × 10−4 |
0.0316 |
5.66 × 10−5 |
Var (ycal) |
0.0110 |
0.0130 |
0.0240 |
0.0240 |
LODmin (%) |
0.5022 |
0.2372 |
0.3275 |
0.6904 |
LODmax (%) |
0.5719 |
0.2709 |
0.3845 |
0.8673 |
LODref (%) |
0.0082 |
0.0045 |
0.0053 |
— |
3.5.2. Wavenumber point quantification with the OPSC algorithm. A simple and efficient wavelength model for multi-index simultaneous determination is notably important for instrument miniaturization and decreased equipment costs. Some innovation wavelength selection methods have been established to search for the key quantitative wavelength points.29,30 In this study, the siPLS algorithm split the data set of a full spectrum into 20 intervals. The optimal combined interval numbers were elaborately selected according to the lowest RMSECV. However, these large wavelength variable inputs (including 310 variables for three intervals and 206 variables for two intervals) make the spectral resolution difficult. Therefore, the CARS algorithm was used to search for the key wavenumber points from the selected intervals by siPLS. Unlike siPLS on the principle of variable selection, the CARS algorithm identifies the wavelengths with large absolute regression coefficients in the PLSR model. However, the disadvantage of CARS is the serious overfitting when uninformative variables with large absolute regression coefficients are introduced (Table 5). When the CARS algorithm is combined with siPLS, siPLS remedies the disadvantages of CARS because siPLS first finds the optimal informative quantitative regions, under which CARS searches for its key wavenumber points. Comparing siPLS with OPSC in Table 5, we observed that a similar predictive effect (Rpre) was achieved by these two types of algorithms. However, OPSC requires even fewer points to fulfill the task of excellent prediction.
Table 5 Comparison of four different wavenumber selection strategies
Compounds |
Wavenumber selection method |
Pretreatment methods |
nVar |
LV |
Rval |
RMSECV |
Rpre |
RMSEP |
CA |
CARS |
One-DC + SNV |
58 |
11 |
0.9959 |
0.0347 |
0.8613 |
0.5033 |
siPLS |
One-DC + SNV |
310 |
16 |
0.9000 |
0.1652 |
0.9451 |
0.2823 |
OPSC |
One-DC + SNV |
10 |
8 |
0.9112 |
0.1553 |
0.9388 |
0.3848 |
3,5-diCQA |
CARS |
One-DC + SNV |
117 |
1 |
0.3008 |
0.1408 |
0.3184 |
0.2403 |
siPLS |
One-DC + SNV |
310 |
9 |
0.8638 |
0.0733 |
0.8691 |
0.1264 |
OPSC |
One-DC + SNV |
16 |
7 |
0.8758 |
0.0700 |
0.8997 |
0.1291 |
Swertiamarin |
CARS |
One-DC + DT |
205 |
3 |
0.4925 |
0.1740 |
0.4113 |
0.2788 |
siPLS |
One-DC + DT |
206 |
13 |
0.8612 |
0.0981 |
0.9321 |
0.1224 |
OPSC |
One-DC + DT |
18 |
10 |
0.9235 |
0.0737 |
0.9137 |
0.1652 |
Vogeloside |
CARS |
None |
58 |
17 |
0.9979 |
0.0526 |
0.7804 |
1.2296 |
siPLS |
None |
310 |
15 |
0.9019 |
0.3500 |
0.9373 |
0.6020 |
OPSC |
None |
13 |
12 |
0.9078 |
0.3376 |
0.9445 |
0.5529 |
As can be seen from the coefficient trend in Fig. 7, during CARS, the regression coefficient (R) increased as the wavelengths with more information were retained, whereas other unimportant ones were eliminated. However, when any key wavelength was removed, the R value sharply declined. Thus, the critical points with the least wavenumbers but R value above 0.9 were retained (the green line), which represents the most valuable and bare-bone spectral information, except for 3,5-diCQA, whose R value under all wavenumber combinations could not reach 0.9. Thus, the inflexion point after which the R value would sharply decrease was selected as the bare-bone spectral information for 3,5-diCQA. Finally, 10, 16, 18, and 13 wavelengths were selected for CA, 3,5-diCQA, swertiamarin, and vogeloside, respectively. In the wavenumber analysis, Fig. 7 illustrates the distribution of the selected variables by the OPSC algorithm. In this way, the fit effect of the four Q-markers is displayed in the fit effect part of Fig. 7.
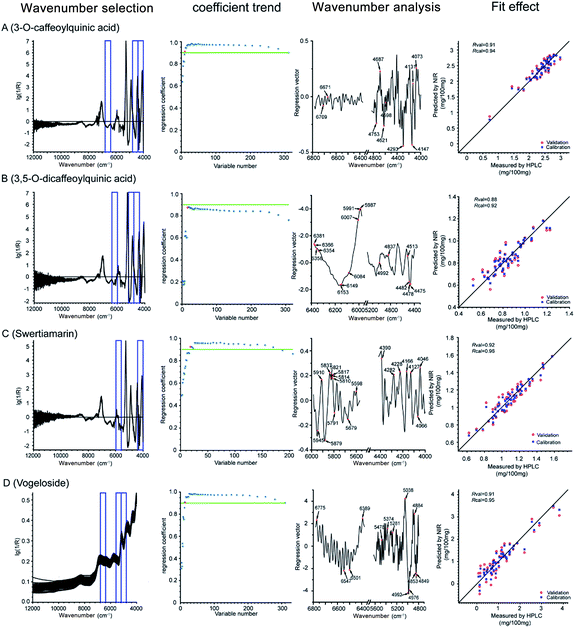 |
| Fig. 7 Wavenumber point quantification with the OPSC algorithm. Every Q-marker compounds has four parts: wavenumber selection by siPLS; regression coefficient trend under different variable numbers; selection of wavenumber points by the OPSC algorithm; correlation diagrams between the NIRS predicted values and the reference values. CA (A); 3,5-diCQA (B); swertiamarin (C); vogeloside (D). | |
3.6. BP-ANN for the bioactivity comprehensive evaluation of the LJF
Because we proved that BP-ANN with the resilient back-propagation algorithm displayed a better non-linear approximation effect than the random forest regression and nu-support vector regression at predicting the anti-inflammation activity in the previous study,31 the machine learning methods BP-ANN were performed to reveal the relationship between NIRS and the holistic anti-inflammation activity via the contents of the four Q-markers. For the bioactivity comprehensive evaluation, 66 batches of LJF were detected for the anti-inflammation activities assay. The ratio of NF-κB inhibition was used to calculate their correlation with the content of Q-markers. The values predicted by the OPSC algorithm were used to build the BP-ANN model. Finally, a three-layered configuration of 4-9-1 nodes was selected to build the relationship between the Q-markers and their holistic activity. As shown in Fig. 8A, the established BP-ANN model displayed an excellent fitting effect, and their corresponding correlation coefficient was 0.95 for the training data and 0.90 for the test data. Then, the contributions for the different Q-markers were determined by systematic analysis to partition the BP-ANN connection weights using Garson's modified algorithm.14 Their contributions to the anti-inflammation activity were ranked as follows: vogeloside (36.1%) > 3,5-diCQA (32.7%) > swertiamarin (18.7%) > CA (12.4%). The contributions of iridoid glycosides were more than the phenolic acid components (Fig. 8B). This result illustrates that the integrated Q-markers and the holistic bioactivity strategy are capable of and practical for the quality control of herb medicines and botanical functional foods.
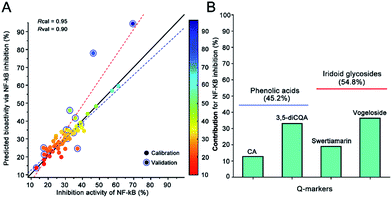 |
| Fig. 8 Predicted inhibition of NF-κB production versus the experimental values using BP-ANN (A); relative importance of each input in determining the anti-inflammation activity for the four Q-markers as estimated using Garson's modified algorithm (B). | |
4. Conclusions
In this paper, we described a quality assessment paradigm that involved a set of integrated strategies to improve the chemical markers to Q-markers in CHM quality management. Although the chemical markers could profile the distinguishing information for identifying LJF and LF, the satisfactory discrimination associated with the bioactive effects were not provided. Q-markers were demonstrated as the key effective and available ingredients and could be used to clarify the complex non-linear relationship between the components and their integral effect in CHMs. The NIRS method based on the key distinctive wavenumber points of Q-markers was proposed suitable for its fast determination. The established Q-marker-coupled NIRS pattern is a convenient and reliable way for quantitative analysis and holistic potency evaluation in herbal medicines or botanical products.
Acknowledgements
This work was supported by a Grant from the National Natural Science Foundation of China (No. 81430095; 81373506).
Notes and references
- K. Chan, X. Y. Hu, V. Razmovski-Naumovski and N. Robinson, European Journal of Integrative Medicine, 2015, 7, 67–75 CrossRef.
- X. M. Liang, Y. Jin, Y. P. Wang, G. W. Jin, Q. Fu and Y. S. Xiao, J. Chromatogr. A, 2009, 1216, 2033–2044 CrossRef CAS PubMed.
- S. P. Li, J. Zhao and B. Yang, J. Pharm. Biomed. Anal., 2011, 55, 802–809 CrossRef CAS PubMed.
- C. X. Liu, S. L. Chen, X. H. Xiao, T. J. Zhang, W. B. Hou and M. L. Liao, Chin. Herb. Med., 2016, 47, 1443–1457 Search PubMed.
- Y. J. Li, W. Y. Cai, X. G. Weng, Q. Li, Y. J. Wang, Y. Chen, W. Zhang, Q. Yang, Y. Guo, X. X. Zhu and H. N. Wang, J. Evidence-Based Complementary Altern. Med., 2015, 2015, 905063 Search PubMed.
- Z. L. Shi, Z. J. Liu, C. S. Liu, M. Q. Wu, H. B. Sun, X. Ma, Y. M. Zang, J. B. Wang, Y. L. Zhao and X. H. Xiao, Front. Pharmacol., 2016, 7 DOI:10.3389/fphar.2016.00012.
- X. Zhang, Q. Guo and B. Y. Yu, J. Sep. Sci., 2015, 38, 4014–4020 CrossRef CAS PubMed.
- R. Yan, J. B. Chen, S. Q. Sun and B. L. Guo, J. Mol. Struct., 2016, 1124, 110–116 CrossRef CAS.
- W. L. Li, Z. W. Cheng, Y. F. Wang and H. B. Qu, J. Pharm. Biomed. Anal., 2013, 72, 33–39 CrossRef CAS PubMed.
- M. Karin and F. R. Greten, Nat. Rev. Immunol., 2005, 5, 749–759 CrossRef CAS PubMed.
- J. S. Rooney, A. McDowell, C. J. Strachan and K. C. Gordon, Talanta, 2015, 138, 77–85 CrossRef CAS PubMed.
- G. Y. Ding, Y. Nie, Y. Y. Hou, Z. H. Liu, A. N. Liu, J. M. Peng, M. Jiang and G. Bai, J. Pharm. Biomed. Anal., 2015, 114, 462–470 CrossRef CAS PubMed.
- Q. Luo, Y. Yun, W. Fan, J. Huang, L. Zhang, B. Deng and H. Lu, RSC Adv., 2015, 5, 5046–5052 RSC.
- F. García-Camacho, L. López-Rosales, A. Sánchez-Mirón, E. H. Belarbi, Y. Chisti and E. Molina-Grima, Algal Res., 2016, 14, 58–64 CrossRef.
- Y. Q. Han, M. G. Zhou, L. Q. Wang, X. H. Ying, J. M. Peng, M. Jiang, G. Bai and G. A. Luo, J. Ethnopharmacol., 2015, 174, 387–395 CrossRef CAS PubMed.
- M. Jiang, Y. Q. Han, M. G. Zhou, H. Z. Zhao, X. Xiao, Y. Y. Hou, J. Gao, G. Bai and G. A. Luo, PLoS One, 2014, 9, e96214, DOI:10.1371/journal.pone.0096214.
- R. K. H. Galvao, M. C. U. Araujo, G. E. Jose, M. J. C. Pontes, E. C. Silva and T. C. B. Saldanha, Talanta, 2005, 67, 736–740 CrossRef CAS PubMed.
- F. Allegrini and A. C. Olivieri, Anal. Chem., 2014, 86, 7858–7866 CrossRef CAS PubMed.
- Y. Khan, Electr. Eng., 2015, 98, 29–42 CrossRef.
- Y. Li, L. Zhang, H. Wu, X. Wu, L. Ju and Y. Zhang, Anal. Methods, 2014, 6, 2247–2259 RSC.
- L. W. Qi, C. Y. Chen and P. Li, Rapid Commun. Mass Spectrom., 2009, 23, 3227–3242 CrossRef CAS PubMed.
- A. Rinnan, Anal. Methods, 2014, 6, 7124–7129 RSC.
- J. Shan, F. Jin, Z. Zhao, X. Kong, H. Huang, L. Lan and Z. Yin, Int. Immunopharmacol., 2009, 9, 1042–1048 CrossRef CAS PubMed.
- S. Puangpraphant, M. A. Berhow, K. Vermillion, G. Potts and E. G. d. Mejia, Mol. Nutr. Food Res., 2011, 55, 1509–1522 CAS.
- S. Saravanan, V. I. Hairul Islam, N. Prakash Babu, P. Pandikumar, K. Thirugnanasambantham, M. Chellappandian, C. Simon Durai Raj, M. Gabriel Paulraj and S. Ignacimuthu, Eur. J. Pharm. Sci., 2014, 56, 70–86 CrossRef CAS PubMed.
- T. L. Meragelman, B. S. Renteria, G. L. Silva, C. Sotomayor and R. R. Gil, Phytochemistry, 2006, 67, 1534–1538 CrossRef CAS PubMed.
- T. J. Zhang, J. Xu, Y. Q. Han, H. B. Zhang, S. X. Gong and C. X. Liu, Chin. Herb. Med., 2016, 47, 1458–1467 Search PubMed.
- Z. S. Wu, Y. F. Peng, W. Chen, B. Xu, Q. Ma, X. Y. Shi and Y. J. Qiao, Bioresour. Technol., 2013, 137, 394–399 CrossRef CAS PubMed.
- B. C. Deng, Y. H. Yun, P. Ma, C. C. Lin, D. B. Ren and Y. Z. Liang, Analyst, 2015, 140, 1876–1885 RSC.
- Y. H. Yun, W. T. Wang, M. L. Tan, Y. Z. Liang, H. D. Li, D. S. Cao, H. M. Lu and Q. S. Xu, Anal. Chim. Acta, 2014, 807, 36–43 CrossRef CAS PubMed.
- G. Y. Ding, B. Q. Li, Y. Q. Han, A. N. Liu, J. R. Zhang, J. M. Peng, M. Jiang, Y. Y. Hou and G. Bai, J. Pharm. Biomed. Anal., 2016, 131, 391–399 CrossRef CAS PubMed.
Footnote |
† Electronic supplementary information (ESI) available. See DOI: 10.1039/c6ra28152d |
|
This journal is © The Royal Society of Chemistry 2017 |