DOI:
10.1039/C6RA08517B
(Paper)
RSC Adv., 2016,
6, 65725-65735
Structure guided design and binding analysis of EGFR inhibiting analogues of erlotinib and AEE788 using ensemble docking, molecular dynamics and MM-GBSA†
Received
2nd April 2016
, Accepted 4th July 2016
First published on 5th July 2016
Abstract
Epidermal growth factor receptor (EGFR) is an important validated drug target for cancer therapy. Some therapeutic agents targeting EGFR have been developed, however these are sometimes ineffective, which calls for the discovery of alternative novel anti-EGFR inhibitors. Thus, we performed ensemble molecular docking and molecular dynamics (MD) against multiple conformations of EGFR to identify, design and validate new analogs of erlotinib and AEE788 that have enhanced binding affinity. To achieve this, curcumin analogues with diverse groups were studied against EGFR using ensemble molecular docking and MD. Based on the preferred orientations and MM-GBSA calculations of the selected top-scoring consensus lead inhibitors, we derived the pharmacophoric features that possess maximum binding affinity for the EGFR binding site. Using these pharmacophoric properties we designed new analogues of two known co-crystallized therapeutic inhibitors of EGFR (erlotinib and AEE788). We then tested the hypothesis using MD that the identified pharmacophoric property increases binding affinity and increases the stability of the designed analogues. Our MD simulations and MM-GBSA calculations confirmed that the occupancy of sub-pocket 2 in the EGFR active site is important for tight EGFR–inhibitor binding. The study thus provides an essential pharmacophoric requirement for design and discovery of new lead candidates as EGFR inhibitors.
Introduction
Epidermal growth factor receptor (EGFR) is one of the well-established drug targets of clinical importance.1 Over the past two decades the association between the EGFR and various types of cancer has been well established. It has been documented extensively that EGFR is over expressed or deregulated in a wide variety of cancers.2,3 In normal cells, EGFR catalyzes the transfer of phosphate from ATP to the tyrosine residue within the catalytic kinase domain of EGFR initiating the signaling cascade that regulates important cellular processes such as cell cycle progression, cell motility, invasion and metastasis.4 However, any functional deregulation in EGFR kinase activity due to either over-expression or mutation results in the disruptive signal transduction process, thereby giving rise to the cancerous state.5 Hence, a large number of tyrosine kinase inhibitors (TKIs) have been developed in recent years and a few of them such as erlotinib and gefitinib are currently available as therapeutics for treating cancer.6
As, inhibition of EGFR has demonstrated to have therapeutic potential, various structure and ligand-based approaches are being widely exploited for identifying new EGFR inhibitor molecules.7–10 In general, designing inhibitors computationally can be useful in accelerating EGFR targeted drug design. Clinically it is well established that protein kinase inhibitors play a major role in the treatment of cancer.6 Recent studies also highlight that the oncology industry has invested considerably on EGFR as a drug target.1 Simultaneously, it is also known that a large majority of drugs that are used in cancer treatment are derived from natural products.11–14 However, in last two decades, due to the reduction in the number of new chemical entities (NCEs) entering the market,15 the interest of pharma companies has shifted back to natural products for their quest of NCEs as combinatorial and high throughput technologies have failed to deliver.16
As the naturally occurring scaffolds are considered superior due to their druggable nature, optimal interaction with biological macromolecule and reduced toxicity issues, we have chosen curcumin analogs for computational screening against EGFR.16 The choice of curcumin is based on the fact that it has great potential to develop as an EGFR antagonist due to its ability to modulate the activity of EGFR as well as it provides ample opportunity for carrying out structural modifications at various positions.8 Therefore, we have used a large and diverse chemical library of curcumin analogs to undertake virtual screening and extensive MD simulation analysis against multiple EGFR conformations to identify the pharmacophoric features that can increase the potency of the inhibitors. Based on the above screening and MD, we designed novel analogues of erlotinib and AEE788, the co-crystallized inhibitors. These newly designed molecules were then re-examined in the binding site of EGFR for determining its binding affinity and stability, using MD simulations. Our MD simulations do indicate that the proposed new analogs fit better than the co-crystallized inhibitor in the cavity, keep the original interactions intact and have an enhanced binding affinity. The present study thus provides novel insight into the ligand-binding process and paves the way for designing next generation EGFR inhibitors.
Methods
Selection and preparation of the protein structures
A number of EGFR crystal structures are reported in the PDB (http://www.rcsb.org/pdb/home/home.do) which capture the different snapshots of the protein conformation and are co-crystallized with diverse inhibitors. Thus, in this study, in order to take into account protein flexibility so as to reduce the chances of identifying false positive leads and increase the likelihood of discovering an active compound during virtual screening, we have used three crystal structures for screening the library of small chemical molecules.8 The three proteins used are 2J6M,17 1M17
18 and 2ITW17 which have been crystallized with AEE788, AQ4 (erlotinib) and AFN941, respectively. To carry out docking studies on the selected crystal structures, the water molecules were removed and hydrogen atoms were added onto the protein structure. The active site of the protein structure was taken as amino acid residues that interact with co-crystallized ligand in the X-ray crystallographic structure. It was observed that the different EGFR crystal structures shared most of the binding site residues, however there were few differences which thereby helps in improving ensemble docking performance. Also, it needs to be noted that we have used residue numbers corresponding to Pdb id: 2J6M throughout the manuscript for consistency. In addition, a table which describes the corresponding residue numbering for the three structures (2J6M, 1M17 and 2ITW) used in this study has been provided (ESI Table S1†).
Preparation of library of curcumin analogues
A systematic literature search was performed to collect a total of 685 curcumin analogues from published research article.19 The 3D structures of molecules were then drawn using ChemDraw Ultra 10.0 followed by their optimization in Discovery Studio client v2.5.0 using default parameters.
Molecular docking using GOLD
Herein, we used GOLD 4.1.2 software (Genetic Optimization for Ligand Docking from Cambridge Crystallographic Data Centre, UK)20 for molecular docking studies of molecules in the 3 selected EGFR protein structures. The GOLD uses genetic algorithm to explore the full range of ligand conformational flexibility. Binding site was defined by providing the list of atoms corresponding to the residues that interact with the co-crystallized ligand in the X-ray crystallographic structure. Standard default settings, consisting of population size-100, number of islands-5, selection pressure-1.1, niche size-2, migrate-2, cross over-95, number of operations-100
000 were adopted for docking of each molecule to the protein.21,22 Also, 100 docking conformations (poses) were generated and the best docked conformation was selected based on the highest Goldscore, for further analysis.
Lead identification using a consensus approach
Thereafter the top scoring 25 ligands for each crystal structure were selected. To identify the potential lead molecules that may inhibit EGFR, we used consensus approach i.e. molecules that are common in the top 25 of all the three dockings were selected. In order to check the stability of the docked ligand in the complex, these selected leads were then taken for MD simulations.
Molecular dynamics simulation
The MD simulations of ligand bound EGFR complexes were performed to calculate binding free energy using MM/GBSA and to study the contribution of each residue in total binding interaction energies, using the AMBER 12 software.23 EGFR–ligand complex structures obtained from molecular docking were used as starting structure for MD simulation studies. We used general amber force field (GAFF) to get the input parameters for ligand and the ff99SB force field was used for protein. Initially, all hydrogen's were added using the Leap program from the AMBER12 package. Each EGFR–ligand complex system was inserted in a rectangular TIP3P water box in which protein atom was at least 8 Å away from the nearest edge of the box. The system was neutralized using counter ions. All simulations were run with SHAKE on hydrogen atoms with a 2 fs time step and langevin dynamics for temperature control. Then, the system was energy minimized as follows, the protein–ligand complex was frozen and solvent molecules with counter ions were allowed to move during a total of 2000 steps of minimization with the 1000 being steepest descent and cut-off of 8 Å to treat the nonbonding interactions. After relaxation, the system was heated up to 300 K for 50 ps and 50 ps of density equilibration with weak restraints (restraint_wt = 2.0) on the complex followed by 500 ps of constant pressure equilibration under NVT conditions. The particle-mesh Ewald (PME) methods for treating electrostatic interaction were used. After heating and density calibration the system was completely equilibrated. Once the EGFR–ligand complex equilibration was achieved, the production MD simulations of 5 ns were performed for all PDB–ligand complexes using NPT ensemble, that is, normal (300 K) temperature and constant pressure of 1 atm, under periodic boundary conditions without any positional constraints. All MD trajectories were analyzed by the ptraj module of the AMBER 12 package and VMD1.9.24 The binding free energy calculation (ΔG) was done on the snapshots extracted throughout 5 ns MD trajectories using MM-GBSA method, as well as per residue decomposition energy was calculated.
Results and discussion
Virtual screening of curcumin analogues using ensemble docking approach
The library of curcumin based analogues was docked against the three protein structures of EGFR (PDB ID: 2J6M, 1M17, 2ITW) using the GOLD docking program in order to identify the probable lead molecules. The consensus of docking results in three structures was used for selecting potential EGFR binders, considering the fact that the use of multiple conformations increases the chances of identifying the true active leads. The top 25 ligands which showed best docking pose, on the basis of Goldscore, were analyzed for each protein structure. The common ligands in all three docking screens identified are 470, 377, 462, 272, and 209 (Table 1 and Fig. 1). The identified five consensus ligands were then critically observed for their interactions with protein residues (Fig. 2).
Table 1 Best ranked 5 curcumin analogues identified by ensemble docking approach. The table shows their docking score (Goldscore) and the corresponding rank in the three EGFR structures. The docking score for the co-crystallized ligands is also indicated
Molecule ID |
PDB ID (co-crystallized ligand, docking score) |
1M17 (AEE-788, 57.56) |
2J6M (AQN, 71.13) |
2ITW (STU, 36.92) |
Goldscore |
Rank |
Goldscore |
Rank |
Goldscore |
Rank |
470 |
81.66 |
1 |
66.09 |
2 |
60.46 |
1 |
377 |
74.43 |
3 |
75.06 |
1 |
54.74 |
13 |
272 |
67.73 |
17 |
56.73 |
18 |
55.66 |
7 |
209 |
67.65 |
18 |
54.69 |
23 |
53.88 |
21 |
462 |
67.14 |
23 |
63.19 |
3 |
53.39 |
22 |
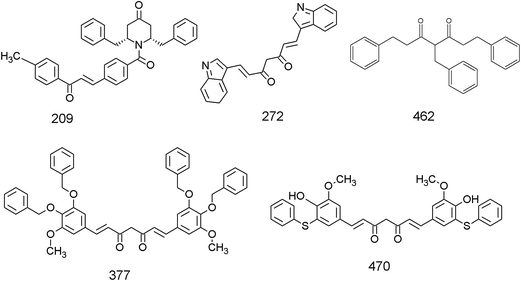 |
| Fig. 1 Chemical structures of the five top-scoring curcumin analogues identified as lead based on the docking against the three EGFR structures. | |
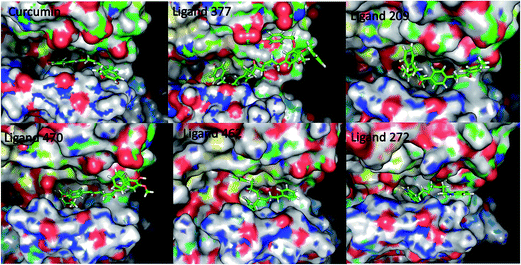 |
| Fig. 2 Ligand binding pose for curcumin analogues in 2J6M EGFR protein structure. | |
Molecular interaction analysis of the five top-scoring curcumin analogues
The molecular docking analysis in 1M17 showed that the Lys745, Met793, and Asp855 form H-bonding interactions with most of ligands, as shown in Table 2. It is clear from Table 2 and interaction data of co-crystallized inhibitors with the three EGFR crystal protein structures that for stable binding of a molecule in active site it is important to have H-bonding interactions with Met793 and Thr790. It is observed that Met 793, Lys745 and Asp855 show H-bonds in three (272, 377 and 470), four (209, 377, 462, and 470) and five (209, 272, 377, 462 and 470) ligands, respectively. Additionally, most of the hydrophobic interactions are also conserved in comparison to the EGFR-TK–erlotinib complex with major contribution coming from Val726, Leu718, Glu762, Leu792, Pro794, Phe723, and Phe795 that are guarding the entry of larger ligands.
Table 2 Molecular interaction analysis of hydrogen and hydrophobic bonding of ligands 209, 272, 377, 462 and 470 with residues of the three protein structures of EGFR
Molecular docking interaction analysis |
Protein PDB ID |
H-bond interactions (AA residues) |
Hydrophobic interactions (AA residues) |
1M17 |
2ITW |
2J6M |
1M17 |
2ITW |
2J6M |
AQN |
STU |
AEE |
AQN |
STU |
AEE |
Co-crystallized ligand |
MET793, THR790 (water mediated) |
GLY719, MET793 |
MET793, water mediated (THR854) |
LEU718, ALA743, LEU788, LEU792, PRO794, PHE795, GLY796, LEU844 |
LEU718, SER720, PHE723, VAL726, ALA743, LYS745, LEU792, PRO794, LEU844 |
ALA743, GLN791, PRO794, GLY796, GLU804, LEU844, ASP855 |
Ligand 209 |
LYS745, ASP855 |
LYS745 |
— |
VAL726, PRO794, ARG841 |
VAL726, CYS775, THR790, PRO794, LEU792, GLY796, ARG841, ASN842 |
ALA743, GLY796, LYS745, LEU788, ARG841, LEU844 |
Ligand 272 |
MET793, ASP855 |
MET793 |
GLU762 |
LEU792, PRO794 |
MET766, PRO794, |
LEU788, PRO794 |
Ligand 377 |
LYS745, MET793, ASP855 |
THR790 |
THR854 |
PHE723, VAL726, LEU792, PRO794, PHE795, GLU804 |
SER720, ALA722, ALA743, PRO794 |
LEU788, CYS775, GLN791, PRO794, PHE795, GLU804 |
Ligand 462 |
LYS745, ASP855 |
— |
THR854 |
VAL726 |
GLY796 |
PRO794, ARG841, ASN842 |
Ligand 470 |
LYS745, MET793, THR854, ASP855 |
GLU762, MET793 |
MET793, ASP855 |
LEU718, LEU792, PHE795 |
ALA743, MET766, LEU792, PRO794, PHE795 |
LEU788, LEU792, PRO794, GLU804 |
The structure of 2ITW, available as co-crystallized with the ligand staurosporine (STU) has multiple ring structures and it is mostly stabilized by the hydrophobic interactions contributed by Ala743, Leu718, Leu792, Leu844, Lys745, Phe723, Ser720, Pro794, and Val726. Two H-bonds are present between the residues, Met793 and Gly719, and ligand staurosporine. We observed that the ligand 272 forms H-bond with Met793, ligand 377 with Thr790, ligand 470 with Met793 and Lys762, and ligand 209 with Lys745 as a result of which it exhibits a high binding affinity of docking scores as shown in Table 2. It is observed that the hydrophobic interacting residues are also well conserved for curcumin analogues.
The 2J6M structure is co-crystallized with ligand AEE. It forms water mediated H-bonds with residues Thr854, while the amino acid residue Met793 is involved in direct H-bonding interactions with ligand AEE. It was observed from docking results that residue Glu762 (in ligand 272), Thr854 (in ligand 377 and 462) and Met793 and Asp855 (in ligand 470) are involved in strong H-bond formation with curcumin analogues. Apart from H-bonding interactions, several hydrophobic interactions were also observed for 2J6M-ligand binding. These residues are Gly796, Lys745, Leu788, Ala743, Gln791, Pro794, Arg841, Leu788, and Leu792 as shown in Table 2. Based on the preliminary molecular docking analysis and analysis of interacting amino acid residues, these 5 selected ligands were subjected to 5 ns MD simulation studies in multiple conformations (PDB ID: 2J6M and 1M17) in order to assess their stability in the binding pocket.
Molecular dynamics simulation analysis of the five selected leads in 1M17 and 2J6M
The MD simulation studies were performed for five ligands (209, 272, 377, 462, and 470) in two EGFR structures viz. 2J6M and 1M17. We selected the protein–ligand complexes with two crystal structures for MD simulation studies in order to study the stable binding orientation and derive affirmative conclusions. The binding free energy for all ligands in the protein structures was calculated using the MMGBSA method (Table 3). The binding energy in both the receptor–ligand complexes for each of the ligands was consistent and in the order 377 > 470 > 272 > 209 > 462 (Table 3). These results do indicate that the five ligands fit well in the active site of the protein and the EGFR cavity exhibits preference for 377 as compared to others. The binding energy for the ligand 377 having highest binding potential was found to be −51.01 kcal mol−1 and −50.52 kcal mol−1 in the protein 2J6M and 1M17 respectively.
Table 3 Shows MMGBSA binding free energy and hydrogen bonding residues along with occupancy for the five lead compounds in 2J6M and 1M17 protein
MD analysis |
Protein |
2J6M |
1M17 |
MMGBSA binding free energy (kcal mol−1) |
H-bond interactions |
MMGBSA binding free energy (kcal mol−1) |
H-bond interactions |
Residue |
Occupancy |
Residue |
Occupancy |
Ligand 377 |
−51.0 ± 3.4 |
CYS797 |
24.20% |
−50.5 ± 5.9 |
HIS805 |
4.80% |
LYS728 |
4.60% |
Ligand 470 |
−36.9 ± 1.5 |
MET793 |
1.80% |
−40.8 ± 3.6 |
MET793 |
44.40% |
LYS745 |
2.40% |
THR790 |
0.40% |
THR790 |
0.20% |
LYS728 |
0.40% |
Ligand 272 |
−33.2 ± 4.4 |
MET793 |
10.40% |
−38.9 ± 2.0 |
MET793 |
6.00% |
ASP855 |
10.20% |
ARG841 |
0.20% |
LYS728 |
0.20% |
Ligand 209 |
−29.2 ± 2.5 |
LYS745 |
0.20% |
−31.1 ± 2.4 |
THR854 |
6.80% |
CYS797 |
0.20% |
LYS745 |
7.00% |
THR790 |
0.20% |
Ligand 462 |
−27.6 ± 3.8 |
THR854 |
2.20% |
−29.9 ± 1.2 |
— |
— |
AQ4 |
— |
— |
— |
−42.1 ± 1.3 |
CYS797 |
7.80% |
MET793 |
10.20% |
THR790 |
0.20% |
AQ4_AN |
— |
— |
— |
−55.3 ± 3.7 |
CYS797 |
6.00% |
MET793 |
11.80% |
THR790 |
0.20% |
AEE |
−50.8 ± 3.2 |
MET793 |
33.40% |
— |
— |
— |
AEE_AN |
−57.1 ± 2.7 |
MET793 |
45.20% |
— |
— |
— |
In search of crucial amino acid residues responsible for optimum binding of ligand 377 to EGFR protein, the residue-wise energy decomposition analysis was performed as shown in Fig. 3. It is observed from residue-wise energy distribution graph that the amino acid residues Val726, Lys728, Phe795, Gly796, Cys797, and Leu844 are the major contributor towards the binding free energy of ligand 377 in 1M17 structure (Fig. 3). The same residues are the major interacting residues for co-crystallized ligand AQ4 in 1M17 structure as shown in Fig. 3. Particularly, the residues Lys728 and His805 have H-bond interactions with ligand 377 and found to have higher H-bond occupancy therefore considered to be important for tight EGFR–ligand interactions. Similarly, the H-bond analysis was done for the ligand 377 in protein 2J6M. It was observed that all H-bond forming residues are conserved in 2J6M structure. Here, the residue Cys797 is the major contributor for H-bonding interactions (Table 3). It is also observed that the amino acid residues Leu718, Val726, Lys728, Ala743, Glu762, Leu788, Thr790, Leu792, Phe795, Gly796, Cys797, Tyr801, Leu844, and Thr854 are the major contributor towards the binding free energy of ligand 377 in 2J6M structure (Fig. 3). The mentioned residues that have interactions with ligand are common in both protein structures. We observed that the residues which have higher contribution towards the binding of co-crystallized ligands AQN and AEE in respective protein structures are well conserved for curcumin analogue 377.
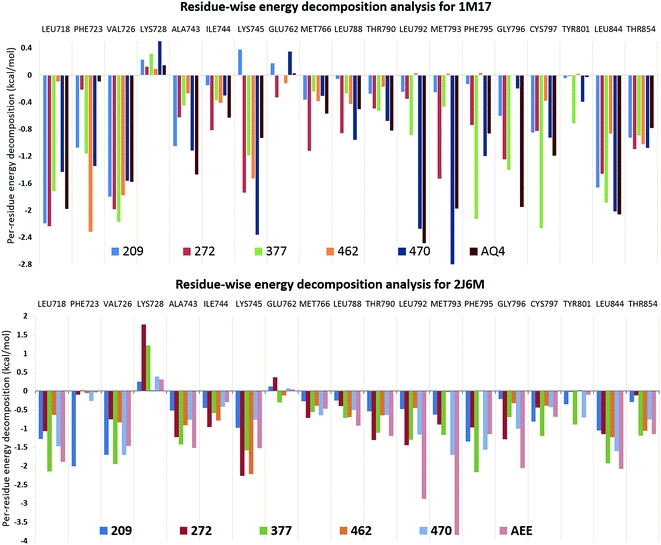 |
| Fig. 3 Residue-wise energy decomposition analysis for curcumin analogues in EGFR structures, 1M17 and 2J6M. | |
The observations suggested that ligand 377 has higher binding affinity (−51.01 kcal mol−1 and −50.52 kcal mol−1) towards EGFR structures, 2J6M and 1M17, than their native ligands, −50.83 kcal mol−1 for AEE and −42.15 kcal mol−1 for AQN, respectively (Table 3). As well as, we observed that the interactions with important residues were conserved in ligand 377, similar to co-crystallized ligands (AEE and AQ4). It gives an excellent opportunity to observe the atomic level interactions in detail and to study the orientation of various groups present in curcumin analogues and co-crystallized ligands.
Pharmacophore analysis
Based on the information about the interacting amino acid residues in EGFR protein and the shape of the ligand binding pocket, the pharmacophoric features required for tight binding of ligands were analyzed using Discovery Studio 2.5. Analysis of curcumin analogues 377 and 470 against the 2 PDB's revealed several consensus residues that exhibit hydrophilic or hydrophobic interactions and their spatial requirements. The consensus H-bonding residues are Lys745, Met793, Cys797, Thr854, and Asp855. Additionally, Ala743, Lys745, Glu762, Met766, Leu788, Thr790, Gln791, Leu792, Met793, Pro794, Gly796, Asp800, Tyr801, Arg841, and Asp855 are observed to form hydrophobic interactions with ligands in the EGFR binding pocket that were also observed in the MD simulation analysis. The amino acid residues Phe723, Phe795, and Tyr801 are found to be involved in pi-stacking interactions with ligands (Fig. 4). It was observed that the ligands show three H-bond acceptor features and four hydrophobic features (Fig. 4). On the other hand, we observed that complementary features are present in the EGFR binding pocket, which has H-bond donor features (residues Cys797 and Thr854) while the amino acid residues, Leu718, Phe723, Val726, Ala743, Ile744, Lys745, Met766, Leu777, Thr790, Leu792, Met793, Pro794, Phe795, Gly796, Tyr801, and Leu844 are satisfying hydrophobic interactions with ligands as shown in Fig. 4. We further observed that the residue Cys797 acts as an H-bond donor for any of the dione oxygen's present in the ligand backbone, which behaves as H-bond acceptor. Another H-bond donor Thr854, stabilizing H-bond acceptor oxygen of hydroxyl or methoxy or benzoxy group is present within these compounds (Fig. 4). As these compounds are symmetrical, the binding pocket (which prefers Y-shaped molecules) is observed to be occupied from one side of the ligand leaving the other side to protrude out of the cavity (Fig. 4). Also, we find that Phe795 and Tyr801 form pi-stacking interactions with one of the benzoxy substituent which resulted in another benzoxy substituent to protrude out of cavity without any interactions. From these observations, it can be inferred that instead of two, only one phenyl substituent is necessary for receptor binding.
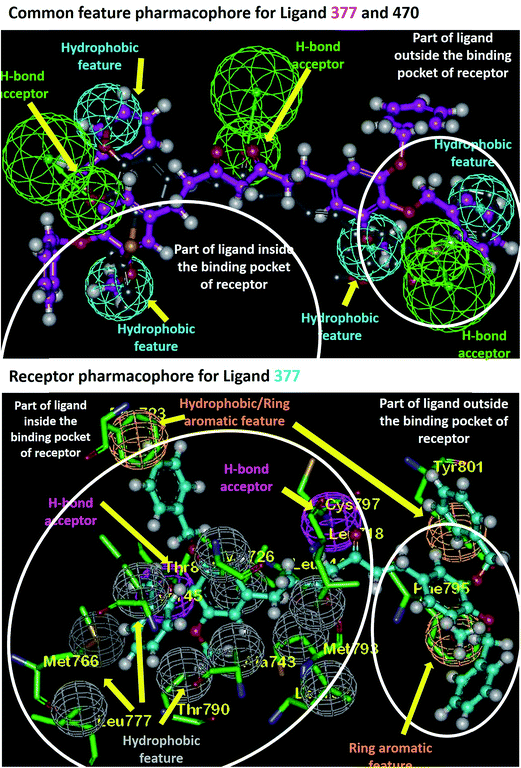 |
| Fig. 4 Pharmacophoric feature analysis of ligand 377 in EGFR binding pocket. | |
Rational for new molecule design
The crystal structure of EGFR bound to co-crystallized ligands AEE and AQ4 (PDB Id: 2J6M and 1M17 respectively), was analyzed to identify the crucial interactions in the active site and gain insight for further design of new ligands. It was observed that the co-crystallized ligands successfully occupy the pocket 1 and 3 (Fig. 5). The H-bonding interactions were observed between the polar nitrogen of ligand and residue Met793. The hydrophobic interactions between phenyl ring of ligand and residues Tyr727, Ala743, Ile744, Leu788, and Ile789 holds the ligand in pocket 1 (Fig. 5). Whereas, remaining part of the ligand is present in pocket 3. It was also observed that pocket 2 is still unoccupied because no functional group is present in ligand which can occupy the space in the pocket. We found that pocket 2 comprises of hydrophobic residues Leu718, Val 726 and aromatic residue Phe723. Therefore, based on the docking and molecular dynamic simulation results of the curcumin analogs, we identified that the addition of a large hydrophobic group on the pyrimidine ring will favor the hydrophobic interactions with residues present in pocket 2 (Fig. 5). It is expected that the proposed modification will lead to occupancy of all 3 pockets in EGFR active site and hence tighter binding of ligands which is necessary for a good EGFR inhibitor. Similar observations are for the ligand AQ4 where the addition of large aromatic hydrophobic group on quinazoline favors the tight binding of ligand in EGFR pocket 2.
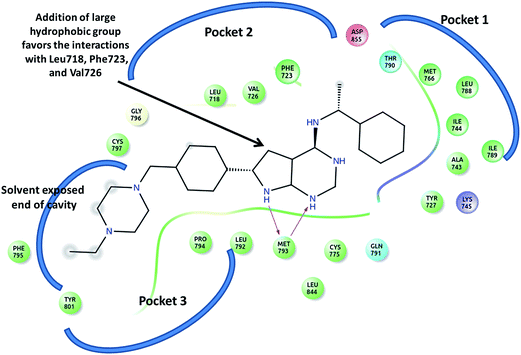 |
| Fig. 5 The ligand AEE and the surrounding residues present in pocket 1, 2 and 3 in active site of EGRF (2J6M). The arrow indicates the position on which modification has been undertaken for designing new analogs. | |
MD simulation studies of novel co-crystal analogues
Based on the observed pharmacophoric features, insights obtained from MD simulation analysis, and crystal structure analysis, we designed novel analogues of co-crystallized ligand, AEE_AN from AEE and AQ4_AN from erlotinib (AQ4), as they are among the few most potent compounds acting on EGFR (Fig. 6). The analogues were designed by adding benzyloxy group at a suitable position in the ligands AEE and AQ4. We expected that the addition of benzyloxy group at this position would lead to formation of pi-stacking interactions with Phe723 as well as hydrophobic interactions with Leu718 and Val726 residues. The additional pi-stacking and hydrophobic interactions are expected to stabilize the ligand and exhibit comparative tighter binding in the EGFR active site cavity. From MD simulation analysis of designed analogues (AQ4_AN and AEE_AN), it was found that the MMGBSA binding free energy increased remarkably as compared to parent molecules, AQ4 and AEE (Table 3). In case of AQ4_AN, it increased to −55.28 kcal mol−1 as compared to AQ4 ligand (−42.15 kcal mol−1). Similarly, it increased to −57.11 kcal mol−1 for AEE_AN in comparison to AEE ligand (−50.83 kcal mol−1). This data clearly establishes that increase in the affinity of ligands towards EGFR was due to the addition of benzyloxy group in the designed molecules (Fig. 7). As hypothesized, we observed that the residues Leu718, Phe723 and Val726 are present in sub-pocket 2, which accommodates the newly substituted benzyloxy group of compound AEE_AN and AQ4_AN (Fig. 7). We found that these interactions of sub-pocket 2 are absent in the case of the parent compound AEE and AQ4. It implies that occupancy of sub-pocket 2 significantly increases the binding of designed analogues to EGFR and hence can be considered as an essential criterion for tighter binding. The hydrogen bonding profile also showed increase in occupancy, that is, the residence time of H-bonds during MD simulations increases from 33.4% (AEE) to 45.2% (AEE_AN) and from 10.2% (AQ4) to 11.8% (AQ4_AN) for interactions between the ligand and residues Met793 (Fig. 7). In case of ligand AQ4_AN the additional H-bond with Cys797 had an occupancy of 7.80%, which was more than that of the co-crystallized ligand (AQ4, 6%). As it is well known that the efficiency of H-bond formation directly affects the ligand binding tendency, we expect that these modifications would result in tighter binding of newly designed molecules with EGFR and thus would enhance potency. We also compared the interaction energies with residues for parent and designed molecules. It was observed that the residues Leu718, Gly719, Phe723, Val726, Pro794, Gly796, and Tyr801 were responsible for higher contribution towards hydrophobic interaction energy for designed analogues as compared to the parent molecule (Fig. 8). Thus, it was clearly indicated that due to the addition of benzyloxy group, interactions with hydrophobic residues present in EGFR active site, Leu718, Phe723, and Val726 increased that in turn resulted in an increase in the total binding energy and subsequently more tight binding with EGFR protein. The designed ligands thus could act as potential EGFR inhibitors as well as anticancer leads.
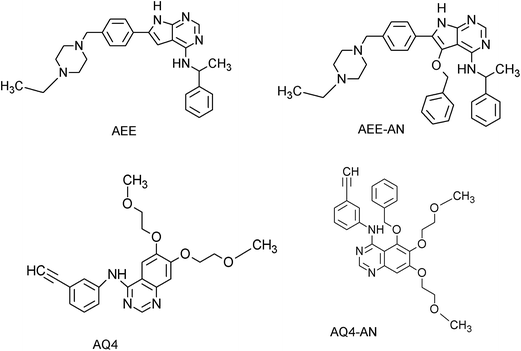 |
| Fig. 6 Chemical structures of the co-crystallized ligands (AEE and AQ4) and newly designed analogues (AEE-AN and AQ4-AN). | |
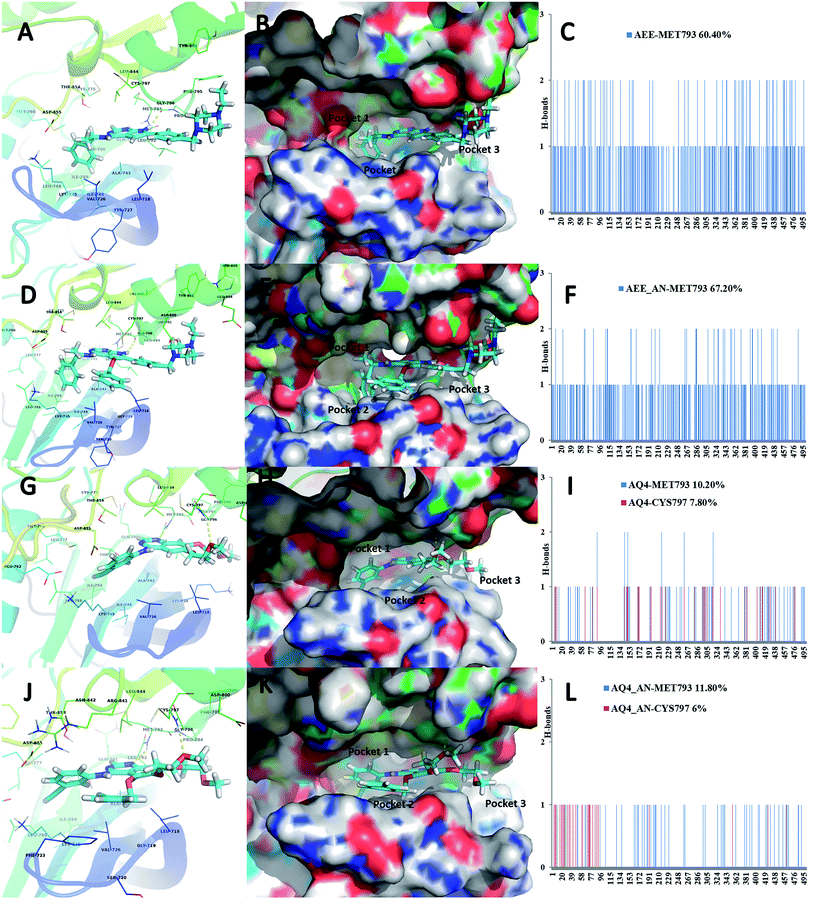 |
| Fig. 7 Ligand binding pose of ligands AEE (A), AEE_AN (D) AQ4 (G) and AQ4_AN (J) in the EGFR binding pocket in carton view showing the H-bonds with surrounding amino acid residues. (B), (E), (H), (K) in surface view with the sub-pockets 1, 2 and 3. (C), (F), (I), (L) show the occurrence of H-bonds observed throughout the 5 ns MD simulation run. | |
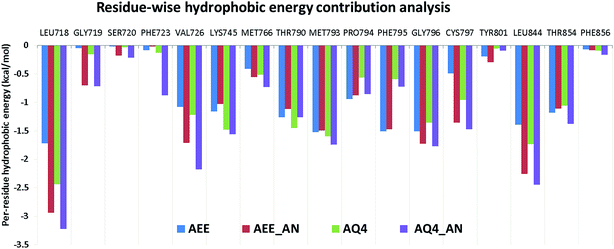 |
| Fig. 8 Residue-wise hydrophobic energy decomposition analysis for co-crystallized ligands and designed analogues in respective EGFR structures. | |
The observed computational results are also in accordance with our previous observations as well as experimental results wherein different investigators have shown that curcumin analogs that occupy sub-pocket 2 have in vitro activity against EGFR as low as 0.0079–1.86 μM (GI50).25,26 Furthermore, such compounds also exhibit inhibition against various lung cancer cell lines including A549 in submicromolar range.27,28 Additionally the two inhibitors i.e. erlotinib and AEE788, experimentally have been shown to bind strongly to EGFR with Kd of 53.5 nM and 10.9 nM respectively.17 As, the designed analogues have much higher binding affinity than the two known inhibitors it is expected that these compounds would atleast show comparable inhibition activity. The present study thus extends the previous experimental observations by providing a detailed structural understanding on the interactions and the rational for the observed activity. The study also provides the guide to design the new molecules having higher binding affinity in EGFR by occupying the sub-pocket 2.
Conclusion
In recent years, a plethora of studies has been performed by researchers to design novel EGFR inhibitors. As finding NCE's for EGFR target still remains a challenge, herein, we study the essential pharmacophoric features required for EFGR inhibitors to become tight binders and hence potential inhibitors. We collected 685 curcumin analogues and undertook molecular docking studies in three EGFR protein structures to identify the potential binders. The five lead compounds identified via docking were studied using MD simulation and analyzed for their MMGBSA binding free energy, H-bonding interactions, and residue-wise energy decomposition. Based on the MD simulation analysis, the critical pharmacophoric features present in ligands that are required for stable protein–ligand interactions and hence tight binding with EGFR were identified. Based on this information, new compounds were designed from parent co-crystallized compounds. We observed using MD that the addition of benzyloxy group to the parent compounds at the appropriate position leads to an increase in binding affinity of compounds as described from MMGBSA binding energy. We also observed that the residues Phe723 (involved in pi-stacking interactions) and Leu718 & Val726 (involved in hydrophobic interactions) occupy sub-pocket 2, which remains empty in case of parent inhibitors. The occupancy of sub-pocket 2 in the EGFR active site by analogues thus has been identified as an important factor for tight EGFR-inhibitor binding. The increase in ligand binding potential was also depicted by increase in H-bonding capacity with MET793 of designed ligands (AEE_AN and AQ4_AN), as compared to parent compounds. We propose that the newly designed analogues AEE_AN and AQ4_AN (erlotinib analogue) could act as potential EGFR inhibitors. The study thus provides an essential pharmacophoric requirement for potential EGFR inhibitors. It is expected that this study will be beneficial for design and discovery of new lead candidates working against EGFR.
Acknowledgements
SMA acknowledges Department of Health Research (DHR) Long Term Fellowship (SEC/DHR/HRD-FELLOW/2(5)/2011).
References
- D. Gonzalez de Castro, P. A. Clarke, B. Al-Lazikani and P. Workman, Clin. Pharmacol. Ther., 2013, 93, 252–259 CrossRef CAS PubMed.
- R. N. Jorissen, F. Walker, N. Pouliot, T. P. Garrett, C. W. Ward and A. W. Burgess, Exp. Cell Res., 2003, 284, 31–53 CrossRef CAS PubMed.
- Y. Yarden and M. X. Sliwkowski, Nat. Rev. Mol. Cell Biol., 2001, 2, 127–137 CrossRef CAS PubMed.
- R. A. Stein and J. V. Staros, BMC Evol. Biol., 2006, 6, 79 CrossRef PubMed.
- D. Raghav, V. Sharma and S. M. Agarwal, Interdiscip. Sci.: Comput. Life Sci., 2013, 5, 60–68 CrossRef CAS PubMed.
- I. S. Yadav, H. Singh, M. I. Khan, A. Chaudhury, G. P. Raghava and S. M. Agarwal, Adv. Anticancer Agents Med. Chem., 2014, 14, 928–935 CrossRef CAS.
- C. La Motta, S. Sartini, T. Tuccinardi, E. Nerini, F. Da Settimo and A. Martinelli, J. Med. Chem., 2009, 52, 964–975 CrossRef CAS PubMed.
- I. S. Yadav, P. P. Nandekar, S. Srivastavaa, A. Sangamwar, A. Chaudhury and S. M. Agarwal, Gene, 2014, 539, 82–90 CrossRef CAS PubMed.
- J. S. Chauhan, S. K. Dhanda, D. Singla, S. M. Agarwal and G. P. Raghava, PLoS One, 2014, 9, e101079 Search PubMed.
- H. Singh, S. Singh, D. Singla, S. M. Agarwal and G. P. Raghava, Biol. Direct, 2015, 10, 10 CrossRef PubMed.
- D. J. Newman and G. M. Cragg, J. Nat. Prod., 2012, 75, 311–335 CrossRef CAS PubMed.
- M. Mangal, P. Sagar, H. Singh, G. P. Raghava and S. M. Agarwal, Nucleic Acids Res., 2013, 41, D1124–D1129 CrossRef CAS PubMed.
- M. Mangal, M. I. Khan and S. M. Agarwal, Adv. Anticancer Agents Med. Chem., 2016, 16, 138–159 CrossRef CAS.
- K. Dhiman and S. M. Agarwal, RSC Adv., 2016, 6, 49395–49400 RSC.
- S. M. Ogbourne and P. G. Parsons, Fitoterapia, 2014, 98, 36–44 CrossRef CAS PubMed.
- A. L. Harvey, R. Edrada-Ebel and R. J. Quinn, Nat. Rev. Drug Discovery, 2015, 14, 111–129 CrossRef CAS PubMed.
- C. H. Yun, T. J. Boggon, Y. Li, M. S. Woo, H. Greulich, M. Meyerson and M. J. Eck, Cancer Cell, 2007, 11, 217–227 CrossRef CAS PubMed.
- J. Stamos, M. X. Sliwkowski and C. Eigenbrot, J. Biol. Chem., 2002, 277, 46265–46272 CrossRef CAS PubMed.
- D. K. Agrawal and P. K. Mishra, Med. Res. Rev., 2010, 30, 818–860 CAS.
- G. Jones, P. Willett, R. C. Glen, A. R. Leach and R. Taylor, J. Mol. Biol., 1997, 267, 727–748 CrossRef CAS PubMed.
- A. Salahuddin, S. M. Agarwal, F. Avecilla and A. Azam, Bioorg. Med. Chem. Lett., 2012, 22, 5694–5699 CrossRef CAS PubMed.
- M. F. Ansari, S. M. Siddiqui, S. M. Agarwal, K. S. Vikramdeo, N. Mondal and A. Azam, Bioorg. Med. Chem. Lett., 2015, 25, 3545–3549 CrossRef CAS PubMed.
- D. A. Case, T. E. Cheatham 3rd, T. Darden, H. Gohlke, R. Luo, K. M. Merz Jr, A. Onufriev, C. Simmerling, B. Wang and R. J. Woods, J. Comput. Chem., 2005, 26, 1668–1688 CrossRef CAS PubMed.
- W. Humphrey, A. Dalke and K. Schulten, J. Mol. Graphics, 1996, 14, 33–38 CrossRef CAS PubMed.
- M. J. Ahsan, H. Khalilullah, S. Yasmin, S. S. Jadav and J. Govindasamy, BioMed Res. Int., 2013, 2013, 239354 Search PubMed.
- P. Qiu, L. Xu, L. Gao, M. Zhang, S. Wang, S. Tong, Y. Sun, L. Zhang and T. Jiang, Bioorg. Med. Chem., 2013, 21, 5012–5020 CrossRef CAS PubMed.
- X. Qiu, Y. Du, B. Lou, Y. Zuo, W. Shao, Y. Huo, J. Huang, Y. Yu, B. Zhou, J. Du, H. Fu and X. Bu, J. Med. Chem., 2010, 53, 8260–8273 CrossRef CAS PubMed.
- Y. Zuo, J. Huang, B. Zhou, S. Wang, W. Shao, C. Zhu, L. Lin, G. Wen, H. Wang, J. Du and X. Bu, Eur. J. Med. Chem., 2012, 55, 346–357 CrossRef CAS PubMed.
Footnotes |
† Electronic supplementary information (ESI) available. See DOI: 10.1039/c6ra08517b |
‡ Equal contribution. |
|
This journal is © The Royal Society of Chemistry 2016 |