DOI:
10.1039/C5MD00556F
(Research Article)
Med. Chem. Commun., 2016,
7, 1007-1015
Predictive proteochemometric models for kinases derived from 3D protein field-based descriptors†‡
Received
4th December 2015
, Accepted 29th March 2016
First published on 31st March 2016
Abstract
Proteochemometrics, a method that simultaneously uses protein and ligand description, was used to model the target-ligand interaction space of 95 kinases and 1572 inhibitors. To build models, we applied 3-dimensional field-based description of the receptors, which allows the visualization of receptor and ligand features relevant for activity within the spatial framework of the binding sites. Receptor fields were derived from knowledge-based potentials and Schrödinger's WaterMaps, while ligands were described by different 1D, 2D and 3D descriptors. Besides good interpretability, which is important for inhibitor design, the obtained proteochemometric models also predicted external test sets with active and inactive ligands or additional protein targets for ligands with more than 80% accuracy and AUCs above 0.8.
Introduction
Protein kinases are enzymes, known to modulate various cellular, metabolic and signaling pathways through phosphorylation.1 Abnormalities in kinase regulation can lead to several diseases, including cancer, diabetes and inflammatory disorders, making kinases one of the most studied drug target classes. Thirty kinase inhibitors are currently available on the market.2 The majority of these inhibitors targets the ATP binding sites, therefore having a high probability for interacting with multiple kinases.3 The similarity of the ATP binding pockets limits the selectivity of kinase inhibitors, often leading to toxic side effects. Better understanding of interactions between multiple ligands and multiple targets can support the design of novel kinase inhibitors with improved efficacy and selectivity.
Employing machine learning approaches to build models that can predict affinity and selectivity of compounds has been a major focus in drug discovery. Proteochemometrics,4,5 a method that simultaneously uses protein and ligand description, can model the target-ligand interaction space. Proteochemometric models are suitable for studying the selectivity profiles of compounds, as they involve the correlation analysis of protein and ligand description with respect to the affinity of the receptors.4,5,6 To date, proteochemometrics has been applied to a wide range of drug targets including kinases, proteases, G protein-coupled receptors, cytochrome P450s and transport proteins.6
Previously conducted proteochemometric studies on kinases7,8 used sequence information to describe the targets and therefore these models had limited interpretability. Our previous study9 has shown that protein-derived fields can be used to create visually interpretable models for kinase inhibition, which highlight features that contribute to selective binding of ligands to certain kinases. However, the predictive capability of field-based descriptors for specific ligands and targets has not been investigated extensively and was therefore a main goal of our current study. We describe the development of proteochemometric models for 1572 inhibitors and 95 kinases, utilizing 3D structural information of kinases and their ligands. Earlier, we have interpreted the ligand features based on 2D Open Babel fingerprints, which do not account for the spatial distribution of ligand groups. In order to consider spatially more meaningful descriptors, we focus in the present article mainly on the interpretation of 4-point pharmacophoric fingerprints.
Methods
Activity data
We compiled interaction data for 1572 ligands and 95 kinases (Table S1 in the ESI‡) from experimental values for Kd, Ki, inhibition % and residual activity published in three articles, Kinase SARfari and CHEMBL18 (GSK and Millipore kinase screening data).10 The dataset contains 63
187 values for bioactivities. Because of different assay conditions, the values were not used as such in modeling; instead we assigned binary values to the data and grouped them as actives (pKd/pKi > 5, inhibition % at 1 μM > 10% and residual activity < 50%) and inactives (all remaining observations/protein–ligand combinations).
Protein structures
A set of 95 unique kinases, where each kinase had activity data for at least 5 compounds, was chosen from a previously collected dataset with 122 DFG-in structures.9 Initially additional chains, water molecules, non-kinase domains and ligands were removed to prepare the protein structures for further processing. In the case of kinases with multiple chains, only the more complete chain was used. All structures were then prepared by using a KNIME workflow11,12 that involved addition of hydrogen atoms, correction of residues with missing atoms, assignment of protonation states of charged amino acids and minimization of hydrogen atoms, keeping the heavy atoms fixed. Additionally, missing residues near the binding site were modeled for 5 kinases, using the PRIME12 side-chain prediction tool in Maestro. Following protein preparation, all kinases were superimposed on a common reference structure (c-Met kinase, PDB id 3A4P) using the protein structure alignment tool in Maestro.12
Ligand structures
Structures of kinase inhibitors extracted from Metz et al.,13 KinaseSarfari and Chembl10 were generated in Maestro12 based on their SMILES notation, whereas structures of those inhibitors published by Karaman et al.14 and Davis et al.15 were downloaded from PubChem database in SDF format. 3D structures of the ligands were generated by using the Ligprep12 module of the Schrödinger package, with default settings. Multiple conformations were created for each ligand, using Confgen12,16 in comprehensive mode, but only the lowest energy conformation of each ligand was used for descriptor calculations. In Confgen, OPLS-2001 and OPLS-2005 force fields were used for initial structure generation and energy minimization respectively.
Description of kinases and ligands
Kinases were described by knowledge-based contact potentials (polar and lipophilic fields)17,18 and WaterMap19,20 derived fields (stable and unstable water fields) of ATP binding sites (for details, see Subramanian et al.9). Ligands were characterized by 1- and 2-dimensional Mold2 descriptors21 (777) and Babel's FP4 fingerprints22,23 (133). Additionally, 4-point pharmacophoric fingerprints (4-PFP) generated by Canvas12,24 were used as 3-dimensional descriptors.
Proteochemometric modeling
Principal component analysis
Due to the large number of descriptors, principal component analyses (PCA) of protein fields and ligand descriptors were performed. PCA analyses were performed separately for the two training sets and used to predict scores for the corresponding test sets. Prior to PCA, the descriptors were scaled to unit variance. Considering the effects of over-fitting, only the principal components with eigenvalues above 1 were extracted. The number of components extracted for each descriptor block along with the variation explained is shown in Table 2.
Table 2 Principal component analysis (PCA) of protein and ligand descriptors
Descriptors |
Components extracted |
Variation explained (%) |
4-Point pharmacophoric fingerprints.
|
Ligand training set |
Polar and lipophilic protein fields, stable and unstable water fields |
36 |
51 |
Open Babel |
39 |
71 |
Mold2 |
74 |
91 |
4-PFPa |
62 |
85 |
|
Target training set |
Polar and lipophilic protein fields, stable and unstable water fields |
29 |
51 |
Open Babel |
40 |
72 |
Mold2 |
73 |
91 |
4-PFPa |
61 |
85 |
Model creation and validation
Non-linear support vector machines (SVM) and random forests (RF) were used in proteochemometric modeling (PCM) to classify the observations as actives and inactives, based on the PCA scores of protein fields and ligand descriptors. PCM classification models were trained on both training datasets. The ligand prediction model was trained on the ligand training set and the target prediction model was trained on the target training set. All RF and SVM models were internally validated by 5-fold cross-validation. During cross-validation, the dataset was divided into 5 groups. Models trained on 4 groups were used to predict the activity classes of the fifth group. This procedure was repeated, until all the groups were predicted at least once. The predictive ability of the ligand and target prediction models was further assessed by means of external prediction of ligand (315 ligands) and target (20 kinases) test sets respectively. Model performance was assessed by area under the ROC curve (AUC) values and Matthews coefficients28 which provide a single balanced model performance measure, considering false positives and false negatives. Additionally, the models were subjected to permutation validation/Y scrambling by re-fitting them to the data with randomly assigned classes. Random classes were assigned 20 times and the models were fitted to the permuted data. The performance of permutation validation was assessed by the intercepts obtained by plotting the correlation coefficient of the original and random classes against the Matthews coefficients obtained from the fitted and cross-validated data.
RF models were trained by using the default parameters of random forest29 function in R. SVM modeling was performed by using the ksvm30 function in R. Prior to SVM modeling the descriptors were centered and scaled to unit variance. The kernel parameter was set to radial basis function (RBF) and the models were trained across a range of sigma (2−10, 2−8, 2−6, 2−4, 2−2, 20, 22, 24) and cost (1, 10, 20, 30, 40, 50, 60, 70) parameters. Best sigma and cost values were identified based on the performance of the models assessed by Matthews coefficients during internal cross-validation (Table S2 in ESI‡).
Model interpretation
Interpretation of SVM models is technically not straightforward; therefore, we focused on RF model interpretation. Models were interpreted by using the Gini index and the descriptors' correlation to the active and inactive class, computed using “importance( )” function in random forest29 package of R. If the variable used for splitting in the decision tree increases the purity of the nodes, it acquires higher decrease of the Gini index. As the Gini index is an estimate of the homogeneity of the nodes, high mean decrease in Gini index implies that the variable is important for classification and vice versa.29 Gini index values may vary during training of different RF models made using the same training set, probably caused by random nature of the bagging algorithm. Due to this inconsistency, we trained a set of 100 different RF models. Gini indices resulting from 100 models were found to vary by 1–1.5% of the total mean for the different descriptors. The variation in Gini index values of the variables is negligible, thereby making them valid for interpretation. Interpretation, purely based on the mean decrease in Gini index does not provide any information about the relevance of the descriptors for activity. Therefore, the correlation of the descriptors to active and inactive class was used as the basis to identify the protein and ligand descriptors that have higher correlation to the active than to inactive class. The correlation values of the variables were extracted from all 100 models and their average values were used to identify the descriptors, for which the difference in correlation between active and inactive class is 1 or more. For each of these descriptors, loadings of the corresponding principal components were examined to identify the protein and ligand features that contribute to activity. Descriptors that had nearly the same correlation to both active and inactive class and those that had higher correlation to the inactive class were not taken into account Apart from considering the correlation to the active class, the relevance of the 4-PFP used for interpretation were further verified by computing the prediction probabilities, after excluding certain fingerprints.
Applicability domain
The applicability domain31 (AD) of a model provides an estimate of the extent of the chemical and target space to which the models can be applied. We used the K-nearest neighbor (K = 3) algorithm to evaluate the AD of the compounds and the targets. For the compound space, we computed the Tanimoto similarities of the ligand test set compounds against the ligand training set compounds, based on the PCA scores of the 4-PFP. For the target space, we calculated the Euclidean distance between the target test set and target training set kinases, based on the PCA scores of the protein field descriptors. We then identified the 3 closest neighbors in the ligand training set for each test set compound and in the target training set for each test set kinase.
Results and discussion
Proteochemometric models
We built proteochemometric models with different combinations of field-based protein and ligand descriptors in order to test the potential of these descriptors to predict ligand and target activities from external test sets. Performances of the ligand and target prediction models with respect to internal cross-validation and external test set prediction are reported in Fig. 1 and Tables 3 and 4. For simplicity, only the receiver operating characteristic (ROC) curves, AUC values and Matthews coefficients are reported. Other performance measures such as sensitivity, specificity, accuracy and kappa coefficient are reported in Tables S3 and S4 of the ESI.‡
 |
| Fig. 1 ROC curves showing the performances of PCM models based on different training and test sets. Blue, sky blue and magenta colored curves represent the performances of PCM models based on Open Babel, Mold2 and 4-PFP descriptors respectively. Continuous lines correspond to the internal validation and dotted lines represent the prediction performances of the test set. | |
Table 3 Performance of proteochemometric (PCM) models in predicting activities of ligand test set (315 ligands). AUCs and Matthews coefficients (in parenthesis) resulting from PCM models based on different ligand descriptors and different machine learning approaches
Ligand descriptors |
Method |
Cross-validation |
External prediction |
Y scrambling |
Fitted intercepts |
Cross-validated intercepts |
Support vector machines.
Random forests.
4-Point pharmacophoric fingerprints.
|
Open Babel |
SVMa |
0.83 (0.47) |
0.70 (0.28) |
−0.006 |
−0.007 |
RFb |
0.86 (0.48) |
0.73 (0.25) |
0 |
0.008 |
Mold2 |
SVMa |
0.87 (0.56) |
0.83 (0.52) |
−0.007 |
−0.011 |
RFb |
0.88 (0.50) |
0.85 (0.47) |
0 |
0.002 |
4-PFPc |
SVMa |
0.86 (0.54) |
0.82 (0.47) |
0.002 |
0.003 |
RFb |
0.87 (0.49) |
0.83 (0.42) |
0 |
−0.002 |
Table 4 Performance of proteochemometric (PCM) classification models in predicting affinities for the target test set (20 kinases). AUCs and Matthews coefficients (in parenthesis) resulting from RF models based on different ligand descriptors
Ligand descriptors |
Method |
Cross-validation |
External prediction |
Y scrambling |
Fitted intercepts |
Cross-validated intercepts |
4-Point pharmacophoric fingerprints.
Random forests.
|
Open Babel |
RFb |
0.86 (0.49) |
0.82(0.39) |
0 |
−0.0016 |
Mold2 |
RFb |
0.87 (0.49) |
0.83(0.42) |
0 |
0.0041 |
4-PFPa |
RFb |
0.87 (0.49) |
0.82(0.41) |
0 |
0.0021 |
As shown in Table 3 and Fig. 1, all ligand prediction PCM models have similar performances with AUCs ranging from 0.83 to 0.88 (Matthews coefficients: 0.48–0.56), irrespective of the descriptors used. Overall, RF models have shown slightly better performance in both internal and external validation of ligands, when compared to SVM models. However, the variation is not significant, as the AUCs increase only by 1–3%. For the prediction of external ligands, models based on Open Babel descriptors have the lowest performance with AUCs of about 0.70. Poor performance of Open Babel descriptor models goes well in line with the results from PCA analysis. PCA of Mold2 and 4-PFP yield almost twice as high scores as with Open Babel descriptors. Open Babel fingerprints just describe the presence or absence of functional groups, which makes their description less detailed, when compared to more complex descriptors like Mold2 and 4-PFP. Better performance of Mold2 and 4-PFP models than the Open Babel models shows that the additional information captured by these descriptors is relevant for good predictability. Considering the performance of target prediction models with respect to internal cross-validation, both RF and SVM models have nearly the same AUCs (0.83–0.87) and Matthews (0.46–0.54) as that of the ligand prediction models (see Tables 4 and S4 in ESI‡). For the prediction of external targets, AUCs for RF models range from 0.82 to 0.83, depending on the ligand descriptors used (see Table 4 and Fig. 1).
The validity of the models was further tested by permutation validation to ensure that the predictions are not obtained by chance. For models with randomly assigned classes, Matthews resulting from model fitting and cross-validation was either close to 0 or negative (see Tables 3 and 4). Low Y intercepts based on fitted and cross-validated Matthews clearly indicate that the models are not over-fitted and are valid enough to be considered for further external prediction and interpretation.
Individual prediction accuracies for kinases and ligands were analyzed by using the target and ligand prediction models based on 4-PFP ligand descriptors. By analyzing the prediction accuracies for individual kinases, we found that excellent test set predictions were obtained for PAK7 and CAMK4 kinases with prediction accuracies over 90%. These kinases are similar to MST3 kinase, for which good performance (accuracy: 97%) was already seen during cross-validation. This suggests that predictability for novel, but similar kinases could be assessed already from cross-validation results. The results from the external target validation therefore indicate that the model is able to predict activity patterns for many kinases, albeit few exceptions exist. Two kinases, EGFR_mut and SLK, have very low prediction accuracies (<60%). EGFR_mut is the only mutant protein in our dataset; the rather small structural differences compared to the EGFR wild type might not be captured well enough by the descriptors. In case of SLK, many false negative predictions occur, which can be attributed to the presence of very few actives for the MST3 kinase, which is most similar to SLK. Additionally, we conducted a systematic analysis to compare the prediction performances of various kinase families (see ESI,‡ Tables S5 and S6). Based on the internal cross-validation results, many false negative predictions were found in the AGC and OPK family, resulting in sensitivity as low as 0.2. Incorrect predictions can be attributed to the presence of only few kinase representatives in these two families. Excluding some of these kinases during cross-validation could have resulted in poor predictions. An exception to this is the TKL family, which is well predicted, despite the presence of only 5 kinase representatives. Better predictions of the TKL family compared to AGC and OPK seem to depend on the presence of several related members of the TK family. Considering the predictions of external targets, no significant trends were observed with respect to different kinase families (Matthews 0.35–0.47).
Analyzing the individual prediction accuracies of ligands (fraction of kinase targets for which a ligand is classified correctly as active or inactive) revealed that 10% of the 315 ligands in the external ligand test set are predicted with 100% accuracy, 49% between 80 and 99% accuracy and 30% of the ligands have prediction accuracies ranging from 51 to 79%. 11% of the ligands have worse prediction accuracies than random (accuracy ≤50%). Poor prediction accuracies for these ligands probably result from low coverage of these chemotypes in the training set.
Performance comparison for different datasets
The data used for PCM modeling was extracted from different sources with different assay conditions. Therefore, a data set dependent variation of the model performance might be expected. When we compared the performances of ligand prediction models based on internal cross validation (see ESI,‡ Table S7), all the four datasets (Ambit, Metz, Millipore and GSK) had nearly the same AUCs ranging from 0.82–0.89, depending on the descriptors and machine learning approaches used. However, with respect to external validation, Ambit and Millipore sets had slightly lower performances than the Metz and GSK sets with 3–15% decreases in AUCs (see ESI,‡ Fig. S2 and Table S7). This variation in AUCs could be associated with the relatively small number of compounds in Ambit (50 pKd values) and Millipore (114 residual activities) sets used for training the models, compared to the Metz (864 pKi values) and GSK (228 inhibition percentages) sets.
Influence of 3D ligand conformations on proteochemometric modeling
Of the 1257 ligands used for training the models, complex X-ray structures are known only for 4 ligands, which could serve as references for bioactive conformations. Among the multiple conformations generated for each ligand, only the conformation with the lowest energy was used for fingerprint calculations. To verify, if the conformation selection influences the PCM model, we trained a set of models using 4-PFP calculated from conformations with different energies and from the PDB structures of the ligands, SKI-606 (Bosutinib) and TAE-684. Comparing their internal cross-validation performances (see ESI,‡ Table S8), we found that the AUCs were about 0.86, irrespective of the conformation used. Considering the prediction probabilities of the active class, conformations with the lowest energies and PDB structures had the highest probability to be predicted as active, when compared to the highest energy conformation. Therefore, choosing the lowest energy conformation is suitable for 3D descriptor calculations.
Additionally, we calculated the root mean square deviations (RMSDs) of the lowest energy conformations against the PDB structures. RMSDs for the ligands SKI-606 and TAE-684 are 6.5 and 6.2 Å respectively. Despite the fact, that the RMSDs are high, PCM models based on PDB structures and lowest energy conformations have nearly the same performance. Similar prediction probabilities of the active class further ascertain that 4-PFP is rather insensitive to the conformation used for descriptor calculations.
Impact of datasets on model quality
Empirical models are susceptible to the quality and variation of experimental data, which for example, depends on assay conditions and errors introduced by automated data collection. Since we extracted data from different sources, building a continuous model by using the exact activity values seems not the preferred choice. One way to use such heterogeneous data in a proteochemometric model is to classify the data into distinct classes based on certain cut-offs. But the predictive power of models is often influenced by the bias introduced by artificial cut-offs, especially affected by observations that lie close to borders between classes.
Another challenge in predictive modelling are unbalanced datasets.32 In the present study, of the 63
187 activity values used in modelling, only 15
729 are classified as actives. The large number of inactives in the dataset is likely to increase the proportion of actives being predicted as inactive by our models. Despite the limitations arising from the smaller number of active representatives and artificial cut-offs, overall AUCs above 0.8 and Matthews coefficients of about 0.5 suggest that a reasonable fraction of the actives is predicted correctly, thereby supporting the validity of the models.
Applicability domain (AD)
AD analysis was conducted to identify the similarity thresholds above which the compounds and targets are predicted with more than 80% accuracy. Based on our analysis of the compound space, we found that a Tanimoto similarity of 4-PFP of at least 0.8 is required for reliable ligand test set predictions (Fig. 2, left). 64% of the compounds with Tanimoto similarity above 0.8 have high accuracy levels (>80%). The remaining 36% of the highly similar test set compounds have an average prediction accuracy of 58%. Despite the high similarity to the descriptor space of the ligand training set compounds, low prediction accuracies are obtained for the 36% of the test set because of the sparse activity space resulting from low coverage (42%) of the dataset used in modeling.
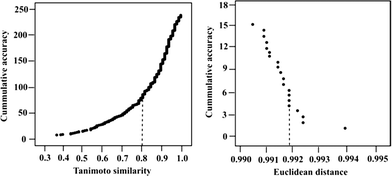 |
| Fig. 2 The applicability domain of the models. The left panel shows the Tanimoto similarity of the test set ligands based on 4-PFP plotted against the cumulative prediction accuracy. The right panel shows the Euclidean distance of the test set kinase field points plotted against cumulative accuracy. Dotted lines in the figures represent the cut-offs for predictions of external ligands and targets. | |
AD analysis for the kinase targets reveals that an Euclidean distance of 0.992 in protein descriptors space or less is required for reliable prediction of external targets (Fig. 2, right). However, the narrow Euclidean distance range of the test set kinases, resulting from few significantly varying field points, makes it difficult to draw conclusions concerning the correlation between Euclidean distance and cumulative accuracy.
Visual interpretation of models
All visual interpretation described in this article is based on the RF ligand prediction models. Since the ligand prediction models have similar performances as the target prediction models and are more balanced in terms of the number of targets (95 versus 75 kinases) included in the modeling, we chose the RF ligand prediction models for interpretation. Protein and ligand features relevant for affinity were interpreted, based on their PCA scores (see Methods) and visualized with MOE.17 In the following, we analyze three examples, where inhibitors show a clear preference for one kinase over another and discuss structural features on the protein and ligand side suggested by the RF models, which strongly determine if a specific protein-ligand combination is active or inactive.
In Fig. 3, we compare pharmacophoric features suggested most relevant for binding of ligands to STK10. 4-PFPs that are expected to influence binding of vandetanib are ADRR, AAAR, AARR and AADR, while only the fingerprint ADRR is considered important for the interaction of the low affinity ligand SB-202190 with STK10. Especially lipophilic features of AAAR, AARR and AADR are suggested to have interactions with the gatekeeper residue and residues in the hydrophobic pocket, contributing to the potency of vandetanib towards STK10. The overall activity profiles of these 2 inhibitors reveal that SB-202190 has a pKi > 6 for only 5% of the kinases in the dataset, whereas vandetanib inhibits 20% of these kinases with similar strength. The activity of vandetanib across a wider range of kinases could be attributed to the presence of strong hinge interactions, illustrated especially by several hydrogen bond features. The presence of just one relevant fingerprint (ADRR) in SB-202190, mostly interacting with gate keeper and hydrophobic pocket residues, seems to make it more selective but less potent for several targets. Excluding AAAR, AADR, AARR and ADRR fingerprints, further reduces the prediction probabilities by 10–15%, (see ESI,‡ Table S9) which highlights the importance of these fingerprints in predicting the activity of vandetanib towards STK10.
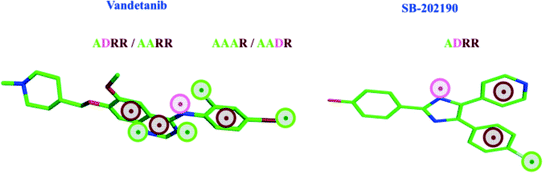 |
| Fig. 3 Pharmacophoric features of vandetanib (ZD6474) relevant for its activity (Kd = 81 nM) on STK10 (left panel). Pharmacophoric features of SB-202190 that is weakly active (Ki > 10 000 nM) on STK10 (right panel). Four 4-PFP (AAAR, ADRR, AARR, AADR) identified as relevant for STK10 inhibition are marked as colored circles with patterns (green – H-acceptor, pink – H-donor, brown – aromatic ring). | |
The example in Fig. 4 shows protein fields and pharmacophoric features relevant for the affinity of the 1.1 nM inhibitor TAE-684 for ALK kinase (left panel). Presence of polar and unstable water field points near the pharmacophoric fingerprint AAAR contributes to the hinge interactions, commonly observed for kinase inhibitors. Lipophilic field points (yellow) and the unstable water field points (red) found in the hydrophobic pocket (a region frequently exploited for selectivity) are predicted to enhance binding. We also speculate that stable water field points (green) close to the pharmacophoric fingerprint AARR indicate water-mediated interactions with residue E1210, thereby improving affinity. Further, the 4-PFPs, AARR and AAAR suggested by our models are highly relevant for affinity as the prediction probabilities of TAE-ALK pair drops from 0.81 to 0.29 (see ESI,‡ Table S9), after excluding these fingerprints.
 |
| Fig. 4 Protein fields and ligand pharmacophoric features important for the interactions of ALK kinase (grey) with TAE-684 (green) (X-ray structure PDB id: 2XB7, left panel). Protein fields of AKT2 kinase (grey) and the low affinity ligand TAE-684 (green) modelled into the binding pocket (right panel). Two 4-PFP (AAAR, AARR) identified as relevant for the affinity to ALK kinase are marked as colored circles (green – H-acceptor, brown – aromatic ring). Polar, lipophilic, unstable and stable water fields that are supposed to influence the kinase affinity are represented as blue, yellow, red and green spheres, respectively. | |
The low affinity of TAE-684 for AKT2 kinase (Ki > 10
000 nM) could be explained by less favorable interactions in the region around F239 (Fig. 4, right), unfavorable interactions with the piperidyl moiety of TAE-684 and absence of stable water areas in the hydrophobic pocket suggested by our models. Additionally, we speculate that the presence of stable water field points in the hydrophobic pocket of AKT2 is likely to interfere with ligand binding, thereby reducing the potency of TAE-684 towards AKT2 (Fig. 4, right).
In another example (Fig. 5), we show the features relevant for the interactions of bosutinib with ABL1 (Ki = 0.12 nM) and PAK7 kinase (Ki > 10
000 nM). Polar and unstable water field points close to the relevant pharmacophoric fingerprint ADRR contribute to the well-conserved hinge interactions. Additionally, the lipophilic and unstable water fields near the pharmacophoric fingerprint AAAR seem to promote better binding of bosutinib to ABL1 than to PAK7. Presence of few unstable water field points and absence of preferred lipophilic interactions at the hydrophobic pocket further explain the lower affinity of bosutinib for PAK7. The importance of 4-PFP AAAR in predicting the affinity of bosutinib towards ABL1 was further confirmed by a 30% decrease in prediction probability, after excluding this fingerprint. The other fingerprint ADRR has only moderate influence on the affinity of bosutinib, as the prediction probability decreases only by 10% (0.58 to 0.47), after its removal.
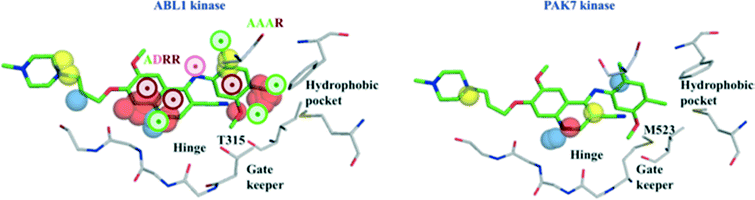 |
| Fig. 5 Protein fields and ligand pharmacophoric features important for the interactions of ABL1 kinase (grey) with bosutinib (green) (left panel, X-ray PDB id: 3UE4). Protein fields of PAK7 kinase (grey) and the ligand bosutinib (green) that weakly interacts with PAK7, modelled into the pocket based on its ABL1 binding mode (right panel). Two 4-PFP (AAAR, ADRR) identified as relevant for the affinity of ABL1 kinase are shown as colored circles (green – H-acceptor, pink – H-donor, brown – aromatic ring). Polar, lipophilic and unstable water fields that influence affinity according to the RF model are represented as blue, yellow and red spheres, respectively. | |
Advantages of classification models using field-based PCM
As shown in the examples discussed above, our field-based PCM models clearly visualize protein and ligand features, critical for a specific kinase-ligand interaction. Earlier, we have shown that three-dimensional field description of proteins can be used for proteochemomectric modelling of continuous activity data.9 However, there are not that many inhibition constants in the public domain and therefore the models with continuous data are limited to a small subset of kinase-ligand interactions. In the present article, we show that one can use protein fields for classification models incorporating also the much larger amount of inactive and single concentration data points, which substantially increases the applicability domain of the models and therefore should better support the prediction of external test sets. Interestingly, it was necessary to apply more sophisticated descriptors and complex machine learning algorithms, indicating that reasons for ligand inactivity are more multifaceted than plain decrease of activity, as observed for continuous models. Additionally, like with the continuous PCM models described in our previous article,9 we obtain visually interpretable information that suggests features contributing to a ligand's activity or inactivity towards certain kinases.
Critical issues with model interpretation
A challenge for the validation of visual interpretation of these models is finding illustrative three-dimensional complex structures for low affinity compounds. The reason for this obviously is that in most cases it is possible to obtain experimental complex structures for potent ligand–receptor pairs, but very few crystal structures are available for poorly active or even inactive compounds. Therefore, it is necessary to generate docking models for most low affinity ligands in order to visualize them, but the quality of these docking poses is frequently questionable. Consequently, we use simple superimposition of kinase structures with different ligands to visualize low affinity ligands in the context of our models and to examine relevant features found in inactive ligand–receptor pairs. One could perhaps question the validity of such estimates especially for inactive ligands, but for the interpretation of PCM models, we observed that the overlays serve better compared to docking poses, as one usually can pinpoint the most relevant reasons for inactivity of the ligand as predicted by the PCM model. Additionally to the three cases mentioned in this article, we identified further examples where relevant model features are in accordance with experimental findings.
Another problem associated with the model interpretation is that the variables identified are relevant only for individual observations and it is difficult to generalize their relevance for all data for a single ligand or kinase. An alternative approach to address this issue could be the estimation of prediction differences in the presence and absence of features. However, implementing this approach would be time consuming and computationally expensive for large datasets like ours, but could be useful for smaller sets. Another critical issue arising out of Gini index based interpretation is the increased preference of ligand over protein descriptors. The ligand descriptors used for training the models were nearly twice as many as the protein descriptors, which might influence the variables chosen for interpretation. The presence of a larger number of ligand descriptors increases their probability to acquire high mean decrease in Gini index values over the protein descriptors. Nevertheless, our interpretation is not biased towards the top 10 variables; we interpret both the protein and ligand descriptors that have positive correlation to activity. The problems discussed above clearly show the need to develop better tools for interpretation of non-linear models. Despite the limitations with Gini index based interpretation, the examples shown in this article agree well with relevant ligand and kinase features described in the literature.
Conclusions
We have shown that field-based proteochemometrics can be used successfully to generate both predictive and visually interpretable models, which is an advantage compared to models using simpler descriptions of target proteins. The present models are not only suitable for the prediction of activities of ligands, but also provide an improved understanding of the protein and ligand features that affect the binding of a compound to certain protein targets. This can directly be exploited in medicinal chemistry programs aiming at the modulation of ligand selectivity or for the understanding of altered interactions e.g. with resistance mutants. The possibility also to predict the protein target space, illustrated by the prediction of activities of 20 kinases not included in the training set (Table 4), makes this a promising approach to estimate potential polypharmacology of newly designed kinase inhibitors. Overall, this study provides clear evidence for the usefulness of field-based proteochemometric approaches in inhibitor design and their advantages compared to low dimensional protein description and “traditional” QSAR. The method combines illustrative capabilities, as docking methods would provide, with advanced correlation methods of experimental values and molecular features provided by proteochemometrics.
Acknowledgements
V. S. acknowledges funding from the Helsinki University Research Foundation and the 3i project (TEKES). The Finnish National Doctoral Program in Informational and Structural Biology and Integrative Life Sciences is thanked for organizing graduate studies and providing support to graduate education.
References
- P. Cohen and D. R. Alessi, ACS Chem. Biol., 2013, 8, 96–104 CrossRef CAS PubMed.
- US Food and Drug Administration approved small molecule protein kinase inhibitors, http://www.brimr.org/PKI/PKIs.htm.
- M. A. Fabian, W. H. Biggs, D. K. Treiber, C. E. Atteridge, M. D. Azimioara, M. G. Benedetti, T. A. Carter, P. Ciceri, P. T. Edeen, M. Floyd, J. M. Ford, M. Galvin, J. L. Gerlach, R. M. Grotzfeld, S. Herrgard, D. E. Insko, M. A. Insko, A. G. Lai, J.-M. Lélias, S. A. Mehta, Z. V. Milanov, A. M. Velasco, L. M. Wodicka, H. K. Patel, P. P. Zarrinkar and D. J. Lockhart, Nat. Biotechnol., 2005, 23, 329–336 CrossRef CAS PubMed.
- P. Prusis, R. Muceniece, P. Andersson, C. Post, T. Lundstedt and J. E. S. Wikberg, Biochim. Biophys. Acta, Protein Struct. Mol. Enzymol., 2001, 1544, 350–357 CrossRef CAS.
-
J. E. S. Wikberg, M. Lapinsh and P. Prusis, Chemogenomics in Drug Discovery: A Medicinal Chemistry Perspective, Wiley-VCH Verlag GmbH & Co. KGaA, Weinheim, FRG, 2004, pp. 289–309 Search PubMed.
- I. Cortés-Ciriano, Q. U. Ain, V. Subramanian, E. B. Lenselink, O. Méndez-Lucio, A. P. IJzerman, G. Wohlfahrt, P. Prusis, T. E. Malliavin, G. J. P. van Westen and A. Bender, Med. Chem. Commun., 2015, 6, 24–50 RSC.
- M. Lapins and J. E. S. Wikberg, BMC Bioinf., 2010, 11, 339 CrossRef PubMed.
- M. Fernandez, S. Ahmad and A. Sarai, J. Chem. Inf. Model., 2010, 50, 1179–1188 CrossRef CAS PubMed.
- V. Subramanian, P. Prusis, L.-O. Pietila, H. Xhaard and G. Wohlfahrt, J. Chem. Inf. Model., 2013, 53, 3021–3030 CrossRef CAS PubMed.
- A. P. Bento, A. Gaulton, A. Hersey, L. J. Bellis, J. Chambers, M. Davies, F. A. Kruger, Y. Light, L. Mak, S. McGlinchey, M. Nowotka, G. Papadatos, R. Santos and J. P. Overington, Nucleic Acids Res., 2014, 42, D1083–D1090 CrossRef CAS PubMed.
-
M. R. Berthold, N. Cebron, F. Dill, G. D. Fatta, T. R. Gabriel, F. Georg, T. Meinl, P. Ohl, C. Sieb and B. Wiswedel, Studies in Classification, Data Analysis, and Knowledge Organization, Springer, Germany, 2007, pp. 319–326 Search PubMed.
-
Maestro, 9.8, Schrödinger, LLC, New York, NY, 2014 Search PubMed;
LigPrep, version 3.0, Schrödinger, LLC, New York, NY, 2014 Search PubMed;
ConfGen, version 2.8, Schrödinger, LLC, New York, NY, 2014 Search PubMed;
Canvas, version 2.0, Schrödinger, LLC, New York, NY, 2014 Search PubMed;
Protein Preparation Wizard, Schrödinger Suite, 2014 Search PubMed;
Epik version 2.2, Schrödinger, LLC, New York, NY, 2014 Search PubMed;
Impact version 5.7, Schrödinger, LLC, New York, NY, 2014 Search PubMed;
Prime version 3.0, Schrödinger, LLC, New York, NY, 2014 Search PubMed.
- J. T. Metz, E. F. Johnson, N. B. Soni, P. J. Merta, L. Kifle and P. J. Hajduk, Nat. Chem. Biol., 2011, 7, 200–202 CrossRef CAS PubMed.
- M. W. Karaman, S. Herrgard, D. K. Treiber, P. Gallant, C. E. Atteridge, B. T. Campbell, K. W. Chan, P. Ciceri, M. I. Davis, P. T. Edeen, R. Faraoni, M. Floyd, J. P. Hunt, D. J. Lockhart, Z. V. Milanov, M. J. Morrison, G. Pallares, H. K. Patel, S. Pritchard, L. M. Wodicka and P. P. Zarrinkar, Nat. Biotechnol., 2008, 26, 127–132 CrossRef CAS PubMed.
- M. I. Davis, J. P. Hunt, S. Herrgard, P. Ciceri, L. M. Wodicka, G. Pallares, M. Hocker, D. K. Treiber and P. P. Zarrinkar, Nat. Biotechnol., 2011, 29, 1046–1051 CrossRef CAS PubMed.
- K. S. Watts, P. Dalal, R. B. Murphy, W. Sherman, R. A. Friesner and J. C. Shelley, J. Chem. Inf. Model., 2010, 50, 534–546 CrossRef CAS PubMed.
-
Molecular Operating Environment (MOE), 2013.08, Chemical Computing Group Inc., 1010 Sherbooke St. West, Suite #910, Montreal, QC, Canada, H3A 2R7, 2015 Search PubMed.
- C. Hoppe, C. Steinbeck and G. Wohlfahrt, J. Mol. Graphics Modell., 2006, 24, 328–340 CrossRef CAS PubMed.
-
WaterMap, version 1.4, Schrödinger, LLC, New York, NY, 2012 Search PubMed.
- D. D. Robinson, W. Sherman and R. Farid, ChemMedChem, 2010, 5, 618–627 CrossRef CAS PubMed.
- H. Hong, Q. Xie, W. Ge, F. Qian, H. Fang, L. Shi, Z. Su, R. Perkins and W. Tong, J. Chem. Inf. Model., 2008, 48, 1337–1344 CrossRef CAS PubMed.
- N. M. O'Boyle, M. Banck, C. A. James, C. Morley, T. Vandermeersch and G. R. Hutchison, J. Cheminf., 2011, 3, 33 Search PubMed.
-
The Open Babel Package, version 2.3.0, http://openbabel.org Search PubMed.
- J. Duan, S. L. Dixon, J. F. Lowrie and W. Sherman, J. Mol. Graphics Modell., 2010, 29, 157–170 CrossRef CAS PubMed.
-
RDKit: Open-source cheminformatics, http://rdkit.org/ Search PubMed.
- M. Ashton, J. Barnard, F. Casset, M. Charlton, G. Downs, D. Gorse, J. Holliday, R. Lahana and P. Willett, Quant. Struct.-Act. Relat., 2002, 21, 598–604 CrossRef CAS.
- J. L. Durant, B. a. Leland, D. R. Henry and J. G. Nourse, J. Chem. Inf. Comput. Sci., 2002, 42, 1273–1280 CrossRef CAS PubMed.
- B. W. Matthews, Biochim. Biophys. Acta, 1975, 405, 442–451 CrossRef CAS.
- A. Liaw and M. Wiener, R News, 2002, 2, 18–22 Search PubMed.
- A. Karatzoglou, A. Smola, K. Hornik and A. Zeileis, J. Stat. Softw., 2004, 11, 1–20 Search PubMed.
- J. Jaworska, N. Nikolova-Jeliazkova and T. Aldenberg, Altern. Lab. Anim., 2005, 33, 445–459 CAS.
- A. V. Zakharov, M. L. Peach, M. Sitzmann and M. C. Nicklaus, J. Chem. Inf. Model., 2014, 54, 705–712 CrossRef CAS PubMed.
Footnotes |
† The authors declare no competing interests. |
‡ Electronic supplementary information (ESI) available: Structures of the training and test set ligands, binary activity data for the ligands, and superimposed and cleaned structures of receptors. See DOI: 10.1039/c5md00556f |
|
This journal is © The Royal Society of Chemistry 2016 |
Click here to see how this site uses Cookies. View our privacy policy here.