DOI:
10.1039/C5MB00460H
(Paper)
Mol. BioSyst., 2016,
12, 233-245
Identification of key active constituents of Buchang Naoxintong capsules with therapeutic effects against ischemic stroke by using an integrative pharmacology-based approach†
Received
10th July 2015
, Accepted 4th November 2015
First published on 6th November 2015
Abstract
Integrative pharmacology has been used to identify the key active constituents (KACs) of Buchang Naoxintong capsules (BNCs), a traditional Chinese medical preparation; this approach involves the evaluation of the content profiles and drug-like properties of the BNC constituents and development of an ingredient-target network. In this study, we used a sensitive analytical method to simultaneously identify and quantify 16 constituents of BNCs. Metabolism of these constituents by gut microbiota and human oral bioavailability were predicted using an in silico approach, followed by construction of networks to analyze the interactions between BNC constituents, their molecular targets, and proteins known to be the molecular targets for Food and Drug Administration-approved colitis medication. Finally, an animal model of ischemic stroke was used to verify the therapeutic effects of the KACs of BNCs. Amygdalin and paeoniflorin were identified as the KACs because they were the 2 most abundant BNC constituents, having appropriate drug-like properties, and produced therapeutic effects against cerebral ischemia. Amygdalin produced an anti-cerebral ischemia effect, likely by interacting with the glucocorticoid receptor (NR3C1) and serpin peptidase inhibitor, clade C (antithrombin), member 1 (SERPINC1). These results form the basis for conducting studies to identify KACs in traditional medicinal preparations; such studies might improve quality control and allow the in vivo evaluation of synergistic interactions between the complex mixtures of compounds.
Introduction
Traditional Chinese medicine (TCM) is one of the oldest medical systems in the world.1 Recently, the focus of the health care paradigm has shifted from disease to TCM therapy with a holistic approach. Some researchers utilize omics and systems biology techniques to elucidate the detailed molecular mechanisms underlying the effectiveness and promote the modernization of TCMs.2,3 At present, chemical analysis, in vivo drug metabolism evaluation, and pharmacological action elucidation are performed separately because of the complexity of TCM formulations. The lack of correlations among these studies has led to the formation of three disciplines (chemistry, pharmacokinetics and pharmacodynamics) in the “three parallel universes”; this has restricted the acceptance of TCMs within Western biomedical practices.4 This limitation can be addressed using integrative pharmacology and systems pharmacology, which allow the determination of the active constituents of TCM formulations and their molecular mechanisms by integrating the knowledge of chemical profiles, drug-like properties, and ingredient-targeted networks.5–7 High-throughput qualitative and quantitative analytical techniques, including ultra-high-pressure liquid chromatography (UHPLC) coupled with linear ion trap/Orbitrap (LTQ-Orbitrap) or a triple quadrupole electrospray tandem mass spectrometry (QQQ), are powerful tools for elucidating the chemical profiles of TCM formulations.8,9 Information on the chemical constituents of TCM ingredients is critical for the accurate assessments of the therapeutic effects of each constituent. However, studies simultaneously evaluating several TCM ingredients are limited by the lack of standard substances and appropriate analytical methods for evaluating multiple constituents. Furthermore, most TCM formulations are consumed orally. Therefore, TCMs need to undergo intestinal metabolism and absorption to enter the body and produce therapeutic actions.10,11 However, experimental determination of these processes can be costly and time consuming. For the rapid estimation of TCM absorption and metabolism in humans, high-throughput in silico screening methods involving the use of Caco-2 cell monolayers and P450 enzymes are considered the most advanced in vitro approaches for assessing new chemical entities.12,13 Systems biology and network pharmacology are valuable tools for better understanding of the molecular mechanisms underlying the therapeutic effects of TCM formulations;14–16 however, their results need to be experimentally validated.
Cardiovascular diseases (CVDs) are the most common cause of death worldwide, especially in developing countries.17 The Buchang Naoxintong capsule (BNC) is one of the TCM prescriptions (BuYangHuanWuTang) and is used to treat CVDs in China. Every BNC weighs 0.4 g and consists of 16 TCMs: Astragalus membranaceus (16.2%), Salvia miltiorrhiza (6.6%), chuanxiong hort (6.6%), Radix paeoniae rubra (6.6%), Szechwan lovage rhizome (6.6%), Semen persicae (6.6%), Carthamus tinctorius L. (3.2%), frankincense (3.2%), myrrh (3.2%), Spatholobus suberectus Dunn (4.9%), Achyranthes bidentate (6.6%), cassia twig (6.6%), Morus alba L. (6.6%), Pheretima hupeiensis (6.6%), Buthus martensii (3.2%), and Whitmania pigra Whitman (6.6%).18 The standardized procedure for the preparation of this TCM is as follows: the 16 TCMs are weighted precisely, and then the two herbs (Pheretima hupeiensis and Scorpionidae) and the remaining 14 ingredients are separately mixed and ground into fine powders. The powders are then mixed and filled in the capsules. In our previous chemical analysis, the UHPLC-LTQ-Orbitrap method was used as a rapid and high-throughput identification or tentative characterization method that allowed the simultaneous analysis of a total of 178 components, including 21 flavones, 6 flavone glycosides, 18 phenanthraquinones, and 22 terpenoids, from the BNCs.19 Furthermore, the UPLC-DAD method was used to quantify 5 constituents of BNCs and obtain the intestinal absorption profiles of 4 of these constituents.20,21
BNCs produce various pharmacological actions, including lipid concentration reduction, anti-platelet effect enhancement, dendritic cell maturation inhibition, and drug-metabolizing enzyme CYP2C19 activity enhancement.22–24 However, the key active constituents (KACs) of BNCs that are beneficial in the cases of CVDs and their molecular mechanisms of action are still unclear. In particular, since the ingredient-target network is not known, the molecular mechanisms of BNC action in CVDs are not yet known. Therefore, in the present study, the integrative pharmacology approach was applied to rapidly identify KACs of BNCs and their potential molecular mechanisms in ischemic stroke; quantitative analysis of multi-constituents, absorption, distribution, metabolism, and excretion (ADME) prediction, network analysis, and experimental validation were also performed. The workflow is shown in Fig. 1.
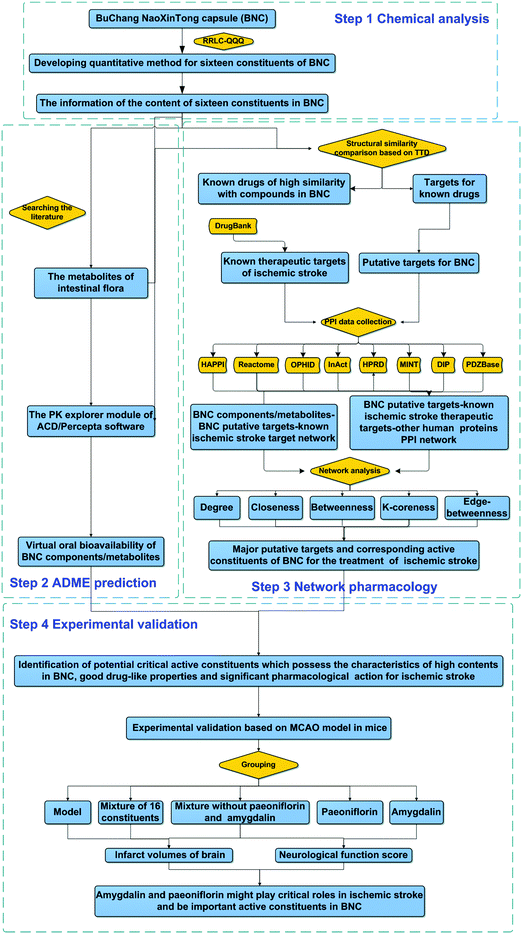 |
| Fig. 1 Schematic diagram of the analytical approach. Schematic diagram of the analytical approach used to identify the critical active constituents of Buchang Naoxintong capsules with therapeutic effects against ischemic stroke by integrating quantitative analysis, pharmacokinetic prediction, network analysis, and experimental validation. | |
Results and discussion
Selection of candidate constituents for quantitative analysis and optimization of sample preparation
Because of the lack of standards and appropriate quantitative methods, contents of only 16 of the 178 BNC components qualitatively identified in our previous study19 (gallic acid, danshensu, hydroxysafflor yellow A, chlorogenic acid, amygdalin, protocatechuic aldehyde, (−)-epicatechin, caffeic acid, albiflorin, ononin, paeoniflorin, rutin, salvianolic acid A, cinnamic acid, formononetin, and dihydrotanshinone I) were determined using the RRLC-QQQ method in this study; the structures of these components are shown in Fig. 2. The best quantitative results were obtained by using variables such as the solvent, procedure, and extraction time.
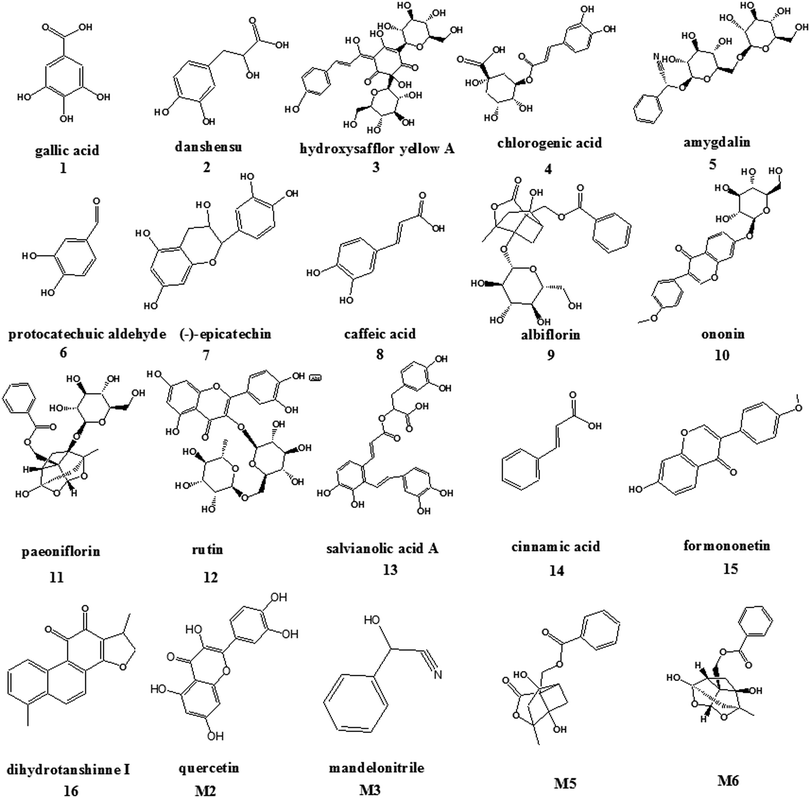 |
| Fig. 2 Chemical structures of the 16 constituents and 4 metabolites of Buchang Naoxintong capsules. | |
Ultrasonic extraction was found to be more convenient and effective than refluxing and Soxhlet extraction because of its simpler extraction process, lower extraction temperature, and higher extraction recoveries of the 16 constituents. In a preliminary experiment, different extraction solvents such as methanol, ethyl acetate, and methylene dichloride were investigated, and methanol showed the best extraction efficiency. Thus, the main parameters that independently influenced the ultrasonic-assisted extraction of the constituent-enriched BNC extract were investigated for the optimum methanol concentration (100%, 75%, and 50%), solvent volumes (25, 50, and 100 mL), and extraction times (30, 40, 50, and 60 min). The results suggested that the compounds of interest were completely extracted from the BNC samples within 40 min when 50 mL of 100% methanol was used.
Optimization of RRLC-QQQ conditions
Good chromatographic behavior and satisfactory MS response were obtained by systematically optimizing the RRLC-QQQ conditions. Liquid chromatographic conditions, including the stationary phase, mobile phase, column temperature, and flow rate, were investigated. The results indicated that the Eclipse XDB-C18 column (Agilent Technologies, Palo Alto, CA) with a small (1.8 μm) particle size showed better separation efficiency in a shorter time, and the aqueous phase with 0.1% formic acid (A)–acetonitrile (B) was better than the other mobile phases. Furthermore, 0.1% formic acid could eliminate the peak tailing of the target compounds. The optimal gradient elution procedure (described in the Methods section) was determined by comparing different procedures. In particular, the organic phase proportion decreased to the initial ratios (15% B) after 8.1 min and could continue to elute the residual target constituents and balance the chromatographic column such that better separation within shorter analytical time could be obtained.
The MS conditions were also optimized systemically. The richest relative abundance of precursor and product ions were obtained by optimizing the parameters for fragmentor energy and collision energy to establish multiple reaction monitoring (MRM) transitions for all target compounds. The representative MRM chromatograms and total ion chromatograms of the 16 tested BNC constituents are shown in Table 1 and Fig. S1 (ESI†), respectively.
Table 1 Retention time (RT) and related mass spectrometry (MS) data of the 16 target compounds detected using rapid resolution liquid chromatography (RRLC)-MS
Maker |
RT (min) |
Ionization mode |
Quasi-molecular ions |
Quantitative ion (m/z) |
Fragmentor (V) |
CE (V) |
Electrospray ionization (ESI)+ and ESI− represent the positive and negative modes of ESI, respectively. |
1 |
3.33 |
ESI− |
169.0 |
125.1 |
100 |
10 |
2 |
3.72 |
ESI− |
197.1 |
135.0 |
100 |
10 |
3 |
4.33 |
ESI− |
611.1 |
325.0 |
250 |
30 |
4 |
5.32 |
ESI− |
353.0 |
191.0 |
100 |
15 |
5 |
6.10 |
ESI− |
502.2 |
323.2 |
100 |
10 |
6 |
6.99 |
ESI− |
137.3 |
108.2 |
100 |
25 |
7 |
7.52 |
ESI− |
289.1 |
245.0 |
100 |
5 |
8 |
7.87 |
ESI− |
179.0 |
135.0 |
100 |
10 |
9 |
8.02 |
ESI− |
525.1 |
121.2 |
150 |
20 |
10 |
8.58 |
ESI− |
475.1 |
267.2 |
100 |
5 |
11 |
8.59 |
ESI− |
525.0 |
449.0 |
100 |
5 |
12 |
8.71 |
ESI− |
609.0 |
300.0 |
200 |
40 |
13 |
8.76 |
ESI− |
493.1 |
295.0 |
150 |
15 |
14 |
9.34 |
ESI− |
147.0 |
103.0 |
100 |
5 |
15 |
9.56 |
ESI− |
267.1 |
252.0 |
100 |
15 |
16 |
11.52 |
ESI+ |
279.0 |
261.0 |
150 |
15 |
Method validation and quantification
In this study, the calibration curves of the 16 compounds showed good linearity (R2 ≥ 0.9933). The LODs were in the range of 0.14–5.62 ng mL−1, whereas the LOQs ranged from 0.48 to 18.72 μg mL−1 (Table S1, ESI†). The relative standard deviation values (%RSD) of the 16 BNC constituents were 0.44–4.14% for intra-day precision and 2.19–4.86% for inter-day precision. Furthermore, the method showed good repeatability, with RSDs for the 16 compounds ranging from 0.61% to 4.31%. The RSD values of the 16 compounds were less than 5%, indicating good stability of the methanol solution within the experimental period (Table S2, ESI†). The mean recoveries of the 16 compounds ranged from 92.8% to 104.3% (RSD, ≤4.94%; Table S3, ESI†).
The established RRLC-QQQ method was successfully applied to quantitatively and simultaneously analyze the 16 compounds of BNCs in 15 batches. The levels of the 16 compounds were determined in triplicate by using calibration curves generated using external standards (Table 2). The results indicated that the contents of the 16 compounds varied considerably, ranging from 4.20% to 1938.42 μg g−1. Amygdalin and paeoniflorin were the most abundant compounds, and their concentrations ranged 1955.11–2323.14 μg g−1 and 1430.18–1938.42 μg g−1, respectively. Among the other analytes, gallic acid, danshensu, hydroxysafflor yellow A, chlorogenic acid, and (−)-epicatechin were identified as the primary active compounds in the BNCs; they were present at a concentration of more than 100 μg g−1. Certain trace constituents, including protocatechuic aldehyde, caffeic acid, rutin, and formononetin, were also quantified. The RSDs of the determination of the 16 compounds ranged from 6.56% to 18.02% in the 15 batches of BNC analysis, suggesting that the chemical profiles of the BNCs were stable across the different batches of the product, probably because of the similar plant origins, growth locations, and manufacturing techniques.
Table 2 Content of each compound in the 15 batches of the Naoxintong capsule sample (μg g−1) and their oral bioavailability (F%)
Sample |
Content of each compound in 15 batches of YZT sample (μg g−1) and oral bioavailability of 16 constituents |
1
|
2
|
3
|
4
|
5
|
6
|
7
|
8
|
9
|
10
|
11
|
12
|
13
|
14
|
15
|
16
|
OB: oral bioavailability. S1–S15: the sample numbers of the BNC capsules obtained from Buchang Pharmaceutical Co. Ltd; compounds 1–16 represent the following 16 markers: 1, gallic acid; 2, danshensu; 3, hydroxysafflor yellow A; 4, chlorogenic acid; 5, amygdalin; 6, protocatechuic aldehyde; 7, (−)-epicatechin; 8, caffeic acid; 9, albiflorin; 10, ononin; 11, paeoniflorin; 12, rutin; 13, salvianolic acid A; 14, cinnamic acid; 15, formononetin; 16, dihydrotanshinone I. |
S1 |
425.36 |
110.69 |
391.14 |
162.80 |
2218.51 |
13.56 |
101.64 |
10.89 |
65.92 |
7.57 |
1647.68 |
12.60 |
56.57 |
27.42 |
16.80 |
14.27 |
S2 |
431.23 |
115.48 |
343.63 |
130.27 |
2105.23 |
12.20 |
109.01 |
9.42 |
62.67 |
7.46 |
1628.94 |
10.01 |
45.65 |
20.61 |
14.79 |
11.69 |
S3 |
450.97 |
110.19 |
355.74 |
153.53 |
2155.17 |
14.67 |
103.52 |
11.19 |
69.94 |
7.79 |
1683.80 |
10.28 |
44.32 |
25.64 |
13.48 |
13.53 |
S4 |
371.60 |
127.43 |
403.99 |
163.81 |
2128.13 |
12.16 |
102.33 |
11.36 |
79.66 |
6.20 |
1703.77 |
10.46 |
52.42 |
22.62 |
12.99 |
13.94 |
S5 |
490.53 |
130.27 |
401.60 |
169.48 |
2311.84 |
15.98 |
112.80 |
10.23 |
74.18 |
7.39 |
1820.96 |
10.55 |
52.09 |
21.92 |
14.22 |
13.56 |
S6 |
410.82 |
152.25 |
279.38 |
153.84 |
1972.93 |
13.91 |
88.01 |
10.11 |
73.79 |
5.81 |
1597.93 |
9.55 |
59.36 |
30.67 |
14.83 |
14.36 |
S7 |
406.37 |
155.30 |
294.16 |
141.88 |
2440.25 |
13.88 |
96.62 |
9.80 |
74.41 |
4.20 |
1651.85 |
10.20 |
61.87 |
36.88 |
14.51 |
13.06 |
S8 |
397.81 |
144.65 |
378.31 |
180.23 |
2312.88 |
15.05 |
101.81 |
10.93 |
80.06 |
6.03 |
1744.54 |
10.13 |
55.31 |
29.45 |
15.50 |
12.50 |
S9 |
328.01 |
136.76 |
357.14 |
186.74 |
1955.11 |
11.21 |
103.18 |
10.48 |
80.81 |
7.20 |
1732.12 |
11.65 |
49.21 |
19.23 |
13.17 |
14.39 |
S10 |
379.67 |
126.60 |
417.21 |
198.17 |
2245.75 |
12.35 |
113.59 |
11.77 |
90.91 |
7.11 |
1927.47 |
12.57 |
51.17 |
21.59 |
14.68 |
15.62 |
S11 |
384.99 |
140.39 |
459.21 |
185.53 |
2323.14 |
15.25 |
116.32 |
13.06 |
90.54 |
6.25 |
1938.42 |
11.25 |
56.15 |
24.86 |
13.88 |
15.73 |
S12 |
351.56 |
125.50 |
391.43 |
176.97 |
2108.98 |
10.85 |
104.90 |
10.94 |
79.31 |
5.84 |
1751.82 |
9.94 |
49.48 |
24.64 |
13.16 |
14.50 |
S13 |
415.92 |
108.43 |
244.88 |
96.89 |
2031.28 |
10.50 |
87.05 |
7.30 |
54.66 |
7.02 |
1430.18 |
8.31 |
43.22 |
24.57 |
13.47 |
10.67 |
S14 |
363.75 |
128.43 |
286.93 |
147.93 |
2315.52 |
14.20 |
130.66 |
10.43 |
43.82 |
4.63 |
1431.05 |
7.59 |
51.42 |
25.75 |
14.62 |
15.94 |
S15 |
363.79 |
136.98 |
376.37 |
159.02 |
2078.71 |
11.69 |
94.37 |
10.21 |
77.95 |
6.78 |
1696.92 |
11.49 |
53.24 |
21.93 |
12.94 |
12.06 |
Mean ± SD |
398.1 ± 41.7 |
129.96 ± 14.7 |
358.74 ± 59.2 |
160.47 ± 25.4 |
2180.23 ± 143.2 |
13.16 ± 1.7 |
104.39 ± 11.2 |
10.54 ± 1.2 |
73.24 ± 12.6 |
6.48 ± 1.1 |
1692.50 ± 144.9 |
10.44 ± 1.4 |
52.10 ± 5.3 |
25.18 ± 4.5 |
14.20 ± 1.1 |
13.72 ± 1.5 |
RSD (%) |
10.48 |
11.32 |
16.49 |
15.84 |
6.56 |
13.07 |
10.74 |
11.92 |
17.13 |
16.38 |
8.56 |
13.18 |
10.02 |
18.02 |
7.54 |
11.11 |
|
OB (%F) |
7.7 |
3.2 |
0.006 |
0.5 |
1.6 |
99.9 |
71.6 |
41.1 |
13.9 |
79.6 |
44.7 |
0.1 |
6.7 |
99.1 |
87.2 |
5.5 |
Reports of the biotransformation of the primary BNC constituents by gut microbiota from the literature and in silico prediction of oral bioavailability
Most TCMs are orally administered as decoctions and might be transformed by intestinal bacteria before absorption in the gastrointestinal tract.25 Therefore, intestinal flora plays an important role in the metabolism of TCM compounds.26,27 Recently, metabolic studies of human intestinal flora have attracted wide attention and provided new insights into the therapeutic mechanisms of TCMs.28 A literature search was conducted using electronic databases, including Medline (Ovid), PubMed, CNKI, Wanfang, and Weipu, to obtain the data regarding the biotransformation of the six quantified BNC constituents (chlorogenic acid, rutin, amygdalin, ononin, albiflorin and paeoniflorin) by gut microbiota. The metabolic pathways of several BNC constituents are summarized in Table 3, and the structures of these constituents are shown in Fig. 2. Following deglycosylation, amygdalin, rutin, ononin, and chlorogenic acid were metabolized to mandelonitrile, quercetin, formononetin, and caffeic acid, respectively, and albiflorin and paeoniflorin were converted to metabolites 5 (M5) and 6 (M6), respectively, by the gut microbiota; deglycosylation is considered a critical step in the absorption and metabolism of dietary flavonoid glycosides in humans.29–37
Table 3 Biotransformation of the six constituents in the gastrointestinal tract identified by comparison with literature searches
Prototype |
Metabolite(s) |
OB(F%) |
Reference(s) |
OB: oral bioavailability. |
Chlorogenic acid |
Caffeic acid, |
40.5 |
28–30
|
Rutin |
Quercetin |
94.3 |
31 and 32
|
Amygdalin |
Mandelonitrile |
99.4 |
33
|
Ononin |
Formononetin |
87.2 |
34
|
Albiflorin |
Metabolites 5 (M5) |
99.9 |
35 and 36
|
After the TCM components are metabolized by the gastrointestinal tract, they are absorbed into the body. Therefore, their oral bioavailability, one of the most important pharmacokinetic parameters, was measured to determine the oral dose of each compound that was sufficient to produce a pharmacological effect. High oral bioavailability suggests that the bioactive molecules have the potential to be used as therapeutic agents.38 Recently, numerous studies have been conducted to develop in silico quantitative models for predicting oral bioavailability.39 The module for determining oral bioavailability involves a combination of probabilistic and mechanistic models to produce quantitative predictions of bioavailability in humans after oral administration (%F); these modules are widely used in research and drug development.40,41 In this study, the pharmacokinetics (PK) explorer module included in the commercially available ACD/Percepta software was used to calculate the absorbed fraction and to predict the oral bioavailability of 16 quantified BNC constituents and 6 metabolites. In all, 7 BNC constituents, including protocatechuic aldehyde, (−)-epicatechin, caffeic acid, ononin, paeoniflorin, cinnamic acid, and formononetin, showed good oral bioavailability (F%, ≥ 40; Tables 2 and 3). Some components, including chlorogenic acid, rutin, amygdalin, and albiflorin, were converted to metabolites by the gut microbiota, which improved their oral bioavailability. Thus, paeoniflorin and its metabolite (M6) had good oral bioavailability.
Identification of KACs of BNCs for the treatment of ischemic stroke
Numerous KACs generally exist in the TCM product; they have the appropriate pharmacokinetic properties and possess therapeutic activity.42 Therefore, the selection of 16 BNC constituents and determination of their contents in different batches of BNCs was a precondition for the evaluation of BNC KACs. In silico prediction of the molecular targets for the 16 BNC constituents and construction and analysis of the constituent-target-disease network is a cost-effective method of predicting the KACs of TCM formulations such as the BNC.
Putative targets for the 16 BNC constituents.
Based on our previously developed target prediction system,43 we identified 80 known drugs with a similar chemical structure as those of the BNC constituents/metabolites and their known targets (n = 227); they were considered as the putative targets of BNC constituents/metabolites. Their details are shown in Table S4 (ESI†). Of the 227 putative targets of BNC constituents, 18 (7.93%; Table S5, ESI†) were known therapeutic targets for the treatment of ischemic stroke. Notably, 14 putative targets of mandelonitrile were known therapeutic targets for the treatment of ischemic stroke, suggesting that M1 played an important role in the therapeutic effect of BNCs.
Network construction and analysis.
The bioactive BNC constituents responsible for the therapeutic effect in ischemic stroke patients were identified by first constructing a BNC component/metabolite-putative target-known ischemic stroke target network (Fig. 3 and Table S6, ESI†). The putative targets of gallic acid (constituent ID: 1), danshensu (2), chlorogenic acid (4), protocatechuic aldehyde (6), (−)-epicatechin (7), caffeic acid (8), paeoniflorin (11), mandelonitrile (M1), and quercetin (M3) directly interacted with known therapeutic targets for the treatment of ischemic stroke.
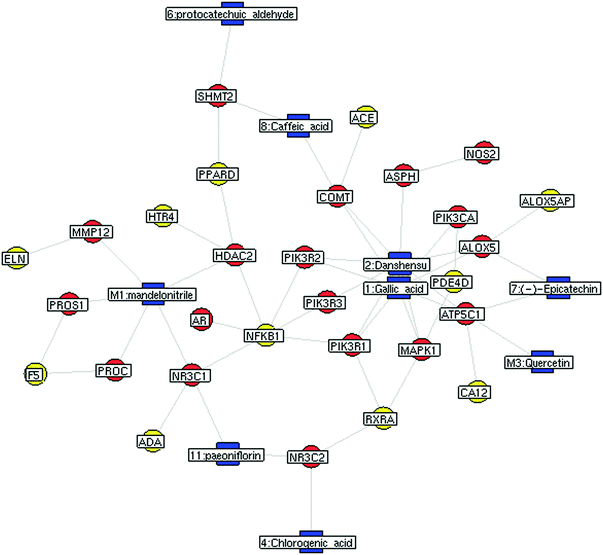 |
| Fig. 3 Network of interactions between Buchang Naoxintong capsule (BNC) constituents and their predicted targets. Interactions between predicted targets and known targets of drugs used to treat ischemic stroke patients were obtained from the DrugBank. The network consists of 38 nodes and 51 edges. Blue nodes represent BNC constituents; red nodes, predicted targets of BNC constituents; yellow nodes, known targets of drugs used to treat ischemic stroke patients obtained from the DrugBank. | |
Next, a PPI network consisting of putative targets of BNC constituents, known therapeutic targets for ischemic stroke, and other human proteins was constructed to identify the bioactive BNC constituents responsible for the therapeutic effect on ischemic stroke (Fig. 4 and Table S7, ESI†). The PPI network consisted of 184 nodes and 423 edges. Four topological features (degree, betweenness, closeness, and k-value) were calculated to screen the major putative targets. In all, 47 major nodes with a higher degree, betweenness, closeness, and k-values than the corresponding median values were identified. Among them, 37 were recognized as the major putative targets of BNC constituents. Notably, the glucocorticoid receptor (NR3C1)—a major putative target of paeoniflorin and mandelonitrile—and serpin peptidase inhibitor, clade C (antithrombin), member 1 (SERPINC1)—a major putative target of amygdalin—had the highest betweenness values, suggesting their large influence on the transfer of information through the network, under the assumption that the information transfer follows the shortest paths. Detailed information on the topological features of the 47 major nodes in the PPI network is shown in Table S8 (ESI†). Detailed information on the 37 major putative targets of BNC constituents is shown in Table S9 (ESI†).
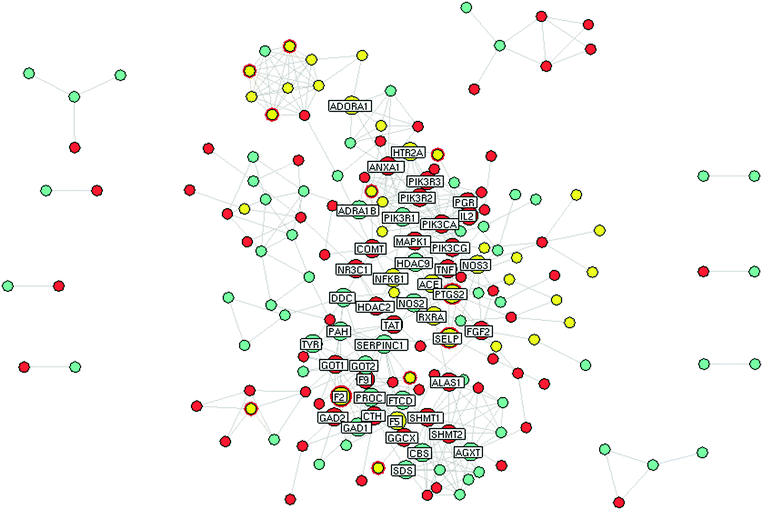 |
| Fig. 4 Network of interactions between predicted targets of Buchang Naoxintong capsule (BNC) constituents, known targets of drugs used to treat ischemic stroke patients obtained from the DrugBank, and other human genes. The network consists of 184 nodes and 423 edges. Red nodes represent predicted targets of BNC constituents; yellow nodes, targets of drugs used to treat ischemic stroke patients obtained from DrugBank; green nodes, other human genes; yellow nodes with red rings, known targets of drugs used to treat ischemic stroke patients that are also predicted targets of BNC constituents. Nodes with gene names refer to key nodes with topological importance according to their degree, closeness, betweenness, and k-value. | |
Furthermore, the edge-betweenness values of each interaction in the BNC putative target/known ischemic stroke therapeutic target/other human protein PPI network were calculated. The NR3C1-TAT (tyrosine aminotransferase), NR3C1-HSD17B6, and SERPINC1-FTCD (formimidoyltransferase-cyclodeaminase) interactions had the three highest edge-betweenness values, suggesting their crucial roles in connecting the other edges in the network (Table 4).
Table 4 Top 10 edges with the highest edge-betweenness value
Node A |
Node B |
Edge-betweenness |
NR3C1 |
TAT |
1332.089 |
NR3C1 |
HSD17B6 |
1036 |
SERPINC1 |
FTCD |
966.4062 |
SLC6A3 |
COMT |
951.6876 |
ANXA1 |
MAPK1 |
900.9778 |
DRD5 |
SLC6A3 |
867.4568 |
RXRA |
ALAS1 |
835.7336 |
TAT |
PTGS2 |
744.5632 |
DDC |
COMT |
736.5258 |
RXRA |
MAPK1 |
715.9232 |
Our results suggested that amygdalin and paeoniflorin might be important bioactive constituents of BNC according to the following observations. First, amygdalin and paeoniflorin were the most abundant BNC constituents, with concentrations greater than 1400 μg g−1 in all the tested samples. Second, in silico pharmacokinetics prediction indicated that paeoniflorin had good drug-like properties, including an oral bioavailability of 44.7%. Although amygdalin had poor absorption and low oral bioavailability, it was easily deglycosylated and converted to mandelonitrile, which had a very good oral bioavailability (F = 99.4%). Third, amygdalin and paeoniflorin, or their metabolites had many putative targets that directly interacted with other known therapeutic targets for ischemic stroke. Finally, NR3C1 and SERPINC1, respectively identified as the putative targets of amygdalin and paeoniflorin, or their metabolites, had the most important topological features in the network.
Experimental validation of the active BNC constituents in a model of ischemic stroke
In rats and mice, the middle cerebral artery occlusion (MCAO) model has been used to represent cerebrovascular stroke; BNC was found to increase the number of masculine nerve cells of calcitonin gene-related peptide (CGRP) and improve learning and memory capacity in this animal model.44,45 In this study, the predicted outcomes on ischemic stroke after treatment with the primary active BNC constituents were investigated by using the MCAO model (Fig. 5a and b). The sham group did not show any infarction volumes, and their neurobehavior was completely normal. In contrast, the control group showed excessive infarction volumes, and their neuronal functions were obviously abnormal, but the positive group (GBE) showed a significant curative effect after treatment with BNC components. The optimal dose was determined by administering the 16 BNC constituents at three concentrations: high (High_16-MS), medium (Medium_16-MS), and low concentrations (Low_16-MS). The Medium_16-MS group showed an infarction rate of 10.50 ± 12.87 and a neurological behavior score of 1.58 ± 0.79, indicating that this concentration produced the best curative effect.
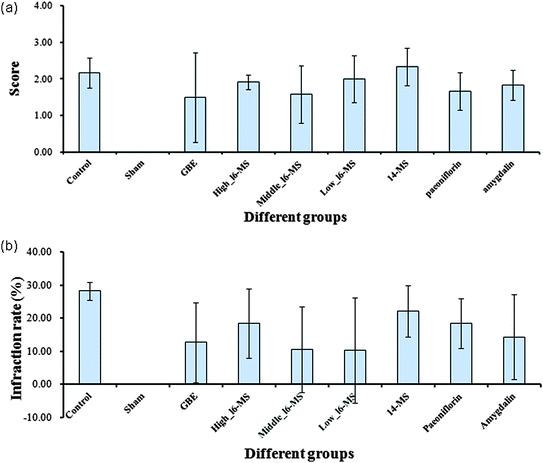 |
| Fig. 5 Evaluation of the effects of different mixtures/active Buchang Naoxintong capsule (BNC) constituents in the middle cerebral artery occlusion (MCAO) model. (a) Infarction rates; (b) neurological deficit scores. High_16-MS, high dose of the mixture of the 16 standards; medium_16-MS, medium dose of the mixture of the 16 standards; low_16-MS, low dose of the mixture of the 16 standards; 14-MS, the mixture of 14 standards excluding amygdalin and paeoniflorin; and GBE, Ginkgo biloba extract. | |
Next, the bioactivities of the primary active BNC constituents (amygdalin and paeoniflorin) and the mixture of the 14 BNC constituent standards (14-MS, excluding amygdalin and paeoniflorin) were determined at doses equivalent to those of the medium-dose 16-MS. The infarction rates and neurological deficit scores of the groups treated with amygdalin were 14.32 ± 12.80% and 1.83 ± 0.41, respectively, and those of the groups treated with paeoniflorin were 18.36 ± 7.50% and 1.67 ± 0.52, respectively. These results suggested that amygdalin and paeoniflorin significantly decreased the infarction volume (p < 0.05) and improved neurological scores compared with those of the control group. However, 14-MS had very low bioactivity in MCAO rats and showed small effects on the infarction volume and neurological function. These findings indicated that amygdalin and paeoniflorin are the KACs of BNCs that play critical roles in the therapeutic effects in ischemic stroke patients. Previous studies showed that paeoniflorin produces a delayed protective effect in ischemia-injured rats by inhibiting MAPK/NF-κB-mediated peripheral and cerebral inflammatory responses, corroborating our network pharmacology results.46,47 However, to our knowledge, this is the first report of the anti-cerebral ischemia effect of amygdalin, which needs to be investigated further for elucidating its underlying molecular mechanism.
Conclusions
Identification of the KACs in TCM formulations is critical for ensuring their efficacy, safety, and quality. In this study, an integrative pharmacology approach involving a combination of quantitative analysis, pharmacokinetics prediction, network pharmacology, and experimental validation was used to identify KACs and the molecular mechanisms involved in the therapeutic effects of BNCs in ischemic stroke patients. First, a RRLC-QQQ technique was used to simultaneously quantify 16 constituents from 15 commercial samples of BNCs. Next, an in silico pharmacokinetics model was applied to predict the oral bioavailability of the tested BNC constituents and 6 plausible metabolites produced by the intestinal flora, which suggested that the BNC constituents had good oral bioavailability. In addition, pharmacological networks were constructed to analyze and visualize the synergistic interactions among the BNC constituents/metabolites, putative BNC targets, and known therapeutic targets of drugs used to treat ischemic stroke patients. Amygdalin and paeoniflorin were identified as KACs of BNCs based on their high abundance in the product, appropriate drug-like properties, and importance to the bioactivity of the mixture of BNC constituents. Finally, the MCAO model was used to show that amygdalin and paeoniflorin significantly decreased the infarction volume and improved neurological scores in ischemic rats. To our knowledge, this is the first report of the anti-cerebral ischemia effect of amygdalin and the involvement of NR3C1 and SERPINC1 in its therapeutic effects. Future studies are warranted to determine the molecular mechanisms underlying the therapeutic effects of BNC constituents, with a focus on compound-target interactions and the synergistic interactions of active constituents at the systemic level.
Materials and methods
Ethics statement
All animal studies were approved by the committee of ethical regulations of the Laboratory Animal Ethics Committee of the Institute of Basic Theory of TCM, China Academy of Chinese Medical Sciences. Animal care and welfare and experimental procedures were complied with the Guide for the Care and Use of Laboratory Animals (National Research Council of the USA, 1996) and related ethical regulations of the China Academy of Chinese Medical Sciences. The approval code of the Ethics Committee was SYXK (Jing) 2005–2008, and the period of validity was from August 2011 to August 2015. All efforts were made to minimize the number of animals used and their suffering.
Reagents and materials
HPLC-grade acetonitrile and formic acid were obtained from Fisher Scientific Company (Thermo Fisher, USA). Analytical-grade formic acid was purchased from Sinopharm Chemical Regent Beijing Co., Ltd (Beijing, China). Purified water was purchased from the Wahaha Company (Hangzhou, China). Reference standards of hydroxysafflor yellow A, chlorogenic acid, amygdalin, protocatechuic aldehyde, L-epicatechin, caffeic acid, albiflorin, paeoniflorin, ononin, rutin, cinnamic acid, and formononetin were purchased from the National Institute for the Control of Pharmaceutical and Biological Products (Beijing, China). Gallic acid, tanshinol, salvianolic acid A, and dihydrotanshinone I were obtained from Chengdu Must Bio-technology Co., Ltd (Chengdu, China). The purities of all the standards were at least 98% and suitable for quantitative analysis. The Ginkgo biloba extract (GBE), trade name Jinnaduo capsule, was purchased from Dr Willmar Schwabe, GmbH & Co. KG (Batch No. H20140768; Germany). Fifteen commercial samples of Buchang Naoxintong capsules (BNCs) were obtained from Buchang Pharmaceutical Co. Ltd (Xian, China). Detailed information regarding the BNCs is provided in Table 5.
Table 5 A summary of the tested samples of BNC capsules from Buchang Pharmaceutical Co. Ltd
Sample no. |
Batch no. |
Production date |
S1 |
131117 |
October 06, 2013 |
S2 |
131118 |
October 06, 2013 |
S3 |
131120 |
October 07, 2013 |
S4 |
131121 |
October 07, 2013 |
S5 |
131122 |
October 07, 2013 |
S6 |
131125 |
October 08, 2013 |
S7 |
131126 |
October 08, 2013 |
S8 |
131127 |
October 08, 2013 |
S9 |
131129 |
October 09, 2013 |
S10 |
131130 |
October 09, 2013 |
S11 |
131131 |
October 09, 2013 |
S12 |
131134 |
October 10, 2013 |
S13 |
131135 |
October 10, 2013 |
S14 |
131137 |
October 10, 2013 |
S15 |
131138 |
October 10, 2013 |
Samples and standard solution preparations
Preparation of standard solutions.
A stock solution containing the 16 standard components was weighed, dissolved in methanol, and diluted serially to provide standard solutions at a range of concentrations, which were used to establish calibration curves. A working solution of each standard was also prepared. All solutions were stored at 4 °C.
Preparation of sample solutions.
The capsule shell was removed, and the BNC powder (0.5 g) was weighed precisely. The samples were ultrasonically extracted in 50 mL methanol at room temperature for 40 min, settled to a volume of 50 mL, and filtered through 0.22 μm nylon membrane filters. The filtrates were analyzed immediately.
Chromatographic and mass spectrometric conditions
Rapid resolution liquid chromatography (RRLC) was performed using an Agilent 1200 Rapid Resolution Liquid Chromatograph (Agilent, MA, USA) equipped with an online vacuum degasser, a binary pump, an autosampler, and a thermostatted column compartment. The analytes were separated on a ZORBAX Eclipse XDB-C18 column (4.6 mm × 50 mm, 1.8 μm) at 25 °C with a mobile phase flow rate of 0.20 mL min−1. The linear gradient conditions for solvent A (0.1% aqueous formic acid) and solvent B (acetonitrile) were as follows: 0–4 min, 15–20% B; 4–5 min, 20–90% B; 5–8 min, 90% B; 8–8.1 min, 90–15% B; 8.1–15 min, 15% B. The injection volume for each analysis was 5.0 μL.
An Agilent G6410 Triple Quadrupole mass spectrometer equipped with an electrospray ion source (ESI; Agilent, MA, USA) was used to quantitatively analyze the 16 BNC components. ESI-MS spectra were acquired in both positive and negative ion modes. The drying gas temperature was maintained at 300 °C at a flow rate of 12 L min−1. The nebulizing gas (N2) pressure was set at 15 psi. The capillary voltage was 4000 V for negative and positive modes. The dwell time was 200 ms. Mass analyzers Q1 and Q3 operating at unit mass resolution were used for all MRM transitions.
Method validation
For each standard compound, linearity, the limit of detection (LOD), the limit of quantification (LOQ), repeatability, stability, precision, and accuracy were experimentally verified.
The linearity of the optimized analytical method was determined by plotting a series of concentrations of standard solutions containing at least 7 non-zero concentrations. Each calibration curve for each analyte was analyzed individually by fitting the area ratio response by using least-square weighted (1/x2) linear regression and excluding the point of origin. The LOD and LOQ, and the signal-to-noise ratio (S/N) of 3 and 10, respectively, were determined by serial dilution of each standard solution under the described conditions.
The precision of the optimized analytical method was validated by determining the intra- and inter-day variance. Intra-day precision was assessed by measuring a standard mixture solution consisting of 16 markers 6 times per day under the optimized conditions. Inter-day precision was evaluated twice per day on 3 consecutive days. Intra-day and inter-day precisions were calculated as relative standard deviation (RSD) and analyzed using one-way analysis of variance (ANOVA) with day as the grouping variable.
The repeatability of the optimized analytical method was tested by preparing and analyzing 6 independent working solutions of the same sample (S15, batch no. 131138). The stability of the sample solutions was tested in triplicate at room temperature every 6 h for 24 h.
In the recovery test, known amounts of the mixed standard solutions with 3 different concentration levels (high, medium, and low) were added to known amounts of BNC sample (S15, batch no. 131138); subsequently, the samples were extracted and analyzed using the optimized analytical method. The experiment was performed in triplicate for each concentration of standard solution. The average recoveries were estimated using the following equation:
Recovery (%) = [(detected amount − original amount)/spiked amount] × 100. |
Literature search for metabolites of intestinal flora and oral bioavailability prediction by using in silico pharmacokinetic models
The metabolites of the 16 BNC constituents produced by the intestinal flora were obtained by searching electronic databases, including PubMed (1950–2014), Medline (1950–2014), and CNKI (China Journals of Full-text database; 1989–2014). Structural information for each component was downloaded from ChemSpider (http://www.chemspider.com/) or drawn using Chemdraw 12.0 and saved in the “.mol” format. The oral bioavailability of the constituents and their metabolites was predicted in silico by using the PK explorer module of ACD/Percepta software 5.07 (ACD/Labs, Toronto, Canada).
Known therapeutic targets of drugs used to treat ischemic stroke
The known therapeutic targets of drugs used to treat ischemic stroke were obtained from 2 resources: the DrugBank database48 (http://www.drugbank.ca/, version: 3.0) and the Online Mendelian Inheritance in the Man (OMIM) database49 (http://www.omim.org/, last updated: October 31, 2013). Only drug–target interactions for drugs approved by the Food and Drug Administration (FDA) for treating ischemic stroke and human gene/protein targets were selected. In all, 70 therapeutic targets of drugs used to treat ischemic stroke were identified. The OMIM database was searched with the keyword “ischemic stroke”; the search yielded 72 therapeutic targets of drugs used to treat ischemic stroke (detailed information in Table S10, ESI†). After redundant entries were removed, 62 therapeutic targets of drugs used to treat ischemic stroke were considered in this study.
Protein–protein interaction data
Protein–protein interaction (PPI) data were imported from 8 existing PPI databases: Human Annotated and Predicted Protein Interaction Database (HAPPI),50 Reactome,51 Online Predicted Human Interaction Database (OPHID),52 InAct,53 Human Protein Reference Database (HPRD),54 Molecular Interaction Database (MINT),55 Database of Interacting Proteins (DIP),56 and PDZBase.57 Detailed information on the PPI databases is shown in Table S11 (ESI†).
Pharmacological mechanism analysis
Prediction of putative BNC targets.
As described in our previous study,58 the Drug Similarity Search tool in the Therapeutic Targets Database (TTD, http://xin.cz3.nus.edu.sg/group/cjttd/ttd.asp, version 4.3.02, released on Feb. 25, 2014) was used to identify drugs similar to the BNC via structural similarity comparisons. Only drugs with a high structural similarity score (>0.70, moderately similar to very similar) with the 16 BNC constituents and their metabolites in the gastrointestinal tract were selected. The therapeutic targets of the identified drugs were also included as putative BNC targets.
Network construction and analysis.
The 16 BNC components and their metabolites and putative targets, as well as known therapeutic targets for drugs used to treat ischemic stroke, were used to construct a BNC component/metabolite/putative target/known ischemic stroke target network and a BNC putative target/known ischemic stroke therapeutic target/other human protein PPI network. Navigator software (version 2.2.1) and Cytoscape (version 2.8.1) were utilized to visualize the networks.
BNC component/metabolite/putative target/known ischemic stroke target network.
This network was constructed by linking the 16 BNC components, their metabolites, and their putative targets that interacted with known therapeutic targets of drugs used to treat ischemic stroke.
BNC putative target/known ischemic stroke therapeutic target/other human protein PPI network.
This network was constructed by linking putative BNC targets, known ischemic stroke therapeutic targets, and other human proteins that directly interact with putative BNC targets and targets of ischemic stroke treatments. According to our previous study,59,60 5 topological features (degree, betweenness, closeness, k-value, and edge betweenness) were chosen to identify the major putative targets by selecting those having values of the 4 features higher than the corresponding median values.
Defining feature set.
The definitions of the 5 measures of topology for each node “i” are as follows. Degree was defined as the number of links to node i. Betweenness was defined as the number of edges running through node i. Closeness was defined as the inverse of the sum of the distances from node i to all other nodes; it can be regarded as a measure of the time required to spread sequentially information from node i to all other nodes. Degree, betweenness, and closeness centralities are correlated with a protein’s topological importance in a PPI network.61k-core analysis is an iterative process in which nodes are sequentially removed from a network, from the least-connected node to the most-connected node.62 The core of maximum order is defined as the main (highest) k-core in a network. A k-core sub-network of an original network can be generated by recursively deleting vertices with a degree less than k from the network, resulting in a series of sub-networks that gradually reveal the globally central region of the original network. The k-value is a measure of the centrality of node i. Edge betweenness is defined as the frequency at which an edge belongs to the shortest paths between all pairs of vertices in a network.63 The edges with the highest betweenness values are most likely to lie between sub-graphs.
The edge betweenness for edge e was defined as
| 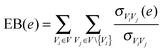 | (1) |
where
σViVj is the number of the shortest paths between nodes
Vi and
Vj in the network, and
σViVj(
e) is the number of the shortest paths between
Vi and
Vj.
Experimental validation
Animals and stroke models.
Wild-type healthy male C57BL/6 mice weighing 19–21 g (6- to 8-week old) were obtained from the Experimental Animal Center of the Peking University Health Science Center (Laboratory animal certificate: scxk 2012-0001; Beijing, China). Anesthesia was induced by 5% isoflurane and maintained using 1% to 2% isoflurane during surgery. In the middle cerebral artery occlusion (MCAO) model,40 focal cerebral ischemia was generated by occluding the left MCA by inserting an intraluminal 5–0 nylon monofilament suture through the common carotid artery to the branch point of the MCA for 6 h. The core body temperature was maintained at 36.5–37.2 °C throughout the experiments, and the arterial pO2, pCO2, and pH were maintained within normal ranges. For measuring acute infarct size, mice were euthanized with isoflurane 6 h after MCAO. Brains were collected and sectioned coronally at 1 mm intervals to produce 5 sections. Each section was stained with a solution of 0.5% 2,3,4-triphenytetrazolium-chloride (TTC). A computerized image analysis system (ImageJ, version 6.0) was used to measure the area of infarction in the inner section. Infarct volumes were calculated from the ischemic area and the thickness of each slice. The neurological function of each mouse was measured 6 h after left MCA occlusion according to the following 0–4 point graded scoring system: 0 = no deficit; 1 = forelimb weakness and torso turning to the ipsilateral side when held by the tail; 2 = circling to the affected side; 3 = falling in the direction contralateral to the infarct; and 4 = depressed level of consciousness without spontaneous movement, as described previously.64,65
Bioassay of the mixtures of 16 BNC constituents and 2 primary active BNC constituents.
The 16 BNC constituents were weighed and dissolved in a solution of 0.5% Tween-80 in water. The ratio of the 16 standards in the standard solution was based on the average relative content of each constituent in the 15 tested batches of BNCs. Before the experiment, the animals were weighed and randomly assigned to 9 groups (10 mice in each group). Group 1 (High_16-MS) was orally administered with the mixture of the 16 standards, which contained gallic acid (4.7 mg kg−1), danshensu (1.5 mg kg−1), hydroxysafflor yellow A (4.2 mg kg−1), chlorogenic acid (1.9 mg kg−1), amygdalin (25.8 mg kg−1), protocatechuic aldehyde (0.2 mg kg−1), (−)-epicatechin (1.2 mg kg−1), caffeic acid (0.1 mg kg−1), albiflorin (0.9 mg kg−1), ononin (0.1 mg kg−1), paeoniflorin (20.0 mg kg−1), rutin (0.1 mg kg−1), salvianolic acid A (0.6 mg kg−1), cinnamic acid (0.3 mg kg−1), formononetin (0.2 mg kg−1), and dihydrotanshinone I (0.2 mg kg−1). Group 2 (Medium_16-MS) was orally administered with the mixture of the 16 standards at 50% of the dose administered to group 1. Group 3 (low dose) was orally administered with the mixture of the 16 standards at 25% of the dose administered to group 1. Group 4, as a positive control group; mice in this group were orally administered with normal saline. Group 5, as a positive control group was orally administered with GBE at a dose of 71.5 mg kg−1. Group 6 was orally administered with a mixture of 14 standards (14-MS, excluding amygdalin and paeoniflorin) at the medium dose administered to group 2. Group 7 was orally administered with amygdalin at a dose of 12.9 mg kg−1. Group 8 was orally administered with paeoniflorin at a dose of 10.0 mg kg−1. Group 9 was the sham group.
Statistical analysis
All data of bioassay experiments are represented as mean ± standard deviation. Student's t-test was used when the analysis involved the comparison of two means, and a P value of less than 0.05 was considered significant. The data were analyzed using SPSS 16.0 software.
Conflict of interests
The authors declare that they have no conflict of interest.
Author contributions
Conceived and designed the experiments: HX, FL, HY. Performed the experiments: YZ, YS, QJ, DL, YZ. Written the manuscript: HX, YZ.
Acknowledgements
Financial support was provided by the National High Technology Research and Development Program of China (No. 2011YZX09201-201-26), the National Natural Science Foundation of China (NO. 81202793 and 81473414) and Operational expenses for basic research of China Academy of Chinese Medical Sciences (NO. Z02063).
References
- F. Cheung, Nature, 2011, 480, 82–83 CrossRef PubMed.
- A. Buriani, M. L. Garcia-Bermejo, E. Bosisio, Q. Xu, H. Li, X. Dong, M. S. Simmonds, M. Carrara, N. Tejedor, J. Lucio-Cazana and P. J. Hylands, J. Ethnopharmacol., 2012, 140, 535–544 CrossRef PubMed.
- T. Ma, C. Tan, H. Zhang, M. Wang, W. Ding and S. Li, Mol. BioSyst., 2010, 6, 613–619 RSC.
- P. S. Xie and A. Y. Leung, J. Chromatogr. A, 2009, 1216, 1933–1940 CrossRef CAS PubMed.
- H. Y. Xu and H. J. Yang, Zhongguo Zhongyao Zazhi, 2014, 39, 357–362 Search PubMed.
- H. Xu, Y. Zhang, Y. Lei, X. Gao, H. Zhai, N. Lin, S. Tang, R. Liang, Y. Ma, D. Li, Y. Zhang, G. Zhu, H. Yang and L. Huang, PLoS One, 2014, 9(7), e101432 Search PubMed.
- P. Li, J. Chen, J. Wang, W. Zhou, X. Wang, B. Li, W. Tao, W. Wang, Y. Wang and L. Yang, J. Ethnopharmacol., 2014, 151, 93–107 CrossRef CAS PubMed.
- H. Pan, W. Yang, Y. Zhang, M. Yang, R. Feng, W. Wu and D. Guo, Anal. Bioanal. Chem., 2015, 407, 6057–6070 CrossRef CAS PubMed.
- Y. Zhang, H. Xu, X. Chen, C. Chen, H. Wang, F. Meng, H. Yang and L. Huang, J. Pharm. Biomed. Anal., 2011, 56, 497–504 CrossRef CAS PubMed.
- H. Xu, K. Li, Y. Chen, Y. Zhang, S. Tang, S. Wang, D. Shen, X. Wang, Y. Lei, D. Li, Y. Zhang, L. Jin, H. Yang and L. Huang, PLoS One, 2013, 8, e81135 Search PubMed.
- P. Artursson and J. Karlsson, Biochem. Biophys. Res. Commun., 1991, 175(3), 880–885 CrossRef CAS PubMed.
- T. Geerts and Y. Vander Heyden, Comb. Chem. High Throughput Screening, 2011, 14, 339–361 CrossRef CAS PubMed.
- T. Hou and J. Wang, Expert Opin. Drug Metab. Toxicol., 2008, 4(6), 759–770 CrossRef CAS PubMed.
- Y. Li, J. Zhang, L. Zhang, X. Chen, Y. Pan, S. S. Chen, S. Zhang, Z. Wang, W. Xiao, L. Yang and Y. Wang, J. Ethnopharmacol., 2015, 30056–30058 Search PubMed.
- H. Li, L. Zhao, B. Zhang, Y. Jiang, X. Wang, Y. Guo, H. Liu, S. Li and X. Tong, J. Evidence-Based Complementary Altern. Med., 2014, 2014, 495840 Search PubMed.
- H. Xu, Y. Tao, P. Lu, P. Wang, F. Zhang, Y. Yuan, S. Wang, X. Xiao, H. Yang and L. Huang, J. Evidence-Based Complementary Altern. Med., 2013, 2013, 658531 Search PubMed.
- S. Mendis, Vasc. Health Risk Manage., 2005, 1, 15–18 CrossRef.
- H. Zhang, W. R. Wang, R. Lin, J. Y. Zhang, Q. L. Ji, Q. Q. Lin and L. N. Yang, J. Ethnopharmacol., 2010, 130, 98–102 CrossRef PubMed.
- S. S. Wang, H. Y. Xu, Y. Ma, X. G. Wang, Y. Shi, B. Huang, S. H. Tang, Y. Zhang, D. F. Li, R. X. Liang and H. J. Yang, J. Pharm. Biomed. Anal., 2015, 111, 104–118 CrossRef CAS PubMed.
- G. Li, F. Y. Meng, H. J. Yang, F. Liu and M. H. Fu, Chin. J. Pharm. Anal., 2013, 33, 15–16 Search PubMed.
- B. Huang, G. Li, Y. F. Guo, S. S. Wang, F. Liu, H. Y. Xu and H. J. Yang, Zhongguo Zhongyao Zazhi, 2013, 38, 889–893 CAS.
- J. Zhao, H. Zhu, S. Wang, X. Ma, X. Liu, C. Wang, H. Zhao, S. Fan, X. Jin, B. Zhao, T. Zhao, L. Jia, K. Wang, Y. Zou, K. Hu, A. Sun and J. Ge, Curr. Pharm. Des., 2013, 19, 5891–5896 CrossRef CAS PubMed.
- H. Chen, Y. Zhang, X. Wu, C. Li and H. Wang, J. Evidence-Based Complementary Altern. Med., 2012, 2012, 430262 Search PubMed.
- F. Zhang, B. Huang, Y. Zhao, S. Tang, H. Xu, L. Wang, R. Liang and H. Yang, J. Evidence-Based Complementary Altern. Med., 2013, 2013, 802784 Search PubMed.
- F. Zuo, Z. M. Zhou, M. Z. Yan, M. L. Liu, Y. L. Xiong, Q. Zhang, H. Y. Song and W. H. Ye, Biol. Pharm. Bull., 2002, 25, 558–563 CAS.
-
S. B. Drasar, Human Intestinal Flora, Academic Press Inc., London, 1974, pp. 53–167 Search PubMed.
- T. Akao, T. Hayashi, K. Kobashi, M. Kanaoka, H. Kato, M. Kobayashi, S. Takeda and T. Oyama, J. Pharm. Pharmacol., 1994, 46, 135–137 CrossRef CAS PubMed.
- Z. J. Shu, Y. Cao and U. Halmurat, Expert Opin. Ther. Targets, 2011, 15, 1147–1152 CrossRef PubMed.
- I. A. Ludwig, M. Paz de Peña, C. Concepción and C. Alan, BioFactors, 2013, 39, 623–632 CrossRef CAS PubMed.
- F. Tomas-Barberan, R. García-Villalba, A. Quartieri, S. Raimondi, A. Amaretti, A. Leonardi and M. Rossi, Mol. Nutr. Food Res., 2014, 58, 1122–1131 CAS.
- M. P. Gonthier, C. Remesy, A. Scalbert, V. Cheynier, J. M. Souquet, K. Poutanen and A. M. Aura, Biomed. Pharmacother., 2006, 60, 536–540 CrossRef CAS PubMed.
- J. Yang, D. Qian, S. Jiang, E. X. Shang, J. Guo and J. A. Duan, J. Chromatogr. B: Anal. Technol. Biomed. Life Sci., 2012, 898, 95–100 CrossRef CAS PubMed.
- K. Keppler, E. M. Hein and H. U. Humpf, Mol. Nutr. Food Res., 2006, 50, 686–695 CAS.
- S. Murakami, R. Nakata, T. Aboshi, N. Yoshinaga, M. Teraishi, Y. Okumoto, A. Ishihara, H. Morisaka, A. Huffaker, E. A. Schmelz and N. Mori, Metabolites, 2014, 4, 532–546 CrossRef PubMed.
- R. P. Pandey, P. Parajuli, N. Koirala, J. H. Lee, Y. I. Park and J. K. Sohng, Mol. Cells, 2014, 37, 172–177 CrossRef PubMed.
- Y. S. Kim, J. J. Kim, K. H. Cho, W. S. Jung, S. K. Moon, E. K. Park and D. H. Kim, J. Microbiol. Biotechnol., 2008, 18, 1109–1114 CAS.
- K. Németh, G. W. Plumb, J. G. Berrin, N. Juge, R. Jacob, H. Y. Naim, G. Williamson, D. M. Swallow and P. A. Kroon, Eur. J. Nutr., 2003, 42, 29–42 CrossRef PubMed.
- X. Li, X. Xu, J. Wang, H. Yu, X. Wang, H. Yang, H. Xu, S. Tang, Y. Li, L. Yang, L. Huang, Y. Wang and S. Yang, PLoS One, 2012, 7, e43918 CAS.
- J. Zhu, J. Wang, H. Yu, Y. Li and T. Hou, Comb. Chem. High Throughput Screening, 2011, 14, 362–374 CrossRef CAS PubMed.
- F. Partosch, H. Mielke, R. Stahlmann, B. Kleuser, S. Barlow and U. Gundert-Remy, Arch. Toxicol., 2015, 89, 941–948 CrossRef CAS PubMed.
- D. P. Reynolds, K. Lanevskij, P. Japertas, R. Didziapetris and A. Petrauskas, J. Pharm. Sci., 2009, 98(11), 4039–4054 CrossRef CAS PubMed.
- T. Lu, J. Yang, X. Gao, P. Chen, F. Du, Y. Sun, F. Wang, F. Xu, H. Shang, Y. Huang, Y. Wang, R. Wan, C. Liu, B. Zhang and C. Li, Drug Metab. Dispos., 2008, 36, 1578–1586 CrossRef CAS PubMed.
- Y. Zhang, X. Guo, D. Wang, R. Li, X. Li, Y. Xu, Z. Liu, Z. Song, Y. Lin, Z. Li and N. Lin, Sci. Rep., 2014, 4, 4154 Search PubMed.
- I. Merchenthaler, T. L. Dellovade and P. J. Shughrue, Ann. N. Y. Acad. Sci., 2003, 1007, 89–100 CrossRef CAS PubMed.
- S. M. Liu, N. X. Su, M. D. He and Z. Wang, Zhongnan Daxue Xuebao, Yixueban, 2007, 32, 899–903 Search PubMed.
- R. B. Guo, G. F. Wang, A. P. Zhao, J. Gu, X. L. Sun and G. Hu, PLoS One, 2012, 7, e49701 CAS.
- N. Y. Tang, C. H. Liu, C. T. Hsieh and C. L. Hsieh, Am. J. Chin. Med., 2010, 38, 51–64 CrossRef CAS PubMed.
- D. S. Wishart, C. Knox, A. C. Guo, D. Cheng, S. Shrivastava, D. Tzur, B. Gautam and M. Hassanali, Nucleic Acids Res., 2008, 36(Database issue), D901–D906 CAS.
- A. Hamosh, A. F. Scott, J. Amberger, C. Bocchini, D. Valle and V. A. McKusick, Nucleic Acids Res., 2002, 30(1), 52–55 CrossRef CAS PubMed.
- J. Y. Chen, S. Mamidipalli and T. Huan, BMC Genomics, 2009, 10(suppl 1), S16 CrossRef PubMed.
- L. Matthews, G. Gopinath, M. Gillespie, M. Caudy, D. Croft, B. de Bono, P. Garapati, J. Hemish, H. Hermjakob, B. Jassal, A. Kanapin, S. Lewis, S. Mahajan, B. May, E. Schmidt, I. Vastrik, G. Wu, E. Birney, L. Stein and P. D'Eustachio, Nucleic Acids Res., 2009, 37(Database issue), D619–D622 CrossRef CAS PubMed.
- K. R. Brown and I. Jurisica, Bioinformatics, 2005, 21(9), 2076–2082 CrossRef CAS PubMed.
- B. Aranda, P. Achuthan, Y. Alam-Faruque, I. Armean, A. Bridge, C. Derow, M. Feuermann, A. T. Ghanbarian, S. Kerrien, J. Khadake, J. Kerssemakers, C. Leroy, M. Menden, M. Michaut, L. Montecchi-Palazzi, S. N. Neuhauser, S. Orchard, V. Perreau, B. Roechert, K. van Eijk and H. Hermjakob, Nucleic Acids Res., 2010, 38(Database issue), D525–D531 CrossRef CAS PubMed.
- T. S. Keshava Prasad, R. Goel, K. Kandasamy, S. Keerthikumar, S. Kumar, S. Mathivanan, D. Telikicherla, R. Raju, B. Shafreen, A. Venugopal, L. Balakrishnan, A. Marimuthu, S. Banerjee, D. S. Somanathan, A. Sebastian, S. Rani, S. Ray, C. J. Harrys Kishore, S. Kanth, M. Ahmed, M. K. Kashyap, R. Mohmood, Y. L. Ramachandra, V. Krishna, B. A. Rahiman, S. Mohan, P. Ranganathan, S. Ramabadran, R. Chaerkady and A. Pandey, Nucleic Acids Res., 2009, 37(Database issue), D767–D772 CrossRef CAS PubMed.
- A. Ceol, A. Chatr Aryamontri, L. Licata, D. Peluso, L. Briganti, L. Perfetto, L. Castagnoli and G. Cesareni, Nucleic Acids Res., 2010, 38(Database issue), D532–D539 CrossRef CAS PubMed.
- B. Lehne and T. Schlitt, Hum. Genomics, 2009, 3(3), 291–297 CAS.
- T. Beuming, L. Skrabanek, M. Y. Niv, P. Mukherjee and H. Weinstein, Bioinformatics, 2005, 21, 827–828 CrossRef CAS PubMed.
- F. Zhu, Z. Shi, C. Qin, L. Tao, X. Liu, F. Xu, L. Zhang, Y. Song, X. Liu, J. Zhang, B. Han, P. Zhang and Y. Chen, Nucleic Acids Res., 2012, 40(Database issue), D1128–D1136 CrossRef CAS PubMed.
- Y. Zhang, D. Wang, S. Tan, H. Xu, C. Liu and N. Lin, J. Evidence-Based Complementary Altern. Med., 2013, 2013, 548498 Search PubMed.
- Y. Zhang, Z. Li, M. Yang, D. Wang, L. Yu, C. Guo, X. Guo and N. Lin, PLoS One, 2013, 8, e85170 Search PubMed.
- Y. Zhang, X. Guo, M. Yang, L. Yu, Z. Li and N. Lin, Mol. BioSyst., 2014, 10, 215–222 RSC.
- Y. Wang, Z. Liu, C. Li, D. Li, Y. Ouyang, J. Yu, S. Guo, F. He and W. Wang, J. Evidence-Based Complementary Altern. Med., 2012, 2012, 698531 Search PubMed.
- S. Wuchty and E. Almaas, BMC Evol. Biol., 2005, 5, 24 CrossRef PubMed.
- S. Ansari, H. Azari, D. J. McConnell, A. Afzal and J. Mocco, J. Visualized Exp., 2011, 51, 2879 Search PubMed.
- E. Z. Longa, P. R. Weinstein, S. Carlson and R. Cummins, Reversible middle cerebral artery occlusion without craniectomy in rats, Stroke, 1989, 20, 84–91 CrossRef CAS PubMed.
Footnotes |
† Electronic supplementary information (ESI) available. See DOI: 10.1039/c5mb00460h |
‡ These authors offer the equal contributions to this paper. |
|
This journal is © The Royal Society of Chemistry 2016 |
Click here to see how this site uses Cookies. View our privacy policy here.