DOI:
10.1039/C5MB00415B
(Paper)
Mol. BioSyst., 2016,
12, 110-119
Correlations of microRNA:microRNA expression patterns reveal insights into microRNA clusters and global microRNA expression patterns†
Received
19th June 2015
, Accepted 3rd November 2015
First published on 3rd November 2015
Abstract
MicroiRNAs are genome encoded small double stranded RNAs that regulate expression of homologous mRNAs. With approximately 2500 human miRNAs and each having hundreds of potential mRNA targets, miRNA based gene regulation is quite pervasive in both development and disease. While there are numerous studies investigating miRNA:mRNA and miRNA:protein target expression correlations, there are relatively few studies of miRNA:miRNA co-expression. Here we report on our analysis of miRNA:miRNA co-expression using expression data from the miRNA expression atlas of Landgraf et al. Our analysis indicates that many, but not all, genomically clustered miRNAs are co-expressed as a single pri-miRNA transcript. We have also identified co-expression groups that have similar biological activity. Further, the non-correlative miRNAs we have uncovered have been shown to be of utility in establishing miRNA biomarkers and signatures for certain tumours and cancers.
Introduction
MicroRNAs (miRNA) are small dsRNAs that regulate protein synthesis through the targeting of homologous mRNAs.1 miRNAs are initially expressed as large pri-miRNA transcripts that are subsequently processed by Dicer to release the pre-miRNA hairpin from the primary transcript.2–4 The pre-miRNA hairpin is then processed by Dicer, in the cytoplasm, to generate the double-stranded ∼22-base-pair mature miRNA which along with a homologous mRNA is subsequently incorporated into RISC leading to decreased protein translation.5,6 With over 2500 known human miRNAs, and each miRNA having ∼300 potential targets,7 miRNAs are pervasive gene regulators, implicated in a broad spectrum of disease as well as in cell differentiation and development. In cancer, dysregulation of miRNA expression is prevalent, with miRNA expression signatures having been proven to be effective diagnostic molecules, able to differentiate between healthy tissue and tumours, but also able to differentiate between tumour subtypes.8–13 Through an in situ hybridization screen,14 Sempere et al. established the miRNA expression signature of breast tumours. Perhaps expectedly, the oncogeneic miRNA, miR-21 is overexpressed, and the tumour suppressive miRNA Let-7, has reduced expression in a number of breast tumours.
miRBase is a freely available miRNA data repository with the current version, miRBase 20, holding 24
521 unique miRNAs.15,16 For each miRNA, miRBase lists all other miRNAs within 10 kb of a given miRNA. miRNAs within 10 kb are referred to as being genomically clustered, and by extension presumed to be co-expressed on the same primary transcript. With a 10 kb cut-off, there are 153 human genomic clusters containing 465 miRNAs which is approximately 20% of total known miRNAs. Except for a few examples, there is little supporting experimental data to indicate that these genomically clustered miRNAs are in fact co-expressed on the same pri-miRNA transcript. In delineation of the stepwise miRNA biogenesis pathway, Lee et al.17 were first to demonstrate co-expression of multiple miRNAs on the same pri-miRNA transcript for the miRNA clusters miR-17∼92 and miR-23a∼24-2. Later work by Ventura et al.18 confirmed the co-expression of the miR-17∼92 constituent miRNAs and showed co-expression of miRNAs from the 106b∼25 miRNA cluster, a paralogue of miR-17∼92. Suh et al. demonstrated two embryonic stem cell specific pri-miRNA clusters, miR-302b∼367 and miR-371∼373. Earlier work by Baskerville et al.19 calculated genomically proximal miRNA:miRNA expression correlations for 175 miRNAs across 24 human tissue samples. This analysis focused on the co-expression of miRNAs with their host mRNA genes. They report miRNA:miRNA expression correlations for miRNAs up to 50 kb apart on the same chromosome, consistent with the average size of a human gene of 55 kb. In another earlier study, Sempere et al.,14 in an effort to identify tissue specificity in miRNA expression, did notice miRNA:miRNA expression correlation for certain miRNA clusters.
We report here our miRNA:miRNA expression correlation analysis using expression data from the miRNA expression atlas developed by Landgraf et al.20 Our rationale was to ascertain whether there are expression patterns between miRNAs which would identify novel insights into miRNA function and regulation. Our analysis covers the top 169 expressed miRNAs along with 12 miRNAs from the Epstein Barr virus and one miRNA from the human cytomegalovirus (HCMV) with expression data taken from 15 human tissue samples and 105 human cell lines. Our analysis provides compelling evidence that many of the genomically clustered miRNAs are indeed co-expressed. While many clustered miRNAs appear co-expressed, this is not universally true. For non-clustered miRNAs, there are both positive and negative expression correlations. Our analysis has uncovered intricate miRNA co-expression patterns, and highlights certain expression cohorts which we believe will be an important consideration when considering miRNA based gene regulation and development of disease biomarkers.
Results and discussion
Genomic clusters
As mentioned above, miRBase lists all other miRNAs within 10 kb of a given miRNA.15,16,21 Thus, a 10 kb cut-off is used as a limit to the size of a genomic cluster of miRNAs. As shown in Fig. 1, a plot of number of clusters versus genomic section size plateaus around 1 kb, with an actual depression at 4 kb. The decrease in number of clusters with a 4 kb cut-off versus 1 kb indicates some overlap between clusters has now reduced the total number. After ∼10 kb the number of clusters grows linearly with increasing cluster size limit, as would be expected when expanding the genomic search area. Thus, 10 kb seems a reasonable cut-off for defining miRNA genomic clusters. With a 10 kb cut-off, there are 153 genomic clusters containing 465 miRNAs which is approximately 20% of total known miRNAs. The miRNA expression atlas data used in our study contains expression data on 203 miRNAs from 34 different clusters.20 At this juncture, we want to explicitly differentiate between genomic miRNA clusters (miRNAs within 10 kb as defined by miRBase)21 and pri-miRNA clusters as miRNAs that are genomically clustered and are co-expressed on the same primary transcript (pri-miRNA).17
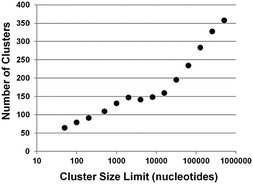 |
| Fig. 1 Genomic clusters. Plot of change in the number of miRNA clusters with clusters increasing size limit. The increase in number of clusters plateaus between size limits of 2000 to 8000 bases. At 10 000 base size limit and beyond, there is log linear growth in the number of clusters with increasing size limit. | |
Correlation analysis of miRNA expression
Using the data from the miRNA expression atlas reported by Landgraf et al.20 we calculated the Pearson correlation coefficients19 for the expression of each miRNA with every other miRNA across 15 human tissue samples and 105 human cell lines. The miRNAs investigated include the top 169 expressed miRNAs (excluding isoMirs) along with 12 miRNAs from the Epstein Barr virus and one miRNA from the human cytomegalovirus (hCMV). While a majority of the 33
124 correlation coefficients (Table S2, ESI†) cluster near zero (Fig. 2A) a significant occurrence of positive correlations are observed which results in an asymmetric distribution of the frequency of correlation coefficients. In cases where the expression of miRNAs that are physically linked, such as those miRNAs on the same pri-miRNA transcript, one predicts a high level of correlations between these miRNAs when comparing their expression levels from these different cells and tissues. An example are miR-17 and miR-20a which are both expressed from the miR17∼92 cluster and exhibit a Pearson correlation coefficient of 0.8 when the expression levels from all the different cells and tissues are analysed (Fig. 2B).
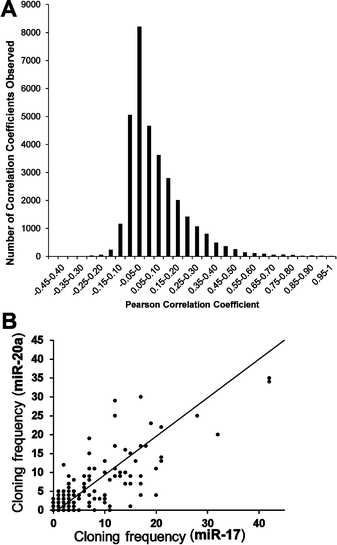 |
| Fig. 2 (A) Frequencies of the Pearson correlation coefficients of the expression of 181 different miRNAs with each other in 120 cell lines and tissues. Correlations of a miRNA with itself have been omitted. (B) Cloning frequencies of miR-20a and miR-17 which are both expressed from the miR-17∼92 pri-miRNA cluster. The Pearson correlation coefficient for these to miRNAs is 0.8. | |
To simultaneously visualize which miRNAs correlate with each other, a graphical Circos plot22 was generated which highlights both the positive and negative correlations (Fig. 3). The graphically presented data immediately reveals many negative correlations with the miR-140-5p and -3p miRNAs and a cluster of strong positive correlations between the EBV miRNAs which arise from a subset of nine cell lines used in compiling the miRNA atlas that express miRNAs from the Epstein Barr Virus.23 In addition to the strong correlations between the EBV miRNAs, miR-146a, miR-155 and 193b also exhibit positive correlation coefficients with the EBV miRNAs, suggesting their expression in these cell lines is in response to EBV infection.24
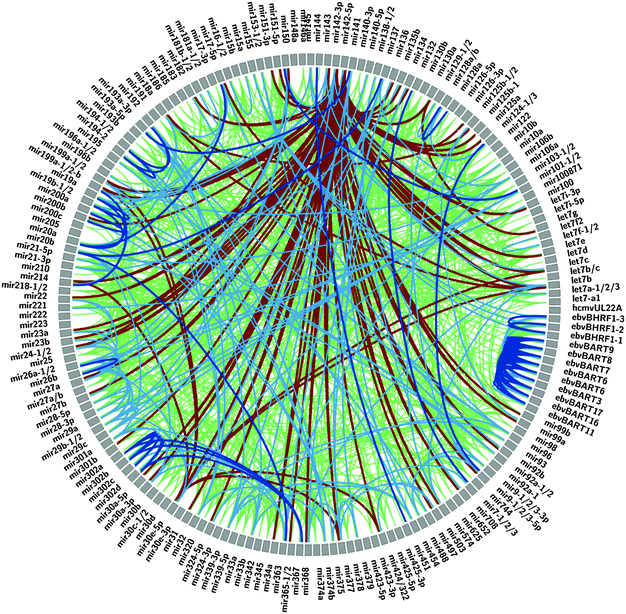 |
| Fig. 3 Circos diagram to depict high correlation coefficients between each miRNA:miRNA pair. ≥0.4 in green, ≥0.6 in light blue, ≥0.8 in dark blue and ≤−0.2 in burgundy. | |
Generally, the positive correlations for clustered miRNAs are stronger than correlations for non-clustered miRNAs. miR-17 of the miR-17∼92 cluster has a correlation coefficient of 0.63, 0.6, 0.8, 0.62 and 0.25 to miRNAs 18a, 19a, 20a, 19b and 92a-1 respectively (the lower correlation of miR-92a-1 with respect to the other clustered miRNAs is consistent with the tertiary structure effects on miRNA expression from this cluster)25–27 (Table S2, ESI†).
So with the high correlations between miRNAs of a miRNA cluster known to be transcribed as a single poly-cistronic pri-miRNA we queried the data for correlations between miRNAs from other known and putative miRNA clusters that are within 10 kb of each other.
We evaluated whether the strengths of the correlations can be used to predict whether genomically clustered miRNAs, miRNAs within 10 kb were actually co-expressed on the same pri-miRNA transcript, and thereby expand the list of known poly-cistronic pri-miRNA clusters. By using a correlation co-efficient threshold of 0.5, we predict there are 24 poly-cistronic pri-miRNA clusters (Table 1). However, there are a couple of genomically clustered miRNAs with significant expression correlations of ≥0.4 that fall outside this cut-off. For some of these clusters, particularly the let-7 clusters, the expression data for an individual miRNA can be the total of up to three different miRNA isoforms (e.g. Let7a-1, Let-7a-2, Let-7a-3), and thus can hide the correlation strength of each individual miRNA of the group. Thus we have expanded the cluster list to 26 to include those clusters with multi-isoform miRNA correlations of 0.4 to 0.6, namely, miR-23b∼27b∼3074∼24-1 and Let-7a-2∼100. Of the 169 miRNAs in our analysis 61 (∼36%) are expressed on pri-miRNA clusters.
Table 1 List of miRNA genomic clusters, with representative miRNA pair correlation coefficients (CC) and inter-hairpin distance (IHD). For genomic clusters with CC values below 0.4, there are intervening transcriptional start sites (ITSS), that suggest the clustered miRNAs are independent transcription units
Genomic cluster |
miRNA pair |
CC |
IHD |
ITSS |
17∼18a∼19a∼20a∼19b-1∼92a-1 |
17∼20a |
0.8 |
700 |
|
106a∼18b∼20b∼19b-2∼92a-2∼363 |
106a∼363 |
0.7 |
800 |
|
106b∼93∼25 |
106b∼25 |
0.6 |
331 |
|
301b∼130b |
301b∼130b |
0.5 |
246 |
|
143∼145 |
143∼145 |
0.7 |
1623 |
|
451a∼144∼4732 |
451a∼144 |
0.9 |
93 |
|
16-1∼15a |
16-1∼15a |
0.8 |
58 |
|
15b∼16-2 |
15b∼16-2 |
0.5 |
60 |
|
181b-1∼181a-1 |
181b-1∼181a-1 |
0.7 |
62 |
|
181a-2∼181b-2 |
181a-2∼181b-2 |
0.7 |
1159 |
|
182∼96∼183 |
182∼96 |
0.7 |
4200 |
|
192∼194-2∼6750 |
192∼194-2 |
0.9 |
109 |
|
195∼497 |
195∼497 |
0.6 |
210 |
|
199a-2∼214 |
199a-2∼214 |
0.8 |
5628 |
|
200b∼200a∼429 |
200b∼200a |
0.9 |
665 |
|
23a∼27a∼24-2 |
23a∼24 |
0.7 |
211 |
|
23b∼27b∼3074∼24-1 |
23b∼27b |
0.7 |
141 |
|
23b∼27b∼3074∼24-1 |
23b∼24 |
0.4 |
1214 |
|
29b-1∼29a |
29b-1∼29a |
0.7 |
649 |
|
29b-2∼29c |
29b-2∼29c |
0.7 |
504 |
|
302b∼302c∼302a∼302d∼367 |
302b∼367 |
0.9 |
522 |
|
424∼503∼542∼450a-2∼450a-1∼450b |
424∼503 |
0.7 |
216 |
|
Let-7a-3∼4763∼Let-7b |
Let-7a∼Let-7b |
0.7 |
774 |
|
Let7a-2∼100 |
Let7a-2∼100 |
0.4 |
5786 |
|
99a∼Let-7c |
99a∼Let-7c |
0.5 |
659 |
|
98∼Let-7f-2 |
98∼Let-7f-2 |
0.5 |
1051 |
|
Let-7e∼125a |
Let-7e∼125a |
0.5 |
390 |
|
99b∼Let-7e∼125a |
99b∼Let-7e |
0.2 |
105 |
Yes |
Let7a-1∼Let7f-1∼Let7d |
Let7a-1∼Let-7f-1 |
0.2 |
311 |
Yes |
|
Let-7a-1∼Let-7d |
0.1 |
2772 |
Yes |
200c∼141 |
200c∼141 |
0.3 |
331 |
Yes |
425∼191 |
425∼191 |
0.4 |
385 |
Yes |
222∼221 |
222∼221 |
0.3 |
727 |
Yes |
30d∼30b |
30d∼30b |
0.2 |
4269 |
Yes |
30e∼30c-1 |
30e∼30c-1 |
0.3 |
2838 |
Yes |
Currently there is insufficient deep sequencing RNA data available to verify our predictions regarding the pri-miRNA clusters. This is a result of the instability of pri-miRNAs as a result of co-transcriptional processing where very few reads from the intervening sequences between pre-miRNA stem loops are observed in RNA-Seq datasets. For documented clusters like miR-17∼92, the data only reveals the mature miRNA sequences.28 Recently the issue of pri-miRNA instability has been circumvented by using cells with impaired microprocessor activity for investigations of the miR-17∼92 cluster,29 but further similar investigations are required to address the question of other pri-miRNA clusters.
To confirm the expression of a panel of pri-miRNA clusters we performed RT-PCR analysis (Fig. 4). There has been some ambiguity in whether the miRNAs from the miR-23b∼27b∼24-1 genomic cluster were actually expressed as a pri-miRNA cluster. In support of our expression correlation data is our RT-PCR assay showing expression of the pri-miR-23b∼27b∼24-1 transcript (Fig. 4B).30,31
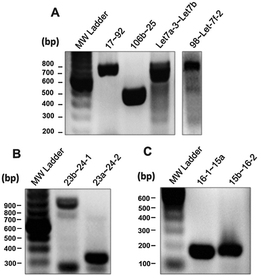 |
| Fig. 4 RT-PCR analysis pri-miRNA clusters. (A) RT-PCR assay of pri-miRNA clusters: miR-17∼92, miR-106b∼25, miR-Let-7a-3∼Let-7b and miR-98∼Let-7f-2. (B) RT-PCR assay of pri-miRNA clusters: miR-23b∼24-2 and miR-23a∼24-2. (C) RT-PCR assay of pri-miRNA clusters miR-16-1∼15a, miR-15b∼16-2. | |
miR-302b∼302c∼302a∼302d∼367 is a pri-miRNA cluster that is remarkable in the strength of correlations amongst the constituent miRNAs. miR-302b and miR-367, which are ∼520 nucleotides apart, have a correlation coefficient of 0.9 while miR-302a and miR-367 at 235 nucleotides apart have a correlation coefficient of 0.97 (Table S2, ESI,† and Table 1). Further, the miRNAs from this cluster are highly and exclusively expressed in the three neuronal tumour cell lines and three testicular germ cell tumour cell lines in the miRNA expression atlas data set. miRNAs from the miR-302b∼367 are part of a subset of miRNAs that are expressed in embryonic stem cells under the regulatory control of the core transcriptional machinery involved in determining stem cell fate.32
From the analysis, poor correlations reveal that there are genomically clustered miRNAs that do not appear to be co-expressed on the same pri-miRNA (Table 1). While there are Let-7 miRNAs expressed as part of a pri-miRNA cluster as with Let7a-3∼Let-7b, there are genomically clustered Let-7 miRNAs that appear to not be co-expressed as part of a pri-miRNA cluster (Table S2, ESI,†Fig. 3). The Let-7 miRNAs from the genomic cluster Let-7a-1∼Let-f-1∼Let7d do not display significant expression correlations nor could we detect pri-Let-7a-1∼Let-f-1∼Let7d by RT-PCR (data not shown). Likewise, there is no significant correlation between the miRNAs from the genomic clusters miR-200c∼141, 425∼191, 30d∼30b, 30e∼30c-1 and 222∼221. An analysis of these genomic loci with the Human Genome Browser reveals that there are intervening RNA Polymerase II and other transcription factor binding sites (Encode Chip-seq). Given the lack of expression correlation and the intervening transcriptional start sites, we propose that these miRNAs are likely to be transcribed independently. Alternatively, there have been several post-transcriptional regulatory mechanisms established for miRNA expression.33,34 One example is the effect of pri-miRNA tertiary structure, which has been shown to regulate constituent miRNA expression.25–27
Through our analysis we have identified 26 miRNA clusters, that express 76 different miRNAs in total. The miR-17∼92, miR-106a∼363, and the 424∼503∼542∼450a-2∼450a-1∼450b clusters have the most miRNAs per pri-miRNA with six each (Table 1). There is a large amount of primary structure diversity within these clusters, with the miR-15 and miR-16 clusters comprising only two miRNAs with a relatively small inter-hairpin distance of ∼60 nucleotides to the 199a-2∼214 cluster with 5628 nucleotides between the two miRNA hairpins in the cluster. The minimum inter-hairpin distance appears to be around 50 nucleotides, which is consistent with what is known about the secondary structural requirements of Drosha processing of pre-miRNA hairpins.35,36
Expression correlations organize miRNAs into functional groups
Expression correlation analysis has not only revealed correlations in expression between constituent miRNAs from a pri-miRNA cluster, but also between non-genomically clustered miRNAs.
As with proteins the oncogene and tumour suppressor nomenclature has been adapted to describe the role of miRNAs involved in cancer.12 While there are exceptions, the bulk of data on the activities of the miR-17∼92 cluster miRNAs demonstrate the constituent miRNAs function as oncogenes.37–39 It has been shown that over-expression of the miRNA cluster drives tumourigeneis in several mouse models.38,39 Similarly, the bulk of cancer studies concerning the role of Let-7 suggest it is typically a tumour suppressive miRNA.40–42 The miRNAs from the miR-17∼92 cluster show positive correlation (≥0.4) with 14 miRNAs beyond its genomic locus (Fig. 5). The correlation pattern is quite complex with miR-378, miR-106b and miR-25 having correlations with four miR-17∼92 miRNAs (17, 18a, 19a, 20a; 17, 18a, 19a, 20a; and 17, 18a, 20a, 92a-1 respectively). The correlation between miR-17∼92 and the miRNAs from the paralogous cluster miR-106b∼93∼25 is consistent with reported expression patterns in mice.18 Conversely, miRNAs 10a, 186, 196, 301b and 32 only correlate with miR-18a (Fig. 5A). Thus, there are quite complex associations among the 20 unique miRNAs from this expression group which suggests multiple levels of regulation for the different miRNAs.
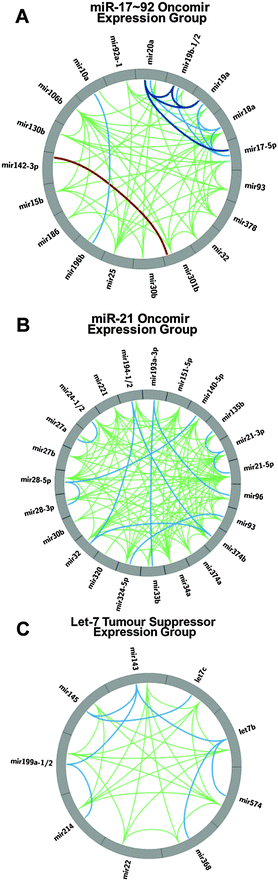 |
| Fig. 5 (A) Circos plot presenting the expression correlations of the miRNAs that exhibit positive Pearson correlations with the miR-17∼92 cluster. Correlation coefficients between each miRNA:miRNA pair. ≥0.4 in green, ≥0.6 in light blue, ≥0.8 in dark blue and ≤−0.2 in burgundy. (B) Circos plot of the expression correlations of miRNAs that exhibit positive Pearson correlations with miR-21-5p and miR-21-3p. (C) Circos plot of the expression correlations of miRNAs that exhibit positive Pearson correlations with Let-7b. | |
miR-21 has a very well established oncogenic role in cancer.43–45 In a miRNA expression screen across 540 samples from lung, breast, stomach, prostate, colon, and pancreatic tumors, miR-21 was found to be over-expressed in all tumour types.11 Interestingly, miR-21 does not significantly correlate with the miR-17∼92 constituent miRNAs but it does exhibit significant positive correlations with a group of miRNAs comprising of miRNAs 135b, 140, 151, 193, 194, 221, 24, 27b, 28, 30b, 32, 320, 324, 33b, 34a, 374, 93 and 96 (Fig. 5B). The only overlap with the miR-17∼92 expression group is miRNAs 30b, 32 and 93. Thus, our miRNA:miRNA expression correlation analysis reveals a second group of highly correlated miRNAs that have been attributed an oncogenic function.
While the expression group reveal general correlations of expression with miR-21, these correlations are not absolute so while their correlations in expression are not predicted to identically match those of miR-21 in clinical biomarker investigations.46,47 Nonetheless these expression correlations may eventfully prove to have utility in the additional stratification and sub-typing of diseases.
Recently, several studies have reported a tumour suppressive role for the miR-143∼145 pri-miRNA cluster in several cancers, including pancreatic, prostate, renal and colorectal cancer.48–50 In pancreatic cancer, the oncogene KRAS suppresses expression of the miR-143∼145 cluster through the Ras responsive element (RRE) binding protein (RREB1) which results in loss of regulation of the MAPK signalling pathway by the cluster's miRNAs. In addition to its well-known role in developmental timing,51 Let-7 also has well established tumour suppressive roles in a wide range of cancers including breast, prostate and lung cancer. Through our co-expression analysis we observe strong correlations between Let-7 (both Let-7b and Let-7c) and miR-143∼145 (Table S2, ESI,† and Fig. 5C). Further, in addition to Let-7 correlation with miR-143∼145, we have identified that miRNAs 199a, 214, 22, 368 (376c), and 574 also correlate with these miRNAs as another co-expression group. These correlations predict that these expression group miRNAs, if detected, would exhibit similar patterns of expression as let-7 miRNA in studies attempting to identify miRNA biomarkers. A recent investigation comparing colorectal cancer and normal tissue52 identified a down regulation of both let-7b and let-7c, further investigation of the reported data also reveals the down regulation of the miR-143∼145 miRNAs.
There is ample literature showing tumour suppressive roles for the miRNAs in this group beyond Let-7 and the miR-143∼145 cluster. miR-199a has a reported tumour suppressive roles in several cancers including prostate, ovarian, breast, bladder, and liver and its reduced expression correlates with histological grade in renal cancer.53 Consistent with a tumour suppressive role, miR-22 is located at 17p13.3, is a site of frequent loss of heterozygosity in ovarian, lung, liver and brain cancer. Further, miR-22 has been shown to have reduced expression in breast and liver cancer, as well as leukemia.54–56 Though not as well established, there are reports of miRNAs 368 (376c), 214 and 574 also having tumour suppressive functions.57,58 While Let-7, miR-143 and miR-22 are in the top 25 expressed miRNAs, 199a, 368 and 574 show high expression specificity, particularly in brain, pancreas, ovary, testes, and uterus as well as USSC stem cells.20 Higher expression in healthy tissue as opposed to tumour derived cell lines is consistent with a tumour suppressive role of this group of miRNAs.
The above mentioned groups of miRNAs that display correlations in their expression patterns is by no means complete, but are presented as examples that can be extracted from the complete set of data. As demonstrated in the frequency of correlations for different miRNA (Fig. 6), the vast majority of miRNAs exhibit multiple positive correlations in expression with other miRNAs so similar analysis can be performed virtually any miRNA of interest. As revealed in Fig. 6, the peak frequency of correlations (>0.35) for a given miRNA is 9–12 positive correlations while a maximal number of correlations for a miRNA is 28.
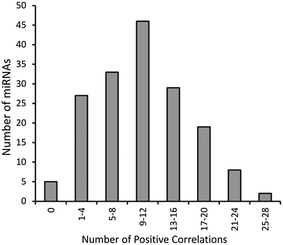 |
| Fig. 6 Frequency plot of the number of correlations (>0.35) for individual miRNAs. | |
Mechanisms of expression correlations
For any given pair or group of co-expressed miRNAs, there are additional mechanistic routes to co-regulate expression beyond transcription on the same pri-miRNA. Regulation through transcriptional activation via a mutual transcription factor is one such route. Thus transcriptional regulation of miRNA expression is quite complex with a given transcription factor able to regulate several different miRNAs, and each miRNA can be regulated by several different transcription factors.59 Using the TransmiR database, a survey of transcription factors known to regulate the constituent miRNAs of the three expression groups revealed that the miRNAs in these groups did not have a transcription factor in common and to transcribe all miRNAs within either group would require several different transcription factors.
For the miR-17∼92 expression group consisting of 19 unique miRNAs, 6 are from the miR-17∼92 cluster, while another 3 are from the paralogous miR-106b cluster. These two clusters and miR-15b are transcriptional targets of transcription factor E2F3 while miRNAs 10a, 130b, 142, 301b and 32 are transcriptional targets of transcription factor EGR1.59,60 Thus these two transcription factors have the potential to co-regulate 15 of the 19 miRNAs in the miR-17∼92 expression group.
In addition to transcriptional regulation, there is post-transcriptional regulation at every step along the miRNA biogenesis pathway.33,34,61 Additionally, mature miRNA turnover can proceed through several different mechanisms. We performed sequence conservation analysis for the miRNAs in each group to search for a common sequence motif for each group. A common sequence motif could potentially be utilized in post-transcriptional regulation of a group of miRNAs. Beyond the GGAG motif in the Let-7 expression group,62 an alignment of the mature miRNA or pre-miRNA sequences of either group did not reveal a common sequence motif.
Negative correlations of miRNA:miRNA expression
While the occurrence of positive correlations of expression between different miRNAs was predicted as a result of multiple mechanisms such as co-transcription as pri-miRNAs and via regulation through the same transcription factors, the observation of numerous negative correlations was somewhat unexpected. While the negative Pearson correlation coefficients occurred at a much lower absolute value than the positive correlation coefficients, the frequency for which some of the negative correlation coefficients occur for some miRNAs was quite striking (Fig. 3). As seen in Fig. 3, miR-142-5p and miR-142-5p exhibit the largest number of negative correlations which range from −0.2 to −0.3 range. The high occurrence of negative correlations for these miRNAs may reflect specific cell differentiation programs as these two miRNAs are only observed in high amounts in cells of hematopoietic origin (Table S1, ESI†).
Non-correlating miRNAs
miRNAs have been shown to be effective biomarkers in classifying different tumours, assessing metastatic potential as well as being of prognostic value in predicting patient outcomes. miRNAs miR-21 and miRNAs from the miR-17∼92 cluster are frequently listed as miRNAs in tumour biomarkers. While they are useful in differentiating the normal tissue and cells from tumours, they are too frequently over-expressed in many cancers to be useful in distinguishing between cancers-lung from colorectal for example.10,11,63 Thus, miRNAs with fewer, rather than more, expression correlations may be of more utility as a biomarker for a specific cancer or disease state. This is a point beginning to emerge regarding many miRNAs which cannot be regarded as independent variables.64 While most miRNAs in this study have many expression correlations, there are several with no significant correlations. miRNAs 148a, 625, 196a-1/2, 205, and 210 do not have correlations of ≥0.35. Since the miRNAs in this group have few to no significant correlations, they may be of higher value as differentiating biomarkers. For example, miRNAs 205 and 210 have been shown to have unique expression profiles in breast and other cancers65–69 but the induced expression of miR-210 by hypoxia70,71 challenges the utility of this miRNA as a cancer specific biomarkers. miRNAs 196a and 148a have recently been established as biomarkers differentiating gastric tumours from normal gastric tissue where miR-196a has increased expression and miR-148a has decreased expression in gastric tumours.72 While decreased miR-625 has been shown to correlate with tumour metastasis and poor prognosis in colorectal cancer.73
Conclusions
We have performed a miRNA:miRNA co-expression analysis by calculating the correlation coefficients for each miRNA:miRNA pair in the miRNA expression atlas compiled by Landgraf et al.20 This analysis has many expression correlations amongst the miRNAs in the atlas, as well as some anti-correlations. Around 20% of the human miRNAs in miRBase are genomically clustered (within 10 kb of another miRNA). It has been generally presumed that genomically clustered miRNAs are co-expressed on the same pri-miRNA. We have used expression correlations to differentiate between genomic clusters and pri-miRNA clusters where the genomically clustered miRNAs are actually co-expressed on the same transcript. We have found that miRNAs co-expressed on a pri-miRNA cluster have stronger expression correlations than those not co-expressed on a pri-miRNA. We use this criterion to provide support for 26 of 33 genomic clusters to be actual pri-miRNA clusters. Additionally, we predict that 7 of the 33 genomic clusters are not pri-miRNA clusters due to the lack of strong expression correlations amongst the constituent miRNAs.
In compiling expression correlations, we found that certain miRNAs organized into co-expression groups consistent with their proposed biological activity. We found two oncogenic expression groups centered on the miR-17∼92 cluster and miR-21. While these expression groups consisted of miRNAs with established oncogenic roles in numerous cancers, there was very little overlap between the two groups, suggesting there are two distinct oncogenic miRNA co-expression groups. Likewise, we also found a tumour suppressive co-expression group centered on Let-7. The miRNAs of this group have well established tumour suppressive roles in various cancers. There is an insignificant expression correlation between the miR-21 expression group and the Let-7 group. This would suggest that only one of these expression groups predominate in the miRNA expression landscape at any given time.
miRNAs have attracted considerable attention as disease biomarkers for defining the disease state, and have demonstrated prognostic value in predicting disease onset and patient response to treatment. miRNAs like miR-21 and the miRNAs from the miR-17∼92 cluster are frequently and generally over-expressed in tumours. While useful in differentiating tumours from healthy tissue, their ubiquitous expression in cancer renders them poor biomarkers in terms of providing a miRNA expression signature for a particular cancer or grading a particular tumour. Thus, miRNAs that are not ubiquitously expressed may be of more utility. We have found miRNAs that do not have significant (≥0.4) expression correlations. Interestingly, these non-correlative miRNAs have been found to be useful in differentiating certain tumours and in breast cancer have shown to be of prognostic value in predicting response to drug treatments.
In conclusion, to date, our analysis is the largest reported miRNA:miRNA co-expression analysis. This analysis has proven useful in predicting the co-expression of genomically clustered miRNAs, and identifying expression groups of common biological activity. miRNAs that show no significant co-expression correlations are known to be useful biomarkers of prognostic value. We believe this analysis will be useful in future studies of gene regulation by miRNAs. The co-expression groups indicate the likelihood that more than one miRNA is up regulated in a given disease state. For example, if it is found that miR-21 has increased expression in a certain tissue or tumour, then it is likely that another miRNA from the miR-21 expression group may also have increased expression. Thus, our identification of these expression groups may facilitate a more thorough understanding of miRNA based gene regulation in a given cell, tissue or tumour.
Experimental
Expression data analysis
miRNA expression data (cloning frequencies) were taken from the miRNA expression atlas of Landgraf et al.20 Only those miRNAs with expression values greater than 50 were included in our analysis. Expression correlations were calculated for each miRNA:miRNA pair using expression data across all 250 samples used in the atlas. Pearson correlation coefficients were calculated in Excel. Correlation data was then used to generate scatter plots (Prism). For circular visualization of the correlation data, Circos v.0.55 was used.22 MicroRNAs were defined as chromosomes and arc drawn according to the correlation values: green ≥ 0.4, light blue ≥ 0.6, dark blue ≥ 0.8 and burgundy ≤ 0.2.
pri-miRNA RT-PCR assay
End point RT-PCR was performed in two steps. cDNA was synthesized using 5 μg of total RNA template, 50 pmol reverse oligo in a 20 μL reaction volume. Prior to the addition of reverse transcriptase, reaction buffer, dithiothreitol and dNTPs, the RNA and reverse oligonucleotide, in water, were thermally denatured for 30 seconds at 95 °C, followed by 5 minutes at 55 °C before cooling on ice for 1 minute. After the thermal denaturation and renaturation step, reverse transcriptase (Invitrogen SuperScript™ III Reverse Transcriptase as per manufacturer protocol) and the reaction components were added and reverse transcription was carried out at 55 °C for 60 minutes, followed by denaturation of reverse transcriptase for 15 minutes at 75 °C. cDNA was then amplified by PCR (Truin Science Taq, as per manufacturer's protocol) and cDNAs were resolved by 1% agarose gels and visualized by ethidium bromide staining. Total RNA source, and forward and reverse oligonucleotides for RT-PCR are listed in Table S3 (ESI†).
Acknowledgements
This work was supported by the Natural Sciences and Engineering Research Council (NSERC) of Canada (Discovery Grant 341453-12 to R. P. F.).
References
- D. P. Bartel, Cell, 2009, 136, 215–233 CrossRef CAS PubMed.
- V. N. Kim, Nat. Rev. Mol. Cell Biol., 2005, 6, 376–385 CrossRef CAS PubMed.
- V. N. Kim, J. Han and M. C. Siomi, Nat. Rev. Mol. Cell Biol., 2009, 10, 126–139 CrossRef CAS PubMed.
- I. Slezak-Prochazka, S. Durmus, B. J. Kroesen and A. van den Berg, RNA, 2010, 16, 1087–1095 CrossRef CAS PubMed.
- V. A. Cherkasova and A. G. Hinnebusch, Genes Dev., 2003, 17, 859–872 CrossRef CAS PubMed.
- W. Filipowicz, S. N. Bhattacharyya and N. Sonenberg, Nat. Rev. Genet., 2008, 9, 102–114 CrossRef CAS PubMed.
- H. C. Martin, S. Wani, A. L. Steptoe, K. Krishnan, K. Nones, E. Nourbakhsh, A. Vlassov, S. M. Grimmond and N. Cloonan, Genome Biol., 2014, 15, R51 CrossRef PubMed.
- C. M. Croce, Nat. Rev. Genet., 2009, 10, 704–714 CrossRef CAS PubMed.
- G. Di Leva and C. M. Croce, Curr. Opin. Genet. Dev., 2013, 23, 3–11 CrossRef CAS PubMed.
- G. Di Leva, M. Garofalo and C. M. Croce, Annu. Rev. Pathol.: Mech. Dis., 2014, 9, 287–314 CrossRef CAS PubMed.
- S. Volinia, G. A. Calin, C. G. Liu, S. Ambs, A. Cimmino, F. Petrocca, R. Visone, M. Iorio, C. Roldo, M. Ferracin, R. L. Prueitt, N. Yanaihara, G. Lanza, A. Scarpa, A. Vecchione, M. Negrini, C. C. Harris and C. M. Croce, Proc. Natl. Acad. Sci. U. S. A., 2006, 103, 2257–2261 CrossRef CAS PubMed.
- R. Garzon, G. A. Calin and C. M. Croce, Annu. Rev. Med., 2009, 60, 167–179 CrossRef CAS PubMed.
- J. J. Xi, Cancer Treat. Res., 2013, 158, 119–137 Search PubMed.
- L. F. Sempere, S. Freemantle, I. Pitha-Rowe, E. Moss, E. Dmitrovsky and V. Ambros, Genome Biol., 2004, 5, R13 CrossRef PubMed.
- A. Kozomara and S. Griffiths-Jones, Nucleic Acids Res., 2014, 42, D68–D73 CrossRef CAS PubMed.
- S. Griffiths-Jones, H. K. Saini, S. van Dongen and A. J. Enright, Nucleic Acids Res., 2008, 36, D154–D158 CrossRef CAS PubMed.
- Y. Lee, K. Jeon, J. T. Lee, S. Kim and V. N. Kim, EMBO J., 2002, 21, 4663–4670 CrossRef CAS PubMed.
- A. Ventura, A. G. Young, M. M. Winslow, L. Lintault, A. Meissner, S. J. Erkeland, J. Newman, R. T. Bronson, D. Crowley, J. R. Stone, R. Jaenisch, P. A. Sharp and T. Jacks, Cell, 2008, 132, 875–886 CrossRef CAS PubMed.
- S. Baskerville and D. P. Bartel, RNA, 2005, 11, 241–247 CrossRef CAS PubMed.
- P. Landgraf, M. Rusu, R. Sheridan, A. Sewer, N. Iovino, A. Aravin, S. Pfeffer, A. Rice, A. O. Kamphorst, M. Landthaler, C. Lin, N. D. Socci, L. Hermida, V. Fulci, S. Chiaretti, R. Foa, J. Schliwka, U. Fuchs, A. Novosel, R. U. Muller, B. Schermer, U. Bissels, J. Inman, Q. Phan, M. Chien, D. B. Weir, R. Choksi, G. De Vita, D. Frezzetti, H. I. Trompeter, V. Hornung, G. Teng, G. Hartmann, M. Palkovits, R. Di Lauro, P. Wernet, G. Macino, C. E. Rogler, J. W. Nagle, J. Ju, F. N. Papavasiliou, T. Benzing, P. Lichter, W. Tam, M. J. Brownstein, A. Bosio, A. Borkhardt, J. J. Russo, C. Sander, M. Zavolan and T. Tuschl, Cell, 2007, 129, 1401–1414 CrossRef CAS PubMed.
- L. Guo, Y. Zhao, H. Zhang, S. Yang and F. Chen, Gene, 2014, 534, 24–32 CrossRef CAS PubMed.
- M. Krzywinski, J. Schein, I. Birol, J. Connors, R. Gascoyne, D. Horsman, S. J. Jones and M. A. Marra, Genome Res., 2009, 19, 1639–1645 CrossRef CAS PubMed.
- Z. L. Pratt, M. Kuzembayeva, S. Sengupta and B. Sugden, Virology, 2009, 386, 387–397 CrossRef CAS PubMed.
- Q. Yin, J. McBride, C. Fewell, M. Lacey, X. Wang, Z. Lin, J. Cameron and E. K. Flemington, J. Virol., 2008, 82, 5295–5306 CrossRef CAS PubMed.
- S. G. Chaulk, G. L. Thede, O. A. Kent, Z. Xu, E. M. Gesner, R. A. Veldhoen, S. K. Khanna, I. S. Goping, A. M. MacMillan, J. T. Mendell, H. S. Young, R. P. Fahlman and J. N. Glover, RNA Biol., 2011, 8, 1105–1114 CrossRef CAS PubMed.
- S. G. Chaulk, Z. Xu, M. J. Glover and R. P. Fahlman, Nucleic Acids Res., 2014, 42, 5234–5244 CrossRef PubMed.
- S. Chakraborty, S. Mehtab, A. Patwardhan and Y. Krishnan, RNA, 2012, 18, 1014–1028 CrossRef CAS PubMed.
- T. Nojima, T. Gomes, A. R. Grosso, H. Kimura, M. J. Dye, S. Dhir, M. Carmo-Fonseca and N. J. Proudfoot, Cell, 2015, 161, 526–540 CrossRef CAS PubMed.
- P. Du, L. Wang, P. Sliz and R. I. Gregory, Cell, 2015, 162, 885–899 CrossRef CAS PubMed.
- Q. Zhou, R. Gallagher, R. Ufret-Vincenty, X. Li, E. N. Olson and S. Wang, Proc. Natl. Acad. Sci. U. S. A., 2011, 108, 8287–8292 CrossRef CAS PubMed.
- F. Sun, J. Wang, Q. Pan, Y. Yu, Y. Zhang, Y. Wan, X. Li and A. Hong, Biochem. Biophys. Res. Commun., 2009, 380, 660–665 CrossRef CAS PubMed.
- A. Marson, S. S. Levine, M. F. Cole, G. M. Frampton, T. Brambrink, S. Johnstone, M. G. Guenther, W. K. Johnston, M. Wernig, J. Newman, J. M. Calabrese, L. M. Dennis, T. L. Volkert, S. Gupta, J. Love, N. Hannett, P. A. Sharp, D. P. Bartel, R. Jaenisch and R. A. Young, Cell, 2008, 134, 521–533 CrossRef CAS PubMed.
- B. N. Davis-Dusenbery and A. Hata, J. Biochem., 2010, 148, 381–392 CAS.
- H. Siomi and M. C. Siomi, Mol. Cell, 2010, 38, 323–332 CrossRef CAS PubMed.
- Y. Zeng and B. R. Cullen, J. Biol. Chem., 2005, 280, 27595–27603 CrossRef CAS PubMed.
- V. C. Auyeung, I. Ulitsky, S. E. McGeary and D. P. Bartel, Cell, 2013, 152, 844–858 CrossRef CAS PubMed.
- V. Olive, Q. Li and L. He, Immunol. Rev., 2013, 253, 158–166 CrossRef PubMed.
- J. T. Mendell, Cell, 2008, 133, 217–222 CrossRef CAS PubMed.
- L. He, J. M. Thomson, M. T. Hemann, E. Hernando-Monge, D. Mu, S. Goodson, S. Powers, C. Cordon-Cardo, S. W. Lowe, G. J. Hannon and S. M. Hammond, Nature, 2005, 435, 828–833 CrossRef CAS PubMed.
- F. Yu, H. Yao, P. Zhu, X. Zhang, Q. Pan, C. Gong, Y. Huang, X. Hu, F. Su, J. Lieberman and E. Song, Cell, 2007, 131, 1109–1123 CrossRef CAS PubMed.
- P. Trang, P. P. Medina, J. F. Wiggins, L. Ruffino, K. Kelnar, M. Omotola, R. Homer, D. Brown, A. G. Bader, J. B. Weidhaas and F. J. Slack, Oncogene, 2010, 29, 1580–1587 CrossRef CAS PubMed.
- J. E. Thornton and R. I. Gregory, Trends Cell Biol., 2012, 22, 474–482 CrossRef CAS PubMed.
- D. Frezzetti, M. De Menna, P. Zoppoli, C. Guerra, A. Ferraro, A. M. Bello, P. De Luca, C. Calabrese, A. Fusco, M. Ceccarelli, M. Zollo, M. Barbacid, R. Di Lauro and G. De Vita, Oncogene, 2011, 30, 275–286 CrossRef CAS PubMed.
- P. P. Medina, M. Nolde and F. J. Slack, Nature, 2010, 467, 86–90 CrossRef CAS PubMed.
- K. Schramedei, N. Morbt, G. Pfeifer, J. Lauter, M. Rosolowski, J. M. Tomm, M. von Bergen, F. Horn and K. Brocke-Heidrich, Oncogene, 2011, 30, 2975–2985 CrossRef CAS PubMed.
- J. X. Zhang, W. Song, Z. H. Chen, J. H. Wei, Y. J. Liao, J. Lei, M. Hu, G. Z. Chen, B. Liao, J. Lu, H. W. Zhao, W. Chen, Y. L. He, H. Y. Wang, D. Xie and J. H. Luo, Lancet Oncol., 2013, 14, 1295–1306 CrossRef CAS PubMed.
- R. M. Silva-Santos, P. Costa-Pinheiro, A. Luis, L. Antunes, F. Lobo, J. Oliveira, R. Henrique and C. Jeronimo, Br. J. Cancer, 2013, 109, 2646–2653 CrossRef CAS PubMed.
- O. A. Kent, R. R. Chivukula, M. Mullendore, E. A. Wentzel, G. Feldmann, K. H. Lee, S. Liu, S. D. Leach, A. Maitra and J. T. Mendell, Genes Dev., 2010, 24, 2754–2759 CrossRef CAS PubMed.
- O. A. Kent, K. Fox-Talbot and M. K. Halushka, Oncogene, 2013, 32, 2576–2585 CrossRef CAS PubMed.
- Y. Ni, L. Meng, L. Wang, W. Dong, H. Shen, G. Wang, Q. Liu and J. Du, Gene, 2013, 517, 197–204 CrossRef CAS PubMed.
- V. Ambros, Curr. Opin. Genet. Dev., 2011, 21, 511–517 CrossRef CAS PubMed.
- R. Vishnubalaji, R. Hamam, M. H. Abdulla, M. A. Mohammed, M. Kassem, O. Al-Obeed, A. Aldahmash and N. M. Alajez, Cell Death Dis., 2015, 6, e1614 CrossRef CAS PubMed.
- Y. Qu, X. Huang, Z. Li, J. Liu, J. Wu, D. Chen, F. Zhao and D. Mu, Am. J. Pathol., 2014, 184, 1541–1549 CrossRef CAS PubMed.
- N. Tsuchiya, M. Izumiya, H. Ogata-Kawata, K. Okamoto, Y. Fujiwara, M. Nakai, A. Okabe, A. J. Schetter, E. D. Bowman, Y. Midorikawa, Y. Sugiyama, H. Aburatani, C. C. Harris and H. Nakagama, Cancer Res., 2011, 71, 4628–4639 CrossRef CAS PubMed.
- Q. F. Xu, Y. W. Pan, L. C. Li, Z. Zhou, Q. L. Huang, J. C. Pang, X. P. Zhu, Y. Ren, H. Yang, H. Ohgaki and S. Q. Lv, Brain Pathol., 2014, 24, 568–583 CrossRef CAS PubMed.
- B. Ling, G. X. Wang, G. Long, J. H. Qiu and Z. L. Hu, J. Cancer Res. Clin. Oncol., 2012, 138, 1355–1361 CrossRef CAS PubMed.
- T. Chiyomaru, S. Yamamura, S. Fukuhara, H. Yoshino, T. Kinoshita, S. Majid, S. Saini, I. Chang, Y. Tanaka, H. Enokida, N. Seki, M. Nakagawa and R. Dahiya, PLoS One, 2013, 8, e70372 CAS.
- J. Iwaki, K. Kikuchi, Y. Mizuguchi, Y. Kawahigashi, H. Yoshida, E. Uchida and T. Takizawa, PLoS One, 2013, 8, e69496 CAS.
- J. Wang, M. Lu, C. Qiu and Q. Cui, Nucleic Acids Res., 2010, 38, D119–D122 CrossRef CAS PubMed.
- K. Woods, J. M. Thomson and S. M. Hammond, J. Biol. Chem., 2007, 282, 2130–2134 CrossRef CAS PubMed.
- S. G. Chaulk, V. J. Lattanzi, S. E. Hiemer, R. P. Fahlman and X. Varelas, J. Biol. Chem., 2014, 289, 1886–1891 CrossRef CAS PubMed.
- I. Heo, C. Joo, Y. K. Kim, M. Ha, M. J. Yoon, J. Cho, K. H. Yeom, J. Han and V. N. Kim, Cell, 2009, 138, 696–708 CrossRef CAS PubMed.
- R. Wu, Y. Jiang, Q. Wu, Q. Li, D. Cheng, L. Xu, C. Zhang, M. Zhang and L. Ye, Tumor Biol., 2014, 35, 8829–8836 CrossRef CAS PubMed.
- A. A. Seyhan, Mol. BioSyst., 2015, 11, 1217–1234 RSC.
- N. Renwick, P. Cekan, P. A. Masry, S. E. McGeary, J. B. Miller, M. Hafner, Z. Li, A. Mihailovic, P. Morozov, M. Brown, T. Gogakos, M. B. Mobin, E. L. Snorrason, H. E. Feilotter, X. Zhang, C. S. Perlis, H. Wu, M. Suarez-Farinas, H. Feng, M. Shuda, P. S. Moore, V. A. Tron, Y. Chang and T. Tuschl, J. Clin. Invest., 2013, 123, 2694–2702 CAS.
- S. Volinia, M. Galasso, M. E. Sana, T. F. Wise, J. Palatini, K. Huebner and C. M. Croce, Proc. Natl. Acad. Sci. U. S. A., 2012, 109, 3024–3029 CrossRef CAS PubMed.
- J. Radojicic, A. Zaravinos, T. Vrekoussis, M. Kafousi, D. A. Spandidos and E. N. Stathopoulos, Cell Cycle, 2011, 10, 507–517 CrossRef CAS PubMed.
- G. Childs, M. Fazzari, G. Kung, N. Kawachi, M. Brandwein-Gensler, M. McLemore, Q. Chen, R. D. Burk, R. V. Smith, M. B. Prystowsky, T. J. Belbin and N. F. Schlecht, Am. J. Pathol., 2009, 174, 736–745 CrossRef CAS PubMed.
- H. Zhang, B. Li, H. Zhao and J. Chang, Int. J. Clin. Exp. Med., 2015, 8, 3034–3043 Search PubMed.
- L. K. Mathew and M. C. Simon, Mol. Cell, 2009, 35, 737–738 CrossRef CAS PubMed.
- X. Huang, L. Ding, K. L. Bennewith, R. T. Tong, S. M. Welford, K. K. Ang, M. Story, Q. T. Le and A. J. Giaccia, Mol. Cell, 2009, 35, 856–867 CrossRef CAS PubMed.
- G. Zheng, Y. Xiong, W. Xu, Y. Wang, F. Chen, Z. Wang and Z. Yan, Oncol. Lett., 2014, 7, 679–684 CAS.
- X. Lou, X. Qi, Y. Zhang, H. Long and J. Yang, J. Surg. Oncol., 2013, 108, 230–235 CrossRef CAS PubMed.
Footnotes |
† Electronic supplementary information (ESI) available: Three supplemental tables are available as a single excel file which include the data from the correlation analysis and primers sequences used for RT-PCR analysis. See DOI: 10.1039/c5mb00415b |
‡ These authors contributed equally to this work. |
|
This journal is © The Royal Society of Chemistry 2016 |