DOI:
10.1039/C5MB00211G
(Paper)
Mol. BioSyst., 2016,
12, 93-101
A deterministic oscillatory model of microtubule growth and shrinkage for differential actions of short chain fatty acids†
Received
25th March 2015
, Accepted 9th November 2015
First published on 9th November 2015
Abstract
Short chain fatty acids (SCFA), principally acetate, propionate, butyrate and valerate, are produced in pharmacologically relevant concentrations by the gut microbiome. Investigations indicate that they exert beneficial effects on colon epithelia. There is increasing interest in whether different SCFAs have distinct functions which may be exploited for prevention or treatment of colonic diseases including colorectal cancer (CRC), inflammatory bowel disease and obesity. Based on experimental evidence, we hypothesised that odd-chain SCFAs may possess anti-mitotic capabilities in colon cancer cells by disrupting microtubule (MT) structural integrity via dysregulation of β-tubulin isotypes. MT dynamic instability is an essential characteristic of MT cellular activity. We report a minimal deterministic model that takes a novel approach to explore the hypothesised pathway by triggering spontaneous oscillations to represent MT dynamic behaviour. The dynamicity parameters in silico were compared to those reported in vitro. Simulations of untreated and butyrate (even-chain length) treated cells reflected MT behaviour in interphase or untreated control cells. The propionate and valerate (odd-chain length) simulations displayed increased catastrophe frequencies and longer periods of MT-fibre shrinkage. Their enhanced dynamicity was dissimilar to that observed in mitotic cells, but parallel to that induced by MT-destabilisation treatments. Antimicrotubule drugs act through upward or downward modulation of MT dynamic instability. Our computational modelling suggests that metabolic engineering of the microbiome may facilitate managing CRC risk by predicting outcomes of SCFA treatments in combination with AMDs.
1. Introduction
Colorectal cancer (CRC) is the fourth most common cancer and second leading cause of cancer-related death in the UK.1 Short chain fatty acids (SCFA) are closely associated with colon epithelia as by-products of anabolic fermentation by gut bacteria.2 The principle SCFAs in the human gut are acetate, propionate, butyrate and valerate. These differ in carbon chain lengths (2, 3, 4 & 5 carbons, respectively). Butyrate is the most researched to date for colon health. It is the preferred energy source for the colonocyte, a potent regulator of cell fate,3 particularly apoptosis,4,5 and is thought to be chemopreventive. SCFAs have been shown to induce acetylation of target proteins, either via histone deacetylation6,7 or by shifting the balance of acetyl-CoA reactions towards acetylation;6,7 however, the mechanistic details of their functions in colonocytes are unclear. Our previous empirical work and others6,8 suggested that carbon chain length may impact on their actions, with odd and even carbon-length SCFAs possessing distinct functions by virtue of different pathways in β-oxidation and entry into the TCA cycle.
Microtubules (MT) are cytoskeletal proteins that perform many critical cellular functions. In addition to maintaining cell structure, motility, intra- and intercellular transport, they are central to accurate formation and control of the mitotic spindle during cell division. MT fibres are composed of typically 13 protofilaments arranged longitudinally, each of which is constructed from αβ-tubulin heterodimers arranged in a head-to-tail manner. This confers the MT fibre with polarity so that polymerisation primarily occurs at the plus end. During assembly, the α-tubulin subunits are bound within the fibre and inaccessible to external proteins; conversely, the β-tubulin subunits are exposed on the surface enabling them to undergo post-translational modifications (PTM) and interact with microtubule associated proteins (MAP), including dynein and kinesin motor proteins. The arrangement of β-tubulin isotypes along the fibre combined with their PTMs has been hypothesised to generate a tubulin code that may direct MT functions and regulate MT behaviour.9,10 In order to perform their molecular functions MTs require the ability to rapidly grow, shrink and change direction in response to cellular cues. These transitions are an essential characteristic of MTs and are termed dynamic instability. The accepted view of MT dynamic instability is the MT capping model.11 Briefly, phosphorylated αβ-tubulin dimers bind to the plus end of the MT fibre to form a protective tubulin–GTP cap. Immediately following polymerisation, the β-tubulin–GTP subunits undergo irreversible hydrolysis to their GDP form. Although this provides the necessary energy to drive MT growth,12 β-tubulin–GDP is unstable and the fibre will rapidly dissociate if the protective GTP cap is not sustained. This is termed catastrophe and occurs when the pool of αβ-tubulin–GTP subunits in the vicinity of the fibre tip falls below a critical concentration.13 Once released into the cytosol, β-tubulin–GDP subunits are free to rephosphorylate, restoring the critical concentration and enabling MT growth to resume, termed rescue. These actions are illustrated in Fig. 1A. Experimental evidence on MT dynamics has primarily been derived from fluorescent tracking microscopy of growing and shrinking MTs in vitro.14–18 The life history of an MT within a cell can range from several minutes to hours and involves extended periods of slow growth, short periods of rapid shrinkage and time spent pausing. Each period of elongation and shortening can consist of multiple catastrophes and rescues (Fig. 1B).17,18 Despite appearing random, MT dynamic instability is a tightly regulated and orchestrated process.
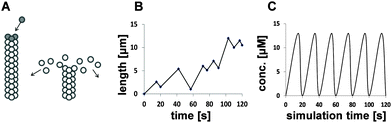 |
| Fig. 1 Qualitative, stochastic and deterministic representations of MT dynamic instability. (A) (left) A growing MT fibre showing the protective cap (dark circles) formed from αβ-tubulin dimers with β-tubulin–GTP subunits; (right) an MT fibre undergoing catastrophe showing rapidly dissociating dimers with hydrolysed β-tubulin–GTP subunits (white circles). (B) The stochastic nature of MT dynamic instability in vivo and in vitro showing the life history of a growing MT as a series of catastrophe and rescue events. (C) A deterministic representation of MT dynamic instability in silico showing regular periodic cycles in MT growth and shrinkage without the need for random perturbations. | |
Polymerisation of a β-tubulin GTP subunit to an MT fibre and its subsequent hydrolysis is a cooperative procedure. Suggested mechanisms include chemical and protein–protein interactions between β-tubulin GTP and GDP subunits;19 conformational changes arising from GTP hydrolysis;20 and facilitated diffusion. The latter mechanism postulated that αβ-tubulin dimers are transported towards the plus end of an MT fibre by virtue of fibre's polarity; as such, the probability that a dimer encounters an MT will increase as the MT lengthens.21
The β-tubulin superfamily comprises of six principle classes thought to have functional significance.22,23 Most β-tubulin isotypes are species, tissue or cell type specific. Their relative proportions within an MT fibre can be regulated by upstream signalling events, suggesting they may influence MT functional activity.24 Consequently, disrupting the fine balance of β-tubulin isotypes can lead to aberrant tubulin behaviour and may have implications in the progression of colonic diseases, including CRC.18,24
Our proteomic analyses investigated proteins differentially regulated in colon cancer cells by treatment with butyrate, propionate and valerate. The results identified several cytoskeletal proteins that were significantly dysregulated:25 all three SCFAs targeted keratin 19; butyrate and propionate targeted actin; and propionate primarily targeted keratins 8 and 18; however, only propionate and valerate significantly targeted β-tubulin isotypes β2c-, β3- and β1-tubulin. These findings were consistent with our high content analyses (HCA) that had revealed valerate and propionate were the most effective in inducing MT breakdown and G2/M cell cycle arrest, despite being weaker effectors in apoptotic functions.5,26 Whereas butyrate induces cytoskeletal breakdown via apoptosis,4,5 these observations suggested that propionate and valerate may act through different mechanisms. Based on this evidence, it was hypothesised that odd- and even-chain SCFAs possessed distinct and unique metabolic functions.
SCFAs act by promoting post-translational acetylation of target proteins. Bioinformatic searches of Reactome and SABiosciences24,27,28 indicated that the activities of several transcriptional regulators (TR) associated with β2c-, β3- and β1-tubulin genes (RFX, PPAR, NSFR and NF-κB) could be altered by acetylation. Histone acetylation also induces chromatin remodelling to promote transcription of target genes and can itself be influenced by upstream acetylation events.29,30 These results suggested that treatment of colonocytes with SCFAs altered the regulation of specific genes, including overexpression of β2c- and β3-tubulin and suppression of β1-tubulin transcription via acetylation of NF-κB.31,32 Both β2c- and β3-tubulin have been implicated in MT destabilisation;24 although less is known about the role of β1-tubulin in MT dynamics.
Computational modelling has contributed to a better understanding of MT functions20,33–36 and the biophysical details of MT dynamic instability. Various approaches have been adopted, including Monte Carlo simulations;20,33 simplified coarse-grained stochastic models;35 one- and three-dimensional modelling;37 and models focusing on different types of lattices and MT fibre–fibre interactions.33,36 The term “structural plasticity” invokes mechanisms more dependent on the structural conformations of bound subunits than their chemical states.19 Simulated systems of dynamic MTs have also advanced understanding of their associations with MAPs, the collective behaviour of MTs and motors, and have been used to replicate mitotic events.38,39 The model of Bayley et al. (1990) was pivotal in demonstrating the validity of the lateral-cap model, in which β-tubulin–GTP assembly is a cooperative stochastic event that is fully coupled with β-tubulin hydrolysis.40 Their hypothesis was supported by Pedigo and Williams (2002) who showed that conformational changes arising from β-tubulin–GTP hydrolysis directly after association was best described using kinetic principles of lattice interactions.20 Computational models have been proposed as a basis for predicting the actions of antimicrotubule drugs (AMDs).40,41 This had particular relevance for our investigation which aimed to explore the links between SCFAs, transcriptional regulation of β-tubulin isotypes, MT integrity and performance, and the potential use of SCFAs in adjuvant therapies in CRC.
A common approach to modelling systems of this nature is to use ordinary differential equations (ODEs). These present biological systems as continuous and deterministic and assume that randomness is not an important factor in the system of interest. An alternative approach is to use stochastic models if there is deemed to be inherent variability within the biological system; a stochastic approach will be used in scenarios whereby a small number of molecules are suggested to be involved in discrete random collisions, such as during the expression of a single gene. The main limitation of stochastic models is that they have a tendency to be computationally intensive, as reviewed in McAuley et al. (2013).42 In this study, we sought to develop a minimal deterministic model based on experimental evidence that might explain or validate our hypothesis:25 that different SCFAs imposed differential effects on the balance of β-tubulin isotypes and their impact on MT integrity. A comparison between the stochastic behaviour of MTs in vivo and in vitro and a deterministic representation in silico is presented in Fig. 1B and C.
2. Methods, kinetic theory and simulations
2.1 Qualitative model and design
The rationale underpinning the selection of a deterministic model made up of ODEs was based on our experimental observations. These had demonstrated that this system was deterministic and the inherent variability was negligible. The schematic diagram in Fig. 2 shows the key events in the hypothesised pathway. Two scenarios are presented: the first represents untreated cells displaying normal MT behaviour (Fig. 2A); the second represents SCFA-treated cells promoting perturbation of MT dynamic instability (Fig. 2B). The first three reactions (k1–k3) are involved in the synthesis of the differentially regulated β-tubulin isotypes (β2c-, β3- and β1-tubulin); the next three reactions (k4–k6) are involved in MT dynamic instability based on the capping model.11 With the exception of the perturbation step, the two parts of the model are distinct; therefore, no feedback loop has been included between the synthesis of βeta in the first part and the concentration of free β-tubulin subunits (tuGTP) in the second part. This was based on the assumption that the total number of β-tubulin subunits (free and bound) in the system is fixed but the relative proportions of stabilising and destabilising isotypes can shift. Consequently, the net upregulation of the three targeted β-tubulin isotypes (βeta) will be compensated by a net downregulation distributed among the remaining β-tubulin isotypes. (Based on our experimental results, none of the other β-tubulin isotypes was individually significantly downregulated.)
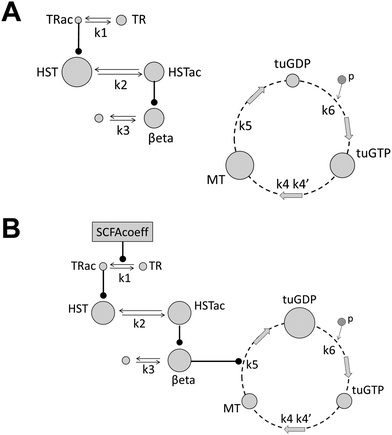 |
| Fig. 2 Schematic diagram representing the hypothesised effects of SCFA treatments on microtubule dynamic instability in colon cancer cells. (A) Butyrate (an even-chain SCFA) has little or no impact on the regulatory pathway (k1→k3) leading to normal synthesis of the three target β-tubulin isotypes (βeta) relative to untreated cells. Therefore MT dynamic instability (k4→k6) remains unperturbed. (B) In contrast, treatment with propionate or valerate (odd-chain SCFAs) induces dysregulation of βeta leading to perturbation of MT dynamicity by promoting depolymerisation of the MT fibre (k6). Arrows show forward and backward reactions; round-ended arrows indicate the target reaction is promoted by the product of the preceding reaction; changes in the concentrations of reactants are represented by changes in the diameters of the circles. The following abbreviations are adopted: TR, transcriptional regulators; HST, histones at the target genes; βeta, the combined weighted fold-change of β3-, β2c- and β1- tubulin isotypes; MT, the MT fibre; tuGTP and tuGDP, total free phosphorylated and hydrolysed β-tubulin subunits, respectively; ac refers to the acetylated forms of TR and HST; p indicates phosphorylation; SCFAcoeff, the SCFA-activation coefficient specific to the hypothesised pathway. | |
The pathway in Fig. 2B (treatment with odd-chain SCFAs) is described below:
• [k1–k3]: Treatment with odd-chain SCFAs drives acetylation of the target transcriptional regulators (TR); the acetylated TRs induce histone acetylation at β2c-, β3- and β1-tubulin genes (denoted βeta); thereby enhancing or extending synthesis of these β-tubulin isotypes.
• [k4–k6]: Free phosphorylated β-tubulin–GTP subunits co-operatively bind to the plus end of the MT fibre and are rapidly hydrolysed to their unstable β-tubulin–GDP form; dissociation of the MT fibre (catastrophe) releases the bound β-tubulin–GDP subunits back into the cytosol where they are re-phosphorylated, enabling MT re-polymerisation (rescue) to resume.
• The cycle then repeats.
The effect of raising the proportion of these three MT-destabilising β-tubulin isotypes in the system was to drive depolymerisation of the MT fibre, thereby inducing catastrophe. In contrast, untreated simulations or treatment with even-chain SCFAs (Fig. 2A) had little impact on the acetylation of target TRs, thereby preserving MT dynamic instability.
The modelled reactions obeyed kinetic rules and the relationships between the reactants were based on published observables and experimental data. Within the specifications of the modelled system, and due to the complexities of biological processes, each reaction in the pathway represented a number of intermediate sub-reactions. This approach of model reduction meant that the kinetic variables of the combined reactions could not be defined.43 Reaction rates can also be altered by post-translational modifications.44 As a result, parameters were set within biologically feasible limits derived from similar reactions reported in curated biomodels.45 However, computational strategies have demonstrated that modelling can be successful in gaining valuable insights into biological mechanisms even under conditions where kinetic or reactant data are limited or unknown.46,47
2.2 Initial concentrations of reactants
The initial reactant concentrations are summarised in the ESI† (Table S1). Our proteomic data was used to define the steady state concentrations of βeta relative to untreated values, (1.07, 1.34 and 1.40 for butyrate, propionate and valerate, respectively). To match these values, the corresponding SCFA activation coefficients (SCFAcoeff) were manually adjusted to 1.2, 2.8, and 3.6, respectively. This is an accepted approach that has been described previously.39 The total concentration of tubulin in the system was set at 10.0 μM based on typical values reported in the literature.11,14,18,48 Although the concentrations of MTs, and bound and free β-tubulin subunits continually fluctuated, the overall concentration was assumed to be constant within the fixed volume of the in silico cell. Therefore, the initial concentrations could be arbitrarily set as MT = 5.5 μM; tuGDP = 3 μM; tuGTP 1.5 μM. These were informed estimates based on the nature of MT dynamic instability. Sensitivity analyses were performed to verify that the estimated values and assumptions were reasonable and appropriate, and to identify those parameters that would have the greatest impact of the model outcomes.
2.3 Kinetic rate laws and ODEs
The rate law interpretation was set as deterministic. The kinetic reactions, rate constants, rate laws and ODEs are summarised in the ESI† (Tables S2 and S3). The three steps of MT dynamic instability (β-tubulin phosphorylation, rescue and catastrophe; Fig. 2B) were inspired by Tyson's two-species model of cell division that displays spontaneous oscillations within set constraints.49 This in turn had been based on the hypothetical model of a mitotic oscillator50 and theoretical Brusselator model of chemical oscillations.51,52 In this study we used a similar approach to construct a minimal oscillating model to describe MT dynamics in which the key requirement was for cooperative/non-linear kinetics within the cycle. By reducing MT dynamic instability to its three fundamental steps required us to ignore many of the additional levels of complexity, such as protein structure and memory effects.53 Neglecting stochastic effects was also a major simplification even though the apparent randomness of dynamic instability is likely to be an important driving force in the true picture. However, the aim of this work was to show that cooperative kinetics for MT growth, dependant on a minimal concentration of free β-tubulin–GTP subunits, and a first order dissociation, could give credible results.
2.4 Simulations and output parameters
The key parameters of MT dynamicity are growth and shrinkage periods and rates and catastrophe and rescue frequencies; catastrophe frequency is defined as the number of transitions from growth to shrinkage relative to the total elongation time; rescue frequency is defined as the number of transitions from shrinkage to growth relative to the total shrinkage time.14,17 The time MTs spend pausing was beyond the scope of this model and not included in the analyses. As described earlier, an MT can undergo multiple sub-events of growth and shrinkage, catastrophes and rescues during its life history. These have been depicted as micro-oscillations.
The ratios of catastrophe:rescue frequencies, growth:shrinkage rates and growth:shrinkage times were calculated from the temporal data in order for equivalent comparisons to be made against the wide range of experimental data.
The simulations were undertaken in COPASI v4.7.54 The simulation times ranged from 0 to 600–1000 s and data were recorded at intervals of 0.5 s. This provided a sufficient number of oscillations at a sensitivity suitable for analysis. The MT dynamicity parameters were evaluated following an initial delay of 500 s to allow β-tubulin synthesis to reach steady state (Fig. 3A).
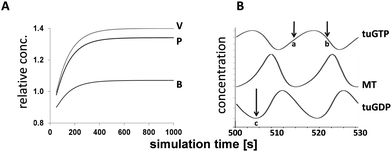 |
| Fig. 3 Temporal simulations showing the relationships between microtubule fibres and subunits in the hypothesised pathway. (A) Treatment with propionate and valerate (odd-chain SCFAs) significantly promotes synthesis of target β-tubulin isotypes (βeta: β2c-tubulin, β3-tubulin, β1-tubulin); whereas butyrate has little influence on βeta synthesis relative to untreated cells. The curves show the relative concentrations of βeta following treatment with valerate (upper), propionate (centre) and butyrate (bottom). Steady state is achieved at approximately 500 s; the simulation time is 1000 s. (B) The stacked curves show the inter-relationships between MT fibres (MT, middle) free β-tubulin GTP and GDP subunits (tuGTP and tuGDP, top and bottom, respectively): the rapid gain and slow decline in free tuGDP is reflected by a rapid loss and slow increase in free tuGTP and consequently rapid shrinkage (catastrophe) and slow growth (rescue) in MT fibres; (a) indicates the point of rescue; (b) and (c) denote the critical concentration of tuGTP at which the protective cap at the end of the MT fibre is established and lost, respectively. Simulation time, 500–530 s. | |
3. Results
3.1 MT dynamicity parameters for SCFA treatments in silico
The inter-relationships between MT fibres and β-tubulin GTP and GDP subunits (MT, tuGTP and tuGDP, respectively; Fig. 3B): MT polymerisation commenced when the concentration of free tuGTP reached a critical concentration (cc); MT dissociation was triggered when the concentration of tuGTP fell below the cc; the subsequent decrease in MT concentration (representing length) was reflected by an increase in the concentration of free tuGDP. This model was based on the assumption that the concentration of free β-tubulin (tuGTP and tuGDP) was not constant but fluctuated in response to MT fibre growth and shrinkage. This implies that the concentration of free β-tubulin–GTP may play a role in MT dynamic instability. Although this supposition is relatively novel, it is not unprecedented: Janulevicius et al. (2006) explored this hypothesis through Monte Carlo simulations in the context of small cellular compartments and fluctuations in the concentration of free tubulin, and by extension, situations that affect the probability of MT association.55 Their model was equated to neuronal growth cones in vivo; our model was based on changes in the local concentration of free β-tubulin–GTP at the fibre tip due to facilitated diffusion,21 and thereby the probability of association.
The temporal simulations for MTs for each SCFA treatment are shown in Fig. 4A. The mean periods of the micro-oscillations were approximately 20 s. This was consistent with published values in vitro and in vivo for fluorescently tagged MTs.17,18,56 Qualitative examination of the profiles showed that there was little difference between MT dynamicity in untreated cells in silico and those treated with butyrate (Fig. 4A). In both cases, the cycle of dynamic instability persisted over the simulation time and the mean concentration of MTs was 94% for butyrate relative to untreated values. In contrast, the MT profiles for propionate and valerate treatments showed increasing dampening of the oscillations, reflecting suppression of MT dynamic instability, and a reduction in the mean concentrations of MTs to 74% and 71% relative to untreated values, respectively. Fig. 4B shows the corresponding rate-profiles in untreated cells and valerate treated cells. These include the rates of concentration changes for MTs and free GTP and GDP β-tubulin subunits. Together, these results demonstrated that odd- and even-chain SCFAs induced distinct actions on MT dynamic instability. The odd-chain SCFAs effectively abolished MT dynamic instability in silico, reflecting dissociation of the fibres and loss of MT integrity in vitro, consistent with our experimental iTRAQ and HCA observations in HCT116 colon cancer cells. Comparisons between the simulated MT-dynamicity values for the three SCFAs relative to untreated values (Table 1) showed that the catastrophe frequencies were increased, the rescue frequencies decreased, the growth:shrinkage times increased and the growth:shrinkage rates decreased in the order butyrate to propionate to valerate, with valerate having the greatest effect.
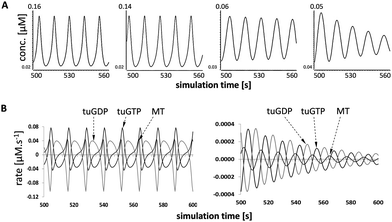 |
| Fig. 4 Temporal simulations showing MT dynamic behaviour under different SCFA treatments. (A) Micro-oscillations depicting catastrophe and rescue events in simulated SCFA treatments during 60 s of the MT's lifespan: (left to right) untreated; butyrate (even-chain, 4 carbons); propionate (odd-chain, 3 carbons); and valerate (odd-chain, 5 carbons). There is little change in MT dynamicity between untreated and butyrate treated cells and MT dynamic instability persists over time; however, treatments with propionate and valerate reduce growth periods, increase shrinkage periods and suppress MT dynamic instability. (B) The rates of changes in MTs and free GDP and GTP β-tubulin (tuGDP and tuGTP) concentrations for untreated and valerate treated simulations show the predominance of second-order cooperative association in untreated MTs (left) and the shift towards first-order dissociation in valerate treated MTs (right). | |
Table 1 Fold-changes in the MT-dynamicity parameters for simulated treatments with SCFAs relative to untreated values in silico
MT dynamicity parameter |
Butyrate |
Propionate |
Valerate |
Catastrophe frequency [s−1] |
0.98 |
1.08 |
1.24 |
Rescue frequency [s−1] |
0.97 |
0.83 |
0.72 |
Catastrophe:rescue frequency |
1.01 |
1.29 |
1.73 |
|
Relative time growing |
1.00 |
0.94 |
0.80 |
Relative time shrinking |
1.01 |
1.21 |
1.39 |
Growth:shrinkage times |
0.99 |
0.77 |
0.58 |
|
Growth rate [μM min−1] |
0.92 |
0.13 |
0.001 |
Shrinkage rate [μM min−1] |
0.91 |
0.10 |
0.000 |
Growth:shrinkage rates |
1.01 |
1.29 |
1.45 |
3.2 Comparisons between MT dynamicity parameters in silico and experimental data in vitro
In order to determine whether the modelled outcomes could plausibly be attributed to MT destabilising treatments in vitro, they were compared to experimental measurements of MT dynamicity parameters in cells at different phases or undergoing different treatments. Experimental observations can yield a wide range of values for MT dynamicity dependent on species, tissue or cell type and external conditions; however they tend to report similar trends and ratios. For this reason, data from two representative reports were selected for interphase and mitotic epithelial cells,17 and MT-destabilising and MT-stabilising treatments in epithelial cells18 to minimise compounding effects. The experimental values are summarised in the ESI† (Table S4). The histograms in Fig. 5 indicate that MT dynamicity ratios in untreated and butyrate treated cells in silico most closely matched those in interphase cells in vitro for growth and shrinkage but were closer to stabilised-control cells for catastrophe and rescue frequencies (Fig. 5A). Whereas, propionate and valerate treated MTs in silico displayed similarities to both mitotic and destabilised MTs in vitro for growth and shrinkage times and rates, but only to MT-destabilising treatments for catastrophe and rescue frequencies (Fig. 5B). Both mitotic and depolymerising MTs are dynamically active; however, these simulations strongly suggested that colon cancer cells treated with odd-chain SCFAs undergo MT-destabilisation as opposed to enhanced mitosis. These results supported our proposition that odd-chain SCFAs at above physiological concentrations may act as anti-microtubule agents.
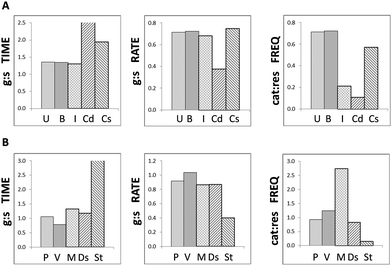 |
| Fig. 5 Goodness of fit of model to empirical data. Comparisons between model predictions and published observations of MTs in vitro show that (A) simulations for untreated and butyrate (even-chain SCFA) treated MTs in silico most closely reflect those in interphase cells (I) for growth:shrinkage [g:s] periods and rates in vitro, and control cells in stabilising experiments (C-s) for catastrophe:rescue [cat:res] frequencies; (B) propionate and valerate (odd-chain SCFAs) treated MTs in silico most closely reflect MTs undergoing destabilising treatments (Ds) in vitro for all three MT dynamicity ratios; although they bear close similarities to mitotic cells (M) for growth:shrinkage periods and rates, they exhibit highly different behaviour from mitotic cells undergoing catastrophe and rescue. Note, the bars for growth:shrinkage times for Cd and St extend beyond the scale of the charts. [U, B, P, V: untreated, butyrate, propionate and valerate treatments in silico, respectively; I, M, Cs, Cd, St, Ds: interphase, mitotic, control cells for stabilising and destabilising treatments, stabilising and destabilising treatments in vitro, respectively.] | |
3.3 Sensitivity analyses: influences of initial concentrations and kinetic rate constants on model behaviour
Sensitivity analyses were carried out using the generic sensitivity framework in COPASI to assess the impacts of the initial reactant concentrations and kinetic rate constants on the reaction fluxes and temporal concentrations of the reactants, respectively. The summarised and scaled results are given in the ESI† (Table S5) and shown graphically in Fig. 6A. The parameters with the greatest influences upstream of MT dynamic instability (k1→k3) were the initial concentration of histones (HST) and the forward kinetic rate constant for synthesis (k3f) of βeta (the three targeted β-tubulin isotypes): a 10% increase in the initial concentration of HST resulted in complete loss of MT dynamicity (Fig. 6B, centre); whereas a 10% increase in the forward rate of βeta synthesis led to over-stabilisation of the MTs (Fig. 6B, right), effectively negating the destabilising effects of valerate treatment (Fig. 6B, left). The dynamicity control factor that had the greatest influence in the cycle of MT dynamic instability was the rate of rephosphorylation of free tuGDP subunits (k6) (Fig. 6A).
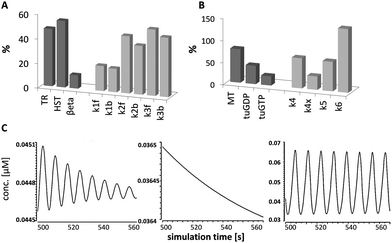 |
| Fig. 6 Sensitivity analyses showing the effects of small changes in the initial model parameters on the overall outcome of the model simulations. The histograms show the summarised scaled sensitivities of the modelled system (y-axes) to changes in each of the initial reactant concentrations or kinetic reaction rates (x-axes) in (A) the βeta synthesis pathway and (B) the MT dynamic instability cycle. The dark coloured bars represent initial concentrations of the reactants; the pale coloured represent kinetic rates (k-rates). The results show that the initial concentration of histones (HST) and the forward rate of βeta synthesis (k3f) have the greatest influences on βeta synthesis; whereas the initial concentration of MTs and the rate of phosphorylation of free tuGDP (k6) have the greatest influences on MT dynamic instability. (C) Representative simulations of MTs undergoing valerate treatment showing the effects of 10% changes in the HST and k3f: (left) no change in either parameter; (centre) a 10% changes in the initial histone concentration (HST) leads to complete loss of MT dynamicity; (right) a 10% change in the forward rate of βeta synthesis (k3f) leads to overstabilisation of MT fibres. The effects of small changes in k6 are not shown due to the inherent instability of the oscillatory system not directly linked to SCFA input. There is no perturbation in untreated systems. The simulation times are 500–600 s. | |
4. Discussion
Antimicrotubule drugs have proved highly successful in the treatment of multiple cancers and other diseases, such as motor neuron disease.57,58 The review by Jordan and Wilson (2004) describes the ability of AMDs to induce apoptosis via mitotic arrest by suppressing MT dynamic instability.59 It was also suggested that cancer cells may dysregulate expression of β-tubulin isotypes in order to enhance resistance to AMDs.60 To date, SCFAs have not been directly identified as antimitotic agents, and experimental investigations have primarily focused on their ability to induce cell death and subsequent cytoskeletal breakdown via mechanisms that include alterations in pro- and anti-apoptotic proteins and tumour suppressor genes through Sp1 and Bcl-2 family proteins.26,61 The importance of MAPs as the driving forces in dynamic instability is becoming increasingly evident.55 This implies that the different β-tubulin isotypes also play critical roles through recruitment of MAPs. We previously reported that propionate and valerate, both odd-chain SCFAs, dysregulated the balance of β-tubulin isotypes towards those associated with MT depolymerisation, and that this was concurrent with impairment of MT structural integrity. To test the plausibility and potential implications of this hypothesis, we constructed a speculative model to explore the possible impact SCFAs might have on MT dynamic instability in colon cancer cells. The initial results showed that simulations of MT dynamic instability in untreated and butyrate treated cells in silico resembled MT behaviour observed in interphase or untreated control cells in vitro;17 whereas simulations of propionate and valerate treatments (odd-chain SCFAs) showed close similarities to MTs under destabilising treatments in vitro.18 The perturbation target for SCFA input in the model was set at the dissociation step of MT dynamic instability; therefore, increased levels of catastrophe relative to rescue had been anticipated. However, the key finding of this study was that the simulated outcomes were closer to those reported for MT-destabilising treatments in vitro than mitotic cells, despite both being associated with high levels of MT dynamicity. This demonstrated that propionate and valerate may possess anti-mitotic functions and suggested a potential role for odd-chain SCFAs as anti-mitotic agents, enabling AMDs to be administered at sub-optimal doses while maintaining efficacy and inhibiting resistance.
5. Conclusions
In combination with earlier experimental results,25 the model presented here supports the hypothesis that propionate and valerate at supraphysiological concentrations trigger an anti-mitotic pathway in colon cancer cells that is unique to odd-chain SCFAs: the simulations provoked changes in MT dynamicity parameters that closely paralleled trends reported in cells undergoing MT-destabilising treatments.18 This study has demonstrated that computational dynamic modelling can make a valuable contribution to such investigations, including the role of SCFAs in the treatment of CRC.
Acknowledgements
JK was supported by an EPSRC studentship (under grant EP/E036252/1).
Notes and references
- Cancer-Research-UK. Cancer Research UK: CancerStats Key Facts 2013 internet, 2013, cited 2013, available from: http://www.cancerresearchuk.org.
- J. H. Cummings, E. R. Beatty, S. M. Kingman, S. A. Bingham and H. N. Englyst, Digestion and physiological properties of resistant starch in the human large bowel, Br. J. Nutr., 1996, 75(5), 733–747 CrossRef CAS PubMed.
- L. H. Augenlicht, J. M. Mariadason, A. Wilson, D. Arango, W. C. Yang and B. G. Heerdt,
et al., Short chain fatty acids and colon cancer, J. Nutr., 2002, 132(12), 3804S–3808S Search PubMed.
- S. Siavoshian, H. M. Blottiere, E. Le Foll, B. Kaeffer, C. Cherbut and J. P. Galmiche, Comparison of the effect of different short chain fatty acids on the growth and differentiation of human colonic carcinoma cell lines in vitro, Cell Biol. Int., 1997, 21(5), 281–287 CrossRef CAS PubMed.
- B. F. Hinnebusch, S. Meng, J. T. Wu, S. Y. Archer and R. A. Hodin, The effects of short-chain fatty acids on human colon cancer cell phenotype are associated with histone hyperacetylation, J. Nutr., 2002, 132(5), 1012–1017 CAS.
- B. M. Corfe, Hypothesis: butyrate is not an HDAC inhibitor, but a product inhibitor of deacetylation, Mol. BioSyst., 2012, 8, 4 RSC.
- E. P. Candido, R. Reeves and J. R. Davie, Sodium butyrate inhibits histone deacetylation in cultured cells, Cell, 1978, 14(1), 105–113 CrossRef CAS PubMed.
- S. M. Astbury and B. M. Corfe, Uptake and Metabolism of the Short-Chain Fatty Acid Butyrate, a Critical Review of the Literature, Curr. Drug Metab., 2012, 13(6), 815–921 CrossRef CAS PubMed.
- P. Verdier-Pinard, E. Pasquier, H. Xiao, B. Burd, C. Villard and D. Lafitte,
et al., Tubulin proteomics: towards breaking the code, Anal. Biochem., 2009, 384(2), 197–206 CrossRef CAS PubMed.
- K. J. Verhey and J. Gaertig, The tubulin code, Cell Cycle, 2007, 6(17), 2152–2160 CrossRef CAS PubMed.
- D. N. Drechsel, A. A. Hyman, M. H. Cobb and M. W. Kirschner, Modulation of the dynamic instability of tubulin assembly by the microtubule-associated protein tau, Mol. Biol. Cell, 1992, 3(10), 1141–1154 CrossRef CAS PubMed.
- A. Desai and T. J. Mitchison, Microtubule polymerization dynamics, Annu. Rev. Cell Dev. Biol., 1997, 13, 83–117 CrossRef CAS PubMed.
- C. Bonfils, N. Bec, B. Lacroix, M. C. Harricane and C. Larroque, Kinetic analysis of tubulin assembly in the presence of the microtubule-associated protein TOGp, J. Biol. Chem., 2007, 282(8), 5570–5581 CrossRef CAS PubMed.
- R. A. Walker, E. T. Obrien, N. K. Pryer, M. F. Soboeiro, W. A. Voter and H. P. Erickson,
et al., Dynamic instability of individual microtubules analyzed by video light-microscopy – rate constants and transition frequencies, J. Cell Biol., 1988, 107(4), 1437–1448 CrossRef CAS PubMed.
- L. D. Belmont, A. A. Hyman, K. E. Sawin and T. J. Mitchison, Real-time visualization of cell cycle-dependent changes in microtubule dynamics in cytoplasmic extracts, Cell, 1990, 62(3), 579–589 CrossRef CAS PubMed.
- L. Cassimeris, Accessory protein regulation of microtubule dynamics throughout the cell cycle, Curr. Opin. Cell Biol., 1999, 11(1), 134–141 CrossRef CAS PubMed.
- N. M. Rusan, C. J. Fagerstrom, A. M. C. Yvon and P. Wadsworth, Cell cycle-dependent changes in microtubule dynamics in living cells expressing green fluorescent protein-alpha tubulin, Mol. Biol. Cell, 2001, 12(4), 971–980 CrossRef CAS PubMed.
- S. L. Kline-Smith and C. E. Walczak, The microtubule-destabilizing kinesin XKCM1 regulates microtubule dynamic instability in cells, Mol. Biol. Cell, 2002, 13(8), 2718–2731 CrossRef CAS PubMed.
- H. Y. Kueh and T. J. Mitchison, Structural plasticity in actin and tubulin polymer dynamics, Science, 2009, 325(5943), 960–963 CrossRef CAS PubMed.
- S. Pedigo and R. C. Williams, Concentration dependence of variability in growth rates of microtubules, Biophys. J., 2002, 83(4), 1809–1819 CrossRef CAS PubMed.
- A. Mechulam, K. G. Chernov, E. Mucher, L. Hamon, P. A. Curmi and D. Pastre, Polyamine Sharing between Tubulin Dimers Favours Microtubule Nucleation and Elongation via Facilitated Diffusion, PLoS Comput. Biol., 2009, 5(1), e1000255 Search PubMed.
- P. G. McKean, S. Vaughan and K. Gull, The extended tubulin superfamily, J. Cell Sci., 2001, 114(Pt 15), 2723–2733 CAS.
- R. F. Luduena, Are tubulin isotypes functionally significant, Mol. Biol. Cell, 1993, 4(5), 445–457 CrossRef CAS PubMed.
- L. J. Leandro-Garcia, S. Leskela, I. Landa, C. Montero-Conde, E. Lopez-Jimenez and R. Leton,
et al., Tumoral and Tissue-Specific Expression of the Major Human beta-Tubulin Isotypes, Cytoskeleton, 2010, 67(4), 214–223 CrossRef CAS PubMed.
- J. Kilner, J. S. Waby, J. Chowdry, A. Q. Khan, J. Noirel and P. C. Wright,
et al., A proteomic analysis of differential cellular responses to the short-chain fatty acids butyrate, valerate and propionate in colon epithelial cancer cells, Mol. BioSyst., 2012, 8(4), 1146–1156 RSC.
- B. G. Heerdt, M. A. Houston and L. H. Augenlicht, Short-chain fatty acid-initiated cell cycle arrest and apoptosis of colonic epithelial cells is linked to mitochondrial function, Cell Growth Differ., 1997, 8(5), 523–532 CAS.
- REACTOME: a manually curated and peer-reviewed pathway database, Reactome internet, 2010, cited 2012, available from: http://www.reactome.org.
- Transcription Regulator Search Portal internet. Qiagen Inc. USA internet, 2010, cited 2012 Aug 01, available from: http://www.sabiosciences.com/home.php.
- Y. Naruse, T. Aoki, T. Kojima and N. Mori, Neural restrictive silencer factor recruits mSin3 and histone deacetylase complex to repress neuron-specific target genes, Proc. Natl. Acad. Sci. U. S. A., 1999, 96(24), 13691–13696 CrossRef CAS.
- P. Kurschat, D. Bielenberg, M. Rossignol-Tallandier, A. Stahl and M. Klagsbrun, Neuron restrictive silencer factor NRSF/REST is a transcriptional repressor of neuropilin-1 and diminishes the ability of semaphorin 3A to inhibit keratinocyte migration, J. Biol. Chem., 2006, 281(5), 2721–2729 CrossRef CAS PubMed.
- L. F. Chen and W. C. Greene, Assessing acetylation of NF-kappa B, Methods, 2005, 36(4), 368–375 CrossRef CAS PubMed.
- N. T. Crump, C. A. Hazzalin, E. M. Bowers, R. M. Alani, P. A. Cole and L. C. Mahadevan, Dynamic acetylation of all lysine-4 trimethylated histone H3 is evolutionarily conserved and mediated by p300/CBP, Proc. Natl. Acad. Sci. U. S. A., 2011, 108(19), 7814–7819 CrossRef CAS PubMed.
- S. R. Martin, M. J. Schilstra and P. M. Bayley, Dynamic Instability of Microtubules – Monte-Carlo Simulation and Application to Different Types of Microtubule Lattice, Biophys. J., 1993, 65(2), 578–596 CrossRef CAS PubMed.
- D. Hall, The effects of Tubulin denaturation on the characterization of its polymerization behavior, Biophys. Chem., 2003, 104(3), 655–682 CrossRef CAS PubMed.
- I. V. Gregoretti, G. Margolin, M. S. Alber and H. V. Goodson, Insights into cytoskeletal behavior from computational modeling of dynamic microtubules in a cell-like environment, J. Cell Sci., 2006, 119(Pt 22), 4781–4788 CrossRef CAS PubMed.
- E. Karsenti, F. Nedelec and T. Surrey, Modelling microtubule patterns, Nat. Cell Biol., 2006, 8(11), 1204–1211 CrossRef CAS PubMed.
- L. Brun, B. Rupp, J. J. Ward and F. Nedelec, A theory of microtubule catastrophes and their regulation, Proc. Natl. Acad. Sci. U. S. A., 2009, 106(50), 21173–21178 CrossRef CAS PubMed.
- B. Ibrahim, S. Diekmann, E. Schmitt and P. Dittrich, In-silico modeling of the mitotic spindle assembly checkpoint, PLoS One, 2008, 3(2), e1555 Search PubMed.
- A. K. Caydasi, M. Lohel, G. Gruenert, P. Dittrich, G. Pereira and B. Ibrahim, A dynamical model of the spindle position checkpoint, Mol. Syst. Biol., 2012, 8, 582 CrossRef PubMed.
- P. M. Bayley, M. J. Schilstra and S. R. Martin, Microtubule dynamic instability – numerical-simulation of microtubule transition properties using a lateral cap model, J. Cell Sci., 1990, 95, 33–48 Search PubMed.
- G. A. Buxton, S. L. Siedlak, G. Perry and M. A. Smith, Mathematical modeling of microtubule dynamics: insights into physiology and disease, Prog. Neurobiol., 2010, 92(4), 478–483 CrossRef CAS PubMed.
- M. T. McAuley, C. J. Proctor, B. M. Corfe, G. J. Cuskelly and K. M. Mooney, Nutrition Research and the Impact of Computational Systems Biology, J. Comput. Sci. Syst. Biol., 2013, 6(5), 271–285 Search PubMed.
- J. Wu, B. Vidakovic and E. O. Voit, Constructing stochastic models from deterministic process equations by propensity adjustment, BMC Syst. Biol., 2011, 5, 187 CrossRef PubMed.
- P. Cheung, K. G. Tanner, W. L. Cheung, P. Sassone-Corsi, J. M. Denu and C. D. Allis, Synergistic coupling of histone H3 phosphorylation and acetylation in response to epidermal growth factor stimulation, Mol. Cell, 2000, 5(6), 905–915 CrossRef CAS PubMed.
- C. Li, M. Donizelli, N. Rodriguez, H. Dharuri, L. Endler and V. Chelliah,
et al., BioModels Database: An enhanced, curated and annotated resource for published quantitative kinetic models, BMC Syst. Biol., 2010, 4, 92 CrossRef PubMed.
- S. J. Wilkinson, N. Benson and D. B. Kell, Proximate parameter tuning for biochemical networks with uncertain kinetic parameters, Mol. BioSyst., 2008, 4(1), 74–97 RSC.
- R. J. Dimelow and S. J. Wilkinson, Control of translation initiation: a model-based analysis from limited experimental data, J. R. Soc., Interface, 2009, 6(30), 51–61 CrossRef CAS PubMed.
- M. Caplow and J. Shanks, Induction of microtubule catastrophe by formation of tubulin – gdp and apotubulin subunits at microtubule ends, Biochemistry, 1995, 34(48), 15732–15741 CrossRef CAS PubMed.
- J. J. Tyson, Modeling the cell-division cycle – cdc2 and cyclin interactions, Proc. Natl. Acad. Sci. U. S. A., 1991, 88(16), 7328–7332 CrossRef CAS.
- J. Tyson and S. Kauffman, Control of mitosis by a continuous biochemical oscillation: Synchronization; spatially inhomogeneous oscillations, J. Math. Biol., 1975, 1(4), 289–310 CrossRef.
- I. Prigogine and R. Lefever, Symmetry breaking instabilities in dissipative systems. II, J. Chem. Phys., 1968, 48(4), 1695–1700 CrossRef.
- J. Tyson, Some Further Studies of Nonlinear Oscillators in Chemical Systems, J. Chem. Phys., 1973, 58, 3919–3930 CrossRef CAS.
- G. J. Brouhard, Dynamic instability 30 years later: complexities in microtubule growth and catastrophe, Mol. Biol. Cell, 2015, 26(7), 1207–1210 CrossRef CAS PubMed.
- S. Hoops, S. Sahle, R. Gauges, C. Lee, J. Pahle and N. Simus,
et al., COPASI–a COmplex PAthway SImulator, Bioinformatics, 2006, 22(24), 3067–3074 CrossRef CAS PubMed.
- A. Janulevicius, J. van Pelt and A. van Ooyen, Compartment volume influences microtubule dynamic instability: a model study, Biophys. J., 2006, 90(3), 788–798 CrossRef CAS PubMed.
- H. Bolterauer, H. J. Limbach and J. A. Tuszyński, Models of assembly and disassembly of individual microtubules: stochastic and averaged equations, J. Biol. Phys., 1999, 25(1), 1–22 CrossRef CAS PubMed.
- S. Don, N. M. Verrills, T. Y. Liaw, M. L. Liu, M. D. Norris and M. Haber,
et al., Neuronal-associated microtubule proteins class III beta-tubulin and MAP2c in neuroblastoma: role in resistance to microtubule-targeted drugs, Mol. Cancer Ther., 2004, 3(9), 1137–1146 CAS.
- P. Singh, K. Rathinasamy, R. Mohan and D. Panda, Microtubule assembly dynamics: an attractive target for anticancer drugs, IUBMB
Life, 2008, 60(6), 368–375 CAS.
- M. A. Jordan and L. Wilson, Microtubules as a target for anticancer drugs, Nat. Rev. Cancer, 2004, 4(4), 253–265 CrossRef CAS PubMed.
- C. A. Burkhart, M. Kavallaris and S. Band Horwitz, The role of beta-tubulin isotypes in resistance to antimitotic drugs, Biochim. Biophys. Acta, 2001, 1471(2), O1–O9 CAS.
- J. S. Waby, H. Chirakkal, C. W. Yu, G. J. Griffiths, R. S. P. Benson and C. D. Bingle,
et al., Sp1 acetylation is associated with loss of DNA binding at promoters associated with cell cycle arrest and cell death in a colon cell line, Mol. Cancer, 2010, 9, 275 CrossRef PubMed.
Footnote |
† Electronic supplementary information (ESI) available: Including literature-derived parameters used in development of the model. See DOI: 10.1039/c5mb00211g |
|
This journal is © The Royal Society of Chemistry 2016 |
Click here to see how this site uses Cookies. View our privacy policy here.