DOI:
10.1039/C5RA16563F
(Paper)
RSC Adv., 2015,
5, 84048-84055
Metabolomics approach to identify therapeutically potential biomarkers of the Zhi-Zi-Da-Huang decoction effect on the hepatoprotective mechanism†
Received
17th August 2015
, Accepted 23rd September 2015
First published on 23rd September 2015
Abstract
Zhi-Zi-Da-Huang decoction (ZZDHD), a Traditional Chinese Medicine (TCM) formula, has been widely used for the treatment of alcohol-induced liver injury for many years. To comprehensively explore the possible mechanism of the hepatoprotective effects of ZZDHD, a nuclear magnetic resonance (NMR)-based metabolomics study, 1H NMR spectra combined with pattern recognition methods including PCA and OPLS-DA, was applied to identify potential plasma and liver biomarkers responsible for the positive effects of ZZDHD on alcohol-induced liver injury in rats. PCA gave a global overview of control, alcohol and ZZDHD group rats. Potential plasma and liver biomarkers that were obtained from OPLS-DA loading plots combined with the corresponding VIP values reflected the hepatoprotective effects of ZZDHD associated with alcohol-induced liver injury. The results suggested that 9 potential plasma biomarkers including, lipoprotein, leucine, valine, dihydrothymine, 3-D-hydroxybutyrate, lactate, alanine, acetate and glucose, and 8 potential liver biomarkers, triglyceride, lactate, alanine, acetate, glutamine, glucose, xanthine and hypoxanthine were identified. Related metabolic pathway analysis found that ZZDHD significantly alleviated the disturbance in energy metabolism, glucose metabolism, amino acid metabolism, lipid metabolism, nucleic acid metabolism, ameliorating lipid peroxidation and permeability change of membrane, and oxidative stress induced by alcohol. The results indicated that ZZDHD could achieve remarkable effects on alcoholic hepatic injury through partially preventing or regulating the perturbed metabolic pathways. The study also demonstrated that NMR-based metabolomics was a useful tool for screening potential biomarkers, further helping to explain the therapeutic mechanism of TCM formula on a comprehensive scale.
Introduction
Metabolomics, a novel research method used to detect subtle metabolic changes in biological samples such as plasma or tissue, has been widely applied to the measurement of multiparametric metabolic responses of living systems to disease states or the biochemical effects of drugs in biological systems.1 It can capture global metabolic changes, investigate the pathogenesis of diseases or the intervention effects of drugs through identified potential biomarkers.2–6 Metabolomics is also an ideal tool to gain insight into the interaction mechanism between traditional Chinese medicine (TCM) and biological organisms.7,8 It can be used to assess the efficacy of TCM and its mechanism through a series of different sample spectra obtained from analytical techniques combined with pattern recognition methods.9 Much recent research has reported the application of metabolomics studies of TCM concentrating on exploring the molecular mechanisms of hepatoprotective effects in liver injury or liver diseases through identified potential biomarkers.10–13
Zhi-Zi-Da-Huang decoction (ZZDHD), a famous Chinese traditional medicine prescription firstly recorded in Jin-Gui-Yao-Lue (Synopsis of Golden Chamber) by Zhongjing Zhang, consists of four crude herbs: Rheum officinale Baill. (Da-Huang), Gardenia jasminoides Ellis (Zhi-Zi), Citrus aurantium L. (Zhi-Shi) and Semen Sojae Preparatum (Dan-Dou-Chi).14 In our nascent research, the optimal hepatoprotective effects of ZZDHD for alcohol-induced liver damage have been determined at a dose of 12 g per kg per day body weight.15 However, the remedial mechanism of the overall effect of ZZDHD remains entirely unclear.
In this study, 1H NMR spectra were used to detect a global profile of endogenous metabolites; following which pattern recognition was performed to discover potential plasma and liver biomarkers of a rat exposed to alcohol and the hepatoprotective effects of ZZDHD. The aim of the present study was to (1) find potential biomarkers in plasma and liver and (2) improve the understanding of the therapeutic efficacy and the underlying hepatoprotective mechanism of ZZDHD.
Materials and methods
Chemicals
Analytical grade alcohol, methanol, sodium chloride, Na2HPO4·12H2O and NaH2PO4·2H2O were bought from Nanjing Chemical Reagent Co., Ltd (Nanjing, China). Deuterium oxide (D2O, 99.9% D) and sodium 3-trimethylsilyl [2,2,3,3-2H4] propionate (TSP) were obtained from Sigma-Aldrich (St. Louis, MO, USA).
Preparation of ZZDHD sample
All the herbs were purchased from Xiansheng Medicine Company (Nanjing, China) and identified by Professor Min-Jian Qin, Department of Medicinal Plants, China Pharmaceutical University. The mixtures of crude herbs (48 g), Rheum officinale Baill., Gardenia jasminoides Ellis, Citrus aurantium L. and Semen Sojae Preparatum at the weight ratio of 1
:
3
:
4
:
8, were extracted thrice under distilled water (1
:
10; w/v), each for 30 min. Finally, the extracted ZZDHD solutions were pooled together, freeze-dried, and stored at −20 °C before use. For the quality control of ZZDHD, the six main ingredients, geniposide, naringin, neohesperidin, daidzein, rhein and emodin were determined by a HPLC-UV system on a LiChrospher-C18 column (250 mm × 4.6 mm, 5 μm, Hanbon Science & Technology Co., Jiangsu, China). The HPLC chromatogram of ZZDHD is shown in ESI Fig. S1.†
Animal handling and sample collection
Male Sprague-Dawley rats (n = 18) weighing 180–220 g were purchased from Animal Experimental Centre of Qinglong Mountain (Nanjing, China). The animals were maintained in an animal room with the following parameters: temperature of 20–25 °C, humidity of 55–65% and artificial light/dark cycles from 08:00–20:00. All animal experiments were approved strictly by the guidance for Experimental Animal Welfare of the National Guidelines (MOST of The People’s Republic of China, 2006) at the Centre for SPF-grade Animal Experiments of Jiangsu Institute for Food and Drug Control.
After 7 days of acclimatization, the rats were randomly divided into three groups of six rats each: control group (CG), alcohol group (AG) and ZZDHD group (ZG). The ZG rats were orally administered the ZZDHD solution (1.0 g ml−1, 12 ml per kg per day) at 15:00 from day 1 to day 9. Concurrently, the ZG rats were given water orally at 17:00 from day 1 to day 2, and then dosed with 50% alcohol at a dose of 14 ml per kg per day at 17:00 from day 3 to day 9. The AG rats were orally administered 50% alcohol at a dose of 14 ml per kg per day between 17:00 and 18:00 for seven consecutive days from day 3 to day 9 to induce an animal model liver injury. The CG rats were always administrated with equivalent water. At 20:00 on day 9, all animals were fasted and then euthanised following isoflurane anaesthesia after 12 hours. The surgical procedures were performed under isoflurane anaesthesia, and all efforts were made to minimize animal suffering.
The liver and blood samples from all three groups were obtained immediately on day 10 (08:00 a.m.). Blood samples were collected into Eppendorf tubes containing sodium heparin to obtain plasma samples, which was immediately snap-frozen in liquid nitrogen and stored at −80 °C for later analysis. Liver tissues were collected and divided into two parts. One part was immediately snap-frozen in liquid nitrogen and stored at −80 °C until NMR spectroscopy, and the other part was used for histological examination.
NMR measurements
Plasma samples were thawed at room temperature and centrifuged at 16
000 rpm for 10 min. 300 μl supernatant was mixed with 150 μl phosphate buffer (0.2 mol l−1, pH 7.4) and 150 μl 10% w/v TSP (100% D2O), then transferred into 5 mm NMR tubes for analysis. The liver tissues (60 mg) were homogenized three times with 600 μl of 50% methanol and separately centrifuged at 16
000 rpm for 15 min. Three combined supernatants were subjected to centrifugation (12
000 rpm, 10 min). Then the resultant supernatants were dried under a stream of nitrogen. Each liver tissue extract was reconstituted into 600 μl phosphate buffer (0.15 mol l−1, pH 7.4) containing 0.006% TSP, 20% D2O. The solution was centrifuged at 16
000 rpm for 10 min and 550 μl supernatant was transferred into a 5 mm NMR tube for analysis. For plasma samples, the Carr–Purcell–Meiboom–Gill (CPMG) sequence (RD-90°-(τ-180°-τ)n-ACQ; RD = 2 s, τ = 350 μs, n = 100) with a total spin-echo delay (2nτ) of 60 ms was used. For liver extracts, the standard noesygppr1d pulse sequence (RD-G1-τ-90°-t1-90°-tm-G2-90°-ACQ; RD = 2 s, t1 = 4 μs, tm = 100 ms) was used to obtain metabolic profiles. All the NMR spectra were Fourier transformed after an exponential line-broadening function of 0.5 Hz was applied.
NMR data processing
Each spectrum was manually phased, baseline corrected and referenced to TSP (CH3, δ 0.0) utilizing TOPSPIN software (version 2.1, Bruker Biospin, Germany). All 1H NMR spectra were processed using the AMIX software package (Bruker Biospin). The regions (δ 4.30–5.12, δ 5.51–6.80) in the plasma spectra and (δ 4.50–5.20) in liver extract spectra were discarded to prevent variation in water signal. The ethyl glucuronide peaks16 were also removed in the plasma spectra. All remaining spectra were integrated into regions with a typical bucket-width of 0.04 ppm using the AMIX software package (Bruker Biospin). Each bucketed region of liver extract sample was normalized by integral normalization to compensate for the effect of variation in concentration, and the plasma spectra was normalized by probabilistic quotient normalization.
Pattern recognition processing and potential biomarker identification
Pattern recognition was performed using the SIMCA-P+ 13 software package (Umetrics, Umea, Sweden). A non-supervised principal component analysis (PCA) was firstly performed with mean-centered NMR data to examine group clustering. Then a supervised orthogonal projection to latent structure discriminant analysis (OPLS-DA) was further carried out with the pareto-scaled NMR data as the X-matrix and the group information as the Y-matrix to discover potential biomarkers. All OPLS-DA models were calculated using a seven-fold cross-validation method. The quality of the models was assessed by the parameters R2X, and the predictability of models as Q2. The models were considered as effective and reliable when these values are more than 0.5. The reliability and predictability are better when these parameters are closer to 1.0. Meanwhile, the models were also tested by the well-known cross-validated analysis of variance (CV-ANOVA) approach and p < 0.05 was considered to be statistically significant. In the OPLS-DA, the samples from different groups were separated into different classes, and differential metabolites responsible for good separation of different groups were obtained from the corresponding loading of the S-line. The differential metabolites were further selected by variable importance in the projection (VIP) values using a VIP ≥ 1. Thus, these endogenous metabolites based on the S-line and VIP values were finally considered as potential biomarkers. Relevant heat maps of the identified potential plasma or liver biomarkers were performed to describe an unknown sample in the test set and classify it according to which class the majority of neighbors in the training set, which are closest to this test sample, belong to. Heat mapping has long been used in pattern recognition, data mining and analysis.
Results and discussion
Histopathology study
Histopathology examination showed that the control rats had normal liver tissue and no pathological changes (Fig. S2a†). Typical pathological features of liver damage, such as fat particles of varying sizes, substantial vacuolization, fatty deposition and lipid accumulation were observed in the alcohol-treated rats (Fig. S2b†). For the ZZDHD-treated rats, pathological abnormalities were noticeably improved, which showed the hepatoprotective function of ZZDHD (Fig. S2c†). The above results indicated that the animal model of alcohol-induced liver injury was successfully, and the hepatic steatosis was ameliorated with administration of ZZDHD.
Pattern recognition analysis of plasma metabolomics data
Typical 1H NMR spectra of plasma from the CG, AG and ZG are shown in Fig. 1 with major metabolites marked. Endogenous metabolites were identified by referencing the previously reported corresponding chemical shifts of the metabolites,17–20 some from publicly available metabolomics databases, such as Madison http://mmcd.nmrfam.wisc.edu/, KEGG http://www.genome.jp/kegg/, HMDB http://www.hmdb.ca/, and Chenomx NMR Suite software (version 7.5, Chenomx, Inc.). Specifically, endogenous metabolites of the plasma samples mainly contained lipoprotein, dihydrothymine, 3-D-hydroxybutyrate, lactate, acetate, creatine, glucose, taurine, formate and a series of amino acids.
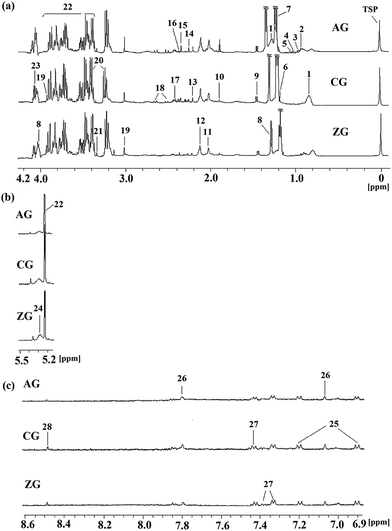 |
| Fig. 1 Typical 500 MHz 1H NMR spectra of plasma from different group rats. Metabolite keys: (1), lipoprotein; (2), leucine; (3), isoleucine; (4), valine; (5), dihydrothymine; (6), 3-D-hydroxybutyrate; (7), ethyl glucuronide; (8), lactate; (9), alanine; (10), acetate; (11), N-acetyl-glycoprotein; (12), O-acetyl-glycoprotein; (13), acetoacetate; (14), pyruvate; (15), succinate; (16), α-oxoglutarate; (17), glutamine; (18), citrate; (19), creatine; (20), taurine; (21), methanol; (22), glucose; (23), phosphocholine; (24), unsaturated lipid; (25), tyrosine; (26), histidine; (27), phenylalanine; (28), formate. | |
To obtain more details about the metabolic profiles and discover potential plasma biomarkers, pattern recognition analysis was performed on the NMR metabolomics data. Firstly, the PCA of the plasma profiles was performed to get a global overview of the metabolic response of the rats exposed to alcohol and ZZDHD administration (Fig. 2a). In the score plot of the PCA, each point represented an individual sample, the AG rats were completely separated from the CG rats, and the ZG rats were also completely separated from the AG. Due to the intervention effects of ZZDHD, the ZG rats were near to the CG rats, which indicated ZZDHD played a significant role in inhibiting alcohol induced liver injury.
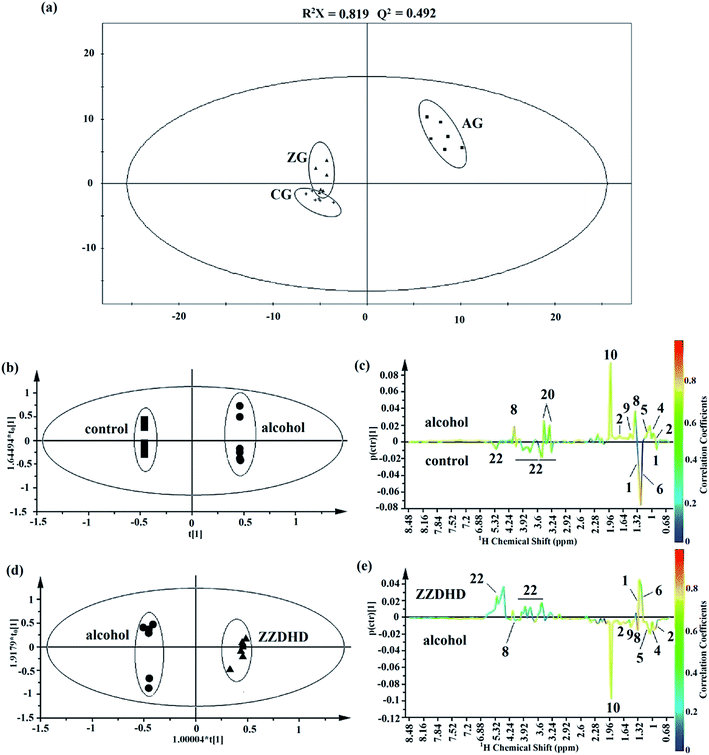 |
| Fig. 2 Multivariate analysis of plasma from CG, AG and ZG rats. A global overview from PCA (a) and potential plasma biomarkers identifications from OPLS-DA model analyses (b–e). | |
Then, OPLS-DA was further conducted to explore significantly differential metabolites responsible for the group differentiation. A clear separation between the AG and CG (Fig. 2b) demonstrated remarkable metabolomics differences with good model quality (R2X = 0.925, Q2 = 0.727, p = 1.64 × 10−3). The corresponding loading plot of OPLS-DA indicated that metabolic changes induced by alcohol were obviously visible including level elevations for leucine, valine, dihydrothymine, lactate, alanine, acetate and taurine together with a level decrease for lipoprotein, 3-D-hydroxybutyrate and glucose compared with controls (Fig. 2c). To further validate the identified differential metabolites, variable importance to projection values (VIP) using a VIP ≥ 1 was necessary. Table 1 showed the VIPs of metabolites responsible for the loading plot. Comprehensive results identified 10 endogenous metabolites as potential biomarkers from the control and alcohol groups. Similarly, for alcohol and ZZDHD groups, a reasonably good separation was shown in the score plot of OPLS-DA with R2X = 0.815, Q2 = 0.943, p = 2.74 × 10−5 (Fig. 2d). Based on the corresponding loading plot (Fig. 2e) and VIP values (Table 1), 9 endogenous metabolites were selected; leucine, valine, dihydrothymine, lactate, alanine, lipoprotein, 3-D-hydroxybutyrate acetate and glucose, which could be considered as plasma potential biomarkers for the positive therapeutic effect of ZZDHD. Then the selected 9 potential biomarkers from the plasma were used to build the prediction model using MeV software. The heat map, an unsupervised clustering, was constructed based on the identified potential significative biomarkers. The parallel heat map visualization (Fig. 3) showed distinct segregation for the alcohol and control rats, but a similar tendency for the control and ZZDHD rats.
Table 1 List of the plasma differential metabolites identified from AG vs. CG and ZG vs. AG
No. |
Potential biomarker |
Chemical shifta (ppm) and multiplicity |
VIPb |
AG vs. CG |
ZG vs. AG |
s, singlet; d, doublet; t, triplet; q, quartet; m, multiplet; dd, double doublet. Variable importance in the projection (VIP) was obtained from OPLS-DA. |
1 |
Lipoprotein |
0.83–0.93(m), 1.27–1.34(m) |
1.82 |
1.94 |
2 |
Leucine |
0.97(d) |
1.43 |
1.85 |
3 |
Valine |
1.04(d) |
1.98 |
2.08 |
4 |
Dihydrothymine |
1.07(d) |
1.71 |
1.76 |
5 |
3-D-Hydroxybutyrate |
1.22(d) |
4.29 |
2.44 |
6 |
Lactate |
1.36(d), 4.14(q) |
2.42 |
1.75 |
7 |
Alanine |
1.50(d) |
1.45 |
1.68 |
8 |
Acetate |
1.94(s) |
4.39 |
4.55 |
9 |
Taurine |
3.28(t), 3.42(t) |
2.06 |
0.60 |
10 |
Glucose |
3.39(t), 3.53(dd), 3.73(dd), 3.76(dd), 3.81(m), 5.26(d), 3.22(dd), 3.42(t), 3.47(m), 3.49(t), 3.72(dd), 3.92(dd) |
1.40 |
1.34 |
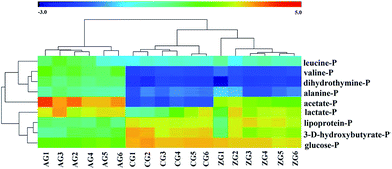 |
| Fig. 3 Heatmap of identified potential plasma biomarkers. Row, samples; columns, potential biomarkers. Color indicates metabolites expression value: lowest (blue) to highest (red). | |
Pattern recognition analysis of liver metabolomics data
Representative 1H NMR spectra of rat liver tissue extracts from the CG, AG and ZG were presented in Fig. 4 with the major metabolites marked. Endogenous metabolites of the liver tissue extract samples mainly contained triglyceride, glucose, amino acids, purines, and pyrimidines. In the alcohol group, the increase in triglyceride was clearly observed.
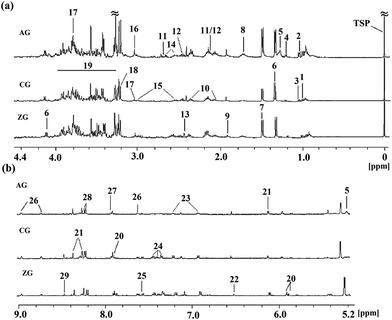 |
| Fig. 4 Typical 500 MHz 1H NMR spectra of liver extract from different groups. Metabolite keys: (1), isoleucine; (2), leucine; (3), valine; (4), 3-D-hydroxybutyrate; (5), triglyceride; (6), lactate; (7), alanine; (8), ornithine; (9), acetate; (10), glutamate; (11), methionine; (12), glutamine; (13), succinate; (14), citrate; (15), oxidized glutathione; (16), creatine; (17), lysine; (18), choline; (19), glucose; (20), uridine; (21), adenosine; (22), fumarate; (23), tyrosine; (24), phenylalanine; (25), uracil; (26), nicotinamide; (27), xanthine; (28), hypoxanthine; (29), formate. | |
To investigate the significance of changes of metabolites, pattern recognition analysis was necessary. As for the liver extract, the score plot of PCA was firstly conducted to get an overview of trends for the control, alcohol and ZZDHD group rats (Fig. 5a). The results showed that the CG and ZG rats were clearly separate from the AG rats, and the CG was also close to the ZG, indicating the hepatoprotective effects of ZZDHD rats exposed to alcohol.
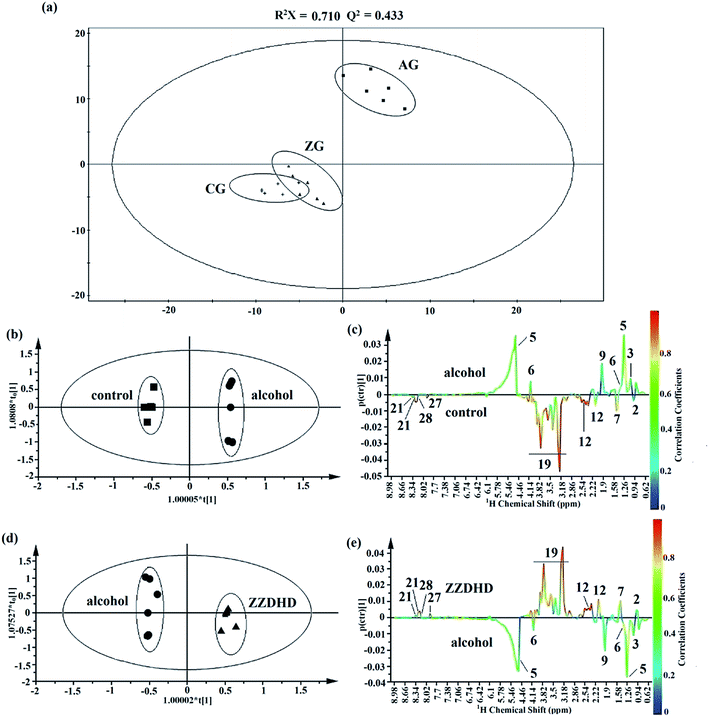 |
| Fig. 5 Multivariate analysis of liver extract from CG, AG and ZG rats. A global overview from PCA analyse (a) and potential liver biomarkers identifications from OPLS-DA model analyses (b–e). | |
The OPLS-DA model was then performed to detect differential metabolites from the control and alcohol group rats. The CG and AG were clearly clustered into two groups from the corresponding score plot of OPLS-DA (Fig. 5b), which were verified with R2X = 0.941, Q2 = 0.928, p = 6.06 × 10−7 and considered as valid. Differential metabolites of liver tissue extract from the two groups were selected through coefficient-coded loading plot (Fig. 5c). Next, the screened differential metabolites were further identified with a VIP value larger than 1.0 (Table 2). Nine metabolites containing triglyceride, lactate, alanine, acetate, glutamine, glucose, xanthine, adenosine and hypoxanthine were finally screened as potential biomarkers, which indicated alcohol disturbed their normal levels. Similarly, the plot of OPLS-DA score (Fig. 5d) obtained from the 1H NMR spectra of liver extract showed a clear separation between the control and alcohol rats (R2X = 0.899, Q2 = 0.916, p = 4.99 × 10−7). The result of the combination of the loading plot (Fig. 5e) and VIP values indicated that 8 endogenous metabolites were considered as potential liver biomarkers responsible for the positive therapeutic effect of ZZDHD. Meanwhile, the heat map of 8 liver biomarkers (Fig. 6) also showed similar results to that of the plasma biomarkers, which indicated alcohol could affect the normal metabolism of rats, and that ZZDHD had clearly regulated the abnormal metabolism.
Table 2 List of the liver extract differential metabolites identified from AG vs. CG and ZG vs. AG
No. |
Potential biomarker |
Chemical shifta (ppm) and multiplicity |
VIPb |
AG vs. CG |
ZG vs. AG |
s, singlet; d, doublet; t, triplet; q, quartet; m, multiplet; dd, double doublet. Variable importance in the projection (VIP) was obtained from OPLS-DA. |
1 |
Leucine |
0.98(t) |
0.78 |
0.93 |
2 |
Valine |
1.04(d) |
0.86 |
0.93 |
3 |
Triglyceride |
1.27(m), 5.20(m) |
2.53 |
2.51 |
4 |
Lactate |
1.34(d), 4.12(q) |
1.01 |
1.15 |
5 |
Alanine |
1.49(d) |
1.71 |
1.14 |
6 |
Acetate |
1.94(s) |
1.72 |
1.79 |
7 |
Glutamine |
2.14(m), 2.45(m) |
1.39 |
1.65 |
8 |
Glucose |
3.39(t), 3.53(dd), 3.73(dd), 3.76(dd), 3.81(m), 5.26(d), 3.22(dd), 3.42(t), 3.47(m), 3.49(t), 3.72(dd), 3.92(dd) |
2.49 |
2.34 |
9 |
Xanthine |
7.91(s) |
1.29 |
1.38 |
10 |
Adenosine |
8.25(s), 8.35(s) |
1.21 |
0.89 |
11 |
Hypoxanthine |
8.20(s), 8.22(s) |
1.20 |
1.21 |
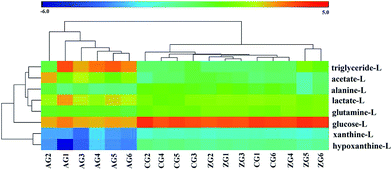 |
| Fig. 6 Heatmap of identified potential liver biomarkers. Row, samples; columns, potential biomarkers. Color indicates metabolites expression value: lowest (blue) to highest (red). | |
The effects of ZZDHD treatment for ALD in metabolic respects
The protective effects of ZZDHD against liver damage induced by alcohol were investigated through 1H NMR spectra coupled with pattern recognition methods. The results showed alcohol affected 10 potential plasma biomarkers, 9 of which were reversed with the effects of ZZDHD intervention. Alcohol altered the normal metabolic profile of 9 potential liver biomarkers, 8 of which were regulated in response to the positive effects of ZZDHD. The related metabolic pathways were analyzed based on the identified potential plasma and liver biomarkers combined with Human Metabolome Database (http://www.hmdb.ca/), Kyoto Encyclopedia of Genes and Genomes (http://www.genome.jp/kegg/) and MetaboAnalyst 3.0.
Energy metabolism and glucose metabolism
Lactate could reflect the supply-to-demand state of tissues.21 It is also the end-product of glucose metabolism with anaerobic conditions.22 In our study, the AG showed increased amounts of lactate in the plasma and liver, while the level of glucose appeared decreased. This phenomenon indicated alcohol could aggravate anaerobic metabolism, causing gluconeogenesis damage, further disrupting the levels of liver or plasma related metabolites. Additionally, alanine could be formed from pyruvate through transamination when amino acids in the muscle transferred an amino group.23 The alanine was transferred to the liver by the blood, and could deaminate back to pyruvate, further generating glucose by the gluconeogenesis pathway.24 Our metabolomics results showed that alanine had obviously decreased in the liver together with an increase in the plasma for the AG. Evidence for gluconeogenesis damage was further supported by the distinct decrease in alanine in the liver.
Alcohol disturbed the energy metabolism and glucose metabolism as revealed by a remarkably increased level of lactate in the liver and plasma, glucose levels decreasing in the liver and plasma, and the decreased level of alanine in the liver together with increase in the plasma. However, the intervention effects of ZZDHD ameliorated the abnormality states by reversing these disturbed potential biomarkers.
Amino acid metabolism
In alcohol-induced liver injury, reactive oxygen species (ROS) and oxidative stress have been proven to play central roles.25 Alcohol could increase the generation of ROS, excessive ROS disrupts the balance between the oxidation and anti-oxidation systems, and further causes oxidative damage such as the lipid peroxidation of membranes and the cell membrane permeability change.26–28 The elevated levels of amino acids (leucine, valine and alanine) suggested that alcohol altered the membrane permeability and made amino acids release into the plasma from damaged hepatic cells. The reductions of glutamine and alanine in the liver confirmed the changes of permeability of intracellular membranes by the influence of alcohol.
ZZDHD could alleviate the membrane disruption as evidenced by regulating amino acid metabolism, such as the reduced levels of leucine, valine and alanine in plasma, and the elevated levels of glutamine and alanine in the liver.
Lipid metabolism and nucleic acid metabolism
Alcohol could inhibit the synthesis of the protein components of lipoprotein in blood, further affecting the secretion of triglyceride (TG) in the liver to the blood.29 During alcohol-induced liver injury, abnormal situations of lipid metabolism could be reversed by ZZDHD, illustrated by the increasing level of lipoprotein in the plasma and the reduction of TG in the liver compared to the CG rats. ROS from alcohol metabolism could lead to the oxidation of membrane lipids,30,31 disrupting both fatty acid oxidation and nucleic acid metabolism, as testified by the decreased levels of 3-D-hydroxybutyrate in the plasma and xanthine, adenosine and hypoxanthine in the liver, together with the increased level of dihydrothymine in the plasma.
ZZDHD could partly attenuate the oxidative stress as evidenced by the elevated level of 3-D-hydroxybutyrate and lipoprotein in the plasma and the reduced level of TG in the liver. The regulation of the disorder of nucleic acid metabolism by ZZDHD was also indicated by restored levels of dihydrothymine, xanthine and hypoxanthine.
Conclusion
In this study, a1H NMR-based metabolomics approach was used to investigate detailed information about the metabolic changes within an organism due to the intervention effects of ZZDHD. 1H NMR spectroscopy coupled with pattern recognition methods identified the specific metabolite changes for the CG, AG and the ZG. 9 potential plasma biomarkers and 8 potential liver biomarkers responsible for the hepatoprotective actions of ZZDHD were detected. Related metabolic pathway analysis of the altered potential biomarkers indicated that ZZDHD significantly regulated lipid peroxidation of membrane, altered cell membrane permeability, and oxidative damage induced by alcohol, alleviating the disturbance in energy metabolism, glucose metabolism, amino acid metabolism, lipid metabolism, and nucleic acid metabolism, thus achieving protective effects on the liver. The methods used in this study did not only explore potential biomarkers but also could provide a comprehensive understanding of the underlying molecular mechanisms of ZZDHD for alcoholic liver disease.
Acknowledgements
The study was supported by National Natural Science Foundation of China (Grant number: 81274063) and a Project Funded by the Priority Academic Program Development of Jiangsu Higher Education Institutions.
References
- J. K. Nicholson, J. C. Lindon and E. Holmes, Xenobiotica, 1999, 29, 1181–1189 CrossRef CAS PubMed
. - F. Y. Ghauri, J. K. Nicholson, B. C. Sweatman, J. Wood, C. R. Beddell, J. C. Lindon and N. J. Cairns, NMR Biomed., 1993, 6, 163–167 CrossRef CAS PubMed
. - J. T. Brindle, H. Antti, E. Holmes, G. Tranter, J. K. Nicholson, H. W. Bethell, S. Clarke, P. M. Schofield, E. McKilligin, D. E. Mosedale and D. J. Grainger, Nat. Med., 2002, 8, 1439–1444 CrossRef CAS PubMed
. - L. L. Wang, L. Y. Zheng, R. Luo, X. S. Zhao, Z. H. Han, Y. L. Wang and Y. X. Yang, RSC Adv., 2015, 5, 281–290 RSC
. - G. M. Liu, G. J. Yang, T. T. Fang, Y. M. Cai, C. M. Wu, J. Wang, Z. Q. Huang and X. L. Chen, RSC Adv., 2014, 4, 23749–23758 RSC
. - M. Huang, Q. L. Liang, P. Li, J. F. Xia, Y. Wang, P. Hu, Z. T. Jiang, Y. X. He, L. Q. Pang, L. D. Han, Y. M. Wang and G. A. Luo, Mol. BioSyst., 2013, 9, 2134–2141 RSC
. - Y. Gu, C. Lu, Q. L. Zha, H. W. Kong, X. Lu, A. P. Lu and G. W. Xu, Mol. BioSyst., 2012, 8, 1535–1543 RSC
. - Y. Huang, Y. Tian, G. Li, Y. Y. Li, X. J. Yin, C. Peng, F. G. Xu and Z. J. Zhang, Anal. Bioanal. Chem., 2013, 405, 4811–4822 CrossRef CAS PubMed
. - H. Dong, A. H. Zhang, H. Sun, H. Y. Wang, X. Lu, M. Wang, B. Ni and X. J. Wang, Mol. BioSyst., 2012, 8, 1206–1221 RSC
. - X. J. Wang, B. Yang, A. H. Zhang, H. Sun and G. L. Yan, J. Proteomics, 2012, 75, 1411–1427 CrossRef CAS PubMed
. - W. F. Yao, H. W. Gu, J. J. Zhu, G. Barding, H. B. Cheng, B. H. Bao, L. Zhang, A. W. Ding and W. Li, Anal. Bioanal. Chem., 2014, 406, 7367–7378 CrossRef CAS PubMed
. - H. Sun, A. H. Zhang, D. X. Zou, W. J. Sun, X. H. Wu and X. J. Wang, Appl. Biochem. Biotechnol., 2014, 173, 857–869 CrossRef CAS PubMed
. - X. J. Wang, H. T. Lv, A. H. Zhang, W. J. Sun, L. Liu, P. Wang, Z. M. Wu, D. X. Zou and H. Sun, Liver Int., 2014, 34, 759–770 CrossRef CAS PubMed
. - J. C. Chen, J. Chin. Med., 2001, 12, 69–80 Search PubMed
. - H. Wang, F. Feng, B. Y. Zhuang and Y. Sun, J. Ethnopharmacol., 2009, 126, 273–279 CrossRef PubMed
. - P. C. Nicholas, D. Kim, F. T. Crews and J. M. Macdonald, Anal. Biochem., 2006, 358, 185–191 CrossRef CAS PubMed
. - W. X. Xu, J. F. Wu, Y. P. An, C. N. Xiao, F. H. Hao, H. B. Liu, Y. L. Wang and H. R. Tang, J. Proteome Res., 2012, 11, 3423–3435 CrossRef CAS PubMed
. - X. L. Kong, X. Q. Yang, J. L. Zhou, S. X. Chen, X. Y. Li, F. Jian, P. C. Deng and W. Li, Oncol. Lett., 2015, 9, 283–289 Search PubMed
. - I. Montoliu, F. P. Martin, S. Collino, S. Rezzi and S. Kochhar, J. Proteome Res., 2009, 8, 2397–2406 CrossRef CAS PubMed
. - F. Dong, L. Zhang, F. Hao, H. Tang and Y. Wang, J. Proteome Res., 2013, 12, 2958–2966 CrossRef CAS PubMed
. - R. J. Connett, Adv. Exp. Med. Biol., 1988, 222, 133–142 CrossRef CAS
. - E. A. Shoubridge and P. W. Hochachka, Science, 1980, 209, 308–309 CAS
. - J. B. Li and L. S. Jefferson, Am. J. Physiol., 1977, 232, E243–E249 CAS
. - R. W. Hanson and L. Reshef, Biochimie, 2003, 85, 1199–1205 CrossRef CAS PubMed
. - D. Wu and A. I. Cederbaum, Alcohol Res. Health, 2003, 27, 277–284 Search PubMed
. - I. Kurose, H. Higuchi, S. Kato, S. Miura, N. Watanabe, Y. Kamegaya, K. Tomita, M. Takaishi, Y. Horie, M. Fukuda, K. Mizukami and H. Ishii, Gastroenterology, 1997, 112, 1331–1343 CrossRef CAS
. - M. Hirokawa, S. Miura, H. Yoshida, I. Kurose, T. Shigematsu, R. Hokari, H. Higuchi, N. Watanabe, Y. Yokoyama, H. Kimura, S. Kato and H. Ishii, Alcohol.: Clin. Exp. Res., 1998, 22, 111S–114S CrossRef CAS
. - A. I. Cederbaum, Y. Lu and D. Wu, Arch. Toxicol., 2009, 83, 519–548 CrossRef CAS PubMed
. - S. H. Choi and H. N. Ginsberg, Trends Endocrinol. Metab., 2011, 22, 353–363 CrossRef CAS PubMed
. - O. Sergent, M. Pereira, C. Belhomme, M. Chevanne, L. Huc and D. Lagadic-Gossmann, J. Pharmacol. Exp. Ther., 2005, 313, 104–111 CrossRef CAS PubMed
. - R. Nordmann, C. Ribiere and H. Rouach, Alcohol Alcohol., 1990, 25, 231–237 CAS
.
Footnote |
† Electronic supplementary information (ESI) available. See DOI: 10.1039/c5ra16563f |
|
This journal is © The Royal Society of Chemistry 2015 |
Click here to see how this site uses Cookies. View our privacy policy here.