DOI:
10.1039/C4AY02470B
(Paper)
Anal. Methods, 2015,
7, 181-186
The use of handheld near-infrared reflectance spectroscopy (NIRS) for the proximate analysis of poultry feed and to detect melamine adulteration of soya bean meal
Received
16th October 2014
, Accepted 7th November 2014
First published on 7th November 2014
Abstract
The use of handheld near infrared (NIR) instrumentation, as a tool for rapid analysis, has the potential to be used widely in the animal feed sector. A comparison was made between handheld NIR and benchtop instruments in terms of proximate analysis of poultry feed using off-the-shelf calibration models and including statistical analysis. Additionally, melamine adulterated soya bean products were used to develop qualitative and quantitative calibration models from the NIRS spectral data with excellent calibration models and prediction statistics obtained. With regards to the quantitative approach, the coefficients of determination (R2) were found to be 0.94–0.99 with the corresponding values for the root mean square error of calibration and prediction were found to be 0.081–0.215% and 0.095–0.288% respectively. In addition, cross validation was used to further validate the models with the root mean square error of cross validation found to be 0.101–0.212%. Furthermore, by adopting a qualitative approach with the spectral data and applying principal component analysis, it was possible to discriminate between adulterated and pure samples.
Introduction
The development of handheld instrumentation for analytical techniques has gained prominence in recent times. This was highlighted in the excellent review1 which discussed characteristics and advancements of opto-sensing portable/handheld instrumentation using for example near infrared (NIR), fourier transform infrared (FTIR), Raman and fluorescence based spectroscopies. NIR spectroscopy has been used routinely for many years within the food and animal feed sectors. Initially, most applications associated with portable/handheld NIR instruments were related to quality parameters of foodstuffs e.g. internal and external quality parameters of mandarins were used to access their ripeness;2 analysis for fat, moisture and protein content of meat;3 analysis for fat content, free acidity and moisture content of intact olives and comparison to benchtop NIRS analysis.4 More recently an NIR device (SCiO),5 which is the size of a matchbox and can be linked by bluetooth to smartphones, has been developed which could revolutionise the approach of future analytical procedures for a low cost outlay ($199–$299) and make NIRS applications accessible and affordable to a wider community.
Melamine (1,3,5-triazine-2,4,6-triamine), is a trimer of cyanamide which is easily polymerised through the reaction with formaldehyde to form a heat-tolerant, fire-resistant resin used in the manufacture of flame retardant materials. In a previous publication by the authors,6 the fraudulent adulteration of foodstuffs and feedstuffs with melamine, to raise the apparent protein content, was highlighted. The discovery of melamine in many food and feedstuffs led the European Commission7–9 to ban the imports of many products from China and set the maximum permitted concentration for melamine in food at 2.5 mg kg−1. However, the limits set by the European Union are at the low contamination levels and related to human health issues. For fraudsters to make profit by economically motivated adulteration (EMA), by turning a low value commodity into a higher value commodity based on protein content, then much higher levels of melamine are needed. For example if 2% of melamine is added to a commodity,10 the nitrogen content of the resulting mixture would be increased by ∼1.3% and the apparent protein content would be increased by over 8% assuming a nitrogen-to protein ratio of 6.25. Due to the notoriety and scandal that developed from 2007 onwards related to melamine adulteration, several reviews have been carried out, including how it evolved and the global effects of the scandal11 and its repercussions for the food safety community and consequent changes to regulations.12
As melamine is not permitted to be added to food and feed commodities, many methods of analysis have been developed in recent years including: (i) Enzyme Linked Immunosorbent Assays (ELISAs);13–16 (ii) Fluorescence Polarisation Immunoassay (FPIA);17 Surface Plasmon Resonance (SPR) biosensor assay;18 Gas-Chromatography Mass-Spectrometry (GC-MS);19 High Performance Liquid Chromatography (HPLC) for infant formula;20 Time of Flight Mass Spectrometry (TOF-MS).21
NIRS is a widely used technique due to its speed, reproducibility, and non-destructive capabilities and also its on-line or at-line applications. More recently, the development of NIRS microscopy and NIRS hyperspectral imaging has led to new applications e.g. (i) for the identification of meat and bone meal in animal feed;22 (ii) to detect undesirable substances in food and feed.23 Due to the health risks associated with melamine in foodstuffs, NIRS procedures have been developed to detect melamine in milk products and infant formulas.24,25 In addition, benchtop NIR6 and NIR microscopy imaging26 have been used to screen soybean meal for the detection of melamine.
In the present study, the main objectives were (i) to compare handheld and benchtop NIRS for the proximate analysis of poultry feed and (ii) to use an NIR handheld/portable instrument to produce spectral data to be used with chemometrics to generate calibration models capable of either qualitatively or quantitatively detecting melamine adulteration in soya and soya product samples destined for animal feed production.
Materials and methods
Sample collection and preparation
For comparison between benchtop and handheld NIR proximate analysis, different batches of the same animal feed diet were collected (n = 21) from John Thompson and Sons Limited (Belfast, Northern Ireland). Batches of commercial soya bean meal samples (hulls, dehulled and toasted, n = 200) were also obtained from John Thompson and Sons Ltd. Samples were ground using an analytical mill (IKA Werke, Germany) and subsequently spiked with a range of concentrations (0, 0.25, 0.5, 0.75, 1, 1.25, 1.5, 1.75 and 2% w/w) of melamine (Sigma Aldrich, UK) as previously described,6 which were used to produce the spectral data and the subsequent calibration models.
Near-infrared reflectance spectroscopy (NIRS)
For benchtop NIRS analysis, the spectra were recorded on an Antaris II FT-NIR (Thermo Fisher Scientific, Dublin, Ireland) as published previously.6 For the proximate analysis comparison, off the shelf INGOT calibrations were used (Aunir, Towcester, UK) and were corrected for bias using reference materials supplied by Aunir.
For handheld NIRS analysis, spectra were recorded on a Thermo Fisher microPHAZIR AG (Antech Ltd, Ireland). The melamine spiked soya samples (10 g) were poured onto the sample cup of the instrument. All the spectra were computed at 8 cm−1 resolution across the spectral range 6250–4167 cm−1, re-filled three times and ran in triplicate (i.e. 9 spectra acquired per sample). The spectra were recorded at ambient temperature and nine spectra collected for each sample averaged. For the proximate analysis comparison, off the shelf INGOT calibrations were used (Aunir, Towcester, UK) and corrected for bias using reference samples from Aunir where the sample was poured into the sample cup and five spectra were collected with a rotation of the cup between each scan. The AUNIR NIR calibrations are based on ∼20 years of wet chemistry data related to the proximate analysis of animal feed.
Chemometric analysis
Multivariate analysis was used for quantitative and qualitative analysis of the NIRS spectroscopic data. The analysis was carried out using the software package TQ Analyst (Thermo Fisher Scientific, Dublin, Ireland) and SIMCA 13 (Umetrics, Sweden).
Principal Components Analysis (PCA) was used for the qualitative modelling in this investigation with standard normal variate technique (SNV), which compensates for differences in pathlengths due to scattering effects, and Pareto scaling using the SIMCA 13 chemometric software. The data generated include R2 which is an estimate of the fit of the model and Q2 which is an estimate of the predictive ability of the model and it is calculated by cross-validation. The latter is calculated by dividing the data into 7 parts, each 1/7th in succession is removed and a model was built on the remaining 6/7th of the data with the omitted data predicted using the new model. This is reiterated until all the data have been predicted using this method of cross validation. By comparison with the original data, the sum of squared errors is calculated for the whole dataset as the Predicted Residual Sum of Squares (PRESS) with the best predictability of the model indicated by a low value. The SIMCA 13 chemometric software automatically converts PRESS into Q2 to resemble the scale of the R2 with good predictions having high Q2.
Partial Least Squares-Regression (PLS-R) was used in the current study for quantitative calibration model building. For the quantitative models, developed using the TQ Analyst software, the conditions used to generate each calibration model was based on obtaining the lowest standard error of prediction when the model accuracy was evaluated. Models were developed using the wavenumber range 9000–4000 cm−1 for the benchtop NIRS data and 6250–4167 cm−1 for the handheld NIRS data. The data pre-treatments were examined using 1st or 2nd order derivatives calculated using the Savitzky–Golay smoothing method and Pareto scaling. With regards to the selection of calibration and validation sample data, TQ analyst uses the information related to the analyte found in the components table, to construct a multidimensional sample space. Statistical guidelines, related to spectral and/or concentration data and built into the software, are then employed to randomly select the calibration/validation standards from the space sample model which is reiterated until a complete set of each is generated. Statistical analysis of the proximate NIR benchtop and handheld data was carried out using IBM SPSS Statistics 21 software (England, UK).
Results and discussion
Proximate analysis comparison between NIRS instruments
To test the comparability of the handheld and benchtop instruments, batches of poultry from the same diet composition were obtained. The results for the NIRS proximate analysis for a range of parameters included protein, moisture and fat. Fig. 1 shows a graphical representation of the mean values for the proximate analysis acquired by handheld and benchtop NIRS which shows a good comparison between the instruments.
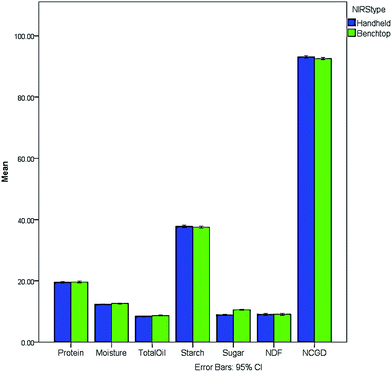 |
| Fig. 1 Mean values (%) for proximate analysis measured by handheld (in blue) and benchtop (in green) NIRS with 95% confidence limits. | |
To further compare the results, statistical analysis of the study, conducted using IBM SPSS Statistics 21 software, was carried out. The results of the proximate analysis given by the handheld and benchtop NIRS were compared by running a paired samples t-test with a confidence interval of 95%, and the results are presented in Table 1. No statistically significant differences could be observed between the mean handheld and benchtop measurements for protein, starch and Neutral Detergent Fibre (NDF) contents. On the other hand, the mean values for moisture, total oil, sugar and Neutral Cellulase Gammanase Digestibility (NCGD) appear to vary significantly depending on the type of instrument as indicated by the p-values obtained. This was especially noticeable for sugar, which has shown the major difference between handheld and benchtop mean values, whereas the differences for total oil and NCGD appear to be slightly less significant. However, due to the wide specifications laid down by the feed manufacturers for each quality parameter, the differences found by the student t-test would not cause undue concern. Within the remit of the current project, only twenty-one samples of poultry feed were used due to the time constraints involved. However any future comparison should include more samples as well as different diets e.g. other poultry feeds or other species feeds. The results indicate that it could be possible to use the handheld instrument for crude nutritional analysis as a replacement for the more expensive benchtop instruments with the added value that it could be used in the field e.g. feed mill or ports of entry.
Table 1 Results of paired samples t-test between handheld and benchtop NIRS results from proximate analysis (NDF: Neutral Detergent Fibre. NCGD: Neutral Cellulase Gammanase Digestibility)
|
Paired differences |
t-value |
Significance level, p (2-tailed) |
Mean difference |
Standard deviation |
Standard error mean |
Protein |
−0.113 |
0.654 |
0.143 |
−0.793 |
0.437 |
Moisture |
−0.287 |
0.154 |
0.034 |
−8.539 |
0.000 |
Total oil |
−0.220 |
0.278 |
0.061 |
−3.632 |
0.002 |
Starch |
0.268 |
0.927 |
0.202 |
1.326 |
0.200 |
Sugar |
−1.638 |
0.633 |
0.138 |
−11.86 |
0.000 |
NDF |
−0.006 |
0.565 |
0.123 |
−0.052 |
0.959 |
NCGD |
0.599 |
0.870 |
0.190 |
3.153 |
0.005 |
Qualitative analysis of melamine adulterated soya
The soya products obtained from the local animal feed company were selected for spiking at adulterated levels with melamine (0.25, 0.5, 0.75, 1, 1.25, 1.5, 1.75 and 2% w/w). Fig. 2 shows the NIRS spectra of 100% soya, 2% melamine in soya ran on both the handheld and benchtop instruments in addition to pure melamine spectrum. As indicated in this figure the range of the handheld instrument is limited to 6250–4167 cm−1 compared to the benchtop instrument whose range could extend to 12
000 cm−1. Although a number of peaks due to melamine structure can be seen in the 6250–4167 cm−1 region, it is noteworthy to point out that the distinct peak observed and highlighted at ∼6800 cm−1 in Fig. 2 in relation to the benchtop data, is due to the first overtone of the amine functional groups present in the molecule. The intensity of this peak decreases as the amount of melamine decreases and due to the limited spectral range of the handheld instrument was not observed in this case. To compare the qualitative data from the instrumentation, the PCA algorithm was applied to spectral data. Fig. 3(a) shows the scores plots for each PCA with pre-processing using standard normal variate (SNV) only. For the model built using the handheld data eleven principal components were generated with the majority of the variation explained by the first two (PC1: 94%; PC2: 2.89%). In contrast, the model built using the benchtop data generated only three PCs with the first two explaining the majority of the variance (PC1: 96.2%; PC2: 3.53%). Visually the PCA scores are very different in that there was very distinct separation between the adulterated and pure soya classes with the benchtop NIR data which was not observed in the other plot for the handheld data, although there was some discrimination observed in the latter especially as the concentration of melamine increased in the adulterated samples. It is postulated that the influence of the peak at ∼6800 cm−1 from melamine which is included in the benchtop instrument data is significant when it comes to qualitative discrimination based on the differences between the PCA models, as this is an unsupervised qualitative technique with its discriminatory power based purely on differences found in the spectroscopic data.
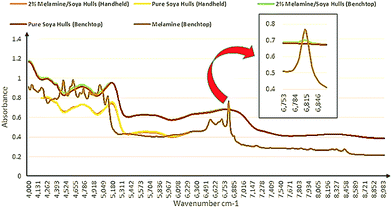 |
| Fig. 2 Typical NIRS spectra from handheld and benchtop instruments. | |
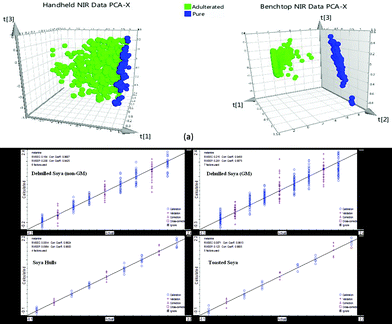 |
| Fig. 3 (a) PCA scores plots for the qualitative analysis of NIRS data from handheld and benchtop instruments. (b) Graphical representation of the quantitative calibration models generated for melamine adulteration of soya products from NIRS data (handheld). | |
Quantitative calibration modelling and validation of melamine adulterated soya data
In a previous publication6 quantitative calibration models were generated using PLS-R (partial least squares regression) and PCR (principal component regression) mathematical algorithms, which convert complex spectral data into analytical parameters, for the benchtop NIR instrument. Table 2 highlights the results for melamine adulterated samples of de-hulled soya (GM & non-GM), soya hulls and toasted soya from calibrations developed from handheld NIR data and compared with the equivalent benchtop data. The data generated for each respective calibration model using the mathematical treatments of the NIRS spectral data includes the corresponding root mean squared error of calibration (RMSEC) and root mean squared error of prediction (RMSEP). These latter values gave an indication of the quality of the calibration models generated. The calibration models for each set of samples were very good with correlation values (R2) between 0.94–0.99 depending on the mathematical algorithm used, the data pre-processing applied and the sample type. The corresponding values for RMSEC and RMSEP were found to be 0.081–0.215% and 0.095–0.288% respectively, again depending on the chemometric treatment of the data and sample type.
Table 2 Partial Least Squares Regression (PLS-R) calibration models generated using spectral data from the handheld (H) and benchtop (B) instruments. R2 is the measure of fit of the calibration model, RMSEC = Root Mean Squared Error of Calibration; RMSEP = Root Mean Squared Error of Prediction; RMSECV = Root Mean Square Error of Cross Validation. For 1st order derivative processing of data, Savitzky–Golay smoothing was applied with Data points = 7 and polynomial order = 2. For 2nd order derivative processing of data, Savitzky–Golay smoothing was applied with data points = 9 and polynomial order = 3
PLS-R |
Dehulled soya/non-GM |
Dehulled soya/GM |
Soya hulls/GM |
Toasted soya/organic |
Data pre-processing |
SNV, 2nd derivative, Savitzky–Golay smoothing |
SNV, 1st derivative, Savitzky–Golay smoothing |
SNV, 1st derivative, Savitzky–Golay smoothing |
SNV, 1st derivative, Savitzky–Golay smoothing |
H |
B |
H |
B |
H |
B |
H |
B |
R
2
|
0.9457 |
0.96320 |
0.9687 |
0.98216 |
0.9924 |
0.94614 |
0.9913 |
0.99156 |
RMSEC% |
0.215 |
0.169 |
0.164 |
0.117 |
0.081 |
0.202 |
0.087 |
0.0810 |
RMSEP% |
0.288 |
0.288 |
0.208 |
0.143 |
0.095 |
0.251 |
0.123 |
0.135 |
Factors |
9 |
9 |
6 |
6 |
4 |
7 |
5 |
10 |
RMSECV% |
0.212 |
0.135 |
0.205 |
0.261 |
0.101 |
0.208 |
0.117 |
0.173 |
To further check on the validation of the calibration models generated, cross validation was used where one standard was removed at a time and this was then predicted by the model and compared to the actual value. The results for the root mean square error of cross validation (RMSECV) are shown in Table 2 with the handheld data given RMSECV: 0.101–0.212% and benchtop data giving RMSECV: 0.135–0.261%. Fig. 3(b) shows the graphical representation for each of the calibration models generated for each soya sample type with actual concentration on X-axis versus calculated (predicted) concentration on the Y-axis (based on the NIR data). The samples used for calibration have been indicated by the circles whilst those used for validation are indicated by crosses. The models generated by the handheld NIRS data are very promising and have been found to be similar to the models generated by the NIRS data from the benchtop instrument in terms of the similarity between the R2, RMSEC and RMSEP calibration and validation parameters respectively as well as the cross validated RMSECV values.
Conclusions
The comparison between proximate analysis results for both the handheld and benchtop NIRS show that there is potential for the handheld instrument to be used as an alternative to the benchtop instrumentation. Although the Student t-test indicated significant differences in four of the quality parameters, these were minimal and the values were well within the specifications as laid down by the animal feed company. The portability of the handheld instrument means that it could be used in the field e.g. feed mills, ports of entry etc. With regards to the detection of adulteration of soya products with melamine, qualitative analysis using the unsupervised PCA algorithm showed that the benchtop NIRS data give better discrimination than the handheld data probably due to the limitations of the wavenumber range on the latter device (6250–4167 cm−1). The quantitative calibration models produced using the NIRS handheld data, with excellent coefficients of determination (R2 = 0.94–0.99) and the subsequent validation data generated, RMSEC (0.081–0.215%), RMSEP (0.095–0.288%) and RMSECV (0.101–0.212%), indicate that this handheld NIRS approach has the potential to be used to detect fraud and adulteration of soya based products used as animal feed ingredients. Routine testing of shipments of soya based products with NIRS at the port of entry or feed mill could have economic benefits for the animal feed sector where the incoming batches of feed materials could be screened for fraudulent activity leading to safer animal feed, healthier animals and safer food for the consumer.
Acknowledgements
The authors are very grateful for the supply of soya based sample sets and animal feed samples used in this study which were kindly donated by John Thompson and Sons Ltd, Belfast. We would also like to thanks Natalie Cook and Dermot Harrington (Antech Ltd, Ireland) and Shailesh Karavadra (Thermo Fisher Scientific, UK) for their help and support during this project. The work was funded through the Food Fortress project (RD0610617) by Invest Northern Ireland and by the European Commission 7th Framework Project; Quality and Safety of Feeds and Food for Europe, QSAFFE (FP7-KBBE-2010-4, 265702).
References
- E. Zamora-Rojas, D. Pérez-Marín, E. De Pedro-Sanz, J. E. Guerrero-Ginel and A. Garrido-Varo, Chemom. Intell. Lab. Syst., 2012, 114, 30–35 CrossRef CAS PubMed.
- M.-T. Sánchez, M.-J. De la Haba and D. Pérez-Marín, Comput. Electron. Agr., 2013, 92, 66–74 CrossRef PubMed.
- L. F. Capitán-Vallvey and A. J. Palma, Anal. Chim. Acta, 2011, 696, 27–46 CrossRef PubMed.
- L. Salguero-Chaparroa, V. Baeten, O. Abbas and F. Peña-Rodríguez, J. Food Eng., 2012, 112, 152–157 CrossRef PubMed.
-
I. McDonald, http://chiefdisruptionofficer.com/spectrometers-and-molecular-sensors/(accessed September 2014).
- S. A. Haughey, S. F. Graham, E. Cancouët and C. T. Elliott, Food Chem., 2013, 136, 1557–1561 CrossRef CAS PubMed.
- Commission Decision 2008/757/EC imposing special conditions governing the import of products containing milk or milk products originating in or consigned from China, Off. J. Europ. Union, 2008, no. L 259/10–11.
- Commission Decision 2008/798/EC imposing special conditions governing the import of products containing milk or milk products originating in or consigned from China, and repealing Commission Decision 2008/757/EC, Off. J. Europ. Union, 2008, No. L 273/18–20.
- Commission Decision 2008/921/EC amending Decision 2008/798/EC, Off. J. Europ. Union, 2008, No. L 331/19–20.
-
E. C. Y. Li-Chan, P. R. Griffiths and J. M. Chalmers, in Applications of Vibrational Spectroscopy in Food Science, Instrumentation and Fundamental Applications, John Wiley and Sons, Chichester, UK, 2010, vol. 1, p. 665, ISBN: 978-0-470-74299-0 Search PubMed.
- K. Sharma and M. Paradakar, Food Security, 2010, 2, 97–107 CrossRef PubMed.
- X. Pei, A. Tandon, A. Alldrick, L. Giorgi, W. Huang and R. Yang, Food Policy, 2011, 36, 412–420 CrossRef PubMed.
- H. Lei, Y. Shena, L. Songa, J. Yanga, O. P. Chevallier, S. A. Haughey, H. Wanga, Y. Suna and C. T. Elliott, Anal. Chim. Acta, 2010, 665, 84–90 CrossRef CAS PubMed.
- H. Lei, R. Su, S. A. Haughey, Q. Wang, J. Yang, Z. Xu, Y. Shen, H. Wang, Y. Jiang and Y. Sun, Molecules, 2011, 16, 5591–5603 CrossRef CAS PubMed.
- E. A. E. Garber and V. A. Brewer, J. Food Prot., 2010, 73(4), 701–707 CAS.
- W. W. Yin, J. T. Liu, T. C. Zhang, W. H. Li, W. Liu, M. Meng, F. Y. He, Y. P. Wan, C. W. Feng, S. L. Wang, X. A. Lu and R. Xi, J. Agric. Food Chem., 2010, 58(14), 8152–8157 CrossRef CAS PubMed.
- Q. Wang, S. A. Haughey, Y. Sun, S. A. Eremin, Z. Li, H. Liu, Z. Xu, Y. Shen and H. Lei, Anal. Bioanal. Chem., 2011, 399, 2275–2284 CrossRef CAS PubMed.
- T. L. Fodey, C. S. Thompson, I. M. Traynor, S. A. Haughey, D. G. Kennedy and S. R. H. Crooks, Anal. Chem., 2011, 83(12), 5012–5016 CrossRef CAS PubMed.
- H. Miao, S. Fan, Y.-N. Wu, L. Zhang, P.-P. Zhou, J. G. Li, H. J. Chen and Y. F. Zhao, Biomed. Environ. Sci., 2009, 22, 87–94 CrossRef CAS.
- G. Venkatasami and J. R. Sowa Jr, Anal. Chim. Acta, 2010, 665(2), 227–230 CrossRef CAS PubMed.
- L. Vaclavik, J. Rosmus, B. Popping and J. Hajslova, J. Chromatogr. A, 2010, 1217(25), 4204–4211 CrossRef CAS PubMed.
- X. Jiang, Z. Yang and L. Han, Anal. Bioanal. Chem., 2014, 406(19), 4705–4714 CrossRef CAS PubMed.
- J. A. Fernández Pierna, P. Vermeulen, O. Amand, A. Tossens, P. Dardenne and V. Baeten, Chemom. Intell. Lab. Syst., 2012, 117, 233–239 CrossRef PubMed.
- R. M. Balabin and S. V. Smirnov, Talanta, 2011, 85, 562–568 CrossRef CAS PubMed.
- L. J. Mauer, A. A. Chernyshova, A. Hiatt, A. Deering and R. Davis, J. Agric. Food Chem., 2009, 57, 3974–3980 CrossRef CAS PubMed.
- Z. Yang, C. Wang, L. Han, J. Li and X. Liu, J. Innovative Opt. Health Sci., 2014, 7(4), 1350072 CrossRef.
|
This journal is © The Royal Society of Chemistry 2015 |
Click here to see how this site uses Cookies. View our privacy policy here.