DOI:
10.1039/C4RA05728G
(Paper)
RSC Adv., 2014,
4, 38672-38681
Dual mechanism of HIV-1 integrase and RNase H inhibition by diketo derivatives – a computational study†
Received
14th June 2014
, Accepted 11th August 2014
First published on 18th August 2014
Abstract
Development of novel therapeutics for treatment of HIV infections is a very challenging process due to the high rate of viral mutation. On this basis, inhibition of more than one HIV replication pathway is a potential efficient way to obtain control over the HIV progression. In the present study we have performed computational analyses in order to investigate the dual inhibitory action of a set of diketo derivatives (carboxylic acid and esters) against RNase H (RNH) and integrase (IN). Docking studies performed with these compounds revealed that the interaction between the ligands and magnesium ions and the surrounding amino acids/water within the protein are important for the dual inhibitory activity of these compounds. Moreover, from a binding mode analysis, the carboxylic acid (series 8) and ester (series 7) derivatives showed distinct binding patterns in RNH and IN, meaning that all compounds bind with magnesium ions through oxygen atoms of the ligands (either enol or carboxylate); however, the orientation of the hydrophobic tail of the ligand is quite different in both systems. Additional validation using a small dataset also strengthens this binding mode hypothesis. The results reported here could be useful for design or screening of small molecules against IN and RNH activity for the development of effective drugs for HIV treatment.
Introduction
UNAIDS (Joint United Nations Program on HIV/AIDS) estimates that currently more than 34 million people worldwide are infected with HIV-1 and that 2.5 million new HIV infections occur every year.1 After introduction of the HAART (highly active anti-retroviral therapy) concepts at the 11th International Conference on AIDS at Vancouver (British Columbia) in 1996 there has been great progress in HIV therapy. This concept suggests that the combination of several antiretroviral drugs slowdown HIV replications and that this combinational therapy is more effective than mono-drug therapy in order to treat HIV.2 Although AIDS related mortality has been reduced by 24% (1.7 million in 2011) compared to 2005 data (2.3 million), the development of improved anti-HIV regiments is still required. To control HIV progression, several viable chemo-targets have been identified in the HIV replication cycle;3,4 however, from a pharmaceutical point of view, only reverse transcriptase (RT) and protease (PR) have been successful targets for HIV therapy. More than 50% of the currently marketed drugs being used for HIV therapy belong to these target classes. However, due to an increasing drug resistance to HIV-1 strains,5 considerable attention has in recent years been paid to other target sites within the HIV replication process6–8e.g., integrase (IN) and RT associated RNase H (RNH),9 which both are essential for viral replication.
Reverse transcriptase (RT) is an enzyme which reverse transcripts the viral genome (single strand RNA) into double strand DNA (dsDNA) through RNA–DNA hybrid formation using polymerase and RNH domains. Integrase takes over the dsDNA for integration with the genome of the host cell. In order to carry out the catalytic process, water molecules (which act as nucleophiles) and magnesium ions (which initiate the deprotonation of water) coordinating with conserved DDE (Asp64, Asp116 and Glu152 for IN) and DDDE (Asp443, Asp498, Asp549 and Glu478 for RNH) residues are essential.10–12 All RT inhibitors approved by the FDA for the treatment of HIV infections particularly inhibit at the polymerase domain;13 however, there are a large number of RNH inhibitors reported and some are even entered into clinical trial even though none of these inhibitors have yet reached the market. On the other hand, two IN inhibitors, named Raltegravir and Dolutegravir, were recently approved by the FDA in 2007 and 2013, respectively, for HIV treatment.14,15
HIV-1 IN is a 32 kDa protein composed of three structural domains: the N-terminal domain (NTD) (residues 1–50), the catalytic core domain (CCD) (residues 51–212) and the C-terminal domain (CTD) (residues 213–288). Among the retroviral classes, the catalytic core domain is highly conserved and CTD plays an essential role in the enzymatic activity. Due to its low solubility the full-length structure has not yet been reported; however, high quality structures for the individual domains have been determined either by NMR or X-ray crystallography.16–21
RNH is one of the two domains of the p66 (66 kDa) subunit of reverse transcriptase. From mutation and X-ray crystallographic studies, the structure of the RNH domain has been well characterized. It is composed of five standard mixed sheets, which are surrounded by four helixes, and eight loops in the center of the domain.22–25
Although the overall structural folds for RNH and IN are quite different, the topology of the catalytic sites are very similar, e.g. both RNH and IN are composed of DDDE and DDE conserved residues, respectively, in addition to catalytically active magnesium ions (Mg2+) and water molecules. Both enzymes execute their catalytic mechanism through the Mg2+ ions which coordinate to the carboxylate groups of the conserved residues. It has been shown that mutation of any of these residues abolishes the catalytic activity. This is because these residues provide a favorable environment for stabilizing metals which is essential for a proper binding and positioning of the substrate and also important for formation of a nucleophile (OH−) from water by deprotonation.24 A schematic representation of the catalytic process of RNH and IN is shown in Fig. 1A.
 |
| Fig. 1 (A) Schematic diagram of the catalytic mechanism of RNase H and integrase. Conserved residues for both systems are shown in red (integrase) and blue (RNase H) letters. (B) Common scaffolds for series 7 and series 8. | |
With the availability of 3D protein structures and deposit of ligands for both systems, ligand and structure-based modeling are readily used to predict compounds (e.g., virtual screening) and rationalize the ligand selectivity.26–31 Recently, two series (1-benzyl-pyrrolyl diketo acid and ester derivatives) belonging to diketo derivatives were synthesized and the effect of substitution at the benzyl ring was investigated against recombinant reverse transcriptase associated RNH and IN function (Fig. 1B).32 The results demonstrated that the majority of the screened compounds showed good HIV-1 replication inhibitory activity (IC50 ranges from >100 to 0.02 μM). Moreover, SAR studies suggest that molecules in the 1-benzyl-pyrrolyl diketo acid series (in here series 8) show good inhibition against IN and that the 1-benzyl-pyrrolyl diketo ester series (in here series 7) is more active as RNH inhibitors. From a modeling point of view, it is interesting to understand the structural features that discriminate or show the dual mechanism of these classes of compounds as the inhibition mechanism of both systems are quite similar. The aim of the present investigation is to understand the dual mechanism of this class of compounds using ligand and structure based modeling.
Computational material and methods
Dataset preparation
The dataset consists of 50 diketo derivatives collected from a recent publication by Costi et al.32 (Table 1). Briefly, two sets of diketo compounds were synthesized, the first series consists of 1-benzyl-pyrrolyl diketo acids (series 8) and the other series consists of 1-benzyl-pyrrolyl diketo esters (series 7). Reported biological activity (IC50) data was converted to pIC50 values. Molecular structures of all the compounds were built in the Maestro module of the Schrödinger suite (v2013-2)33 and saved in sdf format. Subsequently, these 2D structures were converted into 3D structures using the OMEGA tool (v2.0) employing the MMFF95S force field.34 To predict possible tautomers for the diketo group, we used a test system (2,4-dioxohept-5-enoic acid/ester), which mimics the compounds under investigation. For this, energies and geometries were calculated using DFT based on B3LYP/6-311++G** in combination with the PCM solvation model.35 All DFT calculations were performed using Gaussian 09 program.36
Table 1 Structure and activities of the studied compounds
Id |
Substituents |
Activities (pIC50) |
R1 |
R2 |
R3 |
R4 |
R5 |
RNase Ha |
Integraseb |
Activity reported against HIV-1 RT-associated RNase H activity.
Activity reported against HIV-1 IN ST activity.
|
7a |
H |
H |
H |
H |
H |
— |
4.824 |
7b |
Me |
H |
H |
H |
H |
5.523 |
4.495 |
7c |
H |
Me |
H |
H |
H |
5.018 |
5.097 |
7d |
H |
H |
Me |
H |
H |
5.137 |
— |
7e |
F |
H |
H |
H |
H |
5.201 |
6.000 |
7f |
H |
F |
H |
H |
H |
5.046 |
4.959 |
7g |
H |
H |
F |
H |
H |
5.097 |
4.009 |
7h |
H |
Cl |
H |
H |
H |
4.721 |
5.222 |
7i |
H |
H |
Cl |
H |
H |
5.097 |
4.377 |
7j |
CN |
H |
H |
H |
H |
4.495 |
5.046 |
7k |
H |
CN |
H |
H |
H |
5.301 |
4.886 |
7l |
H |
H |
CN |
H |
H |
5.097 |
— |
7m |
OMe |
H |
H |
H |
H |
— |
4.638 |
7n |
H |
H |
OMe |
H |
H |
5.174 |
3.959 |
7o |
OEt |
H |
H |
H |
H |
— |
4.921 |
7p |
H |
Me |
H |
Me |
H |
5.222 |
4.721 |
7q |
F |
F |
H |
H |
H |
5.097 |
6.276 |
7r |
F |
H |
F |
H |
H |
4.721 |
6.000 |
7s |
F |
H |
H |
F |
H |
5.046 |
6.347 |
7t |
F |
H |
H |
H |
F |
4.721 |
5.398 |
7u |
H |
F |
F |
H |
H |
5.523 |
6.222 |
7v |
H |
F |
H |
F |
H |
5.301 |
6.310 |
7w |
Cl |
H |
Cl |
H |
H |
5.046 |
— |
7x |
Cl |
H |
H |
H |
Cl |
4.658 |
5.769 |
7y |
H |
Cl |
H |
Cl |
H |
— |
5.097 |
8a |
H |
H |
H |
H |
H |
4.824 |
7.046 |
8b |
Me |
H |
H |
H |
H |
4.585 |
6.769 |
8c |
H |
Me |
H |
H |
H |
5.337 |
5.886 |
8d |
H |
H |
Me |
H |
H |
4.769 |
5.921 |
8e |
F |
H |
H |
H |
H |
5.194 |
6.009 |
8f |
H |
F |
H |
H |
H |
4.854 |
6.036 |
8g |
H |
H |
F |
H |
H |
5.602 |
7.585 |
8h |
H |
Cl |
H |
H |
H |
5.046 |
6.509 |
8i |
H |
H |
Cl |
H |
H |
5.301 |
5.387 |
8j |
CN |
H |
H |
H |
H |
5.222 |
5.222 |
8k |
H |
CN |
H |
H |
H |
5.301 |
6.125 |
8l |
H |
H |
CN |
H |
H |
5.222 |
5.769 |
8m |
OMe |
H |
H |
H |
H |
4.796 |
6.276 |
8n |
H |
H |
OMe |
H |
H |
5.523 |
5.387 |
8o |
OEt |
H |
H |
H |
H |
4.194 |
6.509 |
8p |
H |
Me |
H |
Me |
H |
4.796 |
5.796 |
8q |
F |
F |
H |
H |
H |
5.222 |
7.229 |
8r |
F |
H |
F |
H |
H |
5.000 |
7.377 |
8s |
F |
H |
H |
F |
H |
5.301 |
7.284 |
8t |
F |
H |
H |
H |
F |
4.301 |
6.824 |
8u |
H |
F |
F |
H |
H |
4.066 |
5.796 |
8v |
H |
F |
H |
F |
H |
4.854 |
5.921 |
8w |
Cl |
H |
Cl |
H |
H |
5.155 |
5.310 |
8x |
Cl |
H |
H |
H |
Cl |
4.553 |
6.770 |
8y |
H |
Cl |
H |
Cl |
H |
4.523 |
6.013 |
Homology modeling
The model of the HIV-1 RT associated RNH domain was constructed from an X-ray crystal structure (resolution of 1.4 Å) obtained from the Protein Data Bank (PDB ID: 3QIO)23 as shown in our previous study.29 In the present study, we modeled the full-length IN structure based on the PFV structure (resolution 2.65 Å, PDB ID: 3OYA) reported by Hare et al.16 Briefly, the HIV-1 IN sequence (288 amino acids, 1148–1435) was obtained from UniProt.37 This sequence was imported into Prime (version 3.4, Schrödinger, LLC, New York, NY, 2013), a homology modeling tool from Schrödinger, and the structure 3OYA was used as a template to build a homology model. The sequence of IN was aligned with the PFV structure as previously reported.16,17 In the secondary structure prediction, the bound ligand (RZL), magnesium ions and three water molecules, which lies close to the magnesium ions, were also included. Models were constructed using a knowledge based method (construct insertion and close the gaps based on the known structure). Subsequently the final model was used for the optimization process in the Protein Preparation Wizard as implemented in Schrödinger.38 This protein structure optimization includes adding hydrogen atoms, assigning correct bond orders and building of di-sulfide bonds. The protonation states of all the ionizable residues were predicted by PROPKA39 provided in the Protein Preparation Wizard in the presence of the Mg2+ ions at the active site. Finally, the optimized model was energy minimized (only hydrogen atoms) using the OPLS 2005 force field.
Docking methodology
The docking experiments were performed using the grid based exhaustive search algorithm implemented in the Glide module of Schrödinger suite.40 Glide uses a series of hierarchical filters to find possible ligand binding poses in the active site, and the program has the option to treat the ligand fully flexible or rigid during the docking run. SP (standard precision) docking and scoring is often recommended for prediction of binding poses, virtual screening and ranking due to its efficient and relative accuracy in pose prediction.41,42 The docking settings used in this study are described elsewhere29 (see also ESI†).
Grid-based fingerprint for ligand and protein (FLAP) modeling
The software FLAP43 was used to build and validate ligand based models. FLAP uses fingerprints derived from GRID molecular interaction fields (MIFs) and GRID atom types are characterized as quadruplets of pharmacophoric features. The GRID approach is a well assessed concept for determining energetically favorable interaction sites in molecules with known structures using chemical probes e.g., H, O, N1, and DRY probes which describe the shape, hydrogen bond acceptor, hydrogen bond donor and hydrophobic interactions, respectively. The distance (i.e. spatial resolution) between two GRID points was set to 0.75 Å.
Protein ligand interaction fingerprint
In order to better understand the protein–ligand interaction patterns of the different binding modes of the diketo derivatives, it is of interest to analyze which residues in the protein and which type of interactions are involved in the binding of the ligands in RNH and IN. To do this, we performed a PLIF (Protein–Ligand Interaction Fingerprint) analysis as implemented in the MOE software44 (see also ESI†).
Evaluation of the models
Reproduction of the bound conformation of co-crystallized ligands based on docking experiments were evaluated in terms of their atom-positional root mean square deviation (RMSD) with respect to the bound conformation of the ligands for both systems. 3D QSAR models were assessed through leave-one-out (LOO) cross-validated squared correlation coefficient (Q2).
Results and discussion
Diketo derivatives bind to IN or RNH through a two-metal–ion chelation mechanism, meaning that either the acid or enolic groups of the ligand chelates with the magnesium ions. Recently, Liao et al.45 have studied the tautomerism of IN inhibitors using density functional theory (DFT). Their study suggests that the carboxylic acid groups are deprotonated and that the enol tautomer is more stable compared to the keto tautomer. Moreover, at biological pH and in presence of magnesium ions, the enolic form may undergo deprotonation which facilitates the chelation formation. Here we have performed free-energy based tautomer stability calculations on a test system i.e. 2,4-dioxohept-5-enoic acid and ethyl 2,4-dioxohept-5-enolate using the keto and enolic forms. According to QM based energy optimizations (B3LYP/6-311++G** level, PCM solvation model) of the test systems, the enolic form 1 was found to be more stable than the enolic form 2 (Fig. 2). The keto form also exists to some extent. This observation is in good agreement with previously reported calculations.45 Therefore, the rest of this study was carried out with the enolic form 1 for both acidic and ester derivatives.
 |
| Fig. 2 Possible tautomers of the test system (2,4-dioxohept-5-enoic acid and ethyl 2,4-dioxohept-5-enolate). | |
Homology model and validation
Due to the unavailability of full-length IN structural models, we have in this work built a full-length homology model based on the recently published PVF X-ray structure.16 In comparison to the PVF structure, the generated model shares very similar overall folds, which includes the catalytic domain, C-terminal domain (CTD) and N-terminal domain (NTD) (Fig. 3A). Although the overall fold is very similar in both cases, the PVF structure has a unique N-terminal extension (NET) domain that is absent in HIV-1 IN (Fig. 3B). By comparison to the crystal structure of the CCD domain reported for HIV-1 (PDB ID: 3L3U, 3NF8, 4DMN and 1BIZ), we find our model to be quite similar with RMSDs between 1.7 and 2.7 Å (a comparison of the catalytic domains of different structures is provided in the ESI,†Fig. 1), and also similar to the homology model reported by Johnson et al.46 In order to validate the docking results and to characterize the binding site our homology model was built with the crystal bound ligand (RLZ). Initially, RLZ was docked into the homology model of HIV-1 integrase in order to validate the docking performance of the program by reproducing the binding pose of RLZ and the RMSD was calculated between the X-ray bound conformation and the pose predicted by the Glide docking. The best 10 poses were analyzed. From the results, the 4 best poses of the 10 docking poses reproduced the crystal bound conformation with a RMSD less than 2.0 Å, moreover, the first ranked pose had a RMSD of 1.09 Å (ESI,†Fig. 2A). Analyzing the binding mode of the best pose, the docked binding pose containing the ligand chelated with magnesium ions within distance <2 Å and the magnesium ions are furthermore strongly coordinated with three bound water molecules with distances ∼2 Å. As reported previously, the halogenated benzyl group and the methyl oxadiazole ring of RLZ is involved in π–π interaction with Pro145 and Phe143 in IN. However, an additional hydrogen bond between the oxygen atom of the oxadiazole ring with Asn144 is absent in the docking pose compared to the bound pose. During the docking, the oxadiazole ring is flipped on the opposite side, which could be the reason for the quite high RMSD value of the docking pose.
 |
| Fig. 3 (A) The structure of the full-length HIV-1 integrase homology model is shown in cartoon representation with magnesium ions (green), water molecules (red) and important residues shown in stick representation. (B) Comparison of the homology model (surface) with the PFV structure (PDB ID: 3OYA). The active site is shown in yellow. | |
On the other hand, the RNH homology model could reproduce the bound conformation of 3-hydroxy-6-(phenylsulfonyl)-quinzoline-2,4-(1H,3H)dione (NHQD) using the same docking protocol as for IN. The RMSD of this docking pose was 0.23 Å and we observed similar interaction with the active site residues as for the bound conformation (ESI,†Fig. 2B); for instance, His539 and magnesium ion (2) interacts with one of the three oxygen atoms of NHQD and the neighboring two oxygen atoms interact with the other magnesium (1) ion and bound water molecules. As seen from both validations, the first ranked docking pose reproduces the bound conformation, thus, the first ranked compounds of both series (series 7 and 8) were analyzed in order to study the dual mechanism.
Binding mode analysis
Initially, the binding poses of series 8 (8a–8y) and series 7 (7a–7y) derivatives were analyzed for RNH. In general, the carboxylate group in the molecules is oriented towards the two magnesium ions and water molecules (especially water no. 17 and 24). The binding modes of all acid derivatives are very similar in terms of their interactions with the magnesium ions by the carboxylate group and the position of the hydrophobic tail of the ligands (Fig. 4). In contrast to series 8, series 7 compounds bind with magnesium ions through “three-oxygen-coordinates”, meaning that the enolic and keto oxygens are arranged in the same plan for chelation. The middle keto-oxygen is slightly inserted between the two magnesium ions and the ethoxy group is pointing out of the active site. However, only the deprotonated enolic oxygen atom binds strongly with the magnesium ions. All series 7 compounds favorably bind with Arg557 which performs π–cation interactions with the pyrrole ring and salt bridge formation between the deprotonated enolic oxygen atom and magnesium ions (so called left-tail mode). Similar to Arg557, the His539 residue is also involved in π–π stacking with the pyrrole ring and hydrogen bonds with the enolic oxygen. Moreover, the majority of series 8 compounds chelate with the two magnesium ions through the terminal mono-oxygen atom, in case of the 8n, 8w and 8x compounds, both enolic and terminal oxygen atoms bind with the magnesium ions, which is also reflected in the relatively high docking score of these compounds (>−8.0 kcal mol−1). This observation is also in good agreement with experiment as these compounds have quite strong RNH inhibition (3–28 μM) compared to the other compounds. Another common interaction of series 8 compounds with RNH is that all compounds are either exposed or involved in π–cation interactions with Lys540 and that the keto-oxygen next to the enolic group binds with water which in turn is coordinated with the conserved residue Asp498. (Binding mode comparison of poor and good inhibitors is provided ESI,†Fig. 3A–F). Although the binding mode of series 8 compounds make chelation and hydrogen bonding with magnesium ions and waters, respectively, orientation of tail of these compounds are very different and majority of them are exposed to large number of hydrophobic residues such as Val536, Ala538 and Pro537 of the RNH, this called right-tail binding mode.
 |
| Fig. 4 Comparison of binding poses of the compounds in series 7 and 8 at the RNase H binding site. (A) Overall view of HIV-1 reverse transcriptase and various sub-domains and ligand binding sites are highlighted, including RNase H domain (blue). Important residues are highlighted in yellow. | |
On the other hand, both series 7 and 8 binding modes in IN show a quite different binding pattern compared to RNH, where series 7 and 8 compounds bind in a left (tail of the ligand is orientated between the residues E152 and P145) or right-tail (tail of the ligand is orientated between the residues F143 and P117) binding mode, respectively. For IN, the majority of the series 8 highly active compounds bind similar to the “right-tail” compounds in the RNH series 7, and the low active series 8 compounds show a “left-middle-right-tail” binding pattern. A comparison between series 7 and 8 compound binding poses in IN is shown in Fig. 5A and B (a binding mode comparison of poor and good inhibitors is provided Supplementary Information, Fig. 4A and B). In order to explain this concept better, the activity ranges were divided into three classes; highly active (pIC50 ≥ 6 μM), moderately active (5 < pIC50 > 6 μM) and low active (pIC50 < 5 μM) compounds and the binding modes were analyzed according to this grouping. As shown in Fig. 6a large portion of the highly active compounds (19 out of 22) lies on the right-tail mode, surprisingly none of them are oriented to the left-tail mode and 3 compounds show middle-tail mode. 9 out of 16 moderate active compounds bind in right-tail mode and only 2 compounds show left-tail binding. Furthermore, out of 13 low active compounds, 5 compounds show left tail and the remaining 5 compounds middle-tail binding mode. Noticeably, only a minor number of compounds (3) show right-tail mode. This analysis suggest that based on the binding orientation of the tail, the compounds can be classified into either highly active or low active compounds. This pattern was not observed in the RNH binding poses, where all series 7 compounds show left-tail mode and series 8 compounds right-tail binding mode, which is also reflected by experiment i.e., series 7 compounds are favorable for RNH inhibition and series 8 compounds bind strongly to IN. Overall, based on the binding orientation of the tail of the molecules in both series, one could suggest that if the compound's tails orient to the left side of the active site, the probability of showing RNH inhibition is quite high and for IN it is a right-tail orientation. A common interaction pattern of series 8 compounds is that the majority of the compounds is found to be involved in π–π interaction with Phe143 and exposed to the Pro142 residue, in particularly the pyrrole ring. At least one of the 3 water molecules participate in hydrogen bonding network with the ligands in addition to the magnesium ions. In terms of chelation, series 8 compounds bind with the two magnesium ions through two reactive oxygen species; in case of series 7, only one oxygen (enol) atom is involved in the chelation with the two magnesium ions. This phenomena could also explain why series 7 compounds are low potent as compared to series 8 against IN.
 |
| Fig. 5 Comparison of series 7 (A) and 8 (B) binding mode in the integrase active site. | |
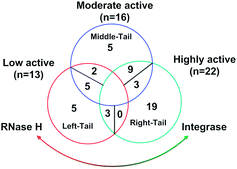 |
| Fig. 6 Venn diagram of classification of RNase H and integrase compounds according to the binding pose of the tail of the series 7 and 8 compounds. | |
In order to analyze the influence of water molecules to the binding mode prediction, an additional docking experiment was performed without considering water molecules at the active site. As seen from Fig. 7A and B the binding pose of series 8 compounds did not change (reproduce the right-tail mode); however ∼50% of the compounds in series 7 follow left-tail mode and the rest of the compounds fall into right-tail mode. This observation indicates that the inclusion of water molecules in the docking experiments play a significant role in the binding pose prediction as observed for the other proteins.47
 |
| Fig. 7 (A) Comparison of binding mode of representative compounds in series 7 (green) and series 8 (cyan) at the binding site of RNase H from the docking experiment without water molecules. Compounds that showed right-tail mode are also shown (violet). Protein–ligand interaction diagram for series 8 compound (B) and series 7 compounds that showed left-tail mode (C) and right-tail mode (D). | |
Validation of binding mode hypothesis
In the following we focus on classifying a small external dataset containing quinolinonyl diketo acid derivatives screened for RNH and IN. These compounds were also screened in the same experimental assay and under the same conditions as the diketo derivatives used for this study.48 In total, 17 compounds were tested against IN and 8 tested against RNH. All compounds were built and preprocessed as described in the method section. The compounds were docked into the RNH and IN models and the poses were subsequently analyzed. Out of 17 compounds docked into the IN binding site, only two active compounds (12b and 12i) misclassified into the left-tail mode as low active compounds and the rest of the compounds in the dataset followed the right-tail binding mode. For RNH, all compounds fall into the left-tail binding mode as proposed previously. Interestingly, compound 2 (IC50 > 100 uM), which possesses a right-tail binding mode, is correctly classified as an inactive compound in accordance to what was proposed earlier based on the large dataset (Fig. 8).
 |
| Fig. 8 Comparison of the binding mode of the external dataset compounds (quinolinonyl diketo acid derivatives) in IN (left) and RNH (right). Misclassified compounds in IN and RNH are shown in green and pink sticks, respectively. | |
Protein–Ligand Interaction Fingerprints (PLIF)
PLIF is a highly valuable analysis tool that helps to examine residue interactions with ligands in a high-throughput screening mode. This method summarizes the different interactions e.g., hydrogen bonds (acceptor and donor), ionic interactions and surface contacts of ligand–protein complexes and could be useful in order to differentiate inhibition differences of a set of particular compounds with respect to the interaction with the receptor (a summary of the PLIF result is provided in the ESI Table 2A and B†).
RNH.
The studied molecules possess nearly 50 interactions with RNH. The major interactions are surface contact (C), side chain donor and/or acceptor (D/A), backbone donor and/or acceptor (d/a), ionic interactions (I), and solvent donor and/or acceptor (O) interactions. It is interesting to note that water molecules such as 17, 562 and 563 have 100% abundance for the solvent acceptor interaction with the hydrogen donor groups in the molecules. Molecules in both series 7 and series 8 bind with the two magnesium ions. However, compounds 8a–8y have a terminal COO− ion in addition to the enolic group and this makes them more strongly binding with magnesium ion compared to the series 7 compounds, which have interactions with the metal ions primarily through the enolic oxygen atoms. Due to the substitution in the terminal carboxylic group with an ethyl group, an overall chelation formation is slightly different, meaning that all compounds are positioned slightly away from the magnesium ions compared to series 8 where the terminal carboxylate ion pointing towards the magnesium ions. Side chain acceptor and ionic interactions were observed to residue Arg557, but the significant difference is that ionic interactions are only observed for compounds 8a–8y and side chain acceptor interactions are observed primarily for the 7a–7y compounds, i.e., the molecules that possess substitutions mainly on the meta (R2) position on the phenyl ring and compounds with CN, F, OCH3, etc. in the ortho (R1) position. Residues such as Asp443, Asn474 and Asp498 possess substantial interaction through side chain donor (Asp443 and Asp498) and surface contact (Asn474) with the compounds containing ester groups (7a–7y). Another important interaction observed for these compounds (7a–7y) is side chain donor interactions with Asp549 (∼25%). The majority of the compounds in series 7 interact with His539 and Ala538 through side chain and backbone acceptor interactions as the binding pose is left-tail mode as shown in the previous section.
Integrase.
Both series 7 and 8 possesses nearly 40 interactions with the amino acids, water and magnesium ions. More than 90% of the compounds in the dataset show interaction with water 400 and 534 either through solvent acceptor or donor. Water 401 also interacts significantly with the compounds (67% abundance). In contrast to RNH, with IN, the magnesium ions have better interaction with the ethyl ester group (7a–7y) containing compounds than the compounds containing a carboxylic group (8a–8y). The magnesium ion (1) possesses better interaction with the carboxylic group than the magnesium ion (2). The majority of the compounds (>90%) in the dataset showed interaction with Pro145 and most of the compounds in series 8 have surface contact with Phe143. It is important to note that conserved residues such as Asp64 and Asp116 primarily interact with series 7 compounds compared to series 8. Gly118 makes surface contact with some of the series 8 compounds and this was nearly absent in series 7 compounds. Glu152 possesses significant side chain donor interactions (>50%) with the molecules containing a carboxylic acid group.
In general, for both enzymes, the metal ions (Mg2+) play an important role for the activity elicitations. The water molecules in the active sites have almost 100% abundance for the interaction through hydrogen bonding (either acceptor or donor). In integrase, the magnesium ion (1) has better coordination with the majority of the compounds compared to magnesium ion (2). In both systems, chelation was observed for all compounds. The carboxylate group containing compounds (8a–8y) have strong interaction with Arg557 in RNH and for series 7 compounds, only the side chain acceptor (a), donor (d) and surface interactions with Asp443, Asn474, Asp498 and Arg557 were observed. The compounds in series 7 and 8 are more exposed to the active site residues of IN compared to RNH, this is due to the tail-mode concept, e.g. right, middle, and left-tail mode.
FLAP models
The docking poses of series 7 and 8 for RNH and IN were used as initial structures in the FLAP modeling. For RNH and IN, we chose the highly active top 5 compounds for alignment and the aligned models were used to generate a pharmacophore psuedomolecule, which consist of pharmacophoric points. The obtained pharmacophore models were used to build 3D-QSAR (Partial Least Square with 5 latent variables) and Leave-one-out cross validation was used to assessed the quality of the final model (ESI,† Table 2). In general, models obtained for RNH were very poor in terms of the cross-validation (Q2), therefore we could not use it for interpretation of the dual mechanism. However, models derived for IN found to be moderate with correlation coefficient of 0.88 (Q2 = 0.42, SDEP = 0.66) for latent variable 3. The MIF (molecular interaction fields) of the highly active compounds and poor inhibitory compounds were analyzed. It is noted from Fig. 9 that hydrogen bond acceptors in the molecule is essential in order to possess the IN and RNH inhibition. The compounds 8g (both RNH and IN active) and 7w (active against RNH and inactive for IN) were analyzed. The result suggests that the psuedomolecule for IN is well suited for series 8 compounds compared to series 7 as compounds in series 7 are slightly moved from the pharmacophoric atom position (Fig. 9A and B) although they share similar structural pattern other than the substituted ethoxyl group. On the other hand, we analyzed the psuedomolecule of RNH (Fig. 9C and D) and compared to the highly active compounds from both series (8g/7b). As expected both molecules aligned very nicely to the pharmacophoric atoms derived from the highly active RNH compounds. Overall this suggests that the series 8 compounds share similar pharmacophore alignment to both system, and series 7 compounds suite only to the RNH pharmacophore atoms. This observation is also in agreement with conclusion derived from experiment.
 |
| Fig. 9 Comparison of MIF derived from RNase H (A and B) and integrase (C and D) pharmacophore models. Here A, B, C, D denotes compound 8g, 7b, 8g, 7w respectively. N1 probe (blue surface with energy level 0.2 kcal mol−1), DRY probe (green surface with energy level −0.1 kcal mol−1) O probe (red surface with energy level −0.5 kcal mol−1). The pseudo-pharmacophore atoms are represented in blue and red spheres. | |
Conclusions
In the present study we have investigated the dual mechanism of diketo derivatives (compounds 7a–7y and compounds 8a–8y) against HIV-1 RNH and IN through computational methods. Although both series share a common scaffold and show RNH and IN activity, the pharmacophore pattern of both systems is quite different in terms of distance and position of hydrophobic sites to hydrogen bond acceptors which is essential for binding to the magnesium ions. As previously reported from experiment, our computational models also suggest that the ester derivatives (7a–7y) are relatively high active against RNH and the carboxylic acid group containing compounds (8a–8y) show better activity against IN. Although all compounds bind to the magnesium ions quite similarly, the most deciding factor for RNH or IN inhibition is how well the ligand's tail orients in both system, e.g., the binding mode or position of the tail is classified into “left, middle, right-tail” binding modes. The majority of series 7 compounds favor “left-tail” mode while the majority of series 8 compounds prefer “right-tail” mode. The orientation of the tail in these classes of compounds dictates a specific type of interaction with residues (Asp443, Asn474, Asp498, Lys540 and Arg557 for RNH and Asp64, Asp116, Gly118 and Glu152 for IN) in the catalytic site and this factor impacts the overall inhibitors activation against IN and RNase H. The presence of an additional formal negative charge on the compound has a significant impact on the activity of IN compared to RNH.
Inclusion of negatively charged functional groups with flexible bonds in the ligands can lead to favorable interactions with the active site metals and water molecules. The aromatic ring/hetero-aromatic rings connected with the charged pharmacophoric group in the compounds can make π–π interaction or hydrogen bonding interactions with the His539 and Arg557 residue in the RNH and Phe143 or Pro145 residues in IN. The observations from this study could be useful in order to design selective or dual inhibition of RNH/IN for the development of effective ant-HIV agents in AIDS therapeutics.
Conflict of interest
The authors declare no conflict of interest.
Acknowledgements
This work has been supported by the Lundbeck Foundation, The Danish Councils for Independent Research and the Villum Foundation. The authors are also thankful to the ChemAxon and OpenEye scientific software for providing an academic license.
References
- UNAIDS, Global report: UNAIDS report on the global AIDS epidemic 2012, Joint United Nations Programme on HIV/AIDS 2012.
-
J. D. Barlett, Medscaps, 2006 Search PubMed (Feb, 8), http://www.medscape.org/viewarticle/523119.
- E. De Clercq, Biochim. Biophys. Acta, Mol. Basis Dis., 2002, 1587, 258–275 CrossRef CAS.
- M. M. Zdanowicz, Am. J. Pharm. Educ., 2006, 70, 100 CrossRef PubMed.
- T. Imamichi, Curr. Pharm. Des., 2004, 10, 4039–4053 CrossRef CAS PubMed.
- W. C. Greene, Z. Debyser, Y. Ikeda, E. O. Freed, E. Stephens, W. Yonemoto, R. W. Buckheit, J. A. Este and T. Cihlar, Antiviral Res., 2008, 80, 251–265 CrossRef CAS PubMed.
- T. L. Hartman and R. W. Buckheit, Jr, Mol. Biol. Int., 2012, 2012, 401965 Search PubMed.
- L. J. Martinez, Res Initiat Treat Action, 2002, 8, 23–25 Search PubMed.
- J. Q. Hang, Y. Li, Y. Yang, N. Cammack, T. Mirzadegan and K. Klumpp, Biochem. Biophys. Res. Commun., 2007, 352, 341–350 CrossRef CAS PubMed.
- M. A. Parniak, K. L. Min, S. R. Budihas, S. F. Le Grice and J. A. Beutler, Anal. Biochem., 2003, 322, 33–39 CrossRef CAS PubMed.
- P. A. Rice and T. A. Baker, Nat. Struct. Biol., 2001, 8, 302–307 CrossRef CAS PubMed.
- C. Marchand, A. A. Johnson, R. G. Karki, G. C. Pais, X. Zhang, K. Cowansage, T. A. Patel, M. C. Nicklaus, T. R. Burke, Jr and Y. Pommier, Mol. Pharmacol., 2003, 64, 600–609 CrossRef CAS PubMed.
- P. Bean, Clin. Infect. Dis., 2005, 41(1), S96–S100 CrossRef CAS PubMed.
- FDA, Antiretroviral drugs used in the treatment of HIV infection, http://www.fda.gov.
- M. Anker and R. B. Corales, Expert Opin. Invest. Drugs, 2008, 17, 97–103 CrossRef CAS PubMed.
- S. Hare, S. S. Gupta, E. Valkov, A. Engelman and P. Cherepanov, Nature, 2010, 464, 232–236 CrossRef CAS PubMed.
- L. Krishnan and A. Engelman, J. Biol. Chem., 2012, 287, 40858–40866 CrossRef CAS PubMed.
- J. C. Chen, J. Krucinski, L. J. Miercke, J. S. Finer-Moore, A. H. Tang, A. D. Leavitt and R. M. Stroud, Proc. Natl. Acad. Sci. U. S. A., 2000, 97, 8233–8238 CrossRef CAS PubMed.
- M. Cai, Y. Huang, M. Caffrey, R. Zheng, R. Craigie, G. M. Clore and A. M. Gronenborn, Protein Sci., 1998, 7, 2669–2674 CrossRef CAS PubMed.
- J. Y. Wang, H. Ling, W. Yang and R. Craigie, EMBO J., 2001, 20, 7333–7343 CrossRef CAS PubMed.
- V. Molteni, J. Greenwald, D. Rhodes, Y. Hwang, W. Kwiatkowski, F. D. Bushman, J. S. Siegel and S. Choe, Acta Crystallogr., Sect. D: Biol. Crystallogr., 2001, 57, 536–544 CrossRef CAS.
- D. M. Himmel, K. A. Maegley, T. A. Pauly, J. D. Bauman, K. Das, C. Dharia, A. D. Clark, Jr, K. Ryan, M. J. Hickey, R. A. Love, S. H. Hughes, S. Bergqvist and E. Arnold, Structure, 2009, 17, 1625–1635 CrossRef CAS PubMed.
- E. B. Lansdon, Q. Liu, S. A. Leavitt, M. Balakrishnan, J. K. Perry, C. Lancaster-Moyer, N. Kutty, X. Liu, N. H. Squires, W. J. Watkins and T. A. Kirschberg, Antimicrob. Agents Chemother., 2011, 55, 2905–2915 CrossRef CAS PubMed.
- J. F. Davies, 2nd, Z. Hostomska, Z. Hostomsky, S. R. Jordan and D. A. Matthews, Science, 1991, 252, 88–95 Search PubMed.
- J. G. Julias, M. J. McWilliams, S. G. Sarafianos, W. G. Alvord, E. Arnold and S. H. Hughes, J. Virol., 2003, 77, 8548–8554 CrossRef CAS PubMed.
- G. M. Ko, A. S. Reddy, R. Garg, S. Kumar and A. R. Hadaegh, Curr. Comput.–Aided Drug Des., 2012, 8, 255–270 CrossRef CAS PubMed.
- A. L. Perryman, S. Forli, G. M. Morris, C. Burt, Y. Cheng, M. J. Palmer, K. Whitby, J. A. McCammon, C. Phillips and A. J. Olson, J. Mol. Biol., 2010, 397, 600–615 CrossRef CAS PubMed.
- S. Distinto, F. Esposito, J. Kirchmair, M. C. Cardia, M. Gaspari, E. Maccioni, S. Alcaro, P. Markt, G. Wolber, L. Zinzula and E. Tramontano, Eur. J. Med. Chem., 2012, 50, 216–229 CrossRef CAS PubMed.
- V. Poongavanam and J. Kongsted, PLoS One, 2013, 8(9), e73478, DOI:10.1371/journal.pone.0073478.
- C. Liao, R. G. Karki, C. Marchand, Y. Pommier and M. C. Nicklaus, Bioorg. Med. Chem. Lett., 2007, 17, 5361–5365 CrossRef CAS PubMed.
- L. Han, Y. Wang and S. H. Bryant, BMC Bioinf., 2008, 9, 401 CrossRef PubMed.
- R. Costi, M. Métifiot, F. Esposito, G. Cuzzucoli Crucitti, L. Pescatori, A. Messore, L. Scipione, S. Tortorella, L. Zinzula, E. Novellino, Y. Pommier, E. Tramontano, C. Marchand and R. Di Santo, J. Med. Chem., 2013, 56, 8588–8598 CrossRef CAS PubMed.
- Schrödinger Release 2014-1: Maestro, version 9.7, Schrödinger, LLC, New York, NY, 2014.
- P. C. Hawkins, A. G. Skillman, G. L. Warren, B. A. Ellingson and M. T. Stahl, J. Chem. Inf. Model., 2010, 50, 572–584 CrossRef CAS PubMed.
- J. Tomasi, B. Mennucci and R. Cammi, Chem. Rev., 2005, 105, 2999–3093 CrossRef CAS PubMed.
-
M. J. Frisch, G. W. Trucks, H. B. Schlegel, G. E. Scuseria, M. A. Robb, J. R. Cheeseman, G. Scalmani, V. Barone, B. Mennucci, G. A. Petersson, H. Nakatsuji, M. Caricato, X. Li, H. P. Hratchian, A. F. Izmaylov, J. Bloino, G. Zheng, J. L. Sonnenberg, M. Hada, M. Ehara, K. Toyota, R. Fukuda, J. Hasegawa, M. Ishida, T. Nakajima, Y. Honda, O. Kitao, H. Nakai, T. Vreven, J. A. Montgomery, Jr., J. E. Peralta, F. Ogliaro, M. Bearpark, J. J. Heyd, E. Brothers, K. N. Kudin, V. N. Staroverov, R. Kobayashi, J. Normand, K. Raghavachari, A. Rendell, J. C. Burant, S. S. Iyengar, J. Tomasi, M. Cossi, N. Rega, J. M. Millam, M. Klene, J. E. Knox, J. B. Cross, V. Bakken, C. Adamo, J. Jaramillo, R. Gomperts, R. E. Stratmann, O. Yazyev, A. J. Austin, R. Cammi, C. Pomelli, J. W. Ochterski, R. L. Martin, K. Morokuma, V. G. Zakrzewski, G. A. Voth, P. Salvador, J. J. Dannenberg, S. Dapprich, A. D. Daniels, Ö. Farkas, J. B. Foresman, J. V. Ortiz, J. Cioslowski and D. J. Fox, Gaussian. Inc, Wallingford, 09 edn., 2009.
- The UniProt Consortium, Nucleic Acids Res., 2014, 42, D191–D198 CrossRef PubMed.
- G. Madhavi Sastry, M. Adzhigirey, T. Day, R. Annabhimoju and W. Sherman, J. Comput.–Aided Mol. Des., 2013, 27, 221–234 CrossRef CAS PubMed.
- H. Li, A. D. Robertson and J. H. Jensen, Proteins, 2005, 61, 704–721 CrossRef CAS PubMed.
- R. A. Friesner, J. L. Banks, R. B. Murphy, T. A. Halgren, J. J. Klicic, D. T. Mainz, M. P. Repasky, E. H. Knoll, M. Shelley, J. K. Perry, D. E. Shaw, P. Francis and P. S. Shenkin, J. Med. Chem., 2004, 47, 1739–1749 CrossRef CAS PubMed.
- S. Kawatkar, H. Wang, R. Czerminski and D. Joseph-McCarthy, J. Comput.–Aided Mol. Des., 2009, 23, 527–539 CrossRef CAS PubMed.
- M. P. Repasky, R. B. Murphy, J. L. Banks, J. R. Greenwood, I. Tubert-Brohman, S. Bhat and R. A. Friesner, J. Comput.–Aided Mol. Des., 2012, 26, 787–799 CrossRef CAS PubMed.
- M. Baroni, G. Cruciani, S. Sciabola, F. Perruccio and J. S. Mason, J. Chem. Inf. Model., 2007, 47, 279–294 CrossRef CAS PubMed.
- Molecular Operating Environment (MOE) (2013.08) Chemical Computing Group Inc., 1010 Sherbooke St. West, Suite #910, Montreal, QC, Canada, H3A 2R7, 2013.
- C. Liao and M. C. Nicklaus, ChemMedChem, 2010, 5, 1053–1066 CrossRef CAS PubMed.
- B. C. Johnson, M. Metifiot, A. Ferris, Y. Pommier and S. H. Hughes, J. Mol. Biol., 2013, 425, 2133–2146 CrossRef CAS PubMed.
- P. Vasanthanathan, J. Hritz, O. Taboureau, L. Olsen, F. S. Jorgensen, N. P. Vermeulen and C. Oostenbrink, J. Chem. Inf. Model., 2009, 49, 43–52 CrossRef CAS PubMed.
- R. Costi, M. Metifiot, S. Chung, G. Cuzzucoli Crucitti, K. Maddali, L. Pescatori, A. Messore, V. N. Madia, G. Pupo, L. Scipione, S. Tortorella, F. S. Di Leva, S. Cosconati, L. Marinelli, E. Novellino, S. F. Le Grice, A. Corona, Y. Pommier, C. Marchand and R. Di Santo, J. Med. Chem., 2014, 57, 3223–3234 CrossRef CAS PubMed.
Footnote |
† Electronic supplementary information (ESI) available. See DOI: 10.1039/c4ra05728g |
|
This journal is © The Royal Society of Chemistry 2014 |