DOI:
10.1039/C4MB00463A
(Paper)
Mol. BioSyst., 2015,
11, 297-306
Acclimatisation-induced stress influenced host metabolic and gut microbial composition change†
Received
5th August 2014
, Accepted 23rd October 2014
First published on 23rd October 2014
Abstract
Understanding the basal gut bacterial community structure and the host metabolic composition is pivotal for the interpretation of laboratory treatments designed to answer questions pertinent to host–microbe interactions. In this study, we report for the first time the underlying gut microbiota and systemic metabolic composition in BALB/c mice during the acclimatisation period. Our results showed that stress levels were reduced in the first three days of the study when the animals were subjected to repetitive handling daily but the stress levels were increased when handling was carried out at lower frequencies (weekly). We also observed a strong influence of stress on the host metabolism and commensal compositional variability. In addition, temporal biological compartmental variations in the responses were observed. Based on these results, we suggest that consistency in the frequency and duration of laboratory handling is crucial in murine models to minimise the impact of stress levels on the commensal and host metabolism dynamics. Furthermore, caution is advised in consideration of the temporal delay effect when integrating metagenomics and metabonomics data across different biological matrices (i.e. faeces and urine).
Introduction
Animal models form an essential part of biomedical research in the 21st century1,2 particularly with the advent of various omics platforms that generate large complex biological datasets.3–5 Increasingly, multiple omics platforms in a single animal model were employed to generate a comprehensive system overview of a physiological state or disease pathophysiology.6–9 A combination of metabonomics and metagenomics investigations has been used to shed light on the host–microbiome interactions and responses to metabolic diseases,10 parasitic infections7 and therapeutic interventions.9,11 Such a system biology approach has contributed greatly to the understanding of the link between the host microbiome inhabiting the gut and host physiological conditions.9–15
The accuracy of host–microbe modelling is highly dependent on the complex microbiota composition and the host basal metabolic phenotype prior to an intervention.16–19 Factors contributing to the heterogeneity of the gut microbiome and metabolic profiles of experimental animals include various physiological factors such as diurnal variation, batch variation, age, gender, and species5,18,20–22 as well as external environmental factors such as food and water intake, bedding and temperature. Amongst them, the latter has been closely correlated with the stress levels of the animals. Previous studies have shown that both invasive (e.g. bleeding, oragastric gavage, etc.) and non-invasive (e.g. cage changing, cleaning and animal lifting, etc.) laboratory routines are likely to exert stress on the animals. The stressed animals usually exhibit abnormal physiological and immunological responses which compromise the reliability of the subsequent experimental observation.23
Thus, in order to normalise the basal physiological and gut microbial compositional difference in the experimental animals, the standardisation of feed, incubation conditions, and animal selection (e.g. weight, age, gender etc.) is normally considered in the development of animal models. In addition, experimental animals usually undergo a period of acclimatisation before treatment to allow the stabilisation of host physiology and the gut ecosystem. Interestingly, however, the period of acclimatisation is usually decided arbitrarily (Table 1). It is also still unclear whether both host metabolism and gut microbial dynamics respond at the same rate during acclimatisation.
Table 1 Period of acclimatisation reported in selected metagenomics or/and metabonomics studies
Study |
Period of acclimatisation |
Type of animals |
Ref. |
Dietary effects on tissue inflammation, hypercholesterolaemia and gut microbiota |
1 week |
Male Balb/c mice |
59
|
Ability of gut microbes to adapt to dietary perturbations |
Not stated |
Male germ-free C57BL/6J mice |
60
|
Immunological alteration and changes of gut microbiota to dextran sulfate sodium administration |
Not stated |
Wild-type female C57BL/6 mice |
61
|
Metabolic profiling on batch and age-related changes |
Not stated |
Female NMRI mice |
27
|
Metabolic phenotyping in the experimental laparotomy model of surgical trauma |
1 week |
Male Wistar rats |
62
|
Metabonomics and microbiological studies of vancomycin-induced changes |
1 week |
Female NMRI mice |
11
|
Metabonomics study of ethionine-induced metabolic changes |
10 days |
Male HanWistar rats |
63
|
Although various studies have been conducted to understand the effect of stress on animals, little attention has been paid on the stress levels exerted and their effect on the animals during acclimatisation prior to experimental treatments. Furthermore, current measures of stress are mainly focused on the behavioural or selected physiological responses of the animal, such as blood pressure, heart beat and corticosterone levels.24–26 Only a few studies have examined the relationship between stress and overall metabolic profiles5,27 or the gut microbiota status.28,29 In addition, an integrated system biology approach utilising both metagenomics and metabolomics platforms has not been widely used to study the basal composition and stress responses of the gut microbiota and the metabolic status of experimental animals.30
Here, we assess the basal metabolic and metagenomics variation and their responses in relation to stress during acclimatisation in the mouse-model for the first time using the nuclear magnetic resonance spectroscopy (NMR)-based metabonomics approach coupled with terminal restriction fragment length polymorphism (TRFLP) analyses. The data obtained from both platforms were statistically co-analysed to allow for system level information recovery. Understanding the boundary of ‘normality’ within the host is paramount to ensure the correct extraction of biological information from the experimental animals. Additionally, the pattern of change in experimental animals during acclimatisation will be essential to aid future experimental design in animal studies and delineating non-study specific biological variations.
Methods
Animals and sample collection
All animals used in this study were BALB/c female mice (5 weeks of age) purchased from the Institute for Medical Research (Kuala Lumpur, Malaysia). Animal experiments were carried out at the International Medical University (Kuala Lumpur, Malaysia), according to the guidelines and requirements of local and national authorities. Briefly, the animals were maintained under controlled environmental conditions (temperature: 26 °C, relative humidity: 60–70%, light/dark cycle: 12/12 hours) with free access to water and standard rodent chow. In this study, urine and faecal samples were collected on day 1, day 2, day 3, day 8 and day 15. Faecal samples were collected for corticosterone assay and TRFLP analyses. Urine samples were collected for metabolic profiling. Faecal and urine sample collection took place between 0800 and 1100 hours to minimise diurnal variation in concentrations of biofluids.18 At least 30 µL of urine and 6 faecal pellets were collected in Petri dishes without cross-contamination by gently rubbing the abdomens of the mice. Samples were transferred into 1.5 mL microcentrifuge tubes, immediately frozen and stored at −80 °C.
Corticosterone enzyme immunoassay of the faecal material
Faecal corticosterone measurement was used as a non-invasive technique to monitor stress levels in the laboratory animals. Corticosterone is a hormone secreted by the adrenal cortex when an animal is stressed and has been shown to be an accurate indicator of the animal status.31 This technique has been proven to be a reliable and accurate method for assessing physiological stress in experimental animals.32,33 The faecal material obtained from mice was measured for the levels of corticosterone using the Corticosterone Enzyme Immunoassay kit (Enzo Life Sciences, USA) with minor modifications to the sample preparation procedure. Prior to use, the faecal samples were dried overnight in an oven at 37 °C and powdered through mashing. The amount of the starting material was weighed and 500 µL of Assay Buffer 15 from the kit was added to each sample. The samples were vortex-mixed for 15 s and left to stand for 5 min at room temperature. The samples were then centrifuged at 7000 rpm for 3 min. 100 µL of the supernatant was used for the assay according to manufacturer's instructions. The level of corticosterone in each sample was calculated based on the standard curve obtained with reference to the weight used for each sample.
Urine sample preparation and 1H NMR spectroscopic analysis
Urine samples were prepared by mixing 20 µL of urine with 30 µL of a phosphate buffer containing 90% D2O and 0.25 mM 3-trimethylsilyl-1-[2,2,3,3-2H4] propionate (TSP) and left to stand for 10 min. The resulting mixtures were then transferred to 5 mm NMR microbore tubes with a 1.7 mm stem (Norell, USA).
A standard 1-dimensional (1-D) 1H NMR spectrum was acquired for each sample with a pulse (recycle delay (RD)–90°–t1–90°–tm–90°–acquire free induction decay (FID)) on a Bruker AVIII 600 MHz spectrometer (Bruker Biospin, Fallenden, Switzerland) with a 5 mm BB(F)O broadband probe operating at 600.13 MHz (ambient probe temperature 27 °C). Samples were automatically delivered to the spectrometer by a Bruker SampleCase™. The field frequency was locked on the D2O solvent. The water peak was suppressed by gradient water presaturation during the RD of 4 s and a mixing time (tm) of 0.01 s. The 90° pulse length was adjusted to ∼10 µs and an acquisition time of 2.65 s was used. In total, 32 transients were collected into 64 K data points using a spectral width of 20 ppm. An exponential line broadening function of 0.3 Hz was applied to the free induction decay prior to Fourier transformation.
Faecal DNA extraction and TRFLP analyses
DNA was extracted using the QiAmp DNA stool mini kit (Qiagen, USA) according to the manufacturer protocol. The TRFLP was carried out as described in Chong et al.34 with minor modifications. Briefly, PCR was conducted using universal primers (27F and 1492R) targeting the bacterial 16S rRNA gene region. Both primers were tagged with the fluorescence dye phosphoramidite fluorochrome 5-carboxyfluorescein (FAM) and 6-carboxy-hexachlorofluorescein (HEX) at 5, respectively. The amplicons were digested using a 4-base cutter MSP-1 before being subjected to electrophoretic separation using ABI 3100 and ABI 3730XL genetic analysers (Applied Biosystems, USA). ROX labeled GeneScan 500 control was used as the size standard. The resulting fragment profiles were scored, aligned and noise filtered using the web-based programme T-REX.35 The noise filtered procedure was carried out by removing peaks which were lower than the overall standard deviation. In addition, alignment was carried out by binning the peaks into TRFs with the clustering threshold of 0.5 bp, starting from the smallest peak among all the profiles.36
Data processing and analysis
For metabonomics analysis, 1H NMR spectra of urine samples were manually phased and baseline-corrected using Bruker TopSpin (version 3.1, Bruker Biospin, Fallenden, Switzerland). All spectra were referenced to the TSP resonance at δ 0.00. The complete spectra (δ 0.0–10.0) were digitised into 7K data points using an in-house developed MATLAB script (O. Cloarec, Imperial College London). The region containing the water resonance (δ 4.5–6.5) was removed from each spectrum to eliminate baseline effects of imperfect water suppression. In addition, the regions δ 0.0–0.5 and δ 9.2–10.0 containing only noise were removed. For each spectrum, normalisation to the total sum of the residual spectrum was carried out prior to pattern recognition analyses followed by the scaling of the data to unit variance. Both unsupervised (principal component analysis, PCA) and supervised multivariate data analysis methods (projection to latent structure-discriminant analysis, PLS-DA and orthogonal partial least squares discriminant analysis, O-PLS-DA37,38) were employed to visualise and interpret experimental differences. The statistical significance and validity of subsequent results were calculated using a permutation test (the number of permutations = 1000).39 The covariance plot was used to aid the interpretation of the significance of each metabolite from the permutation tests. The colours projected onto the spectrum indicate the significance of the metabolites with blue indicating no significant difference at p > 0.05 confidence levels and red indicating highly significant difference at p < 0.05. In this study, only metabolites with p < 0.01 will be considered significant.
The TRFLP data obtained from T-REX were exported to PERMANOVA + add-on of the PRIMER6 multivariate data analysis package (Plymouth Marine Laboratory, UK) for analyses. Alpha-diversity indices including the Shannon diversity index (H′) and Pielou's measure of species evenness (J′) were calculated using the DIVERSE option. For multivariate-analyses, the data were pre-treated with the unit variance normalisation procedure before generating a Euclidean distance matrix which was used to perform the permutational multivariate analysis of variance (PERMANOVA) and PCA. The PERMANOVA was carried out to test for gut microbial compositional difference between the groupings while the PCA was used to visualise the distribution of the samples across the sampling points. The significance of the PERMANOVA was calculated based on 999 permutations and corrected using Monte Carlo correction.
To test for the agreement between urine metabolite profiles and gut microbial composition, the two distance matrices were correlated using the Spearman rank correlation under 999 permutations. Two separate distance based linear modellings (DISTLM) were carried out to regress the urine metabolites on the bacterial assemblage pattern and with the opposite order (i.e. regress the T-RFLP peaks on the metabolites data cloud). The parsimonious model was constructed using stepwise selection under the second-order bias-corrected Akaike information criterion (AICc).40 Specifically, the stepwise selection procedure allows both the forward inclusion and backward elimination of the variables while the AICc was used to account for the large number of predictor variables (q) in relatively small number of samples (N).
Results
Faecal corticosterone measurements
The faecal corticosterone level of samples collected on 5 different days within a 15 day period showed a decreasing trend from day 1 to day 3 (decreasing from the highest level to the lowest level), after which, the level of corticosterone increased from day 3 onwards to day 15 (Table 2). The level of corticosterone in samples collected from days 8 and 15 was not significantly different from that in the samples collected from days 1 and 2. The statistics indicated that the level of corticosterone was only significantly lower on day 3 as compared to day 1.
Table 2 Corticosterone levels and bacterial diversity (mean ± SE) in the faecal samples. Different letters in the superscript denote a significant difference at P < 0.05
Days |
Corticosterone level (log pg mL−1) |
Total TRFLP peaks |
Shannon diversity index (H′) |
Diversity evenness (J′) |
1 |
6.87A ± 0.12 |
309.3B ± 13.1 |
4.69CD ± 0.09 |
0.82CD ± 0.013 |
2 |
6.80AB ± 0.09 |
178.1C ± 33.3 |
4.30D ± 0.19 |
0.87D ± 0.010 |
3 |
6.53B ± 0.05 |
530.9A ± 14.3 |
5.73A ± 0.03 |
0.91A ± 0.005 |
8 |
6.70AB ± 0.03 |
283.4B ± 25.0 |
4.95BC ± 0.17 |
0.88BC ± 0.017 |
15 |
6.75AB ± 0.05 |
337.4B ± 13.1 |
5.34AB ± 0.08 |
0.92AB ± 0.014 |
Effects of acclimatisation and the associated stress on 16S rRNA gene-based gut microbiota profiles
An opposite trend was observed for the bacterial diversity in the faecal samples. The lowest bacterial diversity was observed in the faecal samples collected on day 1 and day 2, while the highest bacterial diversity was found on day 3, corresponding to the lowest level of the stress biomarker (corticosterone). Subsequently, a reduction in diversity was detected from the samples collected on day 8, followed by a slight recovery in diversity on day 15.
The PERMANOVA of the TRFLP data suggested a significant difference in bacterial profiles at P = 0.001 (Pseudo-F = 5.82). A follow-up PCA was conducted to examine the distribution of the data. Fig. 1A showed a clear distinction between samples collected from day 3 as compared to those collected from other time points indicating that the day 3 samples showed the most distinct bacterial TRFLP profiles. It is interesting to note that the distinction is consistent with the sample having the lowest mean corticosterone level among the total collected samples (Table 2). A PCA mean trajectory plot was drawn to detect the presence of finer separation within the sample cluster other than replicates from day 3 (Fig. 1B). From the trajectory plot, the biggest faecal bacteria compositional change occurred from day 2 to day 3 along the first principal component (PC1) followed by a movement along PC1 of almost the same magnitude to day 8 in the opposite direction before shifting along the second principal component (PC2) to day 15 indicating that there was a clear time-dependent faecal bacteria development profile.
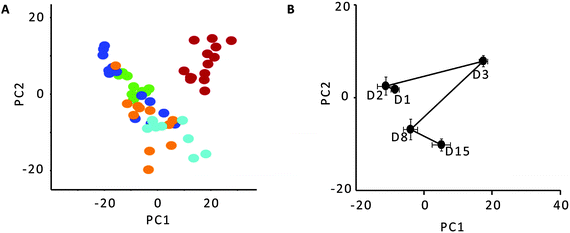 |
| Fig. 1 (A) PCA score plot (R2, 32.1%) derived from the faecal TRFLP of female BALB/c mice from day 1 (green), day 2 (blue), day 3 (red), day 8 (orange) and day 15 (turquoise). (B) Corresponding PCA mean trajectory plot derived from the same TRFLP profiles as (A). The error bar indicates the standard error. Key: D1, day 1; D2, day 2; D3, day 3; D8, day 8; D15, day 15. | |
Effects of acclimatisation and the associated stress on urinary metabolic profiles
Median 1H NMR spectra of urine obtained from all time points showed almost similar metabolic composition dominated by a number of metabolites such as short chain fatty acids (SCFA) i.e. 3-D-hydroxybutyrate and α-hydroxy N-valerate, tricarboxylic acid cycle intermediates i.e. pyruvate and citrate, methylamines i.e. trimethylamine and trimethylamine N-oxide, creatine, creatinine, taurine, phenylacetylglycine and hippurate (Fig. S1, ESI†). The visual inspection of the NMR spectra showed subtle differences in overall metabolic composition among the time points with the exception of urinary NMR spectra obtained on day 8, where relatively low concentrations of urinary hippurate and citrate, and higher concentrations of urinary phenylacetylglycine and taurine were observed.
The urinary metabolic perturbations during acclimatisation were compared using PERMANOVA under Monte Carlo correction (999 permutations). Based on the results, the urine profiles were significantly different at P = 0.001 (Pseudo-F = 4.46). The distribution of the data was further visualised using a principal component analysis based on the entire NMR data set. A total of 5 PCs were calculated for a PCA model using unit variance-scaled spectral data shown in Fig. 2A. In contrast to the gut microbiota profiles where the sample with the lowest stress level (day 3) shows apparent separation from other time points, a clear separation can be observed between urine samples obtained on day 8 as compared to all the other time points along PC1, whereas urine samples collected on day 1 were partially separated from the main cluster of samples along PC2. PCA trajectory analysis was used to give an overview of the metabolic alterations during acclimatisation (Fig. 2B). Three clear clusters can be observed in the urinary metabolic space, separating samples collected on day 1, day 8 and the remaining three time points (day 2, day 3 and day 15). The metabolic trajectory shifted from day 1 to day 2 along the PC2 followed by a slight shift along PC1 from day 2 to day 3. The biggest shift in the metabolic trajectory occurred on day 8 along PC1 before returning to day 2 and day 3 metabolic spaces by day 15 along PC1.
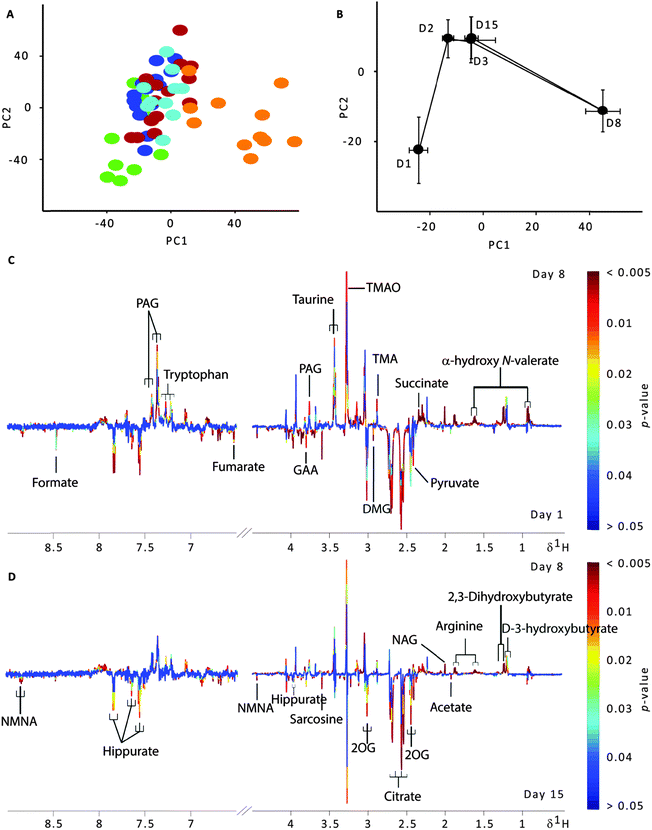 |
| Fig. 2 (A) PCA scores plot (R2, 36.1%) derived from 1H NMR urinary spectra of female BALB/c mice from day 1 (green), day 2 (blue), day 3 (red), day 7 (orange) and day 14 (turquoise). (B) Corresponding PCA mean trajectory plot derived from the same 1H NMR urinary spectra as (A). The error bar indicates the standard error. The covariance plot showing the colour-coded significance of urinary metabolite profiles calculated using permutation test between (C) day 1 and day 8, and (D) day 8 and day 15. Key: D1, day 1; D2, day 2; D3, day 3; D8, day 8; D15, day 15. | |
In order to identify metabolites that are significantly different between each time point pairs, pair-wise O-PLS-DA models were constructed for each comparison of two time points based on two PLS and one orthogonal component by unit variance scaling. In addition, permutation testing was carried out on each pair-wise model to identify metabolites that are significantly different. The total explained variation in the NMR data was indicated by the R2 value and the corresponding parameter indicating the predictive performance of the model (Q2) reflected a high predictive ability (>60% for all pairs except two (day 2 vs. day 3 and day 2 vs. day 15) Table S1, ESI†). All pair-wise models involving urine collected from mice on day 8 exhibited high Q2 values, which is in agreement with the PCA score plot (Fig. 2A) showing that samples from day 8 clearly separated from the other time points. In these models, urine collected from mice on day 8 showed elevated 2,3-dihydroxybutyrate, α-hydroxy N-valerate, D-3-hydroxybutyrate, phenylacetylglycine, succinate, taurine, trimethylamine, trimethylamine N-oxide, arginine and N-acetyls of glycoproteins, together with decreased levels of 2-oxoglutarate, acetate, citrate, dimethylglycine, formate, fumarate, guanidinoacetate, hippurate, N-methyl nicotinate, pyruvate and sarcosine (Table S1, ESI†).
In contrast, changes in urinary metabolites were less pronounced when urine NMR spectra collected from the remaining time points were compared against each other. Elevated urinary creatine, dimethylglycine, guanidinoacetate and phenylacetylglycine, and decreased urinary trimethylamine N-oxide were observed when comparing urine collected on day 1 against urine collected on day 2 and day 3, respectively (Table S1, ESI†). Urine collected on day 1 also showed relatively high levels of 2-oxoglutarate, citrate and pyruvate, and lower levels of taurine, trimethylamine, trimethylamine N-oxide and tryptophan as compared to urine collected on day 15. Urine collected on day 2 and day 3 showed, by far, the least difference when compared with only one significantly different metabolite, 2-oxoglutarate. Similarly, when comparing urine collected on day 3 against urine collected on day 15, only one significantly different metabolite was identified, creatine. The results from O-PLS-DA and permutation tests on the latter were reflective of the scores and mean trajectory plots (Fig. 2A and B), which showed no clear discrimination between these three time points.
Correlation and linear modelling of the urine metabolic profiles and gut microbiota
Due to the difference in the assemblage pattern, no significant correlation was found between the unit variance normalised Euclidean Distance matrices of both urine metabolites and gut microbial profiles (Spearman ρ = −0.04, P = 0.69). We further modelled the relationship separately using urine profiles as the predictor for the gut microbiota distance matrix, followed by matching the distribution of the gut microbiota using the urine metabolite profiles as the predictor. According to the AIC selection criterion (with second order bias correction), 9 TRFLP peaks (Table 3A) were chosen to model the changes of the urine metabolite pattern over the 5 time points (cumulative R2 = 41.11%). We attempted to relate the putative identity of the TRFLP peaks by comparing to the TRF lengths generated via in silico digestion, however the virtual digestion of the 16S rRNA gene database from the ribosomal database project (>1200 bp) did not yield matchable identity (data not shown). Conversely, 6 1H-NMR peaks were selected using the same criterion to model the variation in gut microbial composition over the 15 day acclimatisation experiment (Table 3B). They included dimethylamine (2.77 ppm), fumarate (6.55 ppm), hippurate (3.99 ppm), α-hydroxy N-valerate (0.93 ppm), malonate (3.14 ppm, tentative assignment) and unknown 1 (6.99 ppm). Together, a combination of the 6 variables explained 29.1% of the total variance in the model.
Table 3 Cross-modelling of the urine metabolite and T-RFLP profiles using a stepwise selection procedure
Variablea |
AICc |
SS (trace) |
Pseudo-F |
P
|
Prop. |
Cumul. |
Res.df |
Note: Prop. = explanatory proportion, Cumul. = cumulative explanatory proportion, res.df = residual degree of freedom. +, inclusion; −, exclusion. |
(A) Modelling of the urine metabolite pattern using gut microbial compositions as the predictor |
+59G |
315.35 |
1189.1 |
5.3738 |
0.001 |
8.76 × 10−02 |
8.76 × 10−02 |
56 |
+252G |
314.55 |
630.09 |
2.9464 |
0.003 |
4.64 × 10−02 |
0.13395 |
55 |
+350B |
313.72 |
619.2 |
3.0008 |
0.005 |
4.56 × 10−02 |
0.17955 |
54 |
+217B |
312.64 |
649.46 |
3.2804 |
0.002 |
4.78 × 10−02 |
0.22737 |
53 |
+310G |
311.61 |
618.03 |
3.2544 |
0.002 |
4.55 × 10−02 |
0.27287 |
52 |
+61B |
310.91 |
544.78 |
2.9778 |
0.006 |
4.01 × 10−02 |
0.31299 |
51 |
+372B |
310.66 |
462.65 |
2.6086 |
0.017 |
3.41 × 10−02 |
0.34705 |
50 |
+431G |
310.55 |
435.7 |
2.5319 |
0.071 |
3.21 × 10−02 |
0.37913 |
49 |
+424B |
310.42 |
434.17 |
2.6057 |
0.047 |
3.20 × 10−02 |
0.4111 |
48 |
|
(B) Modelling of gut microbial composition using urine metabolite profiles as the predictor |
+3.982 |
383.58 |
2851 |
3.9732 |
0.001 |
6.62 × 10−02 |
6.62 × 10−02 |
56 |
+2.765 |
381.88 |
2630.6 |
3.8527 |
0.001 |
6.11 × 10−02 |
0.12738 |
55 |
+6.546 |
381.5 |
1704.6 |
2.5677 |
0.001 |
3.96 × 10−02 |
0.16699 |
54 |
+3.142 |
381.16 |
1651.7 |
2.5598 |
0.013 |
3.84 × 10−02 |
0.20536 |
53 |
+3.991 |
380.93 |
1571.1 |
2.504 |
0.002 |
3.65 × 10−02 |
0.24187 |
52 |
−3.982 |
380.15 |
977.69 |
1.5583 |
0.027 |
2.27 × 10−02 |
0.21915 |
53 |
+0.934 |
379.75 |
1631.3 |
2.6532 |
0.001 |
3.79 × 10−02 |
0.25706 |
52 |
+6.993 |
379.63 |
1464.3 |
2.4479 |
0.001 |
3.40 × 10−02 |
0.29109 |
51 |
Temporal effect of urine metabolites and gut microbiota composition
Despite the overall dissimilarity between the distribution pattern of PCAs generated using the urine (Fig. 1) and stool profiles (Fig. 2), a similar trend was observed between the urine metabolite profile obtained from days 2, 3, 8 and 15 and the gut microbiota composition derived from days 1, 2, 3 and 8. We speculated that such a pattern might be indicative of a delayed response of the urine metabolites in comparison to the gut microbial composition. To support our postulation, we repeated the correlation analysis by matching the sample labelling of the urine and stool samples, accounting for one time point response delay in the urine metabolite profile (Fig. S2, ESI†). The results showed a significant correlation at Spearman ρ = 0.209 (P = 0.015). However, DISTLM modelling using the relabelled data did not show a significant improvement in the overall predictive power. R2 of 0.4187 was obtained when the urine profile was modelled using the relabelled gut microbial composition pattern. On the other hand, R2 of 0.2761 was obtained when the relabelled gut microbial distribution was modelled using the urine metabolite composition. Nonetheless, the association of 2,3-dihydroxybutyrate (microbial associated metabolites, 1.223 ppm) to the gut microbial pattern was apparent in the relabelled data, accounting for nearly 14% of the overall variance in the model.
Discussion
By integrating both metagenomics and metabonomics approaches, we were able to show that the basal host metabolic and gut microbiological profiles were significantly affected during acclimatisation. The diversity and compositional changes of the host gut microbe were shown to relate to host stress levels, potentially due to the routine faecal and urine collection procedure; and that the gut microbe composition and host metabolic responses to the acclimatisation-induced stress may be operating on a different time scale. We postulated a potential delayed effect in the latter in comparison to the former. Overall, the stress level, gut microbiota composition and urine metabolite profiles were reasonably stable throughout the duration of the entire study (15 days).
Host responses to laboratory procedure-induced stress
All animals, including humans, undergo an adaptation process known as ‘acclimatisation’ when they are introduced or placed in a new environment.41 Such a process takes time to normalise and usually invokes stress, which in turn induces metabolic changes in the hosts.5 Indeed, studies have shown that experimental animals do habituate to frequencies of handling, which may introduce bias to host sleep homeostasis and stress levels.42,43 As such, sufficient acclimatisation period should be factored into any animal study design to allow for the stabilisation of host metabolic and gut microbe composition to enable the useful extraction of biological information and inference. Our current study showed that the faecal corticosterone level was significantly reduced by day 3 of acclimatisation (Table 2), and subsequently increased by day 8 and day 15. This is further supported by our study sampling regime when sample collection was carried out on days 1, 2, 3, 8 and 15, with the highest frequency of handling in the first three days followed by a longer sampling time interval on days 8 and 15. The changes in corticosterone levels indicated that experimental animals do habituate to repeated handling as observed by the significantly lower levels of faecal corticosterone by day 3, and the subsequent increase in faecal corticosterone levels coincided with the longer sampling time interval on days 8 and 15. Our finding is in line with that of Leussis and Bolivar41 who suggested that habituation is common among rodents and that the level of habituation is strongly related to the duration (i.e. intra session habituation) and frequency (i.e. inter session habituation) of handling. Initially, we speculated that the animals were habituated to the frequency of handling by the third day, but the memory to the handling routine dissipated over time as seen in the elevated corticosterone level in day 8 and day 15 samples. Thus, the interval between handling should be consistent in order to minimise fluctuations in the stress level across the study period.
Our study also showed that animal stress levels may have a direct influence on the host gut microbe diversity (Table 2) as well as composition (Fig. 1A). This was substantiated with the faecal samples collected on day 3 showing the highest bacterial diversity and significantly different gut microbe compositions, both of which coincided with the lowest corticosterone levels. Studies pertinent to the effect of stress on gut microbiota composition dates back to the 70s, when Tannock and Savage44 showed that stressed mice harboured strikingly reduced lactobacilli in their gut. In addition, studies have shown that stress factors such as pup–mother separation, confinement (water avoidance stress) and social disruption affect the diversity of gut microbiota in experimental animal models.28,45,46 Our result provided additional support that the stress induced by non-invasive laboratory routines during acclimatisation can also impact the gut bacterial composition.
Surprisingly, however, the urinary metabolic changes on day 3 were unremarkable. Overall metabolite profiles of day 3 were similar to other samples (Fig. 2) except for a few metabolites that are known to be metabolised both by the host and the commensal flora (microbial–mammalian co-metabolites) such as hippurate that was elevated and trimethylamine N-oxide and 3-D-hydroxybutyrate which were reduced in comparison to the metabolite profiles of day 8 (Table S1, ESI†). It is interesting to note that from the urinary mean trajectory plot (Fig. 2B), although the metabolic composition changes over time, it returned to initially stable levels similar to that of day 2 and day 3 by day 15 as evidenced by the relatively low number of metabolites perturbed (Table S1, ESI†). However, the opposite was observed in the faecal microbe composition where a progressive change in the direction of the compositional trajectory occurred throughout the entire 5 time points indicating that compositional changes occur through time. This observation highlighted one of the commonly overlooked challenges when conducting metagenomics studies wherein the “stability” of the faecal microbe composition in experimental animals is difficult to attain even after fifteen days of study.
Temporal intercompartmental biological variation
In contrast to the corticosterone level as well as the gut microbe compositional variations when samples collected on day 3 showed most significant differences (Fig. 1 and 2), urine samples collected on day 8 showed the biggest metabolic variation as compared to other time points. The discrepancy could be explained by the presence of temporal inter-compartmental biological variation whereby the changes in the gut microbe composition would not be reflected immediately in the urine samples on the same day. Indeed, a significant correlation was obtained when the gut microbiota composition was compared with the relabelled (to account for 1 time point delay) urine metabolite profiles. Furthermore, the reduction in the rodent stress related marker such as hippurate47 in urine of day 8 coincided with the lower stress and elevated bacterial diversity in stool collected on day 3. These findings are in agreement with previous studies, which showed that a high degree of temporal variation exists between faecal and urine samples.27,48 It should also be emphasised that host urinary metabolic composition is a manifestation of the interplay between the host homeostasis and gut microbial interactions with the environment. Hence, urinary metabolic composition is more regulated by the host as compared to faecal microbial composition which interacts directly with the diet and the environment. Our results also indicate the need for extra caution when correlating host–gut microbiome systemic data as the delay in the manifestation of urinary metabolic effect on the gut microbiota could lead to the misinterpretation of the link between host metabolism and the functional role of gut microbiota.
The separation of the urine metabolite profiles collected on day 8 from other time points was mainly due to the relative increase in microbial–mammalian co-metabolites such as trimethylamine, trimethylamine N-oxide and phenylacetylglycine, and a concomitant decrease in tricarboxylic acid cycle intermediates such as 2-oxoglutarate, citrate and pyruvate (Table S1, ESI†). However, the opposite was observed in the level of hippurate, a known microbial–mammalian co-metabolite.11,49,50 Hippurate is formed by the glycine conjugation of benzoate in the liver, which is produced from the bacterial metabolism of plant phenols51 or quinic acid.52 In addition, the gut microbiota is also involved in the catabolism of aromatic amino acids including tyrosin and tryptophan.53 Significant changes in urinary microbial–mammalian co-metabolites observed on day 8 in the current work indicated a change in the bacterial metabolism of phenolics in the animals. A variation in the composition of bacteria in the host could also explain the directionality of change in certain microbial–mammalian co-metabolites such as hippurate and phenylacetylglycine. The evidence of systemic gut microbe modulations was also found in the cross-modelling analyses (Table 3). A series of host bacteria co-metabolites accounted for the highest explanatory values to the gut microbe compositional change, such as dimethylamine (2.77 ppm), fumarate (6.55 ppm), hippurate (3.99 ppm), and α-hydroxy N-valerate (0.93 ppm). The same was observed in the re-labelled table after accounting for temporal delay between the two biological compartments with 2,3-dihydroxybutyrate (1.223 ppm) explaining 50% of the total R2 value (∼27%).
Further indicators of gut microbe perturbation were observed with changes in the metabolites of choline metabolism, methylamines, such as trimethylamine, trimethylamine N-oxide and dimethylglycine.54,55 Choline metabolism involves both mammalian and sym-xenobiotic metabolic pathways that are regulated through complex host–symbiont interaction.56 Methylamines are also known to be co-metabolised by the host and the gut microbiota in the large intestine.54 Changes in choline metabolites were only observed from day 8 onwards possibly indicating that perturbation in gut microbes involved in choline metabolism occurs on day 8. In addition, significant increases in the level of urinary SCFAs such as 3-D-hydroxybutyrate and α-hydroxy N-valerate were also observed from day 8 onwards. SCFAs and lactic acid were products of the bacterial fermentation of carbohydrates in the cecum and colon.57,58 Our observations are consistent with a previous animal study by Li et al.,27 which showed increases in SCFAs during later time points of acclimatisation.
Conclusion
Animal handlings such as lifting, cage changing and biological sample collections are common in animal studies. In this work, we showed that routine laboratory procedures during acclimatisation may affect the stress levels of mice resulting in modifications of the metabolic and gut microbiota composition. We would like to suggest that stress biomarkers such as corticosteroid levels should be monitored when conducting an animal study in order to track animal stress levels throughout the study.32 Additionally, animal handling intervals should be consistent especially in the murine model to minimise variations in stress levels, which could further complicate the extraction and interpretation of biological information.
Abbreviation
FID Free induction decay
NMR Nuclear magnetic resonance
TRFLP Terminal restriction fragment length polymorphism
TSP Sodium 3-(trimethylsilyl) propionate-2,2,3,3-d4
Acknowledgements
We thank the editor and two anonymous reviewers for the helpful comments in improvement of the manuscript. We are grateful to the International Medical University for the support and facilities of this work. This research is supported by the Ministry of Science, Technology and Innovation, Science Fund (02-02-09-SF0033). NMR signal processing and multivariate in-house software were developed by Dr O. Cloarec, Dr T. Ebbels, Dr Kirill A. Veselkov, Dr H. Keun and Dr M. Rantalainen (Biomolecular Medicine, Department of Surgery and Cancer, Imperial College London). The authors also gratefully acknowledge the NMR facilities and assistance provided by Atta-ur-Rahman Institute for Natural Products Discovery, Universiti Teknologi MARA (UiTM), Puncak Alam Campus, Selangor, Malaysia.
References
- N. Rosenthal and S. Brown, Nat. Cell Biol., 2007, 9, 993–999 CrossRef CAS PubMed
.
- S. H. Yang, P. H. Cheng, H. Banta, K. Piotrowska-Nitsche, J. J. Yang, E. C. Cheng, B. Snyder, K. Larkin, J. Liu, J. Orkin, Z. H. Fang, Y. Smith, J. Bachevalier, S. M. Zola, S. H. Li, X. J. Li and A. W. Chan, Nature, 2008, 453, 921–924 CrossRef CAS PubMed
.
- A. L. Goodman, G. Kallstrom, J. J. Faith, A. Reyes, A. Moore, G. Dantas and J. I. Gordon, Proc. Natl. Acad. Sci. U. S. A., 2011, 108, 6252–6257 CrossRef CAS PubMed
.
- J. Saric, J. V. Li, Y. Wang, J. Keiser, K. Veselkov, S. Dirnhofer, I. K. Yap, J. K. Nicholson, E. Holmes and J. Utzinger, J. Proteome Res., 2009, 8, 3899–3911 CrossRef CAS PubMed
.
- M. E. Bollard, E. G. Stanley, J. C. Lindon, J. K. Nicholson and E. Holmes, NMR Biomed., 2005, 18, 143–162 CrossRef CAS PubMed
.
- C. Ansong, A. C. Schrimpe-Rutledge, H. D. Mitchell, S. Chauhan, M. B. Jones, Y. M. Kim, K. McAteer, B. L. Deatherage Kaiser, J. L. Dubois, H. M. Brewer, B. C. Frank, J. E. McDermott, T. O. Metz, S. N. Peterson, R. D. Smith, V. L. Motin and J. N. Adkins, Mol. BioSyst., 2013, 9, 44–54 RSC
.
- B. L. Deatherage Kaiser, J. Li, J. A. Sanford, Y. M. Kim, S. R. Kronewitter, M. B. Jones, C. T. Peterson, S. N. Peterson, B. C. Frank, S. O. Purvine, J. N. Brown, T. O. Metz, R. D. Smith, F. Heffron and J. N. Adkins, PLoS One, 2013, 8, e67155 CAS
.
- S. Madhavan, Y. Gusev, T. G. Natarajan, L. Song, K. Bhuvaneshwar, R. Gauba, A. Pandey, B. R. Haddad, D. Goerlitz, A. K. Cheema, H. Juhl, B. Kallakury, J. L. Marshall, S. W. Byers and L. M. Weiner, Front. Genet., 2013, 4, 236 Search PubMed
.
- A. E. Perez-Cobas, M. J. Gosalbes, A. Friedrichs, H. Knecht, A. Artacho, K. Eismann, W. Otto, D. Rojo, R. Bargiela, M. von Bergen, S. C. Neulinger, C. Daumer, F. A. Heinsen, A. Latorre, C. Barbas, J. Seifert, V. M. dos Santos, S. J. Ott, M. Ferrer and A. Moya, Gut, 2013, 62, 1591–1601 CrossRef CAS PubMed
.
- J. V. Li, H. Ashrafian, M. Bueter, J. Kinross, C. Sands, C. W. le Roux, S. R. Bloom, A. Darzi, T. Athanasiou, J. R. Marchesi, J. K. Nicholson and E. Holmes, Gut, 2011, 60, 1214–1223 CrossRef CAS PubMed
.
- I. K. Yap, J. V. Li, J. Saric, F. P. Martin, H. Davies, Y. Wang, I. D. Wilson, J. K. Nicholson, J. Utzinger, J. R. Marchesi and E. Holmes, J. Proteome Res., 2008, 7, 3718–3728 CrossRef CAS PubMed
.
- P. J. Turnbaugh and J. I. Gordon, Cell, 2008, 134, 708–713 CrossRef CAS PubMed
.
- F. P. Martin, M. E. Dumas, Y. Wang, C. Legido-Quigley, I. K. Yap, H. Tang, S. Zirah, G. M. Murphy, O. Cloarec, J. C. Lindon, N. Sprenger, L. B. Fay, S. Kochhar, P. van Bladeren, E. Holmes and J. K. Nicholson, Mol. Syst. Biol., 2007, 3, 112 CrossRef PubMed
.
- F. P. Martin, N. Sprenger, I. K. Yap, Y. Wang, R. Bibiloni, F. Rochat, S. Rezzi, C. Cherbut, S. Kochhar, J. C. Lindon, E. Holmes and J. K. Nicholson, J. Proteome Res., 2009, 8, 2090–2105 CrossRef CAS PubMed
.
- J. Han, L. C. Antunes, B. B. Finlay and C. H. Borchers, Future Microbiol., 2010, 5, 153–161 CrossRef CAS PubMed
.
- J. G. Fox, Y. Feng, E. J. Theve, A. R. Raczynski, J. L. A. Fiala, A. L. Doernte, M. Williams, J. L. McFaline, J. M. Essigmann, D. B. Schauer, S. R. Tannenbaum, P. C. Dedon, S. A. Weinman, S. M. Lemon, R. C. Fry and A. B. Rogers, Gut, 2010, 59, 88–97 CrossRef CAS PubMed
.
- S. P. Claus, S. L. Ellero, B. Berger, L. Krause, A. Bruttin, J. Molina, A. Paris, E. J. Want, I. de Waziers, O. Cloarec, S. E. Richards, Y. Wang, M. E. Dumas, A. Ross, S. Rezzi, S. Kochhar, P. Van Bladeren, J. C. Lindon, E. Holmes and J. K. Nicholson, mBio, 2011, 2, e00271 CrossRef PubMed
.
- M. E. Bollard, E. Holmes, J. C. Lindon, S. C. Mitchell, D. Branstetter, W. Zhang and J. K. Nicholson, Anal. Biochem., 2001, 295, 194–202 CrossRef CAS PubMed
.
- M. C. Noverr and G. B. Huffnagle, Trends Microbiol., 2004, 12, 562–568 CrossRef CAS PubMed
.
- C. L. Gavaghan, I. D. Wilson and J. K. Nicholson, FEBS Lett., 2002, 530, 191–196 CrossRef CAS
.
- E. G. Stanley, N. J. Bailey, M. E. Bollard, J. N. Haselden, C. J. Waterfield, E. Holmes and J. K. Nicholson, Anal. Biochem., 2005, 343, 195–202 CrossRef CAS PubMed
.
- S. X. Wang and W. C. Wu, World J. Gastroenterol., 2005, 11, 2016–2021 Search PubMed
.
- T. Poole, Lab. Anim., 1997, 31, 116–124 CrossRef CAS
.
- J. P. Balcombe, N. D. Barnard and C. Sandusky, Contemp. Top. Lab. Anim. Sci., 2004, 43, 42–51 CAS
.
- G. Jean Kant, T. Eggleston, L. Landman-Roberts, C. C. Kenion, G. C. Driver and J. L. Meyerhoff, Pharmacol., Biochem. Behav., 1985, 22, 631–634 CrossRef
.
- K. Gouveia and J. L. Hurst, PLoS One, 2013, 8, e66401 CAS
.
- J. V. Li, J. Saric, I. K. Yap, J. Utzinger and E. Holmes, Mol. BioSyst., 2013, 9, 3155–3165 RSC
.
- S. M. O'Mahony, J. R. Marchesi, P. Scully, C. Codling, A. M. Ceolho, E. M. Quigley, J. F. Cryan and T. G. Dinan, Biol. Psychiatry, 2009, 65, 263–267 CrossRef PubMed
.
- N. Sudo, Y. Chida, Y. Aiba, J. Sonoda, N. Oyama, X.-N. Yu, C. Kubo and Y. Koga, J. Physiol., 2004, 558, 263–275 CrossRef CAS PubMed
.
- M. Matsumoto, R. Kibe, T. Ooga, Y. Aiba, S. Kurihara, E. Sawaki, Y. Koga and Y. Benno, Sci. Rep., 2012, 2, 233 Search PubMed
.
- E. Mostl and R. Palme, Domest. Anim. Endocrinol., 2002, 23, 67–74 CrossRef CAS
.
- C. Touma, R. Palme and N. Sachser, Horm. Behav., 2004, 45, 10–22 CrossRef CAS PubMed
.
- O. Kalliokoski, J. Hau, K. R. Jacobsen, C. Schumacher-Petersen and K. S. Abelson, Gen. Comp. Endocrinol., 2010, 168, 450–454 CrossRef CAS PubMed
.
- C. W. Chong, D. A. Pearce, P. Convey and I. K. P. Tan, Antarctic Science, 2012, 24, 249–258 CrossRef
.
- S. W. Culman, R. Bukowski, H. G. Gauch, H. Cadillo-Quiroz and D. H. Buckley, BMC Bioinf., 2009, 10, 171 CrossRef PubMed
.
- C. J. Smith, B. S. Danilowicz, A. K. Clear, F. J. Costello, B. Wilson and W. G. Meijer, FEMS Microbiol. Ecol., 2005, 54, 375–380 CrossRef CAS PubMed
.
- J. Trygg and S. Wold, J. Chemom., 2002, 16, 119–128 CrossRef CAS
.
- O. Cloarec, M. E. Dumas, J. Trygg, A. Craig, R. H. Barton, J. C. Lindon, J. K. Nicholson and E. Holmes, Anal. Chem., 2005, 77, 517–526 CrossRef CAS PubMed
.
-
R. A. S. Fisher, The Design of Experiments, Oliver & Boyd, Edinburgh, London, 1935 Search PubMed
.
- H. Yanagihara, K.-I. Kamo and T. Tonda, Can. J. Stat., 2011, 39, 126–146 CrossRef
.
- M. P. Leussis and V. J. Bolivar, Neurosci. Biobehav. Rev., 2006, 30, 1045–1064 CrossRef PubMed
.
- C. Kopp, F. Longordo, J. R. Nicholson and A. Luthi, J. Neurosci., 2006, 26, 12456–12465 CrossRef CAS PubMed
.
- F. Longordo, J. Fan, T. Steimer, C. Kopp and A. Luthi, Sleep, 2011, 34, 679–681 Search PubMed
.
- G. W. Tannock and D. C. Savage, Infect. Immun., 1974, 9, 591–598 CAS
.
- M. Aguilera, P. Vergara and V. Martinez, Neurogastroenterol. Motil., 2013, 25, e515–e529 CrossRef CAS PubMed
.
- M. T. Bailey, S. E. Dowd, J. D. Galley, A. R. Hufnagle, R. G. Allen and M. Lyte, Brain, Behav., Immun., 2011, 25, 397–407 CrossRef CAS PubMed
.
- S. Zheng, M. Yu, X. Lu, T. Huo, L. Ge, J. Yang, C. Wu and F. Li, Clin. Chim. Acta, 2010, 411, 204–209 CrossRef CAS PubMed
.
- J. Saric, Y. Wang, J. Li, M. Coen, J. Utzinger, J. R. Marchesi, J. Keiser, K. Veselkov, J. C. Lindon, J. K. Nicholson and E. Holmes, J. Proteome Res., 2008, 7, 352–360 CrossRef CAS PubMed
.
- B. L. Goodwin, C. R. Ruthven and M. Sandler, Biochem. Pharmacol., 1994, 47, 2294–2297 CrossRef CAS
.
- H. J. Lees, J. R. Swann, I. D. Wilson, J. K. Nicholson and E. Holmes, J. Proteome Res., 2013, 12, 1527–1546 CrossRef CAS PubMed
.
- A. J. Schwab, L. Tao, T. Yoshimura, A. Simard, F. Barker and K. S. Pang, Am. J. Physiol.: Gastrointest. Liver Physiol., 2001, 280, G1124–G1136 CAS
.
- A. M. Asatoor, Biochim. Biophys. Acta, 1965, 100, 290–292 CrossRef CAS
.
- E. Diaz, A. Ferrandez, M. A. Prieto and J. L. Garcia, Microbiol. Mol. Biol. Rev., 2001, 65, 523–569 CrossRef CAS PubMed
, table of contents.
- J. L. Smith, J. S. Wishnok and W. M. Deen, Toxicol. Appl. Pharmacol., 1994, 125, 296–308 CrossRef CAS PubMed
.
- S. H. Zeisel, K. A. DaCosta and J. G. Fox, Biochem. J., 1985, 232, 403–408 CAS
.
- M. E. Dumas, R. H. Barton, A. Toye, O. Cloarec, C. Blancher, A. Rothwell, J. Fearnside, R. Tatoud, V. Blanc, J. C. Lindon, S. C. Mitchell, E. Holmes, M. I. McCarthy, J. Scott, D. Gauguier and J. K. Nicholson, Proc. Natl. Acad. Sci. U. S. A., 2006, 103, 12511–12516 CrossRef CAS PubMed
.
- G. T. Macfarlane and S. Macfarlane, Curr. Opin. Biotechnol., 2007, 18, 156–162 CrossRef CAS PubMed
.
- D. L. Topping and P. M. Clifton, Physiol. Rev., 2001, 81, 1031–1064 CAS
.
- A. M. Neyrinck, V. F. Van Hee, L. B. Bindels, F. De Backer, P. D. Cani and N. M. Delzenne, Br. J. Nutr., 2013, 109, 802–809 CrossRef CAS PubMed
.
- N. P. McNulty, M. Wu, A. R. Erickson, C. Pan, B. K. Erickson, E. C. Martens, N. A. Pudlo, B. D. Muegge, B. Henrissat, R. L. Hettich and J. I. Gordon, PLoS Biol., 2013, 11, e1001637 CAS
.
- A. Hakansson, N. Tormo-Badia, A. Baridi, J. Xu, G. Molin, M. L. Hagslatt, C. Karlsson, B. Jeppsson, C. M. Cilio and S. Ahrne, Clin. Exp. Med., 2014, 11, 11 Search PubMed
.
- J. M. Kinross, N. Alkhamesi, R. H. Barton, D. B. Silk, I. K. Yap, A. W. Darzi, E. Holmes and J. K. Nicholson, J. Proteome Res., 2011, 10, 277–287 CrossRef CAS PubMed
.
- E. Skordi, I. K. Yap, S. P. Claus, F. P. Martin, O. Cloarec, J. Lindberg, I. Schuppe-Koistinen, E. Holmes and J. K. Nicholson, J. Proteome Res., 2007, 6, 4572–4581 CrossRef CAS PubMed
.
Footnote |
† Electronic supplementary information (ESI) available. See DOI: 10.1039/c4mb00463a |
|
This journal is © The Royal Society of Chemistry 2015 |
Click here to see how this site uses Cookies. View our privacy policy here.