DOI:
10.1039/C2MD20242E
(Review Article)
Med. Chem. Commun., 2013,
4, 80-87
On the origins of drug polypharmacology
Received
16th August 2012
, Accepted 25th October 2012
First published on 29th October 2012
Abstract
The ability of small molecules to interact with multiple proteins is commonly referred to as polypharmacology. The now widely accepted polypharmacology of drugs is of particular interest for human health as it has implications beyond therapeutic efficacy, from anticipating adverse drug reactions to identifying potential repurposing opportunities. There have been a number of studies relating the extent of drug polypharmacology to the physicochemical properties and fragment composition of the drug itself, but also to the protein family and distant binding site similarities of the drug's primary target. Taken together, all these observations lead to speculate that the origins of drug polypharmacology may lie at the heart of protein evolution and that polypharmacology may just be a reminiscent signature of some of the mechanisms of adaptation that primitive biological systems developed to increase the chances of survival in a highly variable early chemical environment.
Introduction
For centuries, the use of traditional remedies from vegetal and animal sources was based on anecdotical evidence of their therapeutic benefits in people for a wide range of discomforts and maladies.1 Just over hundred years ago, the industrial production and commercial exploitation of these materia medica gave rise to the first pharmaceutical companies.2 Advances mainly in synthetic and analytical chemistry, on one side, and in vitro and in vivo pharmacology, on the other side, led progressively to the discovery of the small-molecule active ingredients responsible for the therapeutic action of natural extracts and eventually to the identification of the interacting proteins associated with diseases. With time, these therapeutically relevant proteins will be perceived as “biological targets” for chemicals, and drug discovery will gradually shift from a long and serendipitous phenotype-based investigational work to a more linear, focussed, and manageable target-based research process.3
Based on the one drug–one target premise, one of the main objectives of drug discovery projects in the last two decades was to deliver potent and selective small-molecule candidates against individual protein targets. Unfortunately, due to limited time and resources, small molecules were usually not screened systematically against a large panel of proteins but solely to a handful of them that could include the target of interest to the project and a few phylogenetically related proteins. A major implication of this modus operandi was that our perception of drug selectivity has been for years strongly biased by our limited knowledge of the drug's complete target profile.4
Recently, several public and private initiatives to collect and store drug–target interaction data published in bibliographical sources have contributed significantly to change this biased perception of drug selectivity.5,6 It is now widely recognised that selective drugs are more the exception rather than the rule and that most therapeutically effective molecules tend to interact with multiple proteins.7 This is clearly reflected in Fig. 1 showing the distribution of drugs interacting with different ranges of targets, as derived from data available in public sources.5 As can be observed, only 15% of drugs are currently known to interact with one single target only, whereas over 50% of them interact with more than five targets. This is likely to be a highly conservative view of drug selectivity, still limited strongly by the lack of completeness of drug–target interaction data.4
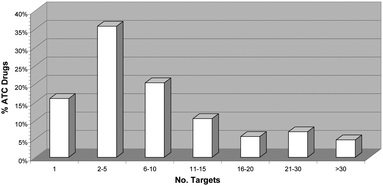 |
| Fig. 1 Distribution of the percentage of drugs in the Anatomical Therapeutic Chemical (ATC) classification10 for which bioactivity on protein targets has currently been reported in public sources of pharmacological data.5 A drug is considered bioactive to a protein if its activity (Act) is better than 10 μM, that is, its pAct = −log(Act) is higher than 5, Act being any Ki, Kd, IC50 or EC50 data available. | |
As a result, the adequacy of optimising bioactive small molecules on affinity and selectivity for individual “biological targets” is now being challenged in favor of a more holistic multitarget view of drug discovery. Following this trend, the ability of a molecule to interact with multiple proteins is popularly referred to as “polypharmacology”. To the best of our knowledge, the word polypharmacology was first used in 1971 by Domino8 as a synonym of polypharmacy, that is, the use of multiple drugs in a single prescription (polypharmakos + logos: the study of multiple drugs). However, in a 1997 article by Kenny et al.,9 the term polypharmacology was used to refer to the lack of α1-subtype adrenoceptor selectivity of the drug indoramin, that was known at the time to interact with other receptors, such as COMPOUND LINKS
Read more about this on ChemSpider
Download mol file of compoundserotonin and COMPOUND LINKS
Read more about this on ChemSpider
Download mol file of compoundhistamine, leading to sedation as a side effect (poly + pharmakologos: the multiple studies of drugs). The use of the term polypharmacology in the latter sense is now widespread among the drug discovery community.
Now that drug polypharmacology is widely proven and recognised, the question is: why do most drugs hit multiple targets? We sought to review the main observations around drug polypharmacology and to learn about what could be the origins of this apparently inherent promiscuity of small molecules when interacting with proteins. Note that, throughout this work, the term promiscuity will be used as a synonym of polypharmacology to refer to the binding of a molecule to multiple proteins and shall not be confused with the behaviour of promiscuous aggregators due to self-association into colloids at high concentrations in biological buffers described elsewhere.11
Chemical sources of polypharmacology
Two main aspects have been highlighted as chemical sources linked to polypharmacology, namely, molecular properties and fragment composition, both very much related to each other. The idea that simple molecules are more likely to bind to multiple proteins than complex molecules was launched over a decade ago12 and it has been one of the main arguments in favour of fragment-based drug discovery.13 However, conflicting results have been obtained recently on this matter from various groups and thus the link between complexity and selectivity may not be as obvious and universal as originally anticipated.
Molecular properties
Using a simplistic model of ligand–protein interactions, Hann et al.12 used patterns of positive and negative features to schematically represent protein binding sites and ligands. Considering a successful recognition event when all features of one sign from the ligand match features of the other sign from the binding site, they deduced that as the number of potentially interacting features in a molecule increases, the chance of measuring a useful interaction (that is, both detectable experimentally and unique in its mode of binding) falls dramatically. Therefore, increasing molecular complexity will tend to limit drug polypharmacology. From a thermodynamics point of view, Ferenczy and Keseru14 analysed the enthalpic and entropic components of the binding affinity. While there exists a direct relationship between heavy atom count and pKd (Kd being the dissociation constant), it was found that the enthalpic component is inversely related to the size of the ligand. This trend agrees well with the simple model of Hann et al.12 Since the enthalpic component is dominated by polar interactions that are directional, a gain in binding enthalpy needs optimal protein–ligand complementarity, the likelihood of which drops dramatically as the ligand size increases. Taking molecular weight (MW) and lipophilicity (clog P) as rough surrogate descriptors of molecular complexity (reflecting size and relative presence of polar and apolar features, respectively), the analysis of a set of 75
000 compounds tested on 220 assays led Hopkins et al.15 to identify an inverse correlation between mean MW and target promiscuity (defined in their work as the number of targets for which a compound has an affinity of pIC50 ≥ 5). In a smaller set of 1098 drug compounds from BioPrint6a they also noted that the most promiscuous drugs tend to be highly hydrophobic (clog P ≥ 3). Similar trends between MW and promiscuity were also found by Morphy and Rankovic16 from an analysis of a limited set of 138 diverse compounds for which affinity data (pKi or pIC50) were available for three phylogenetically related targets. Along the same lines, Mestres et al.17 analysed a set of 802 drugs and concluded that within a given range of MW values, promiscuity tends to increase with hydrophobicity but also that, within a given range of clog P values, promiscuity has a tendency to decrease with size (Fig. 2).
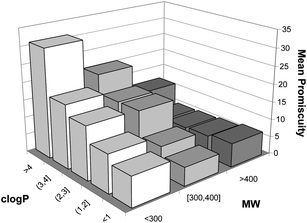 |
| Fig. 2 Variation of the predicted mean promiscuity of 802 drugs contained within value ranges of molecular weight (MW) and hydrophobicity (clog P) (reproduced with permission of the Royal Society of Chemistry). | |
However, even though the connection between hydrophobicity and promiscuity has been generally observed, inconsistent conclusions are obtained with respect to the inverse correlation between MW and promiscuity. For example, using a set of 3138 compounds tested on a panel of more than 50 safety assays, Azzaoui et al.18 showed clearly that promiscuity increased with size. A similar outcome was obtained by Leeson and Springthorpe19 upon analysing a set of 2333 drug-like compounds for which affinity was available on 200 assays. In contrast, just to add more to this particular controversy, Peters et al.20 were not able to find any remarkable correlation between MW and promiscuity in a set of 213 compounds tested across more than 60 assays. In agreement with this result, a more recent study by Gleeson et al.,21 on a large set of 40
408 molecules for which at least three measured affinities (pXC50 ≥ 6) were available in ChEMBL,5b did not find any significant correlation between MW and promiscuity, although when corrected for the number of measurements, large molecules were found significantly more likely to have micromolar affinities than small molecules.
Arguments to explain these contradictions include the realisation of the fact that many cellular activities depend ultimately on the ability of molecules to pass through cell membranes and this requires ever increasing amounts of lipophilicity as MW increases.22 It could well be that this increase in lipophilicity is responsible for the unexpected promiscuity of such large complex molecules. But many other factors could also contribute to this apparent disparity, including the particular nature of the compound set analysed (bias to optimised large molecules), the concrete panel of targets used (bias to safety assays), and even the affinity cut-off taken to define target promiscuity (bias to high affinity). Further analyses on all these aspects are certainly required.
Fragment composition
Over 15 years ago, Bemis and Murcko23 noticed that the structures of a set of 5120 drugs could be described with a reduced number of just 32 molecular frameworks. This motivated the search for privileged structural motifs that could enhance the ability of small molecules to bind to multiple proteins.24,25 The existence of privileged substructures for specific therapeutic classes, such as G protein-coupled receptors (GPCRs) or kinases, has been suggested and incorporated into the design of targeted chemical libraries.26 In general terms, the relationship between the presence of positively charged fragments (e.g., COMPOUND LINKS
Read more about this on ChemSpider
Download mol file of compoundpiperazine) and promiscuity is now widely recognised,18–20,27 whereas the presence of negatively charged groups (e.g., carboxylic acid) has got conflicting reviews in this respect.18,21,25 Also, an early analysis by Oprea28 highlighted the relevance of the number of rings and rigid bonds (aromatic) in the drug-like character of molecules, an aspect that Ertl et al.29 later developed further by identifying the presence of a relatively small number of common aromatic ring fragments in bioactive small molecules.
But apart from rather generic hints on particular functional groups that could enhance or limit target promiscuity and analyses on the frequency of ring fragments in molecules, studies on the polypharmacology of fragments that constitute molecular structures are not abundant. Among them, Chen and Shoichet30 provided crystallographic evidence that high hit rates obtained with fragments were explained by their low affinity to multiple proteins and that, as fragments progressed into larger more complex molecules, both affinity and selectivity improved. Further examples of this inherent binding promiscuity of fragments were reviewed recently.13,31 Combining substructure analysis and molecular descriptors, Roche et al.32 were able to successfully classify a particular class of compounds referred to as “frequent hitters” for their ability to appear as hits in many assays covering a wide range of targets.
To probe the correlation between chemical complexity and target promiscuity, a set of 364
465 unique molecules with binding affinities available from public sources5 were fragmented. The set of unique smallest fragments resulting from breaking each molecule at all possible bonds allowed within some predefined rules33 constitutes what is referred to as “level 1” fragments. Within each molecule, two connected “level 1” fragments form a “level 2” fragment; then, links between “level 1” and “level 2” fragments generate “level 3” fragments and so on, until the molecule is fully reconstructed. A total of 4
575
051 unique fragments from level 1 up to level 28 were generated with this process, and a target promiscuity value was assigned to each of them by counting the number of unique targets for which the molecules that contained them had reported affinity more potent than 10 μM.
A promiscuity analysis was then performed on all fragments up to “level 5” extracted from a set of 1221 drugs fragmented into 5 fragments or more. The results are illustrated in Fig. 3 as a boxplot, showing the distribution of the target promiscuity for all fragments at a given level up to “level 5”, superimposed on a violin plot, reflecting the density of such distributions within each fragment level. Taking the fragment level as a surrogate description of structural complexity within each molecule, it becomes apparent that as fragments become larger and more complex in the process of reconstructing the full molecule, the distribution of target promiscuities tends to concentrate significantly around lower mean promiscuities, very much in agreement with a simple complexity model.12 This provides further support for the success of fragment-based drug discovery13 and the ability of chemical fragments to interact with multiple unrelated protein environments.30,34
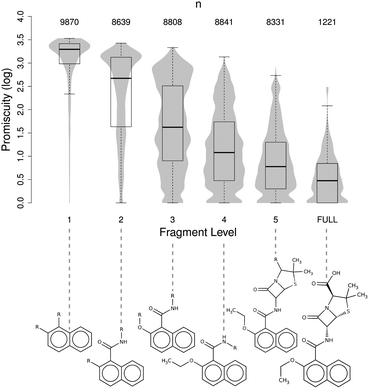 |
| Fig. 3 Boxplot showing the variation of the mean target promiscuity of fragments with increased size and complexity (up to “level 5”) compared to the mean promiscuity observed in 1221 drugs having 5 fragments or more (full). The superimposed violin plots reflect the density of the distribution. For the sake of clarity, sample fragments of COMPOUND LINKS
Read more about this on ChemSpider
Download mol file of compoundnafcillin at each fragment level are provided. | |
Biological sources of polypharmacology
If a small molecule binds to a given protein, there are chances for it to bind also to other proteins related by sequence identity (target phylogeny) and/or binding site similarity. Two targets binding to similar ligands are considered to have related pharmacologies, a property also referred to as cross-pharmacology. Target phylogeny is undoubtedly the major risk factor for cross-pharmacology. But cross-pharmacology relationships among distantly related targets that share similar binding site properties, although rare, also do exist. Therefore, molecules binding to proteins belonging to large families of closely related members and/or having local binding site similarities will be inherently predisposed to polypharmacology.
Target phylogeny
Accumulating evidence that small molecules binding to a given protein tend to have residual affinities for other phylogenetically related proteins motivated the implementation of chemogenomics strategies in drug discovery, aiming at organising research around target families as a means to maximise efficiency of chemical and biological resources and to improve hit rates.35 Among those families, GPCRs26a,36 and kinases,37 but also nuclear receptors,38 are of major concern to polypharmacology. Accordingly, particular focus has been given to data extraction and knowledge generation for the design of targeted chemical libraries directed to these families.39 In addition, cytochrome P450s have been also highlighted among the most promiscuous target classes.40
A recent study attempted to quantify the degree of expected polypharmacology for drugs having affinity for targets belonging to the four main families of therapeutic relevance, namely, enzymes, ion channels/transporters, nuclear receptors, and GPCRs.17 To this aim, the list of 480 targets experimentally known to interact with a set of 802 drugs was organised in those four families. Then, drugs were assigned to each target family if the drug had known affinity for a member of that family. For illustrative purposes, the resulting drug–target networks7 are presented in Fig. 4. The drug–target network derived for enzymes (Fig. 4a) contains 1112 interactions connecting 431 drugs and 191 targets, resulting in an average number of experimentally known interactions per drug of 2.6. Similarly, the corresponding networks derived for ion channels/transporters (Fig. 4b), containing 623 interactions between 268 drugs and 93 targets, and nuclear receptors (Fig. 4c), linking 77 drugs to 19 targets through 155 interactions, result in average numbers of experimentally known interactions per drug of 2.3 and 2.0, respectively. In contrast, the drug–target network for GPCRs represents 2646 interactions between 396 drugs and 106 targets, which correspond to an average number of interactions per drug of 6.7.
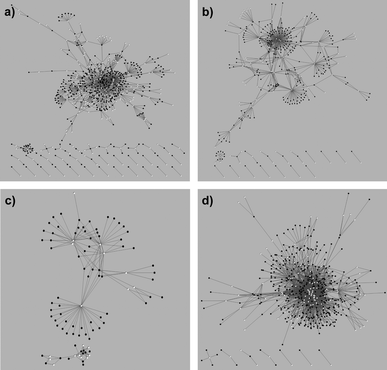 |
| Fig. 4
Drug–target interaction networks derived for the different target families: (a) enzymes, (b) ion channels/transporters, (c) nuclear receptors, and (d) G protein-coupled receptors. Drugs and targets are indicated as black and white circles, respectively. (Reproduced with permission of the Royal Society of Chemistry.) | |
Overall, this analysis emphasised the fact that protein families are implicitly associated to different basal degrees of target promiscuity. In particular, the study anticipated that if a drug has affinity for an aminergic GPCR, there are chances for it to have residual affinity for 6 other GPCRs on average, whereas if a drug has affinity for an enzyme (other than kinases), an ion channel/transporter, or a nuclear receptor, it may have on average residual affinity at most for another target of the same family.
Binding site similarity
The possibility for a small molecule to bind to multiple phylogenetically unrelated proteins is made difficult by the wide diversity observed in the size, shape, and electrostatic features of binding cavities that impose essentially different molecular properties and fragment compositions in bioactive molecules. The availability of pharmacological data on the interaction between thousands of molecules and proteins has allowed one to perform statistical analyses on the differences in molecular properties observed in bioactive molecules to members of the most therapeutically relevant protein families.17,40,41 For instance, Morphy41a analysed a collection of 1860 bioactive molecules extracted from bibliographic sources covering 12 target classes and concluded that the ligands for peptide GPCRs, integrin receptors, proteases, and transferases possessed statistically higher median property values (MW and clog P) than ligands for monoamine GPCRs, oxidases, ion channels, and transporters. Using an entirely different set of 642 marketed drugs, Vieth and Sutherland41b arrived at similar conclusions and showed that, for example, the mean MW for drugs acting on ion channels and proteases is 305.5 and 430.6, both deviating significantly (by defect and excess, respectively) from the average MW of all drugs considered. In the context of polypharmacology, the significant variations observed in the molecular properties of bioactive small molecules across target families imply that, in principle, not all target cross-pharmacologies may be compatible and, if they are, rather different binding efficiencies may be attained.42
In spite of these inherent difficulties, cross-pharmacology relationships beyond phylogeny are encountered. Detailed quantitative analyses of the degree of cross-pharmacology observed and predicted between all main target classes of therapeutic interest have been performed.27,40 The complex drug–target network presented in Fig. 5 illustrates nicely the intricate inter-family cross-pharmacologies observed in current drugs.7 Instead of having isolated network components associated to individual target classes, all target classes appear to be connected in a single large network through several drug hubs that bind to phylogenetically unrelated proteins. For example, even though all aminergic GPCRs are grouped in a dense cluster, evident distant cross-pharmacologies exist, particularly with some peptidic GPCRs and other enzymes.7 Also, cross-pharmacology relationships between kinases and peptidic GPCRs are clearly visible.7 Further confirmatory examples of such cross-pharmacologies between distantly related proteins were reported recently.43
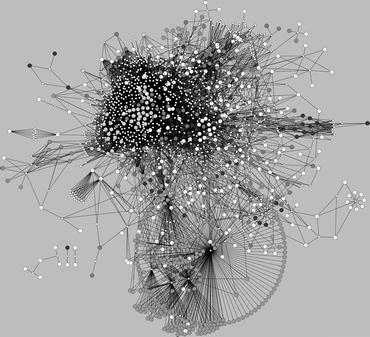 |
| Fig. 5
Drug–target network. Drugs (small white circles) are linked to targets if their affinity is better than 1 μM. | |
Apart from the wealth of pharmacological data available from which distant cross-pharmacologies can be identified,5,6 there is also an increasing amount of protein structure information that can be exploited to detect binding site similarities between phylogenetically unrelated proteins.44 However, experimentally determined protein structures are still very much biased to enzymes, although in recent years great progress has been made towards solving GPCR structures.45 In spite of this target class bias, the development of efficient algorithms to detect binding site similarities46 has led to the successful identification of cross-pharmacologies between distant enzymes.47 Of particular interest to polypharmacology are the applications of these approaches in identifying similar distantly related protein environments.34 In this respect, a recent work by Reisen et al.48 provided an exhaustive list of similar unrelated pocket pairs extracted from the PDBbind database.49 More recently, Jalencas and Mestres34b introduced the concept of chemoisosterism as the property that relates different protein environments interacting with the same chemical fragment. Ligands constituted by fragments linked to a large number of diverse chemoisosteric environments will be predisposed to binding to multiple distantly related proteins.
Implications of drug polypharmacology
As it has been exposed in the previous sections, there is ample evidence that drugs bind to multiple proteins and, based on this information, the main chemical and biological sources that are likely to promote drug polypharmacology have been identified. However, as emphasised above, this is likely to be a highly conservative view of polypharmacology due to the lack of completeness of drug–target interaction data.4 In this respect, the recent development of computational methods for predicting the targets of known drugs is having a profound impact on alleviating the effects of this lack of completeness.50 Just in the last five years, a total of 249 new drug–target interactions have been identified by several of those methods,51 which represent an increase of almost 7% of all drug-target interactions currently known for those targets. The distribution of all 249 experimentally confirmed drug–target affinities is depicted in Fig. 6.
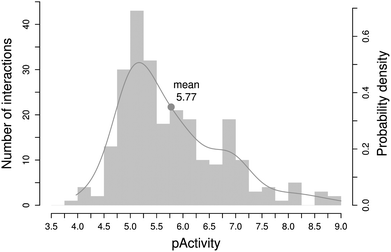 |
| Fig. 6 Distribution of experimental affinities for the 249 novel drug–target interactions identified by computational methods in the last five years. | |
As can be observed, the vast majority of the new drug–target interactions identified are located around the 10 μM range, potent enough to be biologically relevant at high drug doses (overdosage). Therefore, one of the direct implications of drug polypharmacology is that many of these side affinities may be implicated in adverse drug reactions.52 But, in addition, there are also a decent number of new interactions with affinities below 300 nM (ca. pAct > 6.5) that could be biologically relevant at therapeutic drug doses. Should these interactions be with targets associated with therapeutic indications other than the one the drug currently approves, they could represent good opportunities for further exploiting a marketed drug. Accordingly, a second direct implication of drug polypharmacology is in the field of drug repurposing.53Drug toxicity and repurposing are two contrasting aspects of utmost importance in current drug discovery that would highly benefit from a deeper understanding of drug polypharmacology.
Concluding remarks
In their struggle for life, organisms need to constantly adapt to the environment and eventually those developing an optimal fit will be naturally selected to prevail and survive.54 We ought to take here the definition of the environment in its broadest sense and consider also the chemical environment from which organisms will take materials and generate energy. It is in this respect that, as extensively exposed in earlier seminal works,55 living systems are tightly coupled to their chemical milieu. Therefore, it is not difficult to assume that early biological systems evolved towards maximum exploitation of the chemicals available and that systems better prepared for changes in those highly variable environmental conditions may have had higher chances of survival. Under those circumstances, biological systems may have developed mechanisms of adaptation to changes in the chemical composition of the environment. One of them would be increasing plasticity of proteins to favour chemical promiscuity, eventually leading to functional promiscuity.56 But another one may have been retaining all non-damaging randomly generated protein variants and keeping them silent in the genetic background, so that when new chemical entities are formed and become available in the environment, they could be readily assimilated and exploited if an optimal fit were possible with any of those silent proteins. Indeed, it has been shown that the human genome contains a large number of neutral elements unconstrained across mammalian evolution, constituting a warehouse of active elements to natural selection.57 This could well be a plausible reminiscence of that pro-active adaption strategy to chemical environmental changes.
After all these considerations, one is tempted to speculate that the origins of polypharmacology lie precisely at the heart of protein evolution. Primitive biological systems may have been forced to reuse the limited organic material available to increase the chances of survival by replicating protein motifs for different functions (binding site similarity). Later on, the same mechanism may have been used by more advanced organisms to expand the number of members of particular protein families related to complex signalling processes (target phylogeny). Therefore, the levels of polypharmacology observed in current drugs may well just be a latent signature of evolution itself.
Gaining a deeper knowledge on the origins of drug polypharmacology is the first step towards understanding our real capabilities in managing target promiscuity within a therapeutically relevant target space, an aspect that has important implications in anticipating toxicity risks and identifying repurpose opportunities. With recent progress in laboratory evolution,58 this is an area to be further explored in the near future.
Acknowledgements
This research was funded by the Spanish Ministerio de Economía y Competitividad (BIO2011-26669 and PTA2009-1865-P) and the Instituto de Salud Carlos III.
Notes and references
-
(a) B. Schmidt, D. M. Ribnicky, A. Poulev, S. Logendra, W. T. Cefalu and I. Raskin, Metabolism, 2008, 57(suppl. 1), S3 CrossRef CAS;
(b) F. Watkins, B. Pendry, O. Corcoran and A. Sanchez-Medina, Drug Discovery Today, 2011, 16, 1069 CrossRef.
- T. A. Ban, Dialogues Clin. Neurosci., 2006, 8, 335 Search PubMed.
- D. Brown, Drug Discovery Today, 2007, 12, 1007 CrossRef CAS.
- J. Mestres, E. Gregori-Puigjané, S. Valverde and R. V. Solé, Nat. Biotechnol., 2008, 26, 983 CrossRef CAS.
-
(a) D. S. Wishart, C. Knox, A. C. Guo, D. Cheng, S. Shrivastava, D. Tzur, B. Gautam and M. Hassanali, Nucleic Acids Res., 2008, 36, D901 CrossRef CAS;
(b) A. Gaulton, L. J. Bellis, A. P. Bento, J. Chambers, M. Davies, A. Hersey, Y. Light, S. McGlinchey, D. Michalovich, B. Al-Lazikani and J. P. Overington, Nucleic Acids Res., 2012, 40, D1100 CrossRef CAS;
(c) Y. Wang, E. Bolton, S. Dracheva, K. Karapetyan, B. A. Shoemaker, T. O. Suzek, J. Wang, J. Xiao, J. Zhang and S. H. Bryant, Nucleic Acids Res., 2010, 38, D255 CrossRef CAS;
(d) N. H. Jensen and B. L. Roth, Comb. Chem. High Throughput Screening, 2008, 11, 420 CrossRef CAS;
(e) A. J. Harmar, R. A. Hills, E. M. Rosser, M. Jones, O. P. Buneman, D. R. Dunbar, S. D. Greenhill, V. A. Hale, J. L. Sharman, T. I. Bonner, W. A. Catterall, A. P. Davenport, P. Delagrange, C. T. Dollery, S. M. Foord, G. A. Gutman, V. Laudet, R. R. Neubig, E. H. Ohlstein, R. W. Olsen, J. Peters, J. P. Pin, R. R. Ruffolo, D. B. Searls, M. W. Wright and M. Spedding, Nucleic Acids Res., 2009, 37, D680 CrossRef CAS;
(f) T. Liu, Y. Lin, X. Wen, R. N. Jorrisen and M. K. Gilson, Nucleic Acids Res., 2007, 35, D198 CrossRef CAS;
(g) M. L. Benson, R. D. Smith, N. A. Khazanov, B. Dimcheff, J. Beaver, P. Dresslar, J. Nerothin and H. A. Carlson, Nucleic Acids Res., 2008, 36, D674 CrossRef CAS.
-
(a) C. M. Krejsa, D. Horvath, S. L. Rogalski, J. E. Penzotti, B. Mao, F. Barbosa and J. C. Migeon, Curr. Opin. Drug Discovery Dev., 2003, 6, 470–480 CAS;
(b)
M. Olah, M. Mracec, L. Ostopovici, R. Rad, A. Bora, N. Hadaruga, I. Olah, M. Banda, Z. Simon and T. I. Oprea, in Chemoinformatics in Drug Discovery, ed. T. I. Oprea, Wiley-VCH, New York, 2004, p. 223 Search PubMed.
- I. Vogt and J. Mestres, Mol. Inf., 2010, 29, 10 CrossRef CAS.
- E. F. Domino, JAMA, J. Am. Med. Assoc., 1971, 215, 1160 CrossRef CAS.
- B. Kenny, S. Ballard, J. Blagg and D. Fox, J. Med. Chem., 1997, 40, 1293 CrossRef CAS.
-
WHO Collaborating Centre for Drug Statistics Methodology, Guidelines for ATC Classification and DDD Assignment, Oslo, 2012 Search PubMed.
- J. Seidler, S. L. McGovern, T. N. Doman and B. K. Shoichet, J. Med. Chem., 2003, 46, 4477 CrossRef CAS.
-
(a) M. M. Hann, A. R. Leach and G. Harper, J. Chem. Inf. Comput. Sci., 2001, 41, 856 CrossRef CAS;
(b) A. R. Leach and M. M. Hann, Curr. Opin. Chem. Biol., 2011, 15, 489 CrossRef CAS.
- G. Bottegoni, A. D. Favia, M. Recanatini and A. Cavalli, Drug Discovery Today, 2012, 17, 23 CrossRef CAS.
- G. G. Ferenczy and G. M. Keseru, J. Chem. Inf. Model., 2010, 50, 1536 CrossRef CAS.
- A. L. Hopkins, J. S. Mason and J. P. Overington, Curr. Opin. Struct. Biol., 2006, 16, 127 CrossRef CAS.
- R. Morphy and Z. Rankovic, Drug Discovery Today, 2007, 12, 156 CrossRef CAS.
- J. Mestres, E. Gregori-Puigjané, S. Valverde and R. V. Solé, Mol. BioSyst., 2009, 5, 1051 RSC.
- K. Azzaoui, J. Hamon, B. Faller, S. Whitebread, E. Jacoby, A. Bender, J. L. Jenkins and L. Urban, ChemMedChem, 2007, 2, 874 CrossRef CAS.
- P. D. Leeson and B. Springthorpe, Nat. Rev. Drug Discovery, 2007, 6, 881 CrossRef CAS.
- J. U. Peters, P. Schnider, P. Mattei and M. Kansy, ChemMedChem, 2009, 4, 680 CrossRef CAS.
- M. P. Gleeson, A. Hersey, D. Montanari and J. Overington, Nat. Rev. Drug Discovery, 2011, 10, 197 CrossRef CAS.
- M. M. Hann, Med. Chem. Commun., 2011, 2, 349 RSC.
- G. W. Bemis and M. A. Murcko, J. Med. Chem., 1996, 39, 2887 CrossRef CAS.
- X. Q. Lewell, D. B. Judd, S. P. Watson and M. M. Hann, J. Chem. Inf. Comput. Sci., 1998, 38, 511 CrossRef CAS.
- P. J. Hajduk, M. Bures, J. Praestgaard and S. W. Fesik, J. Med. Chem., 2000, 43, 3443 CrossRef CAS.
-
(a) T. Klabunde and G. Hessler, ChemBioChem, 2002, 3, 928 CrossRef CAS;
(b) G. Müller, Drug Discovery Today, 2003, 8, 681 CrossRef;
(c) K. Bondensgaard, M. Ankersen, H. Thoegersen, B. S. Hansen, B. S. Wulff and R. P. Bywater, J. Med. Chem., 2004, 47, 888 CrossRef CAS;
(d) R. W. DeSimone, K. S. Currie, S. A. Mitchell, J. W. Darrow and D. A. Pippin, Comb. Chem. High Throughput Screening, 2004, 7, 473 CrossRef CAS;
(e) D. M. Schnur, M. A. Hermsmeier and A. J. Tebben, J. Med. Chem., 2006, 49, 2000 CrossRef CAS;
(f) M. E. Welsch, S. A. Snyder and B. R. Stockwell, Curr. Opin. Chem. Biol., 2010, 14, 347 CrossRef CAS.
- E. Gregori-Puigjané and J. Mestres, Comb. Chem. High Throughput Screening, 2008, 11, 669 CrossRef.
- T. I. Oprea, J. Comput.-Aided Mol. Des., 2000, 14, 251 CrossRef CAS.
- P. Ertl, S. Jelfs, J. Mühlbacher, A. Schuffenhauer and P. Selzer, J. Med. Chem., 2006, 49, 4568 CrossRef CAS.
- Y. Chen and B. K. Shoichet, Nat. Chem. Biol., 2009, 5, 358 CrossRef CAS.
- S. Barelier and I. Krimm, Curr. Opin. Chem. Biol., 2011, 15, 469 CrossRef CAS.
- O. Roche, P. Schneider, J. Zuegge, W. Guba, M. Kansy, A. Alanine, K. Bleicher, F. Danel, E. M. Gutknecht, M. Rogers-Evans, W. Neidhart, H. Stalder, M. Dillon, E. Sjögren, N. Fotouhi, P. Gillespie, R. Goodnow, W. Harris, P. Jones, M. Taniguchi, S. Tsujii, W. von der Saal, G. Zimmermann and G. Schneider, J. Med. Chem., 2002, 45, 137 CrossRef CAS.
- M. Wagener and J. P. M. Lommerse, J. Chem. Inf. Model., 2006, 46, 677 CrossRef CAS.
-
(a) J. Behnen, H. Köster, G. Neudert, T. Craan, A. Heine and G. Klebe, ChemMedChem, 2012, 7, 248 CrossRef CAS;
(b)
X. Jalencas and J. Mestres, J. Chem. Inf. Model., under revision Search PubMed.
-
(a) S. V. Frye, Chem. Biol., 1999, 6, R3 CrossRef CAS;
(b) P. R. Caron, M. D. Mullican, R. D. Mashal, K. P. Wilson, M. S. Su and M. A. Murcko, Curr. Opin. Chem. Biol., 2001, 5, 464 CrossRef CAS;
(c) M. Bredel and E. Jacoby, Nat. Rev. Genet., 2004, 5, 262 CrossRef CAS;
(d) J. Mestres, Curr. Opin. Drug Discovery Dev., 2004, 7, 304 CAS;
(e) C. J. Harris and A. P. Stevens, Drug Discovery Today, 2006, 11, 880 CrossRef CAS;
(f) T. Klabunde, Br. J. Pharmacol., 2007, 152, 5 CrossRef CAS;
(g) D. Rognan, Br. J. Pharmacol., 2007, 152, 38 CrossRef CAS;
(h) J. Bajorath, Curr. Opin. Chem. Biol., 2008, 12, 352 CrossRef CAS;
(i) L. Jacob and J. P. Vert, Bioinformatics, 2008, 24, 2149 CrossRef CAS;
(j) M. Cases and J. Mestres, Drug Discovery Today, 2009, 14, 479 CrossRef CAS.
-
(a) F. Briansó, M. C. Carrascosa, T. I. Oprea and J. Mestres, Curr. Top. Med. Chem., 2011, 11, 1956 CrossRef;
(b) J. D. Wichard, A. Ter Laak, G. Krause, N. Heinrich, R. Kühne and G. Kleinau, PLoS One, 2011, 6, e16811 CAS;
(c) T. M. Frimurer and T. Högberg, Curr. Top. Med. Chem., 2011, 11, 1882 CrossRef CAS;
(d) H. Zhou and J. Skolnick, Mol. Pharmaceutics, 2012, 9, 1775 CrossRef CAS;
(e) P. K. Madala, D. P. Fairlie and M. Bodén, J. Chem. Inf. Model., 2012, 52, 1401 CrossRef CAS.
-
(a) M. Vieth, J. J. Sutherland, D. H. Robertson and R. M. Campbell, Drug Discovery Today, 2005, 10, 839 CrossRef CAS;
(b) J. T. Metz, E. F. Johnson, N. B. Soni, P. J. Merta, L. Kifle and P. J. Hajduk, Nat. Chem. Biol., 2011, 7, 200 CrossRef CAS.
-
(a) M. Shapira, R. Abagyan and M. Totrov, J. Med. Chem., 2003, 46, 3045 CrossRef;
(b) M. Cases, R. Garcia-Serna, K. Hettne, M. Weeber, J. van der Lei, S. Boyer and J. Mestres, Curr. Top. Med. Chem., 2005, 5, 763 CrossRef CAS;
(c) J. Mestres, L. Martín-Couce, E. Gregori-Puigjané, M. Cases and S. Boyer, J. Chem. Inf. Model., 2006, 46, 2725 CrossRef CAS.
-
(a) J. L. Miller, Curr. Top. Med. Chem., 2006, 6, 19 CrossRef CAS;
(b) E. Gregori-Puigjané and J. Mestres, Curr. Opin. Chem. Biol., 2008, 12, 359 CrossRef;
(c) I. Akritopoulou-Zanze and P. J. Hajduk, Drug Discovery Today, 2009, 14, 291 CrossRef CAS.
- G. V. Paolini, R. H. B. Shapland, W. P. Van Hoorn, J. S. Mason and A. L. Hopkins, Nat. Biotechnol., 2006, 24, 805 CrossRef CAS.
-
(a) R. Morphy, J. Med. Chem., 2006, 49, 2969 CrossRef CAS;
(b) M. Vieth and J. J. Sutherland, J. Med. Chem., 2006, 49, 3451 CrossRef CAS.
- J. Mestres and E. Gregori-Puigjané, Trends Pharmacol. Sci., 2009, 30, 470 CrossRef CAS.
-
(a) R. Hajjo, V. Setola, B. L. Roth and A. Tropsha, J. Med. Chem., 2012, 55, 5704 CrossRef CAS;
(b) X. Lin, X. P. Huang, G. Chen, R. Whaley, S. Peng, Y. Wang, G. Zhang, S. X. Wang, S. Wang, B. L. Roth and N. Huang, J. Med. Chem., 2012, 55, 5749 CrossRef CAS.
- P. W. Rose, B. Beran, C. Bi, W. F. Bluhm, D. Dimitropoulos, D. S. Goodsell, A. Prlic, M. Quesada, G. B. Quinn, J. D. Westbrook, J. Young, B. Yukich, C. Zardecki, H. B. Berman and P. E. Bourne, Nucleic Acids Res., 2011, 39, D392 CrossRef.
-
(a) J. Mestres, Drug Discovery Today, 2005, 10, 1629 CrossRef CAS;
(b) L. Xie and P. E. Bourne, PLoS Comput. Biol., 2005, 1, e31 CrossRef;
(c) R. Garcia-Serna, L. Opatowski and J. Mestres, Bioinformatics, 2006, 22, 1792 CrossRef CAS;
(d) J. A. Salon, D. T. Lodowski and K. Palczewski, Pharmacol. Rev., 2011, 63, 901 CrossRef CAS.
-
(a) M. Stahl, C. Taroni and G. Schneider, Protein Eng., 2000, 13, 83 CrossRef CAS;
(b) S. Schmitt, D. Kuhn and G. Klebe, J. Mol. Biol., 2002, 323, 387 CrossRef CAS;
(c) A. Shulman-Peleg, R. Nussinov and H. J. Wolfson, J. Mol. Biol., 2004, 339, 607 CrossRef CAS;
(d) N. D. Gold and R. M. Jackson, Nucleic Acids Res., 2006, 34, D231 CrossRef CAS;
(e) Z. Zhang and M. G. Grigorov, Protein Sci., 2006, 62, 470 CAS;
(f) N. Westkamp, E. Hüllermeier and G. Klebe, Protein Sci., 2009, 76, 317 CrossRef;
(g) N. Weill and D. Rognan, J. Chem. Inf. Model., 2010, 50, 123 CrossRef CAS;
(h) J. Konc and D. Janezic, Nucleic Acids Res., 2012, 40, W214 CrossRef;
(i) I. Kufareva, A. V. Ilatovskiy and R. Abagyan, Nucleic Acids Res., 2012, 40, W535 CrossRef;
(j) J.-I. Ito, Y. Tabei, K. Shimizu, K. Tsuda and K. Tomii, Nucleic Acids Res., 2012, 40, W541 CrossRef.
- A. Weber, A. Casini, A. Heine, D. Kuhn, C. T. Supuran, A. Scozzafava and G. Klebe, J. Med. Chem., 2004, 47, 550 CrossRef CAS.
- F. Reisen, M. Weisel, J. M. Kriegla and G. Schneider, J. Proteome Res., 2010, 9, 6498 CrossRef CAS.
- R. Wang, X. Fang, Y. Lu, C. Y. Yang and S. Wang, J. Med. Chem., 2005, 48, 4111 CrossRef CAS.
-
(a) D. Rognan, Mol. Inf., 2010, 29, 176 CrossRef CAS;
(b) J. A. Allen and B. L. Roth, Annu. Rev. Pharmacol. Toxicol., 2011, 51, 117 CrossRef CAS;
(c) L. Xie, L. Xie, S. L. Kinnings and P. E. Bourne, Annu. Rev. Pharmacol. Toxicol., 2012, 52, 361 CrossRef CAS.
-
(a) M. J. Keiser, B. L. Roth, B. N. Armbruster, P. Ernsberger, J. J. Irwin and B. K. Shoichet, Nat. Biotechnol., 2007, 25, 197 CrossRef CAS;
(b) M. Campillos, M. Kuhn, A. C. Gavin, L. J. Jensen and P. Bork, Science, 2008, 321, 263 CrossRef CAS;
(c) M. J. Keiser, V. Setola, J. J. Irwin, C. Laggner, A. I. Abbas, S. J. Hufeisen, N. H. Jensen, M. B. Kuijer, R. C. Matos, T. B. Tran, R. Whaley, R. A. Glennon, J. Hert, K. L. Thomas, D. D. Edwards, B. K. Shoichet and B. L. Roth, Nature, 2009, 462, 175 CrossRef CAS;
(d) A. J. DeGraw, M. J. Keiser, J. D. Ochocki, B. K. Shoichet and M. D. Distefano, J. Med. Chem., 2010, 53, 2464 CrossRef CAS;
(e) C. Moneriz, J. Mestres, J. M. Bautista, A. Diez and A. Puyet, FEBS J., 2011, 278, 2951 CrossRef CAS;
(f) J. Mestres, S. A. Seifert and T. I. Oprea, Clin. Pharmacol. Ther., 2011, 90, 662 CrossRef CAS;
(g) A. Schlessinger, E. Geier, H. Fan, J. J. Irwin, B. K. Shoichet, K. M. Giacomini and A. Sali, Proc. Natl. Acad. Sci. U. S. A., 2011, 108, 15810 CrossRef CAS;
(h) E. Lounkine, M. J. Keiser, S. Whitebread, D. Mikhailov, J. Hamon, J. L. Jenkins, P. Lavan, E. Weber, A. K. Doak, S. Côté, B. K. Shoichet and L. Urban, Nature, 2012, 486, 361 CAS;
(i) E. Gregori-Puigjané, V. Setola, J. Hert, B. A. Crews, J. J. Irwin, E. Lounkine, L. Marnett, B. L. Roth and B. K. Shoichet, Proc. Natl. Acad. Sci. U. S. A., 2012, 109, 11178 CrossRef.
-
(a) I. Kola and J. Landis, Nat. Rev. Drug Discovery, 2004, 3, 711 CrossRef CAS;
(b) A. F. Fliri, W. T. Loging, P. F. Thadeio and R. A. Volkmann, Nat. Chem. Biol., 2005, 1, 389 CrossRef CAS;
(c) A. M. Richard, Chem. Res. Toxicol., 2006, 19, 1257 CrossRef CAS;
(d) K. M. Giacomini, R. M. Krauss, D. M. Roden, M. Eichelbaum, M. R. Hayden and Y. Nakamura, Nature, 2007, 446, 975 CrossRef CAS;
(e) A. Bender, J. Scheiber, M. Glick, J. W. Davies, K. Azzaoui, J. Hamon, L. Urban, S. Whitebread and J. L. Jenkins, ChemMedChem, 2007, 2, 861 CrossRef CAS;
(f) J. Scheiber, B. Chen, M. Milik, S. C. Sukuru, A. Bender, D. Mikhailov, S. Whitebread, J. Hamon, K. Azzaoui, L. Urban, M. Glick, J. W. Davies and J. L. Jenkins, J. Chem. Inf. Model., 2009, 49, 308 CrossRef CAS;
(g) R. Garcia-Serna and J. Mestres, Expert Opin. Drug Metab. Toxicol., 2010, 6, 1253 CrossRef CAS;
(h) X. Wang and N. Greene, Mol. Inf., 2012, 31, 145 CrossRef CAS.
-
(a) T. T. Ashburn and K. B. Thor, Nat. Rev. Drug Discovery, 2004, 3, 673 CrossRef CAS;
(b) C. R. Chong and D. J. Sullivan, Nature, 2006, 448, 645 CrossRef;
(c) P. Schneider, Y. Tanrikulu and G. Schneider, Curr. Med. Chem., 2009, 16, 258 CrossRef CAS;
(d) T. I. Oprea, S. K. Nielsen, O. Ursu, J. J. Yang, O. Taboureau, S. L. Mathias, I. Kouskoumvekaki, L. A. Sklar and C. G. Bologa, Mol. Inf., 2011, 30, 100 CrossRef CAS;
(e) T. I. Oprea and J. Mestres, AAPS J., 2012, 14, 759 CrossRef CAS.
-
(a)
P. Matthew, On Naval Timber and Arboriculture, Adam Black, Edinburgh, 1831 Search PubMed;
(b)
R. Chambers, Vestiges of the Natural History of Creation, John Churchill, London, 1844 Search PubMed;
(c)
C. R. Darwin, On the Origin of Species, John Murray, London, 1859 Search PubMed;
(d)
A. R. Wallace, The Geographical Distribution of Animals with a Study of the Relations of Living and Extinct Faunas as Elucidating the Past Changes of the Earth's Surface, Macmillan, London, 1876 Search PubMed.
-
(a)
A. I. Oparin, The Origin of Life, Macmillan, New York, 1938 Search PubMed;
(b)
J. Lovelock, Gaia: New Look at Life on Earth, Oxford University Press, Oxford, 1979 Search PubMed;
(c)
R. J. P. Williams and J. J. R. Fraústo da Silva, The Chemistry of Evolution, Elsevier, Amsterdam, 2006 Search PubMed.
-
(a) R. A. Jensen, Annu. Rev. Microbiol., 1976, 30, 409 CrossRef CAS;
(b) P. J. O'Brien and D. Herschlag, Chem. Biol., 1999, 6, R91 CrossRef CAS;
(c) A. Aharoni, L. Gaidukov, O. Khersonsky, S. McQ Gould, C. Roodveldt and D. S. Tawfik, Nat. Genet., 2005, 37, 73 CAS;
(d) O. Khersonsky, C. Roodveldt and D. S. Tawfik, Curr. Opin. Chem. Biol., 2006, 10, 498 CrossRef CAS;
(e) I. Nobeli, A. D. Favia and J. M. Thornton, Nat. Biotechnol., 2009, 27, 157 CrossRef CAS.
- E. Birney,
et al.
, Nature, 2007, 447, 799 CrossRef CAS.
- K. M. Esvelt, J. C. Carlson and D. R. Liu, Nature, 2011, 472, 499 CrossRef CAS.
|
This journal is © The Royal Society of Chemistry 2013 |
Click here to see how this site uses Cookies. View our privacy policy here.