DOI:
10.1039/C2MB25265A
(Method)
Mol. BioSyst., 2013,
9, 36-43
qPCA: a scalable assay to measure the perturbation of protein–protein interactions in living cells†
Received
6th July 2012
, Accepted 27th September 2012
First published on 27th September 2012
Abstract
One of the most important challenges in systems biology is to understand how cells respond to genetic and environmental perturbations. Here we show that the yeast DHFR-PCA, coupled with high-resolution growth profiling (DHFR-qPCA), is a straightforward assay to study the modulation of protein–protein interactions (PPIs) in vivo as a response to genetic, metabolic and drug perturbations. Using the canonical Protein Kinase A (PKA) pathway as a test system, we show that changes in PKA activity can be measured in living cells as a modulation of the interaction between its regulatory (Bcy1) and catalytic (Tpk1 and Tpk2) subunits in response to changes in carbon metabolism, caffeine and methyl methanesulfonate (MMS) treatments and to modifications in the dosage of its enzymatic regulators, the phosphodiesterases. Our results show that the DHFR-qPCA is easily implementable and amenable to high-throughput. The DHFR-qPCA will pave the way to the study of the effects of drug, genetic and environmental perturbations on in vivo PPI networks, thus allowing the exploration of new spaces of the eukaryotic interactome.
Introduction
Protein–protein interactions (PPIs) are fundamental for all cellular functions.1 In particular, they allow the cell to perceive external stimuli and generate appropriate physiological responses. In the last decade, the development of high-throughput techniques to study PPIs has led to the first maps of the protein interactome of several model organisms.2–7 These maps described new associations within and among functional modules2 and among protein complexes and cellular functions.3 One limitation of these maps is that they have mainly been determined under one single experimental condition. Studying how protein interactomes change under different conditions would allow us to understand how cells adapt to different environments, how they respond to drugs, stressors and genetic perturbations as well as to understand the basis of cellular development and differentiation.8 In order to achieve these objectives, it is necessary to develop new techniques or to adapt existing ones. Ideally, these techniques should be simple, easily implementable and amenable to high-throughput. Because studying how cells respond to perturbations of PPIs requires being able to detect them in their endogenous environments, these assays should also be performed in living cells and among proteins that are natively regulated.
Protein Complementation Assay (PCA) is a family of techniques that is now widely used to study PPI networks.9,10 All different variants of PCA are based on the same principles: two complementary fragments of a reporter protein are fused to two proteins of interest. If the two proteins interact, the activity of the reporter protein is reconstituted such that it provides a detectable signal. One of the most cost-effective PCA techniques is the DHFR-PCA. In this case the reporter protein is a modified murine dihydrofolate reductase (Dhfr) that confers resistance to the chemical methotrexate (MTX).2 Therefore, the presence of an interaction between two proteins of interest can be detected and measured as cellular growth on media supplemented with MTX. This PCA has recently been successfully used to determine a large part of the budding yeast PPI network in living cells.2 In yeast, the assay requires that the coding sequences of the DHFR fragments (DHFR F[1,2] and DHFR F[3]) are inserted into the genome at the 3′-end of two genes of interest to produce proteins with the DHFR fragments fused at the C-termini (Fig. 1). The endogenous gene modification offers the advantage of minimally perturbing the transcriptional regulation of the gene and does not require a modification of the protein native localizations.
![Rationale of the DHFR-PCA. The gene encoding the engineered mouse DHFR is split into two complementary fragments, DHFR F[1,2] and DHFR F[3] that are then fused to the genes encoding two proteins of interest A and B. The concentration of tetrahydrofolate (THF) produced increases with the amount of DHFR complexes formed and is expected to affect growth in a dose-dependent fashion.](/image/article/2013/MB/c2mb25265a/c2mb25265a-f1.gif) |
| Fig. 1 Rationale of the DHFR-PCA. The gene encoding the engineered mouse DHFR is split into two complementary fragments, DHFR F[1,2] and DHFR F[3] that are then fused to the genes encoding two proteins of interest A and B. The concentration of tetrahydrofolate (THF) produced increases with the amount of DHFR complexes formed and is expected to affect growth in a dose-dependent fashion. | |
Here we show that the fitness-based yeast DHFR-PCA,2 combined with high-resolution growth profiling (DHFR-qPCA), can be successfully used to study changes in PPIs in vivo in different conditions and genetic backgrounds, and thus represents a tool that can be used to explore new dimensions of protein interactomes. Using high-resolution growth profiling it is possible to follow the growth of yeast strains in microchambers and in real-time. This allows a precise and sensitive measurement of the growth curves of several strains in parallel and in different growth media.
Results and discussion
The DHFR-qPCA signal reflects the amount of protein complexes formed in the cell
We first examined whether the DHFR-PCA signal provides a quantitative measure of PPIs (Fig. 1), which is a minimal requirement for the measurement of changes in PPIs under different conditions. Here by quantitatively we mean that the PCA signal correlates with the quantity of protein complexes formed by two interacting proteins and that changes in PCA signal can be reproducibly measured. We first tested this hypothesis by combining protein abundance data11 with the PCA data from Tarassov et al.,2 where the PCA signal is measured as colony size on agar plates containing MTX. We found a highly significant correlation between the average abundance of two interacting proteins and PCA signal (ρ = 0.18, p-value < 2.2 × 10−16), and this correlation is significantly improved by considering the abundance of the least expressed protein of the interacting pair (ρ = 0.32, p-value < 2.2 × 10−16, Fig. 2). This result suggests that the PCA signal reflects the abundance of the protein complexes formed in the cell.
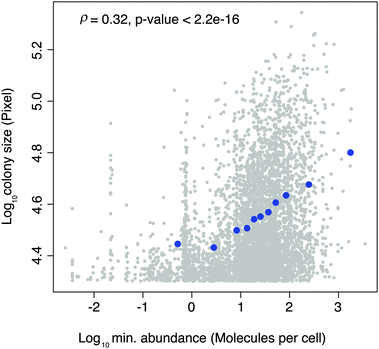 |
| Fig. 2 Relationship between colony size (DHFR-PCA signal) and the abundance of the least expressed protein of interacting pairs. Grey dots represent raw data and blue dots binned data. | |
We then tested whether changes in PPIs could be detected in the absence of modification of protein abundance. We randomly selected 15 PPIs from the yeast DHFR-PCA network2 with high (7 pairs), medium (2 pairs) and low (6 pairs) protein abundances (see Table S1, ESI†).12 We constructed yeast strains carrying different combinations of DHFR tagged genes in diploid cells: one allele of each locus (1:1) or both alleles of each locus (2:2) (Fig. 3A). These constructs allowed us to directly manipulate by four-fold the amount of DHFR reconstituted without modifying protein abundance. When only one allele of each gene is fused to the DHFR fragments (A′ and B′ for the tagged alleles, A and B for the untagged alleles), four types of protein complexes can be formed: A′–B′, A–B′, A′–B, A–B. Therefore in the 1:1 strains, only the A′–B′ complexes (1/4) would provide a DHFR-PCA signal. If both alleles of both genes are tagged (2:2 strains), all complexes would be of type A′–B′ and thus 100% of PPIs of the complex would provide a DHFR-PCA signal. In both cases, the concentrations of proteins A and B are unaltered.
![(A) Fifteen diploid strains with one (1:1) or both alleles (2:2) of two genes (GENE A, light blue and GENE B, yellow) tagged with DHFR fragments (DHFR F[1,2] in red and DHFR F[3] in dark blue, respectively) were constructed in order to test whether the DHFR-PCA signal could be modulated without changing protein abundance (the genes are under the control of their native promoters). In these diploid strains, only the number of alleles of each locus that are fused to the DHFR fragments varies. (B) Parameters used to describe yeast growth curves (slope (ΔOD/Δt), efficiency (ODfinal − ODinitial) and lag time) and their correlations. (C) Example of raw data of a DHFR-qPCA experiment showing the growth profiles for the Vps29–Vps35 interaction. Each curve represents an independent biological replicate. While in DMSO (control) the 1:1 and 2:2 strains have the same growth profile, in MTX the 2:2 has a significantly shorter lag time than the 1:1 (t-test; p-value < 0.001). (D) Results of the DHFR-qPCA test for 14 PPIs (1:1 and 2:2 backgrounds; 15th interaction shown in panel C). All independent replicates are shown for each interaction. Grey points represent growth in DMSO (control), while colored points represent growth in MTX. Red points show interactions among proteins with high expression levels; blue, medium expression and black low expression. Dashed lines associate the same interaction in the two different backgrounds. The significance of the difference in lag time between the 1:1 and 2:2 backgrounds in MTX is shown for each interaction (t-test; ***: p-value < 0.001; *: p-value between 0.01 and 0.05; NS: non-significant). (E) Spot-dilution assays show that difference in growth rates can also be detected on solid medium. Results for the Vps29–Vps35 interaction are shown (cell dilution 1 : 10). An isogenic strain carrying the two DHFR fragments alone expressed on plasmids provides a negative control. DMSO is the MTX solvent and is thus used as a control for growth.](/image/article/2013/MB/c2mb25265a/c2mb25265a-f3.gif) |
| Fig. 3 (A) Fifteen diploid strains with one (1:1) or both alleles (2:2) of two genes (GENE A, light blue and GENE B, yellow) tagged with DHFR fragments (DHFR F[1,2] in red and DHFR F[3] in dark blue, respectively) were constructed in order to test whether the DHFR-PCA signal could be modulated without changing protein abundance (the genes are under the control of their native promoters). In these diploid strains, only the number of alleles of each locus that are fused to the DHFR fragments varies. (B) Parameters used to describe yeast growth curves (slope (ΔOD/Δt), efficiency (ODfinal − ODinitial) and lag time) and their correlations. (C) Example of raw data of a DHFR-qPCA experiment showing the growth profiles for the Vps29–Vps35 interaction. Each curve represents an independent biological replicate. While in DMSO (control) the 1:1 and 2:2 strains have the same growth profile, in MTX the 2:2 has a significantly shorter lag time than the 1:1 (t-test; p-value < 0.001). (D) Results of the DHFR-qPCA test for 14 PPIs (1:1 and 2:2 backgrounds; 15th interaction shown in panel C). All independent replicates are shown for each interaction. Grey points represent growth in DMSO (control), while colored points represent growth in MTX. Red points show interactions among proteins with high expression levels; blue, medium expression and black low expression. Dashed lines associate the same interaction in the two different backgrounds. The significance of the difference in lag time between the 1:1 and 2:2 backgrounds in MTX is shown for each interaction (t-test; ***: p-value < 0.001; *: p-value between 0.01 and 0.05; NS: non-significant). (E) Spot-dilution assays show that difference in growth rates can also be detected on solid medium. Results for the Vps29–Vps35 interaction are shown (cell dilution 1 : 10). An isogenic strain carrying the two DHFR fragments alone expressed on plasmids provides a negative control. DMSO is the MTX solvent and is thus used as a control for growth. | |
We applied high-resolution growth profiling (see Methods) to these strains in DMSO (control) and MTX and estimated growth parameters from the growth profile. As a first step, we determined which growth parameter would maximise the power to detect changes in PPIs. We compared the slope, the efficiency and the lag time required to reach the maximum growth rate (Fig. 3B). We found that all parameters are strongly correlated with each other (Fig. 3B) and thus largely redundant. Because the lag time maximizes the correlation between replicates (ρ = 0.90, p-value < 6.81 × 10−5) and is therefore less sensitive to experimental error, we used it as an estimate of growth and thus of PCA signal. For 14 out of 15 protein pairs, lag time was significantly lower for the 2:2 strains than for the 1:1 strains (Fig. 3C and D). These four-fold differences in DHFR-PCA signal can also be detected on solid medium (Fig. 3E).
We also sought to test whether the PCA signal would reflect the dissociation constant (Kd) of a protein complex. Block et al.13 showed how point mutations in the Ras Binding Domain (RBD) affect the Kd of the Ras–RBD complex. We tested the interactions between Ras and five RBD mutants with different Kds by DHFR-qPCA. As expected, to a decrease in Kd corresponds an increase in PCA signal (Fig. 4). Altogether, these results (Fig. 2–4) indicate that the DHFR-qPCA provides a quantitative readout of the amount of protein complexes formed between interaction partners, even when changes in the amount of complex formed do not involve changes in protein abundance.
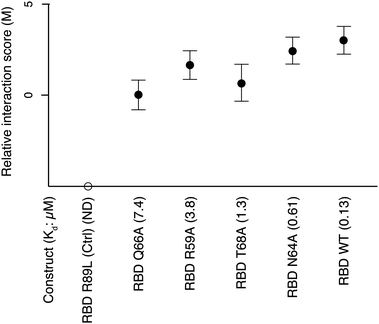 |
| Fig. 4 DHFR-qPCA signal for interactions between Ras and different RBD mutants. PCA signal increases with a decrease in Kd of the different mutant Ras–RBD protein complexes. The R89L mutant shows no interaction and its M score is arbitrarily set on this graph. The numbers in parenthesis indicate the Kd of each complex in μM units. Seventeen independent biological replicates in two independent experiments were used to perform DHFR-qPCA assays for each Ras–RBD complex. | |
DHFR-qPCA allows the measurement of the effects of metabolic, drug and genetic perturbations on protein complexes
We next sought to test directly the approach on a canonical signaling pathway by using different perturbations and combinations of perturbations. For this purpose, we chose the well-characterized protein kinase A (PKA) complex. The PKA is a tetramer formed by two regulatory and two catalytic subunits14 that is regulated by cAMP levels (Fig. 5A). The PKA pathway regulates different processes such as glucose metabolism,15 protein translation,16 ribosome biogenesis,17 stress responses,18 autophagy19 and lifespan.20 In yeast, the regulatory subunits are encoded by the gene BCY1, while the catalytic subunits are encoded by three different genes: TPK1, TPK2 and TPK3.21,22 We studied the interactions between the regulatory subunit (Bcy1) and the catalytic subunits (Tpk1 and Tpk2, respectively) by DHFR-qPCA in response to four different perturbations: (1) galactose, which leads to a decrease in PKA activity relative to glucose;23 (2) caffeine, which was shown to indirectly inhibit the PKA24 through the TORC1 pathway; (3) methyl methanesulfonate (MMS), a DNA damaging agent, which has recently been associated with the PKA pathway25 and was shown to lead to the phosphorylation of the PKA regulatory subunit26 and (4) dosage of the PKA regulator PDE (phosphodiesterase), which negatively regulates the PKA by degrading cAMP.27 Each of these conditions is known or has been hypothesized to affect the PKA pathway in yeast but has not been assessed at the level of the protein complex dissociation.
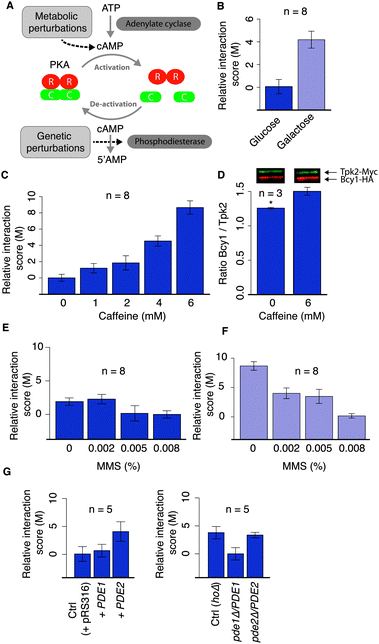 |
| Fig. 5 Condition-dependence of the interactions between the PKA regulatory and catalytic subunits in response to different perturbations. (A) The activation/inactivation of the PKA is regulated by intracellular levels of cAMP, which is modulated among other mechanisms by the enzymes adenylate cyclase and phosphodiesterase (Pde1 and Pde2 in yeast). (B) Comparison of the DHFR-qPCA signal for the Bcy1–Tpk2 interaction in glucose and galactose. (C) DHFR-qPCA signal for the Bcy1–Tpk2 interaction in cells grown in media supplemented with caffeine at different concentrations. (D) Co-immunoprecipitation of Bcy1 and Tpk2 in standard medium (YPD) and in YPD supplemented with caffeine confirms the DHFR-qPCA results (t-test, p-value < 0.05). (E) DHFR-qPCA signal for the Bcy1–Tpk2 interaction in cells grown in media supplemented with methyl methanesulfonate (MMS). (F) DHFR-qPCA signal for the Bcy1–Tpk2 interaction in cells grown in media supplemented with galactose and MMS at different concentrations. (G) DHFR-qPCA signal for the Bcy1–Tpk2 interaction in strains carrying an additional copy (on a low copy number plasmid) or a deletion of one copy (heterozygous strain) of the genes coding for the PDE enzymes (left and right panel, respectively). In all cases, n represents the number of independent biological replicates. | |
We estimated the effect of each perturbation under control conditions (without MTX) and subtracted this effect to estimate the net PCA signal in MTX. We obtained the difference in lag time between MTX and DMSO (ΔL) from which we computed a relative interaction score value (M, see Methods). Our results show that the DHFR-qPCA can detect changes in the PKA activity as a response to metabolic, drug and genetic perturbations (Fig. 5 for Bcy1–Tpk2; Fig. S1, ESI† for Bcy1–Tpk1). We observed more interaction between the regulatory and catalytic subunits of the PKA in galactose (Fig. 5B; Fig. S1A, ESI†) and in the presence of caffeine (Fig. 5C; Fig. S1B, ESI†) when compared with standard growth conditions. This confirms that the PKA is inhibited under these conditions23,24 and this inhibition involves changes in Bcy1–Tpk1 and Bcy1–Tpk2 interactions. Further, our results show that the DHFR-qPCA can detect concentration-dependent effects on the PKA complex, as PKA inhibition increases as caffeine concentration increases (Fig. 5C; Fig. S1B, ESI†). In this particular case, we also measured changes in the PKA complex using co-immunoprecipitation and confirmed this quantitative effect on the PKA (for Bcy1–Tpk2) (Fig. 5D). The DHFR-qPCA signal appears to provide a larger amplitude of changes in the interaction than co-immunoprecipitation, which is most likely due to the fact that the DHFR-qPCA assay is a fitness based assay which serves as a signal amplifier. This property could be exploited for instance for the measurement of subtle changes in PPIs. We also found that the effect of MMS on the PKA was stronger in galactose than in glucose (Fig. 5E and F; Fig. S1C and D, ESI†). The PKA might indeed be maximally activated in glucose and the addition of MMS does not allow to activate it to an extent that can be detected with this assay. It is also possible that DNA damage affects the PKA pathway in a carbon source-dependent fashion, with a greater effect on non-fermentable carbon sources. Finally, we found that modifying the dosage of PDE1 or PDE2 leads to significant changes in the amount of PKA complex formed, consistent with their roles as negative regulators of the PKA complex27 (Fig. 5G; Fig. S1E, ESI†). This result shows that the DHFR-qPCA allows the detection of subtle quantitative genetic perturbations (50% of gene product) that affect PPIs.
Conclusions
PPIs regulate many cellular processes and are therefore expected to be dynamic and condition-dependent, i.e. the degree of association among proteins will depend on the conditions to which cells are exposed. There is therefore a strong need for the development of simple assays to measure changes in PPIs. Here we show that the yeast DHFR-qPCA is a quantitative technique that allows the screening of PPIs under different conditions at low cost. Our results, with those of Schlecht et al.,28 show that the DHFR-qPCA can be used to study PPIs under different conditions and at high-throughput. Ninety-six interactions could be tested simultaneously in a standard plate-reader or more in dedicated instruments (see Methods). Unlike PCA assays based on luciferase,29 this assay does not allow us to measure dynamic PPIs in real-time, because it is based on fitness. However, this offers the advantage that fitness differences among conditions or strains can be amplified through generations and may thus allow us to detect very small changes of interactions. The PCA signal might be saturated for interactions with low-Kd and/or highly abundant proteins. However, we expect that this would occur for a limited number of interactions under natural conditions as we see a strong correlation between the abundance of proteins and PCA signal over five orders of magnitude of protein abundance without saturation (Fig. 2). With the availability of entire yeast collections tagged with the DHFR fragments,2 any pairwise interaction of interest could be investigated under different conditions and in different genetic backgrounds. The DHFR-qPCA will pave the way to the study of the effects of drug, genetic and environmental perturbations on in vivo PPI networks, thus allowing the exploration of new spaces of the model eukaryotic interactome.
Materials and methods
Bioinformatic analysis of previous DHFR-PCA data
The integrated dataset on protein abundance was downloaded from PaxDb.11 Data on colony size were taken from Tarassov et al.2 In order to determine the correlation between PCA signal and protein abundance we calculated the Spearman's rank correlation coefficient as implemented in R.30
Construction of the strains used to test the DHFR-qPCA
Diploid strains with different numbers of DHFR-fused genes were constructed as follows. Haploid strains (BY4741 and BY4742 backgrounds; BY4741: MATa, his3Δ1, leu2Δ0, met15Δ0, ura3Δ0; BY4742: MATα, his3Δ1, leu2Δ0, lys2Δ0, ura3Δ0) carrying a single DHFR fragment (DHFR F[1,2] for MATa strains and DHFR F[3] for MATα strains) were purchased from Open Biosystems (http://www.openbiosystems.com) and were crossed as described by Tarassov et al.2 For each interaction listed in Table S1 (ESI†), we crossed the corresponding BY4741 MATa GENE A-DHFR F[1,2] strain with the BY4742 MATα GENE B-DHFR F[3] strain to generate diploid heterozygous strains GENE A-DHFR F[1,2]/GENE A, GENE B/GENE B-DHFR F[3]. These strains are 1:1 strains, as only one allele of each gene is tagged with a DHFR fragment. Then we sporulated these 1:1 strains, dissected the tetrads and genotyped the segregation of the DHFR fragments31 in order to obtain haploid strains carrying one allele of each gene tagged (MATa GENE A-DHFR F[1,2] GENE B-DHFR F[3] and MATα GENE A-DHFR F[1,2] GENE B-DHFR F[3], respectively). Finally, we crossed these haploid strains to generate 2:2 diploid strains with both alleles of each gene tagged (MATa/MATα GENE A-DHFR F[1,2]/GENE A-DHFR F[1,2], GENE B-DHFR F[3]/GENE B-DHFR F[3]). All strain genotypes are listed in Table S2 (ESI†). We confirmed all integrations by colony PCR32 with oligonucleotides listed in Table S3 (ESI†).
High-resolution growth profiling
Saturated overnight cultures in YPD with suitable antibiotics were diluted to an OD600 of 1 and then diluted 1/30 in 150 μL of SC (0.669% YNB w/o ammonium sulphate w/o amino acids, 2% glucose, drop-out -lys, -met, -ade) with MTX 200 μg mL−1 (Bioshop Canada Inc.) or the MTX solvent DMSO (Bioshop Canada Inc.) in a 100 well honeycomb plate (Growth Curves USA). We measured growth profiles using a Bioscreen C (Growth Curves USA) by reading the OD420–580 every 15 minutes with continuous agitation at 30 °C. For the experiments under different conditions we added caffeine (Bioshop Canada Inc.) and MMS (Sigma-Aldrich, concentration 99%) in a gradient of concentrations (final concentrations: 1 mM, 2 mM, 4 mM, 6 mM and 0.002%, 0.005%, 0.008%, respectively) or we used 2% galactose instead of 2% glucose in the SC medium. Growth curves were measured for at least three replicates from three independent precultures.
Comparison of the different parameters used to estimate growth
For each growth curve, three parameters were measured in order to obtain a quantitative estimate of cellular growth: the maximum growth rate (ΔOD/Δt), the efficiency (ODfinal − ODinitial) and the lag time. The maximum growth rate was calculated as follows. We used a sliding window approach to calculate a regression line for each interval spanning 31 measurements. Then, we sorted all regression coefficients and we determined the 98th percentile. We assumed this value to be the maximum growth rate. This approach allowed us to eliminate any extreme value that could result from experimental errors.33 The lag time was defined as the average time point of the interval where the maximum growth rate was observed. Finally, the efficiency was calculated as difference between the final and initial ODs. All analyses were performed in R.30
Growth curve analysis
In order to measure the relative interaction score (M) we used the following algorithm. We subtracted the lag time calculated in MTX (test) to the lag calculated in DMSO (control), which estimates the effect of the perturbation on the growth rate independently from the interaction. We called this difference ΔL. Then, for each experiment, in order to evaluate the differences in lag times between strains or conditions on a relative scale, we calculated a relative interaction score value (M) as a proxy for effective cellular growth (Fig. S2, ESI†). We first computed the maximum ΔL among the strains or conditions tested (ΔLmax). For each strain or condition we then determined M by subtracting ΔL from ΔLmax. The minimal interaction score is thus arbitrarily set to 0.
Spot assays
An overnight preculture in YPD was diluted to an OD600 = 1 and a ten-fold serial dilution was performed. Five μL of the cell suspensions were inoculated onto SC medium plates with MTX 200 μg mL−1 or DMSO.
Interactions between Ras and different RBD mutants
The DHFR F[1,2] coding sequence was amplified by PCR with oligonucleotides that contain restriction sites BspEI and XhoI, respectively, using the plasmid pAG25-linker-DHFR-F[1,2]-ADHterm2 as template and subcloned in the plasmid p413Gal1-Ras-yCD-F[1]34 cutting with BspEI and XhoI to fuse the Ras coding gene to the DHFR F[1,2]. A sequence encoding the DHFR F[3] was amplified from pAG32-linker-DHFR(3)-ADHterm2 using oligos that contain restriction sites BspEI and XhoI. The resulting PCR fragment was digested with the restriction enzymes BspEI and XhoI and subcloned in the six plasmids p415Gal1-RBD-yCD-F[2]34 that contain the wild-type RBD (residues 55–133) and five mutant RBDs. All constructed plasmids were verified by sequencing. A BY4741 strain was transformed with the plasmids p413Gal1-Ras-DHFR F[1,2] while six BY4742 strains were transformed with the plasmids p415Gal1-RBD-DHFR F[3] containing the wild-type and the five RBD mutants. The BY4741 strain was crossed with all the six BY4742 strains. The resulting diploid strains containing both plasmids were grown overnight in SC-Raffinose medium (0.669% YNB w/o ammonium sulphate w/o amino acids, 2% raffinose, drop-out -lys, -met, -ade, -his, -leu). High-resolution growth profiling experiments were performed in SC-Galactose medium (0.669% YNB w/o ammonium sulphate w/o amino acids, 2% galactose, drop-out -lys, -met, -ade, -his, -leu). Growth curves were measured in two experiments. In the first one, five replicates from five independent pre-cultures were used to test each interaction. In the second one, twelve independent replicates were used to test each interaction. Fig. 4 shows the combined results of the two experiments.
PKA regulatory and catalytic subunits interactions under different conditions
In order to study the perturbation of the PKA complex under different conditions, we constructed diploid strains using strains from the DHFR collection.2 We crossed the MATa strains (BY4741 background) with the TPK1 or the TPK2 genes fused to the DHFR F[1,2] with a MATα strain (BY4742 background) having the BCY1 gene tagged with DHFR F[3] to generate the diploid strains JFL001 and JFL002.
Strains carrying one additional copy of the PDE genes (PDE1 or PDE2) were obtained by transforming the JFL001 and JFL002 strains with plasmids from the MoBY collection.35 Plasmids of the MoBY collection carry one yeast gene (in this case PDE1 or PDE2) under the control of its native promoter and terminator as well as a URA selection cassette and a yeast centromeric sequence (CEN).35 We also transformed the JFL001 and JFL002 strains with an empty pRS316 plasmid, which also has a URA cassette and a CEN sequence, to generate a no insert control strain. We then constructed strains carrying a deletion of one allele of a different PDE gene. We performed two independent transformations to obtain haploid MATα BY4742 strains where TPK1 or TPK2 genes were tagged with the DHFR F[1,2] and BCY1 tagged with the DHFR F[3] (JFL003 and JFL004). Then we crossed the JFL003 and JFL004 strains with the hoΔ (control strain), pde1Δ and pde2Δ MATa strains from the YKO deletion collection.36 The strains of the YKO collection are BY4741 MATa strains where a single gene is interrupted by a fragment containing a KanMX cassette, which provides resistance to the antibiotic G418. We therefore obtained diploid strains that carry a deletion of one allele of a different PDE gene (JFL005, JFL006, JFL007 and JFL008) and control strains (JFL009 and JFL010). All strains were grown in rich medium (YPD) with antibiotics. Strains carrying the DHFR F[1,2] cassette were grown in the presence of Nourseothricin (Werner BioAgents; 100 μg mL−1), those carrying DHFR F[3] were grown in the presence of Hygromycin B (Bioshop Canada Inc.; 250 μg mL−1). Finally, strains carrying a KanMX cassette were grown in the presence of G418 (Bioshop Canada Inc.; 200 μg mL−1).
Co-immunoprecipitation and western blotting of Tpk2–Bcy1 (PKA)
Strain JFL011 used for immunoprecipitation was constructed as follows. Plasmid pYM1737 that contains six repeats of the HA tag and a natNT2 marker was amplified with specific oligonucleotides for the integration at the BCY1 locus (C-terminus) in a BY4742 strain. Then, we amplified plasmid pYM2037 that has nine Myc tags in tandem repeats with oligos for integration at the TPK2 locus (C-terminus). This PCR product was transformed into a BY4741 strain for homologous recombination. All oligonucleotides mentioned above are listed in Table S3 (ESI†). These haploid strains were crossed to generate the JFL011 diploid strain.
Six independent cultures of the strain JFL011 were grown in 5 mL of YPD overnight at 30 °C with shaking. The next day, cells were diluted to OD600 of 0.1 in 100 mL and grown to OD600 of 0.5. Three of the cultures were treated with caffeine (final concentration 6 mM in water) while for the remaining three (controls) treated with the same volume of water. All cultures were incubated for 30 min. After incubation, the equivalent 15 OD600 of cells were collected, washed once with 2 mL of zymolyase buffer (1 M sorbitol, 0.01 M phosphate-buffer pH 7.6, 0.02 M EDTA) and then resuspended in 4 mL with the same buffer. 4 μL of β-mercaptoethanol and 5 μL of zymolyase 20T (20 mg mL−1) were added to the cells and incubated at 37 °C for 23 min with agitation. The spheroplasts were washed with 1 M sorbitol, resuspended in 200 μL of lysis buffer (50 mM Tris–HCl pH 7.4, 0.01 M EDTA, 150 mM NaCl, 1% Triton X-100, PMSF 1 mM, Aprotinin 2 μg mL−1, Leupeptin 20 μg mL−1, Pepstatin A 2 μg mL−1) and incubated for 2 h on ice. Lysates were immunoprecipitated for 2 h at 4 °C with THE™ c-Myc Tag Antibody, mAB, Mouse (GenScript A00704) coupled to Dynabeads® M-280 Tosylactivated (Life Technologies Corporation), washed 3 times with 500 μL of cold washing buffer (0.1 M Na-phosphate pH 7.4, 0.08% Tween 20) and eluted in 40 μL of boiling 2× Laemmli Buffer for 10 min. The primary antibodies for Western blotting were the rabbit Anti-HA antibody (Rockland 600-401-384) for the HA tag, and THE™ c-Myc Tag Antibody. The secondary antibodies were IRDye® 680 conjugated Goat Anti-Rabbit (926-32221) and IRDye® 800 conjugated Goat Anti-mouse (926-32210) (LiCor). Dried membranes were scanned and processed using an Odyssey® Infrared Imaging System. Pixel quantification was performed using ImageJ64.38
Acknowledgements
This work was supported by a Canadian Institutes of Health Research (CIHR) Grant (GMX-191597) and a FRSQ New Investigator grant, and partly by a Human Frontier Science Program grant (RGY0073/2010), a Genome Québec grant and a NSERC discovery grant. LF was supported by a fellowship from the Fonds de recherche du Québec – Nature et technologies (FRQNT). LF and FTQ were supported by fellowships from the Quebec Research Network on Protein Function, Structure and Engineering (PROTEO). We thank all members of the Landry laboratory and Nadia Aubin-Horth for their comments on the manuscript.
Notes and references
- M. Vidal, M. E. Cusick and A. L. Barabasi, Cell, 2011, 144, 986–998 CrossRef CAS.
- K. Tarassov, V. Messier, C. R. Landry, S. Radinovic, M. M. S. Molina, I. Shames, Y. Malitskaya, J. Vogel, H. Bussey and S. W. Michnick, Science, 2008, 320, 1465–1470 CrossRef CAS.
- N. J. Krogan, G. Cagney, H. Y. Yu, G. Q. Zhong, X. H. Guo, A. Ignatchenko, J. Li, S. Y. Pu, N. Datta, A. P. Tikuisis, T. Punna, J. M. Peregrin-Alvarez, M. Shales, X. Zhang, M. Davey, M. D. Robinson, A. Paccanaro, J. E. Bray, A. Sheung, B. Beattie, D. P. Richards, V. Canadien, A. Lalev, F. Mena, P. Wong, A. Starostine, M. M. Canete, J. Vlasblom, S. Wu, C. Orsi, S. R. Collins, S. Chandran, R. Haw, J. J. Rilstone, K. Gandi, N. J. Thompson, G. Musso, P. St Onge, S. Ghanny, M. H. Y. Lam, G. Butland, A. M. Altaf-Ui, S. Kanaya, A. Shilatifard, E. O'Shea, J. S. Weissman, C. J. Ingles, T. R. Hughes, J. Parkinson, M. Gerstein, S. J. Wodak, A. Emili and J. F. Greenblatt, Nature, 2006, 440, 637–643 CrossRef CAS.
- T. Ito, T. Chiba, R. Ozawa, M. Yoshida, M. Hattori and Y. Sakaki, Proc. Natl. Acad. Sci. U. S. A., 2001, 98, 4569–4574 CrossRef CAS.
- J. F. Rual, K. Venkatesan, T. Hao, T. Hirozane-Kishikawa, A. Dricot, N. Li, G. F. Berriz, F. D. Gibbons, M. Dreze, N. Ayivi-Guedehoussou, N. Klitgord, C. Simon, M. Boxem, S. Milstein, J. Rosenberg, D. S. Goldberg, L. V. Zhang, S. L. Wong, G. Franklin, S. M. Li, J. S. Albala, J. H. Lim, C. Fraughton, E. Llamosas, S. Cevik, C. Bex, P. Lamesch, R. S. Sikorski, J. Vandenhaute, H. Y. Zoghbi, A. Smolyar, S. Bosak, R. Sequerra, L. Doucette-Stamm, M. E. Cusick, D. E. Hill, F. P. Roth and M. Vidal, Nature, 2005, 437, 1173–1178 CrossRef CAS.
- S. M. Li, C. M. Armstrong, N. Bertin, H. Ge, S. Milstein, M. Boxem, P. O. Vidalain, J. D. J. Han, A. Chesneau, T. Hao, D. S. Goldberg, N. Li, M. Martinez, J. F. Rual, P. Lamesch, L. Xu, M. Tewari, S. L. Wong, L. V. Zhang, G. F. Berriz, L. Jacotot, P. Vaglio, J. Reboul, T. Hirozane-Kishikawa, Q. R. Li, H. W. Gabel, A. Elewa, B. Baumgartner, D. J. Rose, H. Y. Yu, S. Bosak, R. Sequerra, A. Fraser, S. E. Mango, W. M. Saxton, S. Strome, S. van den Heuvel, F. Piano, J. Vandenhaute, C. Sardet, M. Gerstein, L. Doucette-Stamm, K. C. Gunsalus, J. W. Harper, M. E. Cusick, F. P. Roth, D. E. Hill and M. Vidal, Science, 2004, 303, 540–543 CrossRef CAS.
- L. Giot, J. S. Bader, C. Brouwer, A. Chaudhuri, B. Kuang, Y. Li, Y. L. Hao, C. E. Ooi, B. Godwin, E. Vitols, G. Vijayadamodar, P. Pochart, H. Machineni, M. Welsh, Y. Kong, B. Zerhusen, R. Malcolm, Z. Varrone, A. Collis, M. Minto, S. Burgess, L. McDaniel, E. Stimpson, F. Spriggs, J. Williams, K. Neurath, N. Ioime, M. Agee, E. Voss, K. Furtak, R. Renzulli, N. Aanensen, S. Carrolla, E. Bickelhaupt, Y. Lazovatsky, A. DaSilva, J. Zhong, C. A. Stanyon, R. L. Finley, K. P. White, M. Braverman, T. Jarvie, S. Gold, M. Leach, J. Knight, R. A. Shimkets, M. P. McKenna, J. Chant and J. M. Rothberg, Science, 2003, 302, 1727–1736 CrossRef CAS.
- T. Ideker and N. J. Krogan, Mol. Syst. Biol., 2012, 8, 565 CrossRef.
- M. Morell, S. Ventura and F. X. Aviles, FEBS Lett., 2009, 583, 1684–1691 CrossRef CAS.
- S. W. Michnick, P. H. Ear, E. N. Manderson, I. Remy and E. Stefan, Nat. Rev. Drug Discovery, 2007, 6, 569–582 CrossRef CAS.
- M. Wang, M. Weiss, M. Simonovic, G. Haertinger, S. P. Schrimpf, M. O. Hengartner and C. von Mering, Mol. Cell Proteomics, 2012, 11, 492–500 CAS.
- S. Ghaemmaghami, W. Huh, K. Bower, R. W. Howson, A. Belle, N. Dephoure, E. K. O'Shea and J. S. Weissman, Nature, 2003, 425, 737–741 CrossRef CAS.
- C. Block, R. Janknecht, C. Herrmann, N. Nassar and A. Wittinghofer, Nat. Struct. Biol., 1996, 3, 244–251 CrossRef CAS.
- P. Zhang, E. V. Smith-Nguyen, M. M. Keshwani, M. S. Deal, A. P. Kornev and S. S. Taylor, Science, 2012, 335, 712–716 CrossRef CAS.
- R. Dechant and M. Peter, Curr. Opin. Cell Biol., 2008, 20, 678–687 CrossRef CAS.
- M. P. Ashe, S. K. De Long and A. B. Sachs, Mol. Biol. Cell, 2000, 11, 833–848 CAS.
- D. E. Martin, A. Soulard and M. N. Hall, Cell, 2004, 119, 969–979 CrossRef CAS.
- V. Ramachandran, K. H. Shah and P. K. Herman, Mol. Cell, 2011, 43, 973–981 CrossRef CAS.
- Y. V. Budovskaya, J. S. Stephan, S. J. Deminoff and P. K. Herman, Proc. Natl. Acad. Sci. U. S. A., 2005, 102, 13933–13938 CrossRef CAS.
- V. D. Longo, Exp. Gerontol., 2003, 38, 807–811 CrossRef CAS.
- T. Toda, S. Cameron, P. Sass, M. Zoller and M. Wigler, Cell, 1987, 50, 277–287 CrossRef CAS.
- K. E. Johnson, S. Cameron, T. Toda, M. Wigler and M. J. Zoller, J. Biol. Chem., 1987, 262, 8636–8642 CAS.
- P. Portela, P. Van Dijck, J. M. Thevelein and S. Moreno, FEMS Yeast Res., 2003, 3, 119–126 CAS.
- A. Soulard, A. Cremonesi, S. Moes, F. Schutz, P. Jeno and M. N. Hall, Mol. Biol. Cell, 2010, 21, 3475–3486 CrossRef CAS.
- S. Bandyopadhyay, M. Mehta, D. Kuo, M. K. Sung, R. Chuang, E. J. Jaehnig, B. Bodenmiller, K. Licon, W. Copeland, M. Shales, D. Fiedler, J. Dutkowski, A. Guenole, H. van Attikum, K. M. Shokat, R. D. Kolodner, W. K. Huh, R. Aebersold, M. C. Keogh, N. J. Krogan and T. Ideker, Science, 2010, 330, 1385–1389 CrossRef CAS.
- J. S. Searle, M. D. Wood, M. Kaur, D. V. Tobin and Y. Sanchez, PLoS Genet., 2011, 7, e1002176 CAS.
- P. S. Ma, S. Wera, P. Van Dijck and J. M. Thevelein, Mol. Biol. Cell, 1999, 10, 91–104 CAS.
- U. Schlecht, M. Miranda, S. Suresh, R. W. Davis and R. P. St Onge, Proc. Natl. Acad. Sci. U. S. A., 2012, 109, 9213–9218 CrossRef CAS.
- E. Stefan, S. Aquin, N. Berger, C. R. Landry, B. Nyfeler, M. Bouvier and S. W. Michnick, Proc. Natl. Acad. Sci. U. S. A., 2007, 104, 16916–16921 CrossRef CAS.
- The R Project for Statistical Computing, http://www.r-project.org/.
-
D. A. Treco and F. Winston, Current protocols in molecular biology, 2008, Ch. 13, Unit 13.12-Unit 13.12 Search PubMed.
-
D. C. Amberg, D. J. Burke and J. N. Strathern, Methods in yeast genetics, Cold Spring Harbor Laboratory Press, 2005 Search PubMed.
- J. A. Hill and S. P. Otto, Genetics, 2007, 175, 1419–1427 CrossRef.
- P. H. Ear and S. W. Michnick, Nat. Methods, 2009, 6, 813–816 CrossRef CAS.
- C. H. Ho, L. Magtanong, S. L. Barker, D. Gresham, S. Nishimura, P. Natarajan, J. L. Y. Koh, J. Porter, C. A. Gray, R. J. Andersen, G. Giaever, C. Nislow, B. Andrews, D. Botstein, T. R. Graham, M. Yoshida and C. Boone, Nat. Biotechnol., 2009, 27, 369–377 CrossRef CAS.
- E. A. Winzeler, D. D. Shoemaker, A. Astromoff, H. Liang, K. Anderson, B. Andre, R. Bangham, R. Benito, J. D. Boeke, H. Bussey, A. M. Chu, C. Connelly, K. Davis, F. Dietrich, S. W. Dow, M. EL Bakkoury, F. Foury, S. H. Friend, E. Gentalen, G. Giaever, J. H. Hegemann, T. Jones, M. Laub, H. Liao, N. Liebundguth, D. J. Lockhart, A. Lucau-Danila, M. Lussier, N. M'Rabet, P. Menard, M. Mittmann, C. Pai, C. Rebischung, J. L. Revuelta, L. Riles, C. J. Roberts, P. Ross-MacDonald, B. Scherens, M. Snyder, S. Sookhai-Mahadeo, R. K. Storms, S. Veronneau, M. Voet, G. Volckaert, T. R. Ward, R. Wysocki, G. S. Yen, K. X. Yu, K. Zimmermann, P. Philippsen, M. Johnston and R. W. Davis, Science, 1999, 285, 901–906 CrossRef CAS.
- C. Janke, M. M. Magiera, N. Rathfelder, C. Taxis, S. Reber, H. Maekawa, A. Moreno-Borchart, G. Doenges, E. Schwob, E. Schiebel and M. Knop, Yeast, 2004, 21, 947–962 CrossRef CAS.
- ImageJ, http://rsbweb.nih.gov/ij/.
Footnotes |
† Electronic supplementary information (ESI) available: Supplementary tables and figures. See DOI: 10.1039/c2mb25265a |
‡ These authors equally contributed to this work. |
|
This journal is © The Royal Society of Chemistry 2013 |
Click here to see how this site uses Cookies. View our privacy policy here.