A multi-omic systems approach to elucidating Yersinia virulence mechanisms†
Received 18th July 2012, Accepted 9th October 2012
First published on 24th October 2012
Abstract
The underlying mechanisms that lead to dramatic differences between closely related pathogens are not always readily apparent. For example, the genomes of Yersinia pestis (YP) the causative agent of plague with a high mortality rate and Yersinia pseudotuberculosis (YPT) an enteric pathogen with a modest mortality rate are highly similar with some species specific differences; however the molecular causes of their distinct clinical outcomes remain poorly understood. In this study, a temporal multi-omic analysis of YP and YPT at physiologically relevant temperatures was performed to gain insights into how an acute and highly lethal bacterial pathogen, YP, differs from its less virulent progenitor, YPT. This analysis revealed higher gene and protein expression levels of conserved major virulence factors in YP relative to YPT, including the Yop virulon and the pH6 antigen. This suggests that adaptation in the regulatory architecture, in addition to the presence of unique genetic material, may contribute to the increased pathogenecity of YP relative to YPT. Additionally, global transcriptome and proteome responses of YP and YPT revealed conserved post-transcriptional control of metabolism and the translational machinery including the modulation of glutamate levels in Yersiniae. Finally, the omics data was coupled with a computational network analysis, allowing an efficient prediction of novel Yersinia virulence factors based on gene and protein expression patterns.
Introduction
Yersinia pestis (YP) is a Gram-negative bacterium and the causative agent of plague, an acute and lethal disease responsible for at least three human pandemics that resulted in an estimated 200 million deaths.1 Several lines of evidence suggest that YP has evolved from the gastrointestinal pathogen Y. pseudotuberculosis (YPT) within the last 20
000 years.2 Genomic analyses show YP and YPT to be genetically similar (∼97% identity at the nucleotide level3), yet despite their close genetic relationship, the bacteria exhibit markedly different pathogenecities and modes of transmission.4 YPT causes non-fatal gastrointestinal disease and is transmitted via the fecal oral route, while YP is the causative agent of typically fatal plague and is transmitted via flea bite. Based both on the ability to ferment glycerol and to reduce nitrate, YP strains have traditionally been assigned to one of three biovars: antiqua, medievalis, and orientalis.1 Recently, the new biovar microtus has been identified on the basis of unique pathogenic, biochemical, and molecular features.5 In laboratory studies, microtus strains (also known as Pestoides) are lethal to microtus species (voles), mice, and some other small rodents, however they are avirulent in humans and larger mammals. Whereas antiqua, medievalis, and orientalis biovars cause disease in humans (i.e. epidemic strains), there is no evidence that human plague can be caused by Pestoides (i.e. non-epidemic) strains.6The availability of genome sequences for several Yersinia strains, including YPT and both epidemic and non-epidemic YP variants, has provided an opportunity to explore mechanisms responsible for the differences in pathogenicity. Comparative genomic analyses revealed all human pathogenic Yersinia strains, including YP and YPT share almost identical ∼70 kb virulence plasmids that are essential for virulence.7 This plasmid (pCD1 in YP) encodes two major types of virulence factors: (i) the Yersinia outer proteins (Yops) and V antigen and (ii) the type three secretion system (T3SS) apparatus which is required to translocate Yop effector proteins to the host cytoplasm to modulate host cell function and promote disease progression.8 Additionally, comparison of the genomes of YP and its progenitor YPT reveal a modest number of species-specific chromosomal genes as well as the presence of two plasmids (pMT1 and pCP1) specific to YP that are thought to contribute to YP pathogenesis.1,3,9–14 The pMT1 plasmid harbors genes coding for the capsular antigen F1 and murine toxin, while the pPCP1 plasmid encodes the plasminogen activator. Importantly, these species-specific attributes cannot fully account for the marked difference in pathogenecity between YP and YPT.9,15–21 One hypothesis is that the differential expression of genes common to both organisms, in addition to overt genetic differences, is an important contributing factor to the different pathogenicities and clinical outcomes of YP and YPT.
In this study we have performed a systems level multi-omic analysis of YP CO92 (YPCO) and YPT PB1/+ (YPTS) to gain an understanding as to how an acute and highly lethal bacterial pathogen, such as YP differs phenotypical from its less virulent progenitor YPT. We also compare YP CO92 (YPCO) to the non-epidemic YP strain Pestoides F (YPPF) to provide insights to the mechanism(s) underlying the virulence-restricted phenotype of non-epidemic YP strains compared to epidemic YP strains. The parallel sample-matched transcriptomics and proteomics analysis of multiple pathogenic Yersinia strains in a single study allows for the prediction of genes putatively involved in core pathogenic processes important for virulence mechanisms of Yersinia species. In the present work, cells were grown in a chemically defined medium (pH 7.2) at physiologically relevant temperatures (representative of flea vector and mammalian host environments) and sampled through an 8 hour time course. Transcription was analyzed using a multi-genome microarray and protein and metabolite levels were analyzed by mass spectrometric methods. This experimental design offered the advantage of revealing both transcriptional and post-transcriptional responses to a temperature shift simulating the host–Yersinia interaction through a time course that simulates the progression of a mammalian infection. The data suggests that adaptation in the regulatory architecture, in addition to the presence of unique genetic material, may contribute to the increased pathogenenicity of YP relative to YPT; and also revealed conserved post-transcriptional control of metabolism and the translational machinery in Yersiniae.
Experimental
Reagents
The following reagents were used in sample preparation: Nanopure or Milli-Q quality water (∼18 megohm cm or better); ammonium bicarbonate (NH4HCO3); bicinchoninic acid (BCA) or coomassie protein assay reagents (Pierce, Rockford, IL); urea; thiourea; dithiothreitol (DTT); 3-((3-cholamidopropyl)dimethylammonio)-1-propanesulfonate(CHAPS); calcium chloride; sequencing-grade modified trypsin (Promega); HPLC-grade methanol (MeOH); trifluoroacetic acid (TFA); acetonitrile (ACN); ammonium formate; formic acid; and ammonium hydroxide (NH4OH). All reagents were obtained from Sigma Aldrich (St. Louis, MO) unless otherwise specified.Bacterial strains and culture conditions
Y. pseudotuberculosis PB1/+ (YPTS), Yersinia pestis Pestoides F (YPPF), and a wild-type Yersinia pestis CO92 (YPCO) cured of the pPCP1 plasmid were grown in a chemically defined BCS medium22 in which neutral pH 7.2 was maintained by addition of 50 mM morpholinopropanesulfonic acid (MOPS) as described previously.22,23 Bacterial cultures were grown in Erlenmeyer flasks aerated at 200 rpm at 26 °C or 28 °C. Briefly, a starter culture was grown, diluted to optical density OD600 = 0.1 to begin overnight culture, and grown to an OD600 of ∼3.0. The overnight culture was diluted to OD600 = 0.1 and grown in two flasks at 26 °C. When the cultures reached OD600 ∼0.5, one flask was moved to 37 °C. Similarly cultures grown at 28 °C were shifted to 37 °C. Aliquots from both cultures 26 °C/28 °C and 37 °C were taken at 0, 1, 2, 4, and 8 hours, optical densities measured, and samples prepared as described below for transcriptomic, proteomic, and metabolomic analyses. The different low temperatures were used due to biofilm formation of YPCO at 26 °C and more ideal suspension culturing at 28 °C, results were largely similar for the purposes of our analyses.Transcriptomics
RNA preparation and microarray analysis of transcripts were performed as previously described in ref. 24.Proteomics analysis
Approximately 2 × 1010 bacteria were harvested from the cultures at each time point, pelleted, and immediately frozen at −80 °C. Thawed cell pellets were washed with 100 mM NH4HCO3 (pH 8), lysed via bead beating, and soluble and insoluble protein digestions were performed as described previously.25 Peptides were concentrated in a Speed-Vac (ThermoFisher, Savant) to ∼100 μL, and a BCA protein assay was performed to quantify peptide concentration prior to analysis.Peptide samples were analyzed using the accurate mass and elution time (AMT) tag approach,26 which is enabled by a number of published and in-house tools available for download at omics.pnl.gov27–31 We note that the scale of the experiment in which 24 comparisons within a single MS experiment are being considered (i.e. 3 strains × 2 temperatures × 4 time points) guided our choice of label-free intensity based quantification. Briefly, a reference database of AMT tags for peptides from all three Yersinia strains employed in this study was previously generated through exhaustive 2-dimensional LC-MS/MS analyses, as described,24 and was augmented with additional peptides identified in the LC-MS/MS analyses described here. Samples were blocked and randomized to minimize the effects of systematic biases and ensure the even distribution of known and unknown confounding factors across the entire experiment. Peptides from each of the soluble and insoluble protein preparations were analyzed in triplicate using an custom built capillary LC system32 coupled with an LTQ-Orbitrap mass spectrometer (Thermo Fisher Scientific, San Jose, CA) via an in-house manufactured electrospray ionization interface, as previously described.33 RAW files for these datasets are available at http://omics.pnl.gov and at www.Sysbep.org. The LC elution time and monoisotopic mass (determined using the charge state and high accuracy mass measurement) of each peptide feature observed in the analysis were matched to entries within the AMT tag database using the in-house STAC algorithm,34 which calculates a probability of match. The integrated areas under the elution profiles were used as measures of peptide abundances. Each peptide included for subsequent data analysis was observed in at least one LC-MS analysis with a probability of a correct match being 0.9 and matches for the same peptide in the remaining LC-MS analyses were required to have a minimum probability of 0.5. In addition, at least two unique peptides were required per protein identification. The software program DAnTE35 was employed to perform an abundance roll-up procedure to convert peptide information to protein information, thereby inferring protein abundances.
Metabolomics analysis
For 1H nuclear magnetic resonance (NMR) analysis, 540 μL of spent media were added to 60 μL of 5 mM 2,2-dimethyl-2-silapentane-5-sulfonate (DSS) in 99.9% deuterium oxide (D2O) in 5 mm NMR tubes. DSS is used as an internal standard and to provide a 1H chemical shift reference at δ 0.00 ppm. 1H NMR spectra were acquired on a Varian INOVA-600 MHz NMR spectrometer (Varian Inc., Palo Alto, CA) at 298 K, using a triple resonance 5 mm HCN salt-tolerant cold probe. A one-dimensional NOE pulse sequence adapted from the two-dimensional Varian tnnoesy was used. For each sample, 96 to 512 transients were collected into 64 K data points using a spectral width of 7225.4 Hz with a relaxation delay of 1.0 s, an acquisition time of 4.00 s, and a mixing time of 100 ms. Spectra were processed using Chenomx 6.1. A 0.5 Hz line-broadening function was applied to all spectra prior to Fourier transformation (FT) and baseline correction. The profiler module of Chenomx was used to identify and quantify metabolites.For GC-MS analysis, metabolites were extracted from the cell culture suspensions using four volumes of chilled (−20 °C) chloroform/methanol (2
:
1, v/v). The aqueous layer obtained after centrifugation (12
000 × g, 5 min) was transferred to a new vial and dried in vacuo. All metabolite extracts were then subjected to chemical derivatization to enhance metabolite stability and volatility during analysis.36 Briefly, 20 μL of methoxyamine in pyridine (30 mg mL−1) was added to each dried sample, followed by incubation at 37 °C with shaking (1000 rpm) for 90 min. Next, 80 μL of N-methyl-N-(trimethylsilyl)trifluoroacetamide (MSTFA) containing 1% trimethylchlorosilane (TMCS) was added to each vial, followed by incubation at 37 °C with shaking (1000 rpm) for 30 min. The incubated samples were allowed to cool to room temperature and were then analyzed by GC-MS. The GC-MS system consisted of a 7890A GC-coupled with a single quadrupole MSD 5975C (Agilent Technologies, Santa Clara, CA), and separations were performed on a DB-5MS column (30 m × 0.25 mm × 0.25 μm; Agilent Technologies). The injection mode was splitless, and 1 μL of each sample was injected. The injection port temperature was held at 250 °C throughout the analysis. The GC oven was initially maintained at 60 °C for 1 min and then ramped to 325 °C at 10 °C min−1, followed by a 5 min hold at 325 °C.37 The obtained GC-MS raw data files were processed by MetaboliteDetector.38 Retention indices (RI) were calculated based on the analysis of a mixture of fatty acid methyl esters (FAMEs; C8–C30), and this information was subsequently used to align the retention times of metabolite features detected across GC-MS chromatograms. The chromatographically aligned features were identified using a database, containing mass spectra and retention indices for approximately 700 metabolites.37
Network inference
For network inference analysis transcriptomics and proteomics data from the “ambient” temperature (26 °C and 28 °C) samples and mammalian host temperature (37 °C) samples were used (Table S1, ESI†) to enhance robustness of the network predictions. Transcriptomics and proteomics data were log2 transformed as the ratio of 37 °C to 26 °C samples, or 37 °C to 28 °C samples. Missing values in proteomics data were dealt with as follows: proteins for which there were observations for only one strain were removed. Of the remaining 933 proteins, 670 had a complete complement of data values for all strains and conditions. For the remaining 263, if values were missing for all samples in a single strain, it was assumed the protein was not present in that strain and zeros were filled in, representing a non-changing sample for that protein. In many cases, proteins were detected across all time points at one temperature (in a given strain) but absent at another. For these scenarios, it was assumed the differential in temperature was causing the protein level to fall below the level of detection, and the minimum value for the entire data table was filled in. For instances when only 1 time point was missing for a temperature series, the value from the next earlier time point was filled in. If the missing value was from the first time point, the second time point was filled in. All other missing values were filled in with the minimum value of the entire data table.To determine relationships between transcripts and proteins we used an approach to infer a separate coexpression network for each dataset. To infer edges between network elements, we used context likelihood of relatedness (CLR), an inference algorithm which determines similarity between gene expression profiles based on mutual information between the profiles, and then scores the relationships using a Z-score.39 For each network we used default parameters for inference using 10 bins for binning data and 3 splines for curve fitting (see ref. 39 for details).
Thresholds for considering a relationship to be an edge in a network were chosen to minimize false negatives and false positives. Interestingly, Z-scores for proteomics were noticeably lower than for transcriptomics (proteomics mean positive Z-score = 0.67, transcriptomics mean positive Z-score = 1.04) such that a lower percentage of proteomic edges were preserved than transcriptomic edges at any given cutoff. For this reason, we elected to apply differential Z-score thresholds for transcriptomics (5.0) and proteomics (3.0), yielding 3823 transcriptomic edges and 607 proteomics edges, which is a similar ratio to that of transcriptomic and proteomic entities remaining after the initial filtering process (4020/929). Since locus ID tags were common to both datasets, edgefiles for each were simply merged and redundant edges removed, forming a new integrated network. The full network is provided in a single Cytoscape40 session file as Supplemental Data.
To find clusters in the network, we used the Louvain community-finding algorithm,41 which maximizes modularity between communities and returns the corresponding cluster membership. All clusters with a membership of 10 proteins or more were analyzed for enrichment of functional categories using gene ontology. These functional clusters were visualized using the Cytoscape graph visualization package.40
Results and discussion
Experimental design and omics data generation
To elucidate mechanisms that contribute to the difference in pathogenicity between YP and YPT, high throughput “omics” technologies were used to analyze YP CO92 (YPCO), YPT PB1/+ (YPTS) and YP Pestoides F (YPPF) cells sampled through a time course of 8 hours (1, 2, 4, and 8 h) post-temperature shift from flea vector (26 °C/28 °C) to mammalian host (37 °C) temperature. YPTS typically causes a mild infection in mammals and birds. The microbe is capable of a saprophytic life style, and thus alternates between the natural environment and warm blooded hosts. The non-epidemic YPPF circulates within natural plague reservoirs and is transmitted between common voles by the fleas, similar to epidemic strains of Y. pestis (i.e. YPCO). Thus, a temperature shift model is relevant to all three Yersinia used in our studies. Transcriptomics analyses were performed utilizing a Yersinae multi-genome microarray which has been previously described.24 Unlike a traditional microarray that targets annotated genes for a single strain, this microarray incorporates 7641 unique probes designed against seven sequenced Yersinia strains on a single chip, including the three strains examined in this study. Proteomics analyses were performed using capillary LC-MS combined with the accurate mass and time (AMT) tag approach.26 Overall, microarray-based transcriptomics analyses quantified 5005 genes, and mass spectrometry-based proteomics analyses quantified 1365 proteins across all conditions examined. The data are summarized in Table S1 (ESI†) and provided as a resource for the community (www.sysbep.org). This study focused on the response to temperature shift from flea vector temperature 28 °C to mammalian host temperature 37 °C, represented as log2 transformed ratios of expression at 37 °C relative to 28 °C, unless indicated in the text.Comparative omics analysis of Y. pestis and Y. pseudotuberculosis
While YP and YPT have been shown to be very similar genetically (∼97% identity at nucleotide level),3 the bacteria exhibit markedly different pathogenicities.4 The molecular mechanism(s) underlying the acute character of the plague infection caused by YP in the mammalian host is poorly understood. We hypothesized that differential expression of genes common to both organisms, in addition to overt genetic differences contributes to their significantly different pathogenicities in the mammalian host. To test this hypothesis, thermal-shift transcript and protein profiles of YP CO92 (YPCO) and YPT PB1/+ (YPTS) were analyzed.From our analysis, we identified 70 genes preferentially up-regulated in YPCO relative to YPTS following a shift from flea vector to mammalian host temperature, suggesting a potential functional role in the mammalian host context. Examination of these 70 genes revealed that 66 genes were located on the pCD1 plasmid, which is essential for virulence in all human pathogenic Yersinia strains, with 44 genes annotated as encoding Yops or components of the T3SS (Fig. S1 and Table S2, ESI†). These included secretion apparatus members, chaperones, effectors and low calcium response genes. In agreement with the transcriptional data, all pCD1-encoded Yops and T3SS proteins detected by proteomics were preferentially up-regulated in YPCO relative to YPTS in response to temperature elevation (Fig. 1). In fact, protein expression was not detected for any of these proteins in YPTS across all time points. While it is possible that these proteins were simply not detected due to the stochastic nature of MS-based proteomics analysis methods, a more likely explanation is low protein expression levels in YPTS that fall beneath the detection level of the instruments employed for analysis. YadA is a factor important for YPT pathogenesis but thought to be either absent or non-functional in YP.42 Here our microarray data shows detection of YadA transcripts in both YPCO and YPTS suggesting that expression of this pseudogene does occur in YP (Fig. 1). Examination of the corresponding proteomics data however shows presence of YadA protein in YPTS alone but not YPCO consistent with it being non-functional in YP (Fig. 1). Taken together these observations suggest a role for post-transcriptional regulatory mechanisms in modulating the genotype to phenotype expression of YadA in Yersiniae.
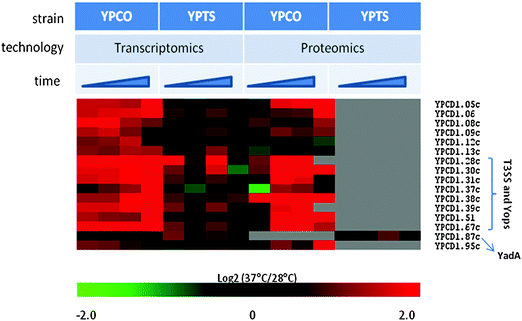 |
| Fig. 1 Heat map illustration of pCD1-encoded proteins, detected by both proteomics and transcriptomics, preferentially up-regulated in YPCO relative to YPTS in response to temperature elevation. Time represents sampling points at 1 h, 2 h, 4 h, 8 h. YPCO, Yersinia pestis CO92; YPTS, Yersinia pseudotuberculosis PB1/+. | |
Three of the four chromosomally-encoded genes preferentially up-regulated in YPCO relative to YPTS following temperature increase are required for the assembly of a functional pH6 antigen (psaABC; Fig. S1, ESI†). The fourth chromosomally-encoded gene is an acid resistance membrane protein (YPO0590). The pH6 antigen (Psa) is conserved across pathogenic Yersinia species and is thought to contribute to both YP and YPT virulence in the mammalian host. In YPT, Psa was reported to be a thermoinducible adhesin that allows binding of the organism to cultured mammalian epithelial cells.43 In YP a psa deletion mutant strain was shown to be attenuated by the intravenous route of infection44 and more recently Psa was shown to promote resistance to phagocytosis further clarifying its role in virulence.45 Taken together, these observations suggest that the increased pathogenicity of YP relative to YPT is not only due to genomic differences, but stems from differences in transcriptional regulatory networks resulting in higher transcript and protein expression of common essential virulence mechanisms.
These observations encouraged examination of the extent that differential expression of other major virulence mechanisms conserved across pathogenic Yersinia species contribute to observed differences in pathogenicity. The high pathogenicity island (HPI)-encoded ybt locus is conserved across pathogenic Yersinia spp with 97–100% identity and is comprised of genes required for the biosynthesis and secretion of yersiniabactin, a siderophore that is essential for Yersinia virulence.46 Transcripts of the genes encoding yersiniabactin biosynthetic proteins (YPO1907-1911), as well as the transcript for the yersiniabactin receptor (YPO1906), were down-regulated following temperature shift in YPCO compared to YPTS (Fig. S2, ESI†). The ferric uptake regulator (Fur) negatively regulates transcription of ybt genes while transcriptional regulator YbtA positively regulates transcription of ybt genes.47 No differences between YPCO and YPTS transcript and protein levels were observed for Fur. Similarly no differences between YPCO and YPTS transcript levels were observed for YbtA. YbtA protein expression was not detected in either organism. Taken together these results suggest that regulation of yersiniabactin biosynthesis and secretion is different in YPCO and YPTS and likely comprises additional yet unrecognized levels of transcriptional or post-transcriptional control that contribute to the differences in pathogenesis between these strains. This is supported by previous work showing that an inactivating mutation in the yersiniabactin receptor YPO1906 causes loss of siderophore production in YPT, but not in YP.48
Comparative omics of Y. pestis CO92 and Y. pestis Pestoides F
We compared YP CO92 (YPCO) to the non-epidemic YP strain Pestoides F (YPPF) to gain insights to the mechanism underlying the virulence-restricted phenotype of non-epidemic YP strains compared to epidemic YP strains. From our analysis, we identified 66 genes preferentially expressed in one organism or the other in response to temperature shift (Table S3, ESI†) as judged by clustering analysis. A majority of these genes, 51, exhibited a coordinated down-regulation of transcript and protein levels following a shift from flea vector temperature to mammalian host temperature suggesting a potential functional role in the flea vector context. The remaining 15 genes were up-regulated following temperature shift in this case, suggesting a potential functional role in the mammalian host context. All 15 genes that were up-regulated following temperature shift were preferentially expressed in YPCO relative to YPPF, with 11 of the 15 genes encoded on the pMT1 virulence plasmid. The pMT1 plasmid is a 100 kb YP-specific plasmid that encodes approximately 100 genes, including the two well characterized virulence factors F1 capsule protein (Caf1) and Yersinia murine toxin (Ymt). Ymt is a phospholipase D (PLD) shown to be required for survival of YP in the midgut of the flea but not required for virulence in mice.12,49 Caf1 was previously demonstrated to be an anti-phagocytic factor that inhibits the internalization of YP by macrophages,9 and additionally promotes transmission by flea bite, increasing the potential for epidemic spread.50 More recent results suggest that Caf1 is required for pathogenesis during bubonic and pneumonic infections.51 It is clear that acquisition of the pMT1 plasmid, and thus the ymt and caf loci, enhanced YP potential for epidemic spread. It is currently unknown but likely that additional virulence determinants are encoded on the pMT1 virulence plasmid. The 11 pMT1 plasmid encoded genes preferentially expressed in YPCO relative YPPF warrant further investigation as potential molecular switches for the epidemic versus non-epidemic phenotypes of YPCO and YPPF.Multi-omics integration for network prediction of putative virulence factors
While the identification of factors that contribute to species-specific pathogenic attributes of YP and YPT is important, equally important is the elucidation of the full repertoire of determinants that play a role in core pathogenic processes required for the virulence mechanism of pathogenic Yersinia spp. Here the context likelihood of relatedness (CLR) algorithm39 was employed to integrate the proteomics and transcriptomics datasets and predict proteins important for Yersiniae pathogenesis in an efficient and systematic manner. Since proteomic expression profiles are often poorly correlated with transcriptomic profiles, there is likely additional regulatory information in the combined results. The value added of an integrated approach, is therefore the incorporation of potential impacts of post-transcriptional regulation on the network analysis. The robustness of the predictions was enhanced by the incorporation of omics data from bacteria grown at both flea vector temperatures, 26 °C and 28 °C, and at the mammalian host temperature (37 °C) (Table S1, ESI†). The proteomics and transcriptomics datasets were integrated at the network level to generate a multi-omics network, and subsequent functional enrichment analyses were used to predict proteins important for pathogenesis as described below. A similar approach was previously employed to predict novel virulence factors in the related pathogen Salmonella Typhimurium which were subsequently verified experimentally.52The proteomic and transcriptomic networks were combined under specific thresholds to create an integrated multi-omics network including only high confidence protein to protein (Z score > 3.0) and transcript to transcript (Z score > 5.0) relationships (see the Materials and Methods section for the fractional contribution of transcriptomics and proteomics). The network was partitioned using a community-finding algorithm,41 and each of the resulting clusters was analyzed for enrichment of functional categories using gene ontology (GO) analysis. Among the most significantly enriched functional clusters was the cluster labeled ‘Type III secretion’ as shown in Fig. 2. To predict proteins potentially important for Yersinia pathogenesis, further analysis was focused on this cluster because of the established characterization of the Type III secretion system (T3SS) as a major Yersinia virulence factor, and proteins co-regulated with it across multiple organisms, conditions and time points are likely to play a role in Yersinia pathogenesis.
 |
| Fig. 2 Association network inferred from integrated proteomic and transcriptomic data. The CLR method was used to infer association relationships between proteins on the basis of their abundance profiles (Z score > 3.0). The resulting network was extended by combining with association relationships inferred from transcriptomics data (Z score > 5.0). The network was visualized in Cytoscape. Examples of significantly enriched functional clusters are indicated in the figure. | |
Examination of the T3SS-associated functional cluster revealed 151 cluster members (Table S4, ESI†), with 34 members encoded on the pCD1 virulence plasmid, including 28 annotated as members of the Yop virulon. Fifty three cluster members are encoded on the pMT1 plasmid including the major virulence determinants Yersinia murine toxin (Ymt) and the F1 capsule protein (Caf1). Sixty four cluster members are chromosomally-encoded, the majority of which are annotated with unknown function. Among the subset of chromosome-encoded cluster members were several proteins that have been suggested to play a role in pathogenesis in Yersinia including PgaA, HmsR, Ail, Asr, RpoS, and NlpD. For example, the lipoprotein NlpD was recently shown to be a novel Yersinia pestis virulence factor.53 Chromosomal deletion of the nlpD gene sequence resulted in a drastic reduction in virulence to an LD50 of at least 107 cfu for subcutaneous and airway routes of infection, and the mutant was unable to colonize mouse organs following infection.53 Given the very high enrichment of virulence-related proteins and proteins important for adaptation to the host environment in this cluster, the remaining uncharacterized members of this cluster are predicted to be enriched for proteins important for Yersinia pathogenesis.
Comparison of omics datasets across multiple Yersinia strains reveals conserved biological processes under post-transcriptional control
In most instances, gene expression in YPCO relative to YPTS or YPPF was confirmed by the proteomics data if the protein of interest was confidently detected. However, this analysis also revealed a number of cases where changes in protein expression were in contrast with the observations from the transcriptional analysis (i.e. transcript appeared unaffected by the temperature shift, while protein displayed substantial change), highlighting the importance of proteomics in addition to transcriptional analyses.Typically, disparities between transcript and protein measurements are often attributed to post-transcriptional regulation,54,55 thus these observations prompted examination of instances of apparent post-transcriptional regulation in response to temperature switch on a global scale. First, transcript and protein responses to temperature shifts were considered for each gene at the same time-point within each of the three organisms by calculating Pearson correlations. A general trend of improved correlation was observed between transcript and protein over time across all three organisms. For example, in the case of YPCO transcript-protein correlations of 0.28, 0.55, 0.65, and 0.53 were observed at 1 h, 2 h, 4 h, and 8 h respectively (Fig. S3, ESI†). It is possible that this general trend of improved correlation may be explained by adaptation to new environmental conditions as the later time points reach a steady-state with only subtle changes in macromolecules needed. This is in contrast to the 1 h time point during which there appears to be a vigorous dynamic adaptation in response to temperature shift occurring, necessitating substantial transcription and translation of newly required biomolecules.
While low transcript-protein correlation is typically interpreted as evidence of post-transcriptional regulation, it is very likely that temporal lags between dynamic changes in transcription and translation at the level of individual genes also represent an important contribution to the observed low correlation; although this has rarely been demonstrated as most studies reporting low correlation have been single time-point studies. The temporal sample-matched global transcript and protein datasets presented here allow us to begin to evaluate this in part with regards to temporal lag on a genome-scale. If the assertion that temporal lags between dynamic changes in transcription and translation represent an important contribution to the low transcript-protein correlations observed is correct, then an improvement in transcript-protein correlation would be expected as a time lag is introduced in analysis of the correlation of the temporal transcript to the protein data. Indeed this was observed across all three strains when transcript and protein response to temperature shift were compared by calculating Pearson correlations with and without a 1h time lag (Fig. S4, ESI†). For YPCO a transcript-protein correlation improvement of 0.28 to 0.57 was observed. Similarly for YPTS and YPPF a transcript-protein correlation improvements of 0.49 and 0.33 to 0.56 and 0.62, respectively, are observed.
Considering the improvement in global transcript-protein correlations associated with time, genome-scale instances of apparent post-transcriptional regulation in response to temperature shift were examined by performing an ANOVA analysis. The goal of this analysis was to identify genes with transcript response significantly different from protein response to temperature shift across all time points. The ANOVA analysis identified 173 YPCO genes with a significant difference (p < 0.05) between mRNA and proteins responses to temperature shift across all time points, with similar numbers of differences for YPPF (194) and YPTS (128) (Fig. 3 and Table S5, ESI†). The Database for Annotation, Visualization and Integrated Discovery (DAVID)56 was used to identify enriched functionally-related genes representing particular biological processes from each of these 3 different gene lists with the following criteria: Benjamini–Hochberg corrected p-value <0.05 and gene count ≥5. Biological processes functionally enriched in the list of genes are shown in Table 1. Observing apparent post-transcriptional control of biological processes across multiple organisms increases the confidence in their assignment. Thus the data were examined for those inferred biological processes under potential post-transcriptional control that were conserved across at least two of the three Yersiniae under investigation (see Table 1; bolded text). Among these post-transcriptional controlled proteins were purine metabolism, pyrimidine metabolism, and amino-acyl tRNA biosynthesis which showed conservation across all three organisms. Pyruvate metabolism and glycolysis/gluconeogenesis were conserved across YPCO and YPPF while ribosomes were conserved across YPPF and YPTS. Interestingly, previous work in the bacterial pathogen Salmonella Typhimurium has also suggested that general metabolism (including purine and pyrimidine metabolism, glycolysis/gluconeogenesis, the TCA pathway, and pyruvate metabolism) and the translational machinery (including aminoacyl-tRNA synthetases) are under post-transcriptional control, mediated to a large extent by the global post-transcriptional regulator Hfq which is required for Salmonella virulence.57–59 Post-transcriptional regulation of general metabolism and the translational machinery, among other processes, is speculated to similarly play an important role in Yersinia adaptation to the mammalian host intracellular environment and in pathogenesis. Indeed the global post-transcriptional regulator Hfq has been shown to be required for virulence in Yersinia.60,61
 |
| Fig. 3 Heat map illustration of genes with transcript response to temperature shift across all time points significantly different from protein response to temperature shift across all time points as determined by ANOVA analysis (p < 0.05) for each organism. Time represents sampling points at 1 h, 2 h, 4 h, 8 h. YPCO, Yersinia pestis CO92; YPTS, Yersinia pseudotuberculosis PB1/+; YPPF, Yersinia pestis Pestoides F. | |
Table 1 Post-transcriptionally regulated biological process. Biological processes functionally enriched in the list of genes with a significant difference (p < 0.05) between mRNA and proteins responses to temperature shift across all time points for YPCO, YPPF and YPTS. Bolded text indicates biological process conserved across at least two of the three Yersiniae strains. YPCO, Yersinia pestis CO92; YPTS, Yersinia pseudotuberculosis PB1/+; YPPF, Yersinia pestis Pestoides F
Organism | Biological process | Gene count | Fold enrichment | Benjamini P value |
---|
YPCO | Pyrimidine metabolism | 11 | 13 | 1.47 × 10−06 |
Purine metabolism | 9 | 7 | 1.70 × 10−03 |
Aminoacyl-tRNA biosynthesis | 6 | 13 | 3.12 × 10−03 |
Glycolysis/gluconeogenesis | 6 | 12 | 4.06 × 10−03 |
Pyruvate metabolism | 6 | 10 | 4.72 × 10−03 |
Citrate cycle (TCA cycle) | 5 | 11 | 1.46 × 10−02 |
Glutathione metabolism | 4 | 16 | 2.55 × 10−02 |
Fatty acid biosynthesis | 4 | 15 | 2.97 × 10−02 |
Arginine and proline metabolism | 5 | 8 | 4.82 × 10−02 |
YPPF | Ribosome | 12 | 9 | 3.44 × 10−06 |
Pyruvate metabolism | 9 | 15 | 3.04 × 10−06 |
Purine metabolism | 9 | 7 | 9.27 × 10−04 |
Aminoacyl-tRNA biosynthesis | 6 | 13 | 1.79 × 10−03 |
Glycolysis/gluconeogenesis | 6 | 12 | 2.58 × 10−03 |
Pyrimidine metabolism | 7 | 8 | 2.91 × 10−03 |
Alanine, aspartate and glutamate metabolism | 5 | 11 | 1.40 × 10−02 |
Glyoxylate and dicarboxylate metabolism | 4 | 13 | 3.16 × 10−02 |
YPTS | Ribosome | 13 | 13 | 6.44 × 10−09 |
Purine metabolism | 9 | 9 | 1.15 × 10−04 |
Pyrimidine metabolism | 7 | 11 | 7.38 × 10−04 |
Peptidoglycan biosynthesis | 5 | 18 | 1.91 × 10−03 |
Aminoacyl-tRNA biosynthesis | 5 | 14 | 4.85 × 10−03 |
Metabolomics analyses suggest post-translational control of glutamate levels
Advances in mass spectrometry and nuclear magnetic resonance (NMR) techniques in the past decade now allow for global, high throughput profiling of metabolites produced by bacteria. Here, NMR was used to analyze spent media samples derived from two YP strains (YPCO and YPPF) and the YPT strain PB1/+ (YPTS) grown at the flea vector and mammalian host temperatures and sampled at 4 h post-culturing. These samples were prepared from the cultures used for transcriptomics and proteomics analyses. A striking observation from this analysis was the difference in glutamate concentrations across the three strains. Glutamate concentration in the YPCO sample was significantly higher than in either YPTS or YPPF samples (data not shown). As an orthogonal measure of the observed differences in this metabolite across the three strains, whole cell cultures of YPCO, YPPF and YPTS (grown at both flea vector and mammalian host temperatures and sampled at 1 h, 2 h, 4 h and 8 h post-culturing) were analyzed by gas chromatography-mass spectrometry (GC-MS). In support of the NMR results, the GC-MS data showed that glutamic acid, which was not an ingredient of the culture media (i.e. an endogenous metabolite), accumulated to high levels in the YPCO cell culture with time, while remaining at background levels in YPTS and YPPF (Fig. S5, ESI†). Interestingly, the level of glutamate in YPCO sample grown at mammalian host temperature reached maximum at the 4 h time point, and dropped significantly at 8 h time point. In contrast, there was little difference in glutamate concentration in YPCO sample grown at flea vector temperature at the 4 h and 8 h time points. These data were confirmed with the measurement of glutamate by using Amplex Red Glutamic Acid/Glutamate Oxidase fluorescence assay kit from Invitrogen (data not shown). It was observed previously, that during adaptation of YP to the flea gut environment, plague bacterium may catabolize L-glutamate group of amino acids, such as glutamine, histidine, arginine, and proline, to give rise to L-glutamate and the TCA cycle intermediates succinate, formate, and α-ketoglutarate.62 We have not detected differences in expression of genes involved in uptake and catabolism of these amino acids between three Yersinia strains, as well as in concentrations of histidine, arginine, proline, succinate, and formate. The example of this comparison for the transcript and protein levels of key enzymes in glutamate metabolism of YPCO relative YPPF and YPTS is shown on Fig. 4. No significant differences were observed in the expression patterns of glutamate dehydrogenase (YPO3971), glutamate synthase alpha (YPO3557) and beta (YPO3558) subunits, or other key enzymes in glutamate metabolism across all three strains. Given that the difference in glutamate levels between the three strains were not reflected at the level of gene transcription or protein translation, these results implicate post-translational control as a previously unappreciated additional regulatory mechanism involved in modulating glutamate levels in vivo. Interestingly, a recent study of nitrogen metabolism in Mycobacterium smegmatis investigated the transcription and specific enzyme activity of glutamine synthetase and glutamate dehydrogenase. The authors found that glutamate dehydrogenase activity was not reflected at the level of gene transcription, thereby implicating post-transcriptional modification as a regulatory mechanism in response to nitrogen availability.63 |
| Fig. 4 Pathway diagram illustrating comparison of transcript and protein levels of key enzymes in glutamate metabolism across all three strains. Inset panel provides a key/legend for interpreting the pathway elements. YPCO, Yersinia pestis CO92; YPTS, Yersinia pseudotuberculosis PB1/+; YPPF, Yersinia pestis Pestoides F. | |
Conclusions
The molecular mechanisms underlying the enhanced pathogenesis of YP relative to YPT (∼97% sequence identity) remain elusive despite several comparative genomic studies. Here a systems biology approach using sample-matched transcriptomic and proteomic profiling appears to support the hypothesis that differential expression of genes common to both YP and YPT, as opposed to the mere presence or absence of species-specific genes, contributes to the striking difference in the diseases caused by these pathogens; and that similar mechanisms may also contribute to the virulence-restricted phenotype of the non-epidemic YP microtus biovar. Of particular interest, the matched omics profiling of multiple Yersinia strains also revealed conserved post-transcriptional control of metabolism and the translational machinery including the modulation of glutamate levels in Yersiniae. Additionally, statistical inference modeling methods were able to predict novel Yersinia virulence factors. This work highlights the utility of a systems approach incorporating multiple omics measurements and computational analyses to provide novel insights into Yersinia biology; and provides an important resource for the Yersinia research community that should aid the understanding of the markedly different pathogenicities of YP and YPT.Acknowledgements
Research described was supported by the National Institute of Allergy and Infectious Diseases NIH/DHHS through Interagency agreement Y1-AI-8401. Proteomics capabilities were developed under support from the U.S. Department of Energy (DOE) Office of Biological and Environmental Research (BER), NIH grant 5P41RR018522-10 and National Institute of General Medical Sciences grant 8 P41 GM103493-10. Metabolomics capabilities were developed under support from the U.S. DOE BER. Work was performed in the Environmental Molecular Sciences Laboratory, a DOE-BER national scientific user facility at Pacific Northwest National Laboratory (PNNL). PNNL is a multi-program national laboratory operated by Battelle Memorial Institute for the DOE under contract DE-AC05-76RLO 1830.References
- R. D. Perry and J. D. Fetherston, Clin. Microbiol. Rev., 1997, 10, 35–66 CAS.
- M. Achtman, G. Morelli, P. Zhu, T. Wirth, I. Diehl, B. Kusecek, A. J. Vogler, D. M. Wagner, C. J. Allender, W. R. Easterday, V. Chenal-Francisque, P. Worsham, N. R. Thomson, J. Parkhill, L. E. Lindler, E. Carniel and P. Keim, Proc. Natl. Acad. Sci. U. S. A., 2004, 101, 17837–17842 CrossRef CAS.
- P. S. Chain, E. Carniel, F. W. Larimer, J. Lamerdin, P. O. Stoutland, W. M. Regala, A. M. Georgescu, L. M. Vergez, M. L. Land, V. L. Motin, R. R. Brubaker, J. Fowler, J. Hinnebusch, M. Marceau, C. Medigue, M. Simonet, V. Chenal-Francisque, B. Souza, D. Dacheux, J. M. Elliott, A. Derbise, L. J. Hauser and E. Garcia, Proc. Natl. Acad. Sci. U. S. A., 2004, 101, 13826–13831 CrossRef CAS.
- R. R. Brubaker, Yersinia pestis and bubonic plague, Springer, New York, 2006 Search PubMed.
- D. Zhou, Z. Tong, Y. Song, Y. Han, D. Pei, X. Pang, J. Zhai, M. Li, B. Cui, Z. Qi, L. Jin, R. Dai, Z. Du, J. Wang, Z. Guo, P. Huang and R. Yang, J. Bacteriol., 2004, 186, 5147–5152 CrossRef CAS.
- A. P. Anisimov, L. E. Lindler and G. B. Pier, Clin. Microbiol. Rev., 2004, 17, 434–464 CrossRef CAS.
- G. R. Cornelis and F. Van Gijsegem, Annu. Rev. Microbiol., 2000, 54, 735–774 CrossRef CAS.
- R. R. Brubaker, Infect. Immun., 2003, 71, 3673–3681 CrossRef CAS.
- Y. Du, R. Rosqvist and A. Forsberg, Infect. Immun., 2002, 70, 1453–1460 CrossRef CAS.
- D. M. Ferber and R. R. Brubaker, Infect. Immun., 1981, 31, 839–841 CAS.
- S. J. Hinchliffe, K. E. Isherwood, R. A. Stabler, M. B. Prentice, A. Rakin, R. A. Nichols, P. C. Oyston, J. Hinds, R. W. Titball and B. W. Wren, Genome Res., 2003, 13, 2018–2029 CrossRef CAS.
- B. J. Hinnebusch, A. E. Rudolph, P. Cherepanov, J. E. Dixon, T. G. Schwan and A. Forsberg, Science, 2002, 296, 733–735 CrossRef CAS.
- K. Lahteenmaki, R. Virkola, A. Saren, L. Emody and T. K. Korhonen, Infect. Immun., 1998, 66, 5755–5762 CAS.
- J. Parkhill, B. W. Wren, N. R. Thomson, R. W. Titball, M. T. Holden, M. B. Prentice, M. Sebaihia, K. D. James, C. Churcher, K. L. Mungall, S. Baker, D. Basham, S. D. Bentley, K. Brooks, A. M. Cerdeno-Tarraga, T. Chillingworth, A. Cronin, R. M. Davies, P. Davis, G. Dougan, T. Feltwell, N. Hamlin, S. Holroyd, K. Jagels, A. V. Karlyshev, S. Leather, S. Moule, P. C. Oyston, M. Quail, K. Rutherford, M. Simmonds, J. Skelton, K. Stevens, S. Whitehead and B. G. Barrell, Nature, 2001, 413, 523–527 CrossRef CAS.
- I. G. Drozdov, A. P. Anisimov, S. V. Samoilova, I. N. Yezhov, S. A. Yeremin, A. V. Karlyshev, V. M. Krasilnikova and V. I. Kravchenko, J. Med. Microbiol., 1995, 42, 264–268 CrossRef CAS.
- Y. Du, E. Galyov and A. Forsberg, Contrib. Microbiol. Immunol., 1995, 13, 321–324 CAS.
- A. A. Filippov, N. S. Solodovnikov, L. M. Kookleva and O. A. Protsenko, FEMS Microbiol. Lett., 1990, 55, 45–48 CrossRef CAS.
- A. M. Friedlander, S. L. Welkos, P. L. Worsham, G. P. Andrews, D. G. Heath, G. W. Anderson, Jr., M. L. Pitt, J. Estep and K. Davis, Clin. Infect. Dis., 1995, 21(Suppl. 2), S178–181 CrossRef.
- V. Kutyrev, R. J. Mehigh, V. L. Motin, M. S. Pokrovskaya, G. B. Smirnov and R. R. Brubaker, Infect. Immun., 1999, 67, 1359–1367 CAS.
- F. Pouillot, A. Derbise, M. Kukkonen, J. Foulon, T. K. Korhonen and E. Carniel, Microbiology, 2005, 151, 3759–3768 CrossRef CAS.
- S. L. Welkos, G. P. Andrews, L. E. Lindler, N. J. Snellings and S. D. Strachan, Plasmid, 2004, 51, 1–11 CrossRef CAS.
- J. M. Fowler and R. R. Brubaker, Infect. Immun., 1994, 62, 5234–5241 CAS.
- R. R. Brubaker, Infect. Immun., 2005, 73, 4743–4752 CrossRef CAS.
- A. C. Schrimpe-Rutledge, M. B. Jones, S. Chauhan, S. O. Purvine, J. A. Sanford, M. E. Monroe, H. M. Brewer, S. H. Payne, C. Ansong, B. C. Frank, R. D. Smith, S. N. Peterson, V. L. Motin and J. N. Adkins, PLoS One, 2012, 7, e33903 CAS.
- H. M. Mottaz-Brewer, A. D. Norbeck, J. N. Adkins, N. P. Manes, C. Ansong, L. Shi, Y. Rikihisa, T. Kikuchi, S. W. Wong, R. D. Estep, F. Heffron, L. Pasa-Tolic and R. D. Smith, J. Biomol. Tech., 2008, 19, 285–295 Search PubMed.
- J. S. Zimmer, M. E. Monroe, W. J. Qian and R. D. Smith, Mass Spectrom. Rev., 2006, 25, 450–482 CrossRef CAS.
- N. Jaitly, M. E. Monroe, V. A. Petyuk, T. R. Clauss, J. N. Adkins and R. D. Smith, Anal. Chem., 2006, 78, 7397–7409 CrossRef CAS.
- G. R. Kiebel, K. J. Auberry, N. Jaitly, D. A. Clark, M. E. Monroe, E. S. Peterson, N. Tolic, G. A. Anderson and R. D. Smith, Proteomics, 2006, 6, 1783–1790 CrossRef CAS.
- M. E. Monroe, J. L. Shaw, D. S. Daly, J. N. Adkins and R. D. Smith, Comput. Biol. Chem., 2008, 32, 215–217 CrossRef CAS.
- M. E. Monroe, N. Tolic, N. Jaitly, J. L. Shaw, J. N. Adkins and R. D. Smith, Bioinformatics, 2007, 23, 2021–2023 CrossRef CAS.
- K. Petritis, L. J. Kangas, B. Yan, M. E. Monroe, E. F. Strittmatter, W. J. Qian, J. N. Adkins, R. J. Moore, Y. Xu, M. S. Lipton, D. G. Camp, 2nd and R. D. Smith, Anal. Chem., 2006, 78, 5026–5039 CrossRef CAS.
- E. A. Livesay, K. Tang, B. K. Taylor, M. A. Buschbach, D. F. Hopkins, B. L. LaMarche, R. Zhao, Y. Shen, D. J. Orton, R. J. Moore, R. T. Kelly, H. R. Udseth and R. D. Smith, Anal. Chem., 2008, 80, 294–302 CrossRef CAS.
- R. T. Kelly, J. S. Page, K. Tang and R. D. Smith, Anal. Chem., 2007, 79, 4192–4198 CrossRef CAS.
- J. R. Stanley, J. N. Adkins, G. W. Slysz, M. E. Monroe, S. O. Purvine, Y. V. Karpievitch, G. A. Anderson, R. D. Smith and A. R. Dabney, Anal. Chem., 2011, 83, 6135–6140 CrossRef CAS.
- A. D. Polpitiya, W. J. Qian, N. Jaitly, V. A. Petyuk, J. N. Adkins, D. G. Camp, 2nd, G. A. Anderson and R. D. Smith, Bioinformatics, 2008, 24, 1556–1558 CrossRef CAS.
- Y. M. Kim, T. O. Metz, Z. Hu, S. D. Wiedner, J. S. Kim, R. D. Smith, W. F. Morgan and Q. Zhang, Rapid Commun. Mass Spectrom., 2011, 25, 2561–2564 CrossRef CAS.
- T. Kind, G. Wohlgemuth, Y. Lee do, Y. Lu, M. Palazoglu, S. Shahbaz and O. Fiehn, Anal. Chem., 2009, 81, 10038–10048 CrossRef CAS.
- K. Hiller, J. Hangebrauk, C. Jager, J. Spura, K. Schreiber and D. Schomburg, Anal. Chem., 2009, 81, 3429–3439 CrossRef CAS.
- J. J. Faith, B. Hayete, J. T. Thaden, I. Mogno, J. Wierzbowski, G. Cottarel, S. Kasif, J. J. Collins and T. S. Gardner, PLoS Biol., 2007, 5, e8 Search PubMed.
- P. Shannon, A. Markiel, O. Ozier, N. S. Baliga, J. T. Wang, D. Ramage, N. Amin, B. Schwikowski and T. Ideker, Genome Res., 2003, 13, 2498–2504 CrossRef CAS.
- V. D. Blondel, J.-L. Guillaume, R. Lambiotte and E. Lefebvre, J. Stat. Mech.: Theory Exp., 2008, 10, P10008 CrossRef.
- Y. W. Han and V. L. Miller, Infect. Immun., 1997, 65, 327–330 CAS.
- Y. Yang, J. J. Merriam, J. P. Mueller and R. R. Isberg, Infect. Immun., 1996, 64, 2483–2489 CAS.
- L. E. Lindler, M. S. Klempner and S. C. Straley, Infect. Immun., 1990, 58, 2569–2577 CAS.
- X. Z. Huang and L. E. Lindler, Infect. Immun., 2004, 72, 7212–7219 CrossRef CAS.
- R. D. Perry and J. D. Fetherston, Microbes Infect., 2011, 13, 808–817 CrossRef CAS.
- M. Marceau, Curr. Issues Mol. Biol., 2005, 7, 151–177 CAS.
- R. D. Perry, P. B. Balbo, H. A. Jones, J. D. Fetherston and E. DeMoll, Microbiology, 1999, 145(Pt 5), 1181–1190 CrossRef CAS.
- J. Hinnebusch, P. Cherepanov, Y. Du, A. Rudolph, J. D. Dixon, T. Schwan and A. Forsberg, Int. J. Med. Microbiol., 2000, 290, 483–487 CrossRef CAS.
- F. Sebbane, C. Jarrett, D. Gardner, D. Long and B. J. Hinnebusch, Infect. Immun., 2009, 77, 1222–1229 CrossRef CAS.
- E. H. Weening, J. S. Cathelyn, G. Kaufman, M. B. Lawrenz, P. Price, W. E. Goldman and V. L. Miller, Infect. Immun., 2011, 79, 644–652 CrossRef CAS.
- H. Yoon, C. Ansong, J. E. McDermott, M. Gritsenko, R. D. Smith, F. Heffron and J. N. Adkins, BMC Syst. Biol., 2011, 5, 100 CrossRef.
- A. Tidhar, Y. Flashner, S. Cohen, Y. Levi, A. Zauberman, D. Gur, M. Aftalion, E. Elhanany, A. Zvi, A. Shafferman and E. Mamroud, PLoS One, 2009, 4, e7023 Search PubMed.
- D. Greenbaum, C. Colangelo, K. Williams and M. Gerstein, Genome Biol., 2003, 4, 117 CrossRef.
- M. Gry, R. Rimini, S. Stromberg, A. Asplund, F. Ponten, M. Uhlen and P. Nilsson, BMC Genomics, 2009, 10, 365 CrossRef.
- W. Huang da, B. T. Sherman and R. A. Lempicki, Nucleic Acids Res., 2009, 37, 1–13 CrossRef.
- C. Ansong, H. Yoon, S. Porwollik, H. Mottaz-Brewer, B. O. Petritis, N. Jaitly, J. N. Adkins, M. McClelland, F. Heffron and R. D. Smith, PLoS One, 2009, 4, e4809 Search PubMed.
- A. Sittka, S. Lucchini, K. Papenfort, C. M. Sharma, K. Rolle, T. T. Binnewies, J. C. Hinton and J. Vogel, PLoS Genet., 2008, 4, e1000163 Search PubMed.
- A. Sittka, V. Pfeiffer, K. Tedin and J. Vogel, Mol. Microbiol., 2007, 63, 193–217 CrossRef CAS.
- J. Geng, Y. Song, L. Yang, Y. Feng, Y. Qiu, G. Li, J. Guo, Y. Bi, Y. Qu, W. Wang, X. Wang, Z. Guo, R. Yang and Y. Han, PLoS One, 2009, 4, e6213 Search PubMed.
- C. A. Schiano, L. E. Bellows and W. W. Lathem, Infect. Immun., 2010, 78, 2034–2044 CrossRef CAS.
- V. Vadyvaloo, C. Jarrett, D. E. Sturdevant, F. Sebbane and B. J. Hinnebusch, PLoS Pathog., 2010, 6, e1000783 Search PubMed.
- C. J. Harper, D. Hayward, M. Kidd, I. Wiid and P. van Helden, BMC Microbiol., 2010, 10, 138 CrossRef.
Footnotes |
† Electronic supplementary information (ESI) available. See DOI: 10.1039/c2mb25287b |
‡ SC, VLM, MBJ, BCF, SNP, ASR, CA contributed to transcriptomics data generation and analysis. SC, VLM, HMB, BDK, JLD, ASR, CA, JNA contributed to proteomics data generation and analysis. SC, VLM, YMK, KM, TOM, CA contributed to metabolomics data generation and analysis. HDM, JEM, CA contributed to computational network analysis. ASR, HDM, YMK, KM, BD, JEM, TOM, SNP, RDS participated in manuscript preparation. CA, VLM, JNA wrote the manuscript. JNA and RDS contributed partial funding. All authors read and approved the final manuscript. |
|
This journal is © The Royal Society of Chemistry 2013 |