DOI:
10.1039/C2RA01345B
(Paper)
RSC Adv., 2012,
2, 3481-3489
Many structurally related drugs bind different targets whereas distinct drugs display significant target overlap
Received
22nd December 2011
, Accepted 19th January 2012
First published on 23rd January 2012
Abstract
Currently available drugs and bioactive compounds have been subjected to a detailed analysis of their structural and target relationships. When single rings are excluded from structural analysis, due to their generic character, ∼65% of all drugs are found to be involved in substructure relationships and/or are topologically equivalent. Furthermore, most targets of experimental drugs differ from targets of approved drugs. Surprisingly, only a small number of all drugs that share the same or overlapping targets are structurally related. Moreover, structurally similar drugs often act against distinct targets. In bioactive compounds, essentially opposite trends were observed. Thus, on the basis of our systematic analysis, structural and target relationships between drugs depart from the intuitive assumption that similar compounds should have similar activities, which is widely accepted in medicinal chemistry. Possible explanations for these rather unexpected findings are discussed.
Introduction
Currently available drugs have been intensely analyzed from different perspectives. Major focal points of systematic studies, often supported by computational means, include the concept of drug-likeness and associated questions1,2 and the emerging paradigm of polypharmacological drug behavior.3,4 Despite a large number of studies that have been carried out over the past 10–15 years including many computational investigations, it is still not possible to rationalize what exactly renders a molecule drug-like, and this might indeed be difficult, if not impossible, for principal reasons.2 For the study of polypharmacology,3 drugs have been subjected to different types of molecular network analyses to identify drugs acting on the same or similar targets, predict off-target effects, or explore possible alternative applications for existing drugs.3,4 For example, network representations and statistical analysis of networks have been utilized to explore drug–target relationships for approved drugs.5 Furthermore, drugs have been classified according to therapeutic effects, and target classes and drugs that might act on previously unexplored targets have also been analyzed via drug–target networks.6 In previous, conceptually related investigations, we have studied scaffold distributions in compounds at different stages of pharmaceutical development7 and searched for bioactive chemotypes with distinct or overlapping target profiles.8,9
Although drug–target network analyses focus on target annotations of drugs and often take drug–drug similarities into account (for example, calculated pair-wise similarity values to establish drug networks), a question we consider highly relevant has thus far not been explored, surprisingly so: how do structural and target relationships between drugs compare to each other? In other words, do structurally related drugs have similar target activity? There might be two reasons why this rather obvious question has thus far not been directly addressed, including a methodological issue and a general aspect. The methodological issue is that currently available studies that relate drug similarity to target annotations mostly have a “local” focus, i.e., they consider selected pairs or small sets of similar drugs and their target annotations, primarily to identify new drug targets on the basis of pair-wise drug similarity. In addition, such studies typically do not globally assess target annotations. Hence, this type of approach does not yield a thorough comparative evaluation of structural and target relationships between drugs. The general aspect is perhaps equally, if not more relevant. The most likely answer to the question whether similar drugs should act against the same or similar targets would be “yes”; this is what one would intuitively expect. Accordingly, it might not appear to be very appealing to address this question. However, this is what we have done in our study. For public domain drugs and bioactive compounds, we have systematically explored structural and target relationships. These relationships were then combined and quantitatively analyzed. Compared to bioactive compounds, our analysis reveals rather unexpected findings concerning structural and target relationships of drugs, as reported herein.
Experimental
Drugs and structural relationships
From DrugBank,10 version 3.0, approved (a-) or experimental (e-) small molecule drugs with available structures and target annotations were selected. According to the DrugBank organization scheme, e-drugs are drug leads in pre-clinical evaluation or candidates in clinical trials. From all qualifying drugs, Bemis and Murcko (BM) scaffolds11 were extracted by removing all R-groups and retaining ring systems and linkers between them. BM scaffolds were further reduced to cyclic skeletons (CSKs)12 by converting all atoms to carbon and all bond orders to one. The generation of BM scaffolds and CSKs is illustrated in Fig. 1. On the basis of BM scaffolds and CSKs, two types of structural relationships were explored for each possible pair of drugs: (1) substructure relationship: a drug scaffold is a substructure of another; (2) topological equivalence: drug scaffolds yield the same CSK. Structural relationships were systematically determined for a- and e-drugs and all combinations of individual a- and e-drugs (inter-set comparison). Single-ring scaffolds were excluded from the assessment of structural relationships to avoid redundancies. In this study, structural relatedness of drugs was assessed on the basis of these two types of well-defined structural relationships. Conventional fingerprint Tanimoto similarity was not calculated for this purpose because this would require the subjective definition of threshold values for “similarity”, which would present an additional variable for the analysis. By contrast, the hierarchical structural and topological similarity criteria applied herein are non-ambiguous. However, it should also be possible to determine meaningful Tanimoto similarity threshold values for this type of analysis.
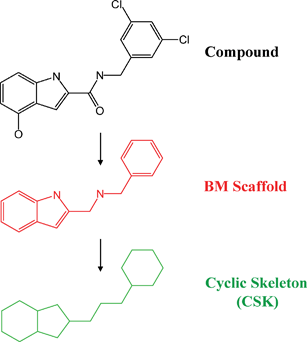 |
| Fig. 1
BM scaffold and CSK. The extraction of a BM scaffold (red) from a compound and its further abstraction to a CSK (green) are illustrated. The BM scaffold is generated by removing all R-groups from the molecule and the CSK is derived from the BM scaffold by converting all heteroatoms to carbon and by setting all bond orders to one. | |
Target and drug hubs
For all drugs, drug–target interactions were determined. Targets with least 20 annotated drugs were considered target “hubs” (in analogy to drug–target network terminology). In addition, from drug–target interactions, relationships between drugs (drug–drug relations) were established by assembling drug pairs sharing one or more targets. Drugs that had overlapping target activity with at least other 50 drugs were designated activity-based drug hubs. In addition, drugs were also identified that were involved in structural relationships with at least 50 other drugs and regarded as structural relationship-based drug hubs. The target activity overlap between each drug hub and the structurally related drugs was quantified by calculating the activity overlap factor (AOF) defined as follows: | 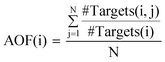 |
(1)
|
Here, #Targets(i) is the number of targets drug hub i is active against, #Targets(i,j) is the number of targets hub i and drug j share, and N is the number of drugs involved in structural relationships with i. AOF ranges from 0 to 1, with 0 indicating distinct target activity for i and all drugs with structural relationships and 1 identical activity. Drug hubs were separately determined for e- and a-drugs. The numbers of drugs used here as criteria (20 and 50, respectively) were set on the basis of the calculated distributions of drugs per target, drug–drug relations, and structural relationships per drug.
Scaffold transformation
Unique scaffold pairs with different structural relationships were identified that represented scaffold transformations among drugs. For each scaffold pair, the number of corresponding drug pairs was determined and the AOF was calculated as follows: |  |
(2)
|
Here, #Targets(ai, bj) is the number of targets drug ai and bj (representing scaffold a and b, respectively) share, #Targets(ai) and #Targets(bj) the numbers of targets the drug ai and bj are active against, respectively, N the number of drugs representing scaffold a, M is the number of drugs corresponding to scaffold b, and ||(ai, bj)|| the number of drug pairs representing the scaffold pair (a, b). Thus, AOF of 0 indicates that a scaffold transformation yields drugs with distinct and 1 with identical activity.
Structure–target relationships for bioactive compounds
As a control, structure–target relationships were also systematically analyzed for bioactive compounds that were assembled from ChEMBL13 (release 10). Compounds active against human targets with explicit potency measurements (i.e. Ki or IC50) were extracted. The annotations with “>”, “<” or “∼” relations were disregarded. In addition, only measurements having the highest confidence level (i.e. ChEMBL target confidence score 9) for direct interactions (target relationship type “D”) were selected. Subsequently, only compounds that had at least 10 μM potency and contained ring systems were retained for further analysis. Substructure relationships and topological equivalences were determined for all compounds. Target hubs, activity-based compound hubs, and structural relationships-based compound hubs were identified, respectively, on the basis of the same criteria applied to drugs. Structural and target relationships were investigated for all target and bioactive compound hubs in a corresponding manner.
ChEMBL also contains potential target annotations for drugs. In part, large numbers of potential targets are reported (on the basis of data from orthologues for a variety of different – and often approximate – assay formats). When applying high confidence data selection criteria to ChEMBL compounds, as stated above, the number of targets is generally reduced, as to be expected. This also applies to drugs available in ChEMBL. For example, for the drugs pyrimethamine, milrinone, and buspirone, the “protein target summary” in ChEMBL reports 22, 42, and 72 potential targets, respectively. By contrast, in DrugBank, the corresponding target numbers are 2, 1, and 2. However, when applying high-confidence data selection criteria specified, the numbers of ChEMBL targets that remain for pyrimethamine, milrinone, and buspirone are 1, 1, and 2, respectively, which match targets reported in DrugBank.
Results and discussion
Structural analysis
Given our selection criteria, 5741 a- and e-drugs were selected that yielded a total of 2538 BM scaffolds and 1275 CSKs. For a-drugs, the molecular weight ranged from 82.1 to 1634.9 Da and for e-drugs, from 68.1 to 2180.3 Da. A total of 194 a-drugs and 483 e-drugs had a weight of more than 500 Da.
On average, each BM scaffold and CSK represented 2.3 and 4.5 drugs, respectively. Overall, 3784 of 5741 drugs (∼66%) were found to be involved in substructural or topological relationships. Structural relationships were separately detected for ∼71% of 1216 a-drugs and ∼63% of 4525 e-drugs. In addition, 843 a-drugs (∼69%) were structurally related to 2312 e-drugs (∼51%). Further details are reported in Table 1. Taken together, the findings reveal a significant and comparable degree of structural relatedness among a- and e-drugs.
Table 1 Drug scaffolds and structural relationshipsa
Type of Drug Set |
Number of |
Drugs |
BM Scaffolds |
CSKs |
Drugs with Structural Relationships |
SUB |
CSK |
SUB + CSK |
Scaffold distributions and structural relationships are reported for all drugs (a- plus e-drugs), a-drugs and e-drugs. SUB stands for substructure.
|
All
|
5741 |
2538 |
1275 |
3111 |
3110 |
3784 (66%) |
Approved
|
1216 |
686 |
413 |
639 |
679 |
865 (71%) |
Experimental
|
4525 |
1985 |
987 |
2273 |
2347 |
2839 (63%) |
Target hubs
The analysis of drug targets is not based on the assumption that all drugs have been tested against all possible targets. Currently available drug–target interactions were systematically identified. A-drugs were active against 873 targets, forming 3744 drug–target interactions and e-drugs against 2885 targets, yielding 8409 interactions. On average, each a-drug was active against ∼3 and each e-drug against ∼2 targets, thus indicating a higher degree of promiscuity of a-drugs. A total of 269 targets were shared for a- and e-drugs. For 2616 targets of e-drugs, no a-drugs existed. Thirty-five target hubs were extracted from a- and 43 from e-drugs. On average, target hubs were associated with 39 a- or 37 e-drugs. Structural relationships were present in all drug sets defined by the 78 target hubs. 31%–97% and 8%–100% of all a- and e-drugs, respectively, associated with target hubs were found to be involved in one or both types of structural relationships (with an average of 64% for a- and 59% for e-drugs). Fig. 2 shows a set of a-drugs for a representative target hub. For the dopamine D4 receptor hub, 14 of 20 drugs were involved in five substructure relationships and seven CSK equivalences. Among a- and e-drugs associated with target hubs, the overall observed fraction of compounds involved in structural relationships only slightly decreased from 71% to 64% and from 63% to 59%, respectively.
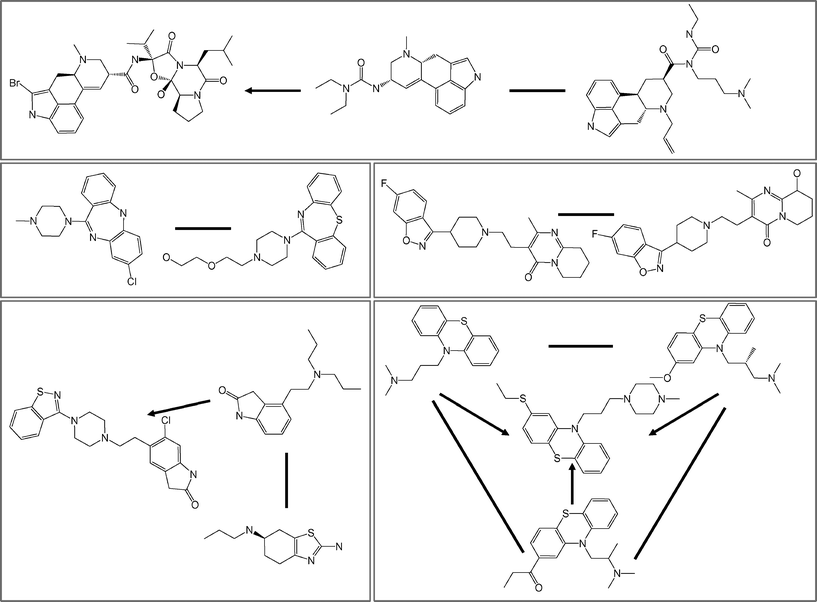 |
| Fig. 2
Representative target hub. Shown are 14 of 20 drugs that were active against the dopamine D4 receptor. Five pairs of drugs have substructure relationships (connected by arrows directed from the smaller to the larger) and seven pairs contain scaffolds yielding the same CSK (connected by lines). | |
Activity-based hubs.
Relations between drugs sharing one or more targets were also determined. A total of 1140 a-drugs (94%) were involved in 22
067 drug–drug relations and 4029 e-drugs (93%) in 51
494 relations. Hence, nearly all drugs shared targets with others. Activity-based drug hubs were then selected that had target overlap with at least 50 other drugs. Details are provided in Table 2. For a-drugs, 322 activity-based hubs were found that, on average, shared targets with 91 other drugs. Structural relationships were detected for drugs associated with 210 of these hubs. However, on average, only eight drug pairs were involved in these relationships. Fig. 3 shows representative hubs and associated drugs. Doxepin is known to act on 20 targets and shares target annotations with 257 other a-drugs, but is structurally related to only nine of these drugs. Olanzapine acts on 25 targets and displays target overlap with other 243 a-drugs. However, olanzapine is not structurally related to any of these drugs. In addition, 514 e-drugs qualified as hubs and for 157 of these hubs, no structural relationships were detected. For the other e-drug hubs, structural relationships were also only formed by, on average, eight drug pairs. Importantly, only ∼10% of drugs sharing target annotations with hubs formed structural relationships, whereas more than 60% of all drugs were involved in such relationships; a striking difference.
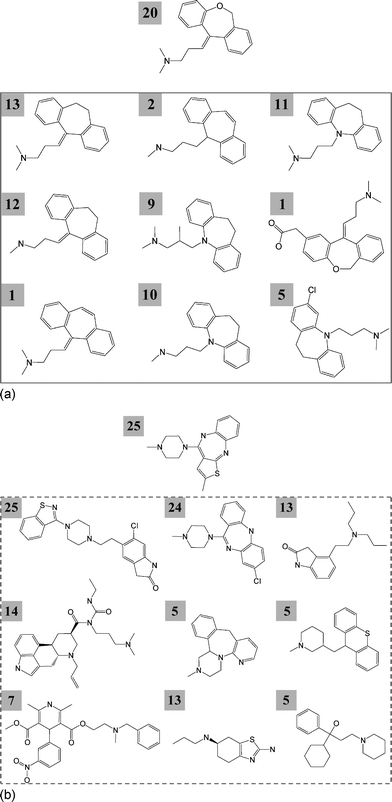 |
| Fig. 3
Representative activity-based drug hubs. Drug hubs are shown at the top with the number of their targets. Selected drugs sharing target activity with a hub are displayed in a box. For each drug, the number of targets shared with the hub is reported. (a) The a-drug doxepin was annotated with 20 targets and shared target annotations with 257 other a-drugs. It displayed structural relationships (CSK equivalences) with only nine of these 257 drugs (bottom). (b) Another a-drug, olanzapine, was active against 25 targets and had overlapping target activity with 243 other a-drugs. Nine exemplary drugs are shown. However, olanzapine did not display structural relationships with any of these 243 drugs. | |
Table 2 Activity-based drug hubsa
Number of |
Approved |
Experimental |
The number of activity-based drug hubs and their average degree (i.e., the number of drugs sharing targets with a hub) are reported for a- and e-drugs. Furthermore, the numbers of hubs with or without structural relationships are provided as well as the average number of drug pairs per hub involved in structural relationships.
|
Drug Hubs
|
322 |
514 |
Degree of Hubs (Avg.)
|
91 |
100 |
With Structural Relationships
|
Hubs
|
210 |
357 |
Drug Pairs (Avg.)
|
8 |
8 |
No Structural Relationships
|
Hubs
|
112 |
157 |
Structural relationship-based hubs.
Target relationships were also determined for structurally-related drugs. Therefore, structural relationship-based drug hubs were selected that formed relations with at least other 50 drugs. In this case, a-drugs were also systematically compared to e-drugs. This inter-set comparison identified a-drug hubs with structural relationships to at least 50 e-drugs and vice versa. Table 3 reports the number of relationship-based drug hubs. For a-drugs, 117 hubs were found representing 25 BM scaffolds and only 5 CSKs and for e-drugs, 971 hubs yielding 339 BM scaffolds and 82 CSKs. Target overlap between a hub and each associated drug was then quantified through calculation of the AOF (for which a value of 0 reflects distinct and of 1 identical target activity). A total of 102 a-drug hubs displayed target overlap, with a maximal AOF of 0.52 (reflecting ∼50% overlap). However, for most of these hubs, only very low AOF values were obtained. In addition, drugs associated with 13% of these hubs had completely distinct target annotations. Furthermore, 706 e-drug hubs were found with a maximal AOF of 0.46 and 27% of these hubs had distinct target annotations. In the inter-set comparison, more a- (188) than e-drug hubs (150) were identified (Table 3). However, for a much higher proportion of these hubs, 68% of a- and 87% of e-drug hubs, all associated drugs had distinct target annotations, yielding an AOF of 0. Thus, taken together, these findings indicate that structurally related drugs often had different target annotations; another unexpected finding.
Table 3 Structural relationship-based drug hubsa
Type of Drug Sets |
Number of Drug Hubs |
All (BM/CSK) |
Target Overlap (Max. AOF) |
Distinct Targets |
The number of structural relationship-based drug hubs is reported for a-, e-drugs, and the inter-set comparison. The numbers of corresponding BM scaffolds and CSKs are given in parentheses. In addition, for each set of drug hubs, the number of hubs with overlapping targets among structurally related drugs is reported and the largest observed AOF is given in parentheses. Furthermore, the number (percentage) of hubs for which all associated drugs have distinct targets is provided.
|
Approved
|
117 (25/5) |
102 (0.52) |
15 (13%) |
Experimental
|
971 (339/82) |
706 (0.46) |
265 (27%) |
Inter-set
|
Approved
|
188 (97/17) |
60 (0.03) |
128 (68%) |
Experimental
|
150 (45/5) |
19 (0.15) |
131 (87%) |
Scaffold pair relationships
All unique scaffold pairs with a substructure or CSK relationship were determined and scaffold pairs with different numbers of drugs and scaffold pair AOF values were analyzed, as reported in Table 4. For all drugs, only small numbers of scaffold pairs were detected that represented more than 10 drug pairs having a target overlap of more than 50% (close to the maximum AOF observed for hubs of approved drugs). Only three and 11 pairs qualifying pairs of scaffolds with substructure relationships were identified for a- and e-drugs, respectively, as shown in Fig. 4a. In addition, five and 26 pairs of scaffolds with CSK equivalences were detected. By contrast, as also reported in Table 4, many more scaffold pairs representing at least 10 drug pairs were identified that had distinct target annotations in a-drugs, e-drugs, and the inter-set comparison, on average ∼260. Examples are given in Fig. 4b. Thus, these findings were consistent with key conclusions drawn from target and drug hub analysis. Taken together, our results revealed that the majority of structurally related drugs had distinct target activity, whereas structurally distinct drugs displayed significant target overlap.
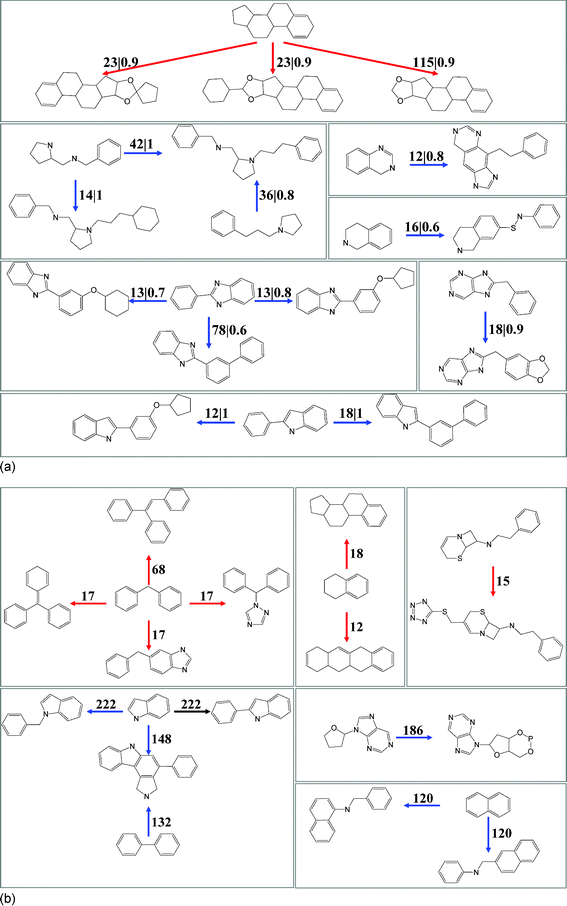 |
| Fig. 4
Structurally related scaffold pairs with distinct target overlap. Scaffold pairs with substructure relationships representing more than 10 pairs of drugs and having different AOF values are shown. (a) AOF > 0.5. These scaffold pairs include three pairs of a-drug (red arrows; directed from the smaller to the larger scaffold) and 11 pairs of e-drug scaffolds (blue arrows). Each pair is annotated with its number of corresponding drug pairs and AOF value. For example, “23|0.9” means that the scaffold pair represents 23 drug pairs and has AOF of 0.9. (b) AOF = 0. Shown are seven representative pairs of a-drug scaffolds and seven representative pairs of e-drug scaffolds. Each pair is annotated with the number of corresponding drug pairs. | |
Table 4 Scaffold pairs from drugs with structural relationshipsa
Number of |
Structural Relationships |
SUB |
CSK |
Approved |
Experimental |
Inter-Set |
Approved |
Experimental |
Inter-Set |
Numbers of drug pairs and corresponding scaffold pairs involved in structural relationships are reported for drugs and the inter-set comparison as well as for five subsets with different AOF and/or pair count characteristics.
|
Drug Pairs
|
2078 |
29,453 |
8550 |
6145 |
55,583 |
23,980 |
Scaffold Pairs
|
All
|
493 |
1986 |
1482 |
1216 |
7854 |
4827 |
Drug Pairs > 10
|
43 |
531 |
172 |
78 |
759 |
368 |
AOF > 0.5
|
78 |
231 |
9 |
84 |
454 |
24 |
AOF > 0.5 Drug Pairs > 10
|
3 |
11 |
0 |
5 |
26 |
0 |
AOF = 0
|
292 |
1543 |
1433 |
955 |
7084 |
4714 |
AOF = 0; Drug Pairs > 10
|
15 |
404 |
166 |
38 |
607 |
335 |
Structure–target relationships for bioactive compounds
From ChEMBL, a total of 71
678 compounds active against 864 human targets were selected that yielded 25
836 unique BM scaffolds. A difference in data composition between bioactive compounds and drugs can be observed. Hence, for comparable numbers of targets (i.e., 864 for bioactive compounds vs. 873 for a-drugs), there were approx. 59-fold more bioactive compounds available than a-drugs. Larger numbers of structural relationships were also found for bioactive compounds (i.e., 97%). Target hubs, activity-based compound hubs, and structural relationship-based compound hubs were also systematically identified for bioactive compounds. Structure–target relationships for hubs originating from bioactive compounds were compared to a- and e-drugs, as reported in Table 5. Approx. 56% of the 864 targets of bioactive compounds were identified as hubs, compared to only ∼4% of a- and ∼1.5% of e-drug targets. In addition, more structural relationships were detected for bioactive compounds binding to target hubs, i.e., 88% compared to 60%. Furthermore, larger numbers of bioactive compounds (80%) shared target activity with at least 50 other compounds (activity-based compound hubs). Only 12% of these hubs formed no structural relationships with activity-related compounds, a percentage much lower than observed for drugs. In addition, 72% of bioactive compounds were involved in structural relationships with at least other 50 compounds (structural relationship-based compound hubs). Less than 3% of these compound hubs had an activity distinct from associated compounds. By contrast, 13% and 27% of these hubs in a- and e-drugs, respectively, displayed distinct activity. Comparable observations were made for scaffold pairs representing structural relationships between bioactive compounds (Table 6). 15% of these scaffold pairs represented more than 10 compound pairs and had an AOF greater than 0.5, whereas less than 5% of such scaffold pairs were found in drugs.
Table 5 Comparison of different types of hubs from bioactive compounds and drugsa
For three sets of compounds, i.e. bioactive compounds, a-drugs, and e-drugs, the numbers and corresponding ratios of different hubs are reported. In addition, for target hubs, the average number of compounds involved in any structural relationship is also given. For activity-based compound hubs, the number of hubs without structural relationships is reported and for structural relationship-based compound hubs, the number of hubs that display distinct activity with structurally related compounds.
|
|
Target Hubs
|
Bioactive
|
Approved
|
Experimental
|
Number of (Ratio)
|
Hubs
|
483 (56%) |
35 (4%) |
43 (1.5%) |
Cpds With Structural Relations (Avg.)
|
196 (88%) |
25 (64%) |
23 (62%) |
|
Activity-based Compound Hubs
|
|
Bioactive
|
Approved
|
Experimental
|
Hubs
|
56989 (80%) |
322 (26%) |
514 (11%) |
No Structural Relations
|
6744 (12%) |
112 (35%) |
157 (31%) |
|
Structural relationship-based Compound Hubs
|
|
Bioactive
|
Approved
|
Experimental
|
Hubs
|
51727 (72%) |
117 (10%) |
971 (21%) |
Distinct Activity
|
1332 (2.6%) |
15 (13%) |
265 (27%) |
Table 6 Scaffold pairs from bioactive compounds with structural relationshipsa
Number of |
Structural Relationships |
SUB |
CSK |
Numbers of bioactive compound pairs and corresponding scaffold pairs involved in structural relationships are reported for five subsets with different AOF and/or pair count characteristics.
|
Compound Pairs
|
5,138,464 |
3,471,477 |
Scaffold Pairs
|
All
|
64,670 |
113,767 |
Compound Pairs > 10
|
36,782 |
30,288 |
AOF > 0.5
|
16,065 |
28,647 |
AOF > 0.5; Compound Pairs > 10
|
6497 (10%) |
5950 (5%) |
AOF = 0
|
39,019 (60%) |
78,982 (69%) |
AOF = 0; Compound Pairs > 10
|
23,301 |
22,193 |
Taken together, these findings illustrate that the structure–target relationships among bioactive compounds differed from those observed for drugs. Fig. 5 shows exemplary drugs with different structure–target relationships. For instance, the four approved drugs tramadol, ketamine, pyrimethamine and milrinone shared the same CSK. However, they were reported to be active against different numbers of targets with different medical indications. By contrast, the other four structurally unrelated approved drugs larazepam, nobilone, ketazolam, and buspirone were anti-anxiety agents but also active against different numbers of targets. However, only one drug–drug relation was detected for lorazepam and ketazolam that shared six targets.
Unexpected structure–target relationships for drugs
Our study of structural and target relationships was focused on target and drug hubs that represented centers of drug–target interactions, target relationships, and drug relations. As such our analysis was conceptually distinct from statistical analysis of recurrent frameworks in drugs11 and from systematic investigations of polypharmacological drug behavior.3 Given our analysis scheme, which involved a systematic comparison of drug–target and drug–drug assignments, we were able to comprehensively account for drug–target and structural relationships. We have quantitatively asserted that structurally related drugs were mostly associated with different targets, whereas structurally distinct drugs often acted on the same or overlapping targets. These observations were not made for bioactive compounds. Hence, for drugs, we observed the tendency to depart from the intuitive assumption that similar compounds should have similar activity, which is often made in medicinal and computational chemistry and generally also referred to as the “similarity-property principle”,14 which has been supported by the findings of different investigations.14–17 Of course, in its generality, the paradigm has its limitations. For example, it is inconsistent with the potential discontinuity of receptor–ligand interactions, leading to the observation that small modifications of a bioactive compound might dramatically alter its activity,18 another often-observed phenomenon in medicinal chemistry. Nevertheless, the similarity-property principle plays an important role in drug research and provides the conceptual basis, for example, for target prediction on the basis of pair-wise (local) drug similarity.4 In light of this situation, we consider the finding that structurally related drugs preferentially act on different targets and distinct drugs on similar ones significant. Although these findings have been unexpected, they have been very clear and there are possible explanations to consider.
Drug–drug similarity is often calculated in a pair-wise manner using chemical descriptors that account for whole-molecule resemblance, which is then expressed as a numerical value.19 Herein, we have systematically detected substructure and topological relationships between drugs and, as a control, bioactive compounds at the level of molecular scaffolds, which represents another global analysis scheme. Also, focusing the analysis on target and drug hubs methodologically differs from systematic pair-wise drug comparison. Furthermore, it should be considered that new drugs for existing targets and therapeutic applications must represent structural novelty as one of the pre-requisites for approval. Thus, there is intrinsic pressure in drug discovery to find structurally diverse compounds acting on similar targets. In addition, popular drug targets are usually good small molecule targets and as such often display a tendency to bind different chemotypes. Moreover, the emerging notion of polypharmacological drug behavior already implies that a given chemotype is recognized by different targets, and this very likely also applies to related compounds. Hence, taken together, these aspects might contribute to the specific features of structure–target relationships identified in our analysis.
Conclusions
In this study, we have systematically analyzed current drugs in order to evaluate their structural and target relationships. For our analysis, we have distinguished between approved and experimental drugs, following the philosophy of DrugBank from which drugs were obtained. It was found that most targets of experimental drugs are distinct from targets of approved drugs; for the majority of targets of experimental drugs, no approved drugs existed. Thus, recent discovery efforts focus on many new targets, which is a rather expected finding. We also found that approved drugs were more promiscuous in nature than experimental drugs for which additional target associations might still be discovered. This is the case because more information is currently available in the literature for a- than for e-drugs. As more experimental data are collected for e-drugs and their targets, additional drug–target pairings might be revealed. However, the results of structural and target relationship analysis were very similar for experimental and approved drugs. We determined that between 60% and 70% of these drugs were involved in substructure or topological relationships. When structural relationships were analyzed in combination with target annotations, we found that structurally related drugs were preferably associated with different targets, whereas structurally distinct drugs often acted on similar targets, which are key results of our study. It is anticipated that these findings will trigger further research to explore relationships between drug structures, target spectra, and therapeutic effects.
References
- P. D. Leeson and B. Springthorpe, Nat. Rev. Drug Discovery, 2007, 6, 881–890 CrossRef CAS.
- O. Ursu, A. Rayan, A. Goldblum and T. I. Oprea, Wiley Interdiscip. Rev.: Comput. Mol. Sci., 2011, 1, 760–781 CrossRef CAS.
- A. L. Hopkins, Nat. Chem. Biol., 2008, 4, 682–690 CrossRef CAS.
- J. A. Metz and P. J. Hajduk, Curr. Opin. Chem. Biol., 2010, 14, 498–504 CrossRef CAS.
- A. Ma'ayan, S. L. Jenkins, J. Goldfarb and R. Iyengar, Mt. Sinai J. Med., 2007, 74, 37–32 CrossRef.
- M. Rask-Andersen, M. S. Almén and H. B. Schiöth, Nat. Rev. Drug Discovery, 2011, 10, 579–590 CrossRef CAS.
- Y. Hu and J. Bajorath, ChemMedChem, 2010, 5, 187–190 CrossRef CAS.
- Y. Hu and J. Bajorath, ACS Med. Chem. Lett., 2011, 2, 523–527 CrossRef CAS.
- Y. Hu and J. Bajorath, ChemMedChem, 2011, 6, 2150–2154 CrossRef CAS.
- C. Knox, V. Law, T. Jewison, P. Liu, S. Ly, A. Frolkis, A. Pon, K. Banco, C. Mak, V. Neveu, Y. Djoumbou, R. Eisner, A. C. Guo and D. S. Wishart, Nucleic Acids Res., 2011, 39, D1035–1041 CrossRef.
- G. W. Bemis and M. A. Murcko, J. Med. Chem., 1996, 39, 2887–2893 CrossRef CAS.
- Y.-J. Xu and M. Johnson, J. Med. Chem., 2002, 42, 912–926 CAS.
-
ChEMBL; European Bioinformatics Institute (EBI): Cambridge, 2011. http:/www.ebi.ac.uk/chembl/ accessed September 1, 2011 Search PubMed.
-
M. A. Johnson, G. M. Maggiora, ed. Concepts and Applications of Molecular Similarity; Wiley: New York, 1990 Search PubMed.
- J. Boström, A. Hogner and S. Schmitt, J. Med. Chem., 2006, 49, 6716–6725 CrossRef.
- Y. C. Martin, J. L. Kofron and L. M. Traphagen, J. Med. Chem., 2002, 45, 4350–4358 CrossRef CAS.
- R. P. Sheridan, B. P. Feuston, V. N. Maiorov and S. K. Kearsley, J. Chem. Inf. Model., 2004, 44, 1912–1928 CrossRef CAS.
- A. M. Wassermann, M. Wawer and J. Bajorath, J. Med. Chem., 2010, 53, 8209–8223 CrossRef CAS.
- H. Geppert, M. Vogt and J. Bajorath, J. Chem. Inf. Model., 2010, 51, 2015–216 Search PubMed.
|
This journal is © The Royal Society of Chemistry 2012 |
Click here to see how this site uses Cookies. View our privacy policy here.