DOI:
10.1039/C1MD00135C
(Concise Article)
Med. Chem. Commun., 2011,
2, 840-852
Chemometric modeling and pharmacophore mapping in coronary heart disease: 2-arylbenzoxazoles as cholesteryl ester transfer protein inhibitors†
Received
25th May 2011
, Accepted 9th June 2011
First published on 1st August 2011
Abstract
The plasma concentration of HDL-C and the prevalence of coronary heart disease (CHD) is inversely related. Inhibition of cholesteryl ester transfer protein (CETP) is considered to be a potential approach to treat dyslipidemia and CHD. 2-arylbenzoxazoles were found to be a potential class of CETP inhibitors. A 2D QSAR study was done on a series of 2-arylbenzoxazoles using PCR, PLS and MLR techniques and externally validated to determine significant models. kNN-MFA 3D QSAR was performed on the same series to correlate the effects of electrostatic, steric and hydrophobic parameters with the CETP inhibitory activity using forward backward regression, genetic algorithm and simulated annealing methods. Pharmacophore mapping was also done on the conformers of the 2-arylbenzoxazoles generated by the BEST and the FAST method. This work may provide a platform for generating leads for novel CETP inhibitors.
Introduction
A reduced level of high-density lipoprotein cholesterol (HDL-C) is a known risk factor for coronary heart disease (CHD).1 Current therapies such as COMPOUND LINKS
Read more about this on ChemSpider
Download mol file of compoundniacin and fibrates suffer from lack of compliance. Thus, novel therapy for raising HDL-C levels is the focus of significant efforts by the cardiovascular medicine community.2Cholesteryl ester transfer protein (CETP) is a 74 kDa plasma glycoprotein responsible for the transfer of cholesteryl esters from high-density lipoprotein (HDL) to very-low-density lipoproteins (VLDLs) and low-density lipoproteins (LDLs).3 A new strategy for raising HDL-C, inhibition of CETP is markedly effective. 2-arylbenzoxazoles represent a class of CETP inhibitors that prevent the transfer of cholesteryl ester from HDL to triglyceride-rich lipoproteins in exchange for triglycerides.4–6 Considering this fact, chemometric modeling through 2D and 3D QSAR studies and pharmacophore mapping were performed on a series of 2-arylbenzoxazoles to find out the structural requirements for more active CETP inhibitors.7–9 The general structure of these compounds is shown in Fig. 1a and 1b.
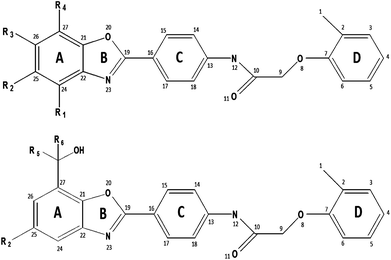 |
| Fig. 1
(a) General structure of Cpd. 1–28 and 43–51 with arbitrary numbering. (b) General structure of Cpd. 29–42 with arbitrary numbering. | |
Forty two 2-arylbenzoxazoles were evaluated for the inhibition of CETP mediated cholesteryl ester transfer by fluorescence transfer assay in the reported work of Smith et al.10 The present work includes the 2D QSAR approaches of principal component regression (PCR), partial least square (PLS) and multiple linear regression (MLR), the 3D QSAR approach of k-Nearest Neighbour Molecular Field Analysis (kNN-MFA) and the pharmacophore mapping of 2-arylbenzoxazoles as CETP inhibitors as a part of our composite program of drug design, discovery and development.11–51 By using different software and statistical methods the pitfalls of any individual method or software could be assumed to be minimized. The importance of several atom based descriptors and molecular descriptors were obtained from the 2D QSAR study. PCR, PLS and MLR were used to develop statistically significant 2D QSAR models.52–55 The kNN-MFA 3D QSAR methodology was applied to the 2-arylbenzoxazole scaffold to correlate the effects of electrostatic, steric and hydrophobic parameters with the inhibition of CETP. In this study, 3D QSAR models were generated by the forward backward regression (FBR), genetic algorithm (GA) and simulated annealing (SA) methods of kNN-MFA.56–58 3D pharmacophore models were developed for the same series of 2-arylbenzoxazoles using the conformers obtained from the FAST and the BEST methods. The 3D pharmacophore model is a ligand-based approach for drug designing. A 3D pharmacophore is a collection of chemical features in 3D space required for a desired biological activity.59 These models were validated by randomizing the data using Fischer's validation technique at the 95% confidence level.60 These analyses may provide a platform for generating leads for novel CETP inhibitors.
Dataset and biological activity
Inhibition of CETP mediated cholesteryl ester transfer was evaluated in vitro using a fluorescence transfer assay.10 The CETP inhibition data for a series of forty two 2-arylbenzoxazoles (1–42) is shown in Table 1. The IC50 (μM) value was converted to the pIC50 (M) value which was taken as the dependent parameter for the QSAR study. For the validation of models generated, an external dataset consisting of nine analogs of 2-arylbenzoxazole (43–51), as shown in Table 1, was taken from the work of Harikrishnan et al.61
Table 1 Substitutions and CETP inhibitory activity of compounds 1–51
Cpd.a |
R1 |
R2 |
R3 |
R4 |
R5 |
R6 |
IC50 (μM) |
pIC50 (M) |
is compound number.
Ref. 10.
Ref. 61.
|
1
b
|
H |
H |
H |
H |
— |
— |
13.000 |
4.886 |
2
b
|
H |
Cl
|
H |
H |
— |
— |
1.100 |
5.959 |
3
b
|
H |
H |
H |
Cl
|
— |
— |
21.000 |
4.678 |
4
b
|
CH3 |
H |
H |
H |
— |
— |
23.000 |
4.638 |
5
b
|
H |
CH3 |
H |
H |
— |
— |
2.000 |
5.699 |
6
b
|
H |
NO2 |
H |
H |
— |
— |
0.940 |
6.027 |
7
b
|
H |
H |
NO2 |
H |
— |
— |
3.200 |
5.495 |
8
b
|
H |
F |
H |
H |
— |
— |
1.900 |
5.721 |
9
b
|
H |
H |
F |
H |
— |
— |
7.500 |
5.125 |
10
b
|
H |
CN |
H |
H |
— |
— |
0.130 |
6.886 |
11
b
|
H |
H |
CN |
H |
— |
— |
0.410 |
6.387 |
12
b
|
H |
H |
H |
CN |
— |
— |
5.200 |
5.284 |
13
b
|
H |
Br
|
H |
H |
— |
— |
1.300 |
5.886 |
14
b
|
H |
OCH3 |
H |
H |
— |
— |
0.840 |
6.076 |
15
b
|
H |
SCH3 |
H |
H |
— |
— |
2.900 |
5.538 |
16
b
|
H |
COCH3 |
H |
H |
— |
— |
1.300 |
5.886 |
17
b
|
H |
CH(OH)CH3 |
H |
H |
— |
— |
3.400 |
5.469 |
18
b
|
H |
CHCH2 |
H |
H |
— |
— |
2.800 |
5.553 |
19
b
|
H |
CCH
|
H |
H |
— |
— |
2.000 |
5.699 |
20
b
|
H |
CN |
CH3 |
H |
— |
— |
1.900 |
5.721 |
21
b
|
H |
Br
|
H |
CH3 |
— |
— |
0.510 |
6.292 |
22
b
|
H |
CN |
H |
CH3 |
— |
— |
0.060 |
7.222 |
23
b
|
H |
CN |
H |
CN |
— |
— |
0.270 |
6.569 |
24
b
|
H |
Cl
|
H |
NO2 |
— |
— |
0.570 |
6.244 |
25
b
|
H |
Br
|
H |
F |
— |
— |
0.910 |
6.041 |
26
b
|
H |
CN |
H |
F |
— |
— |
0.062 |
7.208 |
27
b
|
H |
Br
|
H |
COCH3 |
— |
— |
0.380 |
6.420 |
28
b
|
H |
CN |
H |
COCH3 |
— |
— |
0.086 |
7.066 |
29
b
|
— |
Br
|
— |
— |
H |
CH3 |
0.059 |
7.229 |
30
b
|
— |
CN |
— |
— |
H |
CH3 |
0.046 |
7.337 |
31
b
|
— |
Br
|
— |
— |
CH3 |
CH3 |
0.044 |
7.357 |
32
b
|
— |
CN |
— |
— |
CH3 |
CH3 |
0.028 |
7.553 |
33
b
|
— |
Br
|
— |
— |
CH3 |
CH2CH3 |
0.110 |
6.959 |
34
b
|
— |
CN |
— |
— |
CH3 |
CH2CH3 |
0.031 |
7.509 |
35
b
|
— |
Br
|
— |
— |
CH3 |
n-Pr
|
0.200 |
6.699 |
36
b
|
— |
CN |
— |
— |
CH3 |
n-Pr
|
0.058 |
7.237 |
37
b
|
— |
Br
|
— |
— |
CH3 |
i-Pr
|
0.210 |
6.678 |
38
b
|
— |
CN |
— |
— |
CH3 |
i-Pr
|
0.080 |
7.097 |
39
b
|
— |
Br
|
— |
— |
CH3 |
CCH
|
0.094 |
7.027 |
40
b
|
— |
Br
|
— |
— |
CH3 |
propynyl
|
0.210 |
6.678 |
41
b
|
— |
CN |
— |
— |
CH3 |
propynyl
|
0.160 |
6.796 |
42
b
|
— |
H |
— |
— |
CH3 |
CH3 |
0.440 |
6.357 |
43
c
|
H |
CH2OH
|
H |
H |
— |
— |
1.300 |
5.886 |
44
c
|
H |
CF3 |
H |
H |
— |
— |
0.590 |
6.229 |
45
c
|
H |
H |
CH3 |
H |
— |
— |
13.000 |
4.886 |
46
c
|
H |
H |
H |
CH3 |
— |
— |
1.700 |
5.770 |
47
c
|
H |
H |
H |
COOCH3 |
— |
— |
3.500 |
5.456 |
48
c
|
H |
CH3 |
H |
CH3 |
— |
— |
0.280 |
6.553 |
49
c
|
H |
CH3 |
H |
COCH3 |
— |
— |
0.240 |
6.620 |
50
c
|
H |
CF3 |
H |
CF3 |
— |
— |
0.092 |
7.036 |
51
c
|
H |
Cl
|
H |
NO2 |
— |
— |
0.049 |
7.310 |
Materials and methods
Chemometric modeling
In the field of chemometrics, 2D and 3D QSAR are the two popular tools for designing predictive models.62,63
2D QSAR
Test set selection.
The test set selection was done after arranging these compounds by the Y-based ranking method. In Y-based ranking, compounds were arranged in ascending order of the biological activity. For the test set, 25% of compounds were selected from the arranged set at a specific interval of four data points. Considering the fact that having only one test set–training set combination may include chance factors, three such combinations were developed by this method to ensure proper shuffling of the compounds.
Descriptor generation.
The electrotopological state atom (ETSA) and the refractotopological state atom (RTSA) indices were calculated by the computer program Mouse64 developed in our laboratory. The electrotopological state atom (ETSA) index is a structural descriptor. It encodes the electronic and topological environment of each skeletal atom on a molecule.65–68 The refractotopological state atom (RTSA) index depends on the atomic contribution of the molar refractivity and the topological environment of the atom.69–71 Wang-Ford charge and electrostatic potential were calculated by the Chem 3D Pro 5.0 package of Chembridge Software Inc.72 Electronic descriptors like frontier electron density related to the highest occupied molecular orbital (HOMO) and lowest unoccupied molecular orbital (LUMO) were calculated by the Hyperchem release 7.0 package.73 For the calculation of electronic descriptors, energy minimization of these structures were done according to the semiempirical Austin Model 1 (AM1) by RHF (restricted Hartree–Fock: closed shell) wave function. Different whole molecular descriptors were calculated by the Dragon software.74
Descriptor selection.
A total of 1497 descriptors were calculated. Those descriptors with equal or nearly equal values for all data points were discarded. The remaining descriptors were selected by a forward stepwise method. While developing models, intercorrelated whole molecular descriptors (correlation coefficient >0.6) were grouped together and the descriptor with the highest correlation with the biological activity was selected from that group. In case of atomic descriptors, one more parameter, i.e., the neighbourhood effect of atoms was taken into consideration and descriptors were selected accordingly.
Statistical analyses.
Three statistical methods (PCR, PLS and MLR) were used. All statistical analyses were done by the software Multiregress75 and Least Square76 developed in our laboratory. The overall statistical qualities of equations were justified by the correlation coefficient R, adjusted R2 (R2A), variance ratio (F) and standard error of the estimate (SEE).
Validation of models.
Internal cross-validations of all QSAR equations were done by the Leave-One-Out (LOO) method. The predicted residual sum of square (PRESS), cross-validated R2 (R2cv), standard deviation of PRESS (SPRESS), standard deviation of error of prediction (SDEP) were considered for determining the internal predictive quality of these QSAR models.77 The external predictability of those QSAR models was justified by predicted R2 (R2pred) as well as k, k′ values as suggested by Tropsha et al.52,53 and root mean square (rm2) value as suggested by Roy et al.54
kNN-MFA 3D QSAR
Sphere exclusion principal (SEP).
Selection of the training and the test set for the kNN-MFA 3D QSAR model was done by considering the fact that the test set compounds should represent structural diversity and a range of biological activities similar to that of the training set. The whole dataset was divided into the training set and the test set using a dissimilarity value of 6.5 using the sphere exclusion algorithm (SEP).78 This algorithm allows the construction of training sets covering all the descriptor space areas occupied by representative points. Compounds in the test set allowed one to use one test compound over four training compounds, thus resulting in a more rigorous validation of the training model.
Molecular modeling and alignment.
The 3D QSAR computations were performed on VLife QSAR Plus 1.0 molecular modeling software. The 3D structures of the training and the test set of compounds were drawn using the draw molecule function in VLife QSAR Plus 1.0.79 MMFF94 force field80 and Gasteiger–Marsili charges81 followed by the Austin Model-1 (AM-1) Hamiltonian method were used for the energy minimization of individual molecules as well as for the entire series with a convergence criterion of 0.001 kcal mol−1 Å. The location of each atom is vital for the kNN-MFA study as the descriptor calculation is based on the 3D-space grid. Therefore, the mode to find out the conformation of each molecule and the method to align molecules together are two sensitive parameters to make a reasonable model. The lowest energy conformation of the most active molecule (Cpd. 32) was selected as a reference molecule to fit the training and the test set of compounds by using the align molecules function available with the software. The resulting alignments of molecules were used for building kNN-MFA 3D QSAR models.
Development of models.
To develop the kNN-MFA descriptor fields, a 3D cubic lattice with grid spacing of 2 Å in the x, y, and z dimensions were formed to encompass the aligned molecules. kNN-MFA descriptors were calculated to generate steric field energies and electrostatic fields using an sp3carbon probe atom with a van der Waals radius of 1.52 Å and a charge of +1.0 with a default cutoff energy 30 kcal mol−1. The steric and electrostatic energy values were set at a default value of ±30 kcal mol−1.
Evaluation of models.
The statistical analysis of the kNN field was performed using distance-based weighted averages. The predictive value of the model was evaluated by the standard leave-one-out (LOO) cross-validation method.82,83 The cross-validated correlation coefficient served as a measure of the quality of the model. The predictive r2 calculation was based on molecules in the test set and was used to estimate the predictive power of the kNN-MFA model. The QSAR models were assessed by the number of nearest neighbour (k), cross-validated R2 by leave-one-out method (q2), cross-validated standard error (q2_se), predicted R2 for external test set (pred_r2) and standard error for predicted R2 (pred_r2se). The internal cross validated predictability (q2)55 was evaluated by eqn (1) given below: | 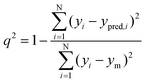 | (1) |
Where the numerator of eqn (1) is the predicted sum of squared deviations between the observed activities (yi) and predicted activities (ypred,i) of the i-th molecule in the training set, while ym is the mean of observed activities of all molecules within the training set. The models were validated externally57 over the external test set and the predictability of the models were denoted by pred_r2 which is obtained using eqn (2): | 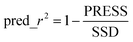 | (2) |
Where PRESS is the sum of squared deviations between the observed and predicted activities of the test set compounds and SSD is the sum of squared deviations between the observed activities of the test molecules and the mean of observed activities of the training molecules.
Pharmacophore mapping
A 3D pharmacophore is a set of chemical features in 3D space essential for a desired biological activity.84 These features include hydrogen bond donors (HBD), hydrogen bond acceptors (HBA), hydrophobic regions (HY), hydrophobic aromatic regions (HY-ARO) hydrophobic aliphatic regions (HY-ALI), ring aromatic regions (RA) and charged/ionizable groups that are assembled appropriately in 3D space to reproduce structural requirements. The success of a pharmacophore hypothesis depends on two theoretical cost calculations. One is the fixed cost and the other is the null cost. Fixed cost represents the simplest model that fits all data points properly. Null cost is the highest cost of a pharmacophore that does not include any feature. It estimates the activity to be the average of all activity of the dataset. A large difference between these two cost values indicates the success of pharmacophore hypothesis. Moreover, the total cost of any pharmacophore hypothesis should be close to the fixed cost. The quality as well as the predictability of a pharmacophore model depends on two other parameters: the configuration/entropy cost and the error cost. The first one is determined by the complexity of the hypothesis space and the second one by the rms deviations between the estimated and actual activities of these compounds. The rms deviations represent the quality of the correlation between the estimated and the observed data.85
Conformation generation.
Conformation generation of the 2-arylbenzoxazoles was performed using the poling algorithm.86 The poling algorithm removes much redundancy in conformation generation and enhances the coverage of conformational space. The number of conformers generated for each molecule was limited to a maximum of 255 with an energy range of 20 kcal mol−1. This is much higher than the number of conformers generated for each molecule. The difference in energy values among different conformers of a compound was less than 20 kcal mol−1. Conformers were generated using both the FAST and the BEST methods.87 The FAST method provides only searches for conformation in torsion space and hence takes less time. The BEST method provides complete and improved searches of conformation by performing rigorous energy minimization and optimizing these conformations in both torsion space and Cartesian space using the poling algorithm. The BEST method performs three steps of conformation generation, i.e., conjugate-gradient minimization in torsion space, conjugate-gradient minimization in Cartesian space and quasi-Newton minimization in Cartesian space.87 The FAST method performs one of the three algorithms depending upon the size of the molecule. The conformational space of small molecules is generated using an efficient systematic search. Conformations with excessive van der Waals clashes are removed. A random search method with poling is used to generate conformations for medium size molecules. The molecule is split into pieces and systematic search is performed for each piece before these pieces are randomly reconnected. If the number of rotatable bonds is greater than 30, one conformation is generated for every possible stereocenter.
3D Pharmacophore generation.
Pharmacophore models were developed using the HypoGen module in Discovery Studio 3.0 with these conformers generated by the BEST and the FAST methods.87 Pharmacophores were developed in consecutive 3 phases: constructive phase, subtractive phase and optimization phase.86 In the constructive phase, common pharmacophores among active molecules were generated. All allowable pharmacophores were generated consisting up to 5 features. The subtractive phase removes the pharmacophores from the data structure which are not likely to be useful. Finally, the optimization is performed using the simulated annealing (SA) algorithm by applying small perturbations to pharmacophores to improve the score. The highest scoring distinctive pharmacophores are then exported.
Ten hypotheses were generated for each of the two sets of conformers (BEST and FAST methods) used. After eliminating the features that do not map active molecules, the following 3 features were selected for subsequent pharmacophore generation: hydrogen bond acceptor (HBA), hydrophobic (HY) and hydrophobic aromatic (HY-ARO).
Fischer's validation.
Validation of the generated pharmacophores was performed using Fischer's validation technique. Fisher's randomization test was done by scrambling the activity data of these molecules and assigning these with new values. The generated pharmacophores using the same features and parameters were regenerated.87 The number of spreadsheets obtained using the randomized test depends on the level of statistical significance considered. At the 95% confidence interval, 19 randomized correlation coefficients were generated. The original hypothesis was believed to be generated by mere chance if the randomized dataset results in the generation of a pharmacophore with a better correlation compared to the original one. The original hypothesis would be significant if its correlation is found to be higher than all the 19 correlation coefficients obtained from the randomized datasets.
Results and discussion
2D QSAR
Y-based ranking.
These 42 compounds were divided into two groups: the training set and the test set. For designing the test set, Y-based ranking was done and 25% compounds were taken in the test set. Three combinations of the test set and the training set (set I–set III) were used in the statistical analyses.88,89 The test set and the training set are shown in Table 2.
Table 2 Training and test set compounds
Set no. |
Training set compound no. |
Test set compound no. |
I |
3, 17, 5, 13, 25, 42, 37, 10, 38, 36, 32, 1, 7, 19, 16, 14, 11, 40, 33, 26, 30, 4, 12, 18, 20, 6, 21, 23, 41, 28, 29, 34 (total = 32) |
9, 15, 8, 2, 24, 27, 35, 39, 22, 31 (total = 10) |
II |
9, 15, 8, 2, 24, 27, 35, 39, 22, 31, 3, 17, 5, 13, 25, 42, 37, 10, 38, 36, 32, 4, 12, 18, 20, 6, 21, 23, 41, 28, 29, 34 (total = 32) |
1, 7, 19, 16, 14, 11, 40, 33, 26, 30 (total = 10) |
III |
9, 15, 8, 2, 24, 27, 35, 39, 22, 31, 1, 7, 19, 16, 14, 11, 40, 33, 26, 30, 4, 12, 18, 20, 6, 21, 23, 41, 28, 29, 34 (total = 31) |
3, 17, 5, 13, 25, 42, 37, 10, 38, 36, 32 (total = 11) |
Principal component regression (PCR).
Factor analysis was performed by the principal component method as the preprocessing step to select descriptors for QSAR equations.90 Fourteen factors were extracted by the principal component method and then rotated by VARIMAX rotation. The factor scores were used as independent parameters for developing QSAR models. Factor scores are shown in the ESI.† Using the forward selection method for the set I training set the following equation was obtained: | pIC50 = 6.269 (±0.071) + 0.591 (±0.072) f1 + 0.175 (±0.080) f2 − 0.265 (±0.072) f14 + 0.278 (±0.088) f9 | (3) |
n = 32, R = 0.892, R2 = 0.795, R2A = 0.764, F(4,27) = 26.16, p < 0.000001, S.E.E. = 0.401, R2cv = 0.729, SSY = 21.184, PRESS = 5.747, SDEP = 0.424, SPRESS = 0.461 |
Where n is the number of data points. Eqn (3) explains 76.4% and predicts 72.9% of the variance of the CETP inhibitory activity. Eqn (1) shows the importance of the factors 1, 2, 14 and 9. Factor 1 is highly loaded with S8, S9, S11, R1, R8, R20, IC2, EP19, nAT, nSK, SNar, w, ww, IDM, TIC2, SEig and moderately loaded with R27, I25, EP23, Sv, Se, Ss, Sp. Factor 2 is highly loaded with S1, S2, S3, S4, S5, S6, S12, S13, S14, S15, S16, S17, S18, S19, S22, S23, R21 and moderately loaded with R14, R15. Factor 14 shows the importance of f(E)2, f(E)4, f(E)L5, f(E)7, Q21 and Q22. Factor 9 shows the significance of I25, nN, nCN. The definition of these terms is given in Table 3.
Table 3 Definition of the terms used in 2D QSAR models
Definition of terms |
IC2 is the Information content index of neighborhood symmetry of 2-order (topological descriptor) |
f(E)2 is the Frontier electron density of the atom number 2 |
Rn is the Refractotopological state atom index of the atom number n |
I25 is the Indicator parameter for the presence of deactivating group at the atom number 25 |
S11 is the Electrotopological state atom index of the atom number 11 |
Q6, Q11, Q19, Q25 are the charges of atom numbers 6, 11, 19 and 25 respectively |
EP21, EP22, EP23 are the electrostatic potential of atom numbers 21, 22 and 23 respectively |
Sv corresponds to the sum of atomic van der Waals volumes scaled on Carbon atom |
Se corresponds to the sum of atomic Sanderson electronegativities scaled on Carbon atom |
Ss is the sum of Kier–Hall electrotopological states |
Sp corresponds to the sum of atomic polarizabilities scaled on Carbon atom |
nN corresponds to the number of Nitrogen atoms |
nCN corresponds to the number of aliphatic nitriles |
nAT corresponds to the number of atoms |
nSK is the number of non-H atoms |
SNar is the log of the Narumi simple topological index |
w corresponds to the detour index |
ww corresponds to the hyper-detour index |
IDM corresponds to the mean information content on the distance magnitude |
TIC2 corresponds to the total information content index neighbourhood symmetry 2-order |
SEig is the absolute eigenvalue sum on the geometry matrix |
Partial least square (PLS).
In this method, five latent variables were selected based on the regression coefficient. Variables with smaller regression coefficients were removed from PLS regression.91,92 The set I training set was used in the partial least square analyses. The final equation is shown below: | pIC50 = −10.985 + 19.902 f(E)2 − 1.510 Q25 − 3.076 EP21 − 6.745 EP23 + 3.417 IC2 | (4) |
n = 32, R = 0.936, R2 = 0.877, R2A = 0.853, F = 37.00, p < 0.0001, S.E.E. = 0.628, R2cv = 0.825, SSY = 21.177, PRESS = 3.706, SDEP = 0.340, SPRESS = 0.378 |
Eqn (4) explains 85.3% of the variance and predicts 82.5% of the variance of the biological activity. The positive coefficients of f(E)2 and IC2 indicate that the higher values of these descriptors may be conducive to the CETP inhibitory activity. The electrophilic attack at the position 2 may be advantageous to the biological activity. The negative coefficient of Q25 indicates that the decrease of the charge at the position 25 may be favourable for the biological activity. The negative coefficients of EP21 and EP23 signify that the decrease of the electrostatic potentials may be beneficial for CETP inhibitory activity. Values of the various descriptors are shown in the ESI.†
Multiple linear regression (MLR).
On the set I training set as used in principal component regression analysis and partial least squares, using forward stepwise method93 the following models were obtained: | pIC50 = −71.731 (±15.006) + 0.773 (±0.161) I25 + 3.957 (±1.023) EP22 + 7.058 (±1.305) S11 + 17.078 (±4.532) Q6 + 11.190 (±4.644) Q11 | (5) |
n = 32, R = 0.923, R2 = 0.852, R2A = 0.824, F(5,26) = 29.937, p < 0.000001, S.E.E. = 0.347, R2cv = 0.767, SSY = 21.184, PRESS = 4.926, SDEP = 0.392, SPRESS = 0.435 |
Eqn (5) explains 82.4% of the variance and predicts 76.7% of the variance of the biological activity.
Another set of new parameters, the R state index of the atom number 1, f(E)2 and EP23 were included in another model shown below:
| pIC50 = −172.694 (±33.307) + 24.178 (±4.632) R1 + 0.691 (±0.139) I25 + 25.886 (±7.256) f(E)2 − 10.354 (±3.374) EP23 | (6) |
n = 32, R = 0.928, R2 = 0.862, R2A = 0.841, F(4,27) = 42.015, p < 0.000001, S.E.E. = 0.330, R2cv = 0.808, SSY = 21.184, PRESS = 4.060, SDEP = 0.356, SPRESS = 0.388 |
Eqn (6) explains 84.1% of the variance and predicts 80.8% of the variance of the CETP inhibitory activity.
The contribution of I25 towards CETP inhibitory activity led to the introduction of another parameter, Q25, which was supposed to be significant for the biological activity of these series of compounds. A new model was developed by using the parameters IC2, EP21, Q19, f(E)2 and Q25 as shown in eqn (7):
| pIC50 = −12.044 (±2.067) + 3.272 (±0.507) IC2 − 3.524 (±0.637) EP21 + 5.935 (±2.375) Q19 + 20.741 (±6.893) f(E)2 − 1.029 (±0.491) Q25 | (7) |
n = 32, R = 0.941, R2 = 0.886, R2A = 0.864, F(5,26) = 40.304, p < 0.000001, S.E.E. = 0.305, R2cv = 0.836, SSY = 21.184, PRESS = 3.469, SDEP = 0.329, SPRESS = 0.365 |
Eqn (7) explains 86.4% of the variance and predicts 83.6% of the variance of the biological activity. While constructing the model, highly collinear molecular descriptors were excluded. I25 was considered to be moderately correlated with Q25 as the later was introduced by modifying the former to describe the models more significantly. Correlation analysis of these descriptors is shown in the ESI.†
To establish the training set independency of the equations another two different combinations of training set were used for developing new models which are comparable with the previous eqn (5), 6 and 7. By taking the set II training set the following QSAR models were developed by multiple linear regression:
| pIC50 = −142.371 (±37.851) + 19.994 (±5.273) R1 + 0.815 (±0.161) I25 + 21.219 (±9.088) f(E)2 − 10.526 (±3.953) EP23 | (8) |
n = 32, R = 0.906, R2 = 0.821, R2A = 0.795, F(4,27) = 31.064, p < 0.000001, S.E.E. = 0.369, R2cv = 0.762, SSY = 20.571, PRESS = 4.890, SDEP = 0.391, SPRESS = 0.426 |
The eqn (8) explains 79.5% and predicts 76.2% of the variance of the biological activity. This equation is comparable to the eqn (6) of the set I. Similarly, descriptors used in eqn (7) were imported to develop eqn (9) on the training set II:
| pIC50 = −11.260 (±2.512) + 3.218 (±0.592) IC2 − 3.021 (±0.668) EP21 + 4.942 (±2.180) Q19 + 28.089 (±8.312) f(E)2 − 1.233 (±0.576) Q25 | (9) |
n = 32, R = 0.918, R2 = 0.843, R2A = 0.813, F(5,26) = 27.947, p < 0.000001, S.E.E. = 0.352, R2cv = 0.776, SSY = 20.571, PRESS = 4.611, SDEP = 0.380, SPRESS = 0.421 |
Eqn (9) explains 81.8% of the variance and predicts 78.5% of the variance of the CETP inhibitory activity. Again a different training set, i.e., set III training set, was used for developing eqn (10)–(12). Eqn (10) is similar to eqn (6) and eqn (8) of set I and set II respectively as the same set of descriptors were used for all these three equations:
| pIC50 = −170.443 (±38.546) + 23.757 (±5.326) R1 + 0.699 (±0.150) I25 + 29.890 (±8.351) f(E)2 − 13.698 (±3.683) EP23 | (10) |
n = 31, R = 0.918, R2 = 0.842, R2A = 0.818, F(4,26) = 34.756, p < 0.000001, S.E.E. = 0.337, R2cv = 0.785, SSY = 18.777, PRESS = 4.037, SDEP = 0.361, SPRESS = 0.394 |
Eqn (10) explains 81.8% of the variance and predicts 78.5% of the variance of the biological activity. Eqn (11) was formed likewise to eqn (5) of set I:
| pIC50 = −84.279 (±17.143) + 0.646 (±0.166) I25 + 2.340 (±1.345) EP22 + 8.409 (±1.461) S11 + 16.329 (±4.215) Q6 + 18.703 (±5.252) Q11 | (11) |
n = 31, R = 0.922, R2 = 0.850, R2A = 0.820, F(5,25) = 28.417, p < 0.000001, S.E.E. = 0.335, R2cv = 0.762, SSY = 18.777, PRESS = 4.466, SDEP = 0.380, SPRESS = 0.423 |
Eqn (11) explains 82.0% of the variance and predicts 76.2% of the variance of the biological activity. Likewise to eqn (7) and eqn (9) of set I and set II respectively, another model was developed as shown in eqn (12):
| pIC50 = −12.371 (±2.237) + 3.341 (±0.533) IC2 − 2.562 (±0.952) EP21 + 6.013 (±2.269) Q19 + 27.335 (±8.347) f(E)2 − 0.930 (±0.454) Q25 | (12) |
n = 31, R = 0.923, R2 = 0.852, R2A = 0.823, F(5,25) = 28.844, p < 0.000001, S.E.E. = 0.333, R2cv = 0.772, SSY = 18.777, PRESS = 4.275, SDEP = 0.371, SPRESS = 0.413 |
Eqn (12) explains 82.3% of the variance and predicts 77.2% of the variance of the CETP inhibitory activity.
As all these models (eqn (3)–(12)) explained and internally predicted variances greater than 50.0% they can be concluded as statistically significant. The important features for CETP inhibitory activity found in the 2D QSAR study are summarized in Fig. 2. Fig. 2 can be correlated with the energy minimized structure of the most active compound (Cpd. 32) shown in Fig. 3.
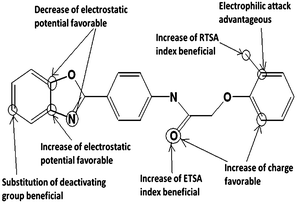 |
| Fig. 2 Important features for CETP inhibitory activity. | |
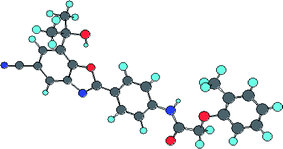 |
| Fig. 3 Energy minimized structure of the most active compound (32). | |
External validation by prediction of test set compounds.
The QSAR models developed on the different training sets were predicted on their respective test sets. Observed (Obs.) and Predicted (Pred.) biological activity obtained from external validation of eqn (3)–(14) are shown in Table 4. The R2pred, rm2, k and k′ values for the eqn (3)–(12) are shown in Table 5. For all equations, predicted R2 (R2pred) and root mean square (rm2) were greater than 0.5 and the k as well as k′ values were in between 0.85 and 1.15. These signify that all these models have good external predictability.52–54
Table 4 Observed (Obs.) and Predicted (Pred.) biological activity obtained from external validation of eqn (3)–(14)
Cpd.a |
Obs. |
Pred. |
eqn (3)
|
eqn (4)
|
eqn (5)
|
eqn (6)
|
eqn (7)
|
eqn (8)
|
eqn (9)
|
eqn (10)
|
eqn (11)
|
eqn (12)
|
eqn (13)
|
eqn (14)
|
is compound number.
|
1
|
4.886 |
— |
— |
— |
— |
— |
5.223 |
4.666 |
— |
— |
— |
— |
— |
2
|
5.959 |
5.638 |
5.770 |
5.854 |
6.157 |
5.564 |
— |
— |
— |
— |
— |
— |
— |
3
|
4.678 |
— |
— |
— |
— |
— |
— |
— |
5.169 |
4.971 |
4.982 |
— |
— |
5
|
5.699 |
— |
— |
— |
— |
— |
— |
— |
5.907 |
5.761 |
5.742 |
— |
— |
7
|
5.495 |
— |
— |
— |
— |
— |
5.479 |
5.699 |
— |
— |
— |
— |
— |
8
|
5.721 |
5.408 |
5.475 |
5.419 |
5.723 |
5.762 |
— |
— |
— |
— |
— |
— |
— |
9
|
5.125 |
4.813 |
5.602 |
5.294 |
5.403 |
5.551 |
— |
— |
— |
— |
— |
— |
— |
10
|
6.886 |
— |
— |
— |
— |
— |
— |
— |
6.623 |
6.356 |
6.384 |
— |
— |
11
|
6.387 |
— |
— |
— |
— |
— |
5.848 |
5.933 |
— |
— |
— |
— |
— |
13
|
5.886 |
— |
— |
— |
— |
— |
— |
— |
5.573 |
5.783 |
5.469 |
— |
— |
14
|
6.076 |
— |
— |
— |
— |
— |
5.987 |
5.990 |
— |
— |
— |
— |
— |
15
|
5.538 |
6.394 |
5.616 |
5.413 |
4.903 |
5.328 |
— |
— |
— |
— |
— |
— |
— |
16
|
5.886 |
— |
— |
— |
— |
— |
6.239 |
5.745 |
— |
— |
— |
— |
— |
17
|
5.469 |
— |
— |
— |
— |
— |
— |
— |
5.408 |
5.500 |
5.831 |
— |
— |
19
|
5.699 |
— |
— |
— |
— |
— |
5.596 |
5.980 |
— |
— |
— |
— |
— |
22
|
7.222 |
6.885 |
6.742 |
6.845 |
6.423 |
6.938 |
— |
— |
— |
— |
— |
— |
— |
24
|
6.244 |
6.366 |
7.111 |
5.989 |
6.412 |
7.227 |
— |
— |
— |
— |
— |
— |
— |
25
|
6.041 |
— |
— |
— |
— |
— |
— |
— |
6.442 |
6.128 |
6.377 |
— |
— |
26
|
7.208 |
— |
— |
— |
— |
— |
6.938 |
6.903 |
— |
— |
— |
— |
— |
27
|
6.420 |
6.415 |
6.575 |
6.502 |
6.317 |
6.707 |
|
|
— |
— |
— |
— |
— |
30
|
7.337 |
|
|
|
|
|
7.046 |
7.161 |
— |
— |
— |
— |
— |
31
|
7.357 |
6.530 |
6.880 |
6.675 |
6.828 |
7.030 |
— |
— |
— |
— |
— |
— |
— |
32
|
7.553 |
— |
— |
— |
— |
— |
— |
— |
7.492 |
7.051 |
7.208 |
— |
— |
33
|
6.959 |
— |
— |
— |
— |
— |
6.879 |
6.789 |
— |
— |
— |
— |
— |
35
|
6.699 |
6.930 |
7.159 |
7.167 |
6.720 |
6.748 |
— |
— |
— |
— |
— |
— |
— |
36
|
7.237 |
— |
— |
— |
— |
— |
— |
— |
7.387 |
7.282 |
7.454 |
— |
— |
37
|
6.678 |
— |
— |
— |
— |
— |
— |
— |
7.578 |
7.775 |
7.137 |
— |
— |
38
|
7.097 |
— |
— |
— |
— |
— |
— |
— |
7.733 |
7.270 |
7.080 |
— |
— |
39
|
7.027 |
7.034 |
7.195 |
6.621 |
6.576 |
6.991 |
|
|
— |
— |
— |
— |
— |
40
|
6.678 |
— |
— |
— |
— |
— |
7.094 |
7.028 |
— |
— |
— |
— |
— |
42
|
6.357 |
— |
— |
— |
— |
— |
— |
— |
6.219 |
6.048 |
6.006 |
— |
— |
43
|
5.886 |
— |
— |
— |
— |
— |
— |
— |
— |
— |
— |
5.737 |
5.536 |
44
|
6.229 |
— |
— |
— |
— |
— |
— |
— |
— |
— |
— |
6.181 |
6.426 |
45
|
4.886 |
— |
— |
— |
— |
— |
— |
— |
— |
— |
— |
5.059 |
5.218 |
46
|
5.770 |
— |
— |
— |
— |
— |
— |
— |
— |
— |
— |
6.100 |
5.924 |
47
|
5.456 |
— |
— |
— |
— |
— |
— |
— |
— |
— |
— |
6.512 |
6.065 |
48
|
6.553 |
— |
— |
— |
— |
— |
— |
— |
— |
— |
— |
6.201 |
6.208 |
49
|
6.620 |
— |
— |
— |
— |
— |
— |
— |
— |
— |
— |
6.051 |
6.617 |
50
|
7.036 |
— |
— |
— |
— |
— |
— |
— |
— |
— |
— |
7.055 |
6.914 |
51
|
7.310 |
— |
— |
— |
— |
— |
— |
— |
— |
— |
— |
7.143 |
7.368 |
Eq. |
R
2
pred
|
rm2 |
k
|
k′ |
3
|
0.628 |
0.555 |
0.983 |
1.012 |
4
|
0.647 |
0.595 |
1.010 |
0.986 |
5
|
0.763 |
0.794 |
0.974 |
1.025 |
6
|
0.671 |
0.729 |
0.967 |
1.030 |
7
|
0.682 |
0.611 |
1.006 |
0.990 |
8
|
0.845 |
0.825 |
0.993 |
1.005 |
9
|
0.882 |
0.854 |
0.988 |
1.011 |
10
|
0.750 |
0.709 |
1.027 |
0.970 |
11
|
0.737 |
0.655 |
1.003 |
0.993 |
12
|
0.831 |
0.816 |
0.999 |
0.998 |
For validation on the external data set eqn (13) and eqn (14) were developed:
| pIC50 = −10.934 (±1.967) + 3.134 (±0.441) IC2 − 3.176 (±0.603) EP21 + 5.055 (±1.898) Q19 + 26.684 (±6.631) f(E)2 − 1.199 (±0.416) Q25 | (13) |
n = 42, R = 0.923, R2 = 0.853, R2A = 0.832, F(5,36) = 41.671, p < 0.000001, S.E.E. = 0.328, R2cv = 0.808, SSY = 26.282, PRESS = 5.056, SDEP = 0.347, SPRESS = 0.375 |
Eqn (13) uses similar descriptors as used in eqn (7), (9) and (12). Eqn (13) explains 92.3% of the variance and predicts 80.8% of the variance of the CETP inhibitory activity. Similarly, eqn (14) was developed using the descriptors similar to the eqn (5) and (11).
| pIC50 = −67.378 (±12.745) + 0.809 (±0.141) I25 + 3.902 (±0.982) EP22 + 6.682 (±1.115) S11 + 14.693 (±3.834) Q6 + 11.629 (±4.223) Q11 | (14) |
n = 42, R = 0.917, R2 = 0.840, R2A = 0.818, F(5,36) = 27.824, p < 0.000001, S.E.E. = 0.342, R2cv = 0.779, SSY = 26.282, PRESS = 5.810, SDEP = 0.372, SPRESS = 0.402 |
Eqn (14) explains 81.8% of the variance and predicts 77.9% of the variance of the CETP inhibitory activity. The R2pred_ext, rm2ext, kext and k′ext values of eqn (13) and eqn (14) are shown in Table 6.
Table 6
R
2
pred_ext, rm2, k and k′ values of eqn (13) and (14)
Eq. |
R
2
pred_ext
|
rm2ext |
k
ext
|
k′ext |
13
|
0.640 |
0.623 |
1.001 |
0.995 |
14
|
0.835 |
0.790 |
1.007 |
0.991 |
It was found that for both these equations (eqn (13) and eqn (14)) the value of R2pred_ext and rm2ext were greater than 0.5 while the Tropsha parameters kext and k′ext lay within 0.85 to 1.15. Thus, prediction on the external dataset gave statistically significant results. These point to the robustness of the QSAR models. The values of the descriptors used for eqn (4)–(14); observed (Obs), calculated (Calc), residual (Res), predicted residual (Press) and LOO predicted (Pred) values; and the t-value and p-value of eqn (3), and (5)–(14) are shown in the ESI.†
kNN-MFA 3D QSAR
These 42 analogs of 2-arylbenzoxazoles were divided into the training set (34 compounds) and the test set (8 compounds) by the sphere exclusion principle. The detail of the dataset division is shown in the ESI.†
According to kNN methodology, pIC50 is a function of independent variables – hydrophobic (H), steric (S) and electrostatic (E) fields. In this study, three statistical methods were used for developing 3D QSAR models:
kNN-MFA Forward backward regression (FBR).
The kNN-MFA forward backward regression (FBR) method yielded validation (q2) and cross validation (pred_r2) of 0.88 and 0.64 respectively. It shows the contribution of the descriptors E_1027, E_933, S_1347, E_1062 and S_1357 in CETP inhibitory activity of the 2-arylbenzoxazoles. E_1027, E_933, S_1347, E_1062 and S_1357 are the electrostatic and steric field energy of interactions between the methyl probe atom and compounds at their corresponding spatial grid points of 1027, 933, 1347, 1062 and 1357.
The summary and the stereoview of the template-based model are shown in Table 7 and Fig. 4 respectively.
Table 7
kNN-MFA forward backward regression summary
F to enter |
3.0 |
|
|
F to remove |
2.9 |
q
2
|
0.8847 |
k Nearest Neighbour |
4 |
q
2_se
|
0.2601 |
n
|
34 |
pred_r2 |
0.6379 |
Degree of freedom |
28 |
pred_r2se |
0.6310 |
Descriptors |
E_1027, E_933,S_1347, E_1062, S_1357 |
|
|
Descriptor range |
E_1027 = −4.11362 to −3.49188 |
|
|
E_933 = 6.60911 to 8.18255 |
|
|
S_1347 = −0.537262 to −0.36639 |
|
|
E_1062 = 1.27896 to 1.71475 |
|
|
S_1357 = −0.368303 to −0.277872 |
|
|
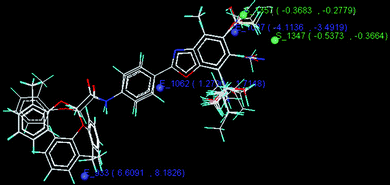 |
| Fig. 4 Stereoview of the kNN-MFA forward backward regression (FBR) model. | |
kNN-MFA Genetic algorithm (GA).
The model developed by the kNN-MFA genetic algorithm (GA) method shows the contribution of the descriptors S_732, H_717, E_1486 in biological activity and explained validation (q2) and cross validation (pred_r2) of 0.67 and 0.82 respectively. S_732, H_717, E_1486 are the steric, hydrophobic and electrostatic field energy of interactions between the methyl probe atom and compounds at their corresponding spatial grid points of 732, 717 and 1486. The details of genetic algorithm study and stereoview of the template based alignment is shown in Table 8 and Fig. 5 respectively.
Table 8
kNN-MFA genetic algorithm details
Population |
100 |
Number of generations |
1000 |
Print after iteration |
100 |
Seed |
0 |
Convergence criteria |
0.005 |
Convergence ending criteria |
5 |
Chromosome length |
3 |
Mutation probability |
0.05 |
Cross over probability |
0.95 |
Statistics |
k Nearest Neighbour |
2 |
n
|
34 |
Degree of freedom |
30 |
Descriptors |
S_732, H_717, E_1486 |
Descriptor range |
S_732 = 0.534455 to 0.604722 |
H_717 = 0.422805 to 0.513086 |
E_1486 = −0.536683 to −0.177957 |
q
2
|
0.6724 |
q
2_se
|
0.4384 |
pred_r2 |
0.8829 |
pred_r2se |
0.4413 |
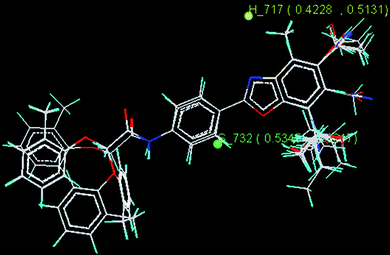 |
| Fig. 5 Stereoview of kNN-MFA genetic algorithm (GA) model. | |
kNN-MFA Simulated annealing (SA).
The best 3D QSAR model was obtained by the kNN-MFA simulated annealing (SA) method which explained validation (q2) and cross validation (pred_r2) of 0.80 and 0.72 respectively. This model shows contribution of H_296, S_721, E_1027, S_740 and E_1472 descriptors in CETP inhibitory activity. H_296, S_721, E_1027, S_740 and E_1472 are the electrostatic, hydrophobic and steric field energy of interactions between the methyl probe atom and compounds at their corresponding spatial grid points of 296, 721, 1027, 740 and 1472. The summary and the stereoview of the model are shown in Table 9 and Fig. 6 respectively.
Table 9
kNN-MFA simulated annealing details
Maximum Temperature |
1000.00 |
Minimum Temperature |
0.01 |
Decrease temperature by |
10.0 |
Iteration at given temperature |
10 |
Terms in model |
5 |
Perturbation limit |
1 |
Seed |
0 |
Statistics |
k Nearest Neighbour |
3 |
n
|
34 |
Degree of freedom |
28 |
Descriptors |
H_296, S_721, E_1027, S_740, E_1472 |
Descriptor range |
H_296 = 0.403633 to 0.423785 |
S_721 = −1.06444 to −1.03944 |
E_1027 = −3.74649 to −3.49188 |
S_740 = −0.226467 to −0.224173 |
E_1472 = 0.111382 to 0.130103 |
q
2
|
0.7951 |
q
2_se
|
0.3467 |
pred_r2 |
0.7194 |
pred_r2se |
0.5555 |
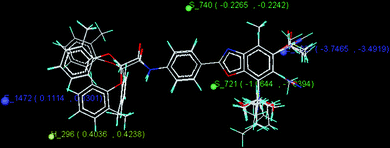 |
| Fig. 6 Stereoview of the kNN-MFA simulated annealing model. | |
Pharmacophore mapping
The FAST and the BEST methods were used to generate diverse conformations of 2-arylbenzoxazoles listed in Table 1. 1796 conformations were obtained by the FAST method and 3957 conformations were obtained by the BEST method.87
Pharmacophore mapping with conformers generated by the BEST method.
10 pharmacophore hypotheses were obtained by pharmacophore generation with conformations generated by the BEST method.86 The hydrogen bond acceptor (HBA), hydrophobic (HY) and hydrophobic aromatic (HY-ARO) features of 2-arylbenzoxazoles were found to contribute towards CETP inhibitory activity. The coefficients of correlation (R) of these hypotheses are in the acceptable range (0.717 to 0.778). The total costs of the hypotheses varied within a small range (183.695 to 188.746) which suggested that the generated hypotheses were homogeneous. These indicated that these developed hypotheses were statistically significant. Among the 10 hypotheses, hypothesis 1 showed maximum correlation. The correlation coefficients, total costs and the features used in the hypotheses are shown in Table 10. Cost differences, error cost, rms values of the hypotheses are shown in the ESI.†
Table 10 Results for the 10 pharmacophore hypotheses generated by using the BEST method of conformer searcha
HT
|
Total cost |
R
|
Features |
Null cost = 199.00, Fixed cost = 160.52, Configuration = 18.12, All cost units are in bits.
|
1 |
183.695 |
0.778 |
HBA, HBA1, HY-ARO, HY, HY1 |
2 |
183.696 |
0.774 |
HBA, HBA1, HY, HY1, HY2 |
3 |
184.217 |
0.768 |
HBA, HBA1, HY, HY1, HY2 |
4 |
186.054 |
0.748 |
HBA, HBA1, HY-ARO, HY, HY1 |
5 |
186.276 |
0.744 |
HBA, HY-ARO, HY-ARO1, HY-ARO2 |
6 |
186.639 |
0.741 |
HBA, HBA1, HY-ARO, HY, HY1 |
7 |
186.826 |
0.739 |
HBA, HBA1, HY-ARO, HY-ARO1, HY |
8 |
187.780 |
0.727 |
HBA, HBA1, HY-ARO |
9 |
188.590 |
0.719 |
HBA, HBA1, HBA2, HY |
10 |
188.746 |
0.717 |
HBA, HY-ARO, HY, HY1, HY2 |
Hypothesis 1 constitutes of five features: HBA, HBA1, HY-ARO, HY and HY1. The distance (Å) between different regions is shown in Fig. 7. In Fig. 8 the most active compound (Cpd. 32) was mapped on the generated pharmacophore of hypothesis 1. It shows the contribution of the hydroxyl group attached with the methyl substituent of the atom number 27 towards the HBA feature and ring A of the benzoxazole towards the HY property. The ring C contributes toward the HY-ARO feature and the oxygen atom (the atom number 11) of the carbonyl group acts as an HBA. Another HY region was found near the methyl group attached with the ring D.
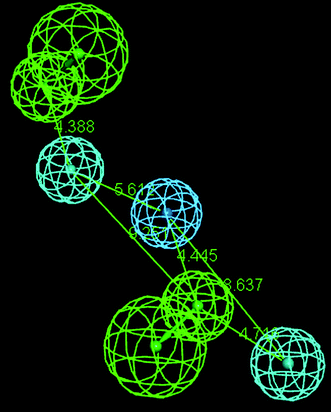 |
| Fig. 7 Distance (Å) between different regions of pharmacophore hypothesis 1 obtained by BEST method of conformer generation. | |
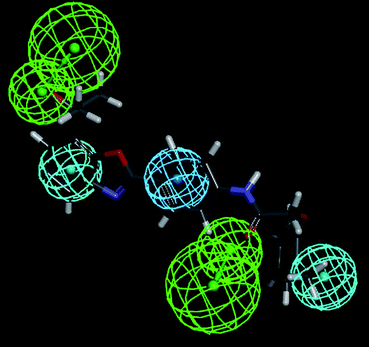 |
| Fig. 8 Most active compound (Cpd. 32) mapped on the pharmacophore hypothesis 1 obtained by the BEST method of conformer generation. | |
Pharmacophore mapping with conformers generated by the FAST method.
The pharmacophore generation was also performed with conformations obtained by the FAST method. Similarly to the BEST method, 10 pharmacophore hypotheses were obtained with HBA, HY and HY-ARO features. The correlation coefficients were within the range of 0.725 to 0.781. The total cost varied within 5 bits (181.908 to 186.708). Such small range of the total costs suggested that the generated hypotheses were homogeneous. The details of the generated hypotheses are shown in Table 11. Cost differences, error cost, rms values of the hypotheses are shown in the ESI.†
Table 11 Results for the 10 pharmacophore hypotheses generated by using the FAST method of conformer searcha
HT
|
Total cost |
R
|
Features |
Null cost = 199.00, Fixed cost = 159.39, Configuration = 16.99, All cost units are in bits.
|
1 |
181.908 |
0.781 |
HBA, HY-ARO, HY-ARO1, HY |
2 |
183.447 |
0.771 |
HBA, HBA1, HY, HY1 |
3 |
183.806 |
0.760 |
HBA, HBA1, HY, HY-ARO |
4 |
184.742 |
0.749 |
HBA, HBA1, HY, HY1, HY2 |
5 |
185.058 |
0.745 |
HBA, HBA1, HY, HY1 |
6 |
185.678 |
0.738 |
HBA, HBA1, HY-ARO, HY |
7 |
186.206 |
0.740 |
HBA, HY-ARO, HY |
8 |
186.576 |
0.728 |
HBA, HY-ARO, HY, HY1, HY2 |
9 |
186.650 |
0.729 |
HBA, HBA1, HY-ARO, HY, HY1 |
10 |
186.708 |
0.726 |
HBA, HY, HY1, HY2, HY3 |
The hypotheses 1 showed the maximum correlation of 0.781. Hypothesis 1 possessed four features: HBA, HY-ARO, HY-ARO1 and HY. The distance between these regions is shown in Fig. 9.
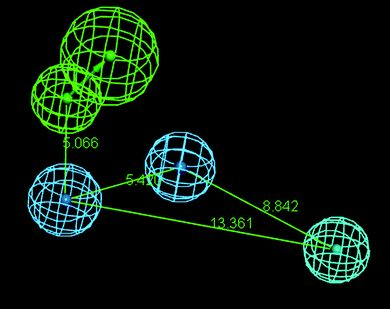 |
| Fig. 9 Distance (Å) between different regions of pharmacophore hypothesis 1 obtained by the FAST method of conformer generation. | |
The most active compound (Cpd. 32) was mapped on the generated pharmacophore of hypothesis 1 and hypothesis 4 which is shown in Fig. 10 and Fig. 11 respectively.
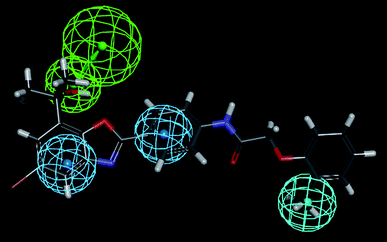 |
| Fig. 10 Most active compound (Cpd. 32) mapped on the pharmacophore hypothesis 1 obtained by the FAST method of conformer generation. | |
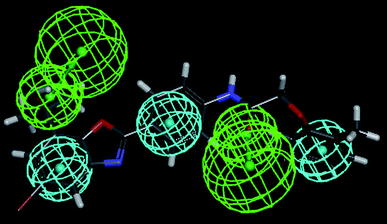 |
| Fig. 11 Most active compound (Cpd. 32) mapped on the pharmacophore hypothesis 4 obtained by the FAST method of conformer generation. | |
Validation of the developed pharmacophores.
The validity of these pharmacophores was assessed using Fischer's validation technique at the 95% confidence level.87 The average correlation coefficient (Rr) of the 19 randomized trials on hypotheses 1 generated by the BEST method was only 0.513. For the FAST method average correlation coefficient (Rr) of the 19 randomized trials on hypotheses 1 and 4 were 0.356 and 0.530 respectively. The result of Fischer's randomization test is given in the ESI.† In all cases the randomized datasets yielded results with inferior correlation coefficients than the original hypotheses. The data showed that the generated hypotheses were robust and statistically significant.
Conclusion
This work gives an insight to the structural requirements of the 2-arylbenzoxazoles for higher CETP inhibitory activity in coronary heart disease. Irrespective of methods, the outcome of the study could be concluded as: the groups attached to the atom numbers 25 and 27 may have significant influence towards the biological activity. Electron withdrawing group substitution to the atom number 25 and hydrogen bond acceptor attachment to the atom number 27 may be favourable to the biological activity. The 2D QSAR models showed that the decrease of electrostatic potential at atom numbers 21 and 23 and the increase of charge at atom numbers 6 and 11 may be beneficial for the biological activity. The increase in the electrostatic potential at the atom number 22 and the decrease in charge at the atom number 25 (i.e., deactivating group attached with the atom number 25) may be favourable for the biological activity. Electrophilic attack at the atom number 2 may be conducive to the biological activity. The increase of structural complexity (denoted by the positive co-efficient of IC2) and E-state index of the atom number 11 and R-state index of the atom number 1 may be contributing to the biological activity. The kNN-MFA 3D QSAR study also signifies the contribution of the electrostatic descriptor E_1027 and the steric descriptor S_1357 near the atom number 25. The distance between the HBA region attached to the atom number 27 and the hydrophobic centre of the benzoxazole moiety appeared to be within 4.38 Å to 5.07 Å. The pharmacophore distance monitoring shows that a 5.42 Å to 5.62 Å distance between the centre of the benzoxazole ring and the ring C may be favourable to CETP inhibitory activity. Another hydrophobic region near the ring D is about 8.5 Å distant from the centre of the hydrophobic region near the ring C. An HBA region at a distance of 4.76 Å from the ring D and 9.25 Å from the centre of benzoxazole moiety may be favourable for the biological activity. The designed pharmacophore can be utilized for searching chemical databases to find leads for novel CETP inhibitors. This work could be extended in future by docking studies using a receptor–ligand co-crystal structure to make a sound basis for the prediction of the CETP inhibitory activity of 2-arylbenzoxazoles.
Acknowledgements
The authors are grateful to the All India Council for Technical Education (AICTE), New Delhi for awarding a research project. Two of the authors (DJ and CM) are thankful to University Grants Commission (UGC), New Delhi for providing post graduate fellowships.
Notes and references
- J. A. Hunt and Z. Lu, Curr. Top. Med. Chem., 2009, 9, 419–427 CrossRef CAS.
- A. Vakalopoulos, C. Schmeck, M. Thutewohl, V. Li, H. Bischoff, K. Lustig, O. Weber, H. Paulsen and H. Elias, Bioorg. Med. Chem. Lett., 2011, 21, 488–491 CrossRef CAS.
- J. J. Kastelein, Am. J. Cardiol., 2007, 100, S47–52 CrossRef.
- E. J. Schaefer and B. F. Asztalos, Am. J. Cardiol., 2007, 100, 25–31 CrossRef.
- J. A. Hunt, S. Gonzalez, F. Kallashi, M. L. Hammond, J. V. Pivnichny, X. Tong, S. S. Xu, M. S. Anderson, Y. Chen, S. S. Eveland, Q. Guo, S. A. Hyland, D. P. Milot, C. P. Sparrow, S. D. Wright and P. J. Sinclair, Bioorg. Med. Chem. Lett., 2010, 20, 1019–1022 CrossRef CAS.
- F. Kallashi, D. Kim, J. Kowalchick, Y. J. Park, J. A. Hunt, A. Ali, C. J. Smith, M. L. Hammond, J. V. Pivnichny, X. Tong, S. S. Xu, M. S. Anderson, Y. Chen, S. S. Eveland, Q. Guo, S. A. Hyland, D. P. Milot, A. M. Cumiskey, M. Latham, L. B. Peterson, R. Rosa, C. P. Sparrow, S. D. Wright and P. J. Sinclair, Bioorg. Med. Chem. Lett., 2011, 21, 558–561 CrossRef CAS.
-
C. D. Selassie, History of quantitative structure–activity relationships, D. J. Abraham ed., in:Burger's medicinal chemistry and drug discovery, Wiley Interscience, Hoboken, 2003, pp. 1–48 Search PubMed.
-
G. L. Patrick, An introduction to medicinal chemistry, 2nd ed., Oxford, New York, 2001 Search PubMed.
-
R. Franke, The theoretical drug design methods, 1st ed., Elsevier, Amsterdam, 1984 Search PubMed.
- C. J. Smith, A. Ali, L. Chen, M. L. Hammond, M. S. Anderson, Y. Chen, S. S. Eveland, Q. Guo, S. A. Hyland, D. P. Milot, C. P. Sparrow, S. D. Wright and P. J. Sinclair, Bioorg. Med. Chem. Lett., 2010, 20, 346–349 CrossRef CAS.
- A. K. Halder and T. Jha, Bioorg. Med. Chem. Lett., 2010, 20, 6082–6087 CrossRef CAS.
- A. K. Halder, N. Adhikari and T. Jha, Chem. Biol. Drug Des., 2010, 75, 204–213 CAS.
- N. Adhikari, M. K. Maiti and T. Jha, Bioorg. Med. Chem. Lett., 2010, 20, 4021–4026 CrossRef CAS.
- N. Adhikari, M. K. Maiti and T. Jha, Internet. Electron. J. Mol. Des., 2010, 9, 1–19 CAS . http://www.biochempress.com/.
- N. Adhikari, M. K. Maiti and T. Jha, Eur. J. Med. Chem., 2010, 45, 1119–1127 CrossRef CAS.
- A. K. Halder, N. Adhikari, M. K. Maiti and T. Jha, Eur. J. Med. Chem., 2010, 45, 1760–1771 CrossRef CAS.
- S. Samanta, Sk.M. Alam, P. Panda and T. Jha, Eur. J. Med. Chem., 2009, 44, 70–82 CrossRef CAS.
- Sk.M. Alam, S. Samanta, A. K. Halder and T. Jha, Eur. J. Med. Chem., 2009, 44, 359–364 CrossRef CAS.
- A. K. Halder, N. Adhikari and T. Jha, Bioorg. Med. Chem. Lett., 2009, 19, 1737–1739 CrossRef CAS.
- T. Jha, P. Chakraborty, N. Adhikari, A. K. Halder and M. K. Maiti, Internet. Electron. J. Mol. Des., 2009, 8, 1–13 CAS . http://www.biochempress.com/.
- T. Jha, S. Samanta, S. Basu, A. K. Halder, N. Adhikari and M. K. Maiti, Internet. Electron. J. Mol. Des., 2008, 7, 234–250 CAS . http://www.biochempress.com/.
- Sk.M. Alam, S. Samanta, Amit Kumar Halder, Soumya Basu and T. Jha, Can. J. Chem., 2007, 85, 1053–1063 CrossRef CAS.
- S. Samanta, Sk.M. Alam, S. Basu, T. Maji, D. K. Roy and T. Jha, Biol. Pharm. Bull., 2007, 30, 2334–2339 CAS.
- P. Panda, S. Samanta, Sk.M. Alam, Soumya Basu and T. Jha, Internet Electron J. Mol. Des., 2007, 6, 280–301 CAS . http://www.biochempress.com/.
- S. Samanta, P. Panda, Sk.M. Alam and T. Jha, Internet Electron J. Mol. Des., 2007, 5, 503–518 Search PubMed . http://www.biochempress.com/.
- S. Samanta, P. Panda, Sk.M. Alam and T. Jha, Internet Electron. J. Mol. Des., 2007, 6, 183–199 CAS.
- A. Basu, S. Gayen, S. Samanta, P. Panda, K. Srikanth and T. Jha, Can. J. Chem., 2006, 84, 458–463 CrossRef CAS.
- B. Debnath, S. Gayen, S. Samanta, A. Basu, B. Ghosh and T. Jha, Ind. J. Chem. A, 2006, 45A, 93–99 CAS.
- S. Samanta, B. Debnath, A. Basu, S. Gayen, K. Srikanth and T. Jha, Eur. J. Med. Chem., 2006, 41, 1190–1195 CrossRef CAS.
- S. Samanta, S. Gayen, B. Ghosh, P. Panda, K. Srikanth and T. Jha, Inter. J. App. Chem., 2006, 2, 169–180 CAS.
- S. Samanta, Sk.M. Alam, P. Panda and T. Jha, Internet Electron J. Mol. Des., 2006, 5, 503–514 CAS . http://www.biochempress.com/.
- S. Gayen, B. Debnath, S. Samanta, B. Ghosh, A. Basu and T. Jha, Internet Electron. J. Mol. Des., 2005, 4, 556–578 CAS . http://www.biochempress.com/.
- S. Samanta, B. Debnath, S. Gayen, B. Ghosh, A. Basu and T. Jha, Farmaco, 2005, 60, 818–825 CrossRef CAS.
- B. Debnath, S. Samanta, S. Gayen, A. Basu, B. Ghosh and T. Jha, Internet Electron. J. Mol. Des., 2005, 4, 393–412 CAS . http://www.biochempress.com/.
- S. Gayen, B. Debnath, S. Samanta and T. Jha, Bioorg. Med. Chem., 2004, 12, 1493–1503 CrossRef CAS.
- S. Samanta, K. Srikanth, S. Banerjee, B. Debnath, S. Gayen and T. Jha, Bioorg. Med. Chem., 2004, 12, 1413–1423 CrossRef CAS.
- B. Debnath, S. Gayen, A. Basu, B. Ghosh, K. Srikanth and T. Jha, Bioorg. Med. Chem., 2004, 12, 6137–6145 CrossRef CAS.
- S. Gayen, B. Debnath and T. Jha, Internet Electron. J. Mol. Des., 2004, 3, 771–780 CAS . http://www.biochempress.com/.
- B. Debnath, S. Gayen, A. Basu, K. Srikanth and T. Jha, J. Mol. Model., 2004, 10, 328–334 CrossRef CAS.
- B. Debnath, S. P. Vishnoi, B. Sa and T. Jha, Internet Electron. J. Mol. Des., 2003, 2, 128–136 CAS . http://www.biochempress.com/.
- B. Debnath, S. Samanta, K. Roy and T. Jha, Bioorg. Med. Chem., 2003, 11, 1615–1619 CrossRef CAS.
- B. Debnath, S. Samanta, S. K. Naskar, K. Roy and T. Jha, Bioorg. Med. Chem. Lett., 2003, 13, 2837–2842 CrossRef CAS.
- T. Jha, B. Debnath, S. Samanta and A. U. De, Internet Electron. J. Mol. Des., 2003, 2, 539–545 CAS . http://www.biochempress.com/.
- B. Debnath, S. Gayen, S. Bhattacharya, S. Samanta and T. Jha, Bioorg. Med. Chem., 2003, 11, 5493–5499 CrossRef CAS.
- B. Debnath, S. Gayen, S. K. Naskar, K. Roy and T. Jha, Drug Des. Discov., 2003, 18, 81–89 CAS.
- K. Srikanth, B. Debnath and T. Jha, Bioorg. Med. Chem., 2002, 10, 1841–1854 CrossRef CAS.
- K. Srikanth, C. A. Kumar, B. Ghosh and T. Jha, Bioorg. Med. Chem., 2002, 10, 2119–2131 CrossRef CAS.
- K. Srikanth, B. Debnath, S. S. Nayak and T. Jha, Ind. J. Pharmacol., 2002, 34, 172–177 CAS.
- B. Debnath, K. Srikanth, S. Banarjee and T. Jha, Internet Electron. J. Mol. Des., 2002, 1, 488–502 CAS . http://www.biochempress.com/.
- K. Srikanth, B. Debnath and T. Jha, Bioorg. Med. Chem. Lett., 2002, 12, 899–902 CrossRef CAS.
- K. Srikanth, C. A. Kumar, D. Goswami, A. U. De and T. Jha, Ind. J. Biochem. Biophys., 2001, 38, 120–123 CAS.
- A. Tropsha, P. Gramatica and V. K. Gomber, QSAR Comb. Sci., 2003, 22, 69–77 CAS.
- A. Golbraikh and A. Tropsha, J. Mol. Graphics Modell., 2002, 20, 269–276 CrossRef CAS.
- P. P. Roy and K. Roy, QSAR Comb. Sci., 2008, 27, 302–313 CAS.
- J. G. Topliss and R. P. Edwards, J. Med. Chem., 1979, 22, 1238–1244 CrossRef CAS.
- V. M. Patil, S. P. Gupta, S. Samanta and N. Masand, Med. Chem. Res., 2010 DOI:10.1007/s00044-010-9435-x , in press.
- S. Nandi and M. C. Bagchi, Mol. Diversity, 2010, 14, 27–38 CrossRef CAS.
- S. Bhandari, K. Bothara, V. Pawar, D. Lokwani and T. Devale, Internet Electron. J. Mol. Des., 2009, 8, 14–28 CAS . http://www.biochempress.com/.
- J. H. V. Drie, Internet Electron. J. Mol. Des., 2007, 6, 271–279 Search PubMed . http://www.biochempress.com/.
- I. Mitra, A. Saha and K. Roy, J. Mol. Model., 2010, 16, 1585–1596 CrossRef CAS.
- L. S. Harikrishnan, M. G. Kamau, T. F. Herpin, G. C. Morton, Y. Liu, C.
B. Cooper, M. E. Salvati, J. X. Qiao, T. C. Wang, L. P. Adam, D. S. Taylor, A. Y. A. Chen, X. Yin, R. Seethala, T. L. Peterson, D. S. Nirschl, A. V. Miller, C. A. Weigelt, K. K. Appiah, J. C. O'Connell and R. M. Lawrence, Bioorg. Med. Chem. Lett., 2008, 18, 2640–2644 CrossRef CAS.
- I. Mitra, A. Saha and K. Roy, Eur. J. Med. Chem., 2010, 45, 5071–5079 CrossRef CAS.
- P. K. Ojha and K. Roy, Eur. J. Med. Chem., 2010, 45, 4645–4656 CrossRef CAS.
-
Mouse is a computer program developed by Jadavpur University.
- L. H. Hall, B. Mohney and L. B. Kier, Quant. Struct.–Act. Relat., 1991, 10, 43–51 CrossRef CAS.
- C. de Gregorio, L. B. Kier and L. H. Hall, J. Comput.-Aided Mol. Des., 1998, 12, 557–561 CrossRef CAS.
- K. Roy, A. U. De and C. Sengupta, Drug Des. Discov., 2002, 18, 33–43 CAS.
-
L. B. Kier and L. H. Hall, Molecular Structure Description: The Electrotopological State, Academic Press, San Francisco, 1999 Search PubMed.
- A. K. Ghose, A. Pritchett and G. M. Crippen, J. Comput. Chem., 1988, 9, 80–90 CrossRef CAS.
- A. K. Ghose and G. M. Crippen, J. Chem. Inf. Comput. Sci., 1987, 27, 21–35 CrossRef CAS.
- R. Carrasco, A. J. Padron and J. Galvez, J. Pharm. Pharm. Sci., 2004, 7, 19–26 CAS.
-
Chem 3D Pro Version 5.0 and Chem Draw Ultra Version 5.0 are software programs developed by Cambridge Soft Corporation, U.S.A.
-
Hyperchem Professional Release 7.0 is a computer program developed by Hypercube Inc., Gainesville, Florida.
-
DRAGON web version 2.1 is a QSAR software developed by Milano Chemometrics and QSAR Research Group, Dipartimento di Scienze dell'Ambiente e del Territorio Universit_degli Studi di Milano – Bicocca, 2002.
-
Multiregress is a statistical software developed in the Department of Pharmaceutical Technology of Jadavpur University.
-
Least Square is a software developed in the Department of Pharmaceutical Technology of Jadavpur University.
-
J. E. Freund and R. E. Walpole, Mathematical statistics, 3rd ed., Prentice-Hall, New Jersy, 1980 Search PubMed.
- A. Golbraikh and A. Tropsha, J. Chem. Inf. Comput. Sci., 2003, 43, 144–154 CrossRef CAS.
-
VLife QSAR Plus 1.0 is a molecular modeling software of VLife Sciences and Technologies, Pune, India. http://www.vlifesciences.com/.
- T. A. Halgren and R. Nachbar, J. Comput. Chem., 1996, 17, 587–615 CAS.
- J. Gasteiger and M. Marsili, Tetrahedron, 1980, 36, 3219–3228 CrossRef CAS.
- S. Wold, Technometrics, 1978, 20, 397–405 CrossRef.
- R. D. Cramer, J. D. Bunce and D. E. Patterson, Quant. Struct.–Act. Relat., 1988, 7, 18–25 CrossRef.
- Y. Kurogi and O. F. Guner, Curr. Med. Chem., 2001, 8, 1035–1055 CAS.
- A. K. Debnath, J. Med. Chem., 2002, 45, 41–53 CrossRef CAS.
- A. Smellie, S. L. Teig and P. Towbin, J. Comput. Chem., 1995, 16, 171–187 CrossRef CAS.
-
Accelrys Inc., Discovery Studio 3.0, San Diego, 2011. http://accelrys.com/ Search PubMed.
-
A. Tropsha, Recent trends in quantitative structure activity relationships, ed. D. J. Abraham, in:Burger's Medicinal Chemistry and Drug Discovery, Vol. 1, John Wiley and Sons Inc., New Jersey, 2003, pp. 49–75 Search PubMed.
- B. Hemmateenejad, K. Javadnia and M. Elyasi, Anal. Chim. Acta, 2007, 592, 72–81 CrossRef CAS.
-
G. W. Snedecor and W. G. Cochran, Statistical Methods, Oxford & Ibh, New Delhi, 1967 Search PubMed.
- S. Wold, M. Sjostrom and L. Eriksson, Chemom. Intell. Lab. Syst., 2001, 58, 109–130 CrossRef CAS.
- J. T. Leonard and K. Roy, Bioorg. Med. Chem., 2006, 14, 1039–1046 CrossRef CAS.
- J. T. Leonard and K. Roy, Bioorg. Med. Chem. Lett., 2006, 16, 4467–4474 CrossRef CAS.
Footnote |
† Electronic supplementary information (ESI) available. See DOI: 10.1039/c1md00135c |
|
This journal is © The Royal Society of Chemistry 2011 |