DOI:
10.1039/C0MD00165A
(Review Article)
Med. Chem. Commun., 2011,
2, 16-30
Proteochemometric modeling as a tool to design selective compounds and for extrapolating to novel targets
Received
21st September 2010
, Accepted 1st October 2010
First published on 1st November 2010
Abstract
‘Proteochemometric modeling’ is a bioactivity modeling technique founded on the description of both small molecules (the ligands), and proteins (the targets). By combining those two elements of a ligand – target interaction proteochemometrics techniques model the interaction complex or the full ligand – target interaction space, and they are able to quantify the similarity between both ligands and targets simultaneously. Consequently, proteochemometric models or complex based models, can be considered an extension of QSAR models, which are ligand based. As proteochemometric models are able to incorporate target information they outperform conventional QSAR models when extrapolating from the activities of known ligands on known targets to novel targets. Vice versa, proteochemometrics can be used to virtually screen for selective compounds that are solely active on a single member of a subfamily of targets, as well as to select compounds with a desired bioactivity profile – a topic particularly relevant with concepts such as ‘ligand polypharmacology’ in mind. Here we illustrate the concept of proteochemometrics and provide a review of relevant methodological publications in the field. We give an overview of the target families proteochemometrics modeling has previously been applied to, and introduce some novel application areas of the modeling technique. We conclude that proteochemometrics is a promising technique in preclinical drug research that allows merging data sets that were previously considered separately, with the potential to extrapolate more reliably both in ligand as well as target space.
1. Introduction: What is ‘proteochemometric modeling’ and what makes it useful for the design of bioactive compounds?
In 1962 Hansch et al. established the COMPOUND LINKS
Read more about this on ChemSpider
Download mol file of compoundwater/COMPOUND LINKS
Read more about this on ChemSpider
Download mol file of compoundoctanol partition coefficient (log P), discovered by Meyer1 and Overton,2 to quantitatively describe the relationship between the structure and biological activity of a substance using regression analysis;3,4 work that can be regarded as the first real quantitative structure-activity relationship (QSAR) study. Over the last decades this field has been greatly expanded, as computational methods can greatly reduce the number of experiments necessary to obtain a viable COMPOUND LINKS
Read more about this on ChemSpider
Download mol file of compoundlead compound. They can do so by removing compounds from the set of candidates based on in silico experimentation before an actual ‘wet lab’ experiment needs to be performed.5 The high expenses of innovative research and development have supported the case of computational research as it can be performed very cost effectively; i.e. it can help to reduce the costs of innovative drug research.6 Furthermore the computational models obtained can be used to predict effects of untested substances, thus successfully finding their way into a virtual screening7 workflow. Basic assumptions in structure activity studies are that (i) compounds sharing some chemical similarity should also share targets and (ii) targets sharing similar ligands should also share similar properties.8–10 To summarize, conventional QSARs represent a very broad collection of computational tools that can model any output variable with input variables in the form of molecular descriptors using statistical approaches. However, QSARs have some limitations and drawbacks; these will be described in more detail below, followed by the extensions proteochemometrics modeling makes to alleviate at least some of those limitations.
Why improve QSAR?
A drawback of QSARs is that they consider the interaction of a group of compounds to only a single target, and often have a minimal ability to extrapolate (and sometimes even interpolate) into novel areas of chemical space.11 This automatically requires that enough data is available on a specific target before a meaningful model can be constructed, which is rarely the case when searching for hits on a recently identified target. Furthermore, as conventional QSAR approaches consider only the ligands, the ability of QSAR approaches alone is very limited for identifying new classes of ligands or new binding modes of similar compounds outside the training set.
Although in practice there are usually multiple similar ligands that bind to a protein with varying affinities, these varying affinities are not caused only by the chemical structure, but also by the binding site. In fact, the concept of simultaneously considering ligand and binding site similarity has caused Kauvar to postulate that binding to anyprotein can be described by a linear combination of binding affinities to ‘orthogonal’ protein binding sites12 – a concept very much resembling current proteochemometrics (and chemogenomics) thinking.
The binding pocket is usually not a rigid pocket but has some flexibility allowing an induced fit of the ligand molecule.13,14 As the pocket is not described by molecular descriptors in the case of QSAR, QSAR will naturally not always be able to describe all aspects of protein–ligand interaction.15 Therefore, scientists should be cautious not to overstep the boundaries of the applicability domain for each QSAR (Fig. 1). Overstepping this boundary might lead to the occurrence of ‘activity cliffs’16–18 in the activity landscape that present the modeler with situations where similar ligands do not always lead to similar activity.13 Possible causes of these cliffs include different binding modes, different binding sites and synergistic effects of chemical features of the ligand with features of the binding pocket, all of which cannot be covered in a QSAR model.
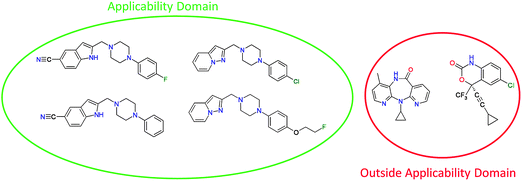 |
| Fig. 1 An example of the applicability domain concept. Depicted on the left side are known structures of Dopamine receptor binding compounds. When a model is trained on these compounds it can only be expected to make reliable predictions for compounds that are chemically similar to this training set. On the right side two HIV reverse transcriptase inhibiting compounds are depicted, as these compounds are chemically very different from the training set the model cannot be expected to make reliable predictions of their possible affinity for the dopamine receptor. | |
Proteochemometric modeling
Contrary to QSAR, proteochemometric (PCM) modeling is based on the similarity of a group of ligands and a group of targets, to the extent that PCM models the so-called ligand-target interaction space.14,19 Like in QSAR modeling, the PCM model is constructed based on chemical descriptors that describe the compound data set and it introduces an additional term, a descriptor of the protein or target (Fig. 2). Therefore a PCM model is constructed on both ligand and target similarity and can be regarded as an extension of conventional QSAR modeling. Furthermore one more additional term can be introduced, which describes effects on both ligand and target and the specific interactions between a compound and a target, called the cross term.19–22 Here the difference with chemogenomic approaches becomes clear, chemogenomics is founded mainly on ligand similarities rather than the combination of the two. Nonetheless, the two techniques are quite similar and even show overlap as reviewed recently.23 In a direct comparison Lapinsh et al. showed PCM to outperform QSAR19 and these findings were corroborated by both Geppert et al.24 and Ning et al.25 However, QSAR and PCM have also been shown to perform nearly identically on an identical training set by Lapinsh et al.,11 although it should be noted that in that particular study a highly simplified form of protein description was used.
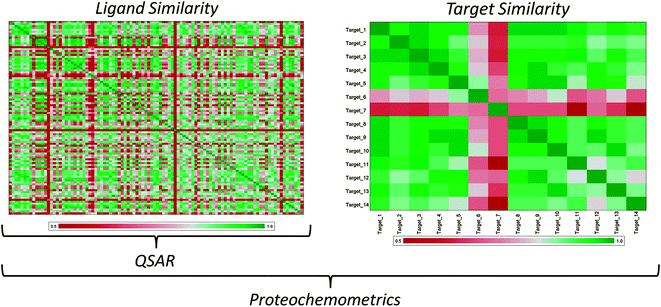 |
| Fig. 2 The difference between QSAR and PCM. The illustration shows two similarity matrices, one for a group of ligands and one for a group of related targets. In the heat maps green illustrates a high similarity while red illustrates a low similarity and white depicts an average similarity. PCM uses both ligand and target similarities for model generation, modeling the interaction complex. However, QSAR only uses ligand similarity, modeling only the left hand side of the ligand – target interaction space. Therefore, while QSAR and PCM are founded on similar principles, PCM can benefit from additional information in model training. | |
The main advantage of PCM is that the model can describe different interactions of a series of compounds to a series of targets while still being able to describe specific interactions of individual compounds to individual targets in the data set. Effectively PCM can thereby connect neighboring QSAR data sets on the basis of the similarity between the targets contained in these data sets. Therefore, in order to create a true PCM model, it is necessary to have activity data of multiple compounds on multiple targets. When creating a PCM model on a single target, the fact that all targets are identical makes this PCM model in reality a QSAR model.21,26
The fact that PCM can connect neighboring QSAR data sets makes it quite similar to inductive learning. Inductive learning is a QSAR based technique consisting of the learning of a property on a data set and to use the extracted knowledge to better learn a related property. (e.g. learn rat blood-brain barrier permeability based on a large rat data set, then use the predicted rat BBB term as a descriptor in a human BBB model. Effectively only the difference between human and rat should then be learned, while letting the rat QSAR model account for the common issues that modulate BBB-permeation in both species). In the example given here, the predicted rat BBB descriptor can be compared with a protein descriptor in the case of PCM. The major difference between the example and PCM is that the former requires two separate steps of model training whereas the latter requires one. Furthermore, the interpretability of PCM will likely be higher as it relates to target (dis)similarity rather than a non-target related descriptor such as ‘rat BBB penetration’
Since PCM contains a target descriptor, the major advantage is that PCM can create a single model predicting a single output variable of the interaction, e.g. affinity, between a very diverse series of compounds or targets and still provide a statistically solid model.11,20 It allows the modeler not only to extrapolate the activity of new compounds on known mutants or targets, but also to extrapolate the activity of known compounds on new mutants or targets (Fig. 3). PCM can also be applied in situations where the 3D information of the targets is unavailable or when the 3D approach is unreliable. Typical examples are situations where no crystal structure is at hand or where only low quality homology models are available.
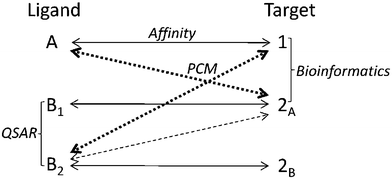 |
| Fig. 3 Possibilities of PCM in a hypothetical data set where the affinity of three different compounds was measured on three different targets. QSAR is able to calculate an output variable based on the similarity of compounds (use the similarity between compound B1 and B2) and Bioinformatics can quantify the similarity between targets (similarity between target 1 and 2A). However PCM can use this information to extrapolate the activity of compounds on targets. (dashed double arrows) In this case a PCM prediction for the activity of B2 on 2A is likely more accurate than the prediction of the activity of B2 on target 1. | |
PCM, like QSAR, can implement a variety of machine learning techniques including both linear and non-linear methods to construct a model.19,27 Furthermore PCM has already been applied to a wide variety of relevant drug targets. To illustrate this versatility we will provide a short overview of the targets PCM has previously been applied to below. Subsequently we will provide an overview of previously used ligand descriptors, target descriptors and cross terms in PCM modeling. Thirdly we will outline some of the machine learning techniques compatible with PCM. We will end the review with some possible pitfalls and disadvantages and the final conclusions.
2. Biochemical applications of PCM techniques
Although PCM has been introduced fairly recently the technique has been tested on a wide variety of relevant drug targets. For a comprehensive overview of targets to which PCM has been applied see Table 1. Overall PCM has mainly been applied to data sets of G protein-coupled receptors (GPCRs), in particular the rhodopsin-like class A receptors. COMPOUND LINKS
Read more about this on ChemSpider
Download mol file of compoundDopamine, COMPOUND LINKS
Read more about this on ChemSpider
Download mol file of compoundhistamine, adrenergic and melanocortin receptors have been described in various ways ranging from binary to local descriptors of protein structures. Interestingly, Lapinsh et al.14 showed that PCM was able to create a viable model from a data set containing multiple related class A receptors based on their transmembrane alpha-helical regions with a pKi error of approximately 0.55 log units, which is a rather well-performing model. It should be noted though that this data set contained limited chemical diversity as it was based on only 22 ligands. The targets side contained 31 GPCRs, being described by 159 TM domain amino acids. In related work, Weil and Rognan also create a model including multiple class A GPCRs. Their model was based on a custom fingerprint encoding both ligand and target features in one fixed length array of bits.28 Using several classification models, they showed that it is in fact possible to create one global GPCRPCM model. Furthermore, they demonstrated that their models are able to retrieve the natural receptor ligands from a decoy-spiked data set of 200,000 ligand – target pairs.
Table 1 List of applications of proteochemometrics modeling (near-comprehensive to the knowledge of the authors, though in particular publications using a different name for the technology might have been missed)a
Year |
Modeling Technique |
Targets |
Prospectively Validated? |
Ligand Descriptors |
Target Descriptors |
Cross Terms |
Reference |
Explanation of abbreviations: Protein Database (PDB), Computed Ligand Binding Energy (CLiBE), GPCR LIgand Database (GLIDA), Human Immunodeficiency Virus (HIV), Partial Least Squares (PLS), Rough Set (RS), Neural Net (NN), Support Vector Machines (SVM), Random Forest (RF), Naïve Bayesian (NB), Decision Tree (DT), Grid Independent Descriptors (GRINDs), one dimensional (1D), two dimensional (2D), three dimensional (3D).
|
2001 |
PLS |
Melanocortin Receptors |
No |
Binary |
Binary |
Multiplication |
111
|
2001 |
PLS |
1a, 1b and 1d Alfa-Adrenergic Receptors |
No |
Binary |
Sequential (Z-scales) |
Multiplication |
19
|
2002 |
PLS |
COMPOUND LINKS
Read more about this on ChemSpider
Download mol file of compoundSerotonin, COMPOUND LINKS
Read more about this on ChemSpider
Download mol file of compoundDopamine, COMPOUND LINKS
Read more about this on ChemSpider
Download mol file of compoundHistamine, and Adrenergic receptors |
No |
GRINDs |
Sequential (Z-scales) |
Multiplication |
59
|
2002 |
SVM
|
CLiBE Selection |
No |
1D Projection of Physicochemical Properties |
Sequential Physicochemical Properties |
— |
40
|
2003 |
PLS |
Melanocortin Receptors |
No |
GRINDs |
TM Identity |
Multiplication |
11
|
2005 |
PLS |
Melanocortin Receptors |
No |
Physicochemical |
Binary |
Multiplication |
20
|
2005 |
PLS |
Set I COMPOUND LINKS
Read more about this on ChemSpider
Download mol file of compoundSerotonin, COMPOUND LINKS
Read more about this on ChemSpider
Download mol file of compoundDopamine, COMPOUND LINKS
Read more about this on ChemSpider
Download mol file of compoundHistamine, Adrenergic receptors, |
No |
GRINDs |
Sequential (Z-scales) |
Multiplication |
82
|
Set II 1a, 1b and 1d Alfa-Adrenergic Receptors |
2005 |
PLS |
COMPOUND LINKS
Read more about this on ChemSpider
Download mol file of compoundSerotonin, COMPOUND LINKS
Read more about this on ChemSpider
Download mol file of compoundDopamine, COMPOUND LINKS
Read more about this on ChemSpider
Download mol file of compoundHistamine, Muscarinic COMPOUND LINKS
Read more about this on ChemSpider
Download mol file of compoundAcetylcholine and Adrenergic receptors |
No |
GRINDs |
Sequential (Z-scales) |
Multiplication |
14
|
2005 |
SVM
|
Orphan GPCRs |
No |
Physicochemical and 2D |
Sequential Physicochemical |
— |
29
|
2006 |
PLS |
Melanocortin Receptors |
Yes |
Binary |
Sequential (Z-scales) |
Multiplication |
21
|
2006 |
RS |
Set I Melanocortin Receptors, |
No |
ASCII String |
ASCII String |
— |
27
|
Set II Melanocortin Receptors, |
Set III Adrenergic Receptors |
2006 |
RS and PLS |
Hydrolases, Lysases, Neuramidases, Anhydrases |
No |
Physicochemical, 1D, 2D, 3D |
Local Descriptors of Protein Structure |
Multiplication |
34
|
2006 |
PLS |
PDBind subset |
No |
2D and 3D |
3D Structural |
Protein-Ligand Interaction |
64
|
2007 |
PLS |
Melanocortin Receptors |
No |
Binary |
Binary/Sequential (Z-scales) |
Multiplication |
62
|
2007 |
PLS |
Antigen recognizing Antibodies |
No |
Sequential (Z-scales) |
Sequential (Z-scales) |
Multiplication |
35
|
2007 |
PLS |
Melanocortin Receptors |
No |
Sequential (Z-scales) |
Sequential (Z-scales) |
Multiplication |
26
|
2008 |
PLS |
(point mutated) HIV Proteases |
No |
GRINDs |
Sequential (Z-scales) |
Multiplication |
31
|
2008 |
PLS |
Cytochrome P450 enzymes |
No |
GRINDs |
Binary and Sequential |
Multiplication |
112
|
2008 |
Linear and NN |
Matrix Metalloproteinases |
No |
2D Autocorrelation vectors |
Amino Acid Sequence Autocorrelation vectors |
— |
89
|
2008 |
PLS |
Dengue Virus NS3 Proteases |
No |
Physicochemical |
Binary |
Multiplication |
33
|
2008 |
SVM
|
Large Crystal Structure data set |
No |
Physicochemical, 1D, 2D, 3D |
Local Descriptors of Protein Structure |
— |
67
|
2008 |
SVM
|
GLIDA subset |
No |
2D and 3D kernels |
Multitask, Hierachy and Binding pocket kernel |
— |
30
|
2009 |
SVM
|
Proteases |
No |
2D Fingerprints |
Sequence Similarity |
— |
24
|
2009 |
PLS |
(Point Mutated) HIV Proteases |
No |
Physicochemical |
Sequential (Z-scales) |
Multiplication |
32
|
2009 |
PLS |
(Point Mutated) HIV Proteases |
Yes |
Sequential (Z-scales) |
Sequential (Z-scales) |
Multiplication |
108
|
2009 |
SVM, RF, NB |
MDL Drug Data Report subset |
No |
2D, Shannon Entropy, Pharmacophoric |
Physicochemical property based phylogenetic tree |
Merger of ligand and target descriptors |
28
|
2009 |
SVM
|
117 Pubchem Targets |
No |
Topological Graph Based |
Sequence Similarity |
— |
25
|
2009 |
SVM
|
DrugBank |
Yes |
2D Graph vector |
Physicochemical property vector |
— |
109
|
2010 |
PLS |
Major Histocompatibility Complex Proteins |
No |
Sequential (Z-scales) |
Sequential (Z-scales) |
Multiplication |
39
|
2010 |
SVM, DT, NB |
Multi Target BindingDB data set |
No |
Physicochemical |
Sequence Similarity (through multiple descriptors) |
— |
44
|
2010 |
DT, NN, SVM, PLS |
Kinase Inhibitors
|
No |
Physicochemical, Geometrical, Molecular |
Sequential (Z-scales), Sequence Similarity (through multiple descriptors) |
Multiplication |
37
|
2010 |
SVM
|
Kinase inhibitors (ProLINT database) |
No |
2D Autocorrelation vectors |
Amino Acid Sequence Autocorrelation vectors |
— |
38
|
Likewise, Bock et al.29 modeled multiple GPCR families in a single model, a PCM based approach, which they applied to orphan GPCRs. Using a Support Vector Machine (SVM) learning approach they were able to separate a small group (2%) of highly active ligand–compound pairs from the bulk of their 1.9 million data point data set. As an extension of this work, Jacob et al.30 applied a PCM approach to the GLIDAGPCR database to predict the ligands of orphan GPCRs. They achieved a prediction accuracy of approximately 90%.
While GPCRs are quite amenable to PCM modeling due to their relationship, the same is true for mutants of enzymes. Hence, another target type that has been adequately covered in PCM modeling are viral proteins, such as HIV Protease,31,32 Dengue virus NS3 Protease33 and Influenza virus A and B Neuraminidase.34 The average similarity between these targets is very high when compared to the average similarity between multiple GPCR families. This is especially true in the case of HIV proteases, where the differences between targets may be a single amino acid.
In addition to these larger target groups, PCM has also been applied to antigen recognizing antibodies,35 matrix metalloproteinases,36 kinases37,38 and Major Histocompatibility Complex (MHC) proteins.39 The ligands for the targets PCM has been applied to included both small molecules and peptides. Furthermore, the output variables modeled by PCM models include classification,28,30 binding affinity20 and even equilibrium binding free energy.40 The nature of the data sets PCM has been applied to confirm the potential of PCM as a versatile technique suitable for any data set. Whether the targets are highly related or more dissimilar, a functional model that provides better extrapolation capabilities than QSAR can be created. However, it should be noted that the type of target description must be tuned to the nature of the data set.
3. Novel applications of PCM
Hit identification for orphan targets
The main advantage of the PCM extension of conventional QSAR modeling is that it allows the merging of data sets that describe the affinity to highly similar but not identical targets; hence it allows for the extrapolation of affinity modeling between these data sets. Thereby PCM can fulfil a need in hit identification for newly identified targets – including applications such as the prediction of polypharmacology or the deorphanization of receptors. PCM not only models similar data sets simultaneously, it also quantifies the distance between the different targets. Therefore it allows the scientist to estimate the reliability of any model prediction depending on the distance of both the ligand and target to the training set, through determining the applicability domain of the model.
Simultaneous modeling of orthosteric and allosteric ligands
PCM includes a target description in addition to the ligand descriptors; hence it is able to quantify the similarity between different binding sites. As a result it could be used for the simultaneous modeling of a series of orthosteric and allosteric41–43 ligands of a single target even though they act through a different binding site. In this case not two (or more) proteins are used for modeling the target side, but rather two binding sites of ligands that are in different parts of the protein which are able to accommodate completely different ligand chemistry. Previously it has already been shown that targets sharing a low similarity and accommodating a completely different chemistry can be modeled successfully with PCM.34,44
Capturing the chemical information of different types of ligands that act on different binding sites on a single target can provide advantages as the increase in information that serves as model input could lead to better extrapolation capabilities of a single target model. The single target model can in this case be seen as a two targets model. (Fig. 4)
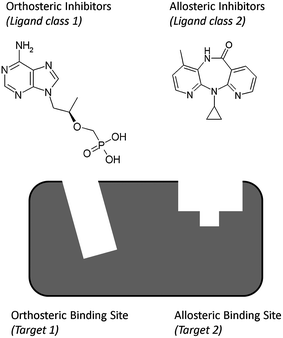 |
| Fig. 4 As an extension of current PCM models, a single PCM could also potentially be used to model both allosteric and orthosteric binders to a given target. Hereby a single target model is effectively turned into a multi target model, with the additional variable being introduced the binding site a given ligand binds to, this being particularly relevant in cases where a single chemical class of molecules can bind to each of the receptor subsites. Shown here are COMPOUND LINKS
Read more about this on ChemSpider
Download mol file of compoundTenofovir (an orthosteric HIV Reverse Transcriptase inhibitor) and Nevirapine (an allosteric HIV Reverse Transcriptase inhibitor). While modeling of this type has not been performed yet and its precise performance remains to be validated in the future, it still illustrates the ability of PCM models to incorporate not only ligand and target variables, but also additional variable types such as those of subpockets of a given protein target. | |
A second possible application is the simultaneous modeling of a drug regimen incorporating both nucleosidereverse transcriptase inhibitors (orthosteric) and non-nucleoside reverse transcriptase inhibitors (allosteric) for a single dominant HIV mutant in a patient (Fig. 4).
Finally, combining allosteric and orthosteric information in one model can provide advantages in preclinical research when looking for novel allosteric inhibitors or enhancers of proteins. Allosteric drugs have been shown to provide advantages in treatment by better resembling physiological signalling.45 Furthermore these models could come in useful when research is targeting a protein for which the orthosteric (natural) ligand is known and part of an essential physiological process. As this process cannot be completely disrupted (orthosteric inhibition) or continuously activated (orthosteric activation or agonism) the possibility of allosteric modulation is very promising here. The addition of the orthosteric inhibitors to this model could potentially detect cross reactivity with the orthosteric binding site at an early stage.
While to our knowledge this research has not been performed yet, it illustrates the versatility of PCM modeling approaches. PCM can cover a much larger bioactivity space than conventional QSAR models alone as well as, introduced here, multiple modes of action
4. Ligand descriptors
Ligand descriptors are merely numerical methods to describe either properties of or differences between the compounds to be modeled, therefore a large number of different descriptor methods for ligands are available. (For reviews see references 8, 10, 13, 46, 47) In this review article we will restrict our focus on ligand descriptors previously used in PCM. There is no optimal descriptor for all data sets and it is therefore wise to sample several different descriptors to identify the optimal descriptor for each setting.13 In the following we will start our discussion with the simplest descriptors used in PCM, namely binary descriptors.
Binary compound descriptors
Binary descriptors are descriptors based on one or more binary flags set to 1 or 0. These descriptors can be based on the differences between functional groups on one common scaffold. Lapinsh et al.19 implemented them in PCM by creating a table listing all possible combinations of three functional groups and assigning unique binary descriptors to each of those combinations. These descriptors are relatively simple and therefore computationally very fast; however results from the model have to be translated back to the functional group combination before model results can be interpreted. Furthermore predicting values outside the range of descriptors is not self-evident, so there is little possibility of numerical inter- and extrapolation.23,48 Therefore, the authors recommend not to use binary descriptors in PCM. Additionally, binary descriptors function on the assumption that it is already known which properties are relevant in the QSAR. Furthermore they are very sensitive to the creation of ‘ties’,49 namely the creation of equidistant similarity distances for non equal compound pairs. Binary descriptors are also more affected by possible overfitting of the data, since they do not allow a fuzzy interpolation of relevant groups.50 One-dimensional (1D) numerical (real-valued) descriptors resolve most of these problems and are better interpretable.
1D and Physicochemical compound descriptors
The main advantage of one-dimensional descriptors is that they are quickly calculated and modeled. Physicochemical descriptors are a subtype of these 1D descriptors, such as molecular weight, polar surface area or polarizability. When compared to binary descriptors, the usage of physicochemical descriptors increases the interpretability of the model and makes a translation step obsolete. The downside of this subtype of descriptors is the high number of possible descriptors that can be calculated for every compound. Therefore it is common to make a selection of descriptors that are important in each interaction model while also removing possible covariance in the descriptors. This goal can be achieved by preprocessing of the descriptors before a reliable model can be constructed, leading to the risk that only those descriptors are selected that are applicable to the training set on which the model is constructed. 1D descriptors have previously been applied successfully in PCM20 allowing the creation of a predictive and highly interpretable model (reaching an R2 of 0.92).
2D Topological compound descriptors
Topological descriptors are two dimensional representations of compounds, visualizing bond properties, atomic properties and the inner atomic distances between the functional groups. These descriptors can be transformed into molecular graphs, a method widely used in substructure searching and clustering. In graph descriptors, the atoms form the nodes of the molecular graph and the bonds the edges.10 Advantages of this type of descriptors are that they are relatively quickly computed and easily interpreted, but a downside can be that they lack three-dimensional (3D) information. (for a recent review see Van der Horst et al.51) To the knowledge of the authors these descriptors have only been used in a PCM-like approach by Ning et al.25 Their particular graph-based descriptor performs quite well and has previously been shown to perform comparably to 2D circular fingerprints,48 which are mentioned below.
2D Circular fingerprints
Descriptors based on 2D fingerprints convert the two dimensional information of the compound structure into a linear binary string. This class of descriptors is overall similar to 2D graph-based approaches, however it tends to be computationally much faster.10 These 2D fingerprints are constructed from several substructures present in the compounds while the substructures are limited by a radius defined by a certain number of covalent bonds.52,53 Circular fingerprints have previously been found to capture a large amount of information and to provide a high retrieval rate while at the same time being chemically interpretable as individual (localized) features.52,54 In recent studies the authors have been applying circular fingerprints in PCM models of HIV Reverse Transcriptase55 producing models with an average prediction error of 0.5 log units.
Alignment based 3D compound descriptors
3D descriptors preserve more information of the compounds by adding information concerning the conformation of the compound.56 However, most 3D descriptors require superposition of the compounds in their active conformation in 3D space. Only then useful information can be obtained, making the calculation more complex. The process of compound superposition is error prone and can introduce more noise than functional information,57 a step which can be avoided by using internal distances between atoms or surface points of a compound. This translation preserves all possible pharmacophore triplets and quadruplets, features of the compound and inter-feature distances but simplifies the calculation step.10 The increase of information in these descriptors also increases their size and consequently the calculation times of the models. To the knowledge of the authors, 3D descriptors have as yet not been used in PCM and the previously mentioned disadvantages might support the usage of GRINDs instead (see next section).
Grid independent descriptors
Grid Independent Descriptors (GRINDs) are descriptors that are obtained starting from a set of molecular interaction fields using different probes. This procedure involves a first step, simplifying the fields, and a second step, encoding the fields into alignment-independent variables using an autocorrelation transform.58 The obtained descriptors can also be used to provide graphical diagrams and the original descriptors can be regenerated from the transformation in order to visualize the results of the analysis graphically in 3D. As one of few 3D descriptors GRINDs have previously been used in PCM11,59 with a prediction error of around 0.5 log units on data sets of GPCRs. This confirms the compatibility of PCM with GRINDs on this data set. In this case, the GRIND descriptors were preprocessed using principal component analysis (PCA), a step to handle the high dimensionality common to many descriptor spaces.11
5.
Protein descriptors
The main difference between ligand and protein descriptors is that the protein is, in general, a larger structure to describe and hence in most cases a selection of a subset of the residues (such as those lining the binding site) would be recommended. This selection can be made on the basis of a crystal structure if available, on data from mutational experiments or from an information-based bioinformatics analysis, e.g. a two-entropy analysis.60
At the level of the residues, a distinction can be made between descriptors that describe a (sub)structure or general property of the protein and those that stand for properties of individual amino acids on a sequential basis. Protein descriptors have been reviewed extensively10,61 and only methods that have been previously used in PCM will be highlighted here.
Binary protein descriptors
Similar to binary ligand descriptors, binary description of proteins can be performed based on several binary flags corresponding to different substructures of the protein. Although the make-up of the data set dictates the binary method used for the description and hence the length of the descriptors, binary descriptors are generally fast from a computational point of view. An example of a binary descriptor in PCM is given by Kontijevskis et al. who created several chimeric proteins divided into five segments based on building blocks obtained from four different receptors.62 Thereby every segment was described by four binary descriptors and every protein by five segments, leading to each protein being described by 20 binary descriptors and to a unique descriptor for every protein. When directly compared with a sequential protein descriptor the binary descriptors (Root Mean Squared Error (RMSE) 0.61) outperformed the sequential descriptors (RMSE 0.76). However, in this form it is far less interpretable than sequential descriptors and limited in extrapolation capabilities.
A related subtype of binary protein descriptors is a feature-based semi-binary protein descriptor. In this descriptor each individual amino acid gets assigned a unique identifier resulting in a unique descriptor for each sequence.55 The final model is then trained on the collection of unique identifiers per sequence. The authors found this type of descriptors to outperform sequential descriptors, as is the case with the previously mentioned binary descriptors. The main advantage of these descriptors is that they are better interpretable than the binary descriptors as important residues can be individually identified rather than identifying an entire important subsection of a protein.
3D Protein descriptors
While the descriptors used above encode only 1D properties of protein sequences, the targets are three-dimensional entities and this information can also be used in PCM modeling. Considering multiple mutants of HIV Reverse Transcriptase in parallel, Van Westen et al. used protein energy fields as descriptors for the investigation of complexes between mutant forms of HIV-RT and different ligands.63 This appeared very useful in understanding the molecular mechanism and in suggesting novel chemistry ideas for anti-resistant inhibitor design. A 3D protein descriptor taking into account Cα coordinates and φ/ψ angles is introduced by Lindström et al.64 Alignment problems and the large number of variables emerging from these descriptors were circumvented by preprocessing the data by PCA and covariance transformations. Lindström et al. show that this form of descriptor contains sufficient information to generate both global and sub-class specific PCM models.64
Contrary to full protein 3D descriptors, an example of a (local) 3D protein descriptor was introduced by Hvidsten and Kryshtafovych.65,66 Here 3D substructures are a collection of continuous short backbone fragments centered on a single residue within a predefined radius. These descriptors provide a highly generalized descriptor of the protein binding pocket and can therefore be used between completely different classes of proteins without the need for a multiple sequence alignment. They have been applied to PCM modeling of a very diverse target library of PDB structures65 by Strombersson et al.34,67 Performance was limited (cross validation prediction error of 1.7 log units), although it should be taken into account that this was a very diverse data set. We conclude that these descriptors can be especially useful when building a PCM model of a diverse data set for which 3D structures are available.
Sequential protein descriptors
As for nearly all possible drug targets (human proteins) the amino acid sequence is available, one can use information derived from this sequence as a protein descriptor. Jacob et al.30 employed this information to create several similarity measures. In this case a hierarchical tree of the sequences guided a division in classes and through these classes a similarity measure of the binding pockets is obtained. They showed that using sequence as a protein descriptor enables the creation of a PCM model, obtaining a prediction accuracy of 90%.30 This performance can most likely be improved by converting a purely sequence based protein descriptor to a sequential descriptor of physicochemical properties of the amino acids, as described below.
Amino acids can also be described according to their physicochemical properties, like descriptors of small molecules. Several descriptor scales are available from the field of peptidedrug modeling.68–71 In PCM, the ‘z-scales’ introduced by Sandberg et al. that describe the properties of the amino acids, have been shown to perform well in many cases.19,64 (Fig. 5) The z-scales are based on a PCA used to compress a large input matrix that describes a broad selection of physicochemical properties into 5 principal components (PCs). In a plot of PC1versusPC2, similar amino acids are located close together and dissimilar amino acids are spread further apart (Fig. 6). PC1 can be interpreted as a lipophilicity scale and PC2 as volume/polarizability scale.68 PC3 mainly describes polarity while PC4 and PC5 are more difficult to interpret. It has been shown that these sequential descriptors can predict contributions to selectivity without 3D information available20 or predict the site of origin of indirect effects on ligand recognition by proteins.21 One possible problem with the z-scales or similar descriptors is the slightly limited interpretability since these descriptors are obtained through a data reduction step performed on an initially large matrix. Furthermore this initial data matrix contains far more amino acids than the 20 natural amino acids needed for the creation of PCMs, and therefore the descriptors might not perform optimally within the 20 natural amino acid space needed for description of protein targets. The authors are currently working on a novel protein descriptor that eliminates some of the issues described above.
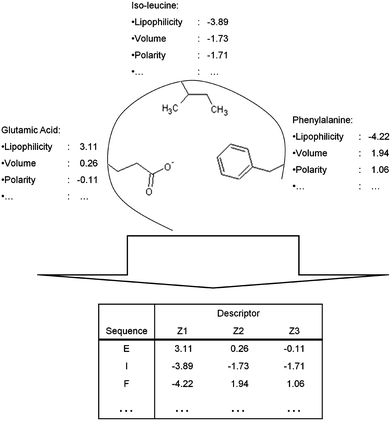 |
| Fig. 5 Conversion of physicochemical properties of amino acids in the binding site into a protein descriptor via sequential protein descriptors using the Z-scales as an example. Based on the physicochemical properties of the amino acids side chains a protein descriptor is constructed. A trained PCM model can subsequently compare the binding sites of the proteins based on the differences in the physicochemical properties of the side chain. | |
6. Cross terms
Descriptor cross terms are used to allow linear machine learning methods like Partial Least Squares (PLS) to model the non-linear interactions that guide ligand-target interactions,72,73 which seem to be present in at least half of all structure–activity data sets.74 Cross terms are influenced by both the ligand and the target part of the data set and they are intended to model particular interactions between the ligand and the target, such as charge interaction sets.19,22 However, when using non-linear machine learning methods in our work in practice, we found that cross terms may in fact deteriorate model performance, likely due to the introduction of a large number of additional parameters, so it is often beneficial to leave them out completely.55 In their simplest forms, cross terms describe the similarity between different compound – target pairs; therefore they can be constructed as any similarity measure.
One problem with cross terms is that their functional form is undefined, and they can be any function of ligand and target properties. In practice often properties of the ligand and target are multiplied.19,62 However, this step follows more the intuition of the user than any thorough theoretical derivation. (For a comprehensive overview of previously used cross terms see Table 1.) Variable selection, mentioned under data pre-processing, can be applied to descriptors prior to cross term calculation when the calculation of all possible cross terms is too time consuming or computationally infeasible.14,34
A second and completely different approach to cross term calculation is provided by Lindstrom et al.64 They applied PCM to model a data set that includes structural protein information, while using the target – ligand interactions as a cross term. The interactions were limited to steric and electrostatic complementarity, the ligand strain energy, logP (COMPOUND LINKS
Read more about this on ChemSpider
Download mol file of compoundoctanol/COMPOUND LINKS
Read more about this on ChemSpider
Download mol file of compoundwater partition coefficient), steric fit, complementary surface area interactions and the number of rotatable bonds in the ligand as described by Head et al.75 Although their final global model had a prediction error of approximately 1.7 log units, the authors showed that any property dependent on both target and ligand features can be used as a cross term.
Weil and Rognan28 extend this work by compressing the ligand and target descriptors to a single fixed length bit-string. They described the ligands and targets by a combination of different descriptors and subsequently they merged both the ligand and target bit-strings into one single protein-ligand fingerprint (PLFP). Thereby they used a single descriptor consisting of a ligand and target part to describe the data set, effectively constructing a model on only the cross term. The authors obtain predictive models with average ROC values of around 0.80 with these descriptors and a variety of machine learning techniques.28
In conclusion a cross term can be any descriptor that depends on properties from the compound and properties from the target. As long as this condition is met there is no difference if the cross term is obtained by mathematically combining the compound and target descriptors (e.g. by multiplication, addition or exponentially) or if the cross term is obtained by introduction of an independent descriptor. As far as the authors know, the different mathematical operators to obtain cross terms have not been thoroughly researched, therefore this provides some interesting research opportunities.
7. Data pre-processing
Before a viable PCM model can be constructed, the data should usually be pre-processed allowing it to be compatible with the machine learning technique of choice and to obtain optimal model performance. Depending on the data and machine learning technique this processing can be very extensive or relatively simple. Here we will provide an overview of data preprocessing steps, with applications in PCM modeling in mind.
Scaling and mean centering
When a PCM model is created using multiple descriptors (descriptor blocks), it should be prevented that a biased model is created, wherein a subset of descriptors mask the influence of the other descriptors. Scaling and mean centering the different descriptors prevents this bias, makes them compatible and is especially necessary when using PLS modeling.76 In block scaling one block of descriptors is scaled according to:Here, COMPOUND LINKS
Read more about this on ChemSpider
Download mol file of compoundNb is the number of descriptors in block b. Block scaling prevents larger blocks to mask small ones26,62 and it is often incorporated in modern modeling tools. In the case of PCM modeling mean centering is always advised.
Covariance removal
To prevent overfitting of the model, which is likely when PLS is used,77 it is important to remove covariance within the descriptor blocks before the final model is built. Covariance can lead to misinterpretations of the final model and poor extrapolation capabilities, and it increases the dimensionality of the model with no apparent benefit. Several methods are available for removal of covariance and determination of the descriptors that best describe the data. Two of the covariance removal methods previously used in PCM will be highlighted, namely variable extraction (e.g.PCA) and variable selection. For a general review about the underlying principles and methods see Wegner et al.78
An example of variable extraction is PCA, a method to find the underlying latent variables present in a data set, e.g. in a set of descriptors. The original multidimensional space defined by the descriptors is summarized by a smaller number of descriptive dimensions which describe the main variation in the data; these are called the principal components.79 PLS is the regression extension of PCA and provides the ability to construct a model based on the extracted principal components. PCA can be used when modeling of the original data set is infeasible14,62 due to reasons such as a high dimensionality of the data set. However, at the same time the interpretability of the model is usually decreased.
Variable selection
Variable selection is the selection and exclusion of variables with negligible importance for the data to be modeled. It is also known as Variable importance projection (VIP) or variable subset selection (VSS). When building a PCM model using PLS, the reliability is heavily influenced by the variable selection before model training. In the case of PLS it can therefore be seen as both a data preprocessing and model tuning procedure.80 Variable selection is an iterative process aimed at selecting a subset of variables from the full set that optimally describes the variance of the data set. Two possible forms of variable selection exist.81 Backward selection consists of iterative elimination starting from the full set, and forward selection consists of iterative addition starting from a single variable. To our knowledge in PCM only backwards selection has been previously used. The iterative process proceeds as follows, firstly a model is constructed on the full data set, subsequently descriptors with negligible importance are removed and a new model on the improved descriptor set is built.19,79,80 This procedure can be repeated until variable selection leads to model deterioration. In models where many variables receive low weights, the variable selection can significantly improve the model.82
8. Modeling techniques in PCM
Apart from statistical methods, both linear machine learning techniques and non-linear machine learning techniques can be and have been used in PCM. We will describe several approaches, starting with modeling learning techniques already used in PCM and subsequently including new suggestions. We will highlight positive and negative consequences of the different techniques, a short summary of which is given in Table 2.
Table 2 Modeling techniques previously used, and which can potentially be used, in proteochemometrics modeling with their main advantages and disadvantagesa
Technique |
Linear? |
Previously used in PCM? |
Advantage |
Disadvantage |
Explanation of abbreviations: Partial Least Squares (PLS), Rough Set (RS), Neural Net (NN), Support Vector Machines (SVM), Naïve Bayesian (NB), Random Forest (RF), Decision Tree (DT), Gaussian Processes (GP).
|
PLS |
Linear |
Yes |
Highly Interpretable |
Requires Cross-Terms |
RS |
Non-linear |
Yes |
Highly Interpretable |
Classification |
NN |
Non-linear |
Yes |
Performs well on complex data |
High Dimensionality |
SVM
|
Non-linear |
Yes |
Very Robust on complex data |
Poorly Interpretable |
NB |
Linear |
Yes |
Performs well on complex data |
Requires Cross-Terms |
RF |
Non-linear |
Yes |
Low risk of Overfitting |
— |
DT |
Non-linear |
Yes |
Highly Interpretable |
Variable performance |
GP
|
Non-linear |
No |
Confidence Estimate |
Long training time |
Partial least squares
By far the most commonly used modeling technique in PCM is PLS.77 PLS is the regression extension of PCA and specifies the relationship between an output variable Y and a set of predictor variables Xi. The final model is able to display the role of the individual predictor variables in the model. Advantages of PLS are that it is highly interpretable and requires low computational expense. A large number of PCM models have been created founded on PLS with a prediction error usually ranging between 0.4 and 0.8 log units. Targets for which PLS-PCM models were constructed include melanocortin receptors,20 HIV Proteases32 and dengue virus NS3 proteases.33 However, as PLS is linear it requires cross terms to be calculated in order to obtain an optimal model21,22,26,59,62,82,83 as opposed to rough set modeling which alleviates this restriction.
Rough set modeling
Non-linear Rough Set (RS) modeling has been introduced to PCM by Strömbergsson et al.28,35 as they proposed that RS might be capable of modeling data to a higher level than PLS because of its non-linearity. RS constitutes a mathematical framework that induces basic IF-THEN decision rules to classify a compound – ligand pair as active or inactive,27 therefore these models are highly interpretable. However, as RS is a classification tool, RS is unable to perform regression and provide a numerical value, e.g. an affinity (pKi) value. RS modeling has been applied to melanocortin and adrenergic receptor data sets27 as well as a data set containing a very broad target collection obtained from the PDB.34 RS modeling performed very well with an area under the retrieval curve (ROC) usually above 0.9, reliably distinguishing between active and inactive on a particular target. However, while the non-linear RS cannot be used in order to model a numerical output variable rather than a class, SVM as described in the following section is a non-linear machine learning technique that is capable of both classification and regression.
Support vector machines
SVM
84 is a non-linear modeling technique also applied multiple times in PCM.24,25,55,67 The main advantage of this machine learning technique is that it has been proven to be very robust and very capable of modeling QSAR data sets,85 especially in the case of many dimensions.86 The disadvantage of SVMs at the moment is the degree to which the models can be interpreted. However, a recent paper by Carlsson et al.87 introduced an approach to improve the interpretation capabilities. When these interpretation methods improve they might lead to SVM models being the machine learning technique of choice in PCM models. Three publications are available that describe PCM modeling based on SVMs. The first one is by Strömbergsson et al.67 who model the entire Enzyme-Ligand interaction space. Although the absolute performance of the PCM model is not very accurate with a prediction error of 1.5 log units, the authors were able to model a very diverse data set. The second publication by Geppert et al.24 showed recovery rates of active compounds from a database of around 60–70%, leading to the conclusion that SVM can successfully extrapolate from a combination of ligand and target information to retrieve new active compounds on a related target. This conclusion is supported by Ning et al.,25 who also used multiple assay based models to gain improved performance compared to single assay models. We share a similar view, having used SVM based PCM models to model an COMPOUND LINKS
Read more about this on ChemSpider
Download mol file of compoundadenosinereceptor data set and an HIV Reverse Transcriptase inhibitor data set. (manuscript in preparation) The SVM models we generated were able to model the data with a prediction error of around 0.5 log units. In conclusion SVM is a robust machine learning technique capable of modeling the complicated PCM data. However, it should be noted that SVM specific parameters like ‘gamma’ and ‘cost’ must always be optimized through a proper model selection, for instance by cross validation.88
Neural net modeling
A well known previously used machine learning technique is neural network (NN) modeling. Fernandez et al.89 have applied NN to PCM modeling using a Bayesian Regularized form of NN. NNs are known for their ability to handle complex input-output relationships and provide robust models of non-linear data. For an extensive review on NN please see Grossberg et al.90 In PCM NNs would not be an optimal machine learning technique since NNs often possess too many parameters for this purpose (as an often mentioned very approximate rule of thumb, when training NNs one should have at least three times more datapoints than variables). In PCM modeling the number of variables is much larger than in QSAR as PCM requires two variables per input dimension. Fernandez et al. used a simplified correlation matrix as ultimate input descriptor, keeping the number of input variables low and using Bayesian Regularization to diminish the inherent complexity of the NN. Therefore their model output requires a translation to the original descriptors before the results can be interpreted. Furthermore, NNs are not easily interpretable by themselves. However, Browne et al.91 have recently described ways of improving the interpretability. A much better interpretable modeling technique known from QSAR models is a Naïve Bayesian classifier. The possibility of using this classifier in PCM models will be described below.
Naïve Bayesian classifier
Naïve Bayesian (NB) classification models have been shown to be able to model data sets with a high number of variables and relate bioactivity predictions from multiple activity classes with each other.92 These two qualities make Bayesian classification another suitable machine learning technique for PCM. However, as Bayesian classification is linear, cross terms might be required to allow confident modeling. Two publications on PCM with a Naïve Bayesian (NB) classifier have appeared, one by Weil and Rognan28 and one by Strömbergsson et al.44 However, in the former the model is created merely on cross terms as descriptors and in the second publication no cross terms are used. We speculate that an NB model will reach a better performance and interpretability when separate ligand, protein and cross term descriptors would be used.
Decision trees algorithm
The decision trees (DT) algorithm has been known from the creation of QSAR models. The decision based output makes a decision tree highly interpretable. The DT algorithm has been applied to PCM by Lapins et al.37 and by Strömbergsson et al.44 In the former publication their performance is somewhat disappointing with a squared correlation coefficient of 0.45, which puts it slightly below PLS. The authors contribute this performance to the high non-linearity of the data set. In the latter publication by Strömbergsson et al. the performance of DT, as a classifier, is superior to both SVM and NB based classification models, with an ROC score of 0.84 and an accuracy of 82%. It can therefore be concluded that the performance of DT can vary with the data set and that further research is required.
Random forest
Random forest modeling techniques93,94 which can be used for both classification and regression, have previously been shown to perform well on QSAR data sets.95 Weil and Rognan28 have also applied this technique to PCM data. In their paper RF performs very well on a number of data sets with a recall larger than 0.5 and precision value higher than 0.7 on average, on average RF performs roughly equal to SVM. However, since RF can provide the following additional features: built-in performance assessment, a measure of relative importance of descriptors, and a measure of compound similarity that is weighted by the relative importance of descriptors, RF might very well be a better choice for usage in PCM models than SVM with its low interpretability.
In conclusion, all machine learning methods that have currently been applied to PCM have their advantages and disadvantages, and none can be considered a universal optimal approach. It might therefore be interesting to apply established machine learning methods that have previously not been used in PCM. One suggestion will be discussed below.
Possible new machine learning techniques to be applied in PCM
One of the most promising machine learning techniques not yet applied in PCM are Gaussian Processes (GP).96 The non-linear GP will not only provide the scientist with a prediction of the output variable but also a measure of reliability for this prediction in the form of variance estimation for each prediction. This quality makes it invaluable in PCM as it directly links the application domain to model predictions and allows the selection of the most reliable predictions for decision making. Previously GPs have been shown to perform very well in the modeling of ADME and physicochemical properties.97,98 Although the training time required surpasses that of the linear PLS technique, the superior performance combined with the prediction confidence parameter tips the scales in favor of GP.
9. Validation of a PCM model
One of the key differences between PCM and QSAR is that PCM significantly increases the number of variables to be modeled as the descriptor space is increased. Therefore there is an increased risk of both chance correlations and model overfitting.82 The modeler should consequently take care to rule out the possibility of a model built on chance correlations. Validation in PCM is based on established validation techniques normally applied in QSAR modeling. The key goal is to get a reliable estimate of the model quality and applicability. This goal can be achieved by calculation of the correlation coefficient, coefficient of determination and RMSE. For an overview of validation techniques please see a set of recent comprehensive publications.99–102
Y-scrambling
Y-scrambling, or response permutation testing is an approach to estimate the risk of chance correlations.99,103 Y-scrambling consists of keeping the X-variables, or the descriptor space, fixed and to randomly shuffle the output or Y-variable and subsequently retraining the model. A typical approach is to create 100 random models and to assess their performance using standard validation parameters and to compare this performance to the actual model, where the performance of the proper model should be significantly higher (for details see above references). Due to the highly increased variable space that a PCM is founded on, this validation technique is very relevant and should always be applied to validate the final model.
Internal validation
Internal validation or cross validation is used to estimate the ability of the model to reliably predict the activity of the data points used in the training set. There are three forms of cross validation, namely Leave-One-Out (LOO)) cross validation, n-fold cross validation and double loop cross validation.82 Double loop cross validation was introduced by Freyhult et al.82 to improve the quality of cross validation performance estimates and prevent overoptimistic assumptions. Two independent loops are used to tune model parameters in the inner loop and provide a performance estimate P2 in the outer loop. Here we will only consider n-fold cross validation as it is currently the state of the art.104 In n-fold cross validation the total training set is divided into ‘n’ equal subsets. Subsequently a model is trained on n − 1 of the subsets and used to predict the activity of the data points in the remaining subset. This process is repeated until all subsets have been left out of the training and the plots of these iterations are used to calculate q2 and cross-validated RMSE.105 Cross validation, while useful, cannot always reliably be used to estimate the performance of the final model on unknown data points as it has been shown that there is no direct correlation between q2 and R2.56,105 However, cross validation provides a very useful framework for tuning modeling parameters like the number of components in PLS and the values for ‘gamma’, ‘epsilon’ and ‘cost’ in SVM.62,85,88
External validation
In external validation a trained model is used to predict the output variable for a set of data points for which an observed activity value is available. These points have been separated from the original data set prior to model training (as well as selection!) or are assembled only after the model has been constructed, and hence they and are completely unknown to the model. Separation of this so called test-set from the training set can be performed using a wide extent of different parameters. (For a full review see Tropsha et al.99) After model training, the performance of the model on the test set is estimated using conventional validation parameters. The main goal of this exercise is to provide a more reliable performance estimate than internal validation to assess the quality of model predictions on a data set of unknown compounds. This performance estimation becomes even more critical when using any sort of model selection,106e.g. feature selection. Otherwise there is a considerable risk that models will become overfitted as we reported earlier,107 and is explained by Wegner et al.78
Prospective validation
The only true validation for any computational model is prospective validation. Prospective validation assesses model performance by experimentally determining compound activity subsequent to model predictions. Unfortunately in the field of PCM not much work has been published containing a prospective validation. Currently, to the knowledge of the authors, only three publications contain prospective validation of modeling results. The first prospectively validated PCM model was constructed on a data set consisting of melanocortin wild-type and chimeric receptors and their ligand α-MSH (and synthetic analogues).21 In this publication a small scale prospective validation was performed in which PCM predicted a correct response of the binding affinity to point mutations in 80% of the datapoints. The second published prospective validated PCM model was constructed on a HIV protease data set (containing point mutations in the protein that change binding affinity) and a selection of octapeptides as ligands.108 Here PCM was able to prospectively predict the affinity of 10 peptides on 4 different protease mutants with an R of 0.63 (R2 of 0.40). Lastly, a prospectively validated PCM modeling study was published by Nagamine et al.109 They used an SVM based model to find androgen receptor ligands from a pool of 19 million compounds while iteratively prospectively validating model predictions. With their model they obtained an area under the ROC curve of 0.717 compared to 0.558 for QSAR. These results firmly establish PCM as a reliable modeling technique with applications in preclinical research.
10. Pitfalls and disadvantages
In any form of statistical modeling there are a large number of possible pitfalls which are caused by the modeling technique, data set preparation or can be the result of a simple bias. An extensive review on risks in statistical modeling has been published by Gedeck et al.;13 here we will focus on specific dangers that can arise when performing PCM.
The main risk present in PCM has also been discussed under validation, and is the fact that the large increase in descriptor variables increases the risk of chance correlation models. This should at all times be kept in mind and an extensive validation (including cross validation, Y-scrambling and prospective testing) is indicated for all PCM models.
A second pitfall comes from the fact that the data to be modeled by PCM is inherently non-linear, whereas many machine learning techniques in QSAR rely on (multiple) linear regression. Therefore the data should be modified in many cases by the introduction of cross terms. The risk of cross terms is that they will account for most of the described variance, as cross terms describe variance of the ligands and the targets simultaneously. If this is the case, cross terms can mask the contributions of the pure compound or protein descriptors. Furthermore, since they rely on both compound and protein contributions, cross terms are not always readily interpretable;27 however, this depends to a significant extent on the precise way in which cross terms are constructed. When using cross terms one needs to ensure that both compound and protein descriptors are compatible, which is not always the case. A workaround that circumvents the use of cross terms, can be the use of non-linear machine learning techniques; however their low interpretability might lead to highly accurate but non-interpretable black box models. Currently research efforts are underway to alleviate this problem,87,91 but for now a decision needs to be made in many cases between better interpretable models or more accurate models. As mentioned above, it also needs to be ensured that cross terms indeed improve model performance, which was not always the case in the experience of the authors.
A disadvantage of PCM is that the calculation time of the models increases compared to QSAR when protein descriptors are added. Depending on the machine learning technique this increase in calculation time can be small, as in PLS, or exponential, as in radial basis function based SVMs.110
11. Conclusions
PCM is a relatively new technique that, by including target descriptors in addition to ligand descriptors, enables modeling of data sets that could previously only be modeled separately using conventional QSAR based techniques. PCM has been applied successfully to a variety of targets, among which are GPCRs, Viral Proteins and Cytochrome P450 enzymes. However, relatively few of those studies have included prospective validations – with the notable exceptions of the studies by Prusis et al.,21 Kontijevskis et al.108 and Nagamine et al.109 Hence, while limited data exists, the authors are of the opinion that PCM modeling should indeed, in many cases, be able to make better use of bioactivity data of molecules than previous QSAR models.
In conclusion, by more comprehensively exploiting the information contained in data sets that were previously considered separate, PCM models allow for improved extrapolation both on the ligand side (by taking more ‘chemistry’ into account) as well as on the target side (by incorporating the relationship between targets into the model). This enables applications in areas such as the deorphanization of compounds or the selection of compounds that are selective, or show a desired bioactivity profile. Until the current stage few prospective PCM studies are available in the literature. However PCM is one of the areas where other research groups are currently active, and where a large-scale validation will be published also by the authors very shortly.
Acknowledgements
The authors would like to thank Olaf O. van den Hoven for his supporting work and discussions. GJPvW thanks Tibotec BVBA for funding.
References
- H. Meyer, Naunyn-Schmiedebergs Arch. Pharmacol., 1899, 42, 109–118.
- E. Overton, Jena, Gustav Fisher, 1901, 45, 195 Search PubMed.
- C. Hansch and T. Fujita, J. Am. Chem. Soc., 1964, 86, 1616–1626 CrossRef CAS.
- C. Hansch, Acc. Chem. Res., 1969, 2, 232–239 CrossRef CAS.
- D. E. Clark, Expert Opin. Drug Discovery, 2006, 1, 103–110 Search PubMed.
- J. A. DiMasi, R. W. Hansen and H. G. Grabowski, J. Health Econ., 2003, 22, 151–185 CrossRef.
- A. Bender and R. C. Glen, J. Chem. Inf. Model., 2005, 45, 1369–1375 CrossRef CAS.
- A. Bender and R. C. Glen, Org. Biomol. Chem., 2004, 2, 3204–3218 RSC.
- T. Klabunde, Br. J. Pharmacol., 2007, 152, 5–7 CrossRef CAS.
- D. Rognan, Br. J. Pharmacol., 2007, 152, 38–52 CrossRef CAS.
- M. Lapinsh, P. Prusis, I. Mutule, F. Mutulis and J. E. S. Wikberg, J. Med. Chem., 2003, 46, 2572–2579 CrossRef CAS.
- L. M. Kauvar, Bio/Technology, 1995, 13, 965–966 CrossRef CAS.
- P. Gedeck, B. Rohde and C. Bartels, J. Chem. Inf. Model., 2006, 46, 1924–1936 CrossRef CAS.
- M. Lapinsh, P. Prusis, S. Uhlen and J. E. S. Wikberg, Bioinformatics, 2005, 21, 4289–4296 CrossRef CAS.
- A. F. Fliri, W. T. Loging, P. F. Thadeio and R. A. Volkmann, J. Med. Chem., 2005, 48, 6918–6925 CrossRef CAS.
- R. Guha and J. H. VanDrie, J. Chem. Inf. Model., 2008, 48, 646–658 CrossRef CAS.
- M. Wawer, L. Peltason and J.r. Bajorath, J. Med. Chem., 2009, 52, 1075–1080 CrossRef CAS.
- J. L. Medina-Franco, K. Martinez-Mayorga, A. Bender, R. M. Marin, M. A. Giulianotti, C. Pinilla and R. A. Houghten, J. Chem. Inf. Model., 2009, 49, 477–491 CrossRef CAS.
- M. Lapinsh, P. Prusis, A. Gutcaits, T. Lundstedt and J. E. S. Wikberg, Biochim. Biophys. Acta, Gen. Subj., 2001, 1525, 180–190 CrossRef CAS.
- M. Lapinsh, S. Veiksina, S. Uhlen, R. Petrovska, I. Mutule, F. Mutulis, S. Yahorava, P. Prusis and J. E. S. Wikberg, Mol. Pharmacol., 2005, 67, 50–59 CrossRef CAS.
- P. Prusis, S. Uhlén, R. Petrovska, M. Lapinsh and J. E. Wikberg, BMC Bioinformatics, 2006, 7, 167 CrossRef.
-
J. E. S. Wikberg, F. Mutulis, I. Mutule, S. Veiksina, M. Lapinsh, R. Petrovska and P. Prusis, in Melanocortin System, ed. D. Braaten, New York, 2003, pp. 21–26 Search PubMed.
- E. Van der Horst, J. E. Peironcely, G. J. P. Van Westen, O. O. Van den Hoven, W. R. J. D. Galloway, D. R. Spring, J. K. Wegner, H. W. T. Van Vlijmen, A. P. Ijzerman, J. P. Overington and A. Bender, Curr. Top. Med. Chem., 2010 Search PubMed In Press.
- H. Geppert, J. Humrich, D. Stumpfe, T. Gaertner and J. Bajorath, J. Chem. Inf. Model., 2009, 49, 767–779 CrossRef CAS.
- X. Ning, H. Rangwala and G. Karypis, J. Chem. Inf. Model., 2009, 49, 2444–2456 CrossRef CAS.
- M. Lapinsh, P. Prusis, R. Petrovska, S. Uhlen, I. Mutule, S. Veiksina and J. E. S. Wikberg, Proteins: Struct., Funct., Bioinf., 2007, 67, 653–660 Search PubMed.
- H. Strombergsson, P. Prusis, H. Midelfart, M. Lapinsh, J. E. S. Wikberg and J. Komorowski, Proteins: Struct., Funct., Bioinf., 2006, 63, 24–34 Search PubMed.
- N. Weill and D. Rognan, J. Chem. Inf. Model., 2009, 49, 1049–1062 CrossRef CAS.
- J. R. Bock and D. A. Gough, J. Chem. Inf. Model., 2005, 45, 1402–1414 CrossRef CAS.
- L. Jacob, B. Hoffmann, V. Stoven and J.-P. Vert, BMC Bioinformatics, 2008, 9, 363 CrossRef.
- M. Lapins, M. Eklund, O. Spjuth, P. Prusis and J. Wikberg, BMC Bioinformatics, 2008, 9, 181 CrossRef.
- M. Lapins and J. E. S. Wikberg, J. Chem. Inf. Model., 2009, 49, 1202–1210 CrossRef CAS.
- P. Prusis, M. Lapins, S. Yahorava, R. Petrovska, P. Niyomrattanakit, G. Katzenmeier and J. E. S. Wikberg, Bioorg. Med. Chem., 2008, 16, 9369–9377 CrossRef CAS.
- H. Strombergsson, A. Kryshtafovych, P. Prusis, K. Fidelis, J. E. S. Wikberg, J. Komorowski and T. R. Hvidsten, Proteins: Struct., Funct., Bioinf., 2006, 65, 568–579 Search PubMed.
- I. Mandrika, P. Prusis, S. Yahorava, M. Shikhagaie and J. Wikberg, Protein Eng., Des. Sel., 2007, 20, 301–307 CrossRef CAS.
- B. Pirard and H. Matter, J. Med. Chem., 2006, 49, 51–69 CrossRef CAS.
- M. Lapins and J. Wikberg, BMC Bioinformatics, 2010, 11, 339 CrossRef.
- M. Fernandez, S. Ahmad and A. Sarai, J. Chem. Inf. Model., 2010, 50, 1179–1188 CrossRef CAS.
- I. Dimitrov, P. Garnev, D. R. Flower and I. Doytchinova, Eur. J. Med. Chem., 2010, 45, 236–243 CrossRef CAS.
- J. R. Bock and D. A. Gough, Mol. Cell. Proteomics, 2002, 1, 904–910 CrossRef CAS.
- A. Christopoulos, Nat. Rev. Drug Discovery, 2002, 1, 198–210 CrossRef CAS.
- Z. G. Gao, Mini-Rev. Med. Chem., 2005, 5, 545 CrossRef CAS.
- V. J. Merluzzi, K. D. Hargrave, M. Labadia, K. Grozinger, M. Skoog, J. C. Wu, C. K. Shih, K. Eckner, S. Hattox and J. Adams,
et al.
, Science, 1990, 250, 1411–1413 CrossRef CAS.
- H. Strömbergsson, M. Lapins, G. J. Kleywegt and J. E. Wikberg, Molecular Informatics, 2010, 29, 499–508 Search PubMed.
- W. Soudijn, I. van Wijngaarden and A. P. Ijzerman, Drug Discovery Today, 2004, 9, 752–758 CrossRef CAS.
- A. Bender, J. L. Jenkins, J. Scheiber, S. C. K. Sukuru, M. Glick and J. W. Davies, J. Chem. Inf. Model., 2009, 49, 108–119 CrossRef CAS.
-
R. Todeschini and V. Consonni, Handbook of Molecular Descriptors, WILEY-VCH, Weinheim, 2000 Search PubMed.
- N. Wale, I. Watson and G. Karypis, Knowledge
and Information Systems, 2008, 14, 347–375 CrossRef.
- J. MacCuish, C. Nicolaou and N. E. MacCuish, J. Chem. Inf. Comput. Sci., 2000, 41, 134–146.
- D. M. Hawkins, J. Chem. Inf. Comput. Sci., 2003, 44, 1–12.
-
E. Van der Horst and A. P. IJzerman, in Fragment-Based Drug Discovery: A Practical Approach, ed. E. R. Zartler and M. J. Shapiro, John Wiley & Sons, Ltd, Chichester, West Sussex, U.K., 2008 Search PubMed.
- R. C. Glen, A. Bender, C. H. Arnby, L. Carlsson, S. Boyer and J. Smith, IDrugs, 2006, 9, 199–204 Search PubMed.
- D. Rogers and M. Hahn, J. Chem. Inf. Model., 2010, 50, 742–754 CrossRef CAS.
- A. Bender, H. Y. Mussa, R. C. Glen and S. Reiling, J. Chem. Inf. Comput. Sci., 2004, 44, 1708–1718 CrossRef CAS.
- M. R. Doddareddy, G. J. P. van Westen, E. van der Horst, J. E. Peironcely, F. Corthals, A. P. IJzerman, M. Emmerich, J. L. Jenkins and A. Bender, Statistical Analysis and Data Mining, 2009, 2, 149–160 Search PubMed.
- H. Kubinyi, F. A. Hamprecht and T. Mietzner, J. Med. Chem., 1998, 41, 2553–2564 CrossRef CAS.
- T. Scior, J. L. Medina-Franco, Q. T. Do, K. Martinez-Mayorga, J. A. Yunes Rojas and P. Bernard, Curr. Med. Chem., 2009, 16, 4297–4313 CrossRef CAS.
- M. M. Pastor, G. G. Cruciani, I. I. McLay, S. S. Pickett and S. S. Clementi, J. Med. Chem., 2000, 43, 3233–3243 CrossRef CAS.
- M. Lapinsh, P. Prusis, T. Lundstedt and J. E. S. Wikberg, Mol. Pharmacol., 2002, 61, 1465–1475 CrossRef CAS.
- K. Ye, E. W. M. Lameijer, M. W. Beukers and A. P. IJzerman, Proteins: Struct., Funct., Bioinf., 2006, 63, 1018–1030 Search PubMed.
- S. Hellberg, M. Sjoestroem, B. Skagerberg and S. Wold, J. Med. Chem., 1987, 30, 1126–1135 CrossRef CAS.
- A. Kontijevskis, R. Petrovska, I. Mutule, S. Uhlen, J. Komorowski, P. Prusis and J. E. S. Wikberg, Proteins: Struct., Funct., Bioinf., 2007, 69, 83–96 Search PubMed.
- G. J. P. van Westen, J. K. Wegner, A. Bender, A. P. IJzerman and H. W. T. van Vlijmen, Protein Sci., 2010, 19, 742–752 CrossRef CAS.
- A. Lindstrom, F. Pettersson, F. Almqvist, A. Berglund, J. Kihlberg and A. Linusson, J. Chem. Inf. Model., 2006, 46, 1154–1167 CrossRef.
- T. R. Hvidsten, A. Kryshtafovych, J. Komorowski and K. Fidelis, Bioinformatics, 2003, 19, 81i CrossRef.
- T. R. Hvidsten, A. Kryshtafovych and K. Fidelis, Proteins: Struct., Funct., Bioinf., 2009, 75, 870–884 Search PubMed.
- H. Strombergsson, P. Daniluk, A. Kryshtafovych, K. Fidelis, J. E. S. Wikberg, G. J. Kleywegt and T. R. Hvidsten, J. Chem. Inf. Model., 2008, 48, 2278–2288 CrossRef.
- M. Sandberg, L. Eriksson, J. Jonsson, M. Sjostrom and S. Wold, J. Med. Chem., 1998, 41, 2481–2491 CrossRef CAS.
- A. Zaliani and E. Gancia, J. Chem. Inf. Comput. Sci., 1999, 39, 525–533 CrossRef CAS.
- H. Mei, Z. H. Liao, Y. Zhou and S. Z. Li, Biopolymers, 2005, 80, 775–786 CrossRef CAS.
- A. G. Georgiev, J. Comput. Biol., 2009, 16, 703–723 CrossRef CAS.
- D. H. Williams, N. L. Davies, R. Zerella and B. Bardsley, J. Am. Chem. Soc., 2004, 126, 2042–2049 CrossRef CAS.
- A. D. Williams, S. Shivaprasad and R. Wetzel, J. Mol. Biol., 2006, 357, 1283–1294 CrossRef CAS.
- Y. Patel, V. J. Gillet, T. Howe, J. Pastor, J. Oyarzabal and P. Willett, J. Med. Chem., 2008, 51, 7552–7562 CrossRef CAS.
- R. D. Head, M. L. Smythe, T. I. Oprea, C. L. Waller, S. M. Green and G. R. Marshall, J. Am. Chem. Soc., 1996, 118, 3959–3969 CrossRef CAS.
- S. Wold, M. Sjöström and L. Eriksson, Chemom. Intell. Lab. Syst., 2001, 58, 109–130 CrossRef CAS.
- P. Geladi and B. Kowalski, Anal. Chim. Acta, 1986, 185, 1 CrossRef CAS.
- J. K. Wegner, H. Froehlich and A. Zell, J. Chem. Inf. Comput. Sci., 2004, 44, 921–930 CrossRef CAS.
- L. Eriksson, P. L. Andersson, E. Johansson and M. Tysklind, Mol. Diversity, 2006, 10, 169–186 CrossRef CAS.
- A. Hoskuldsson, Chemom. Intell. Lab. Syst., 2001, 55, 23–38 CrossRef CAS.
- I. Guyon and A. Elisseeff, Journal of Machine Learning Research, 2003, 3, 1157–1182 Search PubMed.
- E. Freyhult, P. Prusis, M. Lapinsh, J. E. Wikberg, V. Moulton and M. G. Gustafsson, BMC Bioinformatics, 2005, 6 Search PubMed.
- H. Sun and D. Fry, Curr. Top. Med. Chem., 2007, 7, 1042–1051 CrossRef CAS.
-
C. C. Chang and C. J. Lin, LIBSVM: a library for support vector machines, http://www.csie.ntu.edu.tw/cjlin/libsvm Search PubMed.
- X. J. Yao, A. Panaye, J. P. Doucet, R. S. Zhang, H. F. Chen, M. C. Liu, Z. D. Hu and B. T. Fan, J. Chem. Inf. Comput. Sci., 2004, 44, 1257–1266 CrossRef CAS.
- Y. Liu, J. Chem. Inf. Comput. Sci., 2004, 44, 1823–1828 CrossRef CAS.
- L. Carlsson, E. A. Helgee and S. Boyer, J. Chem. Inf. Model., 2009, 49, 2551–2558 CrossRef CAS.
- A. J. Smola and B. Schölkopf, Stat. Comput., 2004, 14, 199–222 CrossRef.
- M. Fernandez, L. Fernandez, J. Caballero, J. I. Abreu and G. Reyes, Chem. Biol. Drug Des., 2008, 72, 65–78 CrossRef CAS.
- S. Grossberg, Neural Networks, 1988, 1, 17–61 CrossRef.
- A. Browne, B. D. Hudson, D. C. Whitley, M. G. Ford and P. Picton, Neurocomputing, 2004, 57, 275–293 CrossRef.
- A. Bender, D. W. Young, J. L. Jenkins, M. Serrano, D. Mikhailov, P. A. Clemons and J. W. Davies, Comb. Chem. High Throughput Screening, 2007, 10, 719–731 CrossRef CAS.
- L. Breiman, Mach. Learn., 2001, 45, 5–32 CrossRef.
- M. R. Segal, Center for Bioinformatics & Molecular Biostatistics, 2004 Search PubMed.
- V. Svetnik, A. Liaw, C. Tong, J. C. Culberson, R. P. Sheridan and B. P. Feuston, J. Chem. Inf. Comput. Sci., 2003, 43, 1947–1958 CrossRef CAS.
-
C. E. Rasmussen, in Advanced Lectures on Machine Learning, 2004, pp. 63–71 Search PubMed.
- O. Obrezanova, G. Csanyi, J. M. R. Gola and M. D. Segall, J. Chem. Inf. Model., 2007, 47, 1847–1857 CrossRef CAS.
- T. Schroeter, A. Schwaighofer, S. Mika, A. Ter Laak, D. Suelzle, U. Ganzer, N. Heinrich and K. R. Müller, ChemMedChem, 2007, 2, 1265–1267 CrossRef CAS.
- A. Tropsha, P. Gramatica and Vijay K. Gombar, QSAR Comb. Sci., 2003, 22, 69–77 CrossRef CAS.
- L. Eriksson, Quantitative structure–activity relationships in environmental sciences-VII SETAC, Pensacola, 1997, 381–397 Search PubMed.
- L. Eriksson and E. Johansson, Chemom. Intell. Lab. Syst., 1996, 34, 1 CrossRef CAS.
-
A. Tropsha, in Handbook of Chemoinformatics Algorithms, ed. J. Faulon and A. Bender, 2010 Search PubMed.
- L. Eriksson, J. Jaworska, A. P. Worth, M. T. D. Cronin, R. M. McDowell and P. Gramatica, Environ. Health Perspect., 2003, 111, 1361–1375 CrossRef CAS.
- K. Baumann, TrAC, Trends Anal. Chem., 2003, 22, 395–406 CrossRef CAS.
- A. Golbraikh and A. Tropsha, J. Mol. Graphics Modell., 2002, 20, 269–276 CrossRef CAS.
- J. Reunanen, Journal of Machine Learning Research, 2003, 3, 1371–1382 Search PubMed.
- J. K. Wegner and A. Zell, J. Chem. Inf. Comput. Sci., 2003, 43, 1077–1084 CrossRef CAS.
- A. Kontijevskis, R. Petrovska, S. Yahorava, J. Komorowski and J. E. S. Wikberg, Bioorg. Med. Chem., 2009, 17, 5229–5237 CrossRef CAS.
- N. Nagamine, T. Shirakawa, Y. Minato, K. Torii, H. Kobayashi, M. Imoto and Y. Sakakibara, PLoS Comput. Biol., 2009, 5, e1000397–11 CrossRef.
- B. Schölkopf, Learning With Kernels, 2002 Search PubMed.
- P. Prusis, R. Muceniece, P. Andersson, C. Post, T. Lundstedt and J. Wikberg, Biochim. Biophys. Acta, Protein Struct. Mol. Enzymol., 2001, 1544, 350–357 Search PubMed.
- A. Kontijevskis, J. Komorowski and J. E. S. Wikberg, J. Chem. Inf. Model., 2008, 48, 1840–1850 CrossRef CAS.
|
This journal is © The Royal Society of Chemistry 2011 |