DOI:
10.1039/D1NA00086A
(Review Article)
Nanoscale Adv., 2021,
3, 2995-3027
Biomolecular interactions of ultrasmall metallic nanoparticles and nanoclusters†
Received
3rd February 2021
, Accepted 16th April 2021
First published on 28th April 2021
Abstract
The use of nanoparticles (NPs) in biomedicine has made a gradual transition from proof-of-concept to clinical applications, with several NP types meeting regulatory approval or undergoing clinical trials. A new type of metallic nanostructures called ultrasmall nanoparticles (usNPs) and nanoclusters (NCs), while retaining essential properties of the larger (classical) NPs, have features common to bioactive proteins. This combination expands the potential use of usNPs and NCs to areas of diagnosis and therapy traditionally reserved for small-molecule medicine. Their distinctive physicochemical properties can lead to unique in vivo behaviors, including improved renal clearance and tumor distribution. Both the beneficial and potentially deleterious outcomes (cytotoxicity, inflammation) can, in principle, be controlled through a judicious choice of the nanocore shape and size, as well as the chemical ligands attached to the surface. At present, the ability to control the behavior of usNPs is limited, partly because advances are still needed in nanoengineering and chemical synthesis to manufacture and characterize ultrasmall nanostructures and partly because our understanding of their interactions in biological environments is incomplete. This review addresses the second limitation. We review experimental and computational methods currently available to understand molecular mechanisms, with particular attention to usNP–protein complexation, and highlight areas where further progress is needed. We discuss approaches that we find most promising to provide relevant molecular-level insight for designing usNPs with specific behaviors and pave the way to translational applications.
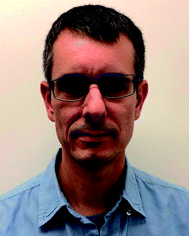 Alioscka A. Sousa | Alioscka Sousa obtained his PhD (2006) in Materials Science & Engineering from Stevens Institute of Technology, USA, and then worked as a post-doctoral researcher (2006–2012) at the National Institute of Biomedical Imaging and Bioengineering (NIBIB). He is now a Professor in the Department of Biochemistry at the Federal University of São Paulo (UNIFESP), Brazil. His current research focuses on the theme of nano–bio interactions, with an emphasis on the mechanisms of nanoparticle–protein and nanoparticle–cell interactions. |
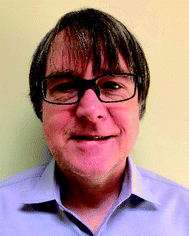 Peter Schuck | Dr Schuck obtained his PhD from the Goethe-University Frankfurt am Main, Germany, where he worked on interactions of integral proteins of the erythrocyte membrane using analytical ultracentrifugation. He received his post-doctoral research training in physical biochemistry with Dr Allen Minton at NIDDK, and joined the Bioengineering and Physical Science Program of NCRR as a Research Fellow in 1997, developing biophysical methods for the study of protein interactions. In 2014 he was appointed an Earl Stadtman Investigator in NIBIB and Chief of the Dynamics of Macromolecular Assembly Section in the Laboratory of Cellular Imaging and Macromolecular Biophysics. |
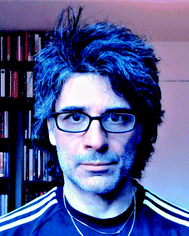 Sergio A. Hassan | Sergio Hassan obtained a PhD in Physics from University of Campinas, São Paulo, Brazil, in the areas of nonequilibrium statistical mechanics and thermodynamics. He joined the Mount Sinai School of Medicine in New York City as a postdoc and became a staff scientist with the Center for Molecular Modeling (NIH) in Bethesda, Maryland, where he developed and applied theoretical and computational methods to study biomolecular interactions. He recently joined the computational structural biology unit of the BCBB (NIAID/NIH) to focus on molecular mechanisms of infectious diseases, including viral replication, drug-resistant bacteria, and parasite-borne infections. His interest in ultrasmall nanoparticles stems from their potential use as antiviral and antimicrobial agents. |
1. Introduction
Metallic particles with core size below ∼3 nm are known as ultrasmall nanoparticles1,2 (usNPs); those below ∼2 nm are usually called nanoclusters3,4 (NCs) and may contain as few as 10–30 atoms, which gives them molecule-like properties. usNPs have garnered interest in recent years because of growing evidence suggesting a safer and more efficient performance in biomedical applications than their larger counterparts. Ultrasmall NPs have distinctive physicochemical properties and unique biological behaviors, including intrinsic catalytic activity,5 efficient renal clearance,6,7 exceptional tumor accumulation,8–11 better cell nucleus penetration,12,13 and reduced toxicity.1,14,15 These attractive features, however, depend critically on the NP surface properties, including the ability to avoid exposure of the metallic core to the biological environment.16–19 These are strong reasons to attract interest in the biomedical community, but their size also makes them unique in precision applications. Indeed, usNPs are protein mimics, comparable in size to an average globular protein. Their interactions with proteins, membranes, and other biological structures, known as nano–bio interactions, can, in principle, be controlled through a judicious choice of design parameters, such as core size and shape, surface chemistry, and core composition. Relative to their classical counterparts, usNPs retain features that make them comparable to drugs,20,21 with the potential for target selectivity and binding specificity to regulate protein function,22–25 broadening the possibilities offered by traditional small-molecule medicine. usNPs have been used in traditional and emerging areas of therapy and diagnosis, including biosensing, biolabeling, protein recognition, drug delivery, and cancer theranostics and radiotherapy. Still, significant challenges remain for regulatory approval and routine clinical applications. Some of these challenges are shared with classical NPs, such as physiological fate; others are more pertinent to the ultrasmall regime, e.g., control of NP–protein binding modes, affinities, and kinetics, all basic concerns in protein engineering as well. Unleashing the full potential of usNPs thus requires detailed knowledge of their interactions with biomolecules and their modulation by the biological microenvironment. Environmental effects include crowding, confinement, and the pH and ionic strength of the solution, all of which change as the particle migrates from the blood to the target site.
This review will focus mainly on ultrasmall gold NPs (usGNPs). However, many of the concepts described here will be valid more generally because the forces underlying usNP–bio interactions are dominated by the core morphology rather than composition and the coating layer properties. Gold as a core material offers several advantages, such as biological inertness, ease of surface modification, and exceptional electro-optical properties, which can be used for imaging,26 photothermal ablation of tissues,27 and other therapeutic and diagnostic applications. Progress is being made in synthesizing heterogeneous metallic nanocores,28 which paves the way for multiple functionalities or anisotropic surface chemistries, adding one more design parameter to control nano-bio interactions.
We will discuss topics of general interest, highlighting areas where further progress is needed to get a clearer picture of the forces operating at the sub-nanometer length scale, especially at usNP/protein interfaces. Classical NPs will be discussed insofar as it helps illustrate contrasting or unique features of usNPs. For example, the vast literature on protein-corona formation, which has dominated the field for the last two decades,29–32 will be seldom mentioned since the concept of adsorbed proteins on the surface of usNPs is not physically sound. Instead, usNP–protein complexes and hybrid usNP/protein aggregates are the relevant structures that emerge and merit consideration. Because of the fast pace of research in the field, reviews on biomedical applications and studies in vivo are also frequent, so these topics will be mentioned mainly to underscore the unique potentialities of usNPs that cannot be realized with classical NPs.
The focus here is on the physics and chemistry of usNP–protein interactions, with emphasis on mechanisms. Although interactions with polyelectrolytes (e.g., RNA, DNA33), phospholipids (e.g., membranes34), and sugars (e.g., polysaccharides35) are not examined explicitly, the interaction mechanisms discussed in this review (e.g., Section 3) are general. Several biophysical, spectroscopic, and computational techniques, some in development, are currently being used, often in combination, to understand and quantify usNP–protein interactions in (near) physiological conditions. This review will focus on approaches we find most promising to provide relevant molecular-level insight to help design usNPs with specific nano-bio behaviors and pave the way for translational applications. After a general introduction in Section 1, we address in Section 2 the physicochemical properties of usGNPs and the dependence of nano-bio interactions on the particle design, focusing on the effects of surface chemistry on usGNP–protein binding and complexation. In Section 3, we discuss the mechanisms that govern nano-bio interactions in general. Experimental characterizations of usNP–protein association mechanisms are presented in Section 4. In Section 5, we describe advances in computational modeling and simulations and emerging approaches for reverse engineering usNPs with desired nano-bio interactions. Biomedical applications of usGNPs are presented in Section 6.
2. Size and surface chemistry of usGNPs and their influence on nano-bio interactions
There is no strict threshold delimiting the onset of the ultrasmall size regime for metallic NPs. Assuming crystal packing, usGNPs, defined as NPs with cores <3 nm across, contain fewer than 1000 atoms (Fig. 1a). Although encompassing a narrow size range within the broader array of nanoscale materials (∼1–100 nm),1 usGNPs can be further subdivided into two distinct size regimes according to their core structures and physicochemical properties (Fig. 1a). On the one hand, usGNPs larger than ∼2 nm present fcc crystalline structures36 and localized surface plasmon resonance bands resembling those in bulk crystalline gold. In contrast, gold cores smaller than ∼2 nm may display non-fcc structure (e.g., icosahedral-based or amorphous-like37,38) and molecule-like electronic configurations that generate optical quantization effects, such as sharp absorbance bands and luminescence emission.39,40 The special class of usGNPs under ∼2 nm, termed gold nanoclusters (GNCs), can be prepared with atomic precision and be assigned exact formulas and atomic structures.41,42 Their atomically precise configurations and protein-like structural complexity43 provide an ideal platform to study GNC interactions with biomolecules and reaction mechanisms. These features provide a venue to control binding specificity more accurately, e.g., by fine-tuning the relative GNC/protein orientation at the binding site. Several reviews have been published on the syntheses and fundamental physical principles of GNCs.3,4
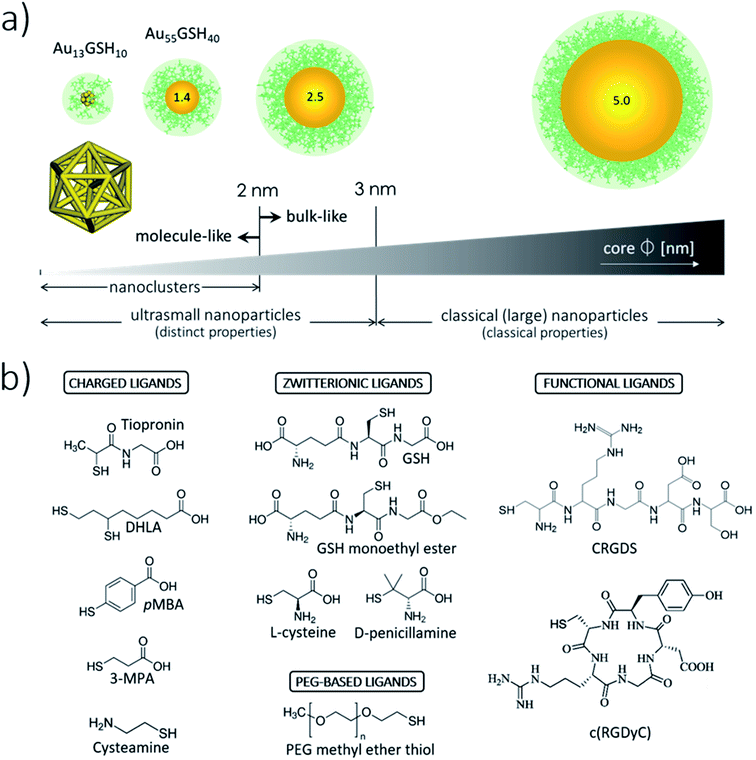 |
| Fig. 1 Illustration of the core and surface architectures and properties of usGNPs. (a) usGNPs have core sizes smaller than ∼3 nm. The central core is protected by an organic monolayer endowing the particles with water solubility, colloidal stability, and functionality. Shown around the cores is a monolayer of GSH molecules represented in green color. GNCs constitute a special class of usGNPs smaller than ∼2 nm. These can be assigned exact formulas and atomic structures; shown is the atomic structure of Au13GSH10. (b) Examples of small thiolated ligands used for the surface passivation of usGNPs. (i) Charged ligands are used in the preparation of charged particles that can interact with biomacromolecules. pMBA, para-mercaptobenzoic acid; 3-MPA, 3-mercaptopropionic acid; DHLA, dihydrolipoic acid. (ii) Zwitterionic and PEG-based ligands are used in the preparation of nonfouling particles. (GSH is commonly referred to as a zwitterionic ligand due to the simultaneous presence of a negative and positive charge; however, the actual net charge on the molecule is −1 at pH 7). (iii) Functional ligands can endow usGNPs with special properties. Shown are RGD-based peptide ligands that recognize the integrin ανβ3 receptor overexpressed on tumor cells.8,330 | |
The metallic core of usGNPs is protected (passivated) by an organic monolayer endowing the particles with water solubility and colloidal stability.44 In certain applications, a passivating shell formed by small molecular weight ligands is preferred to preserve the overall ultrasmall size as much as possible. For example, a 2 nm core-sized usGNP covered with the natural tripeptide glutathione (GSH) would maintain a compact hydrodynamic diameter of ∼4 nm, whereas the same core-sized NP covered with longer and more complex ligands could reach a hydrodynamic diameter as large as ∼10 nm.45Fig. 1b illustrates a few small thiolated ligands that have been used to prepare water-soluble usGNPs. It is clear from the wide diversity of ligands that the surface chemistry of usGNPs can be tuned almost at will with the desired physicochemical properties and functionalities.
The hydrodynamic diameters of usGNPs are commensurate in scale to a small- or mid-sized globular protein. Protein binding to usGNPs, therefore, takes place through a more restricted contact area interface compared to the adsorption of proteins onto larger NPs (Fig. 2a).22 Such a limited binding interface yields usGNP–protein complexes of comparatively low thermodynamic stability and short lifetimes,24,46,47 and also contributes to the preservation of the native conformation and biological activity of bound proteins.48–50 This is in stark contrast to the hard protein corona – the stable layer of partly denatured and permanently adsorbed proteins – that may develop on the surface of larger NPs.29,32,51
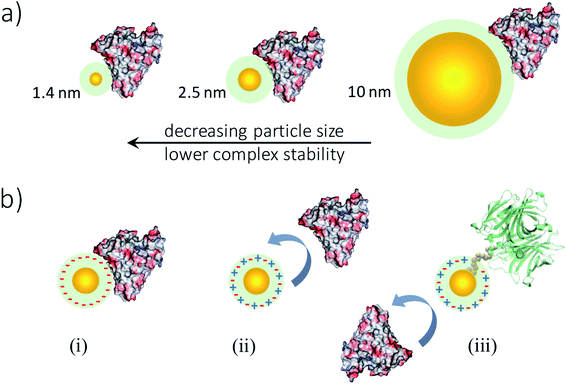 |
| Fig. 2 Influence of size and surface chemistry on usGNP-protein interactions. (a) Influence of size: particles of smaller sizes may form weaker complexes with proteins (albumin is shown as example; drawn to scale). (b) Influence of surface chemistry illustrated through a few selected examples: (i) charged usGNPs (class 1) interact with protein surface regions of opposite charge; (ii) zwitterionic usGNPs (class 2) are resistant against non-specific interactions; (iii) functional usGNPs (class 3) are designed to recognize specific proteins (in green) while avoiding non-specific interactions. See also Table 1. | |
Intriguingly, small variations in nanocore size within the ultrasmall regime can influence usGNP–protein interactions substantially. Using a gel shift assay, Boselli et al. demonstrated that PEG-coated usGNPs of 2 nm in core diameter were effective in minimizing biomolecular interactions in human plasma, whereas 3 nm-sized particles were not.46 We also observed a similarly striking effect of small size variations on particle–particle interactions leading to aggregation.52 We found that GSH-coated usGNPs in biological media supplemented with Ca2+ ions went from being colloidally stable to readily aggregating when crossing a size threshold around 2 nm. The forces underlying aggregation of usNPs have been studied by computer simulations and shown to differ from those acting on classical and colloidal particles53 (cf. Section 5). These examples stress the need for a high degree of control over the uniformity of NPs within the ultrasmall size scale.
The biomolecular interactions of usGNPs of a given size are dictated by the chemical and structural features of the passivating ligands at the surface. Table 1 presents a simple classification system for usGNPs based on their surface passivation and dominant protein interaction modes. Particles in class 1 bind to proteins non-specifically (e.g., Fig. 2b, panel i) and comprehend the largest and most varied subclass owing to their diverse surface chemistries. For example, these particles can be uniformly coated with a wide array of charged ligands54 (see Fig. 1b for examples), non-functional peptides,55 carbohydrates,56 DNA,57 or combinations of different moieties, e.g., charged/hydrophobic or zwitterionic/hydrophobic,58,59 to generate more complex surface architectures. Charged usGNPs, in particular, can bind with high affinity to regions of opposite charge on protein surfaces.23,45,54,60–62 This feature has been exploited in several basic-science studies aimed at the design and use of synthetic usGNPs for protein surface recognition and the modulation of protein structure and function (cf. Section 6.1).23,45,54,62,63 However, the inherently unspecific nature of long-range electrostatic interactions hinders the broad applicability of charged NPs to more complex environments (e.g., in vivo) where binding specificity is required. To overcome this limitation, the Rotello group has explored diverse strategies in monolayer design to influence the regio- and stereo-specificity of the interactions, such as the introduction of hydrophobic moieties alongside charged ligands onto the protecting monolayer or the incorporation of chiral end-groups.64–66 Of note, large NP scaffolds with tunable surface chemistries have also been under development for protein surface recognition and the control of protein binding orientation.67–69 However, despite successful examples in this area, realizing specific protein surface recognition with ultrasmall or larger NPs alike remains an enormous challenge. The reason is that specific recognition requires proper chemical and surface complementarity at the NP/protein binding interface, an accepted notion in high-affinity protein–protein binding and more apparent in usNP–protein associations.70 Nonetheless, by exploiting the tunability of the particle monolayer, usGNPs become well suited for sensing-type applications that require protein-binding selectivity rather than full specificity (cf. Section 6.2).66,71–74 To sum up, usGNPs of class 1 offer a vast array of possibilities to control their protein binding modes, e.g., orientation, affinity, and kinetics, and their ability to modulate protein structure and function. While this can be seen as an advantage, it is also a limitation in the sense that small variations in particle size or surface chemistry (e.g., when replicating someone else's work) can translate into disproportionately large differences in biological outcome.
Table 1 Classification system for usGNPs based on their surface passivation and dominant protein interaction modes
NP classes |
Typical surface chemistries |
Dominant interaction modes |
Example applications |
Class 1 |
Passivating ligands possess charged groups or mixtures of different groups (e.g., charged/hydrophobic) |
NPs interact non-specifically (but often selectively) with proteins |
Protein recognition for the modulation of protein structure and function; biosensing; antimicrobial |
Class 2 |
Passivating ligands include PEG and zwitterionic ligands |
NPs are resistant against protein interactions |
In vivo disease diagnosis and treatment, especially where renal clearance is required |
Class 3 |
Functional ligands can be integrated into class-2 NPs. Alternatively, NPs can be uniformly coated with functional ligands (e.g., peptides) imparting both surface passivation and protein-recognition properties |
Functional NPs bind to specific proteins while showing resistance against non-specific interactions |
Recognition of cell surface receptors in vivo (e.g., for cancer theranostics); bio-imaging probes in vitro |
Class 4 |
NPs are protected by proteins acting both as template and reducing agents |
NP interactions are mostly dictated by the protein template |
In vivo disease diagnosis and treatment; biosensing |
Class 2 comprises an important subclass of NPs for applications in vivo. This is due to their effective protein-repelling characteristics (Fig. 2b, panel ii), an attribute that enables them to be cleared efficiently from circulation by kidney filtration.9 Class-2 NPs are typically passivated with PEG chains or zwitterionic ligands (see Fig. 1b for examples),75,76 the latter being more effective as a nonfouling coating due to the strong electrostatic association of water that creates repulsive forces against protein binding77–79 (cf. Section 3). usGNPs coated with GSH have been one of the most widely investigated for theranostic applications in vivo, especially in the area of cancer nanomedicine.6,80 However, it is important to realize that the GSH molecule has a net charge of −1 at physiological conditions, i.e., it is not a true zwitterion. Taken at face value, this would suggest that proteins might interact with GSH-coated particles mediated by surface patches of positive charge. At any rate, these presumed interactions are likely to be very weak, especially within the smaller nanocluster size regime. True zwitterionic molecules potentially imparting stronger resistance against protein interactions at physiological pH include, among others, betaine-containing ligands78,81 and GSH monoethyl ester (Fig. 1b).82,83
Class 3 comprises NPs that incorporate functional ligands (e.g., peptides) for the specific recognition of proteins (Fig. 2b, panel iii), such as cell surface receptors in cancer cells. usGNPs of class 3 can be prepared, on the one hand, by conjugating the desired functionality directly to class-2 NPs via either chemical crosslinking or ligand exchange.84 Besides this more traditional conjugation approach, usGNPs (GNCs in particular) containing bioactive peptides can be prepared via a one-step biomineralization process, wherein a given peptide acts as both reducing agent and template.85–87 NCs obtained in this way are uniformly coated with the desired peptide, serving the purpose of both surface passivation and molecular recognition.
Class 4 includes the special subclass of protein-protected GNCs, in which proteins are used both as stabilizing and reducing agents during synthesis.88–90 These nanomaterials are particularly attractive for biomedical applications in vivo owing to their expected biocompatibility. Interestingly, fluorescent GNCs can be prepared using immunoglobulin G as a template, which confers the particles with intrinsic recognition properties against specific antigens.91
3. Molecular mechanisms of usNP–protein interactions
Because of their critical size, usNPs have features common to colloidal particles and proteins. As protein-mimics,22,92,93 the forces induced by the aqueous solution control most aspects of their nano-bio interactions, including association and dissociation mechanisms (cf. Section 4). In general, the interaction energy between a NP, classical or ultrasmall, and a protein (or any other structures) in a biological environment can be conceptually divided into the following contributions94–112 (cf.Fig. 3 and ESI†), | V = Velec + VvdW + Vs + Vc + Vd + Vx | (1) |
where Velec and VvdW are the electrostatic and van der Waals terms, Vs is the contribution from solvent-induced forces, Vc are the entropic effects of the coating layers, and Vd and Vx are the depletion and crowding effects of the solution components. In turn, each of these terms may be subdivided into different physical contributions, sometimes acting in opposite directions, e.g., the counter-ionic pressure and direct Coulombic forces94 in Velec (cf. ESI†). Depending on the NP size, shape, and surface physicochemical composition (e.g., types of functional groups, coating density, and length and flexibility of the layer molecules), each term would have a different weight. A central question for understanding NP behavior in the ultrasmall length scale is the right balance between these contributions and how they differ for classical NPs or colloidal systems. Differences are also expected within the ultrasmall regime itself, since the terms in eqn (1) will have different relative weights depending on the type of biomolecular structure interacting with the particle, e.g., another usNP (cf. Section 5.1), a protein, or a membrane. Answering these questions requires examining the physical origin of each contribution in greater detail, which we discuss below (cf. ESI for quantitative details†).
3.1. Electrostatics and van der Waals forces
In the partition of eqn (1), the Velec and VvdW terms arise from interactions in a structureless (continuum) water medium in which (point) ions adopt a spatial distribution determined, for example, by the Poisson–Boltzmann (PB) equation.94,98 These assumptions are common in the treatment of protein–protein interactions and form the basis for the theoretical modeling of colloidal systems. However, even with this simplified view of the aqueous solution, an atomistic representation of the usNP and protein is needed, especially at close contact, because surface topography and specific local interactions at the interfaces (e.g., H-bonds) play a crucial role. These interactions are highly system-dependent and cannot be captured in an analytical form. Thus, in general, Velec and VvdW have to be described in terms of atom-pair interactions, especially at interfaces (cf. Section 5). At longer separations, however, both terms can be described macroscopically using standard approximations in colloidal science, in which case the atomic details at the interface can be ignored. This approach helps make a qualitative connection with classical NPs and colloidal particles, which may be useful for interpreting experimental data qualitatively. In this case, the electrostatic term arises from two separate effects94 (Fig. 3a and e): on the one hand, the water-screened Coulomb interactions between the usNP and the protein and between each of them and the ions; and, on the other hand, the electrostatics-driven pressure of the ions on the usNP and the protein due to the spatial variations of the ions concentration as the usNP approaches the protein (cf. ESI for discussion†). The screened Coulomb interactions between the ions are implicit in their spatial distributions (PB equation); additional interionic forces, e.g., dispersion, would lead to ionic redistribution and a change in this balance of forces. Such ion-correlation effects94,113 can be significant for multivalent ions, leading to a weakening or even a reversal in the direction of the net interparticle forces. The van der Waals term114 (Fig. 3a and e) is determined mainly by the density and polarizability of the core atoms relative to water, properties embedded in the Hamaker constant, and by the particles' shape and size,94 resulting in a relatively long-ranged attractive dispersion decay.
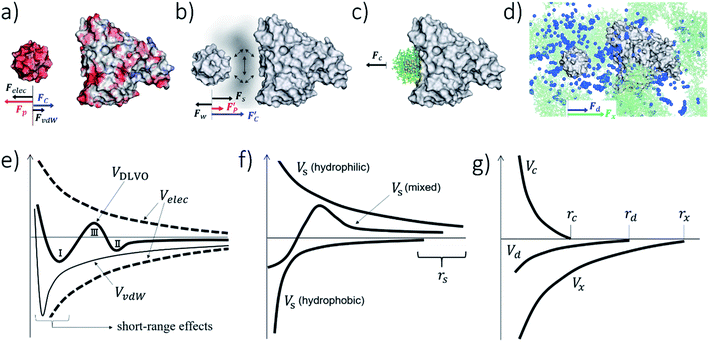 |
| Fig. 3 Components of the usNP–protein interaction energy in an aqueous solution as a function of separation (cf.eqn (1)). These effects are general and applicable to interactions with membranes and other NPs, although their relative importance is system-dependent (cf. ESI†). (a) Electrostatic (Felec) and van der Waals (FvdW) forces can be described within a continuum formalism, leading, e.g., to the DLVO theory (e). Felec is composed of a coulombic force (FC) and electrostatic-driven (entropic, often called osmotic pressure) force (Fp). The combination of dispersion (attractive component of FvdW; thin solid line in e) and electrostatics (attractive or repulsive; dashed lines) lead, in general, to a primary (I) and a secondary (II) energy minimum, and an energy barrier (III) in the potential (thick solid line; cf. ESI†). These features are well characterized in colloidal systems. The continuum approach needs modifications as the size of the particles or the surface-to-surface separation decrease. At close usNP/protein contact, short-range effects (e), such as surface topography and specific interactions (e.g., H-bonds), play a crucial role and need to be described in atomic detail. The major correction to the continuum solvent approximation comes from solvent-induced forces (Fs), originating in the liquid restructuring controlled by the water H-bond network (b). Solvent-induced forces can be dominant in the ultrasmall regime and modulate the thermodynamics and kinetics of the association and dissociation processes (cf. Sections 4 and 5). In pure water, Fs is monotonically repulsive for hydrophilic surfaces and attractive for hydrophobic surfaces (f); usNP and protein surfaces have a mixed composition of polar/nonpolar groups that both attract and repel water. In solution, water–water and water–ion interactions (represented by the vertical arrows in b) determine the interfacial liquid structure, affecting the forces that water and ions exert, independently on the usNP and protein (diagonal arrows). Thus, Fs has a water component (Fw in b) and corrections to both the coulombic and osmotic forces. This combination leads to a “desolvation” barrier (f), seen both in small molecules and usNPs (cf. ESI†). The onset (rs) of Fs depends on the solution makeup and the surface characteristics. Solvent-induced effects are difficult to capture in analytical form and must be studied by computer simulations (cf. Section 5). Entropic effects are of two types: (i) those generated by the coating layers upon binding (c), which induce a repulsive force (Fc) due to the restriction of movement of the layer molecules; and (ii) those generated by the solution components (d), either depletants, which induce an attractive force (Fd), or crowders, which typically induce attraction but may induce repulsion (Fx; cf. Section 3 and ESI†). The onset of these forces (rc, rd, and rx in g) depends on the layers' thickness and the shape, size, and concentration of depletant and crowding agents. Panels (a)–(d) show a 1.4 nm GSH-coated usGNP interacting with an albumin protein, drawn to scale; in (d), depletants are the components of a cell-culture solution represented by atom-based vdW spheres (blue); crowders are albumin proteins at physiological concentration shown in green.53 The relative magnitude and direction of forces depicted in (a)–(d) are for illustration purpose only as they are system-dependent. | |
Under these approximations, the first two terms of eqn (1) (Velec and VvdW) comprise the well-known DLVO theory; the other terms are, by definition, non-DLVO contributions, although this name is typically given to Vs (discussed below). The DLVO theory suffices to explain many experimental observations in colloidal systems and is often invoked in the context of classical NPs. The balance between electrostatic (which may be attractive or repulsive) and dispersion (always attractive) may produce a primary or a secondary minimum in the potential, typically associated with coagulation or flocculation of colloidal particles (Fig. 3e).95–97,99 Computer simulations of usNP–usNP interactions have indeed shown the presence of both minima under certain conditions52,115,116 and may also exist in some usNP–protein interactions.93 However, the competition of forces that gives rise to these minima in the ultrasmall regime may be different from that in the DLVO theory, as atomistic simulations have shown,53 because solvent-mediated effects (Vs in eqn (1); cf. Section 3.2) become more prominent for usNPs (cf. Section 5). Discrepancies between DLVO theory and experiments at small length-scales are not surprising and have led to corrections and alternative formalisms,117,118 although still within the continuum solvent approximation.
3.2. Solvent-mediated forces
Although Velec and VvdW incorporate some of the effects mediated by the aqueous solution, the term solvent-mediated (or -induced) forces refer to explicit corrections (Vs) to the continuum approximation that stem from the structure and dynamics of the interfacial liquid (Fig. 3b and f), which can be quite different from the bulk. The fundamental importance of these forces has long been recognized,94,119–123 and their role in NP interactions discussed.106,107,112 These forces depend critically on the chemical nature of the interfaces and are thus expected to be very different among usNP classes (cf.Table 1). Surface-specific effects become increasingly important at decreasing length-scale and may be dominant in the ultrasmall regime. Aqueous interfaces are the most difficult to characterize owing to the behavior of water itself, dominated by its hydrogen-bond network.
The behavior of water at protein interfaces has long been a topic of basic research. There are still open questions, especially in crowded conditions, e.g., how different is intracellular water from bulk water. The structure and dynamics of water are known to be modified up to several nanometers from certain surfaces, e.g., on colloidal particles or DNA arrays,94,120,124 but the extent of changes at protein surfaces has been contentious. One reason is the conflicting results from the different techniques used to probe local water behavior in complex media.125–129 Data have supported a range of views, from non-bulk behavior affecting large portions of intracellular water to only a few hydration shells;123 the answer likely depends on the local level of crowding or confinement and solution composition. Answering these questions is critically important, especially for charged usNPs, since their interactions would be very different depending on the properties of the liquid in which they are immersed.
Water structure and dynamics affect the interactions between a usNP and a protein directly (hydration forces, dielectric response) and indirectly through their effects on the solution components, ions in particular. The ionic atmospheres that develop in the vicinity of complex surfaces can depart significantly from idealized distributions used in the derivations of Velec and VvdW above. The layers of water and ions that separate a usNP from a protein can be more or less stable depending on their structures, affecting binding affinity and kinetics and, conceivably, the reaction path towards association (e.g., first encounters and secondary binding modes; cf. Section 4.1).
There is no simple analytical expression for the contributions of solvent-induced forces that can be used in practice as successfully as the DLVO theory, especially for usNPs. Each system is unique in its local topography and physicochemical properties, leading to specific liquid behaviors. Nonetheless, a few general comments can be made on the case of water-induced forces. These forces originate in the rearrangement of the hydrogen-bond (HB) network as a usNP approaches a protein. The strength with which water holds on their surfaces can make a difference in the thermodynamic and kinetics of usNP–protein binding. These forces are generally strong and repulsive between hydrophilic surfaces and weaker and attractive between hydrophobic ones (Fig. 3f).94 These trends are seen in a variety of systems and size scales (cf. ESI†). However, usNP and protein surfaces are typically composed of a mix of polar and nonpolar moieties, leading to a complex interplay between hydrophilic repulsion and hydrophobic attraction. As a usNP approaches a protein, there is always a dehydration barrier to overcome because both the NP and protein surfaces contain hydrophilic groups that bind to water. The height of the barrier reflects the water's resistance to being removed from the space between the usNP and the protein. Overcoming this barrier requires a disruption of the water–water, water–usNP, and water–protein HB network. Once this resistance is overcome, a swift or mild attraction may occur as the removed water molecules find new favorable interactions, e.g., with bulk water (Fig. 3f; cf. ESI for details†).
Ion-induced forces are more elusive and difficult to characterize but behave along similar lines. Their spatial distributions are determined indirectly by the structure and dynamics of interfacial water and directly by the surface themselves. Like water, ions must first be removed for the usNP and protein to come in close contact, and this process ultimately determines the binding affinity and kinetic mechanism.47,93 Probing these effects in nano-bio interactions require special techniques, as discussed in Sections 4 and 5.
3.3. Surface-layer forces
The coating layer may constitute a large portion of a usNP and be a non-negligible component of a classical NP (e.g., about 70% in volume for a 3 nm GSH-coated GNP and 10% for a 30 nm NP with the same coating). Thus, the effects of the layer's configurational (or conformational) entropy upon binding need to be considered (Fig. 3c and g). Depending on the usNP class (Table 1), these coating-specific contributions can have different weights in the total energy and likely contribute more to usNP–protein binding than the changes in configuration entropy during protein–protein complexation. The thermodynamic penalty associated with the concomitant restriction of movement of the layer molecules can contribute to usNP–protein repulsion (Fig. 3c and g). Such entropic effects are well characterized in colloidal science and play a significant role in the (steric) stabilization against aggregation and deposition, although the molecular origin is different, namely, mixing and elastic compression of grafted or adsorbed polymers (cf. ESI†).95,97,99
It is unclear how these forces differ among the usNP classes. They are likely more prominent in layers composed of long, flexible chains (e.g., PEG- or some peptide-based) and relatively low coverage density, in such a way that the layer molecules can effectively experience a measurable restriction of movement and elastic adaptation during binding. The effect is probably more limited for dense layers composed of relatively short molecules (e.g., Fig. 1b) with few degrees of freedom. The challenges in estimating the coating layer's entropic contributions are similar to those involved in estimating changes in the configurational entropy during protein–protein binding (e.g., the conformational and vibrational contributions of side chains and flexible loops).130
3.4. Depletion forces
The entropic effects associated with the solution components also affect the nano-bio interactions. Depletants are inert co-solvent molecules much smaller than the NPs, so that they do not restrict the NPs movement (Fig. 3d).109,110 Thus, globular proteins or non-adsorbing polymers can act as depletants for classical NPs, but only smaller species would qualify as depletants for usNPs (see below). Depletion forces stem from changes in the volume accessible to the depletants as a NP approaches a protein or another particle.111,131 The size and concentration of the depletants determine the range and strength of the force. At large usNP–protein separations, the volume excluded by the usNP and protein is maximal; as their separation decreases below a certain threshold (onset of depletion), the volume of the excluded region between them begins to drop, and the configurational entropy of the depletants rises accordingly. The associated thermodynamic reward drives usNP–protein attraction (Fig. 3g). A possible enthalpic component to depletion forces has also been discussed.132 An equivalent, mechanistic interpretation in terms of osmotic pressure of the depletants can also be given (cf. ESI†).
Depletion forces have found use in practice to drive or control various physicochemical processes,133–135 including phase separation and self-assembly and the properties of soft materials, e.g., nanocomposite and polymer–colloid mixtures. Besides these practical applications, depletion forces can be used to modulate the interaction between NPs in vitro, e.g., by systematically varying the size ratio between NPs and depletants. The role of depletion forces in cell biology has begun to be recognized as a possible mechanism in the assembly of subcellular structures, such as the cytoskeleton and chromosomes.109
Depletion forces have been assumed to be important in nano-bio interactions of classical NPs, but their significance in usNPs is less clear. In living organisms, the type or concentrations of the solution constituents cannot be controlled. Besides water itself, only ions, amino acids, short peptides, and other small species are present, with concentrations of ∼250 mM or less. We recently used simulations to estimate the strength of depletion attraction between usNPs in a cell-culture solution.53 We found the interaction energy (defined as the minimum of the interparticle potential of mean force at close contact) to be small (<kT) for cores with diameters of up to ∼2.5 nm. However, the interaction energies became larger than kT for the larger pairs when the solution was crowded with macromolecules at physiological concentrations (Fig. 3d; see Section 3.5). Because depletion forces increase with the number of NPs, they could stabilize small usNP aggregates or usNP/protein complexes in physiological media.
3.5. Crowding forces
Unlike depletants, crowders are components of the solution comparable in size to the particles, or larger, and restrict the particles' mobility (Fig. 3d); confinement is an extreme case. Crowding effects have both entropic and enthalpic contributions (see below), although it is the former that is most commonly acknowledged and more intuitive to understand.100–102
Like depletion, the entropic effects of crowding stem from changes in volume exclusion. Unlike depletion, however, crowding forces can induce attraction or repulsion, depending on whether the usNP–protein association increases or decreases the system's total entropy.102 Attraction is more likely for globular crowders and proteins and occurs when more space becomes available to the crowders as the usNP and protein approach one another, increasing the crowders' configurational entropy (Fig. 3g). The size and shape of the interacting species, and the size, shape, and concentration of the crowders, determine the range and strength of the effective force. This dependence affects the binding modes and affinities of the resulting complexes.136,137
Crowding forces can be exploited in practice to control the structure of supramolecular materials,138 including the assembly of nanomaterials, and improve their performance. Their biological role has been established100,139,140 and are believed to control subcellular processes and organization.
As NPs migrate through the body, they encounter environments that vary broadly in composition and degree of crowding. For example, blood serum contains a total protein concentration of 60–80 mg mL−1,141 whereas the protein content in the intracellular space ranges from 50 to 250 mg mL−1.142 Addressing the influence of crowding (in concert with depletion) on usNP–protein complexation, both at the experimental and theoretical levels, is therefore vital to draw a more detailed understanding of the behavior of usNPs in naturally crowded biological milieus (cf. Section 4.2).
We recently used simulations to examine the strength of these entropic forces on usNPs association in an albumin solution at concentrations comparable to that in blood serum.53 Like depletion, the effects were modest, yielding an interaction energy at close contact close to thermal energy for cores of up to ∼2.5 nm in diameter. However, when the solution was mixed with a cell culture (Fig. 3d), the simulations showed that the energy became larger than kT for the larger pairs. Crowding and depletion are difficult to separate from their combined effects, but the higher concentration of depletants in the (reduced) available volume of the crowded solution may be responsible for the difference. Because both effects are cumulative, albeit not pair additive, they may stabilize small aggregates and complexes containing just a few usNPs. However, our recent experimental studies in vitro show that crowding does have a measurable effect on usNP–protein complexation.93 It may thus be that the non-entropic effects of crowding (see below) rather than the entropic effects just discussed are more significant for usNP–protein association in near-physiological solutions.
Another effect of crowding is the reduced translational and rotational diffusion of usNPs and proteins, which affect all associated processes.102 Thus, if a reaction is diffusion-controlled, the reaction rate will decrease; if reaction-controlled, it will increase because the entropic attraction lowers the energy barrier. The result of these opposing effects is difficult to predict, especially if the path to the final complex involves multiple intermediates, as seems to be the case of usNP–protein associations;47,93 in this case, each reaction and metastable binding mode may be affected separately.
Unlike the entropic effects, enthalpic contributions of crowding and confinement are more difficult to conceptualize. One such effect stems from the fact that crowders and confining structures remove large amounts of water, which is the dielectric medium that modulates the electrostatic interactions. These electrostatic effects of water exclusion are always present and traditionally described with the PB equation, e.g., by assigning a low dielectric permittivity to proteins and a high permittivity to water, or through a position-dependent permittivity, ε(r), if a more detailed representation of interfacial water is desired.143,144 Other enthalpic effects of crowding are closely related to the solvent-induced forces (Vs) discussed in Section 3.2, due to the proximity of the interacting molecules to the crowders or confining surfaces; these effects can be quite intriguing and counter-intuitive,103,123,145 potentially changing the usNP–protein interaction from attraction to repulsion.
Systematic studies of the effects of confinement, i.e., the restriction in the movement of usNPs and proteins by macroscopic surfaces (e.g., inverse micelles, parallel membranes, porous media, or crevices in assemblies, such as the cytoskeleton) might be more challenging than in crowding conditions but could provide valuable information on the nano-bio behaviors of usNPs in vivo. The intracellular environment contains many surfaces and crevices, and a large proportion of intracellular water is interfacial.123 Because usNP interactions within such regions can differ from those in the bulk, an account of these effects is needed to understand and quantify usNP behavior in the living cell or their interactions with cells. This problem has been recognized in the study of protein function. Failing to reproduce realistic intracellular environments in the test tube may conceal features of the usNP–protein interactions critical for in vivo applications.
Studies relevant for in vivo applications also require accounting for other effects not discussed here, e.g., hydrodynamic forces. During circulation, NPs are subject to strong shear forces under flow or in contact with macromolecular complexes and cells. These forces can induce degradation or detachment of the surface molecules,146 or affect the behavior of water, ions, and cosolutes in the local environment, modifying all the solvent-mediated mechanisms of usNPs–protein interactions discussed in this section.
4. Experimental characterization of usNP–protein interactions
To gain proper insight into the molecular mechanisms involved in usNP–protein interactions (cf. Section 3), a detailed experimental characterization of the thermodynamics and kinetics of the binding reaction is required.147–149 Such studies may help dissect the different physical contributions to general nano-bio interactions (cf.eqn (1)). Examining the different effects driving usNP–protein association faces conceptual and technical challenges similar to those encountered in protein–protein association. Electrostatic effects can be probed by studying the interactions at varying ionic strengths, whereas the contributions of H-bond, hydrophobic, and van der Waals forces on complex stability may be gauged from calorimetric data collected at different temperatures.150–152 The effects of crowding and depletion can be evaluated by investigating the strength of the interactions in solutions containing varying concentrations of inert species of suitable sizes, e.g., dextran/PEG and glycerol,101 respectively. Ultimately, a judicious combination of experiments and atomistic simulations is likely the most effective approach to untangle the different physical contributions to the interactions (cf. Section 5 for a systematic simulations study of the terms of eqn (1) in the case of usNP–usNP interactions53).
Because usGNPs are similar to globular proteins in terms of both size and surface chemistry, it is not surprising that many well-established characterization techniques and methods used to study protein–protein complexation can be adapted to investigate usGNP–protein interactions.45,61,93,153–158 However, usNPs are not proteins, and one should be aware of the differences and the potential impact of the implementation of such techniques, especially in quantification. We additionally recall that usGNP–protein complexes are short-lived and thus need to be studied in situ. Experimental methods frequently used to study protein adsorption onto conventionally NPs, such as centrifugal separation followed by dynamic light scattering (DLS) and ζ-potential analysis, perturb the binding equilibrium and are not appropriate.32,159,160
It is important to mention that preceding such detailed investigations, usGNPs must be well characterized with respect to their molar concentrations in solution,161 physicochemical properties,2 and colloidal stability.52 usGNPs uniformly coated with a single ligand type must be characterized at least in relation to ζ-potential, average size, and size uniformity, whereas usGNPs engineered with more complex surface architectures (e.g., containing mixtures of different ligands) demand additional characterization.162,163 At this point, we note that conventional transmission electron microscopy (TEM) and dynamic light scattering (DLS), as commonly employed, do not have adequate sensitivity to characterize the size and uniformity of usGNPs (especially within the NC size regime), whereas annular dark-field scanning TEM and analytical ultracentrifugation (AUC) constitute more powerful choices.52 usGNPs displaying overly broad size distributions are not suitable for rigorous quantitative interaction studies and require prior size purification/separation.164,165 The colloidal stability of usGNPs in the appropriate buffer or biological medium must also be carefully evaluated. A number of biophysical techniques (e.g., fluorescence spectroscopy) cannot yield reliable quantitative data unless NPs and proteins remain individually dispersed. Again, AUC is the gold standard for evaluating usGNP stability in solution due to its high hydrodynamic resolution and sensitivity for trace aggregates.166,167 The reader is referred to many excellent publications covering the issue of NP characterization in greater detail.2,162,163,168–171
4.1. Biomolecular interactions of usGNPs with isolated proteins in dilute solutions
Here, we briefly examine the kinds of structural, thermodynamics and kinetics information that are needed for a detailed description of usGNP–protein complex formation. We focus our discussions on the interactions of class-1 usGNPs with isolated proteins in dilute buffered solutions, where depletion and crowding effects can be ignored. Ideally, a systematic characterization of the binding reaction would entail determination of the following biophysical interaction parameters (Fig. 4a): (i) protein interaction site(s), ideally with knowledge about the amino acid residues involved; (ii) protein conformational changes, both at the global and local levels; (iii) maximum binding capacity of usGNPs; (iv) apparent equilibrium dissociation constant (KD); (v) binding cooperativity; (vi) binding thermodynamics (free-energy, enthalpy, entropy, and heat capacity changes); and (vii) binding kinetics (rate constants for complex association and dissociation). Besides, investigating the dependence of the aforementioned interaction parameters on solution conditions (e.g., temperature, pH, ionic strength) provides additional insight into the molecular mechanism of the binding reaction.
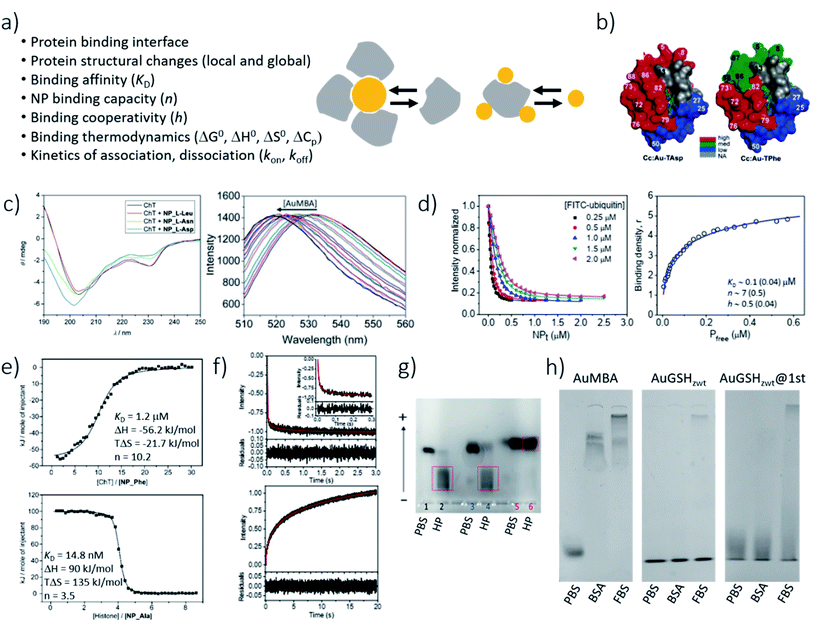 |
| Fig. 4 Experimental characterization of usGNP–protein interactions. (a) Structural, thermodynamics and kinetics information needed for a detailed description of protein binding to usGNPs of class 1 in dilute solutions (see ‘b’ through ‘f’ for illustrative examples). Depending on the physicochemical characteristics of both the usGNPs and the proteins, several proteins (gray) can bind to a single NP (yellow), or vice versa. The occurrence of mutual binding leads to aggregation (cf.Fig. 5c). (b) Protein interaction sites. Solvent protection for cytochrome c in complex with Asp- or Phe-decorated usGNPs determined by H/D exchange mass spectrometry.64 Surface regions depicted in red represent the preferred binding sites for the usGNPs. Facial specificity was observed in the binding of the Phe-decorated particles. (c) Structural changes. Left panel: CD spectra of chymotrypsin (ChT) in the absence or presence of usGNPs decorated with Leu, Asn or Asp.45 Right panel: normalized FITC emission spectra of active site-labeled thrombin titrated with AuMBA. A clear blue-shift was observed upon NP binding.23 (d) Determination of KD, n and h. Left panel: Set of fluorescence quenching titrations of FITC-labeled ubiquitin with AuMBA. Right panel: thermodynamic binding isotherm calculated from the set of titration curves by a model-independent method of analysis. Fitting of the Hill equation (eqn (4)) yielded the binding parameters annotated in the figure. 47 (e) Binding thermodynamics. Integrated heats of reaction obtained by ITC for the binding of ChT and histone proteins to Phe- and Ala-amino acid terminated usGNPs, respectively.152 Fitting according to an independent binding model yielded the thermodynamic parameters annotated in the figure. Binding of ChT to Phe-NPs was enthalpically driven with an unfavorable entropic contribution, whereas the opposite was true for the binding of histone to Ala-NPs. (f) Binding kinetics. Stopped-flow characterization of AuMBA-ubiquitin association and dissociation kinetics.47 Example forward (upper panel) and reverse (lower panel) time traces; red lines represent fits to tri- and four-exponential functions, respectively. The multi-phasic association and dissociation reactions suggested a complex, multi-step binding mechanism (cf. Section 4.1). (g and h) Assessment of interactions in protein-crowded media. (g) Native gel electrophoresis characterization of carboxyl-terminated PEG-coated gold NPs in human plasma (HP).46 Numbered lanes refer to 5 nm (1 and 2), 3 nm (3 and 4) and 2 nm (5 and 6) core-sized NPs. Only the 2 nm particles exhibited no signs of interactions when dispersed in HP. (h) Native gel electrophoresis characterization of usGNPs in 40 mg mL−1 albumin (BSA) and undiluted fetal bovine serum (FBS).83 A significant band shift appeared for AuMBA in both BSA and FBS. No changes in mobility were observed for NPs coated with zwitterionic GSH monoethyl ester (AuGSHzwt), in agreement with their strong resistance against protein binding. The smearing of AuGSHzwt particles monofunctionalized with Strep-tag (AuGSHzwt@1st) was observed in FBS, suggesting the occurrence of weak nonspecific interactions in FBS (but not in BSA). Adapted with permission from ref. 64 ((b); Copyright 2007 American Chemical Society), ref. 45 ((c), left panel; Copyright 2005 American Chemical Society), ref. 23 ((c), right panel; Published by the Royal Society of Chemistry), ref. 47 ((d and f); Published by the Royal Society of Chemistry), ref. 152 ((e); Copyright 2007 American Chemical Society), ref. 46 ((g); Copyright 2017 Wiley-VCH Verlag GmbH & Co. KGaA), and ref. 83 ((h); Copyright 2020 American Chemical Society). | |
Protein interaction sites.
usGNPs may interact with proteins selectively at defined protein surface domains so as to achieve the most thermodynamically stable final state. For example, we showed that anionic usGNPs bind to thrombin primarily through a couple of highly positively charged surface domains known as exosites 1 and 2.23,63 This was verified by fluorescence quenching titrations using exosite-directed aptamers in competition experiments with the usGNPs. In an earlier study, Rotello and co-workers used native gel electrophoresis to demonstrate electrostatically selective binding of charged usGNPs to the highly basic cytochrome c and acidic cytochrome c peroxidase proteins.54 Using amide hydrogen/deuterium (H/D) exchange mass spectrometry, these authors additionally showed facial specificity in the binding of a Phe-decorated anionic particle to cytochrome c (Fig. 4b).64 Nuclear magnetic resonance (NMR) is another powerful technique with the potential to unveil high resolution, residue-specific information on protein binding domains.172–178 For example, NMR-based chemical shift perturbation was employed to identify the preferred binding poses of ubiquitin onto usGNPs surface-coated with multimodal anionic ligands.179 Mass spectrometry-based proteolysis studies have been also carried out to elucidate the preferred binding orientations of proteins adsorbed onto large NPs;180–184 however, similar studies have yet to be implemented in the characterization of usGNP–protein complexes.
Protein structural changes.
Proteins can change conformation upon complexation with usGNPs, and understanding these changes plays an essential part in most biointeraction studies. Circular dichroism (CD) spectroscopy in the far-UV spectral region185 and Fourier transform infrared spectroscopy186,187 are two techniques widely used to monitor global changes in protein secondary structure. For example, CD spectroscopy was used previously to study the impact of different amino acid-terminated usGNPs on chymotrypsin conformation.45 It was observed that NPs bearing polar amino acids destabilized the protein in a time-dependent manner, whereas NPs decorated with hydrophobic amino acids had little influence on protein structure (Fig. 4c, left panel). Fluorescence spectroscopy, on the other hand, can detect NP-induced changes in local protein structure occurring around a fluorophore reporter (intrinsic tryptophan residues or extrinsic probes). The basis of the method rests on the high sensitivity of fluorescence emission to local structural perturbations affecting the local dielectric environment.188,189 For example, using fluorescein as a reporter of environmental changes at the active site of thrombin, we demonstrated the occurrence of allosterically transmitted structural changes to the active site as a result of usGNP interactions to thrombin's exosites (Fig. 4c, right panel).23,63 Techniques such as NMR spectroscopy and proteolytic cleavage followed by mass spectrometry can be used to reveal NP-induced changes to protein structure at the amino acid residue level; however, these methods have generally been limited to investigating protein adsorption onto large NPs.183,184,190,191 One should finally consider the possibility that usGNP complexation could modulate the conformational entropy/internal dynamics of proteins without major accompanied structural changes. Techniques suitable for probing dynamics include time-resolved fluorescence anisotropy,192,193 NMR spectroscopy194 and hydrogen/deuterium exchange mass spectrometry.195 However, the characterization of protein dynamics has remained largely unexplored in usGNP–protein interaction studies.
Binding affinity, stoichiometry, and cooperativity.
In the following discussion, the common situation is assumed in which multiple proteins (defined as the ligands) bind around the surface of a single usGNP (defined as the receptor). We then discuss determination of the microscopic binding affinity (KD) of the interactions, the NP binding capacity or stoichiometry (n), and the degree of binding cooperativity (h) (reflecting whether protein binding gets progressively enhanced or suppressed with each binding event).
Typically, KD, n and h can be experimentally determined by fitting of the Hill equation to a titration curve,196,197 which is, in turn, obtained by monitoring NP–protein complex formation through changes of some physicochemical signal. For example, steady-state fluorescence spectroscopy,155,198 capillary electrophoresis,199,200 isothermal titration calorimetry152,201 and analytical ultracentrifugation202 are some of the techniques that can be used to monitor complex formation through changes in fluorescence emission, electrophoretic mobility shift, evolved heat, and sedimentation coefficient, respectively. However, implicit in the application of these (and other) methods are assumptions on the relationship between the observed signal change (e.g., fluorescence, sedimentation coefficient) and the degree of binding.155,203,204 If underlying assumptions are not met, then fitting of binding models to a single titration curve provides only fitting parameters and not real thermodynamic interaction parameters. To better illustrate these ideas, we discuss below the application of fluorescence quenching titration and analytical ultracentrifugation (AUC) in the quantitative characterization of usGNP–protein interactions.
Characterization of NP–protein interactions by fluorescence quenching titration can be performed in either the direct61,157 or reverse31,199 titration modes. The latter applies when the fluorescence signal originates from the proteins (ligands), in which case their concentration is kept fixed in solution while titrating the NPs (receptors). Upon complex formation, the protein fluorescence is quenched by energy transfer to the metallic core of the particles.205 Fluorescence quench data obtained by reverse titration are often analyzed with the following Hill-type equation expressing the observed fluorescence signal, F, as a function of the free NP concentration, [NP]h:
| 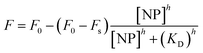 | (2) |
where
F0 is the initial fluorescence signal and
Fs is the signal at saturation. However, the use of
eqn (2) in the present case is wrong.
155Eqn (2) would be actually suitable to analyze data obtained in the direct titration scheme in which the NPs were the ligands and not the receptors. In order to correctly apply the Hill equation toward the analysis of data obtained under reverse titration, it is first necessary to calculate a thermodynamic binding isotherm (
r vs. [P], that is, fractional receptor saturation
vs. concentration of unbound protein) according to
eqn (3) below, and only then fit the Hill equation (
eqn (4)) to the so-obtained isotherm to find the apparent binding parameters
KD,
n and
h.
155,204 | 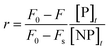 | (3a) |
| 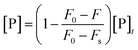 | (3b) |
| 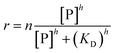 | (4) |
Even so, implementation of this procedure would require that the measured fluorescence signal be proportional to the molar concentration of bound ligand. Unfortunately, it is typically not known a priori whether this proportionality relation holds in any given system. For example, proteins might assume different binding configurations (in terms of orientation, conformation, or both) as a function of binding density, which in turn could affect their net emission signals in the bound state. Fluorescence quench data can be more rigorously analyzed with the Lohman–Bujalowski's and Schwarz's model-independent method of analysis, which enables the conversion of a set of titration curves into a true thermodynamic binding isotherm (r vs. [P]).203,204,206–208 Once the isotherm is generated, it can be modeled according to the Hill equation (eqn (4)) to find the relevant interaction parameters (Fig. 4d).
A different situation is encountered in sedimentation velocity AUC, where a change in the sedimentation coefficient of the NP can be measured as a function of protein concentration and the resulting NP occupancy by protein ligands. A first difficulty arises from the fact that colloidal expressions for sedimentation coefficients as a function of total mass traditionally rely on assumptions of spherically shaped particles.209 This is well-known to be a poor hydrodynamic model for proteins, which are typically highly aspherical and have usually frictional ratios varying anywhere between 1.2 and 2.0. Consequently, sphericity will be a poor assumption for the characterization of usGNP/protein complexes, where the protein contour significantly dominates the shape of the complex. In fact, usGNP with different occupancy will most likely differ from spherical shape to different extent.
As in the case for fluorescence binding signals, the measured change in the sedimentation coefficient (Δs) as a function of protein occupancy (ΔnP) is not linear. Owing to the greater density contrast of usGNPs, complexes will usually sediment slower than the bare usGNPs, which is well documented in the literature. Opposite from protein–protein interactions, the added protein ligand will (at first) make a relatively greater contribution to the hydrodynamic friction than to the total buoyant mass of the NP–protein complex. However, with increasing protein occupancy, a point will be reached where added frictional force from further increase in protein occupancy will match the further increase in buoyant mass, and therefore no further change in sedimentation velocity will be observed. Further increase in protein occupancy will then lead to behavior typical for protein–protein interactions with an increase in sedimentation velocity, i.e. reversing the sign of the binding signal Δs/ΔnP.83 At the inflection point – in the absence of binding signal – it is clear that fitting different binding models will lead to poorly defined binding constants and stoichiometries. Whether or not such a “blind spot” is experimentally observed will depend on the mass and density of the usGNP and the mass and shape of the bound protein. For spherical particles it can be shown theoretically to occur at
| 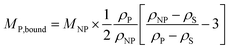 | (5) |
where
MP,bound is the total protein ligand mass,
MNP is the mass of the unbound NP,
ρNP,
ρP, and
ρS are the density of NP, protein, and solvent, respectively. Assuming densities of typical proteins and bulk Au in water the method becomes insensitive when protein mass is 1.6-fold the NP mass; it will occur at lower protein occupancy for coated usGNPs with lower average density, and at higher protein occupancy for non-spherical protein complexes. These problems may be avoided in future developments using compositional information from exploiting spectral properties in multi-signal analyses, as frequently applied to multi-protein complexes.
210,211
Finally, an additional difficulty in AUC analysis arises for proteins that do not form stable complexes with usGNPs but are in a dynamic equilibrium of bound and free states with lifetime shorter than 1000 s. In this case, the sedimentation behavior of free and complexed particles is coupled, and the measured sedimentation velocity does not correspond to that of a complex with ‘average occupancy’. Usually this leads to a ‘reaction boundary’ migrating with the time-average velocity of free and bound usGNP states, which adopts a binding isotherm as a function of total protein of a different form, as described by effective particle theory.211,212 Unfortunately, the case where complexes sediment slower than one of the unoccupied components has not yet been theoretically covered.
The above discussion illustrates how proper quantification of multi-site NP–protein interactions may not be possible based solely on the analysis of a single equilibrium titration curve, or if simplifying assumptions from protein–protein interactions or colloid characterization are uncritically adopted. This limitation could be mitigated to some extent by adopting more sophisticated data acquisition and analysis schemes, as well as pursuing the combination of different techniques. In any event, there is great value to be derived from even a semi-quantitative characterization of the interactions, e.g., in comparative analyses to assess relative differences in binding affinities.
Binding thermodynamics.
Isothermal titration calorimetry (ITC) enables a detailed characterization of the binding thermodynamics and a determination of driving forces in NP–protein interactions.152,179,213–216 The basic principle of the technique relies on the in situ measurement of the evolved heat changes (either heat absorbed or released) that accompany binding events during a step-wise titration series. The interaction enthalpy (ΔH0), the apparent KD and the maximum binding stoichiometry (n) are obtained by fitting of the ITC titration data to an appropriate binding model (typically, to an independent binding site model). The obtained ΔH0 reflects the sum of individual energetic contributions from the molecular events taking place during complexation. In particular, non-covalent bond formation (van der Waals interactions, electrostatics, hydrogen bond formation) at the binding interface is an exothermic process contributing favorably to complex formation, whereas desolvation and ion removal are unfavorable endothermic processes (cf. Section 3). For example, a net ΔH0 < 0 implies that the enthalpy gained from direct intermolecular interactions is greater than the enthalpic penalty for desolvation and ion removal. ΔG0 and ΔS0 are obtained from KD and ΔH0 using the relations ΔG0 = RT
ln
KD and ΔG0 = ΔH0 − TΔS0, respectively. Similarly to ΔH0, ΔS0 reflects the sum of contributions from different molecular events. In particular, local conformational restriction and loss of global rotational freedom upon complexation are unfavorable entropic processes, whereas desolvation and ion removal contribute favorably to the overall system's entropy. Previous ITC studies have revealed that the driving force for usGNP–protein complex formation can be either of a primary enthalpic or entropic nature, thus giving insight into the relative thermodynamic contributions of direct intermolecular interactions vs. desolvation/ion removal toward binding (Fig. 4e).62,152,153
At this point, a word of caution is needed regarding the accurateness of the experimentally determined thermodynamic parameters. First, we note that ΔH0 can be usually determined accurately from ITC since it can be directly determined from the data as the difference between the plateaus at the beginning and end of the titrations; as a result, ΔH0 is not strongly influenced by the choice of binding model. ΔG0 and ΔS0, on the other hand, are determined indirectly from KD. However, the value of KD may depend on the choice of binding model. For example, use of the commonly used independent binding model may produce incorrect estimates of KD in the presence of multi-site binding and cooperative effects.215 In addition, the analysis of single titration curves in a multi-site reaction model in the NP literature implicitly assumes that the cumulative heat of the reaction is proportional to the degree of binding,207,208 but which might not be true in a given system.
As an alternative to the use of ITC, the enthalpy change (ΔH0) of a biomolecular binding reaction can be determined from independent measurements of KD (e.g., by fluorescence titration) as a function of temperature and assuming the van't Hoff approximation: ln (1/KD) = −ΔH0/RT + ΔS0R. However, application of this approach to NP–protein interactions (or to any other multi-site interaction system for that matter)151 is problematic given the uncertainties in the determination of KD as discussed above.
Binding kinetics.
While a detailed thermodynamic dissection of usGNP–protein complex formation is undoubtedly important and necessary, equilibrium properties alone convey limited understanding about microscopic mechanisms of interactions. In contrast, a detailed knowledge of the kinetics of binding can afford unique insights into interaction mechanisms.217,218 For example, given a simple one-step binding model, the association and dissociation reactions can be phenomenologically described by the rate constants kon and koff, which, in turn, are connected to the binding affinity by KD = koff/kon. Briefly, kon is related to the size of the energy barrier for association, whereas koff probes the stability of the binding interface, hence reporting on complex residence time.
Techniques most suitable for the real-time characterization of interaction kinetics include surface plasmon resonance (SPR) biosensing, quartz crystal microbalance biosensing, both of which rely on formation of complexes with surface-immobilized binding partners, and fluorescence stopped-flow spectroscopy, which probes binding kinetics in solution. However, relatively few studies to date have attempted to use these techniques to understand the kinetics of protein binding to and unbinding from nanomaterials.24,93,219–225 Next, we briefly describe our recent work on the use of SPR biosensing and fluorescence stopped-flow spectroscopy for the characterization of usGNP–protein binding kinetics. With regards to SPR, it was implemented to investigate the electrostatically-driven interactions of the model proteins CrataBL and thrombin with pMBA-coated and GSH-coated usGNPs (AuMBA and AuGSH, respectively).24,93 It was determined that protein binding to AuGSH was ∼1000-fold weaker in comparison to AuMBA (∼30 μM vs. 30 nM). This was found to be mainly due to an ∼100-fold smaller apparent kon of the model proteins toward AuGSH. Noting the larger number of ions and the more complex nature of the ionic atmosphere surrounding AuGSH, the difference in kon was explained in terms of a larger energy penalty for desolvation and ion removal from AuGSH (cf. Section 3). Protein interactions with AuGSH were therefore identified as reaction-limited, whereas interactions with AuMBA were characterized as mainly diffusion-limited. The bound complexes were short-lived, displaying residence times in the range of 2–20 s. Borrowing from the terminology used to describe protein adsorption onto larger NPs, one could therefore refer to the proteins as forming a “soft corona” around AuMBA and AuGSH. However, this should not be mistaken for “soft interactions” (ultraweak interactions), which would actually involve KDS in the mM range.
At this point, we note that SPR biosensing suffers from a number of shortcomings such as mass-transport limitations, various surface-related artifacts, and a limited time resolution of the order of 1 second.226,227 Stopped-flow spectroscopy is a solution-based technique that circumvents most of the limitations of SPR. Of particular interest, the millisecond time resolution afforded by the technique enables identification of discrete intermediate steps along the association pathway in a multi-step reaction mechanism. Stopped-flow spectroscopy was therefore applied to investigate the kinetics of AuMBA binding to fluorescently-labeled ubiquitin, which were used as model systems (Fig. 4f).47 The results supported a complex, multi-step reaction model; briefly: (i) complexation proceeded through the formation of a weakly-bound and mostly solvated first-encounter complex (apparent KD ∼8.7 μM at [NaCl] = 5 mM). This was followed by discrete tightening steps of partial desolvation/ion removal and conformational rearrangement, yielding the final bound state (final apparent KD ∼0.1 μM); (ii) the bound complex was weakly stabilized, displaying an average lifetime in the range of seconds; (iii) the interactions were characterized by multiple koff rate constants, which could imply the existence of either multiple intermediate states or multiple final states (or still, a combination of both); (iv) weakening of the interactions at higher ionic strengths was due (in great part) to a destabilization of the encounter complex, whereas the average lifetime of the bound complex was not affected. Overall, these results supported the general notion that NPs in the ultrasmall size regime can be viewed, in some regards, as protein mimics.
4.2. Biomolecular interactions of usGNPs in protein-crowded media
usGNPs of classes 2 and 3 may be used in applications where a high degree of resistance against protein binding is required. For example, usGNPs used as cancer nanomedicines must resist nonspecific interactions in the presence of a high concentration of proteins and other biomolecules (e.g., the plasma concentration of albumin alone is 500–700 μM). Here, resistance against nonspecific interactions is defined as an apparent binding affinity in the mM range (KD > 1 mM) between a usGNP and a protein. Quantifying such ultraweak interactions is important to fully understand the behavior of usGNPs in the crowded biological milieu. However, there are well-known technical difficulties of characterizing ultraweak interactions in highly concentrated environments.
Native gel electrophoresis is one technique that is commonly employed to assess the behavior of usNPs in protein-rich biological media, such as human plasma (HP) or fetal bovine serum (FBS).46,83,228,229 A typical experiment consists in running usGNPs diluted in buffer vs. HP or FBS side-by-side in a gel, then observing any differences in the electrophoretic mobility of the particles as a result of nonspecific protein binding (Fig. 4g and h). As a general rule, “strong” protein interactions produce obvious band shifts, “weak” interactions cause only the smearing of bands, and “ultraweak” interactions generate minor or even no discernable changes in particle mobility (Fig. 4h).83 However, there are important limitations to take into consideration in these types of measurements, such as that samples are not strictly maintained in situ due to the separation of bands, ‘cage effects’ of polymers on the NP interaction,230 and the qualitative nature of the results interpretation.
In contrast to gel electrophoresis, migration in sedimentation velocity AUC can be observed without separation of usGNPs from the bath of surrounding serum proteins, allowing dynamic dissociation rebinding processes to proceed. If as little as 10% protein occupancy of usGNPs is detected, this will allow the detection and quantitation of transient interactions with KD in the low mM range.83 One difficulty for the precise analysis is posed by long-range hydrodynamic interactions altering the sedimentation process of concentrated solutions. Recently, mean-field corrections have been introduced that bring this solution regime in focus for quantitative analysis of weak interactions,231 which we envision will be applicable to usGNP studies.
Compared to class-2 usGNPs, the characterization of class-3 particles in protein-crowded media presents additional challenges. Class-3 usGNPs should be tested not only for their ability to resist nonspecific interactions but also for their capacity to recognize the desired protein receptor under crowding conditions. A lack of receptor recognition could signify either a loss of activity of the functional ligand (e.g., due to detachment from the surface or degradation) or the screening of the interactions by nonspecific protein binding. As a test model, we recently prepared usGNPs passivated with GSH monoethyl ester and functionalized with either single or multiple Strep-tag II peptides.82,83,232 We then verified the ability of the strep-tagged particles to resist nonspecific interactions and to bind Streptactin in the presence of serum. Our results revealed a lower binding efficiency of the multiply functionalized usGNPs over time (apparently due to increased levels of nonspecific serum interactions), suggesting the need for optimizing ligand density onto the surface of usGNPs for improved performance.83
Overall, much remains to be understood about the behavior of class-2 and class-3 usGNPs in the highly concentrated biological milieu. For example, it is typically not known what the order of magnitude is of the nonspecific ultraweak interactions; what the lifetimes of the particle–protein complexes are once they form; the extent to which depletion and crowding effects modulate the interactions (cf. Section 3); whether surface-attached functional ligands behave similarly as when they are free in solution; the extent to which avidity effects may play a role in the ultrasmall particle size regime; and so forth. Tackling such complex questions will require novel ways to addressing usGNP–protein interactions experimentally.
5. Computational modeling and simulations of usNPs
Deeper insight into usNP–bio interactions can be gained with a judicious combination of in vitro experiments and computer simulations. Simulations combine two elements, sampling and forcefield, and this section covers the latter. Sampling techniques are chosen depending on the problem at hand:233–235 stochastic,236 nonequilibrium,237 and enhanced238 sampling techniques may be used to reduce the statistical errors in a calculation or better explore specific regions of the configurational space, whereas plain equilibrium molecular dynamics (MD) is sometimes the most reliable approach to elucidate mechanisms, as it enables direct observation of the sequence of events, including transition states and the structure and dynamic behavior of water and other solvent components. However, the forcefield (FF) embeds the physics of the system and is ultimately the basis for a correct molecular interpretation of experimental data. Some fundamental themes on the simulations of usNP–protein systems are discussed below, with emphasis on results most relevant to the molecular interpretation of the experiments discussed in previous sections.
5.1. All-atom simulations
As protein mimics, simulations of usNP require an atomistic representation. There has been little conceptual innovation in all-atom FFs, mainly ad hoc modifications and parametrizations for specific applications, so the discussion will be limited to recent studies that provide big-picture insight. Of more interest from a methodological perspective are recent developments in multiscale approaches (Section 5.2).
Classical NPs require dealing with two main processes: protein adsorption, which is the primary mechanism of soft and hard corona formation, and the structural changes that usually follow. Different approaches, e.g., QM (quantum mechanical) and hybrid QM/MM (quantum mechanics/molecular mechanics)239,240 for chemisorption or MM241–244 for physisorption and protein restructuring, are used to model binding to surfaces of varying compositions, including oxides and mineral, alloys and metals, and, more relevant to biomedicine, polymeric or self-assembled monolayers of organic molecules (reviewed in ref. 245).
By contrast, usNPs require dealing with problems common to protein simulations. NCs may also require optical or electronic characterization of the nanocore, typically by quantum chemical calculations common in the study of small molecules, e.g., density functional theory or ab initio QM methods. The computational demands of an all-atom representation limit the study of usNPs to relatively small, diluted systems. As summarized below, a great deal can nonetheless be learned from model systems, which have implications for more general nano-bio behaviors, including usNP–membrane, usNP–polyelectrolyte (e.g., DNA and RNA), and usNP–protein binding.
Accurate calculations of potentials of mean force (PMF) in aqueous solutions have shown115,116,246 that, under certain conditions, usNPs coated with either anionic or cationic molecules (class 1) can attract each other, whereas usNPs with charges of opposite signs can repel each other. usNPs covered with net-neutral hydrophilic layers (class 2) repel each other due to the solvent-induced forces discussed in Section 3. As the coating molecules increase in length and flexibility (e.g., PEG-based; class 2), usNP pairs tend to show only a stable secondary minimum and a strong repulsion as the layers begin to overlap. In these simulations, the enthalpic contributions to interparticle repulsion have been identified, an interplay of ionic, surface charges, and hydrophobic interactions; it is also possible that the entropic repulsion of the coating layers (Vc in eqn (1)) play a role as they start to overlap.
The observation that like-charged particles can attract each other is well known in colloidal science, leading to flocculation (secondary minimum) or coagulation (primary minimum), depending on the conditions of the solution, as described by the DLVO and related theories (cf. Section 3.1). It is also known that such theories cannot be applied to usNPs because of the dominant effects of solvent-induced forces and the specific interactions at close contact (cf. Section 3.2). Also, the only attractive force opposing the direct electrostatic repulsion would be (effective) dispersion, which is relatively small for coated NPs with cores < 10 nm and negligible for usNPs;53 calculations115,116,246 have also shown that usNP–usNP attraction begins at a surface-to-surface separation of ∼2–4 nm, which is quite long range. The increasing concentration of counterions in the interparticle space undoubtedly plays a role in like-charge attraction, and the stabilizing effects of ions forming bridges between layer molecules help stabilize the close-contact configurations.52,115,116 Also, the dependence on NP size, e.g., smaller particles tending to form less stable dimers than larger ones, can be partially explained52 in terms of the difference in contact surface areas. Despite these partial explanations, the exact interplay of forces underlying aggregation of usNPs in biological fluids remains obscure.
To better understand the origin of these forces and evaluate the relative importance of each term in eqn (1), including the partial contributions within each of these terms (e.g., coulombic forces vs. pressure in Velec), we conducted MD simulations of two specific pairs of usGNPs.53 The nature of the simulations (plain, equilibrium, brute-force) allowed us to track the behavior of individual ions and water molecules as the usGNPs associate. Each NP pair was composed of identical particles with core diameters of either 1.4 nm or 2.5 nm, covered with L-glutathione, immersed in a cell-culture solution at physiological conditions, so the particles were negatively charged. These particles are ideal for the intended study because the dependence of interparticle forces on the core size was observed experimentally as a reversal from repulsion to attraction at a core diameter of ∼2 nm.52 The analysis showed the dominant role of solvent-induced forces and revealed interaction mechanisms that contrast sharply with the accepted view of classical NPs and colloids. It was shown that Coulomb interactions alone are insufficient to induce attraction, and the van der Waals forces of the solution components (water and ions) are a major factor to drive aggregation. Although the mobile counterions (diffuse layer) induced attraction, the negative co-ions make a substantial contribution. Transient ion-bridging of layer molecules was observed, but no single ion type was responsible for stabilizing the close contact configurations, as is the case for smaller systems (e.g., amino acids247). Direct interparticle dispersion was, as expected, small and almost entirely compensated by water (i.e., the excess polarizability of the NP in the solvent is negligible or, equivalently, the Hamaker constant is nearly zero). Moreover, the contribution of ion dispersion to the interparticle forces was modest, albeit not negligible, and the strongest effects were due to the short-range repulsion from the bound ions (Stern layer, in colloids terminology). The latter creates an imbalance of pressure between each particle's inner and outer hemispheres, which induces interparticle attraction. This imbalance, which vanishes at long interparticle separations (isotropic ion pressure), was not due to any measurable changes in the ion concentrations at the particle surfaces but to a change in either the rate or strength of ion “bombardment” on the surfaces.
The mechanisms just described contrast with those expected from a continuum approximation (e.g., DLVO; cf. Section 3), whereby osmotic pressure of ions induces repulsion and Coulombic forces attraction, with the former typically overcoming the latter, leading to “electrostatic” repulsion opposed to (and, in some cases, overcome by) the attractive interparticle dispersion. Moreover, it was shown53 that just a few ions (1–3) entering or exiting the interparticle space are enough to affect the interparticle forces when the NPs are very close to one another. This sensitivity is expected because the water mobility in the interparticle space is reduced, lowering the water's local dielectric permittivity. Water was shown to form long-range H-bonded wires connecting and even enveloping the usNPs as they draw near each other. These water chains could shift the pKa of ionizable groups on the inner hemispheres, modifying the layers' charge distribution.248 This charge modulation can affect the interparticle forces94 and can be studied by constant-pH MD.249
All these observations, obtained from atomistic dynamics simulations in simple systems, may help develop a parsimonious theory of usNPs in biological media that could be used in practice as readily and intuitively as the DLVO theory is in colloidal systems. Such a theory would entail finding simple analytical approximations for each of the terms in eqn (1).
5.2. Coarse-grained, multiscale, and adaptive multiscaling methods
Experimental studies of nano-bio interactions typically involve many particles and proteins, as exemplified by protein-corona formation on classical NPs. Modeling the behavior of usNPs in blood serum or the cytoplasm, or in test-tube setups designed to reproduce such microenvironments, requires dealing with states of high local concentration or crowding. Simulations of these systems need simplifications, typically through a coarse-grained (CG) model.
Several CG models for biomolecular simulations have been proposed since the early developments on united-atom250 and united-residue251,252 protein FFs. CG approaches involve some structural simplifications where parts of the system are grouped into beads treated as rigid units. The beads interact with one another through bonded and non-bonded energy terms. Carefully developed parameters aim to reproduce atomistic PMFs, so such CG models are knowledge- or statistical-based empirical models.245,253–260 They range from highly coarse ones, e.g., proteins treated as spherical particles (used to study protein adsorption and the kinetics of corona formation261), to models that retain the overall morphology of the proteins (used to study protein/usNP aggregations262 or the effects of protein shape on crowding forces53), to models in which amino acids are treated as single units interconnected along the backbone chain (to study protein conformational changes upon NP adsorption263) or hydrophobic and hydrophilic groups in lipids treated as multi-bead chains (to study passive or active NP transmembrane transport264). Further subdivisions into key groups are introduced depending on the application, e.g., to study specific binding of organic polymers or small ligands or even solvent molecules.
All these models have provided molecular explanations to experimental observations, showing that certain phenomena at the nanometer or even subnanometer length scales are insensitive to structural or chemical details.265 However, it is difficult to know a priori which systems or phenomena fall into this category. Ultimately, different parts of a system may need to be treated at different resolutions to capture its essential physics, leading to multiscale modeling.
One characteristic of multiscale simulations is that the CG model(s), once chosen, is kept fixed throughout the simulation. This approach may be inefficient if parts of the system are treated at high resolution when not needed or, worse, physically incomplete if a low resolution is used when an atomic representation is required. The solution to this practical problem is a self-adaptive multiscaling algorithm to enable transitioning between molecular resolutions in the course of a simulation, speeding up computation while preserving the short- and long-range structural requirements for a complete physical description.266,267 Of particular interest are self-adaptive methods that provide a smooth, reversible transition between the atomic, mesoscopic, and macroscopic scales, as discussed below.
The need for an adaptive multiscale approach is better exemplified by charged usNPs (class 1; cf.Table 1). Electrostatic forces can still operate far from their surfaces, especially in anisotropic or sub-optimal hydration conditions, e.g., during complexation or aggregation or in proximity to membranes. Indeed, nearby structures can exclude large amounts of water, which affects the dielectric screening of the interactions.143 These long-range effects are mostly insensitive to atomic details and can thus be modeled at low resolution. However, the forces that determine the nano-bio interactions depend more critically on the details at interfaces. Modest changes in surface chemistry can have profound effects on usNP–protein interactions.47,63,93 As discussed in Sections 3 and 4, particles of nearly the same sizes and charges can interact with the same protein differently depending on the ions and water behaviors at the usNP/protein interface during binding. In a system composed of hundreds or even thousands of proteins and usNPs, both low and high resolutions need to be accounted for within the same simulation according to demands dictated solely by the system configuration at each point in the simulation.
We have developed a multiscaling method based on a self-adaptive coarsening algorithm that enables on-the-fly adjustment of solvent effects to changing resolutions in the system (Fig. 5a, left panel).34,267 The method prioritizes structural details at the molecular surfaces (e.g., a NP, protein, or lipid) while treating the molecular cores at lower resolutions. Thus, the key elements that determine the nano-bio interactions are maintained, whereas a significant computational speedup can be achieved in crowded systems containing many molecules and NPs, as expected in real biological media. The method preserves physically relevant features of the molecules across scales,262 including hydrodynamic radii, charge distributions, and moments of inertia, and a connection can thus be made between the microscopic (atomic) and macroscopic (thermodynamic, hydrodynamic) representations.149,268
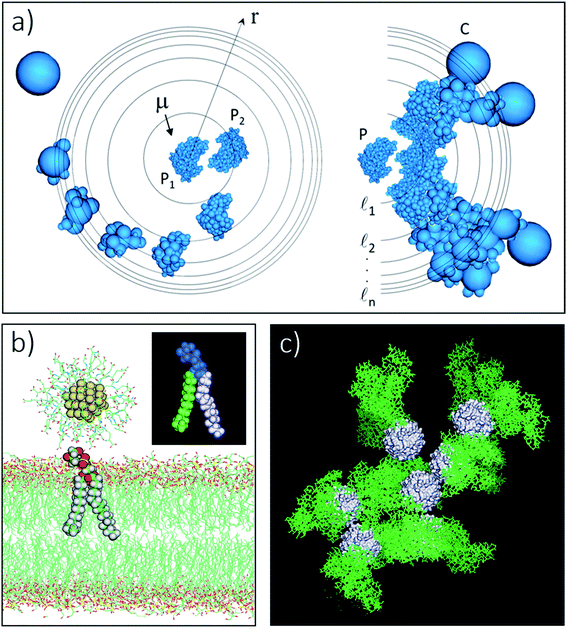 |
| Fig. 5 Adaptive multiscaling method for the simulations of nano-bio interactions. (a) Left: two interacting molecules see each other at different resolutions depending on the surface-to-surface separation (r), with the highest resolution, typically atomistic, at close separation (in practice, when the minimally containing spheres begin to overlap; region μ). The example illustrates how protein P1 sees P2 as their relative configuration changes and crosses different thresholds λi; the situation is symmetrical. In a multiprotein system, a protein sees each of the other proteins at a different resolution depending on their relative spatial configurations and is seen by each of the other proteins at a different resolution. Thus, not only the components of the system are represented at different scales (the standard definition of “multiscale”), but each element of the system is represented at multiple scales, each of which changes as the system evolves in time or configuration (adaptive multiscale). The algorithm captures desirable properties, including a continuous, reversible, and self-guided adaptation of resolutions, all of which are incorporated through the forcefield as a continuous function of the coordinates. Thus, energy is conserved, the forces are continuous, and detailed balance is preserved throughout the simulation. Right: the algorithm can be adapted to accommodate intramolecular gradients of resolutions for a more efficient treatment of large systems; this can be accomplished in practice by dividing C into parts, either arbitrarily or with a pre-established criterion. (b) A GSH-coated usGNP interacting with a POPG lipid bilayer; each lipid molecule may be considered a single molecule subjected to multiscaling34 or as a composite of, e.g., the head group and the hydrocarbon chains (inset). (c) A hybrid usNP/albumin cluster.262 The interactions between the highly charged usNPs and the proteins require both long-range interactions and atomic details at all the interfaces to be accounted for. An adaptive multiscaling approach provides a computationally efficient, physically robust description of such crowded systems. Internal dynamics could be introduced by switching on and off degrees of freedoms as the atoms enter or exit the region μ; this must be done through a thermostat to keep the energy equally partitioned among the active degrees of freedom.266 | |
The computational efficiency can be optimized by introducing intramolecular gradients of resolution (Fig. 5a, right panel). This is done by partitioning the molecule in non-overlapping substructures and treating each as an independent molecule subject to multiscaling. This partition can be entirely arbitrary or follow a structure-based criterion, e.g., by treating the nanocore and the layer of a usNP or the head group and hydrocarbon chains in a lipid molecule separately (Fig. 5b). Such an approach allows the simulations of highly crowded systems, e.g., hybrid usNP/protein aggregates (Fig. 5c) or states of local condensation. The study of aggregates,262,269 in particular, may include their morphologies and kinetics of assembly and dissolution and thus require a statistical analysis of an ensemble of aggregates. Each aggregate may contain many usNPs and proteins, so the computational challenge is great. This bottleneck can be overcome while preserving the system's physical description (short- and long-range interactions) by adaptive multiscaling with intramolecular variations in resolution.
The method just described permits the analytical calculation of forces during dynamics and satisfies detailed balance in MC simulations. The algorithm treats molecules as rigid bodies, limiting its current use to the early stages of complexation, i.e., before interfacial relaxation events. However, this is still a critical step in the hierarchy of relaxation times because it helps identify preferential first-encounter modes34 from which subsequent dynamic processes can follow (e.g., endocytosis264,270 or protein adaptation upon NP adsorption). Simulating such events would require switching to a suitable CG model and a new dynamic simulation started. This discontinuity could be avoided if internal degrees of freedom can be switched on and off on-the-fly within the same simulation, enabling internal dynamics of molecules in the context of multiscaling. Such ideas have been proposed and applied to liquids.266 In this method, the system is divided into regions, with molecules in each region treated at different resolutions. When a molecule moves from one region to another, it must adapt to the new scale, and this is accomplished in practice through an interpolating algorithm. The method accounts for the number of degrees of freedom associated with the different resolutions as the particles hope between regions, so MD simulations can be performed at constant temperature using a thermostat. The conceptual connection between both methods lies in how the system morphs between scales: in one case, through the interpolation of forces,266 in the other, through interpolation of the potentials.267
5.3. Machine learning: prediction of nano-bio behavior and inverse NP design
The in vitro and in silico approaches discussed so far in this review can be combined with a data-driven method in a three-pronged strategy. This third approach falls under the general name of Machine Learning (ML).271,272 It uses available data, learns from them, models a pattern, and produces new data not immediately apparent from the input set. The new information can then be used to design in vitro experiments, novel materials with desirable properties, or improve the FFs for more reliable simulations.
Despite their long history, ML approaches applied to the nanoscale have only recently begun to be exploited and still face many challenges. ML methods encompass a vast array of techniques. A recent survey271 has identified over forty approaches that can be used in nanoscale engineering and inverse design. Among these are traditional, well-established methods, such as artificial neural networks (ANNs), including convolution, recurrent, and deep nets; regression methods; support vector machines; and others still in development.
ML methods used for signal processing (e.g., vision or speech recognition, medical images) benefit from vast amounts of available data, even artificially generated, for training and testing. By contrast, systematic data acquisition in physical or chemical systems is difficult, especially in the nanoscale, for which fine-tune manufacturing and characterization are technically challenging.
The scarcity of training data can be overcome with CG simulations. Multiscaling, in particular, can be used to explore features of the usNP design that are difficult to probe with available technology (e.g., high aspect ratios or coatings with specific densities or compositions). The process was illustrated in a recent systematic study of the interactions of usNPs with phospholipid bilayers.34 The efficiency of the multiscaling method discussed in Section 5.2 allowed us to investigate the dependence of biding modes, energies, and early-stage membrane penetration on usNP shape, size, and layer composition. The data thus generated were used to train an ANN for reverse engineering usNPs with preferential nano-membrane behaviors.70 The use of ANNs for pattern recognition is well established,273 versatile enough to study a variety of physicochemical problems, and can incorporate increasing levels of complexity into the NP design through network arrays (see below). ANNs have recently been used to design multi-layer nanophotonic devices,274 graphene-based metamaterials,275 and metasurfaces276 with desired properties. Different types of ANN architectures have been used depending on the application. We used a single, shallow, multi-output net, the connectivity of which was suggested by the known physics of the system. Incorporating as much physicochemical information as possible in the net design may improve training, reduce redundancy, and enhance efficiency and predictive power.
Safe and efficient use of synthetic usNPs in diagnostics and therapeutics requires multiple independent conditions to be satisfied. For example, aggregation of NPs must be limited in different biological environments; the structural integrity of the coating layer must be preserved during circulation; NPs must have low affinity for plasma proteins and escape detection by the immune system; NPs should accumulate efficiently in target cells, be selective for proteins or membranes, and bind with specificity to target sites to control function or morphology. The ability to integrate data from each of these areas, possibly coming from different laboratories and techniques, may help design usNP for in vivo applications. This data integration could be accomplished through interconnected arrays of ANNs, each optimized independently for a particular property. The array can then be used to find design parameters that best satisfy the many independent behaviors imposed on the NPs. The inherent complexity of the biological environment is thus reduced to several smaller, independent problems, each simpler to handle with its own specially designed ANNs. The concept was illustrated by the design of several usNPs displaying preferential interactions with membranes.70 This kind of approach can be used, for example, in the design of usNPs as antimicrobial agents (cf. Section 6.3), as the mechanisms of action and the structural requirement for the particle to reach and disrupt the inner bacterial membrane is multifaceted.277,278 Similar ML-based ideas are currently being used with encouraging results in the design of antimicrobial peptides.279,280
6. Biomedical applications of metallic usNPs and NCs
Classical NPs have advanced through decades of research and development, and are now used successfully in several biomedical applications, with over a hundred types having already met regulatory approval or currently undergoing clinical trials.281 usGNPs are a relatively new concept, but have found remarkable use in biological and biomedical applications, including but not limited to protein recognition, biosensing, biolabeling, cellular imaging, drug delivery, and disease diagnosis and therapy. Next, we briefly highlight emerging biomedical applications of usGNPs, limiting the discussion to those cases in which non-covalent usGNP–protein interactions are involved in some respect. Due to space limitations, we only provide illustrative examples of applications rather than an exhaustive review of published papers. The reader is referred to additional reports where the myriad biomedical applications of usGNPs are reviewed more broadly.1,14,87,282–285
6.1. Modulation of protein function
usGNPs can be generally viewed as protein mimics. Indeed, both experiments and simulations have shown that usGNP–protein interactions may retain features of a typical biomolecular complexation event, such as a “well-defined” binding site,22,23,64,286 binding reversibility,24 reversibility of conformational changes upon dissociation,63 and similar thermodynamic152 and kinetic signatures.47 usGNPs thus constitute attractive model systems to mimic the structure and action of biomolecules. For example, the Rotello group has systematically explored usGNPs as artificial scaffolds for protein surface recognition and the modulation of protein function, looking specifically into the effects of the NP surface chemistry (including charge and hydrophobicity) on the structure, stability and catalytic properties of enzymes.45,287 One interesting observation was the much higher rates of hydrolysis of cationic substrates over anionic ones when chymotrypsin was complexed to anionic usGNPs.287 To explain the results, it was proposed that the NP monolayer regulated both the capture of substrate by the active site and the release of product via electrostatic interactions. In another study, Jiang and co-workers looked at the effects of the NP surface chemistry on the structure and enzymatic activity of chymotrypsin.25 They reported that DHLA-coated GNCs caused a significant change in the secondary structure of chymotrypsin and a corresponding ∼80% decrease in the activity, whereas GSH-coated GNCs produced only a slight change in structure and no significant inhibition. This difference in behavior was attributed to the occurrence of hydrophobic interactions between the chymotrypsin surface and the carbon chains of DHLA.
Recently, we demonstrated that usGNPs could perform as allosteric modifiers of enzyme activity (Fig. 6a).23,63 Allostery is a powerful concept: it entails changes in substrate binding affinity or catalytic activity at the active site in response to effector binding to allosteric (distal) sites.288,289 Allosteric regulation is unique in that it can access a wide range of functional responses, including enzyme activation, partial or full inhibition. Using α-thrombin as a model enzyme, we showed that anionic AuMBA and AuECYN (passivated with the peptidic Ac-ECYN biomimetic coat) bound to the cationic exosites 1 and 2 on the surface of thrombin, with AuECYN demonstrating a remarkable binding selectivity to exosite 2. NP complexation allosterically transmitted long-range structural changes to the active site, leading to a partial, submaximal inhibition of the activity. It was further established that long-term (24 h) interactions with AuMBA disrupted the optimum active-site geometry and irreversibly inactivated the enzyme, whereas AuECYN sustained a constant level of inhibition over time. In sum, these results revealed how the unique binding modes of different NP types could differentially modulate the structure and function of an enzyme's active site, while all at the same time preserving the overall folded state of the protein.
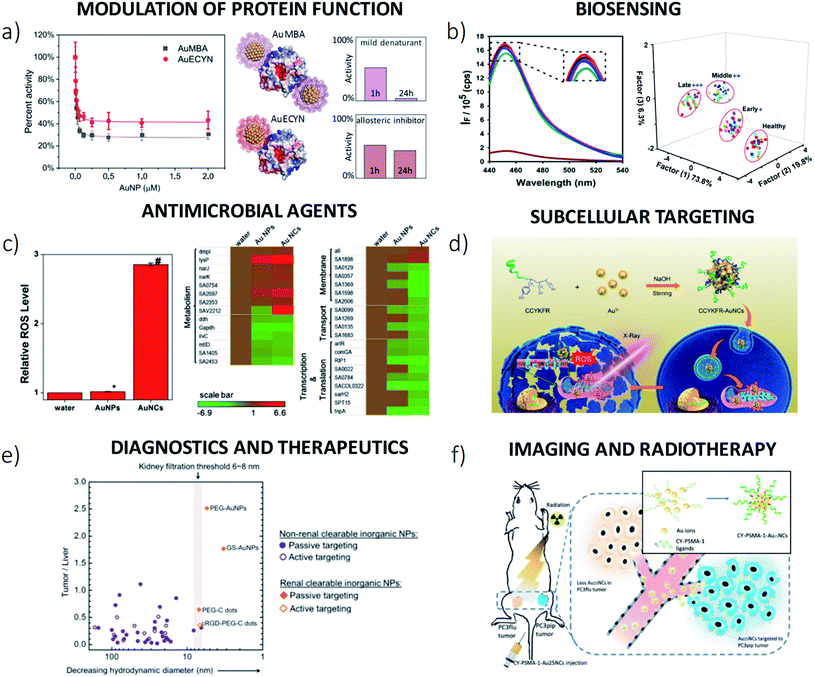 |
| Fig. 6 Applications of usGNPs. (a) Allosteric regulation of α-thrombin enzymatic activity.23 Complexation to exosites 1 and 2 caused partial, sub-maximal inhibition of activity (left panel). Over time, AuMBA inactivated the enzyme, whereas AuECYN sustained a constant level of inhibition (right panels). (b) Biosensing applications. Left panel: sensing of a post-translational modification enzyme, histone deacetylase 1 (HDAC).292 Shown are fluorescence spectral responses of peptide-templated fluorescent GNCs to different proteins: blank (red), HDAC (brown), HDAC plus its specific inhibitor trichostatin A (green), other proteins (black, pink, and blue). Fluorescence quenching observed with HDAC only. Right panel: array sensing for disease diagnosis using six amino acid-decorated fluorescent GNCs.72 Shown is a canonical score plot discriminating serum samples from breast cancer patients at different stages of the disease (early, middle, and late) and healthy subjects. (c) Antimicrobial activity of GNCs.302 GNCs (core size < 2 nm) induced intracellular ROS production in Staphylococcus aureus leading to cell death, whereas standard 6 nm-sized NPs did not (left panel). GNCs greatly affected gene expression related to cell metabolism, substrate transport, membrane integrity, and transcriptomic processes (middle and right panels); red and green designate gene up- and down-regulation, respectively. (d) Targeting of subcellular compartments in cancer cells.316 Schematics of a GNC templated with a short peptide enabling selective targeting of mitochondria following endocytosis. The GNCs acted as radiosensitizers, leading to cell death upon X-ray irradiation. (e) Tumor-to-liver ratios of conventional NPs and renal-clearable usNPs in subcutaneous tumor mouse model following intravenous injection.9 (f) Active in vivo tumor targeting of GNCs.11 Schematics of GNCs templated with a highly selective PSMA ligand for prostate cancer. The GNCs showed enhanced targeting and improved radiation therapy toward PSMA-expressing tumor cells (blue) relative to PSMA-negative tumor cells (light orange). Adapted with permission from ref. 23 ((a); Copyright 2007 American Chemical Society), ref. 292 ((b), left panel; Copyright 2013 American Chemical Society), ref. 72 ((b), right panel; Copyright 2017 Elsevier), ref. 302 ((c), Copyright 2017 American Chemical Society), ref. 316 ((d), published by the Royal Society of Chemistry), ref. 9 ((e), Copyright 2015 American Chemical Society), and ref. 11 ((f), Copyright 2019 Wiley-VCH Verlag GmbH & Co. KGaA). | |
Besides usGNPs, classical NPs also offer an incredible sum of possibilities for the control of protein structure, stability, and function. For example, NP-induced enzymatic enhancement has been impressively demonstrated by means of the covalent attachment of either enzyme or substrate onto colloidal inorganic NPs. More information on this topic can be found in the works of Medintz and Algar, among others.290,291
6.2. Biosensing applications
usGNPs have been successfully developed for both the specific and selective (array-based) sensing of proteins. The former pertains to the use of usGNPs functionalized with recognition elements (such as peptides, proteins, or aptamers) for binding to specific proteins in biofluids, while keeping interactions with nonspecific proteins to a minimum. For example, Wen et al. demonstrated the use of peptide-templated fluorescent gold nanoclusters (GNCs) for sensing of the post-translational modification enzymes histone deacetylase 1 and protein kinase A (Fig. 6b, left panel).292 Accordingly, enzymatic modification of the peptides damaged the protective coating around the metallic core and quenched the particle fluorescence, hence enabling the real-time and label-free detection of the tested enzymes. Peptide-templated usGNPs have also been developed into protease-responsive nanosensors for disease monitoring in vivo, as recently reported by Stevens, Bathia and co-workers.5 Specifically, usGNPs were templated with a peptide substrate containing recognition motifs for thrombin or matrix metalloproteinase 9, as well as a biotin handle for conjugating to a neutravidin carrier protein. Disassembly of the larger neutravidin-particle complex by peptide cleavage in response to dysregulated protease activity led to the release of the usGNPs in the urine, thus enabling a direct calorimetric readout of disease state.
The array-based sensing approach, in turn, relies on selective and cross-reactive analyte recognition by multiple sensor elements to generate unique fingerprints of the sample under study.293–296 The response pattern of sensor arrays is controlled in a synergistic manner by sensor interactions with all sample constituents, including proteins, small molecules, and ions. This sensing approach (reminiscent of olfaction/taste) complements the more traditional detection systems, in which peptides, antibodies or aptamers are employed to identify biomolecules within complex mixtures with high specificity. Array-based sensing is a powerful strategy for disease detection in situations where traditional immunosensing has limitations, such as in the simultaneous detection of multiple proteins for proper diagnosis, or when specific biomarkers are not available. Fluorescent GNCs constitute attractive building blocks for the generation of protein sensing arrays since they can singly act as selective recognition elements and signal transducers (the latter owing to the sensitivity of the fluorescence emission of the GNCs to protein binding). For example, Yuan et al. synthesized eight dual-ligand GNCs and demonstrated the ability of such a sensing system to discriminate among 8 different proteins in buffer.297 In another study, Luo and co-workers prepared six different amino acid-decorated GNCs to use as selective sensors for protein discrimination and disease diagnosis. Significantly, they showed that serum samples from healthy subjects and from breast cancer patients at different stages of the disease could be well distinguished by the sensor array (Fig. 6b, right panel).72 Array-based sensing can also be applied to discriminate cell types (e.g., healthy vs. cancerous) or to assess cellular response to drug administration.298,299 For example, Tao et al. implemented seven dual-ligand GNCs in a sensor array to distinguish healthy, cancerous and metastatic human breast cancer cells.300
The integration of specificity and selectivity within the same sensor platform could provide superior discriminating power and higher stability against background variations.295 For example, class specificity – that is, the ability to interact preferentially to certain classes of analytes – could be incorporated into usGNPs by rational design of their surface chemistries.
6.3. Detection and killing of pathogenic bacteria
usGNPs can be exploited for the efficient detection and killing of pathogenic bacteria.301 For example, Xie and co-workers prepared anionic usGNPs with effective wide-spectrum antimicrobial activity.302 The particles killed both Gram-negative and Gram-positive bacteria by way of inducing a metabolic imbalance in the cells and generating intracellular reactive oxygen species (ROS). Interestingly, a related antimicrobial activity was absent with larger (d = 6 nm) NPs (Fig. 6c). These same authors later investigated the effects of surface chemistry on the antimicrobial properties of GNCs of identical core sizes.303 They found (somewhat surprisingly) that GNCs displaying more negatively charged surfaces exhibited higher bacterial killing efficiencies. This behavior was ascribed to the generation of higher levels of ROS with the more anionic particles. In another study, Rotello and co-workers demonstrated the importance of surface hydrophobicity on the antimicrobial activity of cationic usGNPs against multi-drug-resistant bacteria.304
As illustrated above, usGNPs can kill pathogenic bacteria without having to rely on the recognition of surface receptors. Nonetheless, biospecificity can be conferred to usGNPs by engineering recognition elements on the particle monolayer. For instance, Mukherji et al. synthesized GNCs decorated with quorum sensing signal molecules for the selective detection of bacterial strains expressing quorum sensing receptors.305 Gao and co-workers prepared fluorescent GNCs surface-coated with a peptide having high binding specificity to Staphylococcus aureus.306 Besides enabling bacterial detection, the peptide-templated GNCs displayed effective antimicrobial activity by virtue of membrane disruption and increased ROS production. Chan et al. showed that mannose-capped GNCs could bind selectively to an uropathogenic Escherichia coli strain containing mannose-binding receptors.307 Presumably, usGNPs decorated with different glycan moieties could be employed to detect and kill bacteria expressing distinct glycan receptors.
Finally, Jiang and co-workers reported the preparation of GNCs terminated with quaternary ammonium cations to serve as potent antibiotics against multi-drug-resistant Gram-positive bacteria in vivo.308 The antimicrobial activity of the particles derived from a combination of membrane disruption, metabolic disturbance and ROS generation. The GNCs did not induce drug resistance and, remarkably, showed no detectable toxicity in vivo, thus raising the prospect for further clinical use.
6.4.
In vitro cell biology applications
Luminescent GNCs have been intensively investigated as novel nanoscale probes for cell biology applications, offering many potential advantages in relation to conventional organic dyes and quantum dots, such as ultrasmall sizes, facile synthesis, large Stoke shifts, color tunability, long emission lifetimes (up to the μs range), environmentally sensitive emissions, photo and chemical stability, and biocompatibility.309 Possible cell biology applications of usGNPs and GNCs include bioimaging,309 gene delivery,310 monitoring intracellular pH and temperature changes,311–313 among many others. Of particular interest, GNCs coated with targeting moieties can be used to label specific subcellular compartments for imaging or even the manipulation of cell state and behavior. In an earlier study, Gao and co-workers developed a peptide-templated fluorescent GNC containing a TAT nuclear localization sequence for the targeted staining of cell nuclei.314 The same authors later prepared GNCs functionalized with a short peptide sequence specific for αIIbβ3 integrin receptors.315 The number of receptors per cell was then estimated by quantifying the gold content in single cells using laser ablation ICP-MS. In another application, Liu and co-workers prepared peptide-templated fluorescent GNCs and demonstrated their targeting/accumulation on the mitochondria of cancer cells following endocytosis.316 The internalized GNCs acted as radiosensitizers, inducing significant mitochondrial oxidative stress and DNA damage upon X-ray irradiation, leading to cell death (Fig. 6d).
At this point, we recall that usGNPs functionalized with antibodies or Fab fragments have a long history of use as immunolabeling reagents for electron microscopy.317 Given the new developments in the area of luminescent metallic NCs, it is conceivable that peptide- or protein-templated GNCs could turn into valuable tools for correlative light-electron microscopy studies, insofar as these novel nanomaterials may singly integrate the properties of specific antigen binding, fluorescence emission (thus supporting light microscopy imaging), and high electron scattering power (thus supporting high-resolution electron microscopy imaging318). Incidentally, such a powerful combined approach could furnish a better understanding of the mechanisms of cell entry and intracellular trafficking of usGNPs.319–322
6.5.
In vivo disease diagnosis and therapy
The last decade has seen a surge in the development of usGNP-based platforms for in vivo disease diagnosis and treatment. Due to space limitations, here we consider only applications of usGNPs for cancer therapy.14,323
Studies so far have confirmed that usGNPs are biocompatible,15 at least partly due to the intrinsic short-lived nature of their interactions with proteins.24 usGNPs can be rapidly cleared from blood circulation through the kidneys (clearances over 50% at 24 h p.i. have been routinely reported) and escape liver uptake.9,324 Tumor uptake levels of non-targeted usGNPs are generally around ∼1–15% ID per g of tissue, which is a typical range for larger NPs as well.9 The combined result is that usGNPs may display significantly higher tumor-to-liver rations relative to their classical counterparts (Fig. 6e).9
In a groundbreaking study, Zheng and co-workers reported that the in vivo behavior of atomically precise GNCs was size-dependent.35 They found that smaller particles were more efficiently trapped by the glomerular glycocalyx (an effect similar to the separation principle in size-exclusion chromatography) and displayed longer blood retention times. As a result, a decrease of just a few atoms (Au25 to Au10) in NC size enhanced the passive tumor uptake of GNCs from about 2 to 8% ID g−1. These results underscore the complexity and, sometimes, unpredictability of nano-bio interactions in vivo.
So far, usNPs have been developed as self-therapeutic agents,325 drug delivery vehicles,326 radiosensitizers for cancer radiotherapy,6,327,328 among other applications in cancer nanomedicine. However, despite promising results so far, commonly encountered limitations such as restricted tumor uptake and off-target localization may hamper the clinical applications of usGNPs. Researchers have therefore been developing targeted usGNPs in an attempt to improve their tumor accumulation profiles. For example, Liang et al. observed a higher accumulation of usGNPs modified with a cyclic-RGD peptide in ανβ3-positive tumors relative to their non-targeted counterparts (6.4 ± 1.3% vs. 2.0 ± 1.8% ID/g tissue).8 The enhanced tumor localization of the targeted usGNPs produced a stronger radiosensitizing effect leading to a reduced tumor mass over time. In another study, Basilion and co-workers prepared GNCs covered with a high-affinity targeting ligand for the prostate-specific membrane antigen (PSMA) receptor, which is over-expressed in most prostate cancers. This active targeting strategy significantly improved both tumor accumulation in mice (8.9% ID/g tissue) and the outcome of radiotherapy relative to a passive targeting approach (Fig. 6f).11
Further advancements in the field might benefit from a more quantitative knowledge of the interactions of both targeted and non-targeted usGNPs with proteins. For example, it is typically not known whether targeted ligands on usGNPs are able to maintain the same affinity of binding toward their receptors in comparison to free ligands in solution. In addition, although usGNPs may be deemed highly inert against nonspecific protein binding, the extent of this resistance is generally not known. We recently implemented a simple pharmacokinetic model simulation to understand how the tumor uptake of usGNPs could be modulated by ultraweak (KD > 1 mM) nonspecific interactions between usGNPs and serum proteins.329 The results revealed that under “high” rates of NP plasma clearance and “slow” rates of vascular extravasation, ultraweak interactions – but not active targeting – would increase the tumor accumulation of the particles (by essentially slowing-down particle clearance from circulation). On the other hand, under “high” rates of plasma clearance and “high” rates of vascular extravasation and intravasation, high-affinity active targeting – but not ultraweak interactions – would enhance tumor uptake levels. Despite its underlying simplicity and many approximations, the model has provided a basic framework to quantitatively understand the blood and tumor pharmacokinetics of usGNPs under the influence of ultraweak protein interactions.
7. Outlook and concluding remarks
Compared to their larger counterparts, usGNPs display unique physicochemical properties and interaction modalities with biomolecular structures, providing a new paradigm in nanobiomedical research with potential impact in translational medicine. On the one hand, usGNPs represent powerful tools for fundamental research, helping to generate new knowledge on biosystems, all the way from single proteins to whole-organism physiology. At the same time, usGNPs have been intensively exploited in a remarkable number of biological and biomedical applications, both in vitro and in vivo, including biosensing, bioimaging, drug delivery, and cancer theranostics.
We have emphasized how unleashing the full potential of usGNPs in both fundamental and applied research will require a more in-depth understanding of their biomolecular interactions, much in the same way that a detailed knowledge of protein–protein complexation is a prerequisite to understanding higher-order biological processes. Notwithstanding the current gaps in our knowledge, it is clear that much is already known about the properties and behavior of usGNPs in complex biosystems and how such properties and behavior can be reasonably manipulated by nanoengineering. All in all, usGNPs appear ready to impact real-world clinical applications, as has already been the case for classical NPs.
Future advances in usNP-based biomedicine will likely depend on systematic studies through an integrated approach of experiments in vitro for strict control of environmental effects, computer simulations with advanced multiscaling forcefields, and data-driven techniques for reverse-engineering NPs and NCs with desirable properties. However, progress is needed in all these fronts, including better techniques to manufacture and characterize ultrasmall nanostructures (nanoengineering, chemical synthesis) and a clearer picture of the interfacial forces at the sub-nanometer length scale, particularly in usNP/liquid boundaries (theory and experiments). We have addressed some of the topics in this review.
Conflicts of interest
There are no conflicts to declare.
Acknowledgements
AAS was supported by the São Paulo Research Foundation (FAPESP, grant #19/04372-6); PS was supported by the NIH Intramural Research Program of NIBIB; and SAH was supported by the NIH Intramural Research Program through the CIT and NIAID and utilized the high-performance computational capabilities of the Biowulf HPC cluster at the NIH.
References
- K. Zarschler,
et al., Ultrasmall inorganic nanoparticles: state-of-the-art and perspectives for biomedical applications, Nanomedicine, 2016, 12, 1663–1701 CrossRef CAS PubMed.
- A. Leifert, Y. Pan-Bartnek, U. Simon and W. Jahnen-Dechent, Molecularly stabilised ultrasmall gold nanoparticles: synthesis, characterization and bioactivity, Nanoscale, 2013, 5, 6224–6242 RSC.
- I. Chakraborty and T. Pradeep, Atomically precise clusters of noble metals: emerging link between atoms and nanoparticles, Chem. Rev., 2017, 117, 8208–8271 CrossRef CAS PubMed.
- R. Jin, C. Zeng, M. Zhou and Y. Chen, Atomically precise colloidal metal nanoclusters and nanoparticles: fundamentals and opportunities, Chem. Rev., 2016, 116, 10346–10413 CrossRef CAS PubMed.
- C. N. Loynachan,
et al., Renal clearable catalytic gold nanoclusters for in vivo disease monitoring, Nat. Nanotechnol., 2019, 14, 883–890 CrossRef CAS PubMed.
- X.-D. Zhang,
et al., Ultrasmall glutathione-protected gold nanoclusters as next generation radiotherapy sensitizers with high tumor uptake and high renal clearance, Sci. Rep., 2015, 5, 8669 CrossRef CAS PubMed.
- J. Wang and G. Liu, Imaging nano-bio interactions in the kidney: toward a better understanding of nanoparticle clearance, Angew. Chem., Int. Ed., 2018, 57, 3008 CrossRef CAS PubMed.
- G. Liang, X. Jin, S. Zhang and D. Xing, RGD peptide-modified fluorescent gold nanoclusters as highly efficient tumor-targeted radiotherapy sensitizers, Biomaterials, 2017, 144, 95–104 CrossRef CAS PubMed.
- M. Yu and J. Zheng, Clearance pathways and tumor targeting of imaging nanoparticles, ACS Nano, 2015, 9, 6655–6674 CrossRef CAS.
- C. Lopez-Chavez,
et al., Gold nanoparticles: Distribution, bioaccumulation and toxicity. In vitro and in vivo studies, Nanomedicine, 2018, 14, 1 CrossRef PubMed.
- D. Luo,
et al., Targeted Gold Nanocluster-Enhanced Radiotherapy of Prostate Cancer, Small, 2019, 15, 1900968 CrossRef PubMed.
- V. Sokolova,
et al., Ultrasmall gold nanoparticles (2 nm) can penetrate and enter cell nuclei in an in vitro 3D brain spheroid model, Acta Biomater., 2020, 111, 349–362 CrossRef CAS PubMed.
- S. Huo,
et al., Ultrasmall Gold Nanoparticles as Carriers for Nucleus-Based Gene Therapy Due to Size-Dependent Nuclear Entry, ACS Nano, 2014, 8, 5852–5862 CrossRef CAS PubMed.
- M. Fan,
et al., Ultrasmall gold nanoparticles in cancer diagnosis and therapy, Theranostics, 2020, 10, 4944 CrossRef CAS PubMed.
- J. Xu,
et al., Dose dependencies and biocompatibility of renal clearable gold nanoparticles: From mice to non-human primates, Angew. Chem., Int. Ed., 2018, 130, 272–277 CrossRef.
- Y. Pan,
et al., Size-dependent citotoxicity of gold nanoparticles, Small, 2007, 3, 1941–1949 CrossRef CAS PubMed.
- G. Schmid, W. G. Kreyling and U. Simon, Toxic effects and biodistribution of ultrasmall gold nanoparticles, Arch. Toxicol., 2017, 91, 3011–3037 CrossRef CAS PubMed.
- A. K. Murthy,
et al., Charged gold nanoparticles with essentially zero serum protein adsorption in undiluted fetal bovine serum, J. Am. Chem. Soc., 2013, 135, 7799–7802 CrossRef CAS PubMed.
- T. Mizuhara,
et al., Acylsulfonamide-functionalized zwitterionic gold nanoparticles for enhanced cellular uptake at tumor pH, Angew. Chem., Int. Ed., 2015, 54, 6567–6570 CrossRef CAS PubMed.
- D. An,
et al., A Peptide-Coated Gold Nanocluster Exhibits Unique Behavior in Protein Activity Inhibition, J. Am. Chem. Soc., 2015, 137, 8412–8418 CrossRef CAS PubMed.
- M.-C. Bowman,
et al., Inhibition of HIV Fusion with Multivalent Gold Nanoparticles, J. Am. Chem. Soc., 2008, 130, 6896–6897 CrossRef CAS PubMed.
- M. Kopp, S. Kollenda and M. Epple, Nanoparticle–protein interactions: therapeutic approaches and supramolecular chemistry, Acc. Chem. Res., 2017, 50, 1383–1390 CrossRef CAS PubMed.
- A. L. Lira,
et al., Allosteric inhibition of α-thrombin enzymatic activity with ultrasmall gold nanoparticles, Nanoscale Adv., 2019, 1, 378–388 RSC.
- R. S. Ferreira, A. L. Lira, R. J. Torquato, P. Schuck and A. A. Sousa, Mechanistic Insights into Ultrasmall Gold Nanoparticle–Protein Interactions through Measurement of Binding Kinetics, J. Phys. Chem. C, 2019, 123, 28450–28459 CrossRef CAS.
- W.-Q. Chen,
et al., Thermodynamics,
Kinetics and Mechanisms of Non-competitive Allosteric Inhibition of Chymotrypsin by Dihydrolipoic Acid-Coated Gold Nanoclusters, Langmuir, 2020, 36, 6447–6457 CrossRef CAS PubMed.
- Z. Yang,
et al., Self-assembly of semiconducting-plasmonic gold nanparticles with enhanced optical property for photoacustic imaging and photothermal therapy, Theranostics, 2017, 7, 2177–2185 CrossRef CAS PubMed.
- N. S. Abadeer and C. J. Murphy, recent progress in cancer thermal therapy using gold nanoparticles, J. Phys. Chem. C, 2016, 120, 4691–4716 CrossRef CAS.
- P.-C. Chen,
et al., Interface and heterostructure design in polyelemental nanoparticles, Science, 2019, 363, 959 CrossRef CAS PubMed.
- T. Cedervall,
et al., Understanding the nanoparticle–protein corona using methods to quantify exchange rates and affinities of proteins for nanoparticles, Proc. Natl. Acad. Sci. U. S. A., 2007, 104, 2050–2055 CrossRef CAS PubMed.
- E. Casals, T. Pfaller, A. Duschl, G. J. Oostingh and V. Puntes, Time evolution of the nanoparticle protein corona, ACS Nano, 2010, 4, 3623–3632 CrossRef CAS PubMed.
- S. H. D. P. Lacerda,
et al., Interaction of gold nanoparticles with common human blood proteins, ACS Nano, 2010, 4, 365–379 CrossRef PubMed.
- J. Piella, N. G. Bastús and V. Puntes, Size-dependent protein–nanoparticle interactions in citrate-stabilized gold nanoparticles: the emergence of the protein corona, Bioconjugate Chem., 2017, 28, 88–97 CrossRef CAS PubMed.
- M. Tsoli, H. Kuhn, W. Brandau, H. Esche and G. Schmid, Cellular Uptake and Toxicity of Au55 Clusters, Small, 2005, 1, 841–844 CrossRef CAS PubMed.
- S. A. Hassan, Strong Dependence of the Nano-Bio Interactions on Core Morphology and Layer Composition of Ultrasmall Nanostructures, J. Chem. Phys., 2019, 151, 105102 CrossRef PubMed.
- B. Du,
et al., Glomerular barrier behaves as an atomically precise bandpass filter in a sub-nanometre regime, Nat. Nanotechnol., 2017, 12, 1096–1102 CrossRef CAS PubMed.
- V. Petkov,
et al., Structure of gold nanoparticles suspended in water studied by X-ray diffraction and computer simulations, Phys. Rev. B: Condens. Matter Mater. Phys., 2005, 72, 1954402 CrossRef.
- I. L. Garzon,
et al., Lowest Energy Structures of Gold Nanoclusters, Phys. Rev. Lett., 1998, 81, 1600–1603 CrossRef CAS.
- N. Tarrat, M. Rapacioli and F. Spiegelman, Au147 nanoparticles: ordered or amorphous, J. Chem. Phys., 2018, 148, 204308 CrossRef PubMed.
- Y. Negishi,
et al., A critical size for emergence of nonbulk electronic and geometric structures in dodecanethiolate-protected Au clusters, J. Am. Chem. Soc., 2015, 137, 1206–1212 CrossRef CAS PubMed.
- N. Yan,
et al., Unraveling the long-pursued Au144 structure by X-ray crystallography, Sci. Adv., 2018, 4, eaat7259 CrossRef CAS PubMed.
- P. D. Jadzinsky, G. Calero, C. J. Ackerson, D. A. Bushnell and R. D. Kornberg, Structure of a Thiol Monolayer-Protected Gold Nanoparticle at 1.1 Å Resolution, Science, 2007, 318, 430–433 CrossRef CAS PubMed.
- Q. Yao, X. Yuan, T. Chen, D. Tai Leong and J. Xie, Engineering Functional Metal Materials at the Atomic Level, Adv. Mater., 2018, 30, 1802751 CrossRef PubMed.
- C. Zeng, Y. Chen, K. Kirschbaum, K. J. Lambright and R. Jin, Emergence of hierarchical structural complexities in nanoparticles and their assembly, Science, 2016, 354, 1580–1584 CrossRef CAS PubMed.
- C. J. Ackerson, P. D. Jadzinsky and R. D. Kornberg, Thiolate ligands for synthesis of water-soluble gold clusters, J. Am. Chem. Soc., 2005, 127, 6550–6551 CrossRef CAS PubMed.
- C.-C. You, M. De, G. Han and V. M. Rotello, Tunable inhibition and denaturation of α-chymotrypsin with amino acid-functionalized gold nanoparticles, J. Am. Chem. Soc., 2005, 127, 12873–12881 CrossRef CAS.
- L. Boselli, E. Polo, V. Castagnola and K. A. Dawson, Regimes of biomolecular ultrasmall nanoparticle interactions, Angew. Chem., Int. Ed., 2017, 129, 4279–4282 CrossRef.
- R. S. Ferreira, A. L. Lira and A. A. Sousa, Quantitative Mechanistic Model for Ultrasmall Nanoparticle-Protein Interactions, Nanoscale, 2020, 12, 19230–19240 RSC.
- W. Shang, J. H. Nuffer, J. S. Dordick and R. W. Siegel, Unfolding of ribonuclease A on silica nanoparticle surfaces, Nano Lett., 2007, 7, 1991–1995 CrossRef CAS PubMed.
- W. Shang,
et al., Cytochrome c on silica nanoparticles: influence of nanoparticle size on protein structure, stability, and activity, Small, 2009, 5, 470–476 CrossRef CAS PubMed.
- A. A. Vertegel, R. W. Siegel and J. S. Dordick, Silica nanoparticle size influences the structure and enzymatic activity of adsorbed lysozyme, Langmuir, 2004, 20, 6800–6807 CrossRef CAS PubMed.
- D. Baimanov, R. Cai and C. Chen, Understanding the chemical nature of nanoparticle–protein interactions, Bioconjugate Chem., 2019, 30, 1923–1937 CrossRef CAS.
- A. A. Sousa,
et al., Biointeractions of ultrasmall glutathione-coated gold nanoparticles: effect of small size variations, Nanoscale, 2016, 8, 6577–6588 RSC.
- S. A. Hassan, Computational Study of the Forces Driving Aggregation of Ultrasmall Nanoparticles in Biological Fluids, ACS Nano, 2017, 11, 4145 CrossRef CAS PubMed.
- H. Bayraktar, P. S. Ghosh, V. M. Rotello and M. J. Knapp, Disruption of protein–protein interactions using nanoparticles: inhibition of cytochrome c peroxidase, Chem. Commun., 2006,(13), 1390–1392 RSC.
- S. Xu,
et al., Ultrahighly Efficient and Stable Fluorescent Gold Nanoclusters Coated with Screened Peptides of Unique Sequences for Effective Protein and Serum Discrimination, Anal. Chem., 2019, 91, 13947–13952 CrossRef CAS PubMed.
- F. Compostella, O. Pitirollo, A. Silvestri and L. Polito, Glyco-gold nanoparticles: synthesis and applications, J. Org. Chem., 2017, 13, 1008–1021 Search PubMed.
- Z. Dai, Y. Tan, K. He, H. Chen and J. Liu, Strict DNA Valence Control in Ultrasmall Thiolate-Protected Near-Infrared-Emitting Gold Nanoparticles, J. Am. Chem. Soc., 2020, 142, 14023–14027 CrossRef CAS PubMed.
- D. F. Moyano,
et al., Fabrication of Corona-Free Nanoparticles with Tunable Hydrophobicity, ACS Nano, 2014, 8, 6748–6755 CrossRef CAS PubMed.
- E. Porret,
et al., Hydrophobicity of gold nanoclusters influences their interactions with biological barriers, Chem. Mater., 2017, 29, 7497–7506 CrossRef CAS.
- P. Maffre,
et al., Effects of surface functionalization on the adsorption of human serum albumin onto nanoparticles–a fluorescence correlation spectroscopy study, Beilstein J. Nanotechnol., 2014, 5, 2036–2047 CrossRef PubMed.
- L. Shang,
et al., Nanoparticles interacting with proteins and cells: a systematic study of protein surface charge effects, Adv. Mater. Interfaces, 2014, 1, 1300079 CrossRef.
- K. Chen,
et al., Electrostatic selectivity in protein–nanoparticle interactions, Biomacromolecules, 2011, 12, 2552–2561 CrossRef CAS PubMed.
- A. L. Lira, R. S. Ferreira, M. L. V. Oliva and A. A. Sousa, Regulation of Thrombin Activity with Ultrasmall Nanoparticles: Effects of Surface Chemistry, Langmuir, 2020, 36, 7991–8001 CrossRef CAS PubMed.
- H. Bayraktar, C.-C. You, V. M. Rotello and M. J. Knapp, Facial control of nanoparticle binding to cytochrome c, J. Am. Chem. Soc., 2007, 129, 2732–2733 CrossRef CAS PubMed.
- C. C. You, S. S. Agasti and V. M. Rotello, Isomeric control of protein recognition with amino acid-and dipeptide-functionalized gold nanoparticles, Chem.–Eur. J., 2008, 14, 143–150 CrossRef CAS PubMed.
- K. Chen,
et al., Optimizing the selective recognition of protein isoforms through tuning of nanoparticle hydrophobicity, Nanoscale, 2014, 6, 6492–6495 RSC.
- H. Koide,
et al., A polymer nanoparticle with engineered affinity for a vascular endothelial growth factor (VEGF 165), Nat. Chem., 2017, 9, 715 CrossRef CAS PubMed.
- M. Nakamoto, D. Zhao, O. R. Benice, S.-H. Lee and K. J. Shea, Abiotic Mimic of Endogenous Tissue Inhibitors of Metalloproteinases: Engineering Synthetic Polymer Nanoparticles for Use as a Broad-Spectrum Metalloproteinase Inhibitor, J. Am. Chem. Soc., 2020, 142, 2338–2345 CrossRef CAS PubMed.
- X. Wang,
et al., Chiral surface of nanoparticles determines the orientation of adsorbed transferrin and its interaction with receptors, ACS Nano, 2017, 11, 4606–4616 CrossRef CAS.
- S. A. Hassan, Artificial neural networks for the inverse design of nanoparticles with preferential nano-bio behaviors, J. Chem. Phys., 2020, 153, 054102 CrossRef CAS PubMed.
- M. De,
et al., Sensing of proteins in human serum using conjugates of nanoparticles and green fluorescent protein, Nat. Chem., 2009, 1, 461–465 CrossRef CAS PubMed.
- S. Xu,
et al., Near infrared fluorescent dual ligand functionalized Au NCs based multidimensional sensor array for pattern recognition of multiple proteins and serum discrimination, Biosens. Bioelectron., 2017, 97, 203–207 CrossRef CAS PubMed.
- S. Xu, Y. Wu, X. Sun, Z. Wang and X. Luo, A multicoloured Au NCs based cross-reactive sensor array for discrimination of multiple proteins, J. Mater. Chem. B, 2017, 5, 4207–4213 RSC.
- C.-C. You,
et al., Detection and identification of proteins using nanoparticle–fluorescent polymer ‘chemical nose’sensors, Nat. Nanotechnol., 2007, 2, 318–323 CrossRef CAS PubMed.
- J. Liu,
et al., PEGylation and Zwitterionization: Pros and cons in the renal clearance and tumor targeting of near-IR-emitting gold nanoparticles, Angew. Chem., Int. Ed., 2013, 125, 12804–12808 CrossRef.
- K. P. García,
et al., Zwitterionic-coated “stealth” nanoparticles for biomedical applications: recent advances in countering biomolecular corona formation and uptake by the mononuclear phagocyte system, Small, 2014, 10, 2516–2529 CrossRef PubMed.
- S. Jiang and Z. Cao, Ultralow-fouling, functionalizable, and hydrolyzable zwitterionic materials and their derivatives for biological applications, Adv. Mater., 2010, 22, 920–932 CrossRef CAS PubMed.
- Q. Shao and S. Jiang, Molecular understanding and design of zwitterionic materials, Adv. Mater., 2015, 27, 15–26 CrossRef CAS PubMed.
- Z. G. Estephan, P. S. Schlenoff and J. B. Schlenoff, Zwitteration as an alternative to PEGylation, Langmuir, 2011, 27, 6794–6800 CrossRef CAS PubMed.
- J. Liu,
et al., Passive tumor targeting of renal-clearable luminescent gold nanoparticles: long tumor retention and fast normal tissue clearance, J. Am. Chem. Soc., 2013, 135, 4978–4981 CrossRef CAS PubMed.
- W. Yang, L. Zhang, S. Wang, A. D. White and S. Jiang, Functionalizable and ultra stable nanoparticles coated with zwitterionic poly (carboxybetaine) in undiluted blood serum, Biomaterials, 2009, 30, 5617–5621 CrossRef CAS PubMed.
- L. L. Knittel, P. Schuck, C. J. Ackerson and A. A. Sousa, Zwitterionic glutathione monoethyl ester as a new capping ligand for ultrasmall gold nanoparticles, RSC Adv., 2016, 6, 46350–46355 RSC.
- L. L. Knittel,
et al., Ultrasmall Gold Nanoparticles Coated with Zwitterionic Glutathione Monoethyl Ester: A Model Platform for the Incorporation of Functional Peptides, J. Phys. Chem. B, 2020, 124, 3892–3902 CrossRef CAS PubMed.
- X.-R. Song, N. Goswami, H.-H. Yang and J. Xie, Functionalization of metal nanoclusters for biomedical applications, Analyst, 2016, 141, 3126–3140 RSC.
- Y. N. Tan, J. Y. Lee and D. I. Wang, Uncovering the design rules for peptide synthesis of metal nanoparticles, J. Am. Chem. Soc., 2010, 132, 5677–5686 CrossRef CAS PubMed.
- W. Wang,
et al., Peptide-templated noble metal catalysts: syntheses and applications, Chem. Sci., 2017, 8, 3310–3324 RSC.
- Q. Yuan,
et al., Peptide protected gold clusters: chemical synthesis and biomedical applications, Nanoscale, 2016, 8, 12095–12104 RSC.
- J. Xie, Y. Zheng and J. Y. Ying, Protein-Directed Synthesis of Highly Fluorescent Gold Nanoclusters, J. Am. Chem. Soc., 2009, 131, 888–889 CrossRef CAS PubMed.
- N. Goswami, K. Zheng and J. Xie, Bio-NCs – the marriage of ultrasmall metal nanoclusters with biomolecules, Nanoscale, 2014, 6, 13328–13347 RSC.
- K. Chaudhari, P. Lourdu Xavier and T. Pradeep, Understanding the Evolution of Luminescent Gold Quantum Clusters in Protein Templates, ACS Nano, 2011, 5, 8816–8827 CrossRef CAS.
- Q.-Q. Zhuang,
et al., Immunoglobulin G-Encapsulated Gold Nanoclusters as Fluorescent Tags for Dot-Blot Immunoassays, ACS Appl. Mater. Interfaces, 2019, 11, 31729–31734 CrossRef CAS.
- N. A. Kotov, Inorganic nanoparticles as protein mimics, Science, 2010, 330, 188–189 CrossRef CAS.
- A. L. Lira,
et al., Binding kinetics of ultrasmall gold nanoparticles with proteins, Nanoscale, 2018, 10, 3235–3244 RSC.
-
J. N. Israelachvili, Intermolecular and Surface Forces, Academic Press, 3rd edn, 2011 Search PubMed.
-
J. Lyklema, Fundamentals of Interface and Colloid Science, Academic Press, Oxford, UK, 1991, vol. 1 Search PubMed.
-
J. Lyklema, Fundamentals of Interface and Colloid Science, Academic Press, Oxford, UK, 1995, vol. 2 Search PubMed.
-
J. Lyklema, Fundamentals of Interface and Colloid Science, Academic Press, Oxford, UK, 2005, vol. 5 Search PubMed.
-
H. Ohshima, Electrical Phenomena at Interfaces and Biointerfaces: Fundamentals and Applications in Nano-, Bio-, and Environmental Sciences, John Wiley & Sons, Inc., 2012 Search PubMed.
-
Colloids and Interfaces, ed. T. F. Tadros, Wiley-VCH, Weinheim, 2007, vol. 1.
- R. J. Ellis, Macromolecular Crowding: Obvious but Underappreciated, Trends Biochem. Sci., 2001, 26, 597–604 CrossRef CAS PubMed.
- Y. Phillip and G. Schreiber, Formation of protein complexes in crowded environments. from in vitro to in vivo, FEBS Lett., 2013, 587, 1046–1052 CrossRef CAS.
- H.-X. Zhou, G. Rivas and A. P. Minton, Macromolecular crowding and confinement: biochemical, biophysical, and potential physiological consequences, Annu. Rev. Biophys., 2008, 37, 375–379 CrossRef CAS PubMed.
- J. C. Crocker and D. G. Grier, When like charges attract: the effect of geometrical confinement on long-range colloidal interactions, Phys. Rev. Lett., 1996, 77, 1897 CrossRef CAS.
- J. Israelachvilli and H. Wennerstrom, Role of hydration and water structure in biological and colloidal interactions, Nature, 1996, 379, 219–225 CrossRef PubMed.
- D. F. Moyano, M. Ray and V. M. Rotello, Nanoparticle-protein interactions: water is the key, MRS Bull., 2014, 39, 1069 CrossRef CAS.
- K. Morgenstern, D. Marx, M. Havenith and M. Muhler, Editorial of the PCCP themed issue on Solvation Science, Phys. Chem. Chem. Phys., 2015, 17, 8295–8296 RSC.
- M. Valtiner, A. Erbe and A. Rosenhahn, Ions and solvation at biointerfaces, Biointerphases, 2016, 11, 018801 CrossRef PubMed.
- T. Zemb and E. Leontidis, Equilibrium in soft-matter systems under the influence of competing forces, Curr. Opin. Colloid Interface Sci., 2013, 18, 493–494 CrossRef CAS.
- D. Marenduzzo, K. Finan and P. R. Cook, The depletion attraction: an underappreciated force driving cellular organization, J. Cell Biol., 2006, 175, 681–686 CrossRef CAS PubMed.
- T. Zemb and P. A. Kralchevsky, Depletion forces in single phase and multi-phase complex fluids, Curr. Opin. Colloid Interface Sci., 2015, 20, 1–2 CrossRef CAS.
- A. Trokhymchuk and D. Henderson, Depletion forces in bulk and in confined domains: from Asakura-Oosawa to recent statistical physics advances, Curr. Opin. Colloid Interface Sci., 2015, 20, 32–38 CrossRef CAS.
- C. Pfeiffer,
et al., Interaction of colloidal nanopartivles with their environment: the (ionic) nanoenvironment around nanoparticles is different from bulk and determines the physico-chemical properties of the nanoparticles, J. R. Soc. Interface, 2017, 11, 20130931 CrossRef PubMed.
- R. P. Misra, J. P. de Souza, D. Blankschtein and M. Z. Bazant, Theory of surface forces in multivalent electrolytes, Langmuir, 2019, 35, 11550–11565 CrossRef CAS PubMed.
-
V. A. Parsegian, Van der Waals Forces: A Handbook for Biologists, Chemists, Engineers, and Physicists, Cambridge University Press, 2005 Search PubMed.
- S. A. Alsharif, L. Y. Chen, A. Tlahuice-Flores, R. L. Whetten and M. J. Yacaman, Interaction between functionalized gold nanoparticles in physiological saline, Phys. Chem. Chem. Phys., 2014, 16, 3909–3913 RSC.
- O. D. Villareal, K. Y. Chen, R. L. Whetten and M. J. Yacaman, Ligand-modulated interactions between charged monolayer-protected Au144(SR)60 gold nanoparticles ij physiological saline, Phys. Chem. Chem. Phys., 2015, 17, 3680–3688 RSC.
- I. Sogami and N. Ise, On the Electrostatic Interaction in Macroionic Solutions, J. Chem. Phys., 1984, 81, 6320 CrossRef.
- E. M. V. Hoek and G. K. Agarwal, Extended DLVO interactions between spherical particles and rough surfaces, J. Colloid Interface Sci., 2006, 298, 50–58 CrossRef CAS PubMed.
- V. A. Parsegian, R. P. Rand, N. L. Fuller and D. C. Rau, Osmotic Stress for the Direct Measurement of Intermolecular Forces, Methods Enzymol., 1986, 127, 400 CAS.
- V. A. Parsegian, R. P. Rand and D. C. Rau, Osmotic Stress, Crowding, Preferential Hydration, and Binding: a Comparison of Perspectives, Proc. Natl. Acad. Sci. U. S. A., 2000, 97, 3897 CrossRef PubMed.
- S. A. Hassan, Liquid-structure Forces and Electrostatic Modulation of Biomolecular Interactions in Solution, J. Phys. Chem. B, 2007, 111, 227 CrossRef CAS PubMed.
- P. G. Kusalik and I. M. Svishchev, The Spatial Structure in Liquid Water, Science, 1994, 265, 1219 CrossRef CAS PubMed.
-
S. A. Hassan and E. L. Mehler, in Comprehensive Biophysics, ed. E. Egelman, Academic Press, 2012 Search PubMed.
- V. A. Parsegian and D. C. Rau, Water near Intracellular Surfaces, J. Cell Biol., 1984, 99, 196 CrossRef CAS PubMed.
-
W. Drost-Hansen, in Water and the Cell, ed. G. H. Pollack, I. L. Cameron, and D. N. Wheatley, Springer, 2006 Search PubMed.
- E. Persson and B. Halle, Cell Water Dynamics on Multiple Time Scales, Proc. Natl. Acad. Sci. U. S. A., 2008, 105, 6266 CrossRef CAS PubMed.
- J. Qvist, E. Persson, C. Mattea and B. Halle, Time Scales of Water Dynamics at Biological Interfaces: Peptides, Proteins and Cells, Faraday Disc., 2009, 141, 131 RSC.
- A. Frolich,
et al., From Shell to Cell: Neutron Scattering Studies of Biological Water Dynamics and Coupling to Activity, Faraday Disc., 2009, 141, 117 RSC.
- F. Persson, P. Soderhjelm and B. Halle, The spatial range of protein hydration, J. Chem. Phys., 2018, 148, 215104 CrossRef PubMed.
- I. Andricioaei and M. Karplus, On the calculation of entropy from covariance matrices of the atomic fluctuations, J. Chem. Phys., 2001, 115, 6289–6292 CrossRef CAS.
- R. Tehver, A. Maritan, J. Koplik and J. R. Banavar, Depletion forces in hard-sphere colloids, Phys. Rev. Lett., 1999, 59, R1339–R1342 CAS.
- L. Sapir and D. Harries, Origin of enthalpic depletion forces, J. Phys. Chem. Lett., 2014, 5, 1061–1065 CrossRef CAS PubMed.
-
H. N. W. Lekkerkerker and R. Tuinier, Colloids and the Depletion Interaction, Springer, 2011 Search PubMed.
- R. Tuinier, J. Rieger and C. G. de Kruif, Depletion-induced phase separation in colloid-polymer mixtures, Adv. Colloid Interface Sci., 2003, 103, 1–31 CrossRef CAS PubMed.
- T. Yang, Z. Lei, S. Yang and E.-Q. Chen, Depletion driven self-assembly of block copolymer solutions by homopolymers, Phys. Chem. Chem. Phys., 2019, 21, 2121–2127 RSC.
- B. van den Berg, R. J. Ellis and C. M. Dobson, Effects of macromolecular crowding on protein folding and aggregation, EMBO J., 1999, 18, 6927–6933 CrossRef CAS PubMed.
- A. P. Minton, Implications of macromolecular crowding for protein asembly, Curr. Opin. Struct. Biol., 2000, 10, 34–39 CrossRef CAS PubMed.
- Y. Hata, T. Sawada and T. Serizawa, Macromolecular crowding for material-directed controlled self-assembly, J. Mater. Chem., 2018, 6, 6344–6359 RSC.
- W. T. Snead,
et al., Membrane fission by protein crowding, Proc. Natl. Acad. Sci. U. S. A., 2017, 114, 3258 CrossRef PubMed.
- J. C. Stachowiak,
et al., Membrane bending by protein-protein crowding, Nat. Cell Biol., 2012, 14, 944 CrossRef CAS PubMed.
- J. N. Adkins,
et al., Towards a human blood serum proteome, Mol. Cell. Proteomics, 2002, 1, 947–955 CrossRef CAS PubMed.
- F.-X. Theillet,
et al., Physicochemical Properties of Cells and Their Effects on Intrinsically Disordered Proteins (IDPs), Chem. Rev., 2014, 114, 6661–6714 CrossRef CAS PubMed.
- S. A. Hassan and P. J. Steinbach, Water-exclusion and liquid-structure forces in implicit solvation, J. Phys. Chem. B, 2011, 115, 14668 CrossRef CAS PubMed.
- S. A. Hassan, Self-consistent treatment of the local dielectric permittivity and electrostatic potential in solution for polarizable macromolecular force fields, J. Chem. Phys., 2012, 137, 074102 CrossRef PubMed.
- X.-H. N. Xu and E. S. Yeung, Long-range Electrostatic Trapping of Single-Protein Molecules at a Liquid-Solid Interface, Science, 1998, 281, 1650–1653 CrossRef CAS PubMed.
- M. Jarvis,
et al., Detachment of ligands from nanoparticle surface under flow and endothelial cell contact: Assessment using microfluidic devices, Bioeng. Transl. Med., 2018, 3, 148 CrossRef CAS PubMed.
- C. Rodriguez-Quijada, M. Sánchez-Purrá, H. de Puig and K. Hamad-Schifferli, Physical properties of biomolecules at the nanomaterial interface, J. Phys. Chem. B, 2018, 122, 2827–2840 CrossRef CAS PubMed.
- A. E. Nel,
et al., Understanding biophysicochemical interactions at the nano–bio interface, Nat. Mater., 2009, 8, 543–557 CrossRef CAS PubMed.
- L. A. Lane, X. Qian, A. M. Smith and S. Nie, Physical chemistry of nanomedicine: understanding the complex behaviors of nanoparticles in vivo, Annu. Rev. Phys. Chem., 2015, 66, 521–547 CrossRef CAS PubMed.
- Z. Zeng,
et al., Synthetic Polymer Nanoparticle–Polysaccharide Interactions: A Systematic Study, J. Am. Chem. Soc., 2012, 134, 2681–2690 CrossRef CAS PubMed.
- P. D. Ross and S. Subramanian, Thermodynamics of protein association reactions: forces contributing to stability, Biochemistry, 1981, 20, 3096–3102 CrossRef CAS PubMed.
- M. De, C.-C. You, S. Srivastava and V. M. Rotello, Biomimetic interactions of proteins with functionalized nanoparticles: a thermodynamic study, J. Am. Chem. Soc., 2007, 129, 10747–10753 CrossRef CAS PubMed.
- M.-M. Yin,
et al., A model beyond protein corona: thermodynamics and binding stoichiometries of the interactions between ultrasmall gold nanoclusters and proteins, Nanoscale, 2020, 12, 4573–4585 RSC.
- M.-M. Yin,
et al., Thermodynamics and mechanisms of the interactions between ultrasmall fluorescent gold nanoclusters and human serum albumin, γ-globulins, and transferrin: a spectroscopic approach, Langmuir, 2017, 33, 5108–5116 CrossRef CAS PubMed.
-
A. A. Sousa, in Reviews in Fluorescence, ed. C. D. Geddes and J. R. Lacowicz, Springer, 2018, pp. 53–73 Search PubMed.
- H. Yang,
et al., Conformational-transited protein corona regulated cell-membrane penetration and induced cytotoxicity of ultrasmall Au nanoparticles, RSC Adv., 2019, 9, 4435–4444 RSC.
- L. Shang,
et al., Effect of protein adsorption on the fluorescence of ultrasmall gold nanoclusters, Small, 2012, 8, 661–665 CrossRef CAS PubMed.
- L. Li, Q. Mu, B. Zhang and B. Yan, Analytical strategies for detecting nanoparticle–protein interactions, Analyst, 2010, 135, 1519–1530 RSC.
- L. Böhmert,
et al., Isolation methods for particle protein corona complexes from protein-rich matrices, Nanoscale Adv., 2020, 2, 563–582 RSC.
-
M. P. Monopoli, A. S. Pitek, I. Lynch and K. A. Dawson, in Nanomaterial interfaces in biology, ed. P. Bergese and K. Hamad-Schifferli, Springer, 2013, pp. 137–155 Search PubMed.
- J. Shang and X. Gao, Nanoparticle counting: towards accurate determination of the molar concentration, Chem. Soc. Rev., 2014, 43, 7267–7278 RSC.
- E. Colangelo,
et al., Characterizing self-assembled monolayers on gold nanoparticles, Bioconjugate Chem., 2017, 28, 11–22 CrossRef CAS PubMed.
- Q. Ong, Z. Luo and F. Stellacci, Characterization of ligand shell for mixed-ligand coated gold nanoparticles, Acc. Chem. Res., 2017, 50, 1911–1919 CrossRef CAS PubMed.
- Y. Niihori,
et al., High-performance liquid chromatography mass spectrometry of gold and alloy clusters protected by hydrophilic thiolates, Nanoscale, 2018, 10, 1641–1649 RSC.
- D. Li,
et al., Purification and separation of ultra-small metal nanoclusters, Adv. Colloid Interface Sci., 2020, 276, 102090 CrossRef CAS PubMed.
- J. Liu, S. P. Yadav, J. Andya, B. Demeule and S. J. Shire, Analytical Ultracentrifugation and Its Role in Development and Research of Therapeutical Proteins, Methods Enzymol., 2015, 562, 441–476 CAS.
-
J. F. Carpenter, D. L. Bain and G. R. Johnson, in Analytical Ultracentrifugation, ed. S. Uchiyama, F. Arisaka, W. Stafford and T. Laue, Springer, 2016 Search PubMed.
- Y. Lu and W. Chen, Application of mass spectrometry in the synthesis and characterization of metal nanoclusters, Anal. Chem., 2015, 87, 10659–10667 CrossRef CAS PubMed.
- T. Ruks,
et al., Solution NMR Spectroscopy with Isotope-Labeled Cysteine (13C and 15N) Reveals the Surface Structure of l-Cysteine-Coated Ultrasmall Gold Nanoparticles (1.8 nm), Langmuir, 2018, 35, 767–778 CrossRef PubMed.
- B. Schuetze,
et al., Conjugation of thiol-terminated molecules to ultrasmall 2 nm-gold nanoparticles leads to remarkably complex 1 H-NMR spectra, J. Mater. Chem. B, 2016, 4, 2179–2189 RSC.
- S. Mourdikoudis, R. M. Pallares and N. T. Thanh, Characterization techniques for nanoparticles: comparison and complementarity upon studying nanoparticle properties, Nanoscale, 2018, 10, 12871–12934 RSC.
- Y. Randika Perera, R. A. Hill and N. C. Fitzkee, Protein Interactions with Nanoparticle Surfaces: Highlighting Solution NMR Techniques, Isr. J. Chem., 2019, 59, 962–979 CrossRef CAS PubMed.
- A. Wang, T. Vo, V. Le and N. C. Fitzkee, Using hydrogen–deuterium exchange to monitor protein structure in the presence of gold nanoparticles, J. Phys. Chem. B, 2014, 118, 14148–14156 CrossRef CAS PubMed.
- W. Lin,
et al., Control of protein orientation on gold nanoparticles, J. Phys. Chem. C, 2015, 119, 21035–21043 CrossRef CAS PubMed.
- M. Assfalg,
et al., The study of transient protein–nanoparticle interactions by solution NMR spectroscopy, Biochim. Biophys. Acta, Proteins Proteomics, 2016, 1864, 102–114 CrossRef CAS PubMed.
- S. Zanzoni, M. Pedroni, M. D'Onofrio, A. Speghini and M. Assfalg, Paramagnetic nanoparticles leave their mark on nuclear spins of transiently adsorbed proteins, J. Am. Chem. Soc., 2016, 138, 72–75 CrossRef CAS PubMed.
- A. Bortot, S. Zanzoni, M. D'Onofrio and M. Assfalg, Specific Interaction Sites Determine Differential Adsorption of Protein Structural Isomers on Nanoparticle Surfaces, Chem.–Eur. J., 2018, 24, 5911–5919 CrossRef CAS PubMed.
- L. Calzolai, F. Franchini, D. Gilliland and F. Rossi, Protein− nanoparticle interaction: identification of the ubiquitin− gold nanoparticle interaction site, Nano Lett., 2010, 10, 3101–3105 CrossRef CAS PubMed.
- K. Srinivasan, S. Parimal, M. M. Lopez, S. A. McCallum and S. M. Cramer, Investigation into the molecular and thermodynamic basis of protein interactions in multimodal chromatography using functionalized nanoparticles, Langmuir, 2014, 30, 13205–13216 CrossRef CAS PubMed.
- S. Shrivastava, J. H. Nuffer, R. W. Siegel and J. S. Dordick, Position-specific chemical modification and quantitative proteomics disclose protein orientation adsorbed on silica nanoparticles, Nano Lett., 2012, 12, 1583–1587 CrossRef CAS PubMed.
- J. A. Yang,
et al., Study of wild-type α-synuclein binding and orientation on gold nanoparticles, Langmuir, 2013, 29, 4603–4615 CrossRef CAS PubMed.
- E. J. Tollefson,
et al., Preferential binding of cytochrome c to anionic ligand-coated gold nanoparticles: A complementary computational and experimental approach, ACS Nano, 2019, 13, 6856–6866 CrossRef CAS PubMed.
- Y. Duan, Y. Liu, R. Coreas and W. Zhong, Mapping molecular structure of protein locating on nanoparticles with limited proteolysis, Anal. Chem., 2019, 91, 4204–4212 CrossRef CAS PubMed.
- M. Lundqvist,
et al., Proteolytic cleavage reveals interaction patterns between silica nanoparticles and two variants of human carbonic anhydrase, Langmuir, 2005, 21, 11903–11906 CrossRef CAS PubMed.
- L. Whitmore and B. A. Wallace, Protein secondary structure analyses from circular dichroism spectroscopy: methods and reference databases, Biopolymers, 2008, 89, 392–400 CrossRef CAS PubMed.
- J. Kong and S. Yu, Fourier transform infrared spectroscopic analysis of protein secondary structures, Acta Biochim. Biophys. Sin., 2007, 39, 549–559 CrossRef CAS PubMed.
- M. Wang,
et al., Probing the mechanism of plasma protein adsorption on Au and Ag nanoparticles with FT-IR spectroscopy, Nanoscale, 2015, 7, 15191–15196 RSC.
- A. S. Klymchenko, Solvatochromic and fluorogenic dyes as environment-sensitive probes: design and biological applications, Acc. Chem. Res., 2017, 50, 366–375 CrossRef CAS PubMed.
- C. A. Royer, Probing protein folding and conformational transitions with fluorescence, Chem. Rev., 2006, 106, 1769–1784 CrossRef CAS PubMed.
- M. Lundqvist, I. Sethson and B.-H. Jonsson, High-resolution 2D 1H− 15N NMR characterization of persistent structural alterations of proteins induced by interactions with silica nanoparticles, Langmuir, 2005, 21, 5974–5979 CrossRef CAS PubMed.
- S. Shrivastava,
et al., Identifying specific protein residues that guide surface interactions and orientation on silica nanoparticles, Langmuir, 2013, 29, 10841–10849 CrossRef CAS PubMed.
-
J. R. Lakowicz, Principles of Fluorescence Spectroscopy, Springer, 3rd edn, 2006 Search PubMed.
- A. S. Minazzo, R. C. Darlington and J. A. Ross, Loop dynamics of the extracellular domain of human tissue factor and activation of factor VIIa, Biophys. J., 2009, 96, 681–692 CrossRef CAS PubMed.
- L. E. Kay, NMR studies of protein structure and dynamics, J. Magn. Reson., 2011, 213, 477–491 CrossRef CAS PubMed.
- S. R. Marcsisin and J. R. Engen, Hydrogen exchange mass spectrometry: what is it and what can it tell us?, Anal. Bioanal. Chem., 2010, 397, 967–972 CrossRef CAS PubMed.
-
H. Bisswanger, Enzyme kinetics: principles and methods, John Wiley & Sons, 2017 Search PubMed.
- J. Hühn,
et al., Dissociation coefficients of protein adsorption to nanoparticles as quantitative metrics for description of the protein corona: A comparison of experimental techniques and methodological relevance, Int. J. Biochem. Cell Biol., 2016, 75, 148–161 CrossRef PubMed.
- A. A. Sousa, A note on the use of steady–state fluorescence quenching to quantify nanoparticle–protein interactions, J. Fluoresc., 2015, 25, 1567–1575 CrossRef CAS PubMed.
- S. P. Boulos,
et al., Nanoparticle–protein interactions: a thermodynamic and kinetic study of the adsorption of bovine serum albumin to gold nanoparticle surfaces, Langmuir, 2013, 29, 14984–14996 CrossRef CAS PubMed.
- N. Li, S. Zeng, L. He and W. Zhong, Probing nanoparticle− protein interaction by capillary electrophoresis, Anal. Chem., 2010, 82, 7460–7466 CrossRef CAS PubMed.
- R. Huang and B. L. Lau, Biomolecule–nanoparticle interactions: Elucidation of the thermodynamics by isothermal titration calorimetry, Biochim. Biophys. Acta, Gen. Subj., 2016, 1860, 945–956 CrossRef CAS PubMed.
- A. Bekdemir and F. Stellacci, A centrifugation-based physicochemical characterization method for the interaction between proteins and nanoparticles, Nat. Commun., 2016, 7, 1–8 Search PubMed.
- W. Bujalowski and M. J. Jezewska, Quantitative thermodynamic analyses of spectroscopic titration curves, J. Mol. Struct., 2014, 1077, 40–50 CrossRef CAS PubMed.
- W. Bujalowski and T. M. Lohman, A general method of analysis of ligand-macromolecule equilibria using a spectroscopic signal from the ligand to monitor binding. Application to Escherichia coli single-strand binding protein-nucleic acid interactions, Biochemistry, 1987, 26, 3099–3106 CrossRef CAS PubMed.
- M. Swierczewska, S. Lee and X. Chen, The design and application of fluorophore–gold nanoparticle activatable probes, Phys. Chem. Chem. Phys., 2011, 13, 9929–9941 RSC.
- G. Schwarz, A universal thermodynamic approach to analyze biomolecular binding experiments, Biophys. Chem., 2000, 86, 119–129 CrossRef CAS.
- W. Bujalowski, M. J. Jezewska and P. J. Bujalowski, Signal and binding. I. Physico-chemical response to macromolecule–ligand interactions, Biophys. Chem., 2017, 222, 7–24 CrossRef CAS PubMed.
- W. Bujalowski, M. J. Jezewska and P. J. Bujalowski, Signal and binding. II. Converting physico-chemical responses to macromolecule–ligand interactions into thermodynamic binding isotherms, Biophys. Chem., 2017, 222, 25–40 CrossRef CAS PubMed.
-
W. Maechtle and B. Lars, Analytical Ultracentrifugation of Polymers and Nanoparticles, Springer, 2006 Search PubMed.
- A. Balbo,
et al., Studying multiprotein complexes by multisignal sedimentation velocity analytical ultracentrifugation, Proc. Natl. Acad. Sci. U. S. A., 2005, 102, 81–86 CrossRef CAS PubMed.
-
P. Schuck and H. Zhao, Sedimentation Velocity Analytical Ultracentrifugation. Interacting Systems, CRC Press, 2017 Search PubMed.
- P. Schuck, Sedimentation Patterns of Rapidly Reversible Protein Interactions, Biophys. J., 2010, 98, 2005–2013 CrossRef CAS PubMed.
- R. Huang and B. L. Lau, Biomolecule–nanoparticle interactions: Elucidation of the thermodynamics by isothermal titration calorimetry, Biochim. Biophys. Acta, Gen. Subj., 2016, 1860, 945–956 CrossRef CAS PubMed.
- E. Omanovic-Miklicanin, I. Manfield and T. Wilkins, Application of isothermal titration calorimetry in evaluation of protein–nanoparticle interactions, J. Therm. Anal. Calorim., 2017, 127, 605–613 CrossRef CAS.
- D. Prozeller, S. Morsbach and K. Landfester, Isothermal titration calorimetry as a complementary method for investigating nanoparticle–protein interactions, Nanoscale, 2019, 11, 19265–19273 RSC.
- X. Xu,
et al., Counterion-release entropy governs the inhibition of serum proteins by polyelectrolyte drugs, Biomacromolecules, 2018, 19, 409–416 CrossRef CAS PubMed.
-
C. R. Bagshaw, Biomolecular Kinetics: a step-by-step guide, CRC Press, 2017 Search PubMed.
- K. A. Johnson, Transient-state kinetic analysis of enzyme reaction pathways, Enzymes, 1992, 20, 61 Search PubMed.
- M. F. Engel, C. P. van Mierlo and A. J. Visser, Kinetic and structural characterization of adsorption-induced unfolding of bovine α-lactalbumin, J. Biol. Chem., 2002, 277, 10922–10930 CrossRef CAS PubMed.
- H. Pan, M. Qin, W. Meng, Y. Cao and W. Wang, How do proteins unfold upon adsorption on nanoparticle surfaces?, Langmuir, 2012, 28, 12779–12787 CrossRef CAS PubMed.
- A. Patra,
et al., Component-specific analysis of plasma protein corona formation on gold nanoparticles using multiplexed surface plasmon resonance, Small, 2016, 12, 1174–1182 CrossRef CAS PubMed.
- H. Shibata,
et al., Interaction kinetics of serum proteins with liposomes and their effect on phospholipase-induced liposomal drug release, Int. J. Pharm., 2015, 495, 827–839 CrossRef CAS PubMed.
- Y. Hoshino, M. Nakamoto and Y. Miura, Control of protein-binding kinetics on synthetic polymer nanoparticles by tuning flexibility and inducing conformation changes of polymer chains, J. Am. Chem. Soc., 2012, 134, 15209–15212 CrossRef CAS PubMed.
- H. Koide,
et al., Engineering the binding kinetics of synthetic polymer nanoparticles for siRNA delivery, Biomacromolecules, 2019, 20, 3648–3657 CrossRef CAS PubMed.
- C. Tassa,
et al., Binding affinity and kinetic analysis of targeted small molecule-modified nanoparticles, Bioconjugate Chem., 2010, 21, 14–19 CrossRef CAS PubMed.
-
P. Schuck and H. Zhao, in Surface plasmon resonance, Springer, ed. N. J. Mol and M. J. E. Fischer, 2010, pp. 15–54. Search PubMed.
- J. Svitel, H. Boukari, D. Van Ryk, R. C. Willson and P. Schuck, Probing the functional heterogeneity of surface binding sites by analysis of experimental binding traces and the effect of mass transport limitation, Biophys. J., 2007, 92, 1742–1758 CrossRef CAS PubMed.
- A. Åkesson, M. Cárdenas, G. Elia, M. P. Monopoli and K. A. Dawson, The protein corona of dendrimers: PAMAM binds and activates complement proteins in human plasma in a generation dependent manner, RSC Adv., 2012, 2, 11245–11248 RSC.
- M. Zarei and J. Aalaie, Profiling of nanoparticle–protein interactions by electrophoresis techniques, Anal. Bioanal. Chem., 2019, 411, 79–96 CrossRef CAS PubMed.
- M. G. Fried and J. L. Bromberg, Factors that affect the stability of protein-DNA complexes during gel electrophoresis, Electrophoresis, 1997, 18, 6–11 CrossRef CAS PubMed.
- S. K. Chaturvedi, V. Sagar, H. Zhao, G. Wistow and P. Schuck, Measuring Ultra-Weak Protein Self-Association by Non-ideal Sedimentation Velocity, J. Am. Chem. Soc., 2019, 141, 2990–2996 CrossRef CAS PubMed.
- T. G. Schmidt and A. Skerra, The Strep-tag system for one-step purification and high-affinity detection or capturing of proteins, Nat. Protoc., 2007, 2, 1528 CrossRef CAS PubMed.
-
D. Frenkel and B. Smit, Understanding Molecular Simulations. From algorithms to applications, Academic Press, 2nd edn, 2001..
- K. Karplus, Molecular Dynamics Simulations of Biomolecules, Acc. Chem. Res., 2002, 35, 321 CrossRef PubMed.
-
M. P. Allen and D. J. Tildesley, Computer Simulation of Liquids, Clarendon Press, 1987 Search PubMed.
- W. F. van Gusteren and H. J. C. A. Berendsen, Leap-frog Algorithm for Stochastic Dynamics, Mol. Simulat., 1988, 1, 173–185 CrossRef.
-
B. D. Todd and P. J. Daivis, Nonequilibrium Molecular Dynamics: Theory, algorithms, and applications, Cambridge University Press, 2017 Search PubMed.
- Y. I. Yang, Q. Shao, J. Zhang, L. Yang and Y. Q. Gao, Enhanced sampling in molecular dynamics, J. Chem. Phys., 2019, 151, 070902 CrossRef PubMed.
- E. Brunk and U. Rothlisberger, Mixed quantum mechanical/molecular mechanical molecular dynamics simulations of biological systems in ground and electronically excited states, Chem. Rev., 2015, 115, 6217–6263 CrossRef CAS PubMed.
- U. N. Morzan,
et al., Spectroscopy in Complex Environments from QM–MM Simulations, Chem. Rev., 2018, 118, 4071–4113 CrossRef CAS PubMed.
- B. R. Brooks,
et al., CHARMM: The Biomolecular Simulation Program, J. Comput. Chem., 2009, 30, 1545 CrossRef CAS.
- K. P. Fears,
et al. High-performance nanomaterials formed by rigid yet extensible cyclic β-peptide polymers, Nat. Commun., 2018, 9, 4090 CrossRef PubMed.
- J. Huang,
et al., CHARMM36m: An Improved Force Field for Folded and Intrinsically Disordered Proteins, Nat. Methods, 2017, 14, 71–73 CrossRef CAS PubMed.
- S. Dasetty, P. J. Meza-Morales, R. B. Getman and S. Sarupria, Simulations of interfacial processes: recent advances in force field development, Curr. Opin. Chem. Eng., 2019, 23, 138–145 CrossRef.
- M. Ozboyaci, D. B. Kokh, S. Corni and R. C. Wade, Modeling and simulation of protein–surface interactions: achievements and challenges, Q. Rev. Biophys., 2016, 49, e4 CrossRef PubMed.
- O. D. Villareal, R. O. Rodriguez, L. Yu and T. O. Wambo, Molecular dynamics simulations on the effect of size and shape on the interactions between negative Au18(SR)14, Au102(SR)44 and Au144(SR)60 nanoparticles in physiological saline, Colloids Surf., A, 2016, 503, 70–78 CrossRef PubMed.
- S. A. Hassan, Amino acid side chain interactions in the presence of salts, J. Phys. Chem. B, 2005, 109, 21989–21996 CrossRef CAS PubMed.
- J. Koivisto,
et al., Acid-base properties and surface charge distribution of the water-soluble Au102(pMBA)44 nanocluster, J. Phys. Chem. C, 2016, 120, 10041–10050 CrossRef CAS.
- A. M. Baptista, V. H. Teixeira and C. M. Soares, Constant-pH molecular dynamics using stochastic titration, J. Chem. Phys., 2002, 117, 4184–4200 CrossRef CAS.
- B. R. Brooks,
et al., CHARMM: A Program for Macromolecular Energy, Minimization and Dynamics Calculations, J. Comput. Chem., 1983, 4, 187–217 CrossRef CAS.
- A. Liwo,
et al., A united-residue force field for off-lattice protein-structure simulations. I. Functional forms and parameters of long-range side-chain interaction potentials from protein crystal data, J. Comput. Chem., 1997, 97, 849–873 CrossRef.
- A. Liwo,
et al., A united-residue force field for off-lattice protein-structure simulations. II. Parameterization of short-range interactions and determination of weights of energy terms by Z-score optimization, J. Comput. Chem., 1997, 18, 874–887 CrossRef CAS.
- A. Liwo and C. Czaplewski, Extension of the force-matching method to coarse-grained models with axially symmetric sites to produce transferable force fields: Application to the UNRES model of proteins, J. Chem. Phys., 2020, 152, 054902 CrossRef CAS PubMed.
- S. J. Marrink and D. P. Tieleman, Perspective on the Martini model, Chem. Soc. Rev., 2013, 42, 6801–6822 RSC.
- V. Tozzini, Coarse-grained models for proteins, Curr. Opin. Struct. Biol., 2005, 15, 144–150 CrossRef CAS PubMed.
- G. Brancolini and V. Tozzini, Multiscale modeling of protein interactions with functionalized nanoparticles, Curr. Opin. Colloid Interface Sci., 2019, 41, 66–73 CrossRef CAS.
- I. R. Cooke and M. Deserno, Solvent-free model for self-assembling fluid bilayer membranes: Stabilization of the fluid phase based on broad attractive tail potentials, J. Chem. Phys., 2005, 123, 224710 CrossRef PubMed.
- G. Rossi and L. Monticelli, Gold nanoparticles in model biological membranes: a computational perspective, Biochim. Biophys. Acta, 2016, 1858, 2380–2389 CrossRef CAS PubMed.
- R. C. Van Lehn,
et al., Lipid tail protrusions mediate the insertion of nanoparticles into model cell membranes, Nat. Commun., 2014, 5, 4482 CrossRef CAS PubMed.
- H. M. Ding and Y. Q. Ma, Computational investigations of nanoparticle-biomembrane interactions in cellular delivery, Small, 2015, 11, 1055–1071 CrossRef CAS PubMed.
- F. Tavanti, A. Pedone and M. C. Menziani, Multiscale molecular dynamics simulation of multiple protein adsorption on gold nanoparticles, Int. J. Mol. Sci., 2019, 20, 3539 CrossRef CAS PubMed.
- A. A. Bhirde, S. A. Hassan, E. Harr and X. Chen, Role of albumin in the formation and stabilization of nanoparticle aggregates in serum studied by continuous photon correlation spectroscopy and multiscale computer simulations, J. Phys. Chem. C, 2014, 118, 16199–16208 CrossRef CAS PubMed.
- F. Tavanti, A. Pedone and C. Menziani, Competitive Binding of Proteins to Gold Nanoparticles Disclosed by Molecular Dynamics Simulations, J. Phys. Chem. C, 2015, 119, 22172–22180 CrossRef CAS.
- R. Vacha, F. J. Martinez-Veracoechea and D. Frenkel, Receptor-mediated endocytosis of nanoparticles of various shapes, Nano Lett., 2011, 11, 5391–5395 CrossRef CAS PubMed.
- S. Angioletti-Uberti, Theory, simulations and the design of functionalized nanoparticles for biomedical applications: a soft matter perspective, npj Comput. Mater., 2017, 3, 48 CrossRef.
- M. Praprotnik, L. Delle Site and K. Kremer, Multiscale simulation of soft matter: from scale bridging to adaptive resolution, Annu. Rev. Phys. Chem., 2008, 59, 545–571 CrossRef CAS PubMed.
- S. A. Hassan, Self-Adaptive Multiscaling Algorithm for Efficient Simulations of Many-Protein Systems in Crowded Conditions, Phys. Chem. Chem. Phys., 2018, 20, 28544 RSC.
- L. A. Lane, Physics in nanomedicine: phenomena giverning the in vivo performance of nanoparticles, Appl. Phys. Rev., 2020, 7, 0113116 Search PubMed.
- S. A. Hassan, Computer Simulation of Ion Cluster Speciation in Concentrated Aqueous Solutions at Ambient Conditions, J. Phys. Chem. B, 2008, 112, 10573 CrossRef CAS PubMed.
- X. Shi, A. von dem Bussche, R. H. Hurt, A. B. Kane and H. Gao, Cell entry of one-dimensional nanomaterials occurs by tip recognition and rotation, Nat. Nanotechnol., 2011, 6, 714–719 CrossRef CAS PubMed.
- A.-A. A. Boulogeorgos, S. E. trevlakis, S. A. Tegos, V. K. Papanikolaou and G. K. Karagiannidis, Machine learning in nano-scale biomedical engineering, IEEE Trans. Mol. Biol. and Multi-Scale Commun., 2021, 7, 10–30 Search PubMed.
-
J. Watt, R. Borhani and A. K. Katsaggelos, Machine Learning Refined, Cambridge University Press, 2017 Search PubMed.
-
C. M. Bishop, Neural Networks for Pattern Recognition, Clarendon Press, 1995 Search PubMed.
- D. Liu, Y. Tan, E. Khoram and Z. Yu, Training Deep Neural Networks for the Inverse Design of Nanophotonic Structures, ACS Photonics, 2018, 5, 1365 CrossRef CAS.
- Y. Chen, J. Zhu, Y. Xie, N. Feng and Q. H. Liu, Smart inverse design of graphene-based photonic metamaterials by an adaptive artificial neural network, Nanoscale, 2019, 11, 9749 RSC.
- Z. Liu, D. Zhu, S. P. Rodrigues, K.-T. Lee and W. Cai, Generative Model for the Inverse Design of Metasurfaces, Nano Lett., 2018, 18, 6570–6576 CrossRef CAS PubMed.
- C. D. Fjell, J. A. Hiss, R. E. W. Hancock and G. Schneider, Designing antimicrobial peptides: form follows function, Nat. Rev., 2012, 11, 37–51 CAS.
- N. Raheem and S. K. Straus, Mechanisms of action for antimicrobial peptides with antibacterial and antibiofilm functions, Front. Microbiol., 2019, 10, 1–14 CrossRef PubMed.
- B. Vishnepolsky,
et al., Predictive model of linear antimicrobial peptides active against Gram-negative bacteria, J. Chem. Theory Comput., 2018, 58, 1141–1151 CAS.
- B. Vishnepolsky,
et al., De novo and in vitro testing of antimicrobial peptides against Gram-negative bacteria, Pharmaceuticals, 2019, 12, 1–17 CrossRef PubMed.
- D. Bobo, K. J. Robinson, J. Islam, K. J. Thurecht and S. R. Corrie, A review of FDA-approved materials and clinical trials to date, Pharm. Res., 2016, 33, 2373–2387 CrossRef CAS PubMed.
- D. Su, L. Gao, F. Gao, X. Zhang and X. Gao, Peptide and protein modified metal clusters for cancer diagnostics, Chem. Sci., 2020, 11, 5614–5629 RSC.
- Y. Su,
et al., Luminescent metal nanoclusters for biomedical applications, Nano Res., 2019, 12, 1251–1265 CrossRef CAS.
- E. Porret, X. Le Guével and J.-L. Coll, Gold nanoclusters for biomedical applications: toward in vivo studies, J. Mater. Chem. B, 2020, 8, 2216–2232 RSC.
- Y. G. Srinivasulu, Q. Yao, N. Goswami and J. Xie, Interfacial engineering of gold nanoclusters for biomedical applications, Mater. Horiz., 2020, 7, 2596–2618 RSC.
- T. Ruks,
et al., Targeting the surface of the protein 14-3-3 by ultrasmall gold nanoparticles (1.5 nm), carrying the specific peptide CRaf, ChemBioChem, 2020, 22, 1456–1463 CrossRef PubMed.
- C.-C. You, S. S. Agasti, M. De, M. J. Knapp and V. M. Rotello, Modulation of the catalytic behavior of α-chymotrypsin at monolayer-protected nanoparticle surfaces, J. Am. Chem. Soc., 2006, 128, 14612–14618 CrossRef CAS PubMed.
- M. Merdanovic, T. Mönig, M. Ehrmann and M. Kaiser, Diversity of allosteric regulation in proteases, ACS Chem. Biol., 2013, 8, 19–26 CrossRef CAS PubMed.
- S. Verespy III, A. Y. Mehta, D. Afosah, R. A. Al-Horani and U. R. Desai, Allosteric partial inhibition of monomeric proteases. Sulfated coumarins induce regulation, not just inhibition, of thrombin, Sci. Rep., 2016, 6, 24043 CrossRef PubMed.
- W. R. Algar, T. Jeen, M. Massey, W. J. Peveler and J. Asselin, r. m. Small surface, big effects, and big challenges: Toward understanding enzymatic activity at the inorganic nanoparticle–substrate interface, Langmuir, 2018, 35, 7067–7091 CrossRef PubMed.
- J. N. Vranish, M. G. Ancona, S. A. Walper and I. L. Medintz, Pursuing the promise of enzymatic enhancement with nanoparticle assemblies, Langmuir, 2017, 34, 2901–2925 CrossRef PubMed.
- Q. Wen, Y. Gu, L.-J. Tang, R.-Q. Yu and J.-H. Jiang, Peptide-templated gold nanocluster beacon as a sensitive, label-free sensor for protein post-translational modification enzymes, Anal. Chem., 2013, 85, 11681–11685 CrossRef CAS PubMed.
- K. L. Diehl and E. V. Anslyn, Array sensing using optical methods for detection of chemical and biological hazards, Chem. Soc. Rev., 2013, 42, 8596–8611 RSC.
- Y. Geng, W. J. Peveler and V. M. Rotello, Array-based “Chemical Nose” Sensing in Diagnostics and Drug Discovery, Angew. Chem., Int. Ed., 2019, 58, 5190–5200 CrossRef CAS PubMed.
- W. J. Peveler, M. Yazdani and V. M. Rotello, Selectivity and specificity: pros and cons in sensing, ACS Sens., 2016, 1, 1282–1285 CrossRef CAS PubMed.
- L. Guerrini, E. Garcia-Rico, N. Pazos-Perez and R. A. Alvarez-Puebla, Smelling, Seeing, Tasting - Old Senses for New Sensing, ACS Nano, 2017, 11, 5217–5222 CrossRef CAS PubMed.
- Z. Yuan,
et al., Fluorescent gold nanodots based sensor array for proteins discrimination, Anal. Chem., 2015, 87, 4253–4259 CrossRef CAS PubMed.
- A. Bajaj,
et al., Detection and differentiation of normal, cancerous, and metastatic cells using nanoparticle-polymer sensor arrays, Proc. Natl. Acad. Sci. U. S. A., 2009, 106, 10912–10916 CrossRef CAS PubMed.
- S. Rana,
et al., A multichannel nanosensor for instantaneous readout of cancer drug mechanisms, Nat. Nanotechnol., 2015, 10, 65 CrossRef CAS PubMed.
- Y. Tao, M. Li and D. T. Auguste, Pattern-based sensing of triple negative breast cancer cells with dual-ligand cofunctionalized gold nanoclusters, Biomaterials, 2017, 116, 21–33 CrossRef CAS PubMed.
- M. Tang, J. Zhang, C. Yang, Y. Zheng and H. Jiang, Gold Nanoclusters for Bacterial Detection and Infection Therapy, Front. Chem., 2020, 8, 181 CrossRef CAS PubMed.
- K. Zheng, M. I. Setyawati, D. T. Leong and J. Xie, Antimicrobial gold nanoclusters, ACS Nano, 2017, 11, 6904–6910 CrossRef CAS PubMed.
- K. Zheng, M. I. Setyawati, D. T. Leong and J. Xie, Surface ligand chemistry of gold nanoclusters determines their antimicrobial ability, Chem. Mater., 2018, 30, 2800–2808 CrossRef CAS.
- X. Li,
et al., Functional gold nanoparticles as potent antimicrobial agents against multi-drug-resistant bacteria, ACS Nano, 2014, 8, 10682–10686 CrossRef CAS PubMed.
- R. Mukherji,
et al., Selective imaging of quorum sensing receptors in bacteria using fluorescent Au nanocluster probes surface functionalized with signal molecules, ACS Appl. Mater. Interfaces, 2013, 5, 13076–13081 CrossRef CAS PubMed.
- X. Zhang,
et al., Specific detection and effective inhibition of a single bacterial species in situ using peptide mineralized Au cluster probes, Sci. China: Chem., 2018, 61, 627–634 CrossRef CAS.
- P.-H. Chan,
et al., Photoluminescent gold nanoclusters as sensing probes for uropathogenic Escherichia coli, PLoS One, 2013, 8, e58064 CrossRef CAS PubMed.
- Y. Xie,
et al., Gold Nanoclusters for Targeting Methicillin-Resistant Staphylococcus aureus In Vivo, Angew. Chem., Int. Ed., 2018, 57, 3958–3962 CrossRef CAS PubMed.
- L.-Y. Chen, C.-W. Wang, Z. Yuan and H.-T. Chang, Fluorescent gold nanoclusters: recent advances in sensing and imaging, Anal. Chem., 2015, 87, 216–229 CrossRef CAS PubMed.
- R. Vankayala, C. L. Kuo, K. Nuthalapati, C. S. Chiang and K. C. Hwang, Nucleus-targeting gold nanoclusters for simultaneous in vivo fluorescence imaging, gene delivery, and NIR-light activated photodynamic therapy, Adv. Funct. Mater., 2015, 25, 5934–5945 CrossRef CAS.
- C. Ding and Y. Tian, Gold nanocluster-based fluorescence biosensor for targeted imaging in cancer cells and ratiometric determination of intracellular pH, Biosens. Bioelectron., 2015, 65, 183–190 CrossRef CAS PubMed.
- L. Shang, F. Stockmar, N. Azadfar and G. U. Nienhaus, Intracellular thermometry by using fluorescent gold nanoclusters, Angew. Chem., Int. Ed., 2013, 52, 11154–11157 CrossRef CAS PubMed.
- M. Yu, C. Zhou, J. Liu, J. D. Hankins and J. Zheng, Luminescent gold nanoparticles with pH-dependent membrane adsorption, J. Am. Chem. Soc., 2011, 133, 11014–11017 CrossRef CAS PubMed.
- Y. Wang,
et al., Bifunctional peptides that precisely biomineralize Au clusters and specifically stain cell nuclei, Chem. Commun., 2012, 48, 871–873 RSC.
- J. Zhai,
et al., Facile approach to observe and quantify the αIIbβ3 integrin on a single-cell, Anal. Chem., 2015, 87, 2546–2549 CrossRef CAS PubMed.
- X. Fang,
et al., Mitochondria-targeting Au nanoclusters enhance radiosensitivity of cancer cells, J. Mater. Chem. B, 2017, 5, 4190–4197 RSC.
- J. F. Hainfeld and R. D. Powell, New frontiers in gold labeling, J. Histochem. Cytochem., 2000, 48, 471–480 CrossRef CAS PubMed.
- A. Sousa,
et al., Reprint of “On the feasibility of visualizing ultrasmall gold labels in biological specimens by STEM tomography”, J. Struct. Biol., 2007, 159, 507–522 (
J. Struct. Biol.
, 2008
, 161
, 336–351
) CrossRef CAS PubMed.
- L. Gong, Y. Chen, K. He and J. Liu, Surface coverage-regulated cellular interaction of ultrasmall luminescent gold nanoparticles, ACS Nano, 2019, 13, 1893–1899 CrossRef CAS PubMed.
- K. Saha,
et al., Surface functionality of nanoparticles determines cellular uptake mechanisms in mammalian cells, Small, 2013, 9, 300–305 CrossRef CAS PubMed.
- L. Yang, L. Shang and G. U. Nienhaus, Mechanistic aspects of fluorescent gold nanocluster internalization by live HeLa cells, Nanoscale, 2013, 5, 1537–1543 RSC.
- F. Muraca, L. Boselli, V. Castagnola and K. A. Dawson, Ultrasmall Gold Nanoparticles Cellular Uptake: Influence of Transient Bionano Interactions, ACS Appl. Bio Mater., 2020, 3, 3800–3808 CrossRef CAS.
- J. Yang,
et al., Recent advances in ultra-small fluorescent Au nanoclusters toward oncological research, Nanoscale, 2019, 11, 17967–17980 RSC.
- C. Zhou, M. Long, Y. Qin, X. Sun and J. Zheng, Luminescent gold nanoparticles with efficient renal clearance, Angew. Chem., Int. Ed., 2011, 50, 3168–3172 CrossRef CAS PubMed.
- J. Zhai,
et al., Turning on/off the anti-tumor effect of the Au cluster via atomically controlling its molecular size, ACS Nano, 2018, 12, 4378–4386 CrossRef CAS PubMed.
- S. J. Hale,
et al., DM1 loaded ultrasmall gold nanoparticles display significant efficacy and improved tolerability in murine models of hepatocellular carcinoma, Bioconjugate Chem., 2018, 30, 703–713 CrossRef PubMed.
- J. F. Hainfeld, F. A. Dilmanian, D. N. Slatkin and H. M. Smilowitz, Radiotherapy enhancement with gold nanoparticles, J. Pharm. Pharmacol., 2008, 60, 977–985 CrossRef CAS PubMed.
- X. D. Zhang,
et al., Enhanced Tumor Accumulation of Sub-2 nm Gold Nanoclusters for Cancer Radiation Therapy, Adv. Healthcare Mater., 2014, 3, 133–141 CrossRef CAS PubMed.
- A. A. Sousa, Impact of soft protein interactions on the excretion, extent of receptor occupancy and tumor accumulation of ultrasmall metal nanoparticles: a compartmental model simulation, RSC Adv., 2019, 9, 26927–26941 RSC.
- X. Wang,
et al., Active tumor-targeting luminescent gold clusters with efficient urinary excretion, Chem. Commun., 2016, 52, 9232–9235 RSC.
Footnote |
† Electronic supplementary information (ESI) available. See DOI: 10.1039/d1na00086a |
|
This journal is © The Royal Society of Chemistry 2021 |