DOI:
10.1039/D2LC00814A
(Critical Review)
Lab Chip, 2023,
23, 1258-1278
Advances in droplet digital polymerase chain reaction on microfluidic chips
Received
31st August 2022
, Accepted 30th January 2023
First published on 8th February 2023
Abstract
The PCR technique has been known to the general public since the pandemic outbreak of COVID-19. This technique has progressed through three stages: from simple PCR to real-time fluorescence PCR to digital PCR. Among them, the microfluidic-based droplet digital PCR technique has attracted much attention and has been widely applied due to its advantages of high throughput, high sensitivity, low reagent consumption, low cross-contamination, and absolute quantification ability. In this review, we introduce various designs of microfluidic-based ddPCR developed within the last decade. The microfluidic-based droplet generation methods, thermal cycle strategies, and signal counting approaches are described, and the applications in the fields of single-cell analysis, disease diagnosis, and pathogen detection are introduced. Further, the challenges and prospects of microfluidic-based ddPCR are discussed. We hope that this review can contribute to the further development of the microfluidic-based ddPCR technique.
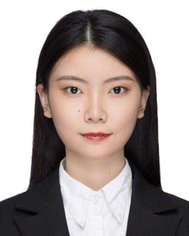 Danfeng Xu | Danfeng Xu received her BS degree from the School of Pharmacy, China Pharmaceutical University in 2020. She is currently a master degree candidate under the guidance of Professor Wei-Fei Zhang at the National Institute of Metrology, China. Her research interests are organs-on-chips and drug metabolism analysis with microfluidic technology. |
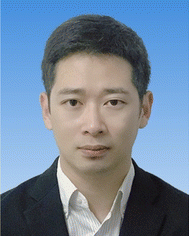 Weifei Zhang | Dr. Weifei Zhang received his PhD degree from Tokyo Metropolitan University in 2017. He was a postdoc at Tsinghua University from 2017–2019. Since 2019 he has been an Associate Professor at the National Institute of Metrology, China. His current research direction is pharmacochemistry metrology and also focuses on the development of microfluidic technology for PCR and drug metabolism analysis. |
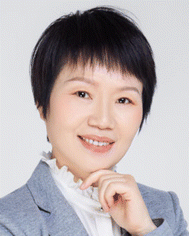 Hongmei Li | Professor Hongmei Li received her BS from Sichuan University in 1986. She is a professor of Chemical Metrology Division, National Institute of Metrology (NIM). She was selected as a committee member of International Committee of Weights and Measures/Consultative Committee on the Quantity of Material (CIPM/CCQM) and Committee on Reference Material (ISO/REMCO). She was also the chairperson of Asia Pacific Metrology Programme (APMP) Food Safety Focus Group (FSFG). Her current research is focused on clinical and pharmaceutical measurement technology standard research and engineering practice. |
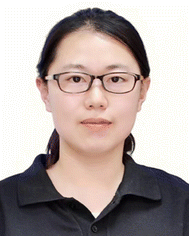 Nan Li | Dr. Nan Li received her PhD degree from Tsinghua University in 2020. She was a postdoctor at Tsinghua University during 2020–2022. Since 2022, she was an associate professor at Beijing University of Chinese Medicine. Her current research direction is pharmaceutical analysis, and she also focuses on the development of microfluidic technology for drug dection. |
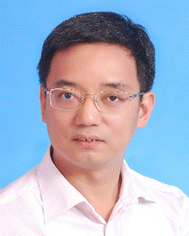 Jin-Ming Lin | Professor Jin-Ming Lin graduated from Fuzhou University in 1984 and received his PhD degree from Tokyo Metropolitan University in 1997. He studied and worked at Showa University and Tokyo Metropolitan University during 1992–2002. He was a full professor at the Research Center for Eco-Environmental Sciences, Chinese Academy of Sciences during 2002–2004, and has been a full professor of the Department of Chemistry, Tsinghua University since 2004. He was selected as a Cheung Kung Scholars Professor of Ministry of Education, China in 2008. He is a Fellow of the Royal Chemical Society, and a deputy director of the Analytical Chemistry Division of Chinese Chemical Society. His current research is focused on cell analysis, microfluidics with mass spectrometry, and chemiluminescence. |
1. Introduction
The COVID-19 pandemic outbreak at the end of 2019 is still disturbing people's lives. To facilitate disease diagnosis, isolate the infection source, and take swift action to stop the epidemic from spreading, early and rapid initial confirmation of COVID-19 is necessary. Polymerase chain reaction (PCR) has been crucial in these situations owing to the advantages of high detection sensitivity and capacity to amplify signals from samples with low amounts of genetic material via repeated cycles of denaturation, annealing, and extension.1–5
Since the high-temperature resistant polymerase (Taqase) was discovered6 and applied to PCR in 1988,7 PCR has progressed through three stages: from the first generation of simple PCR to the second generation of real-time fluorescence quantitative PCR (qPCR) to the third generation of digital PCR (dPCR), accompanied by the improvement of detection ability from qualitative to quantitative to absolute quantitative analysis.8–11 The first-generation PCR uses gel electrophoresis to reflect the amplification results. The first-generation PCR can realize low cost of instruments and reagents. Besides, the PCR products can be purified with gel analysis for molecular biology experiments. However, the cumbersome operation, moderate sensitivity, easy contamination, and narrow dynamic linear range result in failure to detect samples with low concentrations and complex backgrounds. Besides, the first generation is not able to be used for quantitative analysis, and sometimes the target band can be interfered by the non-specific band of the same size as the targets. Therefore, the second-generation PCR, qPCR, was developed in 1992 and has been widely applied.12,13 Fluorescent reporter groups are used for the real-time monitoring of the amplification signal, and the standard curve obtained from standards with a series of concentrations is used for the subsequent relative quantification analysis. However, there are some intrinsic limitations for qPCR, such as relying on a standard curve to quantify the results and potential false-positive interferences caused by contamination. To address these limitations, Bert Vogelstein and Kenneth W. Kinzler first proposed the idea of digital PCR in 1999,14–17 which allowed absolute quantification of nucleic acids based on the amount of positive and negative signals, with no need for an external calibration curve. The current digital PCR can be divided into two main sorts according to the generation of an individual microreactor. One is chamber-based digital PCR, (i.e., cdPCR) containing the slip-chip18–20 and the microarray chip with microwells,21,22 as well as the integrated fluid circuit (IFC) chip.23 Chamber-based chip PCR mainly uses the chip design to seal the nanoliter liquid in a high-throughput microwell or microchannel for subsequent PCR amplification and the amplified results can be interpreted using a fluorescence microscope directly. The other is droplet digital PCR based on water-in-oil droplet generation which is the focus of the review. Hindson et al. reported a microfluidic-based droplet ddPCR method for high throughput and absolute quantification in 2011, which was one of the theoretical foundations of the now-mature ddPCR.24 During the past decade, ddPCR techniques have been experiencing a fast development with advances in microfluidic droplet manipulation.25,26 Microscale-sized monodisperse aqueous droplets (1 pL–10 nL) can be quickly generated by introducing an immiscible oil phase in the microfluidic channel, enabling high sensitivity of the analysis. Besides, these droplets are physically and chemically isolated, with the advantages of low cross-contamination and ease of counting.27–29
With the advantages of absolute quantification, high throughputs, and high sensitivity, the ddPCR technique is considered to have a wide range of applications. In the field of life analysis, ddPCR can be used to detect pathogens including bacteria and viruses in biological samples,30–34 and can also be used for disease diagnosis35–37 and single cell analysis,38–41 including the test of circulating tumor cells (CTCs)42–44 and the analysis of mutated genes.45–47 Additionally, ddPCR is also popular in the environmental field33,48–51 and food field5,52,53 for the detection of harmful microorganisms. With the continual enlargement of the application range, a large number of commercial ddPCR apparatuses have been developed based on microfluidic techniques, which can automatically generate micro-droplets to wrap the reactants in independent space for amplification reactions before being automatically counted and exhibiting results. So far, however, although ddPCR has a great advantage over qPCR, it is far from being as universal as qPCR due to the expensive cost. Therefore, developing more mature ddPCR techniques with low cost and convenience is significantly important.
In this review, we introduce various designs of ddPCR on microfluidic chips developed within the last decade. First, the microfluidic-based droplet generation methods are described. Then, different thermal cycle strategies for the amplification process including space domain and time domain cycles are discussed, followed by the description of signal counting approaches. Next, some representative examples of applications are introduced (Fig. 1). Further, this review also discusses the challenges and prospects of microfluidic-based ddPCR, and provides important references for the development of techniques and applications.
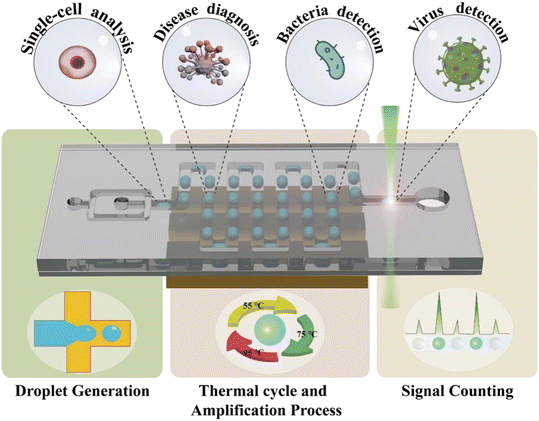 |
| Fig. 1 Schematic diagram of ddPCR and applications. | |
2. Droplet generation
Droplet generation is one of the essential steps in ddPCR, which is influenced by the properties of the liquid itself including viscosity and surface tension, as well as external factors such as temperature, flow rate, and the geometry of the channel. Generally, temperature changes affect the reaction kinetics, while the flow rate of both the dispersed phase and continuous phase, the properties of fluid and the geometry of the microfluidic channel affect the size and stability of droplet generation.54,55 Droplet generation methods can be divided into passive and active generation depending on the way of acting forces. The passive droplet generation method is mainly based on the fluid dynamics method, by constructing different shapes of geometric flow channels, so that the liquid can overcome surface tension and generate micro-droplets when the steady state of flow is disrupted by impact including shear force.56–58 It doesn't need extra input force but it relies on the hydrodynamic pressure to change the surface energy of the flow. The active droplet generation method uses external forces such as electricity, magnetism, light and heat to generate droplets.41,59,60 The external force applied in the active method has more precise control over droplet generation, and the droplet changes faster according to the external force. Moreover, some active methods can generate droplets on demand by an external force. Either way, the generated monodisperse droplets can be used as a stand-alone chamber for the substance encapsulation and reaction without cross-interference, facilitating subsequent droplet counting and absolute quantitative analysis for ddPCR. Herein, several of the mentioned droplet generation methods are described in detail below.
2.1. Passive generation
There are usually four geometric shapes of the channel by the passive droplet generation method: T-junction structure, flow-focusing structure, co-flowing structure, and step structure. At present, the most common geometric channel structure in the passive method is the flow-focusing structure. Passive generation of droplets allows for fast, automated, and high-throughput analysis.
2.1.1. T-junction.
The T-junction channel was first proposed by Thorsen et al. The structure consists of a T-junction tube with the sample solution as the dispersed phase in the vertical direction and the oil phase as the continuous phase in the horizontal direction,61 as shown in (Fig. 2A). Thorsen et al. believed that the competition between the shear force and the surface tension of the dispersed phase at the outflow geometry of the tube caused the steady state of the liquid itself to be disrupted, and eventually the sample solution was squeezed into droplets by the shear stress greater than the surface tension. Guillot et al. attributed droplet generation in the T-junction channel to the blocking-pinch mechanism subjected by flow rate conservation.62 Menech et al. demonstrated that the main reason for droplet formation is the equilibrium of hydrostatic pressure in the immiscible two-phase fluid rather than shear stress. T-junction channels generate droplets either by the squeezing or jet mechanism, jets are only generated at high flow rates and low interfacial tension while the squeezing mechanism occurs at lower velocity of flow.63 Although the T-junction is simple to fabricate and operate, it might generate bubbles or heterogeneous droplets at the beginning of droplet generation. To attain good reproducibility, V-junction (Fig. 2D) or K-shaped channels are developed as variants of T-junction channels, in which the extra channel is used as an outlet for the waste droplets, allowing the selection of the generated droplets.64,65 Traditional methods for adjusting the channel size are complicated and require fabrication of new chips in general. González-Estefan et al. investigated the effect of the swelling characteristic of PDMS on the geometry of the generated flow channels. The geometry of the chip can be adjusted by fine-tuning the microfluidic chip in situ with the swelling of the PDMS material, and the method can reduce the volume of the generated droplets by two orders of magnitude for T-junction channels.66
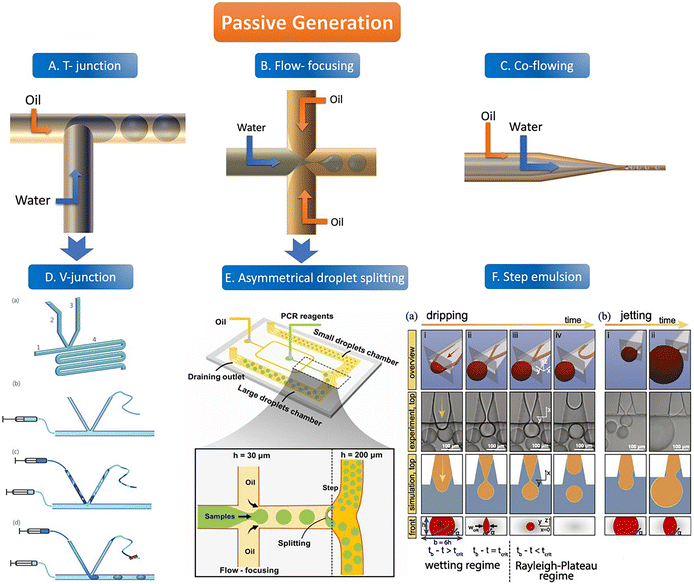 |
| Fig. 2 The abridged general view of passive generation of droplets. A–C: The orange arrow represents the flow direction of the oil phase (continuous phase) and the blue arrow represents the flow direction of the water phase (dispersed phase). A. T-junction structure. B. Flow-focusing structure C. Co-flowing structure. D. V-junction (the variant of T-junction). (a) Schematic of the V-junction structure containing an oil inlet (1), a side channel (2) for water introduction, a side channel (3) for control, and a main channel and an observation zone (4). (b–d) Schematic illustration of chip operation. Copyright 2015 by RSC.65 E. Asymmetrical droplet splitting (following behind the flow-focusing structure). Copyright 2022 by Elsevier.71 F. Step emulsion. (a) Droplet formation in a step emulsification device at different times (a, i–iv). (b) For high flow rates, the thread remains stable and the bulb continues to grow until it is finally sheared off in the reservoir (b, i–ii). Copyright 2018 by PNAS.74 | |
2.1.2. Flow-focusing structure.
The most commonly used droplet-generating structure on chips for microdroplet generation is the flow-focusing structure because this method can generate size-controllable and uniform droplets with high frequency, which can reach kHz.67,68 The flow-focusing structure uses a crossover type channel, where the liquid flowing in the horizontal channel is the dispersed phase, and the oil flowing in the vertical flow path on both sides is the continuous phase. When the dispersed phase and the continuous phase meet at the crossover, the dispersed phase is interrupted by the continuous phase under the pressure of the liquid flowing in the two sides of the channel, thus forming droplets (Fig. 2B).57,69 To enable droplet generation and transport for analytical purposes, the droplet can be regulated by adjusting the design of the microchannel structure, the ion concentration of the surfactant and the properties of the substrate surface.70 Wei et al. have added an asymmetrical droplet-splitting microstructure after the flow-focusing, which allows the large droplets generated by the flow-focusing structure to split into smaller droplets (Fig. 2E). This method can produce 30
000 droplets with a molecular quantitative dynamic range of DNA of more than 104 times.71 The dynamic range (DR) (d) is the span of detected concentrations of the sample. The use of droplets of different sizes is to expand the upper limit of quantification (ULQ) (λU) and reduce the lower quantitative limit (LDL) (λL),
. When d is larger, the span of sample concentration which can be detected is larger. ULQ is defined as the concentration with a 95% chance of generating at least one negative droplet. Accordingly, LDL is defined as the concentration with a 95% chance of generating at least one positive droplet. DR is determined predominantly by the volume of individual droplets, especially the small droplets. When detecting samples of different concentrations, ddPCR requires pre-experiments to explore an appropriate PCR sample concentration. The critical parameters of the low-end and high-end limits of detection are fluorescence intensity and the diameter of droplets. The fluorescence intensity has a positive correlation to the percentage of positive droplets. Thus, based on the percentage of positive droplets and the total volume of droplets, the copy number can be calculated. The system has been validated with an amplification of 20 to 12
500 copies per μL of chicken DNA. Thus, a wide range of concentrations can be detected by the system.
2.1.3. Co-flowing structure.
The co-flowing structure uses a channel similar to a coaxial casing, where a small-diameter channel is contained in the center of a large-diameter channel. The small diameter channel is filled with the dispersed phase, while the large diameter channel contains the continuous phase. When both of them flow together from the outlet of the channel, the dispersed phase in the small-diameter channel is crushed by the sidewall and broken up into droplets, followed by being wrapped in the continuous phase (Fig. 2C).58,72 Cramer et al. studied the droplet kinetics from drop at low flow velocity to jet at high flow velocity, as well as the interfacial tension and viscosity ratio between the continuous and dispersed phases during droplet generation, which was more comparable to the mechanism of spraying or breaking at the tip of a needle. The higher the flow velocity, the smaller the droplet generated.72 Utada et al. investigated the mechanism of droplet dropping from the capillary tip to the jet, and proposed that this mechanism was dependent on the capillary number of the external liquid and the Weber number of the internal liquid. In addition, it emphasized the importance of coflowing viscous liquid in the droplet dropping to jet process.58
2.1.4. Step emulsion.
The step emulsification method is one of the microfluidic droplet generation methods using the spontaneous formation of droplets induced by Laplace pressure differences from the geometric structure of the microchannel, with the characteristics of low shear force, insensitive flowing, and simple structure as well as ease of integration. The basic structure of step emulsion includes a narrow channel and a slit-like terrace.73 In the classical step emulsion method, the water phase flows in the narrow channel before coming to the step structure. Then it splits into microdroplets spontaneously when crossing the reservoir which contains the oil phase in the step structure. Fig. 2F shows how the dispersed phase flows through a shallow wedged channel to a deeper reservoir with a continuous phase and generates a pinch-off droplet later on. The emulsion procedure can be controlled by the wetting conditions which influence the diameter of droplets. Besides, the throughput is related to the surface wetting angle.74 The designed geometry structure also determines the size of droplets. The confined gradient is produced by the nonparallel top and bottom walls which leads to an inclined angle of the reservoir. When the angle is flat, the droplet will expand in the reservoir with the continuous phase infinitely and cannot be pinched off theoretically. However, a tiny inclined angle will change the situation. The dispersed phase becomes an elongated tongue in the narrow channel, and then the thread with the tongue shrinks and generates droplets suddenly before being pinched off.75
Some step emulsion devices based on the classical structure have been improved. Schuler et al. made a ddPCR on a disk and combined the step emulsion structure with a centrifugal device which can split the droplet from a narrow nozzle to a center zone containing a continuous phase. The monodisperse droplets enter the deeper reservoir with the centrifugal force and float on the top of the reservoir into a monolayer which is easier to count.76 Park et al. designed a pushbutton-activated microfluidic generator for ddPCR instead of a complex micropump. After adding the continuous and dispersed phase solutions containing the sample to the droplet dispenser, tens of thousands of droplets can be prepared in the PCR tube for ddPCR detection at the push of a button along with the air being crushed. The droplet size can be adjusted by changing the shape of the microchannel, and the frequency of droplet generation can be manipulated by the number of button presses. The nozzle of the dispersed phase is inserted into the reservoir with a continuous phase. Compressed by air, the water phase is squeezed into the oil phase and becomes a W/O droplet floating in it and being collected.77
2.2. Active generation
The active method of droplet generation is mainly used to generate droplets by breaking the liquid flow with the help of external forces. Compared to the passive generation of droplets, the devices of active generation are more complex and skillfully manufactured. The active generation device has better droplet control ability and can generate various droplets to meet the analytical requirements.
2.2.1. Micropillar array for droplet generation.
For meeting the requirement of the wide dynamic range of detection, especially for samples with large concentration differences, a micropillar array is designed for generating multiple microreactors which not only keeps the dynamic range wide but also reduces the number of reaction vessels. The droplet generation with a micropillar array relies on the surface-assisted technique rather than the continuous flow method. Liu et al. developed a surface-assisted multifactor fluid segmentation (SAMFS) combined with robotic liquid handling and a hydrophilic micropillar array to realize precise control for droplet generation. The different-diameter micropillar arrays were fabricated on a chip to generate various sizes of droplets. The water phase was driven out of the capillary tip while it scanned the surface of the hydrophilic micropillar array. The chip was moving around below the capillary tip until the growing water phase on the tip touched the micropillar array. The water phase was then captured and formed droplets on the micropillar array, and after that each micropillar tip had a microdroplet as shown in (Fig. 3A).78
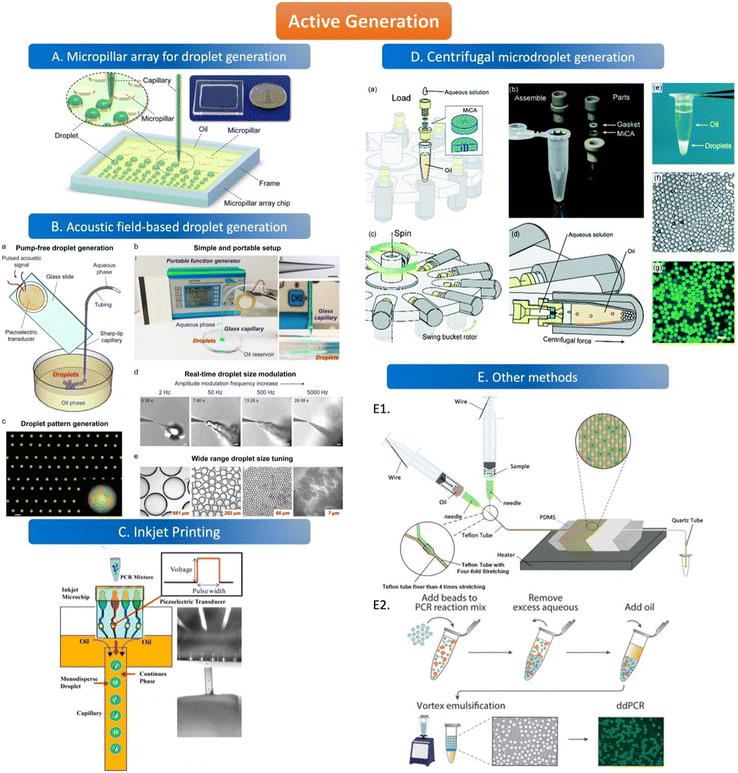 |
| Fig. 3 Active generation. A. Micropillar array for droplet generation. Schematic diagram of the micropillar array chip. Copyright 2016 by ACS.78 B. Acoustic field-based droplet generation. Concept and features of droplet generation based on a vibrating sharp-tip capillary. (a) Schematic of pump-free droplet generation by a vibrating sharptip capillary. (b) Simple and portable setup of the system: i. image of the real system setup; ii. image of glass capillary with the tip OD of 20 μm, scale bar = 100 μm; iii. Side view of droplet generation from the vibrating sharp-tip capillary. (c) Printed droplet array by incorporating a X–Y stage.(d) Real-time droplet size modulation. (e) Wide tuning range of droplet size. Copyright 2021 by Elsevier.79 C. Inkjet printing. Principle of monodisperse droplet generation by the inkjet system. Copyright 2018 by ACS.85 D. Centrifugal microdroplet generation. Construction and operation principle of the MiCA-emulsifier. (a) Assembly of a container with MiCA. The main body was made of PEEK with a PTFE gasket ring. (b) The components. (c) The swing buckets with microcentrifuge tubes and MiCA inserts will flip centripetally when spinning. (d) During spinning the centrifugal force is perpendicular to the MiCA plate, breaking the solution into small droplets which then form emulsion in the receiving oil. (e) Emulsion is stably sitting at the bottom of a microcentrifuge tube after centrifugation. (f) Microphotograph of emulsion droplets after 40 thermal cycles of PCR. (g) Fluorescence microphotograph indicating the digital amplification within the emulsion. Scale bars: 100 μm. Copyright 2017 by RSC.87 E. Other methods. E1. Schematic of the compressed air-driven continuous-flow thermocycle digital PCR. Copyright 2020 by MDPI.60 E2. Particle-templated emulsification. Copyright 2018 by ACS.96 | |
2.2.2. Acoustic field-based droplet generation.
Droplet generation using acoustic flow vibration does not require additional pressurization devices but uses a pointed capillary instead of a solid tip, by using acoustic waves to vibrate the capillary tip so that droplets flow in the neck of the capillary tip, and when there is no vibration, the flow immediately pinches off at the neck to generate droplets automatically (Fig. 3B). The droplet size and generation frequency can be achieved by adjusting the amplitude and waveform of the acoustic wave. This method has the advantage of integration and low power consumption without the need to design microfluidic channels and perform surface modifications.79
2.2.3. Inkjet printing.
Inkjet printing ejects a volume of microliters or even nanoliters of liquid droplets with the help of the printer's nozzle. Since digital PCR analyzes cells in biological samples or tissues containing the genetic material etc., to prevent the sample from being destroyed due to heating, thermal bubble printing cannot be adopted for droplet generation while piezoelectric inkjet printing is usually used.80 The principle of piezoelectric inkjet is to apply piezoelectric materials such as piezoelectric crystals or ceramics to produce a piezoelectric effect when driven by an electric field, which deforms and squeezes the liquid out of the nozzle to form microdroplets.81–84 Zhang et al. developed a droplet-based digital PCR based on inkjet printing, in which the reaction solution was placed in an ink cartridge and the nozzle was placed in an oily dispersion phase. The tiny droplets ejected by squeezing the nozzle were immediately wrapped by the dispersed phase to form dispersed O/W droplets, enabling online droplet amplification without any droplet transfer step, which reduced cross-contamination and reagent consumption (Fig. 3C).85
2.2.4. Centrifugal emulsion.
The centrifugal emulsion is based on a designed channel for the dispersed phase and a centrifugal device. The geometry of the droplet generation channel can be T-junction, co-flowing, flow focusing86 structures, etc. The classical centrifugal emulsion device can refer to the ddPCR on disk.76 The droplets are split out of the nozzle with centrifugal force. Chen et al. designed a device with a centrifugal microchannel array. The device in Fig. 3D indicates that the water phase is split into droplets through the micro-channel array in the vessel and collected in it containing with oil phase which can continue thermal cycling and amplification as well as detection directly. Without the transition of droplets, the contamination of the sample can be reduced greatly. Besides, the sample solution can be divided into several microcentrifuge tubes to realize detection simultaneously. The size of the droplet is determined by the revolving rate and the diameter of the microchannel.87
2.2.5. Other methods.
The electric field-based droplet generation method mainly relies on digital microfluidic technology. Based on the dielectric wetting phenomenon, a voltage is applied at one end of the reservoir electrodes, causing the liquid to drag out into a column of water, after which the voltage is disconnected as the column of water is cut off between the electrode units to form small droplets.59 The position and droplet size together with the stability of the micro-droplets produced by dielectric wetting depend on the amplitude and frequency of the applied voltage, the surface tension and wettability of the liquid, and also the shape and parameters of the electrodes.88,89 The generation of small droplets from large droplets can be achieved using the integration of multiple electrodes.90 Using this satellite droplet generation method, Li et al. applied a digital microfluidic approach to control the initiation signal to regulate the droplet ejection position and volume by turning on/off the switch at the actuation signal, and the sudden actuation above the threshold voltage triggered the release of the satellite droplet.91 Integrating multiple electrodes on a single chip reduces the bulkiness of the droplet generation device, which may have a potential application in ddPCR.
The way of droplet generation by magnetic actuation depends on the response of the magnetic fluid to the magnetic field. The only fluids that can be applied in this method are magnetic fluids rather than non-magnetic fluids. Ferromagnetic fluids can be used as both oil and water phases because of their paramagnetic characteristics, which are magnetic in the presence of an external magnetic field and non-magnetic in the absence of an external magnetic field. The droplet size and density generated by a magnetic fluid under the control of an external magnetic field are related to the position of the magnet and the magnitude of the magnetic force.92,93 The inhomogeneous magnetic field increases the wettability of the hydrophobic surface and thus reduces the surface tension between the droplets and the solid surface.94 Magnetic actuation does not require any external source and can also be manually controlled. This approach may be applied to ddPCR in the future.
The droplet generation devices can also be simple equipment without complicated structure or design. A concise schematic diagram (Fig. 3E1) shows microdroplet generation by compressing the needle cylinder. The combination of a syringe and a microfluidic tube can produce uniform droplets without complicated experimental setups and operations. The Teflon tube is designed to maintain a consistent droplet flow rate and generate droplets with good stability. The two syringes contain the oil and aqueous phases of the solution, and the needle on the syringe is the key to droplet generation. When the syringe is pushed, the air inside the tubing is squeezed by the piston at a pressure higher than atmospheric pressure, allowing the two phases to be pumped to the droplet generator.60 The benefit of microdroplet generation is reducing droplet fusion due to the air plug. Besides, water-in-oil droplets can be generated by vortexing the continuous phase and dispersed phase simultaneously which doesn't need an extra geometry structure for droplet generation.95 Hatori et al. invented a method of particle-templated emulsification (PTE) which can obtain a defined size of droplets using appropriate particles as a template to generate droplets. The template particles are first thoroughly mixed with the PCR reagent and samples, and the small molecules can diffuse into the particles but the large molecules like genomic DNA can only be contained in the aqueous phase. Then, the mixture is centrifuged to obtain PCR reagent-containing particles and large-molecule samples, followed by adding the oil phase to generate monodispersed droplets with vortex. Thus, each droplet is composed of a PCR reagent-containing template particle inside and samples outside which disperses in a thin layer of the aqueous phase surrounding on surface of the particle (Fig. 3E2). Thus, the size of the droplet is similar to the particle which can ensure the uniformity of the droplet.96
3. Thermal cycle and amplification process
The thermal amplification steps in ddPCR can be divided into two categories according to the principle: spatial domain amplification and time domain amplification.
3.1. Space domain ddPCR
The geometric channels used for spatial domain amplification are usually serpentine, radial, and spiral channels. The spatial domain amplification is based on a long geometric channel designed with heating modules of different temperatures placed in different areas of the channel, the temperature of these modules is kept at a stable value (95 °C, 55 °C, 72 °C). When the sample flows through the channel, the target nucleic acid molecules are denatured in the 95 °C region, and primers are attached in the 55 °C region and extended in the 72 °C region through the different heating modules. The temperature control element consists of either a resistive heater, for instance, a heat conductor with a temperature sensor, or a new composite material, which uses an outer optimization loop and an inner infinite element to achieve the desired temperature change of the channel.97,98 Resistive heating devices can be modified to integrate several electrochemical sensors and resistive heaters using circuit boards to create microcontrollers.99
In the serpentine channel, the droplet will pass through the entire channel and amplify the nucleic acid in different temperature-controlled zones of the channel. A single amplification occurs when a droplet passes through a repetitive unit in the serpentine channel. Exponential amplification is achieved by passing the droplet sequentially through the repetitive units of the serpentine channel, with the amplification index determined by the length of the channel. The PCR chip prepared by Kopp et al. used a serpentine structure for the heated amplification of Neisseria gonorrhoeae. The three heating modules were set at 95 °C, 77 °C, and 60 °C, and the samples were amplified by passing through the serpentine channel in the chip through the three temperature-controlled zones in sequence, achieving 20 cycles in 18.7 to 1.5 min.100 The results illustrate the possibility of using the serpentine structure for heated amplification of PCR. The flow of liquid in the serpentine channel required external force pumping, but the capillary self-service transport method was developed to deliver 1600 mm of PCR solution flow in 15 minutes without external pumping, and the flow path was designed according to the time required for the liquid to remain in the temperature-controlled zone.101 When using liquid plug-flow, compared with traditional continuous flow, plug-flow has interfacial tension between temperature gradients, which makes the flow speed and transit time of plug-flow change dramatically. This variation is reflected in the slow heating process but fast cooling speed (Fig. 4A).97
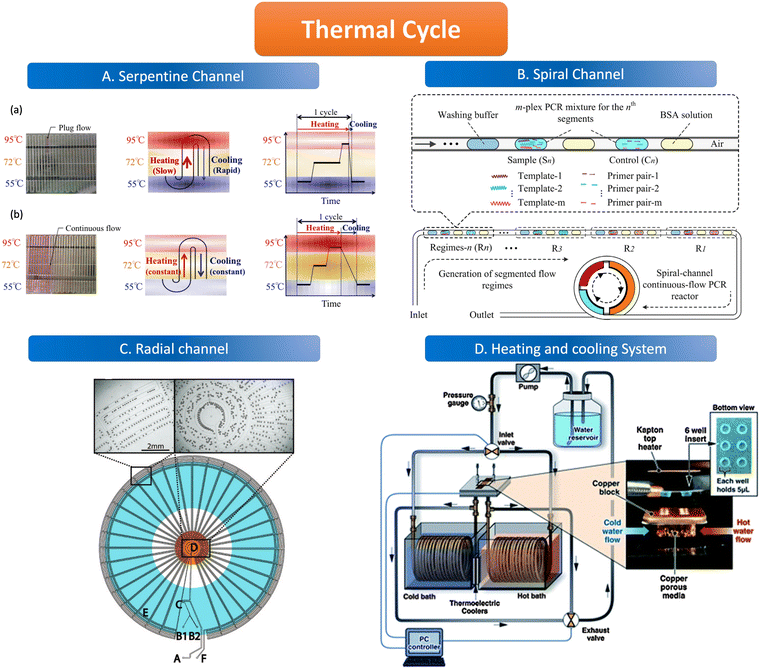 |
| Fig. 4 Features of the thermal cycle. A. Serpentine channel. (a) Plug flow-based thermal cycling technique presented by using a temperature gradient between 95 °C and 55 °C; (b) continuous flow-based thermal cycling. Copyright 2014 by MDPI.97 B. Spiral channel. Illustration of design principle of spiral-channel segmented continuous-flow multiplex PCR amplification. Copyright 2014 by Elsevier.102 C. Radial channel. The device contains an oil inlet (A) that joins two aqueous inlet channels (B1 and B2) to form droplets at a T-junction (C). The droplets pass through the inner circles (500 μm wide channels) in the hot zone (D) to ensure initial denaturation of the template and travel to the periphery in 200 μm wide channels where primer annealing and template extension occur (E). The droplets then flow back to the center, where the DNA is denatured and a new cycle begins. Finally, the droplets exit the device after 34 cycles (F). Copyright 2009 by ACS.103 D. Heating and cooling system. System schematic with an expanded view in the insert, wells and Kapton heater. Copyright 2011 by RSC.105 | |
The channel line containing the droplets is wound in a hollow spiral, and the cylindrical part wrapped in the center is the heating module, in which the central cylinder is divided into several different temperature modules. When the droplet containing the substance to be examined passes through one circumference of the spiral tube, (i.e., undergoes denaturation, annealing, and extension processes) to achieve an amplification cycle, the total number of cycles depends on the number of turns the spiral tube is wrapped around. The length of the amplification region is twice as long as the denaturation and annealing regions thus allowing for better amplification (Fig. 4B).102
The flow of liquid droplets in the radial channel is a cycle from the edge to the center and then to the edge. From the center to the edge in the order of denaturation–retrogeneration–annealing regions or vice versa, the different temperature-controlled regions are of concentric circular structure (Fig. 4C).103 Madadelahi et al. applied diamond nanoparticles in two phases to create a droplet-based continuous flow PCR device that coupled a serpentine channel for droplet generation and a helical channel for heated amplification. The addition of the appropriate concentration of diamond nanoparticles to the PCR reaction solution increased the thermal conductivity and improved the PCR performance by a factor of 5 and saved time.104
3.2. Time domain ddPCR
Time domain amplification does not depend on the slow flow of the sample through the geometric channel, but rather uses a variable temperature heating module that sequentially heats the droplets in the channel to 95 °C, 55 °C, and 72 °C to denature–relapse–extend the target nucleic acid molecule which requires heating and cooling procedures. As the sample is dispersed into the droplet, it remains stationary in the chamber while the heating module sequentially varies according to the set time and temperature to amplify the nucleic acid molecules. The number of copies of the nucleic acid molecules amplified depends on the number of cycles of temperature variation performed by the temperature control module. The samples are immobile in the reaction chamber but are transferred to signal-counting devices later on.
Time domain heaters for ddPCR are classified into several types, including infrared light and a similar device like tungsten light to heat the sample without convection, as well as a silicon device with a heating resistive module and a temperature sensor. The cooling procedure is alternated with the heating process which can be realized by the heating exchange or the active cooling system (Fig. 4D) such as mini fans in temperature control devices.105
4. Signal counting
The common method for signal counting is based on the in-line measurement of the droplet. The detectors include fluorescence microscopes, customized high-throughput fluorescence detectors, or flow cytometers. Optical-based detection methods are mainly established on the foundation of qPCR, which is combined with fluorescent probes to count positive droplets using microscopy imaging before the target nucleic acid in the sample is copied.106 The target sequence will emit fluorescence by specifically binding with the probe, and the PCR detector will collect the fluorescence signal, which represents the information related to the target DNA molecule. After the optical signal is output by the photoelectric detection circuit, it is transmitted to the chip of the PCR detector for processing. In the chip, the digital fluorescence signal needs to detect the peak value, and the number of positive and negative droplets can be obtained by the peak value detection. If the detection result of the peak value is not accurate, it will lead to false positive droplet and false negative droplet identification, thus affecting the quantitative analysis of target DNA molecules. Usually, the detector is equipped with a double-emission wavelength laser, coaxial optical path, microscopic optical path, and splitter optical path. Hatch et al. designed a wide-field fluorescence imaging system that could detect more than 1 million monodisperse droplets of 50 picoliters with a low-cost 21-megapixel digital camera and macro lens with an 8–12 cm2 field-of-view at 1× to 0.85× magnification, greatly increasing the throughput and imaging capability of the assay (Fig. 5A).106 To improve the instability and inefficiency of ddPCR using small field microscopy that requires image stitching to observe imaging, Shen et al. developed a rapid nucleic acid quantification system based on a white laser light source and a large field of view. This system uses a white laser light source as the excitation light to enhance the fluorescence signal compared to the discontinuous spectrum emitted by a mercury lamp, and prolonging the exposure time increases the brightness without the need for an image stitching technique, thus improving the detection efficiency of ddPCR.107 The electrochemical-based detection methods rely on the changes in the impedance or capacitance of the droplet. The chemical signal of the droplet is converted into an electrical signal output by the action of electrodes, which is more conducive to chip integration and miniaturization than optical detection methods.108
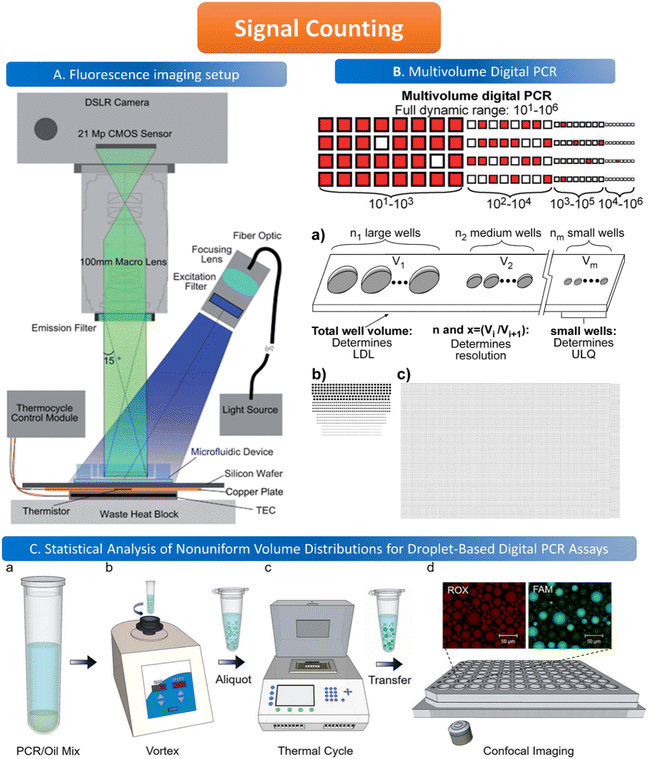 |
| Fig. 5 Illustration of signal counting. A. Wide field of view fluorescence imaging setup. Copyright 2011 by RSC.106 B. Multivolume digital PCR. (a) General schematic of the multivolume system used for digital PCR (MV digital PCR), with relationship between device features and performance abilities. Two hypothetical devices with identical dynamic range and with equal spacing (300 μm) between wells; (b) a model MV digital PCR system (160 wells each at 125, 25, 5, and 1 nL); and (c) a single volume digital PCR system (12 000 wells at 2.08 nL). Copyright 2011 by ACS.28 C. Statistical analysis of nonuniform volume distributions for droplet-based digital PCR assays. a and b. A PCR/oil mixture was vortexed to create an emulsion of nonuniform volume distribution. c. The emulsion aliquots underwent PCR in a thermal cycler and then were transferred to a well-plate for imaging using scanning confocal microscopy. d. The red fluorescence image displays a signal from the ROX dye (present in all droplets); the green image displays a signal from the FAM dye (significant only in occupied droplets). Copyright 2019 by ACS.110 | |
When using microfluidics to generate uniform and stable droplets in ddPCR, the copy number of nucleic acid molecules contained in the droplets should theoretically follow the Poisson distribution,109 (i.e.,
) where λ is the average copy number of template nucleic acid molecules in each droplet, and k is the copy number of nucleic acid molecules contained in the droplet. As a result, the probability of the number of positive droplets to the total number of droplets, (i.e., 1 − P(0)), was substituted into the formula to calculate the value of P(0) to obtain λ = −ln[1 − P(0)], and the total number of positive droplets was solved by K = λN, given that the total number of microdroplets was N. The concrete procedure of mathematical simulation can be referred to the research of Majumdar et al.109 The original digital analysis is based on multiple small-volume droplets which require the uniformity of droplets. However, it's difficult to avoid contamination and fusion during the transfer of droplets. A new method for signal counting doesn't need uniform droplets. It measures the size of each droplet using a microscope but does not need high precision and does not use Poisson distribution to calculate the occupancy (Fig. 5B and C).28,110 The simulation of nonuniform droplet division indicates that the dynamic range has a significant improvement in comparison with the uniform droplets, and the error is acceptable.
5. Applications
5.1. Single-cell analysis
Liquid can be dispersed into tiny droplets as independent reaction chambers in ddPCR, which is comparable to the volumes of cells. Thus ddPCR is suitable for the high-throughput and high-sensitivity analysis of single cells, especially for effectively analyzing small amounts of genetic material in single cells including genetic materials in the nucleus, organelles, or trace nucleic acids in the cytosol.71 Usually, extraction and purification of DNA are important steps before single-cell analysis, which include lysis, washing, binding, and elution. These operations consume a lot of time, and the amount of DNA after purification limits the quantitative results of the assay.
In response to the many problems in DNA extraction and purification, ddPCR, which has the advantage of high throughput and sensitivity, is later also demonstrated to be directly applicable to the analysis of the genetic material in single cells without purification of DNA. O'Hara et al. applied ddPCR to quantitatively analyze mitochondrial DNA (mtDNA).41 The traditional method for mtDNA detection is still qPCR. A series of problems exist in this method, such as poor reproducibility of the results due to the trace amounts of purified mtDNA, which are easy to be lost, differences in the amplification efficiency of mtDNA and housekeeping genes, and different values of mtDNA/nDNA obtained by different methods. In contrast, ddPCR can be performed directly using cell lysates or individual cells without a purification step, and ddPCR has the advantage of absolute quantification and solves the problem of poor reproducibility, and allows quantitative assessment of the heterogeneity of mtRNA deletion.33 Dahotre et al. developed an approach based on a DNA barcode-labeled peptide–MHC (pMHC) tetramer and ddPCR with high sensitivity that allows labeling of T cells in complex high background matrices without subjecting them to destruction. This method can be used for monitoring tumorigenesis (Fig. 6).111
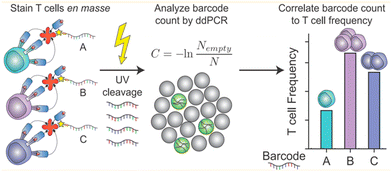 |
| Fig. 6 A mixture of different antigen-specific T cells can be stained with barcoded tetramers en masse. Barcodes are isolated by irradiation with UV light and analyzed by ddPCR. Copyright 2019 by ACS.111 | |
ddPCR can also be used for the detection of RNA in single cells. The difficulty in detecting the mutant type of messenger RNA in single cells is that the cells contain a lot of wild-type RNA (wtRNA), which can cause severe background interference.
The sensitivity and specificity of the traditional detection method, qPCR, are not strong enough to distinguish quite small amounts of mutant RNA in single cells, so new methods are needed to detect and analyze mutant mRNA in single cells. Using ddPCR, 0.01% of mRNA mutants can be identified in wild-type mRNAs with a high background. Sun et al. designed a PNA clip combined with ddPCR technology to detect mRNA mutations, and only droplets containing a single nucleotide mutated sequence (mutRNA) can interact with the TaqMan probe to turn on the fluorescence, thus making the droplets positive. In the case of droplets containing wtRNA, the wtRNA is not amplified by the PNA which makes the droplet negative.112
Detecting and analyzing miRNA in a single cell is significant to find the correlation between miRNA and single-cell function. Tian et al. also created a miRNA analysis method in a single cell by ddPCR (Fig. 7). This method doesn't need to design complicated reverse transcription but makes two target-specific oligonucleotide probes which are complementary to the half-sequence of the target miRNA. The miRNA can be detected by ddPCR after the miRNA ligates with the templates. The miRNA in a single-cell lysate can be detected with an LDL of 12 copies per microliter which realizes the precise quantification.113
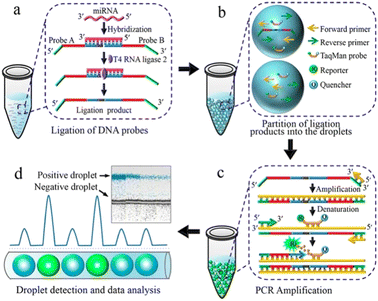 |
| Fig. 7 Principle of the ddPCR-based miRNA assay. a. Ligation of DNA probes. b. Partition of ligation products into the droplets. c. PCR amplification. d. Droplet detection and data analysis. Copyright 2016 by ACS.113 | |
5.2. Disease diagnosis
ddPCR has also played an important role in the field of disease diagnosis in recent years by relying on its advantages of achieving amplified signals of targets with small sample consumption, absolute quantification, and ultra-high sensitivity. Clinical applications such as liquid biopsy, molecular diagnostics, and cancer screening have widely used ddPCR technology to extract and detect trace amounts of target substances in a limited sample volume for clinical diagnostic purposes.
With the development of medical testing technology, the treatment of tumors has advanced to the early screening stage, which is a key step in the diagnosis and treatment of cancers. In addition, the biopsy is also the most direct and rapid way to diagnose tumors, and the testing of patients' body fluid samples can also evaluate the efficacy of the treatment. Puncturing the patient's lesion to obtain a sample and test it is a common method to diagnose tumors in clinical practice. For example, Xu et al. applied ddPCR to detect the BRAF V600E mutation in the fine needle aspiration (FNA) sample from papillary thyroid carcinoma (PTC) patients.114 The regular methods to detect the mutation such as immunohistochemistry, amplification-refractory mutation system (ARMS), and sequencing are not so precise and sensitive for the trace mutant cells compared to ddPCR. The small sample volume and invasiveness of the puncture biopsy method also expose patients to the risk of infection, puncture failure, or contamination of surrounding tissue due to improper handling, making patient compliance with the test poor. The puncture procedure must also be operated by an experienced physician, which places high demands on the surgeon. Considering the problems mentioned above of puncture biopsy, the concept of liquid biopsy has been proposed in recent years and is gradually being promoted and applied in the process of adjuvant tumor diagnosis because of its characteristics of minimal invasiveness, accuracy, and feasibility. Cancer patients usually have a small amount of circulating tumor cells (CTCs) in their blood, and some of the necrotic cells may also release cell-free DNA (cfDNA), so the detection of CTCs or cfDNA in blood with the help of ddPCR is one of the main ways of liquid biopsy, which can help rapid diagnosis.
ddPCR can be used to assess the extraction efficiency of cfDNA in plasma and its recovery after modification with bisulfite.115 Aya-Bonilla et al. used ddPCR to analyze transcripts and studied CTC heterogeneity in blood samples from melanoma patients as one of the bases for clinical diagnosis.43 Wang et al. used ddPCR to quantify T790M transcripts in enriched CTCs (Fig. 8).116 Ou et al. also developed an integrated comprehensive droplet digital detection (IC3D) digital PCR system that also allows the high-sensitive analysis of total tumor cell DNA isolated from blood samples without the pre-enrichment of CTCs (Fig. 9).117
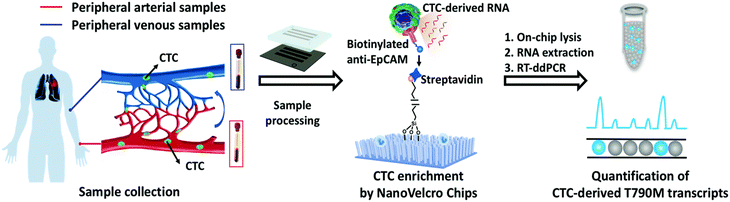 |
| Fig. 8 Workflow of the NanoVelcro CTC-digital assay for detecting the EGFR T790M mutation. Copyright 2020 by RSC.116 | |
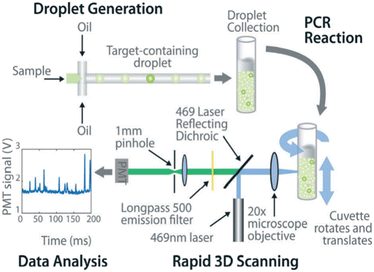 |
| Fig. 9 IC3D ddPCR workflow diagram for a typical clinical sample. The IC3D technology involves partitioning a sample into millions of picoliter-sized droplets, thermocycling the droplets to amplify specific fluorescence signals, and detecting/quantifying droplets that are positive for one or more specific targets. Unlike commercial dPCR systems, the IC3D technology can analyze large sample volumes with a great number of partitions, which can collectively improve the sensitivity of ctDNA detection. Copyright 2019 by RSC.117 | |
One of the cancers which have been getting the most attention recently is a variety of squamous cell carcinomas caused by HPV, including anal, oral, and cervical locations. It can be diagnosed and quantified by ddPCR detection of circulating tumor DNA (ctDNA), which is very essential in determining disease progression before therapy.118 Veyer et al. applied ddPCR to examine HPV-related CTCs and explored the function of ctDNA content in the kinetics of HPV tumor formation to determine the prognosis of cancer.35 Galati et al. assessed whether the magnetic bead-based HPV genotyping test for HPV16 can be utilized as a biomarker for cervical cancer (CC) in ddPCR.119 Additionally, the detection of cell-free DNA (cfDNA) in saliva by ddPCR, which has strong sensitivity for HPV16-OPC, can help identify HPV-induced oropharyngeal cancer (OPC).120,121
In addition to CTCs for liquid biopsy to diagnose cancer, ddPCR also detects mutated genes or tumor markers to allow a comprehensive determination of the extent of disease progression. Decraene et al. developed a ddPCR approach for unknown mutations as a diagnostic method for tumors. It can detect more mutated genes by consuming minimum samples with single reaction ddPCR which is more convenient than multiple reaction ddPCR needed for separate detection aimed at each mutation.122 Cheng et al. determined that the Kras (G12D) mutation status was associated with high levels of regulatory T cells (Treg) infiltration in resectable pancreatic cancer tissues by ddPCR.46 Goss et al. detected Kras mutations and MAP2K1 mutations by ddPCR, thus identifying mutated genes in “intramuscular capillary hemangioma type” (IHCT) lesions.123 Similarly, Callens et al. validated ddPCR to screen for ESR1 mutations in patients with metastatic breast cancer for therapeutic intervention in tumors.124
Additionally, ddPCR has been used to examine tumors after treatment and to analyze carcinogenesis. Ahn et al. discovered that the existence of uncommon ESR1 mutant clones in the initial tumor was related to primary endocrine resistance throughout treatment. They employed ddPCR to examine the pattern of relapse and primary endocrine resistance in patients with non-metastatic ER+ breast cancer.45 Moreover, ddPCR can be used to examine the possibility of negative effects and the need to stop using tyrosine kinase inhibitors (TKIs) in patients with chronic myelogenous leukemia (CML), assisting doctors in deciding how much medication to give each patient.37 Furthermore, minimal residual disease (MRD) surveillance of leukemia relapse has demonstrated the excellent sensitivity of ddPCR.125
5.3. Pathogen detection
ddPCR can be used to detect a variety of pathogens, such as bacteria, fungi, parasites, and viruses, which can pose a significant risk to human health in the living environment and agricultural products, as well as in animals and humans. Since ddPCR is capable of absolute quantification, even samples with minimal nucleic acid content can be detected, greatly improving detection efficiency. In the following, we will introduce the application of ddPCR in pathogen detection in recent years.
5.3.1. Bacteria detection.
One of the main issues in the area of food safety has been the discovery of the over-colonization of agricultural goods. Owing to the advantages of high sensitivity and selectivity, ddPCR-based technologies can be widely used to determine the number of bacteria in foods like poultry, meat, eggs, and milk126 (Fig. 10). The pathogen contents of raw milk and raw milk products, as well as the DNA of Coxiella burnetii, were both quantified through the use of ddPCR in the evaluation of goat and sheep dairy products that cause Q fever.127 The results demonstrated that ddPCR, with higher sensitivity and shorter preincubation time, might increase the detection efficiency when qPCR and ddPCR were compared for the detection of Salmonella typhimurium in milk.128 For the routine detection of VBNC bacteria in food, Lv et al. used immunomagnetic beads modified with antibodies to collect C. sakazakii and detect it by ddPCR with a detection limit of 5.6 copies per g.129 When qPCR and ddPCR were put side by side, ddPCR proved to be more sensitive in detecting Yersinia pestis in vegetables.130
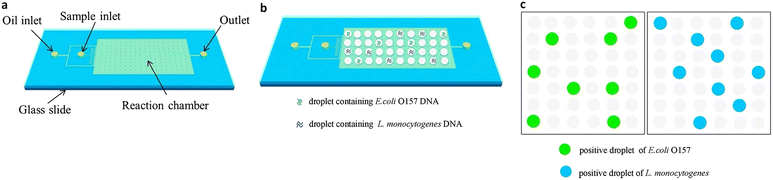 |
| Fig. 10 Droplet digital PCR workflow for simultaneous detection of E. coli O157 and L. monocytogenes. (a) Fabrication of mineral oil saturated PDMS (OSP) microfluidic chip. (b) Generation of droplets. (c) On-chip amplification under many times of thermal cycles followed by fluorescence readout. Copyright 2015 by Elsevier.126 | |
To effectively prevent and diagnose bacterial infections, ddPCR can be used to detect dangerous bacteria in both the environment and food sources. The origin and survival time of microbial groups, the traceability of microorganisms in aerosols and on surfaces in the wards of newborn preterm infants, and the comparison with metadata on the infant's gut flora can be determined using the ddPCR technique, which can aid healthcare professionals in creating disinfection plans.131 Bivins et al. in India used dead-end filtration (DEFU) to identify waterborne pathogens in water samples. The presence of the typical norovirus, Shigella, and E. coli suggests that the water may be tainted with feces.132
In terms of common pathogenic bacteria, ddPCR can be used to detect Mycobacterium tuberculosis, which is difficult to diagnose because of the small number of Mycobacterium tuberculosis and the difficulty of culturing it.133 The ability to detect Mycobacterium tuberculosis DNA in classroom air, which was counted using the Poisson distribution and compared to sputum samples from patients, suggests that the risk of exposure to air containing Mycobacterium tuberculosis in classrooms is close to that of exposure in public clinics, which is undoubtedly another major health risk. Detection of enteric microorganisms in aerosol samples and wastewater using ddPCR allows investigation and traceability of disease transmission in cities with poor sanitation, as well as identification of colonized DNA and RNA in aquatic sediments to obtain information on changes in colonization.49,134 For the quantification of bacteria and fungi in the environment, ddPCR has better accuracy, sensitivity, reproducibility and stability than qPCR, and is more resistant to PCR inhibitors.135 For bacterial detection in human samples, ddPCR can be used directly for rapid taxonomic identification and antibiotic susceptibility analysis of bacteria in human whole blood samples, especially in blood samples with low bacterial DNA content, eliminating the need for processes such as bacterial culture and pre-treatment, greatly reducing experimental time and playing an important role in early diagnosis and treatment monitoring against bacterial diseases.136,137 In addition, feces and other excreta are a large source of bacterial survival in large numbers in humans and animals, and the use of ddPCR allows quantitative analysis of fecal–oral pathogens in human and animal feces. Some samples of human origin also include saliva and nasopharyngeal swab samples, and compared to qPCR, ddPCR has better reproducibility and better specificity, shorter detection time, and better sensitivity.50,138 This is reflected in the lower detection limit of ddPCR, which avoids false negative results due to insufficient pathogen load. The advantage is valuable for laboratory analysis and clinical diagnosis as well as epidemiological studies.30,139
5.3.2. Virus detection.
Many diseases are caused by viruses. Viruses are tiny, non-cellular beings that most of them usually have only one kind of nucleic (DNA or RNA) acid as their genetic material. Viruses have an incubation period in the host and can survive in the environment for several days to months after leaving the host, making them an important source of infectious diseases. These characteristics of viruses make them one of the major threats to human life and health, and people have been fighting against viruses for thousands of years. In recent years, with the advancement of detection technology, ddPCR has been increasingly used for virus detection.
HIV has posed a serious threat to global health since its discovery in the 1980s. The HIV-DNA level is an indicator of the effectiveness of HIV treatment and it also requires the quantification of DNA subtypes of the pre-HIV virus (Fig. 11).140–142 ddPCR allows the detection of HIV-DNA in peripheral blood mononuclear cells (PBMCs) with minimal viral reservoirs and the total amount of HIV-DNA in them, which is an important reference for the progression of the disease in treated patients.143
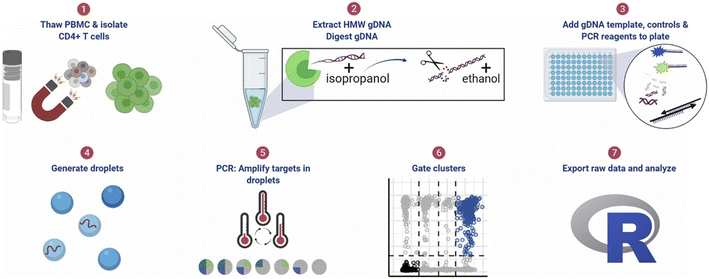 |
| Fig. 11 ddPCR protocol workflow for detecting pre-HIV. (1) Thaw cryopreserved cells and isolate CD4+ T cells. For tissue samples, CD4+ T cells were not isolated. (2) Extract high molecular weight genomic DNA (gDNA) using guanidinium salts and isopropanol precipitation and then digest gDNA with the restriction enzyme BglI and precipitate the DNA with ethanol. (3) Add specimen gDNA, control plasmids, and PCR reagents to a plate. (4) Generate droplets. (5) Conduct PCR for amplification of targets in droplets. (6) Gate populations in QuantaSoft AP. (7) Analyze results in R. Copyright 2021 by Elsevier.142 | |
SARS-CoV-2, which has caused global pandemic pneumonia since late 2019, is also an RNA virus that has been detected not only in humans but also in animals and the environment. Although the current gold standard for detecting SARS-CoV-2 is qPCR which is prone to false-negative results due to factors such as mutations in the probe or insufficient viral load in the sample, ddPCR, with its high sensitivity, low sample volume consumption, and absolute quantification, can be used as a complementary method to qPCR for detection.5
Li et al. used ddPCR to analyze clinical samples from different parts of patients' bodies infected with COVID-19 which yielded the highest positive detection rate for nasopharyngeal swabs, followed by body fluid samples such as anal swabs and saliva blood, which is a good reference for the development of detection standards for SARS-CoV-2.3 The current method for initial determination of SARS-CoV-2 in various countries is PCR amplification of RNA for SARS-CoV-2 in nasopharyngeal swab samples. Cassinari et al. used RT-ddPCR to detect the SARS-CoV-2 virus in saliva and nasopharyngeal swab specimens, and compared the results with qPCR, which concluded that ddPCR was more sensitive and produced fewer false positive results.144
Research on SARS-CoV-2 is not limited to nasopharyngeal swab specimens and serology, but researchers have also conducted many experiments on SARS-CoV-2 in environmental samples to study its epidemiology and transmission routes. For example, in Italy, which was severely affected by the epidemic when the COVID-19 pandemic first started in 2020, Chirizzi et al. used ddPCR to detect SARS-CoV-2 concentrations in aerosols from the southern and northern coasts of Italy to analyze the impact of outdoor airborne transmission on the COVID-19 epidemic.48 There are also several cases in which ddPCR was used to quantify SARS-CoV-2 in wastewater (Fig. 12),145–147 such as the detection of SARS-CoV-2 in commercial airliner and cruise ship wastewater148 and community wastewater,149 making an important contribution to public health studies concerning the epidemic of COVID-19.
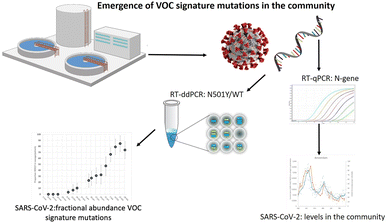 |
| Fig. 12 Droplet digital reverse transcription PCR to detect SARS-CoV-2 signature mutations of variants of concern in wastewater. Copyright 2021 by Elsevier.147 | |
6. Challenges and prospects
Microfluidic-based ddPCR has significant advantages over the previous two generations of PCR in terms of high sensitivity and low sample consumption. Besides, ddPCR does not rely on the standard curve to achieve absolute quantification, which is considered to be a major breakthrough. ddPCR has its own advantages in pathogen detection, disease diagnosis, single-cell analysis, and environmental and agricultural testing. In theory, ddPCR can be used in the field of life analysis to achieve more accurate detection. In practice, ddPCR relies on theoretical calculations that are limited by strict requirements when translated into practical applications. For example, the generated droplets must be homogeneous monodisperse droplets, which can theoretically be used as a single reaction vessel, and there is a possibility of fragmentation and fusion between the actual generated droplets. It is a major challenge to ensure efficient homogeneous droplet generation and no collisional cleavage during droplet transfer. The new method which doesn't rely on uniform droplets but measures the size of each droplet is another research approach as shown in signal counting. Besides, the second challenge is that the number of nucleic acid copies in each droplet is indeterminate. Although a droplet can theoretically contain at most one nucleic acid copy, in practice, it may contain more than one nucleic acid copy, which makes it difficult to ensure the accuracy of the final statistics. In addition, for some genes, base mismatches and low reverse transcription efficiency can lead to false negative results. In the case of some targets, the choice of the assay method has a strong impact on the reproducibility of the results.140 Poor differentiation of fluorescence amplitude between negative and positive droplets is also conducive to false negative results in the final statistics.149 Moreover, ddPCR needs higher throughput and lower cost to replace qPCR in the massive bioanalysis technically.
In response to the problems of ddPCR, new methods are designed to overcome these shortcomings. For example, to address the loss of target or droplet aggregation during droplet transfer, Chen et al. have developed a capillary-based integrated digital PCR, which uses the T-shaped structure of HPLC as a droplet generator linked to a capillary cytometer to achieve quantitative detection with a good linear range and low detection limit.150 To realize high-order multiplexed detection of nucleic acids and prevent interactions between the primers/probes, Xie et al. invented a microarray dPCR with different partitions for separating the multiple target-specific primers/probes which allow a single fluorophore to label all targets and eliminate spectral overlap caused by different probes. Moreover, the probes are prestored in the store part on the chip and can be rehydrated using a dissolvable delay valve which enhances the spatial uniformity of reaction conditions.151 In addition, in the detection of circulating tumor DNA, which is present in very small amounts in biological samples, denaturation-enhanced ddPCR is used to obtain results that improve the stability and precision of the detection of mutated genes. With the continuous efforts of researchers, ddPCR will eventually replace qPCR as a widely used nucleic acid detection tool in the future and play an increasingly important role in the field of life analysis. Besides, the droplet digital loop-mediated isothermal amplification (ddLAMP) and droplet digital recombinase polymerase amplification (ddRPA) are also becoming popular research trends in the nucleic acid detection field. ddLAMP doesn't need complicated and expensive thermal cyclers and the reaction can be finished at a constant temperature. ddLAMP also has high tolerance to the inhibitors compared to ddPCR. ddLAMP can detect the samples well in the presence of inhibitors like SDS or humic acid.152–154 ddRPA is called a technique that can replace PCR. The whole process is very fast, generally within 10 min to obtain a detectable level of amplification products. ddRPA can not only be rapidly amplified,155 but also support the simultaneous amplification of multiple reactions in the same tube.156 Thus, it can meet the requirement of rapid detection. For example, Schulz et al. invented an point-of-care testing (POCT) system based on bi-plex ddRPA instead of the complicated laborious workflow in ddPCR for detecting Staphylococcus aureus (MRSA) and the resistance against methicillin while other bacteria exist in the sample and the nuances cannot be discerned by qPCR.157 However, ddLAMP mostly relies on fluorescence dyes and fluorescence detectors are expensive as well as the high requirements of primers. ddRPA is difficult to avoid the combinations between primers which cause non-specific amplification.158 Therefore, low-cost, convenient, precise, and rapid detection of nucleic acids is the key to research.
7. Conclusions
The microfluidic-based ddPCR technique has been considered as a promising tool for single-cell analysis, disease diagnosis, and pathogen detection in environmental or food fields owing to its advantages of high throughput, high sensitivity, low reagent consumption, low cross-contamination, and absolute quantification ability. In this review, we introduced various designs of microfluidic-based ddPCR and also described the droplet generation methods, thermal cycle strategies, and signal counting approaches in detail. Although there are some challenges for ddPCR to be used as universally as qPCR, we still believe that those shortcomings will be overcome with the development of more mature new techniques. We hope that this review can provide novel enlightenment and an effective guide for new research enthusiasm.
Author contributions
Danfeng Xu wrote the original draft and modified the manuscript. W. F. Zhang organized the framework and figures, drew the TOC graph, reviewed the writing and supervised the project. H. M. Li reviewed the writing and supervised the project. Nan Li reviewed and edited the writing and contributed to the challenges and prospects. J.-M. Lin identified the topic, reviewed the writing and supervised the project.
Conflicts of interest
The authors declare that they have no known competing financial interests or personal relationships.
Acknowledgements
This work was supported by the National Key R&D Program of China (No. 2021YFF0600700), the National Natural Science Foundation of China (No. 22034005, 21621003, 22204155) and the National Institute of Metrology Fundamental Research Project (No. AKYZD2114-2).
Notes and references
- F. Yu, G. Xie, S. Zheng, D. Han, J. Bao, D. Zhang, B. Feng, Q. Wang, Q. Zou, R. Wang, X. Yang, W. Chen, B. Lou and Y. Chen, Front. Cell. Infect. Microbiol., 2021, 11, 685640 CrossRef CAS PubMed
.
- K. Cassinari, E. Alessandri-Gradt, P. Chambon, F. Charbonnier, S. Gracias, L. Beaussire, K. Alexandre, N. Sarafan-Vasseur, C. Houdayer, M. Etienne, F. Caron, J. C. Plantier and T. Frebourg, Clin. Chem., 2021, 67, 736–741 CrossRef PubMed
.
- L. Li, C. Tan, J. Zeng, C. Luo, S. Hu, Y. Peng, W. Li, Z. Xie, Y. Ling, X. Zhang, E. Deng, H. Xu, J. Wang, Y. Xie, Y. Zhou, W. Zhang, Y. Guo and Z. Liu, J. Transl. Med., 2021, 19, 30 CrossRef CAS PubMed
.
- S.-S. Lee, S. Kim, H. M. Yoo, D.-H. Lee and Y.-K. Bae, Anal. Bioanal. Chem., 2022, 414, 1773–1785 CrossRef CAS PubMed
.
- T. Suo, X. Liu, J. Feng, M. Guo, W. Hu, D. Guo, H. Ullah, Y. Yang, Q. Zhang, X. Wang, M. Sajid, Z. Huang, L. Deng, T. Chen, F. Liu, K. Xu, Y. Liu, Q. Zhang, Y. Liu, Y. Xiong, G. Chen, K. Lan and Y. Chen, Emerging Microbes Infect., 2020, 9, 1259–1268 CrossRef CAS PubMed
.
- A. Chien, D. B. Edgar and J. M. Trela, J. Bacteriol., 1976, 127, 1550–1557 CrossRef CAS PubMed
.
- R. K. Saiki, D. H. Gelfand, S. Stoffel, S. J. Scharf, R. Higuchi, G. T. Horn, K. B. Mullis and H. A. Erlich, Science, 1988, 239, 487–491 CrossRef CAS PubMed
.
- C. Wong, C. E. Dowling, R. K. Saiki, R. G. Higuchi, H. A. Erlich and H. H. Kazazian, Jr., Nature, 1987, 330, 384–386 CrossRef CAS PubMed
.
- K. Mullis, F. Faloona, S. Scharf, R. Saiki, G. Horn and H. Erlich, Cold Spring Harbor Symp. Quant. Biol., 1986, 51(Pt 1), 263–273 CrossRef CAS PubMed
.
- S. J. Scharf, G. T. Horn and H. A. Erlich, Science, 1986, 233, 1076–1078 CrossRef CAS PubMed
.
- L. M. Powell, S. C. Wallis, R. J. Pease, Y. H. Edwards, T. J. Knott and J. Scott, Cell, 1987, 50, 831–840 CrossRef CAS PubMed
.
- M. V. Ouspenskaia, D. A. Johnston, W. M. Roberts, Z. Estrov and T. F. Zipf, Leukemia, 1995, 9, 321–328 CAS
.
- R. Higuchi, C. Fockler, G. Dollinger and R. Watson, Nat. Biotechnol., 1993, 11, 1026–1030 CrossRef CAS PubMed
.
- B. Vogelstein and K. W. Kinzler, Proc. Natl. Acad. Sci. U. S. A., 1999, 96, 9236–9241 CrossRef CAS PubMed
.
- H. C. Fan and S. R. Quake, Anal. Chem., 2007, 79, 7576–7579 CrossRef CAS PubMed
.
- L. Warren, D. Bryder, I. L. Weissman and S. R. Quake, Proc. Natl. Acad. Sci. U. S. A., 2006, 103, 17807–17812 CrossRef CAS PubMed
.
- G. Pohl and M. Shih Ie, Expert Rev. Mol. Diagn., 2004, 4, 41–47 CrossRef CAS PubMed
.
- F. Shen, W. Du, J. E. Kreutz, A. Fok and R. F. Ismagilov, Lab Chip, 2010, 10, 2666–2672 RSC
.
- Y. Yu, Z. Yu, X. Pan, L. Xu, R. Guo, X. Qian and F. Shen, Analyst, 2022, 147, 625–633 RSC
.
- F. Shen, W. Du, E. K. Davydova, M. A. Karymov, J. Pandey and R. F. Ismagilov, Anal. Chem., 2010, 82, 4606–4612 CrossRef CAS PubMed
.
- S. Lindström, M. Hammond, H. Brismar, H. Andersson-Svahn and A. Ahmadian, Lab Chip, 2009, 9, 3465–3471 RSC
.
- P. Mao, L. Cao, Z. Li, M. You, B. Gao, X. Xie, Z. Xue, P. Peng, C. Yao and F. Xu, Analyst, 2021, 146, 6960–6969 RSC
.
- Q. Tian, B. Yu, Y. Mu, Y. Xu, C. Ma, T. Zhang, W. Jin and Q. Jin, RSC Adv., 2015, 5, 81889–81896 RSC
.
- B. J. Hindson, K. D. Ness, D. A. Masquelier, P. Belgrader, N. J. Heredia, A. J. Makarewicz, I. J. Bright, M. Y. Lucero, A. L. Hiddessen, T. C. Legler, T. K. Kitano, M. R. Hodel, J. F. Petersen, P. W. Wyatt, E. R. Steenblock, P. H. Shah, L. J. Bousse, C. B. Troup, J. C. Mellen, D. K. Wittmann, N. G. Erndt, T. H. Cauley, R. T. Koehler, A. P. So, S. Dube, K. A. Rose, L. Montesclaros, S. Wang, D. P. Stumbo, S. P. Hodges, S. Romine, F. P. Milanovich, H. E. White, J. F. Regan, G. A. Karlin-Neumann, C. M. Hindson, S. Saxonov and B. W. Colston, Anal. Chem., 2011, 83, 8604–8610 CrossRef CAS PubMed
.
- L. B. Pinheiro, V. A. Coleman, C. M. Hindson, J. Herrmann, B. J. Hindson, S. Bhat and K. R. Emslie, Anal. Chem., 2012, 84, 1003–1011 CrossRef CAS PubMed
.
- J. Juárez, C. A. Brizuela and I. M. Martínez-Pérez, Inf. Sci., 2017, 429, 130–146 CrossRef
.
- D. Pekin, Y. Skhiri, J.-C. Baret, D. Le Corre, L. Mazutis, C. Ben Salem, F. Millot, A. El Harrak, J. B. Hutchison, J. W. Larson, D. R. Link, P. Laurent-Puig, A. D. Griffiths and T. Valérie, Lab Chip, 2011, 11, 2156–2166 RSC
.
- J. E. Kreutz, T. Munson, T. Huynh, F. Shen, W. Du and R. F. Ismagilov, Anal. Chem., 2011, 83, 8158–8168 CrossRef CAS PubMed
.
- Y. Ouyang, G. R. M. Duarte, B. L. Poe, P. S. Riehl, F. M. dos Santos, C. C. G. Martin-Didonet, E. Carrilho and J. P. Landers, Anal. Chim. Acta, 2015, 901, 59–67 CrossRef CAS PubMed
.
- J. Luo, J. Li, H. Yang, J. Yu and H. Wei, J. Clin. Microbiol., 2017, 55, 2946–2955 CrossRef CAS PubMed
.
- P. Cremonesi, C. Garofalo, C. Picozzi, B. Castiglioni, N. Mangieri, V. Milanović, A. Osimani and L. Aquilanti, Food Microbiol., 2022, 101, 103894 CrossRef CAS PubMed
.
- S. Moron-Lopez, S. Telwatte, I. Sarabia, E. Battivelli, M. Montano, A. B. Macedo, D. Aran, A. J. Butte, R. B. Jones, A. Bosque, E. Verdin, W. C. Greene, J. K. Wong and S. A. Yukl, PLoS Pathog., 2020, 16, e1009060 CrossRef CAS PubMed
.
- T. Grudda, H. S. Hwang, M. Taddese, J. Quinn, M. S. Sulkowski, R. K. Sterling, A. Balagopal and C. L. Thio, J. Clin. Invest., 2022, e161818 CrossRef PubMed
.
- A. Stevenson, K. Wakeham, J. Pan, K. Kavanagh, D. Millan, S. Bell, D. McLellan, S. V. Graham and K. Cuschieri, J. Clin. Virol., 2020, 129, 104505 CrossRef CAS PubMed
.
- D. Veyer, M. Wack, M. Mandavit, S. Garrigou, S. Hans, P. Bonfils, E. Tartour, L. Bélec, S.-F. Wang-Renault, P. Laurent-Puig, H. Mirghani, B. Rance, V. Taly, C. Badoual and H. Péré, Int. J. Cancer, 2020, 147, 1222–1227 CrossRef CAS PubMed
.
- M. Fitarelli-Kiehl, F. Yu, R. Ashtaputre, K. W. Leong, I. Ladas, J. Supplee, C. Paweletz, D. Mitra, J. D. Schoenfeld, S. Parangi and G. M. Makrigiorgos, Clin. Chem., 2018, 64, 1762–1771 CrossRef CAS PubMed
.
- E. Atallah, C. A. Schiffer, J. P. Radich, K. P. Weinfurt, M.-J. Zhang, J. Pinilla-Ibarz, V. Kota, R. A. Larson, J. O. Moore, M. J. Mauro, M. W. N. Deininger, J. E. Thompson, V. G. Oehler, M. Wadleigh, N. P. Shah, E. K. Ritchie, R. T. Silver, J. Cortes, L. Lin, A. Visotcky, A. Baim, J. Harrell, B. Helton, M. Horowitz and K. E. Flynn, JAMA Oncol., 2021, 7, 42–50 CrossRef PubMed
.
- J. S. L. Vong, L. Ji, M. M. S. Heung, S. H. Cheng, J. Wong, P. B. S. Lai, V. W. S. Wong, S. L. Chan, H. L. Y. Chan, P. Jiang, K. C. A. Chan, R. W. K. Chiu and Y. M. D. Lo, Clin. Chem., 2021, 67, 1492–1502 CrossRef PubMed
.
- T. Profaizer and P. Slev, Clin. Chem., 2020, 66, 229–238 CrossRef PubMed
.
- S. Lei, X. Gu, W. Xue, Z. Rong, Z. Wang, S. Chen and Q. Zhong, Front. Microbiol., 2020, 11, 1727 CrossRef PubMed
.
- R. O'Hara, E. Tedone, A. Ludlow, E. Huang, B. Arosio, D. Mari and J. W. Shay, Genome Res., 2019, 29, 1878–1888 CrossRef PubMed
.
- G. Beinse, B. Borghese, M. Métairie, P.-A. Just, G. Poulet, S. Garinet, B. Parfait, A. Didelot, C. Bourreau, N. Agueeff, A. Lavollé, B. Terris, C. Chapron, F. Goldwasser, K. Leroy, H. Blons, P. Laurent-Puig, V. Taly and J. Alexandre, Clin. Chem., 2022, 68, 782–793 CrossRef PubMed
.
- C. A. Aya-Bonilla, M. Morici, X. Hong, A. C. McEvoy, R. J. Sullivan, J. Freeman, L. Calapre, M. A. Khattak, T. Meniawy, M. Millward, M. Ziman and E. S. Gray, Br. J. Cancer, 2020, 122, 1059–1067 CrossRef CAS PubMed
.
- A. Strati, M. Zavridou, P. Economopoulou, S. Gkolfinopoulos, A. Psyrri and E. Lianidou, Clin. Chem., 2021, 67, 642–652 CrossRef PubMed
.
- S. G. Ahn, S. J. Bae, Y. Kim, J. H. Ji, C. Chu, D. Kim, J. Lee, Y. J. Cha, K.-A. Lee and J. Jeong, npj Breast Cancer, 2022, 8, 58 CrossRef CAS PubMed
.
- H. Cheng, K. Fan, G. Luo, Z. Fan, C. Yang, Q. Huang, K. Jin, J. Xu, X. Yu and C. Liu, Cancer Lett., 2019, 446, 103–111 CrossRef CAS PubMed
.
- E. Jeannot, L. Darrigues, M. Michel, M.-H. Stern, J.-Y. Pierga, A. Rampanou, S. Melaabi, C. Benoist, I. Bièche, A. Vincent-Salomon, R. El Ayachy, A. Noret, N. Epaillard, L. Cabel, F.-C. Bidard and C. Proudhon, Oncogene, 2020, 39, 2987–2995 CrossRef CAS PubMed
.
- D. Chirizzi, M. Conte, M. Feltracco, A. Dinoi, E. Gregoris, E. Barbaro, G. La Bella, G. Ciccarese, G. La Salandra, A. Gambaro and D. Contini, Environ. Int., 2021, 146, 106255 CrossRef CAS PubMed
.
- O. Ginn, L. Rocha-Melogno, A. Bivins, S. Lowry, M. Cardelino, D. Nichols, S. N. Tripathi, F. Soria, M. Andrade, M. Bergin, M. A. Deshusses and J. Brown, Environ. Sci. Technol., 2021, 55, 14758–14771 CrossRef CAS PubMed
.
- J. P. Nshimyimana, M. C. Cruz, S. Wuertz and J. R. Thompson, Water Res., 2019, 159, 192–202 CrossRef CAS PubMed
.
- A. Bivins, S. Lowry, S. Wankhede, R. Hajare, H. M. Murphy, M. Borchardt, P. Labhasetwar and J. Brown, Water Res., 2021, 201, 117301 CrossRef CAS PubMed
.
- J. A. Capobianco, M. Clark, A. Cariou, A. Leveau, S. Pierre, P. Fratamico, T. P. Strobaugh, Jr. and C. M. Armstrong, Int. J. Food Microbiol., 2020, 319, 108499 CrossRef CAS PubMed
.
- E. S. Noh, Y. J. Park, E. M. Kim, J. Y. Park, K. B. Shim, T.-J. Choi, K.-H. Kim and J.-H. Kang, Food Chem., 2019, 275, 638–643 CrossRef CAS PubMed
.
- C. N. Baroud, F. Gallaire and R. Dangla, Lab Chip, 2010, 10, 2032–2045 RSC
.
- L. Shang, Y. Cheng and Y. Zhao, Chem. Rev., 2017, 117, 7964–8040 CrossRef CAS PubMed
.
- P. Garstecki, M. J. Fuerstman, H. A. Stone and G. M. Whitesides, Lab Chip, 2006, 6, 437–446 RSC
.
- S. L. Anna, N. Bontoux and H. A. Stone, Appl. Phys. Lett., 2003, 82, 364–366 CrossRef CAS
.
- A. S. Utada, A. Fernandez-Nieves, H. A. Stone and D. A. Weitz, Phys. Rev. Lett., 2007, 99, 094502 CrossRef PubMed
.
- H. Geng, J. Feng, L. M. Stabryla and S. K. Cho, Lab Chip, 2017, 17, 1060–1068 RSC
.
- K. Wang, B. Li and W. Wu, Molecules, 2020, 25, 5646 CrossRef CAS PubMed
.
- T. Thorsen, R. W. Roberts, F. H. Arnold and S. R. Quake, Phys. Rev. Lett., 2001, 86, 4163–4166 CrossRef CAS PubMed
.
- P. Guillot and A. Colin, Phys. Rev. E: Stat., Nonlinear, Soft Matter Phys., 2005, 72, 066301 CrossRef PubMed
.
- M. De Menech, P. Garstecki, F. Jousse and H. A. Stone, J. Fluid Mech., 2008, 595, 141–161 CrossRef
.
- R. Lin, J. S. Fisher, M. G. Simon and A. P. Lee, Biomicrofluidics, 2012, 6, 024103 CrossRef PubMed
.
- Y. Ding, X. Casadevall i Solvas and A. deMello, Analyst, 2015, 140, 414–421 RSC
.
- J. H. González-Estefan, M. Gonidec, T. T. Vu, N. Daro and G. Chastanet, Adv. Mater. Technol., 2019, 4, 1900232 CrossRef
.
- S.-Y. Teh, R. Lin, L.-H. Hung and A. P. Lee, Lab Chip, 2008, 8, 198–220 RSC
.
- L. Yobas, S. Martens, W.-L. Ong and N. Ranganathan, Lab Chip, 2006, 6, 1073–1079 RSC
.
- B. Morin, Y. Liu, V. Alvarado and J. Oakey, Lab Chip, 2016, 16, 3074–3081 RSC
.
- L. He, D. J. Simpson and M. G. Gänzle, Food Microbiol., 2020, 90, 103466 CrossRef CAS PubMed
.
- C. Wei, C. Yu, S. Li, J. Meng, T. Li, J. Cheng and J. Li, Sens. Actuators, B, 2022, 132473 CrossRef CAS
.
- C. Cramer, P. Fischer and E. J. Windhab, Chem. Eng. Sci., 2004, 59, 3045–3058 CrossRef CAS
.
- S. Sugiura, M. Nakajima and M. Seki, Langmuir, 2002, 18, 5708–5712 CrossRef CAS
.
- M. L. Eggersdorfer, H. Seybold, A. Ofner, D. A. Weitz and A. R. Studart, Proc. Natl. Acad. Sci. U. S. A., 2018, 115, 9479–9484 CrossRef CAS PubMed
.
- R. Dangla, S. C. Kayi and C. N. Baroud, Proc. Natl. Acad. Sci. U. S. A., 2013, 110, 853–858 CrossRef CAS PubMed
.
- F. Schuler, M. Trotter, M. Geltman, F. Schwemmer, S. Wadle, E. Domínguez-Garrido, M. López, C. Cervera-Acedo, P. Santibáñez, F. von Stetten, R. Zengerle and N. Paust, Lab Chip, 2016, 16, 208–216 RSC
.
- J. Park, K. G. Lee, D. H. Han, J.-S. Lee, S. J. Lee and J.-K. Park, Biosens. Bioelectron., 2021, 181, 113159 CrossRef CAS PubMed
.
- W.-W. Liu, Y. Zhu, Y.-M. Feng, J. Fang and Q. Fang, Anal. Chem., 2016, 89, 822–829 CrossRef PubMed
.
- Z. He, J. Wang, B. J. Fike, X. Li, C. Li, B. L. Mendis and P. Li, Biosens. Bioelectron., 2021, 191, 113458 CrossRef CAS PubMed
.
- N. Scoutaris, M. R. Alexander, P. R. Gellert and C. J. Roberts, J. Controlled Release, 2011, 156, 179–185 CrossRef CAS PubMed
.
- P. Calvert, Chem. Mater., 2001, 13, 3299–3305 CrossRef CAS
.
- F. Chen, Y. Zhang, Y. Nakagawa, H. Zeng, C. Luo, H. Nakajima, K. Uchiyama and J.-M. Lin, Talanta, 2013, 107, 111–117 CrossRef CAS PubMed
.
- F. Chen, L. Lin, J. Zhang, Z. He, K. Uchiyama and J.-M. Lin, Anal. Chem., 2016, 88, 4354–4360 CrossRef CAS PubMed
.
- H. Li, J. Liu, K. Li and Y. Liu, Sens. Actuators, A, 2019, 297, 111552 CrossRef CAS
.
- W. Zhang, N. Li, D. Koga, Y. Zhang, H. Zeng, H. Nakajima, J.-M. Lin and K. Uchiyama, Anal. Chem., 2018, 90, 5329–5334 CrossRef CAS PubMed
.
- S. Haeberle, R. Zengerle and J. Ducrée, Microfluid. Nanofluid., 2007, 3, 65–75 CrossRef
.
- Z. Chen, P. Liao, F. Zhang, M. Jiang, Y. Zhu and Y. Huang, Lab Chip, 2017, 17, 235–240 RSC
.
- X. Rui, S. Song, W. Wang and J. Zhou, Biomicrofluidics, 2020, 14, 061503 CrossRef CAS PubMed
.
- P.-C. Chen and C.-M. Tsai, Sens. Actuators, B, 2017, 259, 1123–1132 CrossRef
.
- A. D. Ruvalcaba-Cardenas, P. Thurgood, S. Chen, K. Khoshmanesh and F. J. Tovar-Lopez, ACS Appl. Mater. Interfaces, 2019, 11, 39283–39291 CrossRef CAS PubMed
.
- H. Li, R. Shen, C. Dong, T. Chen, Y. Jia, P.-I. Mak and R. P. Martins, Lab Chip, 2020, 20, 3709–3719 RSC
.
- M. Latikka, M. Backholm, A. Baidya, A. Ballesio, A. Serve, G. Beaune, J. V. I. Timonen, T. Pradeep and R. H. A. Ras, Adv. Sci., 2020, 7, 2000359 CrossRef CAS PubMed
.
- M. R. Hassan, J. Zhang and C. Wang, Colloid Interface Sci. Commun., 2021, 40, 100333 CrossRef CAS
.
- M. R. Hassan, J. Zhang and C. Wang, Langmuir, 2021, 37, 5823–5837 CrossRef CAS PubMed
.
- C. Sun, L. Liu, H. N. Vasudevan, K.-C. Chang and A. R. Abate, Anal. Chem., 2021, 93, 9974–9979 CrossRef CAS PubMed
.
- M. N. Hatori, S. C. Kim and A. R. Abate, Anal. Chem., 2018, 90, 9813–9820 CrossRef CAS PubMed
.
- Y. Fuchiwaki and H. Nagai, Sensors, 2014, 14, 20235–20244 CrossRef PubMed
.
- A. Harandi and T. Farquhar, J. Micromech. Microeng., 2014, 24, 115009 CrossRef
.
- H.-Y. Tseng, V. Adamik, J. Parsons, S.-S. Lan, S. Malfesi, J. Lum, L. Shannon and B. Gray, Sens. Actuators, B, 2014, 204, 459–466 CrossRef CAS
.
- M. U. Kopp, A. J. D. Mello and A. Manz, Science, 1998, 280, 1046–1048 CrossRef CAS PubMed
.
- H. Tachibana, M. Saito, K. Tsuji, K. Yamanaka, L. Q. Hoa and E. Tamiya, Sens. Actuators, B, 2015, 206, 303–310 CrossRef CAS
.
- B. Shu, C. Zhang and D. Xing, Anal. Chim. Acta, 2014, 826, 51–60 CrossRef CAS PubMed
.
- Y. Schaerli, R. C. Wootton, T. Robinson, V. Stein, C. Dunsby, M. A. A. Neil, P. M. W. French, A. J. deMello, C. Abell and F. Hollfelder, Anal. Chem., 2009, 81, 302–306 CrossRef CAS PubMed
.
- M. Madadelahi, E. Ghazimirsaeed and A. Shamloo, Anal. Chim. Acta, 2019, 1068, 28–40 CrossRef CAS PubMed
.
- E. K. Wheeler, C. A. Hara, J. Frank, J. Deotte, S. B. Hall, W. Benett, C. Spadaccini and N. R. Beer, Analyst, 2011, 136, 3707–3712 RSC
.
- A. C. Hatch, J. S. Fisher, A. R. Tovar, A. T. Hsieh, R. Lin, S. L. Pentoney, D. L. Yang and A. P. Lee, Lab Chip, 2011, 11, 3838–3845 RSC
.
- J. Shen, J. Zheng, Z. Li, Y. Liu, F. Jing, X. Wan, Y. Yamaguchi and S. Zhuang, Lab Chip, 2021, 21, 3742–3747 RSC
.
- B. P. Cahill, R. Land, T. Nacke, M. Min and D. Beckmann, Sens. Actuators, B, 2011, 159, 286–293 CrossRef CAS
.
- N. Majumdar, S. Banerjee, M. Pallas, T. Wessel and P. Hegerich, Sci. Rep., 2017, 7, 9617 CrossRef PubMed
.
- G. S. Yen, B. S. Fujimoto, T. Schneider, J. E. Kreutz and D. T. Chiu, J. Am. Chem. Soc., 2019, 141, 1515–1525 CrossRef CAS PubMed
.
- S. N. Dahotre, Y. M. Chang, A. M. Romanov and G. A. Kwong, Anal. Chem., 2019, 91, 2695–2700 CrossRef CAS PubMed
.
- Y. Sun, H. Tian, C. Liu, D. Yang and Z. Li, ACS Sens., 2018, 3, 1795–1801 CrossRef CAS PubMed
.
- H. Tian, Y. Sun, C. Liu, X. Duan, W. Tang and Z. Li, Anal. Chem., 2016, 88, 11384–11389 CrossRef CAS PubMed
.
- X. Xu, X. Ma, X. Zhang, G. Cao, Y. Tang, X. Deng, Z. Kang, M. Li and M. Guan, Clin. Chim. Acta, 2019, 491, 91–96 CrossRef CAS PubMed
.
- S. K. Goh, D. R. A. Cox, B. K. L. Wong, A. Musafer, T. Witkowski, H. Do, V. Muralidharan and A. Dobrovic, Clin. Chem., 2021, 67, 1201–1209 CrossRef PubMed
.
- J. Wang, N. Sun, Y.-T. Lee, Y. Ni, R. Koochekpour, Y. Zhu, H.-R. Tseng, S. Wang, L. Jiang and H. Zhu, J. Mater. Chem. B, 2020, 8, 5636–5644 RSC
.
- C.-Y. Ou, T. Vu, J. T. Grunwald, M. Toledano, J. Zimak, M. Toosky, B. Shen, J. A. Zell, E. Gratton, T. J. Abram and W. Zhao, Lab Chip, 2019, 19, 993–1005 RSC
.
- K. Chatfield-Reed, V. P. Roche and Q. Pan, Oral Oncol., 2021, 115, 104958 CrossRef CAS PubMed
.
- L. Galati, J.-D. Combes, F. Le Calvez-Kelm, S. McKay-Chopin, N. Forey, M. Ratel, J. McKay, T. Waterboer, L. Schroeder, G. Clifford, M. Tommasino and T. Gheit, Microbiol. Spectrum, 2022, 10, e0148021 CrossRef PubMed
.
- G. J. Hanna, C. J. Lau, U. Mahmood, J. G. Supplee, A. R. Mogili, R. I. Haddad, P. A. Jänne and C. P. Paweletz, Oral Oncol., 2019, 95, 120–126 CrossRef CAS PubMed
.
- M. Pichon, A. Gaymard, L. Josset, M. Valette, G. Millat, B. Lina and V. Escuret, Antiviral Res., 2017, 145, 160–167 CrossRef CAS PubMed
.
- C. Decraene, A. B. Silveira, F.-C. Bidard, A. Vallée, M. Michel, S. Melaabi, A. Vincent-Salomon, A. Saliou, A. Houy, M. Milder, O. Lantz, M. Ychou, M. G. Denis, J.-Y. Pierga, M.-H. Stern and C. Proudhon, Clin. Chem., 2018, 64, 317–328 CrossRef CAS PubMed
.
- J. A. Goss, D. J. Konczyk, P. J. Smits, H. P. W. Kozakewich, A. I. Alomari, A. Al-Ibraheemi, A. H. Taghinia, B. H. Dickie, D. M. Adams, S. J. Fishman, J. B. Mulliken, M. L. Warman and A. K. Greene, Angiogenesis, 2019, 22, 547–552 CrossRef CAS PubMed
.
- C. Callens, F.-C. Bidard, A. Ø. Curto-Taribo, O. Trabelsi-Grati, S. Melaabi, S. Delaloge, A.-C. Hardy-Bessard, T. Bachelot, F. Clatot, T. De La Motte Rouge, J.-L. Canon, L. Arnould, F. Andre, S. Marques, M.-H. Stern, J.-Y. Pierga, A. Vincent-Salomon, C. Benoist, E. Jeannot, F. Berger, I. Bieche and A. Pradines, Anal. Chem., 2022, 94, 6297–6303 CrossRef CAS PubMed
.
- S. Dulucq, F. E. Nicolini, D. Rea, P. Cony-Makhoul, A. Charbonnier, M. Escoffre-Barbe, V. Coiteux, P. Lenain, F. Rigal-Huguet, J. Liu, A. Guerci-Bresler, L. Legros, J.-C. Ianotto, M. Gardembas, P. Turlure, V. Dubruille, P. Rousselot, J. Martiniuc, H. Jardel, H. Johnson-Ansah, B. Joly, T. Henni, E. Cayssials, P. Zunic, M. G. Berger, B. Villemagne, F. Robbesyn, S. Morisset, F.-X. Mahon and G. Etienne, Haematologica, 2022, 107(12), 2859–2869 CrossRef CAS PubMed
.
- X. Bian, F. Jing, G. Li, X. Fan, C. Jia, H. Zhou, Q. Jin and J. Zhao, Biosens. Bioelectron., 2015, 74, 770–777 CrossRef CAS PubMed
.
- M. G. Basanisi, G. La Bella, G. Nobili, D. A. Raele, M. A. Cafiero, R. Coppola, A. M. Damato, R. Fraccalvieri, R. Sottili and G. La Salandra, Int. J. Food Microbiol., 2022, 366, 109583 CrossRef CAS PubMed
.
- M. Wang, J. Yang, Z. Gai, S. Huo, J. Zhu, J. Li, R. Wang, S. Xing, G. Shi, F. Shi and L. Zhang, Int. J. Food Microbiol., 2018, 266, 251–256 CrossRef CAS PubMed
.
- X. Lv, L. Wang, J. Zhang, X. He, L. Shi and L. Zhao, Food Microbiol., 2021, 99, 103831 CrossRef CAS PubMed
.
- D. Cristiano, M. F. Peruzy, M. Aponte, A. Mancusi, Y. T. R. Proroga, F. Capuano and N. Murru, Int. J. Food Microbiol., 2021, 354, 109321 CrossRef CAS PubMed
.
- B. Brooks, M. R. Olm, B. A. Firek, R. Baker, D. Geller-McGrath, S. R. Reimer, K. R. Soenjoyo, J. S. Yip, D. Dahan, B. C. Thomas, M. J. Morowitz and J. F. Banfield, Microbiome, 2018, 6, 112 CrossRef PubMed
.
- A. Bivins, S. Lowry, S. Wankhede, R. Hajare, H. M. Murphy, M. Borchardt, P. Labhasetwar and J. Brown, Water Res., 2021, 201, 117301 CrossRef CAS PubMed
.
- M. Antonello, R. Scutari, C. Lauricella, S. Renica, V. Motta, S. Torri, C. Russo, L. Gentile, V. Cento, L. Colagrossi, G. Mattana, L. R. Codecasa, C. Vismara, F. Scaglione, S. M. Veronese, E. Bonoldi, A. Bandera, A. Gori, E. Mazzola, C. F. Perno and C. Alteri, Front. Microbiol., 2021, 12, 727774 CrossRef PubMed
.
- J. K. Pearman, L. Biessy, J. D. Howarth, M. J. Vandergoes, A. Rees and S. A. Wood, Mol. Ecol. Resour., 2022, 22, 877–890 CrossRef CAS PubMed
.
- D. Wang, S. Wang, X. Du, Q. He, Y. Liu, Z. Wang, K. Feng, Y. Li and Y. Deng, Mol. Ecol. Resour., 2022, 1–12 Search PubMed
.
- T. J. Abram, H. Cherukury, C.-Y. Ou, T. Vu, M. Toledano, Y. Li, J. T. Grunwald, M. N. Toosky, D. F. Tifrea, A. Slepenkin, J. Chong, L. Kong, D. V. Del Pozo, K. T. La, L. Labanieh, J. Zimak, B. Shen, S. S. Huang, E. Gratton, E. M. Peterson and W. Zhao, Lab Chip, 2020, 20, 477–489 RSC
.
- B. Chen, Y. Xie, N. Zhang, W. Li, C. Liu, D. Li, S. Bian, Y. Jiang, Z. Yang, R. Li, Y. Feng, X. Zhang and D. Shi, Front. Microbiol., 2021, 12, 700008 CrossRef PubMed
.
- K. Zhu, B. Suttner, A. Pickering, K. T. Konstantinidis and J. Brown, Water Res., 2020, 183, 116085 CrossRef CAS PubMed
.
- P. Gao, C. Wu, J. Zhang, S. Wang, Y. Huang, Y. Dong, T. Liu, C. Ye, X. Xu and W. Xin, Front. Microbiol., 2022, 13, 860992 CrossRef PubMed
.
- K. J. Bosman, A. M. Wensing, A. E. Pijning, W. J. van Snippenberg, P. M. van Ham, D. M. de Jong, A. I. Hoepelman and M. Nijhuis, J. Int. AIDS Soc., 2018, 21, e25185 CrossRef PubMed
.
- N. A. J. Cassidy, C. S. Fish, C. N. Levy, P. Roychoudhury, D. B. Reeves, S. M. Hughes, J. T. Schiffer, S. Benki-Nugent, G. John-Stewart, D. Wamalwa, K. R. Jerome, J. Overbaugh, F. Hladik and D. A. Lehman, iScience, 2022, 25, 103615 CrossRef CAS PubMed
.
- C. N. Levy, S. M. Hughes, P. Roychoudhury, D. B. Reeves, C. Amstuz, H. Zhu, M.-L. Huang, Y. Wei, M. E. Bull, N. A. J. Cassidy, J. McClure, L. M. Frenkel, M. Stone, S. Bakkour, E. R. Wonderlich, M. P. Busch, S. G. Deeks, J. T. Schiffer, R. W. Coombs, D. A. Lehman, K. R. Jerome and F. Hladik, Cell Rep. Med., 2021, 2, 100243 CrossRef CAS PubMed
.
- C. Gálvez, V. Urrea, J. Dalmau, M. Jimenez, B. Clotet, V. Monceaux, N. Huot, L. Leal, V. González-Soler, M. González-Cao, M. Müller-Trutwin, A. Sáez-Cirión, F. García, J. Blanco, J. Martinez-Picado and M. Salgado, EBioMedicine, 2020, 57, 102830 CrossRef PubMed
.
- K. Cassinari, E. Alessandri-Gradt, P. Chambon, F. Charbonnier, S. Gracias, L. Beaussire, K. Alexandre, N. Sarafan-Vasseur, C. Houdayer, M. Etienne, F. Caron, J. C. Plantier and T. Frebourg, Clin. Chem., 2021, 67, 736–741 CrossRef PubMed
.
- K. E. Graham, S. K. Loeb, M. K. Wolfe, D. Catoe, N. Sinnott-Armstrong, S. Kim, K. M. Yamahara, L. M. Sassoubre, L. M. Mendoza Grijalva, L. Roldan-Hernandez, K. Langenfeld, K. R. Wigginton and A. B. Boehm, Environ. Sci. Technol., 2021, 55, 488–498 CrossRef CAS PubMed
.
- J. A. Rothman, T. B. Loveless, J. Kapcia, 3rd, E. D. Adams, J. A. Steele, A. G. Zimmer-Faust, K. Langlois, D. Wanless, M. Griffith, L. Mao, J. Chokry, J. F. Griffith and K. L. Whiteson, Appl. Environ. Microbiol., 2021, 87, e0144821 CrossRef PubMed
.
- L. Heijnen, G. Elsinga, M. de Graaf, R. Molenkamp, M. P. G. Koopmans and G. Medema, Sci. Total Environ., 2021, 799, 149456 CrossRef CAS PubMed
.
- W. Ahmed, P. M. Bertsch, N. Angel, K. Bibby, A. Bivins, L. Dierens, J. Edson, J. Ehret, P. Gyawali, K. A. Hamilton, I. Hosegood, P. Hugenholtz, G. Jiang, M. Kitajima, H. T. Sichani, J. Shi, K. M. Shimko, S. L. Simpson, W. J. M. Smith, E. M. Symonds, K. V. Thomas, R. Verhagen, J. Zaugg and J. F. Mueller, J. Travel Med., 2020, 27(5), taaa116 CrossRef PubMed
.
- P. M. D'Aoust, E. Mercier, D. Montpetit, J.-J. Jia, I. Alexandrov, N. Neault, A. T. Baig, J. Mayne, X. Zhang, T. Alain, M.-A. Langlois, M. R. Servos, M. MacKenzie, D. Figeys, A. E. MacKenzie, T. E. Graber and R. Delatolla, Water Res., 2021, 188, 116560 CrossRef PubMed
.
- J. Chen, Z. Luo, L. Li, J. He, L. Li, J. Zhu, P. Wu and L. He, Lab Chip, 2018, 18, 412–421 RSC
.
- T. Xie, Y. Luo, P. Wang, L. Wu, X. Cui, B. Sun and G. Li, Anal. Chem., 2022, 94, 13223–13232 CrossRef CAS PubMed
.
- B. Xiao, R. Zhao, N. Wang, J. Zhang, X. Sun and A. Chen, TrAC, Trends Anal. Chem., 2023, 158, 116836 CrossRef CAS
.
- C. Wu, L. Liu, Z. Ye, J. Gong, P. Hao, J. Ping and Y. Ying, Anal. Chim. Acta, 2022, 1233, 340513 CrossRef CAS PubMed
.
- H. Yuan, Y. Chao and H. C. Shum, Small, 2020, 16, 1904469 CrossRef CAS PubMed
.
- F. Schuler, F. Schwemmer, M. Trotter, S. Wadle, R. Zengerle, F. von Stetten and N. Paust, Lab Chip, 2015, 15, 2759–2766 RSC
.
- J. Q. Cui, F. X. Liu, H. Park, K. W. Chan, T. Leung, B. Z. Tang and S. Yao, Biosens. Bioelectron., 2022, 202, 114019 CrossRef CAS PubMed
.
- M. Schulz, S. Calabrese, F. Hausladen, H. Wurm, D. Drossart, K. Stock, A. M. Sobieraj, F. Eichenseher, M. J. Loessner, M. Schmelcher, A. Gerhardts, U. Goetz, M. Handel, A. Serr, G. Haecker, J. Li, M. Specht, P. Koch, M. Meyer, P. Tepper, R. Rother, M. Jehle, S. Wadle, R. Zengerle, F. von Stetten, N. Paust and N. Borst, Lab Chip, 2020, 20, 2549–2561 RSC
.
- N. Zhang, C. Li, X. Dou, Y. Du and F. Tian, Crit. Rev. Anal. Chem., 2022, 52, 1969–1989 CrossRef CAS PubMed
.
|
This journal is © The Royal Society of Chemistry 2023 |