DOI:
10.1039/D3NR02662K
(Paper)
Nanoscale, 2023,
15, 13466-13472
Early-stage oral cancer diagnosis by artificial intelligence-based SERS using Ag NWs@ZIF core–shell nanochains†
Received
6th June 2023
, Accepted 24th July 2023
First published on 24th July 2023
Abstract
Surface-enhanced Raman spectroscopy (SERS) has great potential in the early diagnosis of diseases by detecting the changes of volatile biomarkers in exhaled breath, because of its high sensitivity, rich chemical molecular fingerprint information, and immunity to humidity. Here, an accurate diagnosis of oral cancer (OC) is demonstrated using artificial intelligence (AI)-based SERS of exhaled breath in plasmonic-metal organic framework (MOF) nanoparticles. These plasmonic-MOF nanoparticles were prepared using a zeolitic imidazolate framework coated on Ag nanowires (Ag NWs@ZIF), which offers Raman enhancement from the plasmonic nanowires and gas enrichment from the ZIF shells. Then, the core–shell nanochains of Ag NWs@ZIF prepared with 0.5 mL Ag NWs were selected to capture gaseous methanethiol, which is a tumor biomarker, from the exhalation of OC patients. The substrate was used to collect a total of 400 SERS spectra of exhaled breath of simulated healthy people and simulated OC patients. The artificial neural network (ANN) model in the AI algorithm was trained with these SERS spectra and could classify them with an accuracy of 99%. Notably, the model predicted OC with an area under the curve (AUC) of 0.996 for the simulated OC breath samples. This work suggests the great potential of the combination of breath analysis and AI as a method for the early-stage diagnosis of oral cancer.
Introduction
Oral cancer (OC) is the cause of a significant proportion of global cancer morbidity and mortality. At present, imaging, clinical and histopathological examinations are used to diagnose OC.1–4 Invasive tissue biopsy followed by histopathological examination is the gold standard for OC diagnosis. However, these methods are invasive, inconvenient, and patient unfriendly. Therefore, using exhaled breath for diagnosis is a promising non-invasive method. Gas chromatography-mass spectrometry (GC-MS) is the main method for detecting biomarkers in the exhaled breath of oral cancer patients.5 This method determines that volatile sulfur compounds (i.e. H2S, CH3SH and (CH3)2S) are ideal biomarkers for non-invasive screening of oral cancer. However, the analytical techniques generally require complex analytical procedures or time-consuming processes, such as preliminary enrichments or preconcentration procedures. Therefore, developing a simple, fast, non-invasive, and highly sensitive method for on-site screening and identification of volatile sulfur compounds to achieve efficient early diagnosis of oral cancer remains an urgent issue.
Surface-enhanced Raman spectroscopy (SERS) is considered a powerful spectroscopic analytical technology that shows high specificity and sensitivity in detecting molecules.6–11 The Raman enhancement in SERS mainly comes from the collective oscillation of free electrons at a metal surface excited by light, which are called surface plasmons (SPs). This collective oscillation reaches resonance at a suitable frequency of incident light, which could greatly confine light at the metal surface in a nanoscale region (hot spot).12 So far, a small amount of work has reported that SERS technology has been successfully applied to diagnose oral cancer from saliva of oral cancer patients based on highly active nanostructures such as gold nanoparticles,13 silver nanoparticles,14 Ag TiO2 SERS nanostructures15,16 and so on. However, there have been no reports on the use of SERS technology to diagnose oral cancer based on the biomarkers of exhaled gas from cancer patients. The method of fast and highly-sensitive SERS detection of aldehydes still needs to be expanded owing to the low number density and fast diffusion of aldehydes in exhaled breath. One of the most effective strategies used is the enrichment of the target molecules prior to analysis.
Metal–organic frameworks (MOFs), which are highly ordered porous crystalline materials consisting of metal cations or cluster nodes linked by organic ligands, have recently attracted considerable attention because of their intriguing features, such as tunable pore size, extremely high surface area, and versatile functionalities.17–19 The plasmonic-MOF nanostructures have unique optical properties and a porous organic MOF nanoshell, which is beneficial for improving the performance of a plasmonic-MOF nanostructure based SERS platform.20 At present, there are only a few reports on the SERS detection of aldehydes exhaled by cancer patients based on plasmonic-MOF nanostructures, such as lung cancer21–23 and gastric cancer.24 The progress of SERS research based on breath analysis indicates that SERS technology has great potential for the diagnosis of cancer.
However, to the best of our knowledge, the application of exhaled SERS to the discrimination of oral cancer has not yet been reported in the literature. Meanwhile, due to the complexity of the exhaled breath system, the interpretation and application of SERS spectra of exhaled breath often require the assistance of multivariate analysis and advanced learning models. In recent years, multivariate statistical methods and machine-learning technologies, including artificial intelligence (AI), have made identifying these SERS spectra more precise and easy.25–28 AI algorithms have shown superior performance in analyzing spectroscopic signals including Raman spectroscopy in complex samples including biological samples.29 AI algorithms are combined with SERS spectra to successfully identify certain cancer types, such as breast cancer,30 prostate cancer,31 lung cancer,32 and so on.33 Therefore, for clinical diagnosis of oral cancer, there is an urgent need to develop a biomarker platform that can intelligently detect oral cancer from the exhaled breath of patients using SERS technology.
Here, an accurate diagnosis of oral cancer (OC) is demonstrated using artificial intelligence (AI)-based SERS of exhaled breath in plasmonic-metal organic framework (MOF) nanoparticles. These plasmonic-MOF nanoparticles were prepared using a zeolitic imidazolate framework coated on Ag nanowires (Ag NWs@ZIF), which offers Raman enhancement from the plasmonic nanowires and gas enrichment from the ZIF shells. Then, the core–shell nanochains of Ag NWs@ZIF prepared with 0.5 mL Ag NWs were selected to capture gaseous methanethiol, which is a tumor biomarker, from the exhalation of OC patients. The substrate was used to collect a total of 400 SERS spectra of exhaled breath of simulated healthy people and simulated OC patients. Principal component analysis (PCA), partial least-squares discriminant analysis (PL-SDA) and artificial neural network (ANN) models in the AI algorithm were constructed here to train these SERS spectra. Notably, the ANN model could classify them with an accuracy of 99% and predict OC with an area under the curve (AUC) of 0.996 for the simulated OC breath samples.
Experiments and methods
Fabrication of the Ag NWs@ZIF core–shell nanochains
The Ag NWs@ZIF core–shell nanochains were prepared by in situ growth of ZIF on the as-synthesized Ag NWs.34 To begin with, 25 mL of Zn(NO3)2 solution (25 mM) and 0.5 mL or 1 mL of synthetic Ag NWs (10 mg mL−1) were sequentially added to 25 mL of 2-methylimidazole solution (25 mM) while stirring. Then, the mixture was reacted at 30 °C for 2 h without stirring. After that, the residual reagents were removed by centrifugation and then washed twice with ethanol to obtain the Ag NWs@ZIF core–shell nanochains. Finally, the Ag NWs@ZIF core–shell nanochains were deposited on a Si film to dry for further use. Detailed methods for synthesizing Ag NWs@ZIF core–shell nanochain nanostructures are included in the ESI.† In addition, the ESI† includes gas adsorption, SERS characterization and specific experimental details of simulated oral cancer samples.
Artificial intelligence analysis
AI algorithms are used to analyze SERS data from simulated healthy and cancer exhalations. SERS data were preceded by preprocessing done using Labspec 5 software (Horiba; standard values: 4 degrees, 9 dimensions), including smoothing and baseline subtraction (function: polynomial type, 8 degrees). The simulated SERS spectra of healthy breath and OC breath were analyzed in the 400–3000 cm−1 range (4111 1). In the data of the SERS spectra, the simulated healthy samples are considered positive and the simulated OC samples are considered negative. The partial least-squares discriminant analysis (PLS) model, principal component analysis (PCA) model, and the artificial neural network (ANN) model are constructed. The ANN model consists of three parts: an input layer, a hidden layer, and an output layer. The input data width of the ANN model is composed of 4111 one-dimensional floating data. The ANN model parameters are set to randomly combine the hidden layers from 1 to 4, 1 to 50 neurons, and an RMSprop optimizer (lr = 3 × 10−4–3 × 10−3). At the same time, EarlyStopping (5 steps) and ModelCheckpoint methods are used to obtain the optimal model.35,36
Results and discussion
Synthesis and characterization of the Ag NWs@ZIF core–shell nanochains
The Ag NWs@ZIF core–shell nanochains were prepared by in situ growth of ZIF on the as-synthesized Ag NWs. The SERS substrate is prepared with a glass capillary loaded with ZIF-coated Ag nanowires (Ag NWs@ZIF), which offers Raman enhancement from the Ag nanowires and gas enrichment from the ZIF shells. The core–shell nanochains are confirmed by scanning electron microscopy (SEM) and transmission electron microscopy (TEM) images shown in Fig. 1. According to the SEM images of Ag NWs (Fig. 1a) and Ag NWs@ZIF (Fig. 1b), the length of the Ag nanowire is about 10 μm and the diameter is 100 nm ± 10 nm, whereas the thickness of the ZIF shell is about 200 ± 30 nm. The core–shell structure of the Ag NWs@ZIF composite is further observed by TEM. As shown in Fig. 2c, the Ag NWs penetrate through the ZIF-8 polyhedron and connect the adjacent particles together. In addition, the energy-dispersive X-ray spectroscopy (EDX) elemental mapping of Ag, Zn, C and N indicates the homogeneous coating/distribution of ZIF on the surface of Ag NWs (Fig. 1d). To demonstrate that ZIF shells can effectively adsorb gas molecules, the bare Ag NWs and the Ag NWs@ZIF composite are functionalized with methanethiol gas molecules to collect the SERS spectra. As shown in Fig. 1e, two characteristic peaks are observed at 684 cm−1 and 2981 cm−1, which can be assigned to υCS and υSH. In addition, according to the simulated SERS spectra of methanethiol shown in Fig. S1,† these two characteristic peaks are the characteristic peaks of methanethiol. It should be noted that the SERS intensity of methanethiol gas molecules is weaker in the control Ag platform without ZIF. These comparisons demonstrate the importance of creating a crack-free ZIF membrane to selectively capture and enrich target molecules over the encapsulated SERS active surfaces. The concentration changes of the gas passing through the Ag NWs and Ag NWs@ZIF are simulated, as shown in Fig. 1f. The numerous cavities of ZIF structures slow down the airflow, resulting in a longer reaction time between methanethiol gas molecules and Ag NW cores. However, gaseous methanethiol could easily flow away from smooth Ag NW surfaces. The reason is that the gaseous fluid flows through the porous ZIF shell, leading to cavity-diffusion effects.
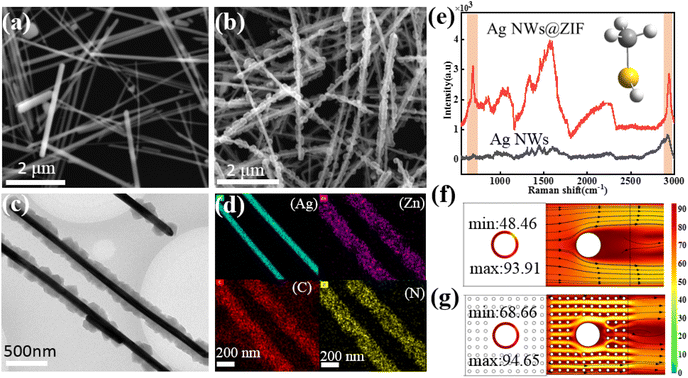 |
| Fig. 1 (a) SEM micrographs of the synthesized Ag nanoparticles and (b) Ag NWs@ZIF. Scale bar: 2 μm. (c) TEM micrographs of Ag NWs@ZIF. Scale bar: 500 nm. (d) The corresponding energy-dispersive X-ray spectroscopy (EDX) elemental mapping of Ag, Zn, C and N. Scale bar: 200 nm. (e) SERS spectra of (red) Ag NWs@ZIF and (black) Ag-only platforms for adsorbing gaseous methanethiol. The velocity distribution pattern for (f) Ag NW substrates and (g) Ag NWs@ZIF structures. | |
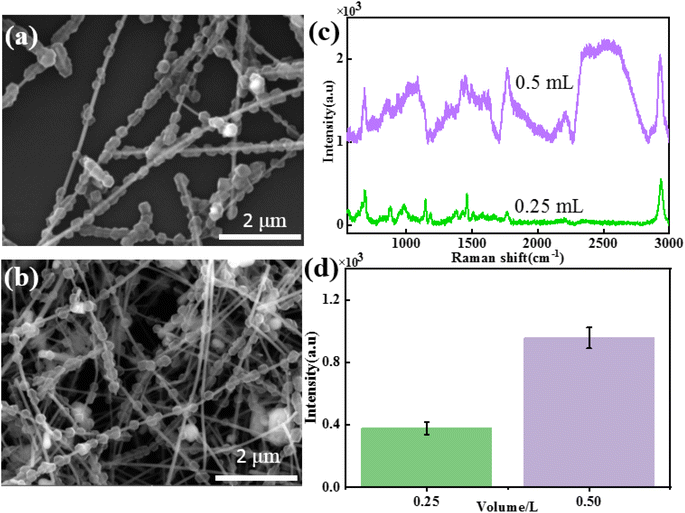 |
| Fig. 2 SEM images of the Ag NWs@ZIF core–shell nanochains prepared with volumes of (a) 0.25 mL and (b) 0.5 mL of Ag NWs. (c) SERS spectra of methanethiol gas molecules (10−4 M) collected on Ag NWs@ZIF substrates with different volumes of Ag NWs. (d) The corresponding histogram of the SERS intensity at 2931 cm−1. | |
Condition optimization for preparing Ag NWs@ZIF core–shell nanochains
To study the influence of the addition amount of Ag NWs on the morphology and SERS performance of Ag NWs@ZIF core–shell nanochains, Ag NW-dependent reactions are further carried out under the same conditions. The SEM images of core–shell structures, when the amounts of Ag NWs added to the system were 0.25 mL and 0.5 mL, respectively, are shown in Fig. 2a and b. It is found that when the amount of Ag NWs added is 0.25 mL, ZIF nanoparticles (500 ± 60 nm) are completely stacked on Ag NWs, with almost no bare Ag NWs exposed (Fig. 2a). As the amount of Ag NWs added increased, the particle size of ZIF nanoparticles on Ag NWs gradually decreased (0.5 mL: 300 ± 50 nm), and a discontinuous distribution was formed on the Ag NWs (Fig. 2b).
In order to further study the SERS properties of core–shell structures, methanethiol gas molecules are used to evaluate the SERS properties of these two Ag NWs@ZIF composites. Fig. 2c and d show the SERS spectra of methanethiol gas molecules (10−4 M) collected on the Ag NWs@ZIF substrate prepared with different amounts of Ag NWs and the corresponding Raman intensities of 2931 cm−1. The results show that with the increase of ZIF covering density and particle size, the SERS intensity of methanethiol gas molecules decreased, which may be related to the enrichment effect of the ZIF shell. Excessive ZIF loading inhibits the absorption of light by Ag NWs, resulting in a weakened SERS “hot spot” between Ag NWs.
According to the above results, the core–shell nanochains of Ag NWs@ZIF prepared with 0.5 mL of Ag NWs have better SERS properties, which are further selected as the SERS substrate to verify the capability of quantitative detection. The Ag NWs@ZIF nanocomposite is used to detect methanethiol gas molecules at different concentrations. As shown in Fig. 3a, the SERS spectra of methanethiol gas molecules with concentrations ranging from 1.0 × 10−7 to 1.0 × 10−3 M are acquired. The Raman signal at 2931 cm−1 gradually decreased with the decreasing methanethiol gas molecule concentration. The limit of detection (LOD) was 10−7 M. The relationship between the concentration of methanethiol gas molecules and the SERS intensity at 2931 cm−1 can be described by the equation: I2931 = 538.14log[C–SH] + 3874.57 with R2 (goodness of fit) = 0.95 (Fig. 3b). It is demonstrated that the Ag NWs@ZIF substrate exhibits a highly sensitive spectral response to methanethiol gas molecules. Meanwhile, Fig. 3c presents the SERS spectra of methanethiol gas molecules (10−6 M) collected from 10 random sites on the Ag NWs@ZIF substrate. Moreover, the relative standard deviation of the signal changes is less than 16.5% in 10 time measurements at the characteristic peak 2931 cm−1, which indicates that the SERS signals enhanced by the Ag NWs@ZIF SERS substrate demonstrate excellent reproducibility. Therefore, the Ag NWs@ZIF core–shell nanochains (0.5 mL) are practically applicable in breath analysis with good reproducibility and accuracy.
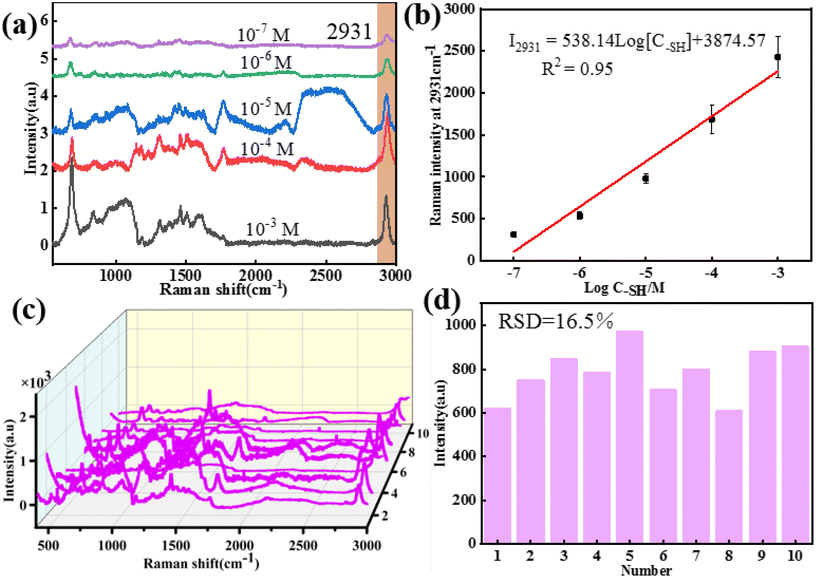 |
| Fig. 3 (a) SERS spectra of methanethiol gas molecules with the concentration ranging from 10−3 to 10−7 M for the core–shell nanochains of Ag NWs@ZIF prepared with 0.5 mL of Ag NWs. (b) Plots of SERS intensity versus logarithmic methanethiol gas molecule concentration at 2931 cm−1. (c) SERS spectra of methanethiol gas molecules (10−6 M) collected from 10 random sites on the Ag NWs@ZIF substrate. (d) Relative standard deviation for methanethiol gas molecules at the characteristic peak 2931 cm−1. | |
AI model for diagnosing oral cancer in exhaled breath via Ag NWs@ZIF
Exhaled breath has complex components and contains low levels of target molecules. The gaseous methanethiol in human breath is released as a result of tumor-specific tissue composition and metabolism and has therefore been recognized as a classic oral cancer biomarker. As a practical application, the SERS substrate is used for breath analysis of simulated breath samples. Simulated breath is supposed to have a composition similar to that of real breath samples. In order to simulate the actual detection environment, the test was carried out in a simulated oral cancer breath containing hydrocarbons, alcohols, organic acids, aromatic amines, aromatic compounds, ethers, etc. At present, AI algorithms are being widely used for the recognition of SERS spectra to detect cancer.33,37 The AI models trained using a supervised learning approach, where the input data are the SERS spectra and the output is the type of breath, are shown in Fig. 4a. Considering that different algorithms often lead to differences in prediction results, principal component analysis (PCA), partial least-squares discriminant analysis (PL-SDA) and artificial neural network (ANN) models are constructed. The 200 SERS spectra from four simulated healthy breath samples and the 200 SERS spectra from four simulated OC breath samples are collected. Before the AI model is constructed, SERS spectra derived from all three receptors are baseline corrected, normalized, and concatenated as a single SERS superprofile. The spectral data ranged from 400 to 3000 cm−1 and consisted of 4111 one-dimensional float data. For binary classification, the simulated healthy breath samples and the simulated OC breath samples are labeled with 0 and 1, respectively. As shown in Fig. 4b and c, the PCA and PLS models classify simulated breath samples with 92% and 85% accuracy when distinguishing simulated healthy breath and simulated OC breath profiles, respectively. However, ANN exhibits a superior accuracy of 99% for simulated breath sample classification. The detailed predicted training data and test data set results are shown in Fig. S2a and b.† This method achieves sufficiently high accuracy with an area under the curve (AUC) of the receiver operating characteristic (ROC) curves of 0.996 for simulated breath samples (Fig. S3†). Therefore, the combination of the AI algorithm and SERS method analysis has also demonstrated excellent ability in diagnosing oral cancer.
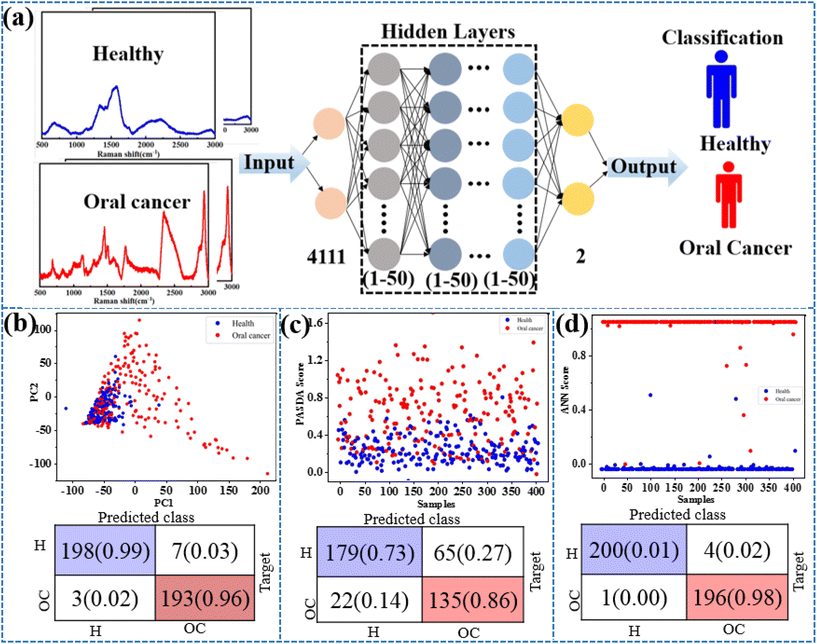 |
| Fig. 4 (a) The machine learning model is composed of several interconnected components. (b)–(d) A two-dimensional PCA, PLS, and ANN score plot derived from the classification of simulated healthy and OC breath profiles and the corresponding classification outcomes. | |
Conclusions
In conclusion, a breath analysis method based on an efficient SERS substrate – Ag NWs@ZIF is developed for the intelligent detection of oral cancer patients. The Ag NWs@ZIF substrate offers Raman enhancement from the plasmonic nanowires and gas enrichment from the ZIF shells. The Ag NWs@ZIF substrate synthesized with 0.5 mL of Ag NWs exhibited the highest SERS activity, reproducibility and long-term stability in detecting exhaled biomarkers of oral cancer. The substrate is used to collect a total of 400 SERS spectra of exhaled breath of simulated healthy people and simulated OC patients. The artificial neural network (ANN) model in the AI algorithm is trained with these SERS spectra and could classify them with an accuracy of 99%. Notably, the model predicted OC with an area under the curve (AUC) of 0.996 for the simulated OC breath samples. This work suggests the great potential of the combination of breath analysis and AI as a method for the early-stage diagnosis of oral cancer.
Author contributions
X. X., Y. Z. H., and L. W. designed the study and developed the concept. S. H. L. collected samples and data. W. R. Y. and J. J. Y. built the models and wrote the algorithm codes. X. X., L. Z. L., and Y. X. L. prepared the SERS substrate and collected the Raman data. X. X. and Z. X. C. designed and optimized experimental performance. X. X., Y. Z. H., and W. R. Y. analyzed data. Y. X. L. and X. X. conducted data visualization and made the figures. X. X. and Y. Z. H. wrote the paper. Y. Z. H., and L. W. conducted the funding acquisition.
Conflicts of interest
The authors declare no conflicts of interest.
Acknowledgements
This research was supported by the National Natural Science Foundation of China (11974067 and 62105044), the Chongqing Medical Scientific Research Project (Joint Project of Chongqing Health Commission and Science and Technology Bureau, No.2023QNXM018) and the Sharing Fund of Chongqing University's Large-scale Equipment.
References
- Y. Yang, M. Zhou, X. Zeng and C. Wang, BMC Oral Health, 2021, 21, 1–11 CrossRef CAS PubMed.
- M. W. Lingen, J. R. Kalmar, T. Karrison and P. M. Speight, Oral Oncol., 2008, 44, 10–22 CrossRef PubMed.
- J. Ferlay, I. Soerjomataram, R. Dikshit, S. Eser, C. Mathers, M. Rebelo, D. M. Parkin, D. Forman and F. Bray, Int. J. Cancer, 2015, 136, E359–E386 CrossRef CAS PubMed.
- C. M. L. Bollen and T. Beikler, Int. J. Oral Sci., 2012, 4, 55–63 CrossRef CAS PubMed.
- I.-J. Kwon, T.-Y. Jung, Y. Son, B. Kim, S.-M. Kim and J.-H. Lee, BMC Oral Health, 2022, 22, 268 CrossRef CAS PubMed.
- S. Y. Ding, J. Yi, J. F. Li, B. Ren, D. Y. Wu, R. Panneerselvam and Z. Q. Tian, Nat. Rev. Mater., 2016, 1, 16021 CrossRef CAS.
- C. Zong, M. Xu, L.-J. Xu, T. Wei, X. Ma, X.-S. Zheng, R. Hu and B. Ren, Chem. Rev., 2018, 118, 4946–4980 CrossRef CAS PubMed.
- R. Zhang, Y. Zhang, Z. C. Dong, S. Jiang, C. Zhang, L. G. Chen, L. Zhang, Y. Liao, J. Aizpurua, Y. Luo, J. L. Yang and J. G. Hou, Nature, 2013, 498, 82–86 CrossRef CAS PubMed.
- Q. Chen, L. Zhao, H. Liu, Q. Ding, C. Jia, S. Liao, N. Cheng, M. Yue and S. Yang, Biosens. Bioelectron., 2022, 202, 114004 CrossRef CAS PubMed.
- H. Zhao, W. Li, J. Li, Y. Sun, Q. Yang and M. Sun, Appl. Spectrosc. Rev., 2023, 1–30, DOI:10.1080/05704928.2023.2168688.
- L. Cui, R. Li, T. Mu, J. Wang, W. Zhang and M. Sun, Spectrochim. Acta, Part A, 2022, 264, 120283 CrossRef CAS PubMed.
- J. Langer, D. Jimenez de Aberasturi, J. Aizpurua, R. A. Alvarez-Puebla, B. Auguié, J. J. Baumberg, G. C. Bazan, S. E. J. Bell, A. Boisen, A. G. Brolo, J. Choo, D. Cialla-May, V. Deckert, L. Fabris, K. Faulds, F. J. García de Abajo, R. Goodacre, D. Graham, A. J. Haes, C. L. Haynes, C. Huck, T. Itoh, M. Käll, J. Kneipp, N. A. Kotov, H. Kuang, E. C. Le Ru, H. K. Lee, J.-F. Li, X. Y. Ling, S. A. Maier, T. Mayerhöfer, M. Moskovits, K. Murakoshi, J.-M. Nam, S. Nie, Y. Ozaki, I. Pastoriza-Santos, J. Perez-Juste, J. Popp, A. Pucci, S. Reich, B. Ren, G. C. Schatz, T. Shegai, S. Schlücker, L.-L. Tay, K. G. Thomas, Z.-Q. Tian, R. P. Van Duyne, T. Vo-Dinh, Y. Wang, K. A. Willets, C. Xu, H. Xu, Y. Xu, Y. S. Yamamoto, B. Zhao and L. M. Liz-Marzán, ACS Nano, 2019, 14, 28–117 CrossRef PubMed.
- H. Yang, Y. Xiang, X. Guo, Y. Wu, Y. Wen and H. Yang, Sens. Actuators, B, 2018, 271, 118–121 CrossRef CAS.
- J. M. Connolly, K. Davies, A. Kazakeviciute, A. M. Wheatley, P. Dockery, I. Keogh and M. Olivo, Nanomedicine, 2016, 12, 1593–1601 CrossRef CAS PubMed.
- C. M. Girish, S. Iyer, K. Thankappan, V. V. D. Rani, G. S. Gowd, D. Menon, S. Nair and M. Koyakutty, J. Mater. Chem. B, 2014, 2, 989–998 RSC.
- G. Chundayil Madathil, S. Iyer, K. Thankappan, G. S. Gowd, S. Nair and M. Koyakutty, Adv. Healthcare Mater., 2019, 8, 1801557 CrossRef PubMed.
- J. Li, Z. Liu, D. Tian, B. Li, L. Shao and Z. Lou, Nanoscale, 2022, 14, 5561–5568 RSC.
- H.-C. Zhou, J. R. Long and O. M. Yaghi, Chem. Rev., 2012, 112, 673–674 CrossRef CAS PubMed.
- H. Furukawa, K. E. Cordova, M. O’Keeffe and O. M. Yaghi, Science, 2013, 341, 1230444 CrossRef PubMed.
- L. B. T. Nguyen, Y. X. Leong, C. S. L. Koh, S. X. Leong, S. K. Boong, H. Y. F. Sim, G. C. Phan-Quang, I. Y. Phang and X. Y. Ling, Angew. Chem., Int. Ed., 2022, 61, e202207447 CAS.
- Z. Xia, D. Li and W. Deng, Anal. Chem., 2021, 93, 4924–4931 CrossRef CAS PubMed.
- A. Li, X. Qiao, K. Liu, W. Bai and T. Wang, Adv. Funct. Mater., 2022, 32, 2202805 CrossRef CAS.
- Y. Huang, T. Xie, K. Zou, Y. Gu, G. Yang, F. Zhang, L.-L. Qu and S. Yang, Nanoscale, 2021, 13, 13344–13352 RSC.
- L. Huang, Y. Zhu, C. Xu, Y. Cai, Y. Yi, K. Li, X. Ren, D. Jiang, Y. Ge, X. Liu, W. Sun, Q. Zhang and Y. Wang, ACS Sens., 2022, 7, 1439–1450 CrossRef CAS PubMed.
- H. Shin, B. H. Choi, O. Shim, J. Kim, Y. Park, S. K. Cho, H. K. Kim and Y. Choi, Nat. Commun., 2023, 14, 1644 CrossRef CAS PubMed.
- N. E. Dina, A. M. R. Gherman, V. Chiş, C. Sârbu, A. Wieser, D. Bauer and C. Haisch, Anal. Chem., 2018, 90, 2484–2492 CrossRef CAS PubMed.
- X. Chen, X. Li, J. Xie, H. Yang and A. Liu, Anal. Chim. Acta, 2022, 1191, 339296 CrossRef CAS PubMed.
- C. D. Bocsa, V. Moisoiu, A. Stefancu, L. F. Leopold, N. Leopold and D. Fodor, Nanomedicine, 2019, 20, 102012 CrossRef CAS PubMed.
- R. Di Santo, S. Romanò, A. Mazzini, S. Jovanović, G. Nocca, G. Campi, M. Papi, M. De Spirito, F. Di Giacinto and G. Ciasca, Nanomaterials, 2021, 11, 1476 CrossRef PubMed.
- Y. Xie, X. Su, Y. Wen, C. Zheng and M. Li, Nano Lett., 2022, 22, 7910–7918 CrossRef CAS PubMed.
- X. Chen, X. Li, H. Yang, J. Xie and A. Liu, Spectrochim. Acta, Part A, 2022, 267, 120571 CrossRef CAS PubMed.
- H. Shin, S. Oh, S. Hong, M. Kang, D. Kang, Y.-G. Ji, B. H. Choi, K.-W. Kang, H. Jeong, Y. Park, S. Hong, H. K. Kim and Y. Choi, ACS Nano, 2020, 14, 5435–5444 CrossRef CAS PubMed.
- Y. Chen, Y. Zhang, F. Pan, J. Liu, K. Wang, C. Zhang, S. Cheng, L. Lu, W. Zhang, Z. Zhang, X. Zhi, Q. Zhang, G. Alfranca, J. M. de la Fuente, D. Chen and D. Cui, ACS Nano, 2016, 10, 8169–8179 CrossRef CAS PubMed.
- J. Yang, M. Pan, X. Yang, K. Liu, Y. Song and S. Wang, Food Chem., 2022, 395, 133623 CrossRef CAS PubMed.
- W. Yu, X. Xie, L. Wang, Q. Lv, Y. Jiang, W. Zhang, C. Huang, L.-A. Wang and Y. Huang, ACS Appl. Nano Mater., 2022, 5, 15142–15149 CrossRef CAS.
- D. Zappi, M. M. Ramma, V. Scognamiglio, A. Antonacci, G. Varani and M. T. Giardi, Biosensors, 2020, 10, 176 CrossRef CAS PubMed.
- X. Lin, D. Lin, Y. Chen, J. Lin, S. Weng, J. Song and S. Feng, Adv. Funct. Mater., 2021, 31, 2103382 CrossRef CAS.
|
This journal is © The Royal Society of Chemistry 2023 |
Click here to see how this site uses Cookies. View our privacy policy here.