DOI:
10.1039/D2NA00608A
(Review Article)
Nanoscale Adv., 2023,
5, 538-570
Machine learning-augmented surface-enhanced spectroscopy toward next-generation molecular diagnostics†
Received
7th September 2022
, Accepted 6th November 2022
First published on 7th November 2022
Abstract
The world today is witnessing the significant role and huge demand for molecular detection and screening in healthcare and medical diagnosis, especially during the outbreak of COVID-19. Surface-enhanced spectroscopy techniques, including Surface-Enhanced Raman Scattering (SERS) and Infrared Absorption (SEIRA), provide lattice and molecular vibrational fingerprint information which is directly linked to the molecular constituents, chemical bonds, and configuration. These properties make them an unambiguous, nondestructive, and label-free toolkit for molecular diagnostics and screening. However, new issues in molecular diagnostics, such as increasing molecular species, faster spread of viruses, and higher requirements for detection accuracy and sensitivity, have brought great challenges to detection technology. Advancements in artificial intelligence and machine learning (ML) techniques show promising potential in empowering SERS and SEIRA with rapid analysis and automatic data processing to jointly tackle the challenge. This review introduces the combination of ML and SERS/SEIRA by investigating how ML algorithms can be beneficial to SERS/SEIRA, discussing the general process of combining ML and SEIRA/SERS, highlighting the molecular diagnostics and screening applications based on ML-combined SEIRA/SERS, and providing perspectives on the future development of ML-integrated SEIRA/SERS. In general, this review offers comprehensive knowledge about the recent advances and the future outlook regarding ML-integrated SEIRA/SERS for molecular diagnostics and screening.
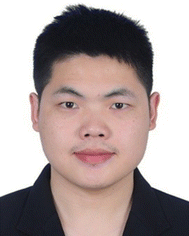 Hong Zhou | Dr Hong Zhou received his B.Eng. degree in Mechanical Engineering from Central South University, China, and his PhD degree in Instrument Science and Technology from Chongqing University, China. He is now a Research Fellow at the Department of Electrical & Computer Engineering, NUS. His research interests are focused on mid-IR metamaterials and nanophotonics for sensing applications. |
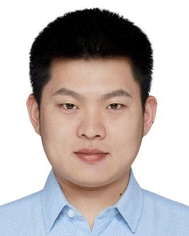 Liangge Xu | Liangge Xu is pursuing his PhD at Harbin Institute of Technology from 2018 to the present. His current research mainly focuses on the optical film and photoelectric film in the infrared region and introduces them into more advanced sensor devices. |
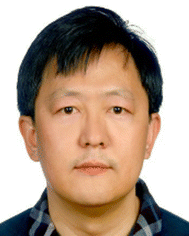 Jiaqi Zhu | Prof. Jiaqi Zhu received his PhD degree from the Harbin Institute of Technology, Harbin, China, in 2007. He is the Director of the Group for Infrared Films and Crystals, Yangtze River Scholar Chair Professor, Outstanding Young Investigator Award, and Leading Talent of China. His research focuses on the design and fabrication of advanced infrared optical films and devices. |
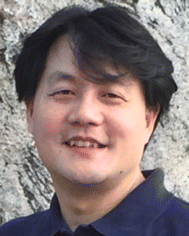 Chengkuo Lee | Prof. Chengkuo Lee received the M.Sc. degree from the Department of Materials Science and Engineer, National Tsing Hua University, Hsinchu, Taiwan, in 1991, M.Sc. degree from the Department of Industrial and System Engineering, the State University of New Jersey, Brunswick, NJ, USA, in 1993, and the PhD degree from the Department of Precision Engineering, the University of Tokyo, Tokyo, Japan, in 1996. He is the Director of Center for Intelligent Sensors and MEMS at National University of Singapore, Singapore. His research interest includes energy harvesting, nanophotonics, and MEMS. |
1. Introduction
Surface-enhanced spectroscopy is a significant spectroscopic technique and could be classified as Surface-Enhanced Raman Scattering (SERS) spectroscopy,1 surface-enhanced infrared absorption spectroscopy (SEIRA) spectroscopy,2 and surface-enhanced fluorescence (SEF).3 SERS and SEIRA provide lattice and molecular vibrational fingerprint information, respectively, which is directly related to the molecular constituents, chemical bonds, and configuration.4–8 This correlation makes them powerful analytical tools for unambiguous, nondestructive, and label-free detection of substances in biology, medicine, electrochemistry, catalysis, materials science, etc.9 Since the intrinsic mechanisms of SEF and the other two are quite different, we will not discuss them in this review. The discovery of SERS stems from the unprecedentedly intense Raman spectra of molecules adsorbed on specially prepared roughened silver electrodes, as demonstrated by Fleischmann et al. in 1974.10 This stronger-than-expected spectrum was further investigated and carefully calculated as a million-fold enhancement by Van Duyne et al. in 1977 and then it was dubbed the SERS effect.11 Serval years later (in 1980), a similar phenomenon in infrared spectroscopy was observed by Hartstein et al. using films of randomly distributed silver nanoparticles in the attenuated-total-reflection (ATR) setup,12 which is known as SEIRA. Since the underlying mechanism of SEIRA and SERS is the interaction between molecules and plasmonic resonance, their excitation is geometry-dependent and their substrate dimensions are on the micro-/nano scale. Along with the rapid development of nanofabrication and nano-synthesis techniques in recent years, SEIRA and SERS technologies have advanced rapidly and various types of substrates and applications were demonstrated, such as single-molecule (SM) SERS,13 Tip-Enhanced Raman Spectroscopy (TERS),14 scattering-type scanning near-field optical microscope (s-SNOM),15 shell-isolated nanoparticle-enhanced Raman spectroscopy (SHINERS),9 resonant SEIRA,16 graphene-based SEIRA,17 and more. Nonetheless, their technical limitations have become increasingly prominent in the process of technological evolution and application.
First of all, it is challenging and time-consuming to deal with the huge volumes of spectral data in many applications such as the detection of multiple biomarkers and viruses and the monitoring of biological responses in multiple processes. Especially, the volume of spectral data is inevitably ever-increasing with the development of sophisticated SERS/SEIRA-based applications.1 Furthermore, the processing of each set of spectral data is also complex and time-consuming, which generally includes normalization, baseline calibration, and feature signal extraction. Another issue for SERS and SEIRA is the spectral overlapping of various molecules, which greatly limits the application scope of SERS and SEIRA. For instance, almost all kinds of protein molecules suffer from IR spectral overlapping between 1600 and 1700 cm−1, where the amide I and amide II vibration bands are located (proteins are special types of amides).18 Third, anomalies and artifacts are critical challenges for SERS and SEIRA, which restricts SERS and SEIRA to low accuracy, poor stability and reliability. Factors that cause anomalies and artifacts are various, ranging from instrumental effects, sample effects, to contamination in sampling procedures.19 More specifically, the instrumental effects include shifts in the wavenumber scale, multi-passing errors, detector effects, noise effects, dark noise, etc. The sample effects contain sample heating, fluorescence interference in Raman spectra, and matrix absorption. The contamination in sampling procedures includes ambient lighting, air, sample support surfaces, sample containers, and sample movement. Finally, the manual design of surface-enhanced spectroscopy substrates is time-consuming and inefficient and various analytes require customized structure designs to ensure the matching of molecular vibrations and plasmonic resonances, which is particularly prominent in SEIRA spectroscopy.20 Therefore, the automatic design of substrates is highly desirable and helps to facilitate the practical application of the technology. In general, in response to the above-mentioned bottlenecks, researchers are looking for breakthroughs in other aspects.
A potential solution to the bottleneck is an algorithm analysis technique, which was widely employed in early chemometrics.21 Specifically, it is used to analyze and mine chemical data and to design optimal experiments or choose measurement procedures. The well-known algorithms include principal component analysis (PCA), principal component regression (PCR), multiple linear regression (MLR), linear discriminant analysis (LDA), and more.22,23 However, the implementation of these algorithms requires the support of high-performance computing. Therefore, the addressing of these issues can not only rely on algorithms, but also requires the assistance of computers. Artificial intelligence (AI) is a part of computer science that tries to enable machines to perform tasks that typically require human intelligence. It utilizes the computing power of machines and intelligent algorithms to free people from complicated data analysis.24 Therefore, AI could provide novel strategies for overcoming the challenges faced by surface-enhanced spectroscopy, which also makes common SEIRA and SERS intelligent tools and analysis platforms. One of the basic requirements for AI is learning, and it is generally agreed by most researchers that there is no AI without learning.25 Therefore, machine learning (ML) is one of the most rapidly developing and significant subfields of AI research.26–33 At the very beginning of ML development (1950s–1960s), there are three major branches, that is, symbolic learning proposed by Hunt et al., statistical methods by Nilsson, and neural networks by Rosenblatt.34 Nowadays, these branches develop advanced methods and can be divided into four categories, that is, classification, regression, clustering, and dimensionality reduction.35–44 The algorithms for these branches include support vector machine (SVM), κ-nearest neighbor (κNN), decision tree (DT), convolutional neural network (CNN), k-means, PCA, etc.45–50 These algorithms have been well employed in SEIRA and SERS. The development path of AI-augmented surface-enhanced spectroscopy is shown in Fig. 1.51–60 During the exploration, the researchers have demonstrated many advantages of ML-augmented SEIRA and SERS over conventional approaches. ML offers the inimitable possibility of solving pressing challenges in the field of surface-enhanced spectroscopy and is receiving increasing attention.
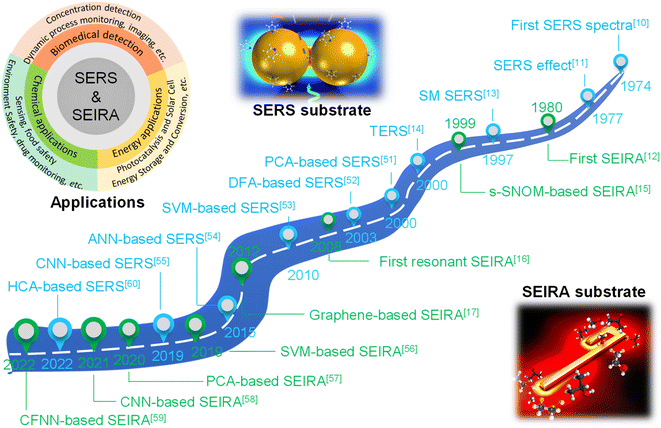 |
| Fig. 1 Development path of AI-augmented surface-enhanced Raman and infrared spectroscopy. The common SERS substrate is nanoparticles with dimensions ranging from tens to hundreds of nanometers61 and the common SEIRA substrate is nanoantennas with micro-level dimensions. SEIRA and SERS are widely used in biochemical and energy fields. SERS: Surface-Enhanced Raman Scattering; SEIRA: surface-enhanced infrared absorption; SM SERS: single-molecule SERS; s-SNOM: scattering-type scanning near-field optical microscope; TERS: tip-enhanced Raman spectroscopy; PCA: principal component analysis; DFA: deterministic finite automaton; SVM: support vector machine; ANN: artificial neural network; CNN: convolutional neural network; CFNN: cascade forward neural network; HCA: hierarchical cluster analysis. Reproduced with permission.61 Copyright 2020 Royal Society of Chemistry. | |
As noted above, although ML and surface-enhanced spectroscopy are complementary in terms of technical characteristics, ML-augmented SEIRA and SERS are still in their infancy and their efficient combination is challenging. The landmark work of many researchers has greatly advanced the field, but their technical approaches are diverse and their perspectives are somewhat distinct. Therefore, the purpose of this review is to summarize some of the masterpieces in the technological evolution and to provide a timely discussion and perspectives of ML-augmented SEIRA and SERS and their applications in molecular diagnostics, the most representative and active field according to our literature survey. Firstly, we introduce the concept of ML-augmented surface-enhanced spectroscopy and discuss the benefit of ML for SEIRA and SERS. Then, we present the development and application of ML-augmented SEIRA and SERS from the perspective of substrate design, data processing, and decision-making. Finally, we conclude and look ahead to the future of advanced technologies.
2. Concept of machine learning enhancing SERS and SEIRA
2.1 Benefits of machine learning for SERS and SEIRA
The principles of SERS and SEIRA include electromagnetic field enhancement and chemical effect.62 The underlying mechanisms of electromagnetic field enhancement are mainly about the interaction of molecules and plasmons excited in a SERS/SEIRA substrate.63–68 The detailed theoretically analysis of SERS and SEIRA can be found in Note S1 (ESI†). Fig. 2a–d is the schematic of electromagnetic field enhancement for SERS and SEIRA. Another mechanism for SERS and SEIRA is the chemical effect. It refers to contributions that are associated with the transfer of electrons between adsorbed molecules and the SERS/SEIRA substrate. It can be achieved by electron transfer in the ground or excited states of the molecule-metal system. The commonly used SERS substrates include anisotropic nanoparticles, core–shell nanoparticles, single-nanoparticle dimers, self-assembled nanoparticles, nanostructure based on hole-mask colloidal lithography, nanopillars, nanostructured dielectrics and hybrids, and so on.69–72 The SEIRA substrates are sub-wavelength nanoantennas or metamaterials which are artificial sub-wavelength structures with extraordinary physical properties distinct from the intrinsic properties of naturally available materials.73–85 The nanofabrication technologies for preparing SEIRA/SERS substrates include chemical preparation methods, photolithography, electron beam lithography, magnetron sputtering, electron beam evaporation, and more.86–107 The widely used theory for modeling SERS/SEIRA includes perturbation theory,108 temporal coupled-mode theory,109,110 coupled harmonic oscillator theory,111 and so on. As mentioned earlier, machine learning is complementary to SERS/SEIRA and offers unparalleled possibilities for solving pressing challenges related to spectral artifacts, overlapping, and huge volumes of spectral data (Fig. 2e). Based on the current reported work, the benefits of machine learning for SERS and SEIRA can be summarized as follows.45,112–125
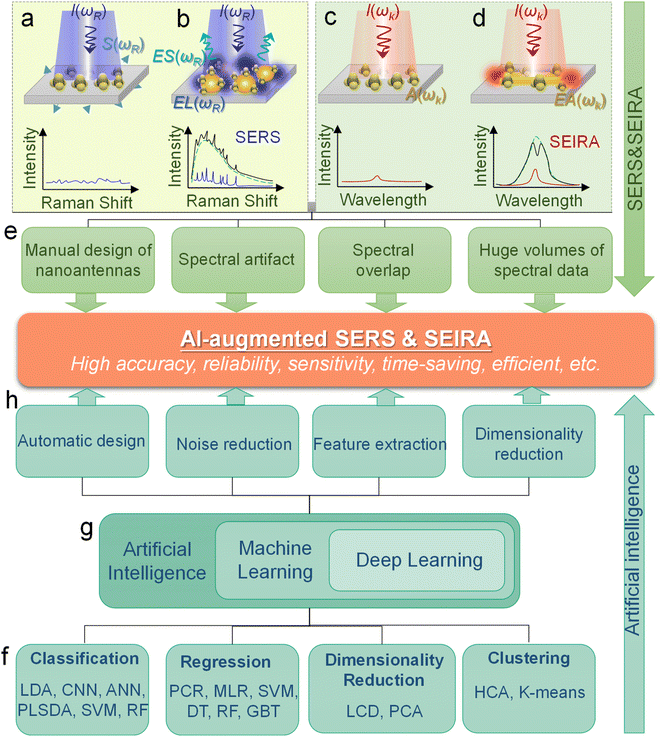 |
| Fig. 2 Concept of machine learning-enhanced SERS and SEIRA. (a) Conventional Raman scattering Sωr and Raman spectrum with incident radiation Rω0. (b) Surface-enhanced Raman scattering using nanoparticle substrates. Enhanced local field (ELω0) is achieved by utilizing the localized surface plasmon polariton (LSP) of nanoparticles, and then the excitation and radiation efficiency of Raman scattering is improved by the interaction with LSP, which is expressed as ESωr. (c) Conventional IR absorption and spectrum Aω0 of molecules with incident IR radiation Iω0. (d) Surface-enhanced infrared absorption. Enhanced absorption of molecules (EAω0) is realized by the near-field coupling of LSP and molecules. (e) Challenges and issues of SEIRA and SERS. (f) Model categories of machine learning for SERS and SEIRA. (g) Relationship between AI, ML, and DL. (h) Benefits of ML brought to SERS and SEIRA. | |
Machine learning algorithms enable the automated design of SERS/SEIRA substrates to avoid time-consuming and onerous design processes. Taking SEIRA's antenna design as an example,126 its first step is to analyze the infrared spectrum of the analyte molecule and obtain the position of the molecular vibration. Then, an appropriate antenna structure is chosen to excite plasmonic resonances that match the molecular vibrational frequencies. There is a lot of repetitive work involved here. First, the selection of the structural shape is a critical and continuous optimization process. It requires designers to utilize simulation software (such as FDTD solutions) to compare the figures of merit of different shapes, such as sensitivity, enhancement factor, bandwidth, and so on. While design experience can reduce the number of iterations in the process, it could lead to design deviations due to personnel differences. Another iterative work at this stage is to match antenna resonances and molecular vibrations via the tuning of antenna dimensions, since zero detuning allows for more efficient molecule-plasmon coupling. Additionally, a multiband design is necessary to enhance SEIRA's ability to identify molecules if the detection target is a specific molecule in the mixture. Furthermore, the limitations of nanofabrication are also issues to be considered at the device design stage. A good design considering all of these factors takes a lot of effort and time. Fortunately, these time-consuming and repetitive tasks are easy and efficient for ML-assisted design systems. For example, genetic algorithms were used to automatically generate highly sensitive antenna structures that match well with molecular peaks.126 Furthermore, the number of iterations and machine learning efficiency can be improved by employing the physical constraints of causality to directly learn the response functions of antennas.127 In common deep neural networks, the function in the hidden layers to output predictions is unknown like a black box. It works but it is unknown how or why it works. By incorporating physical knowledge into hidden layers, the network is able to learn the physical relationships between the input physical parameters. Automatic learning of the underlying physics can improve the accuracy of prediction results.
Machine learning algorithm also helps SERS/SEIRA reduce anomalies and artifacts. As mentioned earlier, anomalies and artifacts are common in SERS/SEIRA, mainly caused by instrumental effects, sample effects, to contamination in sampling procedures. The presence of anomalies and artifacts limits SERS/SEIRA to high noise, low accuracy and resolution, poor stability and reliability. Machine learning can address these issues in the following ways.128,129 Well-trained ML model can identify and reduce noise by the difference with the sample signal in changing frequency and spectral location. More specifically, the change time of the sample is typically on the second or even minute level, while some noise signals such as background noise from the instrument are usually high frequency. The position of the signal of some contaminants in the substrate in the spectrum could also be distinct from the sample. Based on these differences, a well-trained ML model can distinguish and attenuate noise. Additionally, ML can identify the “right” signals and correct anomalies to improve the accuracy, resolution, and stability of SERS/SEIRA. More specifically, during the training of the ML model, the correct signal is used and “remembered” by the model via extracting features.130 When anomalous and artifact signals are fed into a trained model, its features will be outside the normal range. Models can ignore or even correct anomalies and artifacts depending on what they were trained on.131 These improvements are greatly crucial for SERS/SEIRA when it comes to on-site applications.
Machine learning algorithms are beneficial for solving problems about spectral overlap in SERS/SEIRA. Spectral overlap of analytes is a common challenge for spectral detection methods, and for SERS and SEIRA, it impairs the identification capability and limits the scope of application. Fortunately, the spectra of different analytes partially overlap, which presents an opportunity for ML to address this issue.117,118 By extracting the complete spectral signature of an analyte, ML can quickly and automatically identify and classify analytes. For example, nucleotides and sucrose are spectrally overlapping around 1000–1200 cm−1 due to their C–O stretching vibrations. However, there is no spectral overlap around 1400–1600 cm−1. Nucleotides are infrared active in this region due to the C–N stretching vibration, while sucrose lacks it. Therefore, well-trained ML algorithms taking advantage of this distinction can accurately identify and classify them in mixed analytes.58
Finally, machine learning algorithms assist SERS and SEIRA to analyze and process data quickly, directly, and automatically, and relieve the pressure of massive spectral data through dimensionality reduction. In the real-time monitoring application of SERS and SEIRA, the output data is three-dimensional information, including spectral intensity, wavelength, and time. Additionally, if multiple analytes are targeted, the information becomes four-dimensional (category information added).58,128 All these factors lead to the generation of a huge amount of spectral data. Fast and accurate analysis and processing of these data is a major challenge for SERS and SEIRA. Some ML algorithms such as PCR can reduce the dimension of information on the premise of preserving information features, thereby reducing the amount of data, decreasing data complexity, simplifying data processing, and quickly outputting test results.132 It can be foreseen that the increase in the amount of data is inevitable due to the technological development of SERS and SEIRA and the emergence of new applications. Therefore, the dimensionality reduction of spectral data by using ML algorithms is of great help to SERS and SEIRA.
2.2 General process for combining ML and SEIRA/SERS
Machine learning is a powerful analytical tool that is widely used in the Internet of things (IoT),133–142 wearable sensors,143–158 human–machine interaction (HMI),159–169 and smart sensing.154,155,170–184 According to the function of the algorithm and the problem it solves, machine learning can be divided into classification, regression, clustering, and dimensionality reduction, as shown in Fig. 2f. From the perspective of learning methods, machine learning is inspired by human learning mechanisms, and can be divided into supervised, unsupervised, and semi-supervised learning (Fig. 3a). Supervised learning is the most common way.185 Supervised ML algorithms first train a model with known labeled or annotated input data (learning set) and responses (output), and then use the trained model to predict the response on new input data. This approach provides the strictest framework and strongest guarantees. Due to the relevance of the learning set and new data, the accuracy of the response prediction of the model in supervised learning depends on the training of the learning set. Common supervised ML algorithms include linear or logistic regression, decision trees and random forests, support vector machines, convolutional neural networks, and recurrent neural networks. When data is not annotated or labelled, the ML is called unsupervised learning. Most unsupervised tasks are related to probability density estimation. Representative unsupervised ML algorithms include autoencoders, dimensionality reduction (e.g., principal component analysis), and clustering (e.g., k-means).186 So far, the use of unsupervised ML has been more limited than supervised learning. Semi-supervised ML is a hybrid configuration between supervised and unsupervised.187 In semi-supervised ML, the expected output of the learning set is partially known. One representative case is that semi-supervised ML is used to cluster and then label the data space to assist a supervised algorithm in finding the decision boundary.188 Some ML algorithms that are relevant to SERS/SEIRA and have great research potential are listed in Note S2 (ESI†).
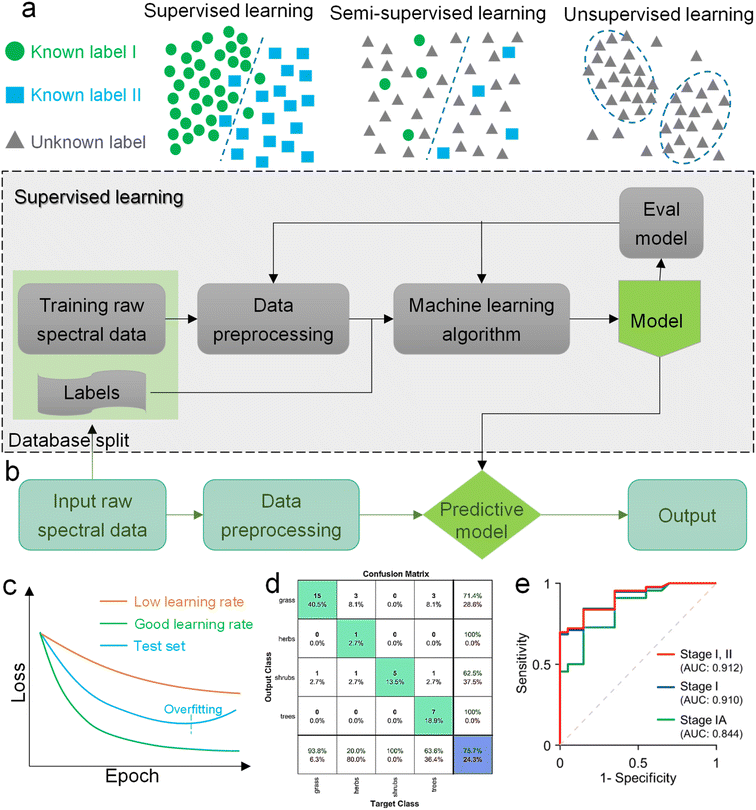 |
| Fig. 3 Machine learning algorithm. (a) Schematic diagram of the three classic learning frameworks. (b) General process of ML-based SERS/SEIRA data training and testing. (c) Loss curve during the training process. The loss of the test set increases when overfitting, indicating that fitting should be stopped within the dashed line. (d) Representation of a confusion matrix showing the performance of the classification models for the test data.195 Copyright 2019 MDPI. (e) ROC curves and corresponding AUC values.196 Copyright 2020 American Chemical Society. | |
The process of combining supervised and unsupervised ML algorithms with SEIRA/SERS is different. Supervised learning requires supervision to train a model, and unsupervised learning finds patterns in data, as shown in Fig. 3b, where the model training presented in the dashed box is performed under supervision. The top priority for the whole process is to select an appropriate ML algorithm based on the task objective. Then, the preprocessing of raw spectral data is required for both supervised and unsupervised ML algorithms.189 Since baseline wander is a common problem in analysis with IR and Raman spectroscopy, baseline correction is the first step in the preprocessing of raw spectral data. For infrared spectroscopy, the common method includes polynomial baseline correction, adaptive smoothness parameter penalized least squares method,190 Lorentz fitting, and constrained base-line correction based on a peak shift.191 In terms of Raman spectroscopy, the Savitsky–Golay-smoothing method is used to correct the baseline.192 Then, by sequentially subtracting the signal from the baseline and normalizing, a valid signal containing the target molecular features is obtained, which is used as the input to the ML algorithm.55 Normalization is necessary because it can compare the errors of the models and also reduce the impact of abnormal samples on the training process. In many cases, PCA is also used for noise reduction and dimensionality reduction during preprocessing.193 It is worth noting that some informative features in the original data may be accidently removed during the preprocessing. Therefore, it is necessary to pay attention to the change of features in preprocessing. The learning of the ML model consists of three stages, namely training, validation, and testing. The datasets corresponding to the three stages are training set, validation set, and test set, respectively. Therefore, the raw data needs to be divided into training set, validation set, and test set in preprocessing, and the ratio could be 60%, 20%, and 20%.194 The training dataset is the example dataset used during the learning process to find the best hyperparameters for the model. The validation set is an example dataset used to tune the hyperparameters of the model throughout the model development process, adding a feedback loop to the training of the model. The test dataset is a separate dataset from the training and validation dataset and is used to evaluate the final model chosen during the validation process. Since the signal to the target is obtained from the same SERS/SEIRA platform, the test dataset follows the same probability distribution as the training dataset.
In neural network models, weight initialization is a crucial design choice.197 Its purpose is to avoid layer activation outputs exploding or vanishing during the forward pass through the models. Common initialization methods include constant initialization and random initialization.198 For constant initialization, all weights in the neural network are initialized to a constant value C (typically 0). While constant initialization is simple, it is nearly impossible to break activation symmetry, making some models inefficient to learn. Random initialization can break the symmetry and let each neuron learn a different function of its input, but high or low weights could lead to exploding or vanishing gradients. Some new initialization techniques, such as He199 and Xavier200 initialization, are proposed to achieve a good starting point for initialization. After weight initialization, hyperparameter tuning becomes a critical task for the ML model.201 Common hyperparameter types include (i) κ in κ-NN, (ii) regularization constant, kernel type, and constants in SVMs, and (iii) number of layers, number of units per layer, and regularization in a neural network. To find the optimal value for hyperparameters, the tuning methods such as grid search, random search, and Bayesian optimization could be implemented. After hyperparameter tuning, cross-validation is often utilized to estimate the prediction performance of a model with the hyperparameter. An appropriate hyperparameter helps avoid under-fitting (high training and test errors) and overfitting (low training error but high test error). Under-fitting and overfitting can be observed in a loss curve which reflects the model error and answers the question “how bad our model is doing”.202 As shown in Fig. 3c, the loss decreases over time as the model learns, and the lower the loss, the better the model performance. Notably, it means overfitting starts when the loss on the test/validation set transitions from decreasing to increasing.203 The performance of the classification models for the test data can be displayed in a confusion matrix, as shown in Fig. 3d. It is represented in the form of a matrix and divided into two dimensions, the actual and predicted results along with the total number of predictions.204 It shows the error of the model performance, hence also called the error matrix. Another representative evaluation parameter is the receiver operating characteristics (ROC) curve, which reveals the performance of the classification model across all classification thresholds, as shown in Fig. 3e. The area under the ROC curve is called AUC. AUC provides an aggregated measure of performance across all possible classification thresholds and represents the trade-off between sensitivity and specificity.196 In addition to the confusion matrix, ROC and AUC, there are many evaluation parameters, such as accuracy, precision, recall, specificity, f1 score, and precision-recall curve.205,206 The key metrics that are commonly used for evaluating and reporting the data processing performance include mean squared error (MSE), root mean squared error (RMSE), mean absolute error (MAE), and average standard deviation of the mean (SDM). MSE is computed as
, where Y and Y are the measured and predicted values, respectively. RMSE is computed as
. MAE is calculated as the sum of absolute errors, that is,
, where ei is the absolute error. SDM is expressed mathematically as
, where
. MSE, RMSE, and MAE are often used to evaluate the difference between measured and predicted results. After introducing the benefits, algorithm types, and general process of ML-based SERS/SEIRA, we will explain and demonstrate it in the later section by reviewing the detailed application of machine learning in SEIRA from the perspective of substrate design, data processing, and decision-making.
3. Machine learning-enhanced substrate design of SERS and SEIRA
As discussed in previous chapters, machine learning algorithms enable the automated design of SERS/SEIRA substrates, thereby avoiding time-consuming and tedious design processes. Many efforts have been invested in it.207–215 In 2018, Jafar-Zanjani and co-workers demonstrated that an adaptive GA simplifies and automates the design of increasingly complex SEIRA substrates.216 To achieve algorithmic manipulation of substrate patterns, digitized binary elements are proposed as alternative high-dimensional building blocks for complex customizable multifunctional applications, as shown in Fig. 4a-i. Binary-pattern antennas provide more degrees of freedom for complex designs targeting multi-functional design goals under the support of powerful computing resources. GAs are inherently parallel algorithms capable of speeding up optimization tasks by a factor close to the number of parallel workers. Therefore, it has advantages in dealing with multi-dimensional function domains and discrete solution domain problems such as binary-pattern antenna design. For conventional GA, the weights of different objective functions are constant throughout the optimization process, and important objectives have higher priority and weight. However, the small weights given to less important objectives could deviations in the optimization and very slow convergence. The adaptive GA they proposed could overcome this problem by only considering the non-zero cost of high-priority objectives and updating the objective update criteria for the objective function in the optimization process (Fig. 4a-ii). As a result, the spectrum of the binary-pattern antennas automatically designed by adaptive GA is in good agreement with the target spectrum (Fig. 4a-iii).
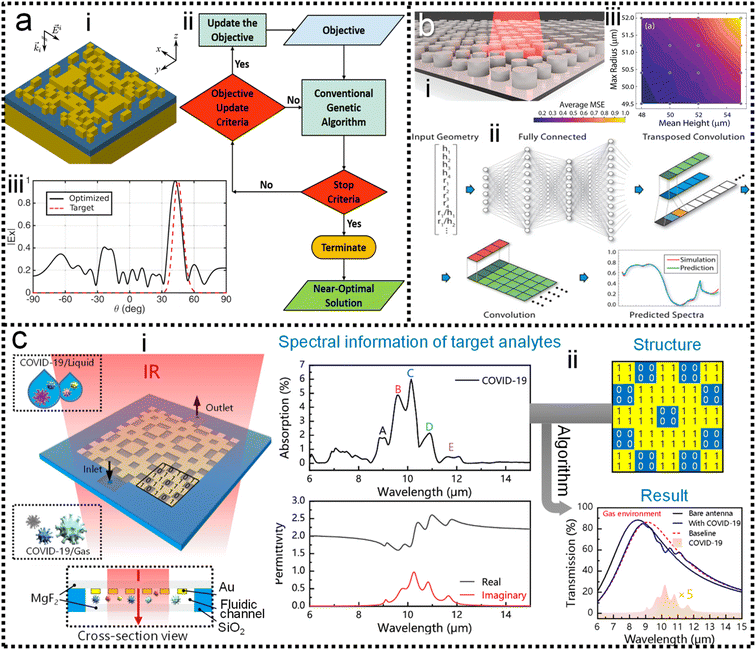 |
| Fig. 4 Machine learning-enhanced substrate design. (a) Schematic view of SEIRA substrate designed by using an adaptive genetic algorithm.216 (i): 3D view of the substrate. (ii): Design process using GA. (iii): Comparison of the predicted and simulated spectra. Copyright 2018 Springer Nature Limited. (b) Illustration of the all-dielectric SEIRA substrate designed by using neural network architecture.217 (i): 3D view of the substrate. (ii): Design process using neural network algorithm. (iii): MSE error in cross-validation. Copyright 2019 Optical Society of America. (c) SEIRA substrate design using a genetic algorithm for the detection of COVID-19.126 (i): 3D view of the substrate. (ii): Design process. Copyright 2021 American Chemical Society. | |
Of course, binary-pattern antennas are not the solution that all designers want. In most cases, with the antenna pattern already selected, researchers only need algorithms to optimize the performance of the antenna to high-level goals. In 2019, Nadell and co-workers demonstrated the use of deep neural networks (DNN) to model complex all-dielectric antennas and achieve performance optimization, as shown in Fig. 4b-i.217 A DNN is a kind of ANN with multiple layers between the input layer and the output layer. It finds the correct mathematical manipulation of whether the input and output are linear or non-linear. The geometric parameters x of the antenna such as radius, height along with ratios were used as the input layer of the DNN, and the corresponding spectra S was set as the predictions of the output layer in the training process. While DNNs could build hidden layers by exploiting additional network parameters during training, it increased the difficulty of optimization and led to poorer generalization. Taking knowledge of the underlying physics as input and pre-learning these quantities during training could improve network performance and reduce the difficulty of optimization (Fig. 4b-ii). The agreement of the prediction spectrum of the model with the target spectrum demonstrated the power of the DNN in the optimization of SEIRA substrate design. The MSE for the cross-validation set after training can be used to evaluate the prediction accuracy of the model (Fig. 4b-iii).
When considering coupled molecules in substrate design, machine learning is required to model the vibrational behavior of molecules and optimize the enhancement performance of the SEIRA signal. In 2021, Li and co-workers utilized a GA-based ML to automatically design a sensitive SEIRA substrate for COVID-19 (severe acute respiratory syndrome coronavirus 2) detection, as shown in Fig. 4c-i.126 Currently, the commercialized COVID-19 diagnostic methods include reverse transcription-polymerase chain reaction (RT-PCR) test, serological test or immunoassay, and chest computed tomography (CT). These methods suffer from their respective disadvantages, such as low sensitivity for CT and time-consuming for RT-PCR. Plasmonic methods with the potential for point-of-care (POC) diagnostics are highly desirable. The first step for modeling the vibrational behavior of molecules was the extraction of molecular complex permittivity from its infrared spectrum. Then, the optimal solution, with high sensitivity, zero detuning of plasmonic resonance and molecular vibration, high enhancement factor, and so on, is rapidly found from multi-design parameter problems by exploiting the excellent parallel capabilities of GA (Fig. 4c-ii). Furthermore, the mutation of COVID-19, which poses a great challenge to common diagnostic methods, can be easily distinguished by SEIRA methods by comparing the intensity and frequency of peaks of the virus. Overall, the use of machine learning in the design of SEIRA antennas is booming, while its use in SERS substrate design is rare. Because antennas for SEIRA are generally on the micrometer scale, wherein antenna patterns with various design parameters can be realized by using top-down fabrication-based photolithography and direct writing technique. That is, the multi-parameter complex design of antennas for SEIRA requires the help of powerful technical resources of machine learning. In terms of SERS, its signals are large and complex in many applications. Studies employing ML to aid SERS process data and make decisions are more common.
Systematic inverse design based on machine learning can accelerate the design of SEIRA/SERS substrates to achieve tailored optical responses. The underlying idea of the ML-assisted reverse design is to train an ML model by learning the relationship between physical responses and structures, and then provide structural patterns based on the desired physical responses. A well-trained model can remove the need of computationally intensive numerical simulations from the pattern. Fig. 5a shows the simultaneous inverse design of material and structural parameters of core–shell nanoparticle-based nanophotonic substrate, demonstrated by So and co-workers.218 Since the structural parameters are continuous quantities and the material parameters are discrete quantities, it is difficult to achieve the simultaneous inverse design of these two parameters using an algorithm. By combining both regression and classification into the same implementation (Fig. 5a-ii), core–shell nanoparticles are designed in reverse to meet the user-drawn spectra (Fig. 5a-i). In the model training process, a large number of parameters and corresponding spectra obtained through forward design are required. After training, the model is tested by using a hand-drawn Lorentzian function with peaks at specific locations (Fig. 5a-iv). The parameters of the core–shell nanoparticle are obtained and the predicted spectra match well with the target spectra (Fig. 5a-v).
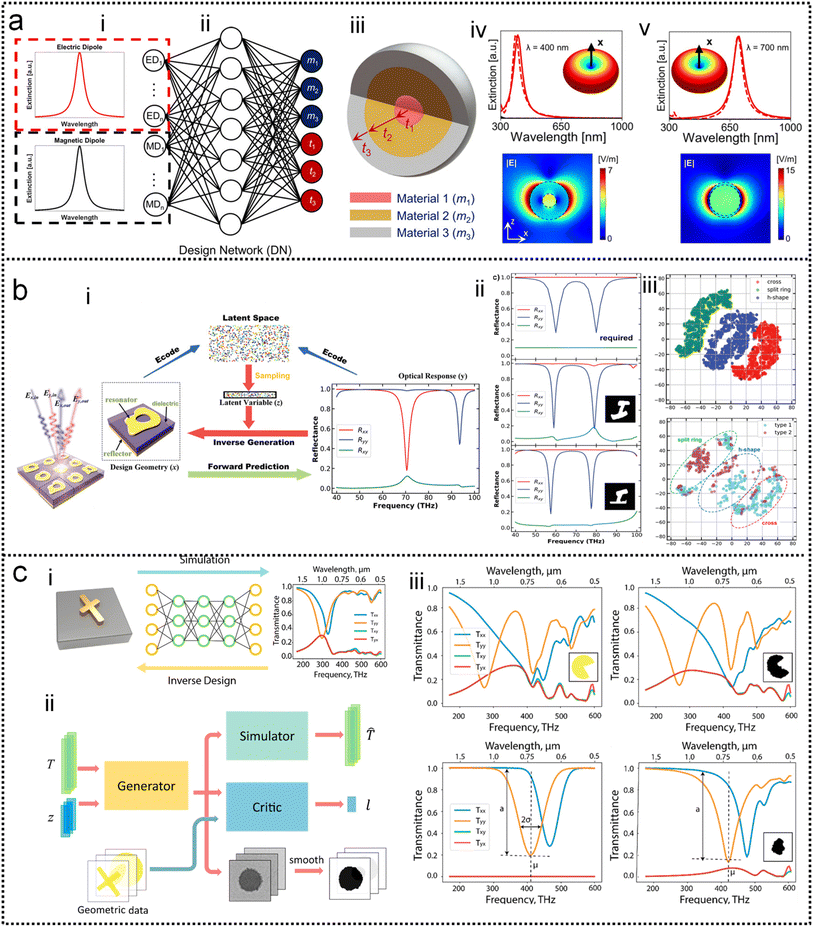 |
| Fig. 5 Inverse design of nanophotonic devices. (a) Simultaneous material and structural inverse design through a supervised deep learning algorithm.218 (i): Target spectrum. (ii): Schematic diagram of the machine learning model used in the reverse design. (iii): Schematic representation of core–shell nanoparticle-based substrates. (iv): Inverse design example of core–shell nanoparticle at resonant wavelengths of 400 nm. (v): At resonant wavelengths of 500 nm. Copyright 2019 American Chemical Society. (b) Inverse design of nanophotonic devices using a semi-supervised deep learning algorithm.219 (i): A schematic of inverse design. (ii): The required reflection spectra (upper panel), the results of inverse design (middle, bottom panel). Insets are the design pattern through algorithms. (iii): Visualization of the latent space. Copyright 2019 John Wiley and Sons. (c) Inverse design of nanophotonic devices using an unsupervised deep learning algorithm.220 (i): Schematic illustration of transitioning device design from a traditional trial-and-error approach to machine learning-mediated inverse design. (ii): Network architecture to inverse design structural images. (iii): Examples of the results of the inverse design. Copyright 2018 American Chemical Society. | |
Semi-supervised learning algorithms can also be used in the reverse design, which reduces the amount of data for training, where both labeled and unlabeled data are used for training. Ma and collaborators propose a network that differs from other inverse design methods.219 It takes input geometry and encodes the structural design and optical responses as latent variables with predefined distributions (Fig. 5b-i). Randomly sampling and decoding these latent variables from the latent space can reconstruct the original structural geometry, thereby enabling inverse design. Fig. 5b-ii shows the simulated spectra using the parameters obtained from the inverse design (middle and bottom pane in Fig. 5b-ii), which matches well with the required spectrum (upper panel). The sampling process provides a variety of outputs for the same target spectrum, that is, it generates many candidates for reverse design. Furthermore, they demonstrated that the proposed model can automatically learn to distinguish different shapes through encoding–decoding training iterations on labeled and unlabeled data (Fig. 5b-iii).
Besides, inverse design of nanophotonic structures using unsupervised learning algorithms is also feasible. Liu and workers adopted a generative adversarial network (GAN) in the network model to reverse design the arbitrary geometry of the substrate (Fig. 5c).220 GAN is an unsupervised learning architecture. It consists of two networks, namely a generator and a critic. They compete against each other and learn simultaneously to create authentic pattern. The generator receives the random noise and generates a pattern of the structure that should have the desired optical properties. The pattern is judged by the critic and then the critic decides whether it comes from the structural geometry of interest. The objective of the generator network is to deceive the critic network by generating authentic pattern. After training, the generator model can create designs that resemble patterns in the actual geometric data (Fig. 5c-i). In addition to a generator and a critic required by traditional GAN models, the authors also add a simulator network to approximate the optical properties of the generated design patterns (Fig. 5c-ii). After unsupervised training, the model is able to provide structural patterns for a given spectrum. For specific spectral requirements, the simulated spectra of the test pattern and the generated pattern (via reverse design) are well matched (Fig. 5c-iii).
4. Machine learning-enhanced data processing of SERS and SEIRA
As discussed in previous chapters, machine learning algorithms assist SERS and SEIRA to analyze and process data quickly, directly, and automatically, and achieve high accuracy and resolution through noise reduction. Taking SEIRA as an example, Meng and colleagues demonstrated noise reduction by using a frame averaging model.122 The sensor signals recorded by the system are generally data including noise. Noise in the data can be effectively suppressed by taking the recorded data without the analyte as a reference and normalizing the raw data, as shown in Fig. 6a. Furthermore, the error bars of the data are significantly reduced by increasing the frequency (frame) of data acquisition and performing frame averaging (Fig. 6a-ii). It shows that proper data preprocessing to generate datasets for training ML models is critical and beneficial to the improvement of ML model accuracy. John-Herpin and co-workers compared the performance of SEIRA-based biosensors with/without using ML models in monitoring the dynamics of biomolecular reactions, as shown in Fig. 6b.58 The biological reaction is the release of nucleotides and sucrose in the liposomes by utilizing melittin to perforate the lipid membrane of liposomes (Fig. 6b-i). The results of the biosensor is analyzed using a MLR model. The hypothesis of MLR is h(x) = θ0 + θ1x1 + θ2x2 + … + θnxn, where θ is called regression coefficient and x is input measurement data. The cost function is
. By obtaining the optimal value of θ from the training set, the result of monitoring the molecular change in dynamic process is extracted, as shown in Fig. 6b-ii. The detailed mathematics behind MLR can be found in Note S3 of ESI.† By using the DNN model (Fig. 6b-iii–v) to process the data of the biosensor, new results could be obtained, as shown in Fig. 6b-vi. Overall, the trends in results with and without machine learning were similar. Significant increases in sucrose and nucleotide signals were observed after the first injection of Melittin. The liposome signal stabilized, while the sucrose and nucleotide molecule signals stabilized at t = 100 min. Notably, compared to results without machine learning, the Melittin signal had few false negatives and remained stable around zero before it was introduced. This shows that the DNN method helps to effectively reduce the interference effect of the matrix, and the information extracted by DNN yielded superior performance.
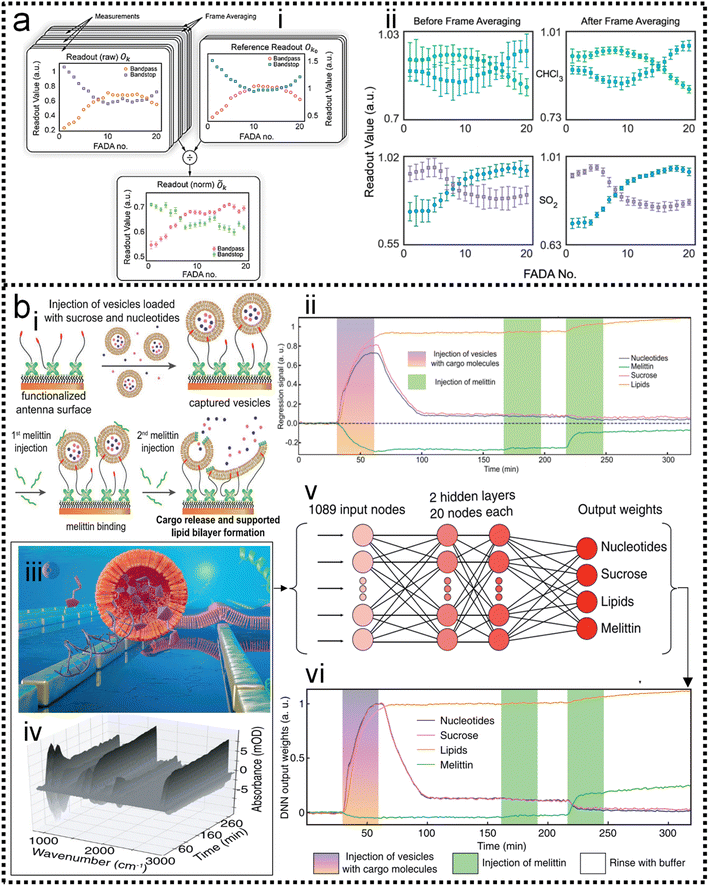 |
| Fig. 6 Machine learning-enhanced data processing in SEIRA. (a) Data preprocessing using frame averaging model for noise reduction.122 (i): Schematic diagram of the conversion from raw noisy data to ML data set. (ii): Data before and after frame averaging showing the result of noise reduction. Copyright 2021 American Chemical Society. (b) Data processing using DNN algorithm for dynamic biomonitoring.58 (i): Schematic depiction of the dynamic process of biological reaction. (ii): Measurement results of the dynamic process without using ML for data processing. (iii): Schematic diagram of SEIRA-based biosensor. (iv): Raw spectral data obtained by biosensors in biological reactions. (v): Schematic diagram of the DNN algorithm for raw spectral data processing. (vi): Results of the dynamic process using DNN for data processing. Copyright 2021 John Wiley and Sons. | |
In terms of SERS, spectral statistics and data processing methods have grown significantly. The first step, before spectral comparison and further data processing, is to normalize the data. Fluorescence removal, filters' signal removal, baseline subtraction, intensity normalization, smoothing, and more are commonly used routines. A brief overview of the possible use of these tools, as well as several ways to analyze and compare spectra, is presented: (1) the construction of spectral libraries and automatic searches; (2) the determination of flowcharts and criteria for the unambiguous characterization of the studied materials, and (3) the use of chemometrics for data classification. Some examples relying on these statistical tools to advance our knowledge on the aging of materials or the determination of individual components in mixtures are also presented. The importance of data processing in the use of surface-enhanced Raman spectroscopy is significant. But current progress is limited by cumbersome and intensive data collection steps. More important, a typical lab-scale SERS experiment requires the user to evaluate the quality and reliability of the result as the data are being collected. There is an urgent need to develop to simplify and accelerate data processing steps. The challenge can be addressed by ML-enhanced data processing in SERS.221–224
Alstrom and co-workers225 proposed a Bayesian Non-negative Matrix Factorization (NMF) approach to identify the locations of target molecules. This method can successfully analyze the spectra and extract the target spectrum, as shown in Fig. 7a. A visualization of the loadings of the basis vector is created and the results show a clear SNR enhancement. Compared to traditional data processing, the NMF approach enables a more reproducible and sensitive sensor. It was able to identify the estradiol glow base spectrum as one of the basis vectors at high concentrations. This allows for more accurate and robust data analysis when the SERS substrate is contaminated by unknown molecules, as shown in Fig. 7a-iv. A further advantage of using NMF for SERS data is that the method is interpretable, because the basis vectors identified can be related to the expected physical effects (Fig. 7a-v). At the same time, both the high demand for mass data and the low interpretability of the mysterious black-box operation significantly limit the well-trained model to real systems in practical applications. Aiming at these two issues, Luo and co-workers226 constructed a novel machine learning algorithm-based framework (Vis-CAD), integrating visual random forest, characteristic amplifier, and data augmentation (Fig. 7b). The introduction of data augmentation significantly reduced the requirement of mass data, and the visualization of the random forest clearly presented the captured features, which helps to determine the reliability of the algorithm. For instance, the trace analysis of individual polycyclic aromatic hydrocarbons in a mixture achieves a confidence accuracy of no less than 99% under optimized conditions. The visualization of the algorithm framework clearly shows that the captured features are closely related to the characteristic Raman peaks of each individual, as shown in Fig. 7b-i. Moreover, the sensitivity to trace individuals can be improved by at least 1 order of magnitude compared to the naked eye. The lesser need for the proposed algorithm for massive data and the visualization of the operational process provides new avenues for the indestructible application of machine learning algorithms, and it helps the SERS field to improve the limits of sensitivity for both qualitative and quantitative analysis of traces.
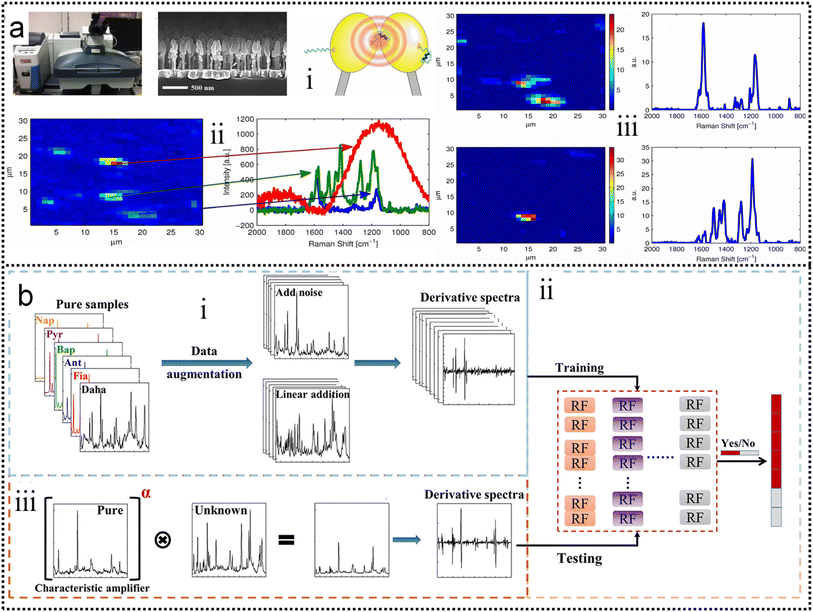 |
| Fig. 7 (a) Improving the robustness of SERS by NMF.225 (i): The Raman microscope used to collect data. (ii): A side-view up of a Raman substrate depicting the nanopillars. (iii): Illustration of the principle behind the SERS substrates. (iv): An example of a Raman map that has been locally contaminated (red and green spectra). (v): Noise filtering using NMF. The left-hand side is the loadings in the left drawn as Raman maps and the right-hand side is the corresponding basis vector in S. Copyright 2014 IEEE. (b) Schematic flowchart of the Vis-CAD.226 (i): Data preparation and processing. (ii): Stochastic Forest chain model. (iii): Model testing. Copyright 2022 American Chemical Society. | |
5. Machine learning-enhanced decision-making of SERS and SEIRA
ML-assisted decision-making, such as the automatic determination of analyte types through classification algorithms, is the most widely used and well-established in SEIRA and SERS. Its detection targets are diverse, mainly including small molecules, biomarkers, circulating tumour cells, pathogens, proteins, bacteria, and DNA.
5.1 ML-enabled detection of small molecules
In SEIRA, simultaneous detection of multiple molecules is challenging because of spectral overlap. By training the ML model to become familiar with the spectra of molecules, it can accurately distinguish and identify multiple molecules in a mixed environment. For example, the IR spectra of glucose and fructose partially overlap due to the presence of C
O vibrations in both. Kühner and co-workers demonstrated the discrimination and detection of physiological levels of glucose and fructose by developing a PCA-assisted SEIRA biosensor, as shown in Fig. 8a.56 It works by placing a SEIRA substrate in internal reflection mode on a flow cell (Fig. 8a-ii), which is flushed through an attached tube connector to deliver analytes in and out of the flow cell (Fig. 8a-i). Then PCA algorithm is used to decompose measurement data and represented them as a set of orthogonal and uncorrelated eigenfunctions called principal components (PC) and eigenvalues defined as termed scores (SCs). The first-order PC corresponds to the variance that contributes the most, and thus constitutes the largest contribution. Similarly, the second-order PC contributes the second largest, and so on. The more significant the correlation between the various datasets is, the less PC is needed to describe the features of the entire dataset. The PCs and corresponding SCs are shown in Fig. 8a-iii and iv. Clearly, the PCA algorithm can extract solutions of different concentrations, which is a key element of an automated evaluation procedure. To achieve automatic evaluation, the PCs data obtained by using PCA requires further supervised learning through classification algorithms. Meng and co-workers demonstrated chemical material identification by using the PCA algorithm and SVM classifier to process the data of a plasmonic mid-infrared filter array-detector array.122 The sensor integrates a plasmonic filter array with an IR detector array, as shown in Fig. 8b-i. First, the data of the detector is calculated as PC1, PC2, and PC3 via PCA algorithms (Fig. 8b-ii). The PCA begin with the standardization of the continuous variables of the dataset. The next is to construct the covariance matrix by
, where Xi and Yi are the specific training dataset from variables X and Y, and μx and μy are the means of the variables. The eigenvector of a matrix A is calculated by A
= λ
, where λ is the eigenvalue and
is the eigenvector. The 1st PC v1 is the eigenvector of the sample covariance matrix A associated with the largest eigenvalue. The 2nd PC v2 is the eigenvector of the sample covariance matrix A associated with the second largest eigenvalue. Then, the SVM model is trained to identify which chemical is present and determine the concentration of a specific analyte. The hyperplane is often used to separate the different classes in SVM. It can be expressed as ω·x − b = 0, where ω is a weights that determines the orientation of the hyperplane and b is the bias. The distance of any point x in dataset to the hyperplane is calculated as d = |ωT·x − b|/‖ω‖. The training task is to maximize the distance d using the training dataset (see Note S3 of ESI† for details). The confusion matrix demonstrates good automatic identification of the different analytes with a 10-fold cross-validated accuracy value of 95.34%. The sensitivity and bandwidth of SEIRA are often limited by the small overlap between molecules and sensing hotspots, as well as by sharp plasmonic resonance peaks. Our group developed a wavelength-multiplexed hook-shaped nanoantenna array for continuous broadband detection to capture multiple absorption peaks in the fingerprint region, as shown in Fig. 8c-i.121 For different analytes with the same functional group, their SEIRA spectra overlap in many regions (Fig. 8c-ii). Therefore, it is difficult to distinguish them in mixtures using narrow-band SEIRA substrates. With the help of PCA and SVM algorithms, 100% recognition accuracy is achieved (Fig. 8c-iii–vi). This strategy can be designed to cover the entire infrared fingerprint range, that is, by designing the antenna length for specific molecular monitoring, such as proteins, sugars, lipids, nucleic acids, and volatile organic compounds.
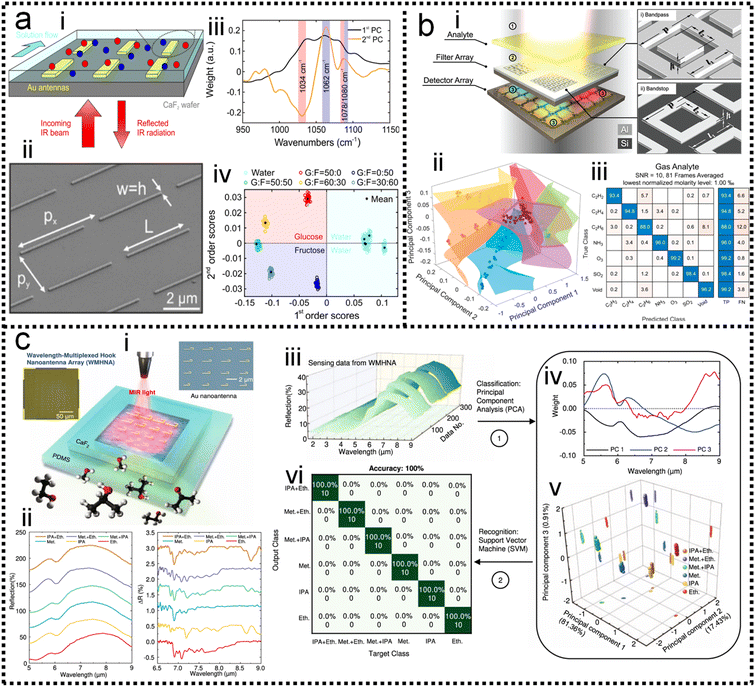 |
| Fig. 8 ML-enhanced detection of small molecules. (a) Glucose detection using PCA-assisted SEIRA.56 (i): Schematic diagram of the biosensor. (ii): SEM image of the biosensor. (iii): First and second principal components of the measurement data using PCA. (iv): Visualization of classification results. Copyright 2019 American Chemical Society. (b) Multiple chemical detection using SVM-assisted SEIRA.122 (i): Schematic diagram of the SEIRA-based sensor. (ii): Visualization of classification results for different analytes. (iii): Confusion matrix for analyte classification results. Copyright 2021 American Chemical Society. (c) Volatile organic compounds (VOCs) detection using PCA/LDA-assisted SEIRA.121 (i): Schematic diagram of the SEIRA-based VOC sensor. (ii): The measured spectra of analytes showing spectral overlapping, which is difficult to distinguish clearly with the classic data processing methods. (iii): The measured raw spectral data. (iv): Data preprocessing using PCA. (v): The weight of scores of each spectrum in 3D space. (vi): The confusion map for analyte classification results. Copyright 2022 Springer Nature Limited. | |
5.2 ML-enabled detection of biomarkers
Although SERS has been applied to monitor biomarkers for different disorders (neurological, infectious, and genetic diseases), the direct addition of complex biological media onto a plasmonic substrate may give rise to SERS spectra where the presence of individual biomarkers cannot be identified. Most studies are based on the screening of biomarkers that display both high affinity toward metal surfaces and high Raman cross sections. When such molecules are present in the probe solution, they can easily dominate the SERS signal, masking the presence of other analytes while their characteristic fingerprint is obvious in the spectra. This screening, even at very low concentrations, is highly advantageous, therefore, reaping the reward of fast SERS monitoring. This may be the reason why similar biomolecules are reported in most ongoing applications. In the scenario of impaired signal enhancement, classical methods for SERS spectral analysis, where a direct correlation between vibrational peaks and the presence of individual metabolites can be established, are no longer sufficient. Therefore, chemometrics methods have emerged as a reliable alternative to conventional approaches. In this context, multivariate and machine learning algorithms can be devised to substantially improve data processing, such as the calibration and classification of multicomponent samples and the identification of interferences in complex biochemical systems. A wide range of multivariate statistical and machine learning methods are currently available to decipher the optically rich and complex information contained in SERS spectra. For instance, some groups have tackled quantification challenges of biomarkers by using a so-called SERS digital procedure, which considers the number of SERS event counts rather than SERS intensities and couples them with machine learning.26,227–232
Kim and co-authors demonstrated the detection of extracellular vehicles (EVs) as an excellent resource of diagnostic biomarkers in serum samples from normal controls individuals, and chronic pancreatitis and pancreatic cancer patients using a SERS-based immunoassay technique,227 as shown in Fig. 9a. Applying a machine learning algorithm (Fig. 9a-iv) to the analysis of the expression level of EVs biomarkers in pancreatic cancer, chronic pancreatitis, and normal control individuals, the sensitivity and specificity were measured as 0.95 and 0.96, respectively, which suggests the great potential of using this biomarker to differentiate pancreatic cancers from chronic pancreatitis. Dual-modal (FRGBRSERS, FRRSERS) fluorescence–SERS quantum dot (QD)-embedded silver bumpy nanoparticles (Fig. 9b-i) are developed by Cha and co-authors for high-throughput multiplex analysis.233 Each FRRSERS nanoprobe produces strong SERS and fluorescence signals for multiplex analysis (Fig. 9b-ii). Based on this dual-modality, a barcode-based machine learning algorithm that transforms spectra into barcodes and identifies chemical information is created, as shown in Fig. 9b-iv. The multiplex detection platform comprising the FRRSERS nanoprobes and the high-throughput analysis algorithm will be extremely useful for analyzing and encoding biological targets.
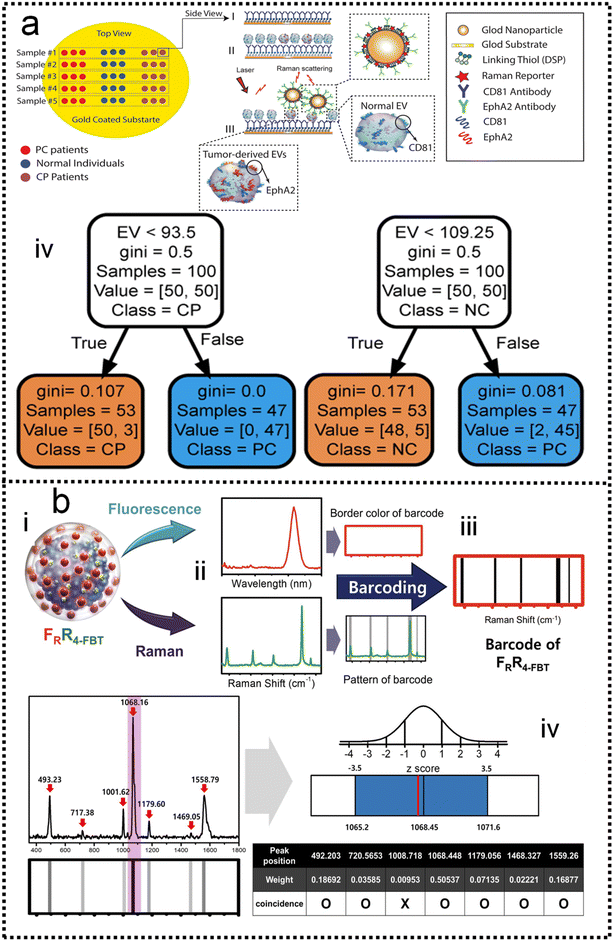 |
| Fig. 9 (a) A SERS-based immunoassay for pancreatic cancer (PC) tumor-derived extracellular vesicles (EVs) quantification227 (i): functionalizing gold substrate with thiol and CD81 antibody. (ii): Capturing normal and tumor-derived EVs present in the serum sample. (iii): Loading EphA2-NPs-reporter to enhance Raman signal and selectively label tumor-derived EVs. (iv): The classification tree trained with the whole dataset of peak-value Raman shifts with depth = 2. Copyright 2021 John Wiley and Sons. (b) Dual-modal fluorescence–SERS quantum dot (QD)-embedded silver bumpy nanoparticles are developed for biomarkers high-throughput multiplex analysis.233 (i): Nanoprobes are prepared from silica-coated silver bumpy nanoshells (AgNS@SiO2). (ii) Each dual-modal (FRGBRSERS, FRRSERS) nanoprobe produces strong SERS and fluorescence signals for multiplex analysis. (iii): A barcode-based machine learning algorithm that transforms spectra into barcodes and identifies chemical information is created. (iv): The result of applying density-based spatial clustering of applications with noise (DBSCAN) to the spectrum of 4-bromobenzenethiol (4-BBT), and the corresponding barcode. Copyright 2021 Elsevier. | |
5.3 ML-enabled detection of circulating tumor cells
Cancer metastasis is the main reason for cancer-related death. Circulating tumor cells (CTCs) shed from primary or metastatic tumor cells at the very early stages of cancer may enter into the human peripheral blood and metastasize to distant tissues. CTCs can be accurately examined in vitro by cell culture, phenotype, and genotype analysis. Hence, CTCs are important tumor markers for clinical analysis such as early tumor diagnosis, postoperative evaluation, monitoring of cancer treatment, and diagnosis of recurrence or metastasis. The SERS method possesses the features of remarkable detection sensitivity, a non-destructive nature, label-free detection, a quick spectrum response, and a molecular fingerprint spectrum. In the past decade, SERS technology serving as a bio probe has been increasingly applied to detect circulating tumor cells due to its unique detection advantages.234–244 In the past few years, the detection of CTCs using SERS has been extensively studied. Wu and co-authors developed an AuNP-MBA-rBSA-FA SERS bioprobe for the direct detection of CTCs in blood with high specificity and high sensitivity. The SERS peak intensity and the number of cancer cells in the range of 5–500 cells per mL exhibited a good linear relationship (R2 = 0.9935), indicating that this SERS bioprobe could be applied to quantitative analysis of CTCs in human peripheral blood.245 Pang and co-workers developed a Fe3O4@Ag magnetic NP and Au@Ag nanorod complex SERS bioprobe. The limit of detection for hepatocellular carcinoma CTCs can reach 1 cell per mL in human peripheral blood samples based on dual signal-enhancing strategies.246 Sensitivity is an important factor for CTC detection, but the feature of SERS spectral stability, biocompatibility, and anti-interference ability should also be optimized in CTC analysis systems.
The SERS spectra of blood cells and various tumor cells are measured (Fig. 10a-iii) with the silver film substrate by Fang and co-workers.247 It is found that there are significant differences in nucleic acid-related characteristic peaks between most tumor cells and blood cells. These spectra are classified by the feature peak ratio method, the principal component analysis combined with K-nearest neighbor, and residual network, which is a kind of deep learning algorithm. The results showed that the ratio method and PCA-κNN can only distinguish SERS spectra that come from blood cells and some kinds of distinct tumor cells, while the built residual network (ResNet) model can perfectly classify SERS spectra of blood cells and all kinds of tumor cells with an identification accuracy of 100%. The research studies demonstrate that the silver film SERS substrate can stably enhance the Raman scattering signal of cells, and the deep learning algorithm can quickly and effectively identify the SERS spectra of tumor cells and blood cells, as shown in Fig. 10a-iv and v. Erzina and co-workers248 presented the advanced approach for the detection of compositional changes in culture medium arising from the metabolic activity of tumor or normal cells (Fig. 10b). The approach combines innovative techniques from the field of machine learning. First, the functionalized nanoparticles, after the interaction with biosamples, were deposited on the gold grating surface to achieve plasmonic coupling and high SERS enhancement. Subsequently measured SERS spectra are used as the input for advanced CNN training and validation, while the kind of nanoparticles surface functionalization serves as additional parameters, increasing the flexibility and reliability of the method. The CNN classification results were then translated into sensitivity and specificity for each cell kind and 100% accuracy in the discrimination of tumour and normal cell's cultivation media was achieved, as shown in Fig. 10b-vii. Such an approach is useful to make clinical decisions rapidly and accurately possible.
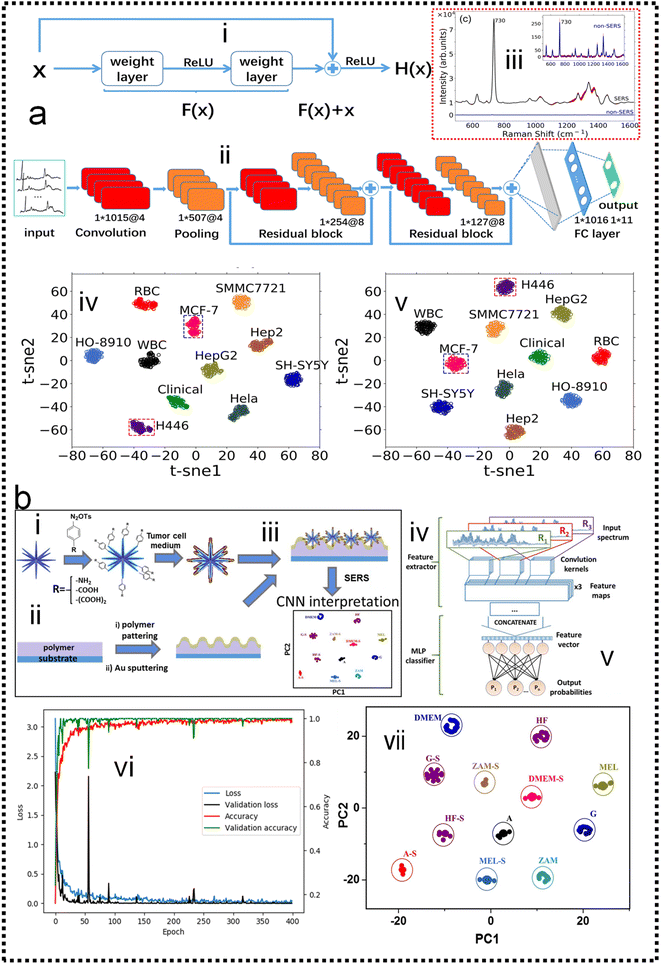 |
| Fig. 10 (a) Fast discrimination of tumor cells by label-free SERS and deep learning247 (i): structure of the residual network unit. (ii): The basic structure of ResNet, which consists of a convolutional layer, pooling layer, two residual blocks, and two fully connected layers. (iii): The Raman spectra of tumor cells are enhanced by the silver film substrate and aluminum plate substrate. (iv): t-distributed stochastic neighbor embedding (t-SNE) diagram after extracting original data features by the ResNet model which contains two residual blocks. (v): t-SNE diagram after extracting original data features by the ResNet model which contains five residual blocks. Copyright 2021 AIP Publishing LLC. (b) Schematic representation of the proposed experimental concept.248 (i): preparation of functional AuMs and their interaction with culture medium sample. (ii): simultaneous preparation of gold grating. (iii): deposition of AuMs on the surface of the gold grating. (iv): SERS measurements. (v): implementation of CNN for SERS result interpretation. (vi): Accuracy and loss evolution during the CNN learning and validation. (vii): The visualization of predictive ability of the proposed SERS/CNN approach. Copyright 2020 Elsevier. | |
5.4 ML-enabled detection of pathogens
SERS has enabled the detection of pathogens at extremely low levels. In a typical SERS process, test targets are directly attached to the surface of NPs to generate enhanced Raman signals.249 However, the exposed surface of metal nanoparticles easily absorbs other interfering molecules, resulting in insufficient SERS signal stability.250 Pathogen detection using label-free SERS methods is mainly accomplished by mixing noble metal nanoparticles with bacterial cells in solution, or by forming nanoparticle films on substrates for bacterial attachment. The adsorption sites mainly include nanostructured metal surfaces and noble metal NPs surfaces.251–253 In addition, the multifunctional SERS254 and microfluidic255 platforms are also used for pathogen detection to improve SERS activity, pathogen isolation ability, and detection speed. Despite some progress, current methods and platforms still face challenges in qualitative and quantitative analysis of real samples. Machine learning has strong advantages in improving qualitative and quantitative analysis capabilities, improving high sensitivity, reproducibility of results, and shortening data analysis time.
Combining SERS sampling and machine learning data analysis, Hong designed and developed a simple, fast, and inexpensive optical sensing platform.256 The pre-processing of spectral measurement employed gold nanoparticle colloid mixing with the serum from patients with colorectal cancer (CRC) to predict the disease. Portable Raman spectrometer and the PCA were used to determine the phenotypic variations of fungal cells Candida albicans (C. Albicans) under the influence of different antifungals with various mechanisms, and unknown antifungals were predicted using the established PCA model.257 In another study, Tang and co-workers analyzed SERS through machine learning algorithms to discriminate bacterial pathogens quickly and accurately.258 Surface-enhanced Raman spectroscopy combined with machine learning techniques enables rapid discrimination between methicillin-resistant and methicillin-sensitive Gram-positive Staphylococcus aureus strains and Gram-negative Legionella pneumophila (controls).118 A total of 10 methicillin-resistant S. aureus (MRSA), 3 methicillin-sensitive S. aureus (MSSA) and 6 L. pneumophila isolates were used. The obtained spectra indicated high reproducibility and repeatability with a high signal-to-noise ratio, as shown in Fig. 11a-i. PCA, HCA, and various supervised classification algorithms were used to discriminate both S. aureus strains and L. pneumophila. The results indicate that SERS combined with machine learning can be used for the detection of antibiotic-resistant and susceptible bacteria and this technique is a very promising tool for clinical applications. Tang and co-workers116 compared three unsupervised machine learning methods and 10 supervised machine learning methods, respectively, on 2752 SERS spectra from 117 Staphylococcus strains belonging to nine clinically important Staphylococcus species to test the capacity of different machine learning methods for bacterial rapid differentiation and accurate prediction. According to the results, density-based spatial clustering of applications with noise (DBSCAN) showed the best clustering capacity (Rand index 0.9733) while CNN topped all other supervised machine learning methods as the best model for predicting Staphylococcus species via SERS spectra (ACC 98.21%, AUC 99.93%). As shown in Fig. 11b-ii. This study shows that machine learning methods are capable of distinguishing closely related Staphylococcus species and therefore have great application potential for bacterial pathogen diagnosis in clinical settings. Thrift and co-workers presented the first SERS odor compass (Fig. 11c-i).259 Using a grid array of SERS sensors, machine learning analysis enables reliable identification of multiple odor sources arising from the diffusion of analytes from one or two localized sources. Specifically, CNN and SVM classifier models achieve over 90% accuracy for a multiple odor source problem. This system is then used to identify the location of an Escherichia coli biofilm via its complex signature of volatile organic compounds. Thus, the fabricated SERS chemical sensors have the needed limit of detection and quantification for diffusion-based odor compasses. Solving the multiple odor source problem with a passive platform opens a path toward an Internet of things approach to monitoring toxic gases and indoor pathogens.
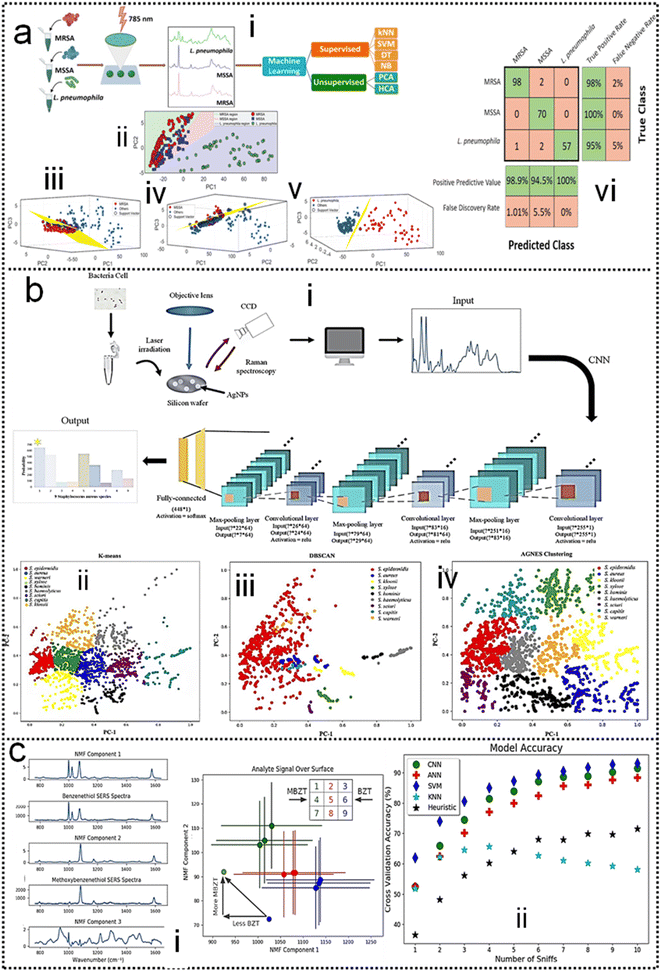 |
| Fig. 11 (a) Identification of methicillin-resistant Staphylococcus aureus bacteria using SERS and ML.118 (i): Scheme of using surface-enhanced Raman spectroscopy combined with machine learning techniques for rapid identification of pathogens. (ii): Multiple class boundaries of the SVM classifier with linear kernel function in a 2-dimensional PCA plane. SVM hyperplanes found by the one-vs.-all method and linear kernel function. (iii): MRSA-vs.-all. (iv): MSSA-vs.-all. (v): L. pneumophila-vs.-all. (vi): 5-Fold cross-validated confusion matrix of the k-nearest neighbor classifier for the identification of Staphylococcus aureus strains and Legionella pneumophila. Copyright 2020 Royal Society of Chemistry. (b) Comparative Analysis of Machine Learning Algorithms on SERS of clinical Staphylococcus species116 (i): Schematic illustration of CNN data flow during processing SERS spectra of Staphylococcus species. (ii): Clustering results of nine Staphylococcus species via (iii): k-means, (iv): DBSCAN, and (v): agglomerative nesting (AGNES). Copyright 2021 Frontiers Media SA. (c) SERS-Based Odor Compass: Locating Multiple Pathogens259 (i): NMF components determined from analyte training datasets. (ii): Cross-validation accuracy of the models used. Copyright 2019 American Chemical Society. | |
5.5 ML-enabled detection of proteins
Protein detection and identification are important for proteomics and early diagnosis of various diseases. SERS is a powerful assay that is non-destructive and non-invasive. In addition, SERS has been used to directly detect proteins without any Raman labels and has the property to effectively distinguish target molecules in complex environments. Kahraman and co-workers have proposed a sensitive and reproducible method for label-free detection of proteins based on the self-assembly of AgNPs and proteins on hydrophobic surfaces.260 SERS spectral features and reproducibility were observed. The lowest detectable concentration was as low as 0.5 μg mL−1. To improve the sensitivity and selectivity of immunoassays, nanostructure-based SERS nanotags have attracted increasing attention.261,262 Multiplexed protein detection is also an important topic in the field of SERS immunosensors.263–266 In addition to traditional SERS strategies, the combination of SERS with machine learning has attracted increasing attention in protein detection.
Barucci and co-workers193 performed in situ rapid and highly sensitive analysis by label-free SERS. Then resorted to a hybrid analytical method consisting of PCA obtained by band-fitting the SERS spectra of protein samples. Compared to the application of standard PCA to raw spectral data, the method succeeded in classifying proteins excellently and preserving the biological differences contained in their SERS spectra. The scheme is shown in Fig. 12a-i. The field of application of this method combined with machine learning involves the detection and analysis of proteins and can also be extended to other biomolecular species. The expression of the S-protein in the coronavirus makes it a marker for detecting the virus. Yang and co-workers have developed a functionalized gold “virus-traps” nanoarray as a novel COVID-19 SERS sensor to capture and identify viruses with extremely high sensitivity and specificity.267 The S protein is specifically immobilized within a region of strong electromagnetic field enhancement 10 nm from the nanoneedle surface, which includes “lightning rod” and “hot spot” effects that can enhance the highly enhanced Raman signal unique to the S protein of the SARS-CoV-2 virus. The identification standard of SERS signals established by machine-learning and identification techniques has been utilized to identify simulated COVID-19 from urines with a viral load of as low as 80 copies mL−1 as short as 5 min. Viruses are captured by functionalized surfaces, generating enhanced signals, and ancillary processes of machine learning, as shown in Fig. 12b-ii, which is of great significance for achieving real-time monitoring and early warning of coronavirus.
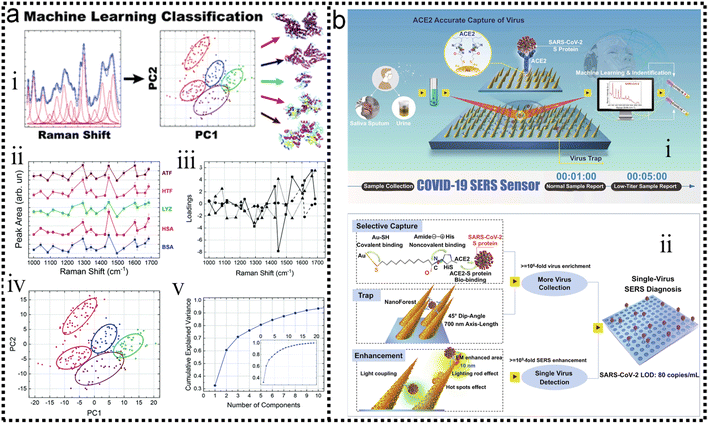 |
| Fig. 12 (a) Label-free SERS detection of proteins based on machine learning classification of chemostructural determinants.193 (i): Scheme of label-free SERS detection of proteins based on machine learning classification of chemo-structural determinants. (ii): Average area intensity values upon fitting the SERS spectra. (iii): Exemplary cross-validated PC1 vs. PC2 score plot of area intensity values. (iv): PCA loading plot. (v): PCA explained variance. Copyright 2020 Royal Society of Chemistry. (b) Schematic diagram of COVID-19 SERS sensor design and single-virus detection mechanism.267 (i): SARS-CoV-2 can be localized by “virus-traps” nanoforest composed of the oblique gold-nanoneedles array (GNAs). Through machine learning and identification techniques, the identification standard of virus signals is established and utilized for virus diagnoses. (ii): Schematic diagrams of single-virus detection by selectively capturing and trapping virus, and the multi-SERS enhancement mechanism. Copyright 2021 Springer Nature Limited. | |
5.6 ML-enabled detection of bacteria
SERS has many advantages in particular in terms of analysis time, sensitivity, and sample preparation, gaining tremendous interest in the past few years for the detection, identification, and quantification of bacteria. There are two main approaches to SERS analysis of bacteria: “label-free” and “label-based” methods. The “label-free” approach is based on the detection of bacterial Raman fingerprints. SERS studies using label-free methods have typically focused on the analysis of pathogenic bacteria such as Staphylococcus aureus,268–270Escherichia coli,271–275 or Salmonella typhimurium.268,270,276 SERS nanoprobes were developed and applied for indirect detection, identification, or quantification of bacteria with higher sensitivity, specificity, and reproducibility than label-free methods.277,278 Thus, in scenarios used for quantification purposes, label-based methods are more commonly used. To date, unambiguously observed spectral signatures in bacterial SERS spectra have remained difficult due to overlapping signals from various species. It is an efficient method to improve spectral classification and shorten detection time through machine learning.279,280 Researchers propose a pathogen-classification system combining machine learning and the SERS platform, expressing the task as a cost-sensitive classification problem, and taking state-of-the-art cost-sensitive classification algorithms from machine learning to complete the task.281 This study validates the usefulness of machine learning algorithms in building the system. Coincidentally, when using machine learning algorithms to analyze conditioned media from bacterial cultures in a complex environment, 1 ng mL−1 limit of detection of pyocyanin and robust quantification of concentration cover 5 orders of magnitude can be achieved.282
Rapid antimicrobial susceptibility testing (AST) is an integral tool to mitigate the unnecessary use of powerful and broad-spectrum antibiotics that leads to the proliferation of multi-drug-resistant bacteria. Using a sensor platform composed of SERS sensors (Fig. 13a-i) with control of nanogap chemistry and machine learning algorithms (Fig. 13a-ii) for analysis of complex spectral data, bacteria metabolic profiles after post-antibiotic exposure are correlated with susceptibility. DNN models can discriminate the responses of Escherichia coli and Pseudomonas aeruginosa to antibiotics from untreated cells in SERS data in 10 min after antibiotic exposure with greater than 99% accuracy. Deep learning analysis is also able to differentiate responses from untreated cells with antibiotic dosages up to 10-fold lower than the minimum inhibitory concentration observed in conventional growth assays. Unsupervised Bayesian Gaussian mixture analysis achieves 99.3% accuracy in discriminating between susceptible versus resistant to antibiotic cultures in SERS using the extended latent space. Discriminative and generative models rapidly provide high classification accuracy with small sets of labeled data, which enormously reduces the amount of time needed to validate phenotypic AST with conventional growth assays. Thus, this work outlines a promising approach toward practical rapid AST, the schematic diagram is shown in Fig. 13a-iii.283 Rahman and co-workers report the application of concanavalin A lectin-modified Bacterial cellulose nanocrystals (BCNCs) for bacterial isolation and label-free SERS detection of bacterial species using Au nanoparticles (AuNPs).284 The SERS spectral dataset was analyzed using machine learning-based SVM techniques, and the SVM classifier demonstrated high overall accuracy of 87.7% in correctly distinguishing bacterial strains, as shown in Fig. 13b.
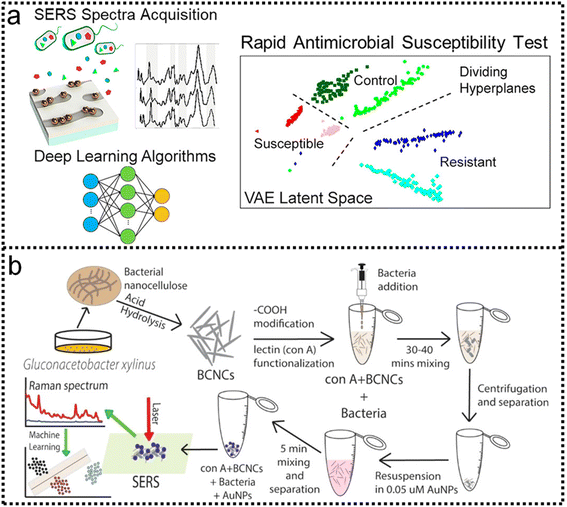 |
| Fig. 13 (a) Deep Learning Analysis of Vibrational Spectra of Bacterial Lysate for Rapid Antimicrobial Susceptibility Testing. Using a sensor platform consisting of a SERS substrate.283 (i): Sensor with nanogap chemistry control and machine learning algorithms (ii): for analyzing complex spectral data, the rapid antimicrobial susceptibility test (AST) (iii): distinguishes between E. coli and P. aeruginosa responses to antibiotics and untreated cells with over 99% accuracy in less than 10 minutes. Copyright 2022 American Chemical Society. (b) Schematic illustration of284 (i): synthesis and (ii): functionalization of BCNCs, (iii): bacteria detection assay, (iv): SERS, and v: machine-learning applications. Copyright 2020 American Chemical Society. | |
5.7 ML-enabled detection of DNA
In the previous study, many studies concerning label-free detection of unmodified DNA sequences were reported, mostly adsorbed on the surface of AgNPs or AuNPs.285,286 Modifying DNA with an extrinsic Raman label or constructing SERS nanotags is the other general approach to DNA detection, which has much broader applications than label-free methods. Wang and co-workers287 presented a hairpin DNA-assisted silicon-based SERS sensor for ultrahigh sensitivity and specificity detection of a deafness gene. This detection strategy could efficiently discriminate deafness-causing mutations in a real sample at the femtomolar level, a concentration about 100 times lower than that detected by conventional detection methods. Sensitive multiplex DNA detection using SERS biosensors is growing in popularity. SERS is an attractive method for multiplex DNA detection because it can easily differentiate between mixtures of fluorescent labels. Fast, sensitive, and accurate analysis of spectral data is another important development, and machine learning is a fascinating solution.288–294 Dixit and co-workers give a review on the mechanisms of gene sequence classification using machine learning techniques,295 which includes a brief detail on bioinformatics, a literature survey, and key issues in DNA Sequencing using Machine Learning. Taking advantage of the unique merits of SERS methodology in the collection and construction of a database (e.g., abundant intrinsic fingerprint information, noninvasive data acquisition process, strong anti-interfering ability, etc.). Based on SERS measurements showed changes in the spectra of spermatozoa, suggesting DNA damage.119 In addition, machine learning (ML) methods were used to evaluate the performance of three classification algorithms (ANN, κ-NN, and SVM) in the identification task of the groups exposed to difenoconazole. The results obtained by ML algorithms were very promising with accuracy ≥ 90% and validated the hypothesis that exposure to difenoconazole reduces sperm quality. More, the DNA probes, developed, target a specific segment of the nucleocapsid phosphoprotein (N) gene of SARS-CoV-2 with high binding efficiency.296 cervical squamous cell carcinoma (CSCC) detection.297
Nguyen and co-workers report on a room temperature inhomogeneous broadening as a function of the increased adenine concentration and employ this feature to develop one-dimensional and two-dimensional chemical composition classification models of 200 long single-stranded DNA sequences.298 Afterward, they develop a reservoir computing chemical composition classification scheme of the same molecules and demonstrate enhanced performance that does not rely on manual feature identification, as shown in Fig. 14a-iii. Taking advantage of the unique merits of SERS methodology in the collection and construction of a database (e.g., abundant intrinsic fingerprint information, noninvasive data acquisition process, strong anti-interfering ability, etc.), herein Shi et al.299 set up a SERS-based database of deoxyribonucleic acid (DNA), suitable for artificial intelligence (AI)-based sensing applications (Fig. 14b-i). The database is collected and analyzed by silver nanoparticles (Ag NPs)-decorated silicon wafer (Ag NPs@Si) SERS chip, followed by training with a deep neural network (DNN). As proof-of-concept applications, three kinds of representative tumor suppressor genes, i.e., p16, p21, and p53 fragments, are readily discriminated in a label-free manner, as shown in Fig. 14b-iv. Prominent and reproducible SERS spectra of these DNA molecules are collected and employed as input data for DNN learning and training, which enables selective discrimination of DNA target(s). The AI-based sensing method for distinguishing single DNA targets and DNA mixtures, with accuracy rates of 90.28 (Fig. 14b-iii) and 74.83%, respectively (Fig. 14b-vi).
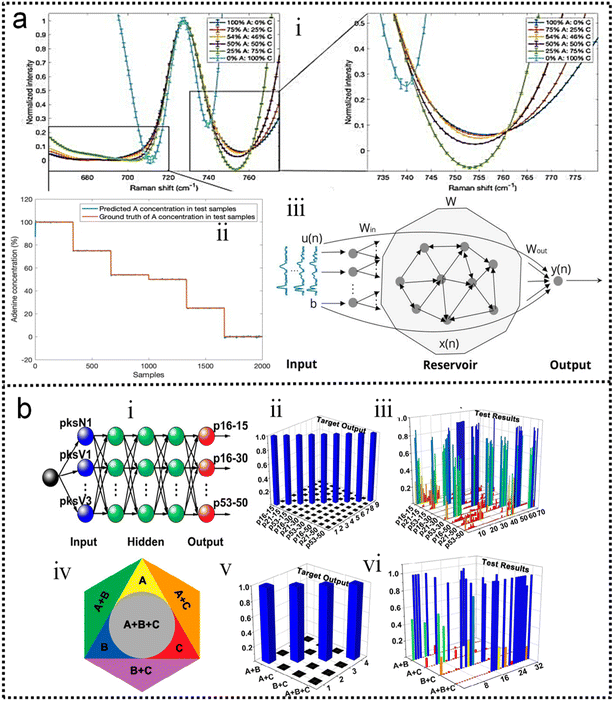 |
| Fig. 14 (a) SERS-based ssDNA composition analysis.298 (i): Experimental results of SERS spectra presenting the adenine-related peak for different adenine concentrations in ssDNA molecules. All peaks are normalized and shifted so their Raman shifts are aligned to enhance the visibility of the peak broadening. (ii): Comparison between the RC-predicted concentration of adenine bases in ssDNA molecules and the actual value. (iii): Schematic description of the proposed RC architecture for SERS spectra chemical composition analysis/classification. Copyright 2022 AIP Publishing LLC. (b) DNA discrimination from SERS database in DNN mode.299 (i): Schematic illustration of three-layer feed-forward network for DNA discrimination from SERS database. (ii): Target output for the vector definition of nine DNA targets. (iii): Test results of verification topologies after training of the deep neural network and DNA mixture discrimination from the SERS database in DNN mode. (iv): Scheme of binary and ternary mixtures of p16, p21, and p53 (30 bp). (v): Target output for the vector definition of four DNA mixture targets. (vi): Corresponding test results of verification topologies after training of the deep neural network. Copyright 2018 American Chemical Society. | |
5.8 ML-enabled disease diagnosis and therapy
SERS have been used in a variety of different applications for the detection and therapy of disease. For example, detecting the activity of intracellular enzymes,300–304 breast cancer305,306 and more. Chou and co-workers demonstrated the detection of the β-amyloid peptide, which is a key component in plaques in sufferers of Alzheimer's disease.307 Analysis of the SERS signals found that it was possible to discriminate between the different protein forms, in particular, α-helices could be discriminated from β-sheets, and between the different β-sheet forms discrimination was also possible. Having a method by which to characterize the disease process is hugely beneficial and it could be used as a method to monitor disease development. The rich and diverse applications of the remarkable SERS for disease detection are a direct result of the numerous advantages they confer, due to their specificity of spectra. Improved robustness, reliability of results through machine learning improved robustness, reliability, reproducibility, and sensitivity of results through machine learning. This technology is a major contender to improve our ability to detect diseases.
Combining machine learning algorithms and acquired SERS spectra to develop a brand new monitoring platform, Kazemzadeh and co-workers findings demonstrate the platform's ability to effectively fingerprint and efficiently classify, for the first time, three distinct subtypes of breast cancer EVs following the application.308 This platform and characterization approach will enhance the viability of EVs and nanoplasmonic sensors towards clinical utility for breast cancer and many other applications to improve human health. Grieve and co-workers developed a cell-free, label-free SERS approach using gold nanoparticles (nano SERS) to classify hematological malignancies referenced against two control cohorts: healthy and noncancer cardiovascular disease.309 A predictive model was built using machine-learning algorithms to incorporate disease burden scores for patients under standard treatment. Results show linear- and quadratic-discriminant analysis distinguished three cohorts with 69.8 and 71.4% accuracies, respectively. Koster and co-workers using label-free SERS to demonstrate that a dual-isolation method is necessary to isolate EVs from the major classes of lipoprotein.69 However, combining SERS analysis with machine learning-assisted classification, they show that the disease state is the main driver of distinction between EV samples, and is largely unaffected by choice of isolation. The study describes a convenient SERS assay to retain accurate diagnostic information from clinical samples by overcoming differences in lipoprotein contamination according to the isolation method. Shin and co-workers demonstrate an accurate diagnosis of early-stage lung cancer, using deep learning-based SERS of the exosomes, as shown in Fig. 15a.196 Their approach was to explore the features of cell exosomes through deep learning and figure out the similarity in human plasma exosomes, without learning insufficient human data. The deep learning model was trained with SERS signals of exosomes derived from normal and lung cancer cell lines and could classify them with an accuracy of 95%. In 43 patients, including stage I and II cancer patients, the deep learning model predicted that plasma exosomes of 90.7% of patients had higher similarity to lung cancer cell exosomes than the average of the healthy controls. The similarity was proportional to the progression of cancer. Notably, the model predicted lung cancer with an area under the curve (AUC) of 0.912 for the whole cohort and stage I patients with an AUC of 0.910. These results suggest the great potential of the combination of exosome analysis and deep learning as a method for early-stage liquid biopsy of lung cancer. Karunakaran and co-workers have stepped up on a strategic spectroscopic modality by utilizing the label-free ultrasensitive SERS technique to generate a differential spectral fingerprint for the prediction of normal (NRML), high-grade intraepithelial lesion (HSIL) and cervical squamous cell carcinoma (CSCC) from exfoliated cell samples of the cervix, as shown in Fig. 15b-ii.301 Three different approaches i.e., single-cell, cell-pellet and extracted DNA from the oncology clinic as confirmed by Pap test and HPV PCR were employed. Gold nanoparticles as the SERS substrate favored the increment of Raman intensity and exhibited signature identity for amide III/nucleobases and carotenoid/glycogen respectively for establishing the empirical discrimination. Moreover, all the spectral invention was subjected to chemometrics including the SVM which furnished an average diagnostic accuracy of 94%, 74%, and 92% in the three grades. Combining SERS read-out and machine learning techniques in field trials promises to reduce the incidence in low-resource countries.
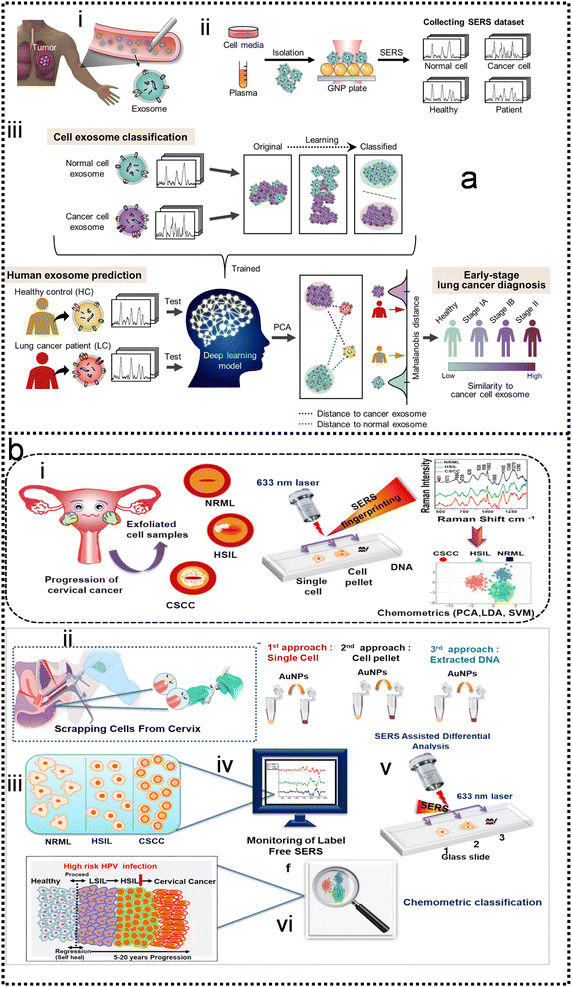 |
| Fig. 15 Machine learning-enhanced intelligent disease diagnosis and therapy in SERS. (a) Schematic illustration of deep learning-based circulating exosome analysis for lung cancer diagnosis.196 (i): Circulation of lung cancer tumor exosomes in the bloodstream. (ii): Collection of spectroscopic data of exosomes by SERS. (iii): Overview of deep learning-based cell exosome classification and lung cancer diagnosis using exosomal SERS signal patterns. Copyright 2020 American Chemical Society. (b) Schematic illustration of experimental design for differentiating three grades viz. normal (NRML), high-grade intraepithelial lesion (HSIL), cervical squamous cell carcinoma (CSCC) using SERS.301 (i): Scrapping cells from the cervix using cytobrush. (ii): Progression pattern of cervical cancer. (iii): Set 1: single cell, set 2: cell pellet, set 3: extracted DNA (mixed with AuNPs). (iv): Independent SERS analysis of (1) single cell, (2) cell pellet, (3) extracted DNA in the glass slide. (v): empirical signal monitoring of the three grades. (vi): Chemometric analysis. Copyright 2020 Elsevier. | |
The demonstration of the identification program for machine learning-augmented SERS is shown in Fig. 16. First, a large amount of measurement data is collected through the SERS substrate to form a database (Fig. 16a). Then, the SERS database provides great support for fast, sensitive, label-free, and non-destructive molecular detection and identification with the assistance of appropriate ML algorithms. Fig. 16b shows several representative ML analysis results with the best models in the corresponding application scenarios. However, choosing an appropriate algorithm for a specific database remains challenging. Because, with the large volumes of data generated during SERS analysis, not all algorithms could achieve relatively high accuracy. Many machine learning algorithms have not been explored, which may be appropriate for the analysis of Raman spectra and worthy of further investigation. Most of the diagnostic treatments for diseases are based on biomarkers, which are biological molecules produced in the body or at the patient's site of the disease. The biomarker is an indicator that evaluates normal biological processes, pathogenic processes, and response to exposure or intervention. Biomarker tests help to characterize changes in disease. Despite the availability of approved algorithms for disease diagnosis and treatment, however, many clinicians remain wary of ML because they are concerned about the “black box” nature of many ML models. While not all ML algorithms are uninterpretable, most ML algorithms that produce state-of-the-art results (including deep learning) have this limitation. The lack of interpretability of predictive models can undermine trust in these models, especially in healthcare, where many decisions are a matter of life and death, so clinician oversight is essential. “If used carefully, this technology could improve performance in health care and potentially reduce inequities”, Ghassemi says.310 “But if we're not actually careful, technology could worsen care”. Finally, we list some important related work on machine-enabled SEIRA and SERS technologies in the table for better reference, as shown in Table S1† (ESI†).
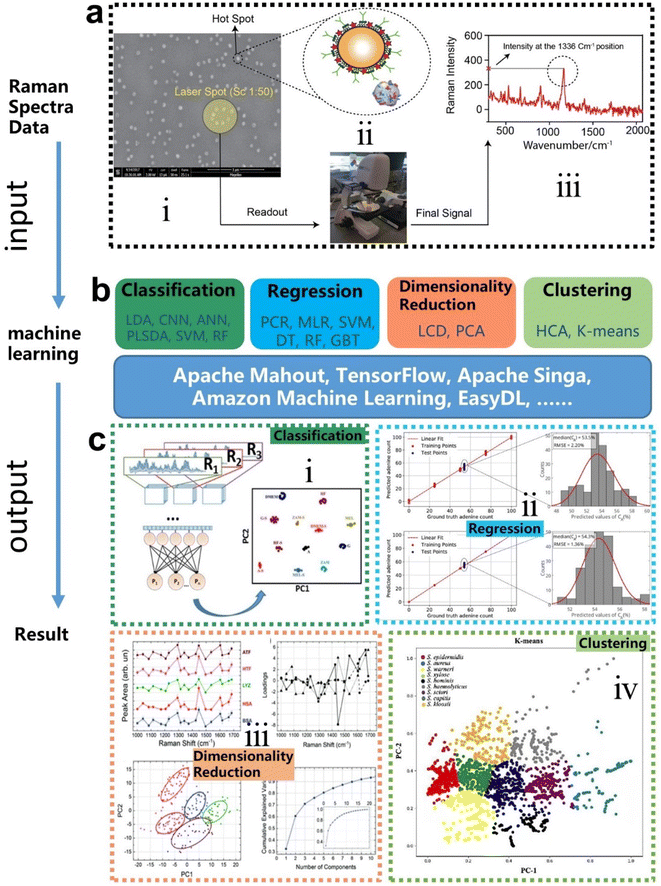 |
| Fig. 16 Flow chart of machine learning-enhanced SERS (a) the formation process of the SERS database.227 (i): SEM image of captured extrinsic Raman label on the gold-coated substrate. (ii): collecting the SERS signal using Raman spectroscopy. (iii): The concentration was quantified intensity at 1336 cm−1. Copyright 2021 John Wiley and Sons. (b) Model categories and tools of machine learning for SERS. (c) Demo for the results of different algorithms (i): classification.248 (ii): Regression.311 (iii): Dimensionality.193 (iv): Clustering.116 Copyright 2020 Optical Society of America. | |
6. Conclusions and future perspectives
In this review, we briefly investigate and offer a glance at the artificial intelligence-augmented surface-enhanced spectroscopies (SEIRA and SERS) and their applications in biomedical detection. Generally, machine learning algorithms benefit SEIRA and SERS in four ways. First, enables the automated design of SEIRA substrates to avoid time-consuming and onerous design processes. Then, it helps to reduce anomalies and artifacts and improve the ability of SEIRA/SERS to identify and classify various analytes from their overlapping spectra. Finally, it assists SERS/SEIRA to analyze and process data quickly, directly, and automatically, reducing the amount of spectral data through dimensionality reduction. Currently, as a powerful technology, ML is well integrated into SEIRA/SERS and allows their complex applications in biomedical detection, which is quite difficult and challenging for conventional SERS/SEIRA technology. Furthermore, the focus of ML combined with SEIRA is different from that with SERS. Since the design of SEIRA substrates is complex and strongly related to their patterns, ML has great potential to assist the automated design of SEIRA substrates. In terms of SERS, it is widely used in the detection of various analytes in biomedicine, so ML-enabled SERS diagnostic technology is quite promising. Furthermore, considering that sufficient data is required for ML models, substrates with multi-pixel, multi-resonant, broadband, and high-throughput characteristics are desirable for combining ML and SEIRA/SERS.
Although machine learning has improved SEIRA and SERS research, the introduction of algorithms still poses many challenges that require further investigation. The most critical issue is data preparation for training, validation, and testing. First, supervised learning algorithms are highly data-demanding. But it is difficult for operators to obtain large sets of independent SERS and SEIRA data. In most cases, large sets of data are obtained by repeated measures, which are not independent of each other. Second, the generalization ability of a well-trained model is questionable. A model that performs well on one dataset may output unsatisfactory results on another dataset due to overfitting. Finally, the results and accuracy obtained by different algorithm models are different. The selection and optimization of algorithms could increase the technical difficulty and complexity of the molecular screening process using SEIRA and SERS. Despite the challenges, the benefits of machine learning to SEIRA and SERS outweigh the disadvantages.
Going forward, ML-assisted SEIRA and SERS are attractive for point-of-care testing (POCT). POCT is a medical diagnostic test performed at the time and place of patient care. It provides rapid test results and has the potential to improve patient care. The huge market of POCT makes it a good choice for the practical application and commercialization of SEIRA and SERS technologies. To ensure the correctness of the diagnostic results, POCT has high requirements on the stability, accuracy, and speed of the detection. The rapid, automated and noise-reduced technological advantages of ML can improve the performance in these aspects, thereby representing an opportunity for the evolution of SEIRA into POCT. In particular, portable Raman/IR spectrometers integrated with ML algorithms can serve as powerful tools for SERS/SEIRA substrates regarding direct signal readout, fast data processing, and accurate decision-making. In this case, it could advance SERS/SEIRA-based POCT towards home testing or self-testing.
All in all, ML-based SEIRA and SERS technologies have achieved breakthroughs in the past 15 years, including automated design of complex substrates, signal processing, decision-making, and more. However, the demand for molecular diagnostics has changed dramatically in recent years, such as demographic shifts, new infectious agents, and labor shortages in the healthcare industry. All of these will drive advances in diagnostic technology, from the laboratory to the field or POCT, manual processing to unattended operation, single-function testing to all-in-one diagnostics,312 and more. It is promising that SEIRA and SERS technologies embrace ML algorithms to address challenges and move toward next-generation molecular diagnostics.
Conflicts of interest
There are no conflicts to declare.
Acknowledgements
This work is supported by the RIE Advanced Manufacturing and Engineering (AME) Programmatic Grant Project (Grant A18A5b0056, WBS: A-0005117-02-00), and the Advanced Research and Technology Innovation Centre (ARTIC) Project (WBS: A-0005947-20-00).
References
- J. Langer, D. J. de Aberasturi, J. Aizpurua, R. A. Alvarez-Puebla, B. Auguie, J. J. Baumberg, G. C. Bazan, S. E. J. Bell, A. Boisen, A. G. Brolo, J. Choo, D. Cialla-May, V. Deckert, L. Fabris, K. Faulds, F. J. G. de Abajo, R. Goodacre, D. Graham, A. J. Haes, C. L. Haynes, C. Huck, T. Itoh, M. Ka, J. Kneipp, N. A. Kotov, H. Kuang, E. C. Le Ru, H. K. Lee, J.-F. Li, X. Y. Ling, S. A. Maier, T. Mayerhofer, M. Moskovits, K. Murakoshi, J.-M. Nam, S. Nie, Y. Ozaki, I. Pastoriza-Santos, J. Perez-Juste, J. Popp, A. Pucci, S. Reich, B. Ren, G. C. Schatz, T. Shegai, S. Schlucker, L.-L. Tay, K. G. Thomas, Z.-Q. Tian, R. P. Van Duyne, T. Vo-Dinh, Y. Wang, K. A. Willets, C. Xu, H. Xu, Y. Xu, Y. S. Yamamoto, B. Zhao and L. M. Liz-Marzan, ACS Nano, 2020, 14, 28–117 CrossRef CAS PubMed.
- E. J. Osley, C. G. Biris, P. G. Thompson, R. R. F. Jahromi, P. A. Warburton and N. C. Panoiu, Phys. Rev. Lett., 2013, 110, 087402 CrossRef CAS PubMed.
- E. Fort and S. Grésillon, J. Phys. D: Appl. Phys., 2008, 41, 013001 CrossRef.
- H. Zhou, D. Li, Z. Ren, X. Mu and C. Lee, InfoMat, 2022, e12349 CAS.
- H. Zhou, D. Li, X. Hui and X. Mu, Int. J. Optomechatronics, 2021, 15, 97–119 CrossRef.
- D. Li, H. Zhou, X. Hui, X. He, H. Huang, J. Zhang, X. Mu, C. Lee and Y. Yang, Adv. Sci., 2021, 8, e2101879 CrossRef PubMed.
- X. Hui, C. Yang, D. Li, X. He, H. Huang, H. Zhou, M. Chen, C. Lee and X. Mu, Adv. Sci., 2021, 8, e2100583 CrossRef PubMed.
- H. Zhou, X. Hui, D. Li, D. Hu, X. Chen, X. He, L. Gao, H. Huang, C. Lee and X. Mu, Adv. Sci., 2020, 7, 2001173 CrossRef CAS PubMed.
- J. F. Li, Y. J. Zhang, S. Y. Ding, R. Panneerselvam and Z. Q. Tian, Chem. Rev., 2017, 117, 5002–5069 CrossRef CAS PubMed.
- M. Fleischmann, P. J. Hendra and A. J. McQuillan, Chem. Phys. Lett., 1974, 26, 163–166 CrossRef CAS.
- D. L. Jeanmaire and R. P. V. Duyne, J. Electroanal. Chem. Interfacial Electrochem., 1977, 84, 1–20 CrossRef CAS.
- A. Hartstein, J. R. Kirtley and J. C. Tsang, Phys. Rev. Lett., 1980, 45, 201–204 CrossRef CAS.
- K. Kneipp, Y. Wang, H. Kneipp, L. T. Perelman, I. Itzkan, R. R. Dasari and M. S. Feld, Phys. Rev. Lett., 1997, 78, 1667–1670 CrossRef CAS.
- M. S. Anderson, Appl. Phys. Lett., 2000, 76, 3130–3132 CrossRef CAS.
- B. Knoll and F. Keilmann, Nature, 1999, 399, 134–137 CrossRef CAS.
- F. Neubrech, A. Pucci, T. W. Cornelius, S. Karim, A. Garcia-Etxarri and J. Aizpurua, Phys. Rev. Lett., 2008, 101, 157403 CrossRef PubMed.
- J.-T. Liu, N.-H. Liu, J. Li, X. Jing Li and J.-H. Huang, Appl. Phys. Lett., 2012, 101, 052104 CrossRef.
- C. K. Akhgar, G. Ramer, M. Zbik, A. Trajnerowicz, J. Pawluczyk, A. Schwaighofer and B. Lendl, Anal. Chem., 2020, 92, 9901–9907 CrossRef CAS PubMed.
- R. Ramos and M. J. Gordon, Appl. Phys. Lett., 2012, 100, 213111 CrossRef.
- F. Zangeneh-Nejad, D. L. Sounas, A. Alu and R. Fleury, Nat. Rev. Mater., 2021, 6, 207–225 CrossRef.
- R. G. Brereton, J. Chemom., 2014, 28, 749–760 CrossRef CAS.
- S. Stewart, M. A. Ivy and E. V. Anslyn, Chem. Soc. Rev., 2014, 43, 70–84 RSC.
- K. L. Diehl and E. V. Anslyn, Chem. Soc. Rev., 2013, 42, 8596–8611 RSC.
- B. Huang, Z. Li and J. Li, Nanoscale, 2018, 10, 21320–21326 RSC.
- D. Jakhar and I. Kaur, Clin. Exp. Dermatol., 2020, 45, 131–132 CrossRef CAS PubMed.
- C. L. Lin, S. S. Liang, Y. S. Peng, L. Long, Y. Y. Li, Z. R. Huang, N. V. Long, X. Y. Luo, J. J. Liu, Z. Y. Li and Y. Yang, Nano-Micro Lett., 2022, 14, 75 CrossRef CAS PubMed.
- N. Shauloff, A. Morag, K. Yaniv, S. Singh, R. Malishev, O. Paz-Tal, L. Rokach and R. Jelinek, Nano-Micro Lett., 2021, 13, 112 CrossRef CAS PubMed.
- Z. Y. Shi, L. X. Meng, X. L. Shi, H. P. Li, J. Z. Zhang, Q. Q. Sun, X. Y. Liu, J. Z. Chen and S. R. Liu, Nano-Micro Lett., 2022, 14, 141 CrossRef CAS PubMed.
- C. Wei, W. S. Lin, S. F. Liang, M. J. Chen, Y. J. Zheng, X. Q. Liao and Z. Chen, Nano-Micro Lett., 2022, 14, 131 CrossRef CAS PubMed.
- W. Q. Yan, K. Wang, H. Xu, X. Y. Huo, Q. H. Jin and D. X. Cui, Nano-Micro Lett., 2019, 11, 7 CrossRef CAS PubMed.
- Y. Yang, Y. S. Peng, C. L. Lin, L. Long, J. Y. Hu, J. He, H. Zeng, Z. R. Huang, Z. Y. Li, M. Tanemura, J. L. Shi, J. R. Lombardi and X. Y. Luo, Nano-Micro Lett., 2021, 13, 109 CrossRef CAS PubMed.
- X. Yin, J. Yang, F. Xiao, Y. Yang and H. B. Shen, Nano-Micro Lett., 2018, 10, 2 CrossRef PubMed.
- B. W. Zheng and G. X. Gu, Nano-Micro Lett., 2020, 12, 181 CrossRef CAS PubMed.
- I. Kononenko, Artif. Intell. Med., 2001, 23, 89–109 CrossRef CAS PubMed.
- Z. S. Ballard, D. Shir, A. Bhardwaj, S. Bazargan, S. Sathianathan and A. Ozcan, ACS Nano, 2017, 11, 2266–2274 CrossRef CAS PubMed.
- M. Kotzabasaki, I. Sotiropoulos, C. Charitidis and H. Sarimveis, Nanoscale Adv., 2021, 3, 3167–3176 RSC.
- J. Z. Cai, X. Chu, K. Xu, H. B. Li and J. Wei, Nanoscale Adv., 2020, 2, 3115–3130 RSC.
- H. T. Wen, X. X. Xu, S. S. Cheong, S. C. Lo, J. H. Chen, S. L. Y. Chang and C. Dwyer, Nanoscale Adv., 2021, 3, 6956–6964 RSC.
- L. D. Geoffrion and G. Guisbiers, Nanoscale Adv., 2021, 3, 4254–4270 RSC.
- H. P. Su, S. Lin, S. W. Deng, C. Lian, Y. Z. Shang and H. L. Liu, Nanoscale Adv., 2019, 1, 2162–2166 RSC.
- A. Chandrashekar, P. Belardinelli, M. A. Bessa, U. Staufer and F. Alijani, Nanoscale Adv., 2022, 4, 2134–2143 RSC.
- A. R. Wei, H. Ye, Z. L. Guo and J. Xiong, Nanoscale Adv., 2022, 4, 1455–1463 RSC.
- S. M. Neumayer, S. Jesse, G. Velarde, A. L. Kholkin, I. Kravchenko, L. W. Martin, N. Balke and P. Maksymovych, Nanoscale Adv., 2020, 2, 2063–2072 RSC.
- Q. X. Luo, E. A. Holm and C. Wang, Nanoscale Adv., 2021, 3, 206–213 RSC.
- N. M. Ralbovsky and I. K. Lednev, Chem. Soc. Rev., 2020, 49, 7428–7453 RSC.
- A. Chen, X. Zhang and Z. Zhou, InfoMat, 2020, 2, 553–576 CrossRef CAS.
- J. D. Dan, X. X. Zhao and S. J. Pennycook, InfoMat, 2019, 1, 359–375 CrossRef CAS.
- W. X. Sha, Y. Li, S. Tang, J. Tian, Y. M. Zhao, Y. Q. Guo, W. X. Zhang, X. F. Zhang, S. F. Lu, Y. C. Cao and S. J. Cheng, InfoMat, 2021, 3, 353–361 CrossRef CAS.
- J. Wei, X. Chu, X. Y. Sun, K. Xu, H. X. Deng, J. G. Chen, Z. M. Wei and M. Lei, InfoMat, 2019, 1, 338–358 CrossRef CAS.
- M. Xu, M. Xu and X. S. Miao, InfoMat, 2022, 4, e12315 CAS.
- C. Eliasson, A. Loren, K. V. Murty, M. Josefson, M. Kall, J. Abrahamsson and K. Abrahamsson, Spectrochim. Acta, Part A, 2001, 57, 1907–1915 CrossRef CAS PubMed.
- R. M. Jarvis and R. Goodacre, Anal. Chem., 2004, 76, 40–47 CrossRef CAS PubMed.
- A. Walter, A. Marz, W. Schumacher, P. Rosch and J. Popp, Lab Chip, 2011, 11, 1013–1021 RSC.
- X. Li, T. Yang, S. Li, L. Jin, D. Wang, D. Guan and J. Ding, Opt. Express, 2015, 23, 18361–18372 CrossRef CAS PubMed.
- W. J. Thrift and R. Ragan, Anal. Chem., 2019, 91, 13337–13342 CrossRef CAS PubMed.
- L. Kühner, R. Semenyshyn, M. Hentschel, F. Neubrech, C. Tarin and H. Giessen, ACS Sens., 2019, 4, 1973–1979 CrossRef PubMed.
-
S. H. Huang, R. Delgado and G. Shvets, in Biomedical Vibrational Spectroscopy 2020: Advances in Research and Industry, ed. W. Petrich and Z. Huang, SPIE, San Francisco, 2020, p. 1123601 Search PubMed.
- A. John-Herpin, D. Kavungal, L. von Mucke and H. Altug, Adv. Mater., 2021, 33, e2006054 CrossRef PubMed.
- S. Bashir, S. Ali, H. Nawaz, M. I. Majeed, M. Mohsin, A. Nawaz, N. Rashid, F. Tahir, A. u. Haq, M. Saleem, M. Z. Nawaz and K. Shahzad, Anal. Lett., 2022, 55, 1833–1845 CrossRef CAS.
- E. Corcione, D. Pfezer, M. Hentschel, H. Giessen and C. Tarin, Sensors, 2022, 22, 7 CrossRef CAS PubMed.
- A. I. Perez-Jimenez, D. Lyu, Z. Lu, G. Liu and B. Ren, Chem. Sci., 2020, 11, 4563–4577 RSC.
- H. L. Wang, E. M. You, R. Panneerselvam, S. Y. Ding and Z. Q. Tian, Light: Sci. Appl., 2021, 10, 161 CrossRef CAS PubMed.
- D. Hasan and C. Lee, Adv. Sci., 2018, 5, 1700581 CrossRef PubMed.
- J. Wei, Y. Li, Y. Chang, D. M. N. Hasan, B. Dong, Y. Ma, C. W. Qiu and C. Lee, ACS Appl. Mater. Interfaces, 2019, 11, 47270–47278 CrossRef CAS PubMed.
- Y. Chang, D. Hasan, B. Dong, J. Wei, Y. Ma, G. Zhou, K. W. Ang and C. Lee, ACS Appl. Mater. Interfaces, 2018, 10, 38272–38279 CrossRef CAS PubMed.
- J. Xu, Z. Ren, B. Dong, X. Liu, C. Wang, Y. Tian and C. Lee, ACS Nano, 2020, 14, 12159–12172 CrossRef CAS PubMed.
- W. X. Liu, Y. M. Ma, X. M. Liu, J. K. Zhou, C. Xu, B. W. Dong and C. K. Lee, Nano Lett., 2022, 22, 6112–6120 CrossRef CAS PubMed.
- W. Liu, Y. Ma, Y. Chang, B. Dong, J. Wei, Z. Ren and C. Lee, Nanophotonics, 2021, 10, 1861–1870 CrossRef CAS.
- H. J. Koster, T. Rojalin, A. Powell, D. Pham, R. R. Mizenko, A. C. Birkeland and R. P. Carney, Nanoscale, 2021, 13, 14760–14776 RSC.
- N. M. Culum, T. T. Cooper, G. A. Lajoie, T. Dayarathna, S. H. Pasternak, J. Liu, Y. Fu, L.-M. Postovit and F. Lagugne-Labarthet, Analyst, 2021, 146, 7194–7206 RSC.
- G. C. Phan-Quang, N. Yang, H. K. Lee, H. Y. F. Sim, C. S. L. Koh, Y. C. Kao, Z. C. Wong, E. K. M. Tan, Y. E. Miao, W. Fan, T. Liu, I. Y. Phang and X. Y. Ling, ACS Nano, 2019, 13, 12090–12099 CrossRef CAS PubMed.
- S. Yan, J. Qiu, L. Guo, D. Li, D. Xu and Q. Liu, Appl. Microbiol. Biotechnol., 2021, 105, 1315–1331 CrossRef CAS PubMed.
- J. Wei, C. Xu, B. Dong, C.-W. Qiu and C. Lee, Nat. Photonics, 2021, 15, 614–621 CrossRef CAS.
- Z. Ren, B. Dong, Q. Qiao, X. Liu, J. Liu, G. Zhou and C. Lee, InfoMat, 2021, 4, e12264 Search PubMed.
- X. Liu, W. Liu, Z. Ren, Y. Ma, B. Dong, G. Zhou and C. Lee, Int. J. Optomechatronics, 2021, 15, 120–159 CrossRef.
- Z. Ren, J. Xu, X. Le and C. Lee, Micromachines, 2021, 12, 946 CrossRef PubMed.
- J. Wei, Z. Ren and C. Lee, J. Appl. Phys., 2020, 128, 240901 CrossRef CAS.
- J. Wei, Y. Li, L. Wang, W. Liao, B. Dong, C. Xu, C. Zhu, K. W. Ang, C. W. Qiu and C. Lee, Nat. Commun., 2020, 11, 6404 CrossRef CAS PubMed.
- Y. H. Chang, J. X. Wei and C. K. Lee, Nanophotonics, 2020, 9, 3049–3070 CrossRef.
- Z. Ren, Y. Chang, Y. Ma, K. Shih, B. Dong and C. Lee, Adv. Opt. Mater., 2019, 8, 1900653 CrossRef.
- H. Zhou, C. Yang, D. Hu, D. Li, X. Hui, F. Zhang, M. Chen and X. Mu, Appl. Phys. Lett., 2019, 115, 143507 CrossRef.
- H. Zhou, C. Yang, D. Hu, S. Dou, X. Hui, F. Zhang, C. Chen, M. Chen, Y. Yang and X. Mu, ACS Appl. Mater. Interfaces, 2019, 11, 14630–14639 CrossRef CAS PubMed.
- H. Zhou, D. Hu, C. Yang, C. Chen, J. Ji, M. Chen, Y. Chen, Y. Yang and X. Mu, Sci. Rep., 2018, 8, 14801 CrossRef PubMed.
- C. Xu, Z. Ren, J. Wei and C. Lee, iScience, 2022, 25, 103799 CrossRef CAS PubMed.
- J. Kim, Y. Yang, T. Badloe, I. Kim, G. Yoon and J. Rho, InfoMat, 2021, 3, 739–754 CrossRef.
- F. Zhang, X. Wang, C. Chen, H. Zhou, J. Yang, L. Bai, Y. Xu, Y. Cheng, S. Zhang and X. Mu, IEEE Trans. Ultrason. Eng., 2019, 67, 131–135 Search PubMed.
- F. Zhang, C. Chen, S. Dou, H. Zhou, J. Yang, D. Wang, Y. Chen, Y. Cheng, Z. Shang and X. Mu, Appl. Phys. Lett., 2018, 113, 203502 CrossRef.
- S. Dou, M. Qi, C. Chen, H. Zhou, Y. Wang, Z. Shang, J. Yang, D. Wang and X. Mu, AIP Adv., 2018, 8, 065315 CrossRef.
- S. Dou, J. Cao, H. Zhou, C. Chen, Y. Wang, J. Yang, D. Wang, Z. Shang and X. Mu, Appl. Phys. Lett., 2018, 113, 093502 CrossRef.
- C. Chen, Z. Shang, F. Zhang, H. Zhou, J. Yang, D. Wang, Y. Chen and X. Mu, Appl. Phys. Lett., 2018, 112, 243501 CrossRef.
- C. Chen, Z. Shang, J. Gong, F. Zhang, H. Zhou, B. Tang, Y. Xu, C. Zhang, Y. Yang and X. Mu, ACS Appl. Mater. Interfaces, 2018, 10, 1819–1827 CrossRef CAS PubMed.
- C. Chen, L. Bai, J. Zhang, L. L. Tian, Q. Zhou, H. Zhou, D. Li and X. Mu, IEEE Trans. Ultrason. Eng., 2022, 69, 1452–1460 Search PubMed.
- Y.-S. Lin and Z. Xu, Int. J. Optomechatronics, 2020, 14, 78–93 CrossRef.
- J. Xu, Y. Du, Y. Tian and C. Wang, Int. J. Optomechatronics, 2021, 14, 94–118 CrossRef.
- G. Zhou, Z. H. Lim, Y. Qi, F. S. Chau and G. Zhou, Int. J. Optomechatronics, 2021, 15, 61–86 CrossRef.
- D. Hasan, P. Pitchappa, J. Wang, T. Wang, B. Yang, C. P. Ho and C. Lee, ACS Photonics, 2017, 4, 302–315 CrossRef CAS.
- Q. Qiao, X. Liu, Z. Ren, B. Dong, J. Xia, H. Sun, C. Lee and G. Zhou, ACS Photonics, 2022, 9, 2367–2377 CrossRef CAS.
- N. Chen, D. Hasan, C. P. Ho and C. Lee, Adv. Mater. Technol., 2018, 3, 1800014 CrossRef.
- F. A. Hassani, G. G. L. Gammad, R. P. Mogan, T. K. Ng, T. L. C. Kuo, L. G. Ng, P. Luu, N. V. Thakor, S. C. Yen and C. Lee, Adv. Mater. Technol., 2018, 3, 1700184 CrossRef.
- Y. M. Ma, B. W. Dong, J. X. Wei, Y. H. Chang, L. Huang, K. W. Ang and C. Lee, Adv. Opt. Mater., 2020, 8, 24856–24863 Search PubMed.
- M. Manjappa, P. Pitchappa, N. Wang, C. Lee and R. Singh, Adv. Opt. Mater., 2018, 6, 1800141 CrossRef.
- P. Pitchappa, A. Kumar, H. D. Liang, S. Prakash, N. Wang, A. A. Bettiol, T. Venkatesan, C. K. Lee and R. Singh, Adv. Opt. Mater., 2020, 8, 2000101 CrossRef CAS.
- L. Huang, B. Dong, X. Guo, Y. Chang, N. Chen, X. Huang, W. Liao, C. Zhu, H. Wang, C. Lee and K. W. Ang, ACS Nano, 2018, 13, 913–921 CrossRef PubMed.
- Y. Ma, Y. Chang, B. Dong, J. Wei, W. Liu and C. Lee, ACS Nano, 2021, 15, 10084–10094 CrossRef PubMed.
- L. Huang, W. C. Tan, L. Wang, B. Dong, C. Lee and K.-W. Ang, ACS Appl. Mater. Interfaces, 2017, 9, 36130–36136 CrossRef CAS PubMed.
- Y. P. Ma, X. J. Shao, J. Li, B. W. Dong, Z. L. Hu, Q. L. Zhou, H. M. Xu, X. X. Zhao, H. Y. Fang, X. Z. Li, Z. J. Li, J. Wu, M. Zhao, S. J. Pennycook, C. H. Sow, C. Lee, Y. L. Zhong, J. P. Lu, M. N. Ding, K. D. Wang, Y. Li and J. Lu, ACS Appl. Mater. Interfaces, 2021, 13, 8518–8527 CrossRef CAS PubMed.
- J. X. Wei, Y. Li, Y. H. Chang, D. M. N. Hasan, B. W. Dong, Y. M. Ma, C. W. Qiu and C. Lee, ACS Appl. Mater. Interfaces, 2019, 11, 47270–47278 CrossRef CAS PubMed.
- N. S. Mueller and S. Reich, Front. Chem., 2019, 7, 470 CrossRef CAS PubMed.
- S. Fan, W. Suh and J. D. Joannopoulos, J. Opt. Soc. Am. A, 2003, 20, 569–572 CrossRef PubMed.
- H. Zhou, Z. Ren, C. Xu, L. Xu and C. Lee, Nano-Micro Lett., 2022, 14, 207 CrossRef CAS PubMed.
- B. T. O'Callahan, M. Hentschel, M. B. Raschke, P. Z. El-Khoury and A. S. Lea, J. Phys. Chem. C, 2019, 123, 17505–17509 CrossRef.
- J.-W. Tang, J.-Q. Li, X.-C. Yin, W.-W. Xu, Y.-C. Pan, Q.-H. Liu, B. Gu, X. Zhang and L. Wang, Front. Microbiol., 2022, 13, 843417 Search PubMed.
- A. Rahman, S. Kang, W. Wang, Q. Huang, I. Kim and P. J. Vikesland, ACS Appl. Nano Mater., 2022, 5, 259–268 CrossRef CAS.
- S. Park, J. Lee, S. Khan, A. Wahab and M. Kim, Sensors, 2022, 22, 596 CrossRef CAS PubMed.
- P. Moitra, A. Chaichi, S. M. Abid Hasan, K. Dighe, M. Alafeef, A. Prasad, M. R. Gartia and D. Pan, Biosens. Bioelectron., 2022, 208, 114200 CrossRef CAS PubMed.
- J.-W. Tang, Q.-H. Liu, X.-C. Yin, Y.-C. Pan, P.-B. Wen, X. Liu, X.-X. Kang, B. Gu, Z.-B. Zhu and L. Wang, Front. Microbiol., 2021, 12, 696921 CrossRef PubMed.
- H. Li, Y. Cao and F. Lu, J. Innovative Opt. Health Sci., 2021, 14, 2141002 CrossRef.
- F. U. Ciloglu, A. M. Saridag, I. H. Kilic, M. Tokmakci, M. Kahraman and O. Aydin, Analyst, 2020, 145, 7559–7570 RSC.
- V. R. Pereira, D. R. Pereira, K. C. de Melo Tavares Vieira, V. P. Ribas, C. J. Leopoldo Constantino, P. A. Antunes and A. P. Alves Favareto, Environ. Sci. Pollut. Res., 2019, 26, 35253–35265 CrossRef CAS PubMed.
- W. Hu, S. Ye, Y. Zhang, T. Li, G. Zhang, Y. Luo, S. Mukamel and J. Jiang, J. Phys. Chem. Lett., 2019, 10, 6026–6031 CrossRef CAS PubMed.
- Z. Ren, Z. Zhang, J. Wei, B. Dong and C. Lee, Nat. Commun., 2022, 13, 3859 CrossRef CAS PubMed.
- J. Meng, J. J. Cadusch and K. B. Crozier, ACS Photonics, 2021, 8, 648–657 CrossRef CAS.
- S. V. Kalinin, K. M. Roccapriore, S. H. Cho, D. J. Milliron, R. Vasudevan, M. Ziatdinov and J. A. Hachtel, Adv. Opt. Mater., 2021, 9, 2001808 CrossRef CAS.
- J. L. Lansford and D. G. Vlachos, Nat. Commun., 2020, 11, 1513 CrossRef CAS PubMed.
- F. Cui, Y. Yue, Y. Zhang, Z. Zhang and H. S. Zhou, ACS Sens., 2020, 5, 3346–3364 CrossRef CAS PubMed.
- D. Li, H. Zhou, X. Hui, X. He and X. Mu, Anal. Chem., 2021, 93, 9437–9444 CrossRef CAS PubMed.
- O. Khatib, S. Ren, J. Malof and W. J. Padilla, Adv. Opt. Mater., 2022, 10, 2200097 CrossRef CAS.
- F. Lussier, D. Missirlis, J. P. Spatz and J.-F. Masson, ACS Nano, 2019, 13, 1403–1411 CAS.
- Y. X. Leong, Y. H. Lee, C. S. L. Koh, G. C. Phan-Quang, X. Han, I. Y. Phang and X. Y. Ling, Nano Lett., 2021, 21, 2642–2649 CrossRef CAS PubMed.
- M. L. Giger, J. Am. Coll. Radiol., 2018, 15, 512–520 CrossRef PubMed.
- A. Morellos, X.-E. Pantazi, D. Moshou, T. Alexandridis, R. Whetton, G. Tziotzios, J. Wiebensohn, R. Bill and A. M. Mouazen, Biosyst. Eng., 2016, 152, 104–116 CrossRef.
- S. K. Gahlaut, D. Savargaonkar, C. Sharan, S. Yadav, P. Mishra and J. P. Singh, Anal. Chem., 2020, 92, 2527–2534 CrossRef CAS.
- Q. F. Shi, Z. D. Sun, Z. X. Zhang and C. Lee, Research, 2021, 2021, 6849171 CAS.
- L. Liu, X. G. Guo and C. Lee, Nano Energy, 2021, 88, 106304 CrossRef CAS.
- L. Liu, X. Guo, W. Liu and C. Lee, Nanomaterials, 2021, 11, 2975 CrossRef CAS.
- A. Haroun, X. Le, S. Gao, B. Dong, T. He, Z. Zhang, F. Wen, S. Xu and C. Lee, Nano Express, 2021, 2, 022005 CrossRef.
- B. Dong, Q. Shi, Y. Yang, F. Wen, Z. Zhang and C. Lee, Nano Energy, 2021, 79, 105414 CrossRef CAS.
- F. Wen, T. He, H. Liu, H. Chen, T. Zhang and C. Lee, Nano Energy, 2020, 78, 105155 CrossRef CAS.
- Q. F. Shi, B. W. Dong, T. Y. Y. He, Z. D. Sun, J. X. Zhu, Z. X. Zhang and C. Lee, InfoMat, 2020, 2, 1131–1162 CrossRef.
- Q. Shi, Z. Zhang, T. He, Z. Sun, B. Wang, Y. Feng, X. Shan, B. Salam and C. Lee, Nat. Commun., 2020, 11, 4609 CrossRef CAS.
- C. Qiu, F. Wu, C. Lee and M. R. Yuce, Nano Energy, 2020, 70, 104456 CrossRef CAS.
- S. Lee, Q. Shi and C. Lee, APL Mater., 2019, 7, 031302 CrossRef.
- Z. Zhang, F. Wen, Z. Sun, X. Guo, T. He and C. Lee, Adv. Intell. Syst., 2022, 4, 2100228 CrossRef.
- Q. Zhang, T. Jin, J. Cai, L. Xu, T. He, T. Wang, Y. Tian, L. Li, Y. Peng and C. Lee, Adv. Sci., 2022, 9, 2103694 CrossRef CAS PubMed.
- M. Zhu, Z. Sun, T. Chen and C. Lee, Nat. Commun., 2021, 12, 2692 CrossRef CAS PubMed.
- T. He and C. Lee, IEEE Open J. Circuits Syst., 2021, 2, 702–720 Search PubMed.
- Y. Chang, S. Xu, B. Dong, J. Wei, X. Le, Y. Ma, G. Zhou and C. Lee, Nano Energy, 2021, 89, 106446 CrossRef CAS.
- M. Zhu, Z. Sun, Z. Zhang, Q. Shi, T. He, H. Liu, T. Chen and C. Lee, Sci. Adv., 2020, 6, eaaz8693 CrossRef CAS PubMed.
- Z. Zhang, T. He, M. Zhu, Z. Sun, Q. Shi, J. Zhu, B. Dong, M. Yuce and C. Lee, npj Flexible Electron., 2020, 4, 29 CrossRef.
- F. Wen, Z. Sun, T. He, Q. Shi, M. Zhu, Z. Zhang, L. Li, T. Zhang and C. Lee, Adv. Sci., 2020, 7, 2000261 CrossRef CAS PubMed.
- B. Dong, Q. Shi, T. He, S. Zhu, Z. Zhang, Z. Sun, Y. Ma, D. L. Kwong and C. Lee, Adv. Sci., 2020, 7, 1903636 CrossRef CAS PubMed.
- T. He, Z. Sun, Q. Shi, M. Zhu, D. Anaya, M. Xu, T. Chen, M. Yuce, A. Thean and C. Lee, Nano Energy, 2019, 58, 641–651 CrossRef CAS.
- T. He, H. Wang, J. Wang, X. Tian, F. Wen, Q. Shi, J. S. Ho and C. Lee, Adv. Sci., 2019, 6, 1901437 CrossRef CAS PubMed.
- T. Chen, M. Y. Zhao, Q. F. Shi, Z. Yang, H. C. Liu, L. N. Sun, J. Y. Ouyang and C. K. Lee, Nano Energy, 2018, 51, 162–172 CrossRef CAS.
- T. Chen, Q. F. Shi, M. L. Zhu, T. Y. Y. He, L. N. Sun, L. Yang and C. Lee, ACS Nano, 2018, 12, 11561–11571 CrossRef CAS PubMed.
- Y. Wang, Z. Y. Hu, J. P. Wang, X. Y. Liu, Q. F. Shi, Y. W. Wang, L. Qiao, Y. H. Li, H. Y. Yang, J. H. Liu, L. Y. Zhou, Z. Q. Yang, C. K. Lee and M. Y. Xu, ACS Appl. Mater. Interfaces, 2022, 14, 24832–24839 CrossRef CAS PubMed.
- M. Zhu, Z. Sun and C. Lee, ACS Nano, 2022, 16, 14097–14110 CrossRef CAS.
- F. Wen, Z. Sun, T. He, Q. Shi, M. Zhu, Z. Zhang, L. Li, T. Zhang and C. Lee, Adv. Sci., 2020, 7, 2000261 CrossRef CAS PubMed.
- B. Dong, Z. Zhang, Q. Shi, J. Wei, Y. Ma, Z. Xiao and C. Lee, Sci. Adv., 2022, 8, eabl9874 CrossRef CAS PubMed.
- Z. Q. Bai, T. Y. Y. He, Z. X. Zhang, Y. L. Xu, Z. Zhang, Q. F. Shi, Y. Q. Yang, B. G. Zhou, M. L. Zhu, J. S. Guo and C. Lee, Nano Energy, 2022, 94, 106956 CrossRef CAS.
- Z. X. Zhang, Q. F. Shi, T. Y. Y. He, X. G. Guo, B. W. Dong, J. Lee and C. Lee, Nano Energy, 2021, 90, 106517 CrossRef CAS.
- Z. Sun, M. Zhu, Z. Zhang, Z. Chen, Q. Shi, X. Shan, R. Yeow and C. Lee, Adv. Sci., 2021, 8, 2100230 CrossRef PubMed.
- L. Liu, Q. F. Shi, Z. D. Sun and C. K. Lee, Nano Energy, 2021, 86, 106154 CrossRef CAS.
- M. Zhu, T. He and C. Lee, Appl. Phys. Rev., 2020, 7, 031305 CAS.
- G. Tang, Q. Shi, Z. Zhang, T. He, Z. Sun and C. Lee, Nano Energy, 2020, 81, 105582 CrossRef.
- H. C. Liu, W. Dong, Y. F. Li, F. Q. Li, J. J. Geng, M. L. Zhu, T. Chen, H. M. Zhang, L. N. Sun and C. K. Lee, Microsyst. Nanoeng., 2020, 6, 16 CrossRef PubMed.
- B. Dong, Y. Yang, Q. Shi, S. Xu, Z. Sun, S. Zhu, Z. Zhang, D.-L. Kwong, G. Zhou, K.-W. Ang and C. Lee, ACS Nano, 2020, 14, 8915–8930 CrossRef CAS PubMed.
- Q. F. Shi, Z. X. Zhang, T. Chen and C. K. Lee, Nano Energy, 2019, 62, 355–366 CrossRef CAS.
- M. Zhu, Q. Shi, T. He, Z. Yi, Y. Ma, B. Yang, T. Chen and C. Lee, ACS Nano, 2019, 13, 1940–1952 CAS.
- X. Le, Q. Shi, Z. Sun, J. Xie and C. Lee, Adv. Sci., 2022, 9, 2201056 CrossRef CAS PubMed.
- M. Zhu, Z. Yi, B. Yang and C. Lee, Nano Today, 2021, 36, 101016 CrossRef.
- J. X. Zhu, Z. D. Sun, J. K. Xu, R. D. Walczak, J. A. Dziuban and C. Lee, Sci. Bull., 2021, 66, 1176–1185 CrossRef CAS PubMed.
- J. Zhu, M. Cho, Y. Li, T. He, J. Ahn, J. Park, T. Ren, C. Lee and I. Park, Nano Energy, 2021, 86, 106035 CrossRef CAS.
- Q. Zhang, Z. Zhang, Q. Liang, Q. Shi, M. Zhu and C. Lee, Adv. Sci., 2021, 8, 2004727 CrossRef CAS PubMed.
- Q. Zhang, L. Li, T. Wang, Y. Jiang, Y. Tian, T. Jin, T. Yue and C. Lee, Nano Energy, 2021, 90, 106501 CrossRef CAS.
- Q. F. Shi, Z. X. Zhang, Y. Q. Yang, X. C. Shan, B. Salam and C. K. Lee, ACS Nano, 2021, 15, 18312–18326 CrossRef CAS PubMed.
- X. G. Guo, T. Y. Y. He, Z. X. Zhang, A. X. Luo, F. Wang, E. J. Ng, Y. Zhu, H. C. Liu and C. K. Lee, ACS Nano, 2021, 15, 19054–19069 CrossRef CAS PubMed.
- T. Jin, Z. D. Sun, L. Li, Q. Zhang, M. L. Zhu, Z. X. Zhang, G. J. Yuan, T. Chen, Y. Z. Tian, X. Y. Hou and C. Lee, Nat. Commun., 2020, 11, 5381 CrossRef CAS PubMed.
- H. Wang, J. Wang, T. He, Z. Li and C. Lee, Nano Energy, 2019, 63, 103844 CrossRef CAS.
- Q. F. Shi, C. K. Qiu, T. Y. Y. He, F. Wu, M. L. Zhu, J. A. Dziuban, R. Walczak, M. R. Yuce and C. Lee, Nano Energy, 2019, 60, 545–556 CrossRef CAS.
- Q. F. Shi and C. K. Lee, Adv. Sci., 2019, 6, 1900617 CrossRef PubMed.
- T. Chen, Q. F. Shi, M. L. Zhu, T. Y. Y. He, Z. Yang, H. C. Liu, L. N. Sun, L. Yangt and C. Lee, Nano Energy, 2019, 60, 440–448 CrossRef CAS.
- T. Chen, Q. F. Shi, Z. Yang, J. C. Liu, H. C. Liu, L. N. Sun and C. Lee, Nanomaterials, 2018, 8, 613 CrossRef PubMed.
- Y. Lee, S. H. Cha, Y. W. Kim, D. Choi and J. Y. Sun, Nat. Commun., 2018, 9, 1804 CrossRef PubMed.
- X. Wang, X. Lin and X. Dang, Neural Network., 2020, 125, 258–280 CrossRef PubMed.
- A. Glielmo, B. E. Husic, A. Rodriguez, C. Clementi, F. Noe and A. Laio, Chem. Rev., 2021, 121, 9722–9758 CrossRef CAS PubMed.
- J. E. van Engelen and H. H. Hoos, Mach. Learn., 2019, 109, 373–440 CrossRef.
- M. Peikari, S. Salama, S. Nofech-Mozes and A. L. Martel, Sci. Rep., 2018, 8, 7193 CrossRef PubMed.
- M. Nadif and F. Role, Briefings Bioinf., 2021, 22, 1592–1603 CrossRef CAS PubMed.
- F. Zhang, X. Tang, A. Tong, B. Wang, J. Wang, Y. Lv, C. Tang and J. Wang, Spectrosc. Lett., 2020, 53, 222–233 CrossRef CAS.
- R. Adato and H. Altug, Nat. Commun., 2013, 4, 2154 CrossRef PubMed.
- R. J. Dijkstra, W. J. Scheenen, N. Dam, E. W. Roubos and J. J. ter Meulen, J. Neurosci. Methods, 2007, 159, 43–50 CrossRef CAS PubMed.
- A. Barucci, C. D'Andrea, E. Farnesi, M. Banchelli, C. Amicucci, M. de Angelis, B. Hwang and P. Matteini, Analyst, 2021, 146, 674–682 RSC.
- V. Romeo, S. Maurea, R. Cuocolo, M. Petretta, P. P. Mainenti, F. Verde, M. Coppola, S. Dell'Aversana and A. Brunetti, J. Magn. Reson. Imag., 2018, 48, 198–204 CrossRef PubMed.
- A. S. Mondol, M. D. Patel, J. Ruger, C. Stiebing, A. Kleiber, T. Henkel, J. Popp and I. W. Schie, Sensors, 2019, 19, 4428 CrossRef CAS PubMed.
- H. Shin, S. Oh, S. Hong, M. Kang, D. Kang, Y.-g. Ji, B. H. Choi, K.-W. Kang, H. Jeong, Y. Park, S. Hong, H. K. Kim and Y. Choi, ACS Nano, 2020, 14, 5435–5444 CrossRef CAS PubMed.
- M. V. Narkhede, P. P. Bartakke and M. S. Sutaone, Artif. Intell. Rev., 2021, 55, 291–322 CrossRef.
- M. Shirobokov, S. Trofimov and M. Ovchinnikov, Acta Astronaut., 2021, 186, 87–97 CrossRef.
-
K. He, X. Zhang, S. Ren and J. Sun, Presented in Part at the Proceedings of the IEEE International Conference on Computer Vision, 2015 Search PubMed.
-
X. Glorot and Y. Bengio, Understanding the difficulty of training deep feedforward neural networks, Proceedings of the thirteenth international conference on artificial intelligence and statistics, JMLR, Chia Laguna Resort, 2010 Search PubMed.
- P. Probst, M. N. Wright and A. L. Boulesteix, Wiley Interdiscip. Rev.: Data Min. Knowl. Discov., 2019, 9, e1301 Search PubMed.
- L. Yang and A. Shami, Neurocomputing, 2020, 415, 295–316 CrossRef.
- J. Yang, J. Xu, X. Zhang, C. Wu, T. Lin and Y. Ying, Anal. Chim. Acta, 2019, 1081, 6–17 CrossRef CAS PubMed.
- Y. Yang, Q. Shi, Z. Zhang, X. Shan, B. Salam and C. Lee, InfoMat, 2022, e12360 Search PubMed.
- J. Miao and W. Zhu, Evol. Intell., 2021, 15, 1545–1569 CrossRef.
- J. Z. Feng, Y. Wang, J. Peng, M. W. Sun, J. Zeng and H. Jiang, J. Crit. Care, 2019, 54, 110–116 CrossRef PubMed.
- A. D. Phan, C. V. Nguyen, P. T. Linh, T. V. Huynh, V. D. Lam, A.-T. Le and K. Wakabayashi, Crystals, 2020, 10, 125 CrossRef CAS.
- M. V. Zhelyeznyakov, S. Brunton and A. Majumdar, ACS Photonics, 2021, 8, 481–488 CrossRef CAS.
- C. Yeung, J.-M. Tsai, B. King, B. Pham, D. Ho, J. Liang, M. W. Knight and A. P. Raman, Nanophotonics, 2021, 10, 1133–1143 CrossRef.
- F. Wen, J. Jiang and J. A. Fan, ACS Photonics, 2020, 7, 2098–2104 CrossRef CAS.
- I. Tanriover, W. Hadibrata and K. Aydin, ACS Photonics, 2020, 7, 1957–1964 CrossRef CAS.
- O. Hemmatyar, S. Abdollahramezani, Y. Kiarashinejad, M. Zandehshahvar and A. Adibi, Nanoscale, 2019, 11, 21266–21274 RSC.
- J. He, C. He, C. Zheng, Q. Wang and J. Ye, Nanoscale, 2019, 11, 17444–17459 RSC.
- Y. Chen, J. Zhu, Y. Xie, N. Feng and Q. H. Liu, Nanoscale, 2019, 11, 9749–9755 RSC.
- X. Han, Z. Fan, Z. Liu, C. Li and L. J. Guo, InfoMat, 2020, 3, 432–442 CrossRef.
- S. Jafar-Zanjani, S. Inampudi and H. Mosallaei, Sci. Rep., 2018, 8, 11040 CrossRef PubMed.
- C. C. Nadell, B. Huang, J. M. Malof and W. J. Padilla, Opt. Express, 2019, 27, 27523–27535 CrossRef CAS PubMed.
- S. So, J. Mun and J. Rho, ACS Appl. Mater. Interfaces, 2019, 11, 24264–24268 CrossRef CAS PubMed.
- W. Ma, F. Cheng, Y. Xu, Q. Wen and Y. Liu, Adv. Mater., 2019, 31, 1901111 CrossRef PubMed.
- Z. Liu, D. Zhu, S. P. Rodrigues, K. T. Lee and W. Cai, Nano Lett., 2018, 18, 6570–6576 CrossRef CAS PubMed.
- K. Gao, H. Zhu, B. Charron, T. Mochizuki, C. X. Dong, H. R. Ding, Y. Cui, M. D. Lu, W. Peng, S. G. Zhu, L. Hong and J. F. Masson, J. Phys. Chem. C, 2022, 126, 9446–9455 CrossRef CAS.
- T. Rojalin, D. Antonio, A. Kulkarni and R. P. Carney, Appl. Spectrosc., 2022, 76, 485–495 CrossRef CAS PubMed.
- H. Y. Shi, H. Y. Wang, X. Y. Meng, R. Z. Chen, Y. S. Zhang, Y. Y. Su and Y. He, Anal. Chem., 2018, 90, 14216–14221 CrossRef CAS PubMed.
- F. Lussier, V. Thibault, B. Charron, G. Q. Wallace and J.-F. Masson, TrAC, Trends Anal. Chem., 2020, 124, 115796 CrossRef CAS.
-
T. S. Alstrom, K. B. Frohling, J. Larsen, M. N. Schmidt, M. Bache, M. S. Schmidt, M. H. Jakobsen and A. Boisen, 2014 IEEE International Workshop on Machine Learning for Signal Processing, MLSP, 2014 Search PubMed.
- S. H. Luo, W. L. Wang, Z. F. Zhou, Y. Xie, B. Ren, G. K. Liu and Z. Q. Tian, Anal. Chem., 2022, 94, 10151–10158 CrossRef CAS PubMed.
- N. Banaei, J. Moshfegh and B. Kim, J. Raman Spectrosc., 2021, 52, 1810–1819 CrossRef CAS.
- M. G. Cha, W. K. Son, Y. S. Choi, H. M. Kim, E. Hahm, B. H. Jun and D. H. Jeong, Appl. Surf. Sci., 2021, 558, 149787 CrossRef CAS.
-
N. H. Othman, K. Y. Lee, A. R. M. Radzol and W. Mansor, IEEE Engineering in Medicine and Biology Society (EMBC), IEEE, Berlin, 2019 Search PubMed.
- D. Paria, A. Convertino, V. Mussi, L. Maiolo and I. Barman, Adv. Healthcare Mater., 2021, 10, 2001110 CrossRef CAS PubMed.
-
T. A. Saifuzzaman, K. Y. Lee, A. R. M. Radzol, P. S. Wong and I. Looi, IEEE Engineering in Medicine and Biology Society (EMBC), IEEE, Montreal, 2020 Search PubMed.
- Y. Yang, B. Xu, J. Haverstick, N. Ibtehaz, A. Muszynski, X. Chen, M. E. H. Chowdhury, S. M. Zughaier and Y. Zhao, Nanoscale, 2022, 14, 8806–8817 RSC.
- M. G. Cha, W. K. Son, Y.-S. Choi, H.-M. Kim, E. Hahm, B.-H. Jun and D. H. Jeong, Appl. Surf. Sci., 2021, 558, 149787 CrossRef CAS.
- S. Bhana, E. Chaffin, Y. M. Wang, S. R. Mishra and X. H. Huang, Nanomedicine, 2014, 9, 593–606 CrossRef CAS PubMed.
- M. Czaplicka, K. Nicinski, A. Nowicka, T. Szymborski, I. Chmielewska, J. Trzcinska-Danielewicz, A. Girstun and A. Kaminska, Cancers, 2020, 12, 3315 CrossRef CAS PubMed.
- A. Kaminska, T. Szymborski, E. Witkowska, E. Kijenska-Gawronska, W. Swieszkowski, K. Nicinski, J. Trzcinska-Danielewicz and A. Girstun, Nanomaterials, 2019, 9, 366 CrossRef CAS PubMed.
- Y. F. Pang, C. W. Wang, R. Xiao and Z. W. Sun, Chem.–Eur. J., 2018, 24, 7060–7067 CrossRef CAS PubMed.
- M. Y. Sha, H. X. Xu, M. J. Natan and R. Cromer, J. Am. Chem. Soc., 2008, 130, 17214–17215 CrossRef CAS PubMed.
- C. L. Sun, R. Zhang, M. X. Gao and X. M. Zhang, Anal. Bioanal. Chem., 2015, 407, 8883–8892 CrossRef CAS PubMed.
- X. X. Wu, L. Q. Luo, S. G. Yang, X. H. Ma, Y. L. Li, C. Dong, Y. C. Tian, L. E. Zhang, Z. Y. Shen and A. G. Wu, ACS Appl. Mater. Interfaces, 2015, 7, 9965–9971 CrossRef CAS PubMed.
- J. R. Xiong, C. Dong, J. J. Zhang, X. Y. Fang, J. Ni, H. Y. Gan, J. X. Li and C. Y. Song, Biosens. Bioelectron., 2022, 213, 114442 CrossRef CAS PubMed.
- Y. P. Xu, D. H. Zhang, J. Lin, X. X. Wu, X. W. Xu, O. U. Akakuru, H. Zhang, Z. W. Zhang, Y. J. Xie, A. G. Wu and G. L. Shao, Biomater. Sci., 2022, 10, 1812–1820 RSC.
- T. Xue, S. Q. Wang, G. Y. Ou, Y. Li, H. M. Ruan, Z. H. Li, Y. Y. Ma, R. F. Zou, J. Y. Qiu, Z. Y. Shen and A. G. Wu, Anal. Methods, 2019, 11, 2918–2928 RSC.
- Y. J. Zhang, Q. Y. Zeng, L. F. Li, M. N. Qi, Q. C. Qi, S. X. Li and J. F. Xu, Laser Phys., 2019, 29, 045602 CrossRef CAS.
- X. Wu, Y. Xia, Y. Huang, J. Li, H. Ruan, T. Chen, L. Luo, Z. Shen and A. Wu, ACS Appl. Mater. Interfaces, 2016, 8, 19928–19938 CrossRef CAS PubMed.
- Y. Pang, C. Wang, R. Xiao and Z. Sun, Chemistry, 2018, 24, 7060–7067 CrossRef CAS PubMed.
- X. Fang, Q. Zeng, X. Yan, Z. Zhao, N. Chen, Q. Deng, M. Zhu, Y. Zhang and S. Li, J. Appl. Phys., 2021, 129, 123103 CrossRef CAS.
- M. Erzina, A. Trelin, O. Guselnikova, B. Dvorankova, K. Strnadova, A. Perminova, P. Ulbrich, D. Mares, V. Jerabek, R. Elashnikov, V. Svorcik and O. Lyutakov, Sens. Actuators, B, 2020, 308, 127660 CrossRef CAS.
- Y. Q. Shan, M. L. Wang, Z. L. Shi, M. L. Lei, X. X. Wang, F. G. Wu, H. H. Ran, G. M. Arumugam, Q. N. Cui and C. X. Xu, J. Mater. Sci. Technol., 2020, 43, 161–167 CrossRef CAS.
- T. Y. Liu, K. T. Tsai, H. H. Wang, Y. Chen, Y. H. Chen, Y. C. Chao, H. H. Chang, C. H. Lin, J. K. Wang and Y. L. Wang, Nat. Commun., 2011, 2, 538 CrossRef PubMed.
-
N. Baig, S. Polisetti, N. Morales-Soto, S. J. B. Dunham, J. V. Sweedler, J. D. Shrout and P. W. Bohn, Biosensing and Nanomedicine IX, SPIE, 2016 Search PubMed.
- A. E. Grow, L. L. Wood, J. L. Claycomb and P. A. Thompson, J. Microbiol. Methods, 2003, 53, 221–233 CrossRef CAS PubMed.
- Y. F. Xie, L. Xu, Y. Q. Wang, J. D. Shao, L. Wang, H. Y. Wang, H. Qian and W. R. Yao, Anal. Methods, 2013, 5, 946–952 RSC.
- M. Rippa, R. Castagna, M. Pannico, P. Musto, G. Borriello, R. Paradiso, G. Galiero, S. B. Censi, J. Zhou, J. Zyss and L. Petti, ACS Sens., 2017, 2, 947–954 CrossRef CAS PubMed.
- L. Rodriguez-Lorenzo, A. Garrido-Maestu, A. K. Bhuniapio, B. Espina, M. Prado, L. Dieguez and S. Abalde-Cela, ACS Appl. Nano Mater., 2019, 2, 6081–6086 CrossRef CAS.
- Y. Hong, Y. Q. Li, L. B. Huang, W. He, S. X. Wang, C. Wang, G. Y. Zhou, Y. M. Chen, X. Zhou, Y. F. Huang, W. Huang, T. X. Gong and Z. G. Zhou, J. Biophotonics, 2020, 13, 120360 CrossRef PubMed.
- H. Li, Y. B. Cao and F. Lu, J. Innovative Opt. Health Sci., 2021, 14, 2141002 CrossRef.
- J. W. Tang, J. Q. Li, X. C. Yin, W. W. Xu, Y. C. Pan, Q. H. Liu, B. Gu, X. Zhang and L. Wang, Front. Microbiol., 2022, 13, 838808 CrossRef PubMed.
- W. J. Thrift, A. Cabuslay, A. B. Laird, S. Ranjbar, A. I. Hochbaum and R. Ragan, ACS Sens., 2019, 4, 2311–2319 CrossRef CAS PubMed.
- M. Kahraman, B. N. Balz and S. Wachsmann-Hogiu, Analyst, 2013, 138, 2906–2913 RSC.
- C. Y. Song, L. H. Min, N. Zhou, Y. J. Yang, B. Y. Yang, L. Zhang, S. Su and L. H. Wang, RSC Adv., 2014, 4, 41666–41669 RSC.
- C. Y. Song, J. Chen, Y. P. Zhao and L. H. Wang, J. Mater. Chem. B, 2014, 2, 7488–7494 RSC.
- M. H. Shin, W. Hong, Y. Sa, L. Chen, Y. J. Jung, X. Wang, B. Zhao and Y. M. Jung, Vib. Spectrosc., 2014, 72, 44–49 CrossRef CAS.
- H. Chon, S. Lee, S. Y. Yoon, E. K. Lee, S. I. Chang and J. Choo, Chem. Commun., 2014, 50, 1058–1060 RSC.
- L. G. Xu, W. J. Yan, W. Ma, H. Kuang, X. L. Wu, L. Q. Liu, Y. Zhao, L. B. Wang and C. L. Xu, Adv. Mater., 2015, 27, 1706–1711 CrossRef CAS PubMed.
- N. Guarrotxena and G. C. Bazan, Adv. Mater., 2014, 26, 1941–1946 CrossRef CAS PubMed.
- Y. Yang, Y. Peng, C. Lin, L. Long, J. Hu, J. He, H. Zeng, Z. Huang, Z.-Y. Li, M. Tanemura, J. Shi, J. R. Lombardi and X. Luo, Nano-Micro Lett., 2021, 13, 109 CrossRef CAS PubMed.
- J. Sundaram, B. Park, Y. Kwon and K. C. Lawrence, Int. J. Food Microbiol., 2013, 167, 67–73 CrossRef CAS PubMed.
- C. C. Lin, Y. M. Yang, P. H. Liao, D. W. Chen, H. P. Lin and H. C. Chang, Biosens. Bioelectron., 2014, 53, 519–527 CrossRef CAS PubMed.
- H. Y. Chu, Y. W. Huang and Y. P. Zhao, Appl. Spectrosc., 2008, 62, 922–931 CrossRef CAS PubMed.
- D. T. Yang, H. B. Zhou, C. Haisch, R. Niessner and Y. B. Ying, Talanta, 2016, 146, 457–463 CrossRef CAS PubMed.
- P. A. Mosier-Boss, K. C. Sorensen, R. D. George and A. Obraztsova, Spectrochim. Acta, Part A, 2016, 153, 591–598 CrossRef CAS PubMed.
- D. Cam, K. Keseroglu, M. Kahraman, F. Sahin and M. Culha, J. Raman Spectrosc., 2010, 41, 484–489 CrossRef CAS.
- E. Akanny, A. Bonhomme, C. Commun, A. Doleans-Jordheim, C. Farre, F. Bessueille, S. Bourgeois and C. Bordes, J. Raman Spectrosc., 2020, 51, 619–629 CrossRef CAS.
- B. Ankamwar, U. K. Sur and P. Das, Anal. Methods, 2016, 8, 2335–2340 RSC.
- W. R. Premasiri, D. T. Moir, M. S. Klempner, N. Krieger, G. Jones and L. D. Ziegler, J. Phys. Chem. B, 2005, 109, 312–320 CrossRef CAS PubMed.
- K. S. Yuan, Q. S. Mei, X. J. Guo, Y. W. Xu, D. T. Yang, B. J. Sanchez, B. B. Sheng, C. S. Liu, Z. W. Hu, G. C. Yu, H. M. Ma, H. Gao, C. Haisch, R. Niessner, Z. J. Jiang and H. B. Zhou, Chem. Sci., 2018, 9, 8781–8795 RSC.
- E. Temur, I. H. Boyaci, U. Tamer, H. Unsal and N. Aydogan, Anal. Bioanal. Chem., 2010, 397, 1595–1604 CrossRef CAS PubMed.
- Q. Y. Fu, X. L. Fang, Y. Zhao, X. Qiu, P. Wang and S. X. Li, Spectrosc. Spectral Anal., 2022, 42, 1339–1345 Search PubMed.
- S. S. Yan, J. X. Qiu, L. Guo, D. Z. Li, D. P. Xu and Q. Liu, Appl. Microbiol. Biotechnol., 2021, 105, 1315–1331 CrossRef CAS PubMed.
-
T.-K. Jan, H.-T. Lin, H.-P. Chen, T.-C. Chern, C.-Y. Huang, B.-C. Wen, C.-W. Chung, Y.-J. Li, Y.-C. Chuang, L.-L. Li, Y.-J. Chan, J.-K. Wang, Y.-L. Wang, C.-H. Lin and D.-W. Wang, IEEE International Conference on Bioinformatics and Biomedicine, IEEE, Atlanta, 2011 Search PubMed.
- C. Q. Nguyen, W. J. Thrift, A. Bhattacharjee, S. Ranjbar, T. Gallagher, M. Darvishzadeh-Varcheie, R. N. Sanderson, F. Capolino, K. Whiteson, P. Baldi, A. I. Hochbaum and R. Ragan, ACS Appl. Mater. Interfaces, 2018, 10, 12364–12373 CrossRef CAS PubMed.
- W. J. Thrift, S. Ronaghi, M. Samad, H. Wei, D. G. Nguyen, A. S. Cabuslay, C. E. Groome, P. J. Santiago, P. Baldi, A. I. Hochbaum and R. Ragan, ACS Nano, 2020, 14, 15336–15348 CrossRef PubMed.
- A. Rahman, S. Kang, W. Wang, Q. S. Huang, I. Kim and P. J. Vikesland, ACS Appl. Nano Mater., 2022, 5, 259–268 CrossRef CAS.
- E. Papadopoulou and S. E. Bell, Chemistry, 2012, 18, 5394–5400 CrossRef CAS PubMed.
- Z. Yi, X. Y. Li, F. J. Liu, P. Y. Jin, X. Chu and R. Q. Yu, Biosens. Bioelectron., 2013, 43, 308–314 CrossRef CAS PubMed.
- H. Wang, X. Jiang, X. Wang, X. Wei, Y. Zhu, B. Sun, Y. Su, S. He and Y. He, Anal. Chem., 2014, 86, 7368–7376 CrossRef CAS PubMed.
- E. Crew, H. Yan, L. Q. Lin, J. Yin, Z. Skeete, T. Kotlyar, N. Tchah, J. Lee, M. Bellavia, I. Goodshaw, P. Joseph, J. Luo, S. Gal and C. J. Zhong, Analyst, 2013, 138, 4941–4949 RSC.
- M. M. Harper, J. A. Dougan, N. C. Shand, D. Graham and K. Faulds, Analyst, 2012, 137, 2063–2068 RSC.
- I. Khalil, W. A. A. Yehye, M. N. Julkapli, A. A. I. Sina, S. Rahmati, W. J. Basirun and A. Seyfoddin, Analyst, 2020, 145, 1414–1426 RSC.
- Y. J. Sun, F. G. Xu, Y. Zhang, Y. Shi, Z. W. Wen and Z. Li, J. Mater. Chem., 2011, 21, 16675–16685 RSC.
- Y. Q. Wen, W. Q. Wang, Z. L. Zhang, L. P. Xu, H. W. Du, X. J. Zhang and Y. L. Song, Nanoscale, 2013, 5, 523–526 RSC.
- S. Yuksel, L. Schwenkbier, S. Pollok, K. Weber, D. Cialla-May and J. Popp, Analyst, 2015, 140, 7254–7262 RSC.
- J. N. Zhang, P. Joshi, Y. Zhou, R. Ding and P. Zhang, Chem. Commun., 2015, 51, 15284–15286 RSC.
-
P. Dixit and G. I. Prajapati, Advanced Computing & Communication Technologies, 2015 Fifth International Conference on Advanced Computing & Communication Technologies, IEEE, Rohtak, 2015 Search PubMed.
- P. Moitra, A. Chaichi, S. M. A. Hasan, K. Dighe, M. Alafeef, A. Prasad, M. R. Gartia and D. Pan, Biosens. Bioelectron., 2022, 208, 114200 CrossRef CAS PubMed.
- V. Karunakaran, V. N. Saritha, M. M. Joseph, J. B. Nair, G. Saranya, K. G. Raghu, K. Sujathan, K. S. Kumar and K. K. Maiti, Nanomedicine, 2020, 29, 102276 CrossRef CAS PubMed.
- P. H. L. Nguyen, S. Rubin, P. Sarangi, P. Pal and Y. Fainman, Appl. Phys. Lett., 2022, 120, 023701 CrossRef CAS.
- H. Shi, H. Wang, X. Meng, R. Chen, Y. Zhang, Y. Su and Y. He, Anal. Chem., 2018, 90, 14216–14221 CrossRef CAS PubMed.
- S. Y. Fang, Y. R. Zhao, Y. Wang, J. M. Li, F. L. Zhu and K. Q. Yu, Front. Plant Sci., 2022, 13, 802761 CrossRef PubMed.
- V. Karunakaran, V. N. Saritha, M. M. Joseph, J. B. Nair, G. Saranya, K. G. Raghu, K. Sujathan, K. S. Kumar and K. K. Maiti, Nanomedicine, 2020, 29, 102276 CrossRef CAS PubMed.
- M. Kazemzadeh, M. Martinez-Calderon, S. Y. Paek, M. Lowe, C. Aguergaray, W. L. Xu, L. W. Chamley, N. G. R. Broderick and C. L. Hisey, ACS Sens., 2022, 7, 1698–1711 CrossRef CAS PubMed.
- W. Kim, J. C. Lee, J. H. Shin, K. H. Jin, H. K. Park and S. Choi, Anal. Chem., 2016, 88, 5531–5537 CrossRef CAS.
- W. Kim, S. H. Lee, J. H. Kim, Y. J. Ahn, Y. H. Kim, J. S. Yu and S. Choi, ACS Nano, 2018, 12, 7100–7108 CrossRef CAS PubMed.
- K. K. Maiti, A. Samanta, M. Vendrell, K. S. Soh, M. Olivo and Y. T. Chang, Chem. Commun., 2011, 47, 3514–3516 RSC.
- J. H. Kim, J. S. Kim, H. Choi, S. M. Lee, B. H. Jun, K. N. Yu, E. Kuk, Y. K. Kim, D. H. Jeong, M. H. Cho and Y. S. Lee, Anal. Chem., 2006, 78, 6967–6973 CrossRef CAS PubMed.
- I. H. Chou, M. Benford, H. T. Beier, G. L. Cote, M. Wang, N. Jing, J. Kameoka and T. A. Good, Nano Lett., 2008, 8, 1729–1735 CrossRef PubMed.
- M. Kazemzadeh, C. L. Hisey, A. Artuyants, C. Blenkiron, L. W. Chamley, K. Zargar-Shoshtari, W. L. Xu and N. G. R. Broderick, Biomed. Opt. Express, 2021, 12, 3965–3981 CrossRef PubMed.
- S. Grieve, N. Puvvada, A. Phinyomark, K. Russell, A. Murugesan, E. Zed, A. Hassan, J. F. Legare, P. C. Kienesberger, T. Pulinilkunnil, T. Reiman, E. Scheme and K. R. Brunt, Nanomedicine, 2021, 16, 2175–2188 CrossRef CAS PubMed.
- M. Ghassemi and E. O. Nsoesie, Patterns, 2022, 3, 100392 CrossRef PubMed.
- P. H. L. Nguyen, B. Hong, S. Rubin and Y. Fainman, Biomed. Opt. Express, 2020, 11, 5092–5121 CrossRef CAS PubMed.
- Y. Seok, B. S. Batule and M. G. Kim, Biosens. Bioelectron., 2020, 165, 112400 CrossRef CAS PubMed.
|
This journal is © The Royal Society of Chemistry 2023 |