DOI:
10.1039/D0AN01972K
(Minireview)
Analyst, 2021,
146, 789-802
Recent advances in the use of stimulated Raman scattering in histopathology
Received
5th October 2020
, Accepted 15th December 2020
First published on 4th January 2021
Abstract
Stimulated Raman histopathology (SRH) utilises the intrinsic vibrational properties of lipids, proteins and nucleic acids to generate contrast providing rapid image acquisition that allows visualisation of histopathological features. It is currently being trialled in the intraoperative setting, where the ability to image unprocessed samples rapidly and with high resolution offers several potential advantages over the use of conventional haematoxylin and eosin stained images. Here we review recent advances in the field including new updates in instrumentation and computer aided diagnosis. We also discuss how other non-linear modalities can be used to provide additional diagnostic contrast which together pave the way for enhanced histopathology and open up possibilities for in vivo pathology.
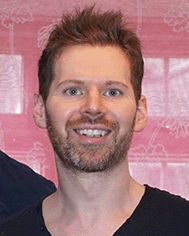 Martin Lee | Martin Lee studied Biochemistry at the University of Bristol and completed his PhD in Biomedical Engineering at the University of Edinburgh. His PhD work focused around Coherent anti-Stokes Raman imaging and he has continued this interest by joining the Cancer Research UK Edinburgh Centre as an Imaging Engineer helping to apply these imaging methodologies to Cancer Research. |
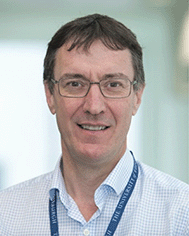 C Simon Herrington | C Simon Herrington is Professor of Molecular Cancer Pathology at the University of Edinburgh and a consultant pathologist in the UK National Health Service. His research aims to improve the diagnostic assessment of tumours using molecular analysis and optical imaging. Specific interests include the application of Raman spectroscopy and light sheet microscopy to cancer diagnosis; and the molecular stratification of ovarian tumours. |
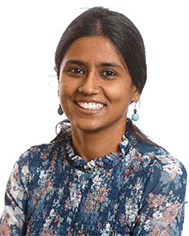 Manasa Ravindra | Manasa Punaha Ravindra finished her bachelors in pharmacy from Jawaharlal Nehru Technological University (Hyderabad, India) and moved to the United States to do a PhD in medicinal chemistry at Duquesne University during which her project was to develop tumor-targeted antimetabolites. Following her interest in drug development, she joined the University of Edinburgh as a PDRA in the Hulme lab, where her Cancer Research UK-funded project work focuses on using the principles of drug design in developing novel bioorthogonal Raman tags with improved physicochemical properties to enhance the preclinical evaluation of drugs. |
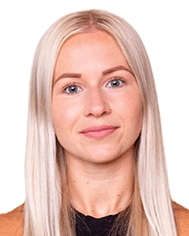 Kristel Sepp | Kristel Sepp received her MChem in Medicinal and Biological Chemistry from the University of Edinburgh. Subsequently, she joined the interdisciplinary OPTIMA doctoral training programme in 2016 under the supervision of Prof. Valerie Brunton and Prof. Alison Hulme. Her PhD project focused on the application of label-free SRS imaging to study drug localisation in live cells and spheroids. |
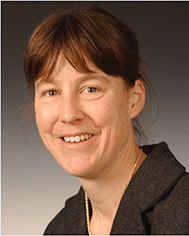 Alison N. Hulme | Alison Hulme is a Professor of Synthesis and Chemical Biology at the University of Edinburgh. Her research integrates synthetic methodology development with chemical biology to provide molecular level insight to challenges in biology and medicine. Specific interests include the efficient construction of complex macrocyclic targets using alkene/alkyne metathesis reactions; the development of small molecules and stapled peptides that can disrupt protein–protein interactions; and the visualisation of intracellular drug concentrations using stimulated Raman scattering microscopy. |
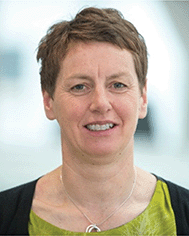 Valerie G. Brunton | Valerie Brunton is a Professor of Cancer Therapeutics at the University of Edinburgh. Her research uses multimodal coherent Raman scattering and fluorescence imaging to monitor the interaction of cancer cells with the tumor microenvironment and provide read-outs of cancer phenotypes in real time. Other research is focused on exploiting the benefits of stimulated Raman scattering microscopy to monitor drug distribution within tissues. |
1. Introduction
Diagnosis by histopathology is the clinical gold standard in a wide variety of diseases but, despite refinement using immunohistochemical and molecular techniques, remains limited by the need for tissue removal and laboratory processing. Whilst tissue sections can often be assessed the following day after fixation, embedding, cutting, staining and, in some laboratories, digitisation, there is a need for more rapid and accurate diagnostic processing in the operating room. To help guide resection of diseased tissue during an operation, especially where an unexpected finding may require modification or even termination of the surgery, an intra-operative diagnosis of the tissue may be performed. Time in the operating theatre is an expensive commodity so a rapid approach to obtaining a reliable diagnostic slide is taken in the form of a frozen section of tissue stained with haematoxylin and eosin (H&E). Concordance of frozen section histopathology to permanent formalin fixed sections is typically very high,1 though there are a number of potential limitations around sample processing that may cause difficulty in diagnostic interpretation. Technical issues, particularly in challenging tissue types such as brain matter, are introduced when samples are frozen, which can lead to bloated cell morphology, uneven sectioning and poor staining.2,3 Whilst turn-around times are often reported in the region of 20 minutes this is the time taken for a pathologist to assess the specimen and excludes tissue transport and preparation time.4 These steps also require highly trained technical personnel and facilities which add further costs to the endeavour so there is great interest in providing new, adjunctive, tools for use in this environment.
The ability to produce ‘H&E-like’ images using optical approaches can reduce, or potentially eliminate, the costs associated with laboratory processing whilst maintaining the information provided by this gold-standard staining method. Moreover, the additional chemical information provided by optical methods such as stimulated Raman scattering (SRS) can enhance the information generated from the tissue. Whilst it could be argued that there is a cost-shift associated with training other professionals to interpret the images, particularly those generated in the operating room, the involvement of pathologists in the interpretation of the images can be achieved easily by remote viewing of digital images. In addition, any additional costs must be balanced against the cost savings associated with reduced laboratory processing, which involves complex laboratory equipment and reagents, and multiple professional groups. The reduction in time taken to produce the images also provides significant advantages in terms of reduction in anaesthetic time and improved operating theatre efficiency. Finally, the generation of images in ‘real-time’ allows a more interactive approach, particularly to intra-operative diagnosis, where surgeons and pathologists can collaborate iteratively to solve a particular clinical problem. This can be a significant problem particularly when assessing tumour resection margins, where multiple specimens need to be sent from the operating theatre to the laboratory, compounding the time taken to achieve an appropriate outcome for the patient. The generation of serial optical images, without the need for specimen transport or processing, would be a significant advance, with even greater reduction in anaesthetic time and improvement in operating theatre efficiency.
Vibrational spectroscopy has long drawn interest as a diagnostic tool for differentiating normal and diseased tissue by assessing changes in the chemical composition via the interaction with light. Typically, two approaches to vibrational spectroscopy can be taken, infrared (IR) spectroscopy, where the absorbance of light is measured, or Raman spectroscopy where the inelastic scattering of photons are measured. Both techniques have been explored as potential diagnostic markers for a wide variety of diseased states but their use in imaging applications is limited by the relatively long acquisition times.5 Biological Raman spectra are usually divided into three distinct regions as show in Fig. 1A: a high frequency region, often called the C–H stretch region between 2800 and 3200 cm−1 mainly containing broad C–H and O–H bond vibrations; a silent region between 1700 and 2800 cm−1 where no cellular vibrations occur and a fingerprint region between 500 and 1700 cm−1 that contains many overlapping peaks used to identify biochemical information associated with proteins, lipids and DNA.
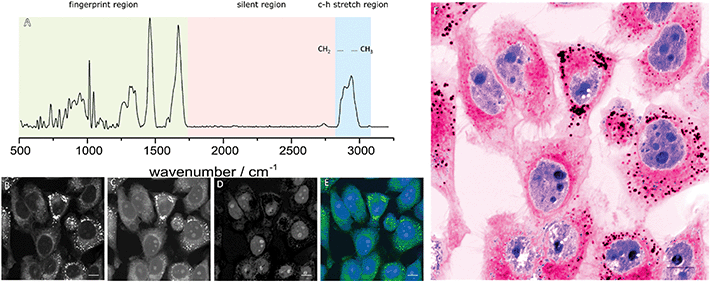 |
| Fig. 1 Generation of SRH images. (A) Raman spectra of a typical cell showing the location of the fingerprint, silent and C–H stretch regions. Images of HeLa cells at (B) CH2 stretching vibration at 2840 cm−1, (C) CH3 stretching vibration 2930 cm−1, (D) thresholded CH3-CH2 difference image, (E) composite image generated from (B and D) with green channel showing the cell body and blue channel highlighting nuclear morphology, (F) recoloured image of (E) to mimic H&E staining colours. Scale bar 10 μm. Images captured at 1024 × 1024 with 20 μs pixel dwell time with a frame time of 22.3 s per image. | |
The development of coherent Raman imaging (CRI) and particularly stimulated Raman scattering (SRS) however has opened the door for vibrational imaging at a speed and resolution that can start to compete with optical methods. SRS uses two lasers that are separated in frequency to generate a stimulated emission when the frequency difference matches a Raman vibrational mode of interest. By modulating one of the lasers in amplitude this stimulated emission can be measured in the other at the modulation frequency using a lock-in amplifier. SRS is not affected by fluorescence from the sample and the signal closely resembles that of spontaneous Raman whilst being linearly correlated with the concentration of individual vibrational motifs, allowing quantitative analysis.6
The abundance of CH, CH2 and CH3 stretches from proteins and lipids in the high-frequency region generates strong contrast allowing rapid image acquisition. The differing chemical composition of these macromolecules give rise to subtly differing spectra in this region, with proteins typically showing a peak around 2930 cm−1 due to higher CH3 content and lipids showing a peak around 2850 cm−1 due to their CH2 content. Images at these wavelengths (Fig. 1B and C) highlight different features of the cell which can be further enhanced through image subtractions. Typically, the CH2 contrast is used to highlight cytoplasmic features whilst a difference image of the two wavelengths highlights the nuclei of the cells (Fig. 1D and E). By applying colour look up tables (LUTs) an image can be produced to mimic familiar H&E staining as shown in Fig. 1F.7 Technical details of the methods behind this process are summarised in two excellent reviews from Camp et al.,8 and Yang et al.,9 whereas this review will update on the current clinical applications of SRH.
Multiphoton techniques, such as CRI, are attractive due to the potential advantages that they offer over traditional histopathology methods in terms of time and resource saving. The ability to generate contrast without the need for staining and an inherent optical sectioning ability allows them to be used on unsectioned tissue biopsies without any further processing. By bringing CRI to the operating theatre, there is potential for a new, rapid pathway compared to conventional frozen sections as shown in Fig. 2. This allows for operator agnostic histopathological details to be viewed directly from excised tissue within minutes. Moreover, these images can be shared across networks allowing intraoperative consultation with either diagnostic algorithms or remote experts as required.
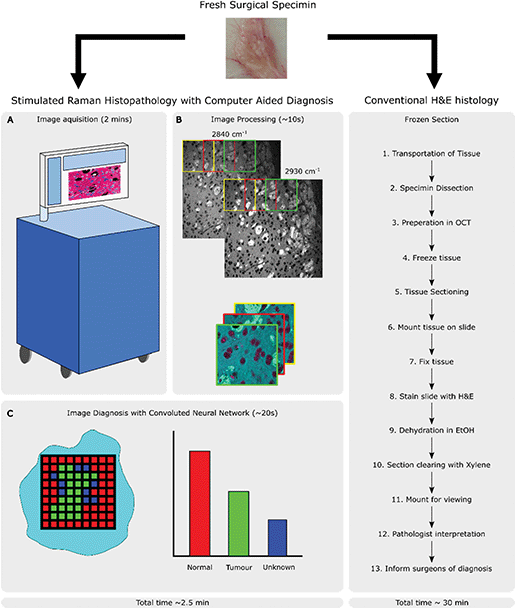 |
| Fig. 2 Intraoperative workflows of both conventional H&E staining histopathology and SRH with computer aided diagnosis. (A) Small freshly excised specimens can be imaged directly in the operating theatre. (B) CH2 and CH3 images are aligned and processed to provide inputs for a convolutional neural network (CNN). (C) The CNN classifies regions of the images as either normal, tumour or as unknown. This process can produce results in under 2.5 min from a 1 mm2 sample, compared to the frozen section route that may take over 30 minutes (and may require larger specimens) and relies on the presence of a trained pathologist. | |
2. Stimulated Raman histopathology in the operating theatre
Whilst laboratory-based SRS systems are often designed with little regard for space requirements, stability or user experience, clinical systems will have stricter design limitations as well as the need to focus on costs. Clinical applications will generally come in one of two formats: imaging previously biopsied material or an in situ scanning device designed to image from either an exposed surface or endoscopically from the patient.
Biopsy imaging systems designed for use in a clinical setting are already involved in many of the trials reported on in this review.10–12 The design shares many similar components to those found in a lab with the sample being scanned by a traditional microscope objective and the sample housed on a moving stage to allow large areas of tissue to be collected (this setup is shown in Fig. 4A). The divergence occurs from the need to construct a system that requires no adjustments to maintain alignment over both long-term use and with shocks that might come from moving equipment around as needed. A fibre laser has been shown to be a good candidate to replace optical parametric oscillators as they are comparatively inexpensive and the difference frequency of the two major fibre gain media, Erbium and Ytterbium, correspond to the high-wavenumber region of Raman spectra used in SRH. Light guided through a fibre core also avoids misalignments that can be introduced by free space optics in uncontrolled environments. Fibre lasers do exhibit more high frequency laser noise though, but it has been demonstrated that this can be removed using an autobalanced detection scheme to achieve shot noise sensitivity.13 A final consideration is the need to package the device into a portable self-contained unit that can be moved around and disinfected as required. Orringer et al.,14 reported that their test system was able to remain in operation in a clinical environment service-free without the need for realignment for years, including shipping across states.
Imaging in situ is a greater challenge requiring a complete redesign of the optics involved to allow them to move to the area of interest, it also requires an epi-detection setup due to the impracticality of collecting transmitted light in most conditions. epi-Detection has been demonstrated in a single frequency system in vivo by using a polarising beam splitter and quarter wave plate to separate the backscattered laser light.15 Bae et al.16 showed that using an epi-detection setup they could differentiate glioblastoma subtypes in bulk brain tissue with hyperspectral SRS imaging.
A simple model of a handheld SRS system created by Liao et al.,17 used a photonic crystal fibre to connect the output of a laser to a handheld combined scanhead and lens which could then be moved to the subject rather than vice versa allowing for measurements from exposed surfaces. Ultimately endoscopic probes could be used to allow SRS histopathology diagnosis rather than requiring biopsy material, however this is a difficult challenge due to the need to carry two spatially and temporally overlapped pulses in a flexible fibre. Lombardini et al.18 demonstrated that by using a hollow-core Kagomé-lattice double-clad fibre that the parasitic signals usually generated within other fibre types due to mixing of the two laser pulses were greatly reduced. Coupled with a set of miniaturised optics this created a 4.2 mm wide probe that allowed for Coherent Raman images to be taken with a sub-micron resolution and a 320 μm field of view, and with further development could be optimised for SRS imaging.
2.1 Clinical trials of the NIO system
Orringer et al.14 conducted the first application of SRS in an operating room using a custom designed system built in collaboration with Invenio Imaging, which later developed into the NIO imaging system. Having previously developed a methodology for SRH, this study demonstrated that they were capable of transferring SRH to the theatre by imaging fresh surgical specimens from central nervous system (CNS) tumours. A trial using 101 neurosurgical patients established that they were able to recapitulate the H&E like stain on fresh tissue by squashing the sample between slide and coverslip. Comparisons between H&E and SRH images from the NIO Imaging system are shown in Fig. 3. Using only two wavelengths in the C–H stretching region (2930 and 2845 cm−1) they showed that key differences in cellularity, vascular pattern and nuclear architecture were visible and could be used to distinguish tumours. 30 of the patients who had undergone intraoperative diagnosis were enrolled in a study to assess the concordance among pathologists who compared adjacent portions of the same specimen that had undergone SRH and frozen section H&E staining. Using SRH pathologists were very accurate in distinguishing lesional from non-lesional tissues (98%), glial from non-glial tumours (100%), and predicting diagnosis (92.2%). Diagnostic discrepancies were thought to be due to the different appearance of nuclear atypia and mitotic features between the two techniques.
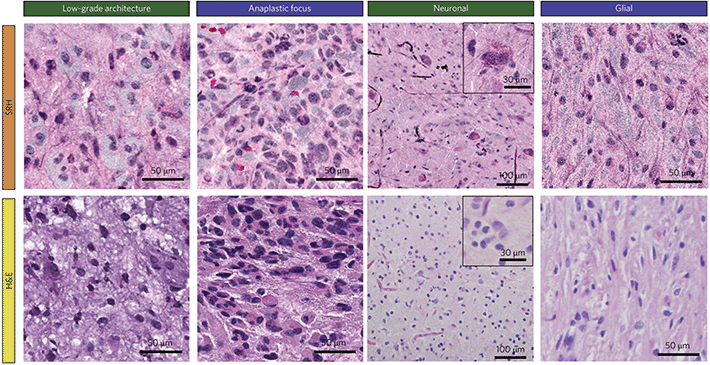 |
| Fig. 3 Comparison of formalin-fixation, paraffin-embedding, H&E-stained images and SRH in selected tumours. SRH reveals architectural differences between a shallow tissue biopsy (green headings) where disorganized binucleated dysplastic neurons predominate, and a deeper biopsy (blue headings), where architecture is more consistent with a hypercellular glioma. Adapted and reproduced from ref. 14 with permission from Springer Nature Publishing Group, copyright 2017. | |
Orringer and colleagues led a further trial on 20 paediatric brain tumours.10 Using the intraoperative pathological diagnosis as ground truth both SRH and frozen section H&E images provided accurate diagnosis (SRH, 92–96%; H&E 92–100%). SRH images highlighted a number of unique features that could aid diagnostics such as high intracellular lipid content in the cytoplasm of tumour associated macrophages and well visualised axons that help differentiate between infiltrative and well-circumscribed tumours. SRH was shown to avoid some tissue processing artefacts associated with freezing that disrupted cytological features.
Eichberg et al.11 led a study whose main objective was to compare the time difference between using the onsite SRH imaging system with that of frozen section analysis. The study compared a variety of CNS tumour types with SRH generated images and produced a time to diagnosis (from specimen removal) that was 30 (±13) minutes quicker than that of frozen section analysis whilst achieving the same diagnostic correlation of 91.5% to permanent sections. The inclusion criteria did mean that well-circumscribed and obvious tumours were included which would not normally require consultation.
The largest trial utilising the NIO Imaging system to date was reported by Hollon et al. consisting of a multi-centre non-inferiority clinical trial of brain tumour diagnosis in 278 patients.12 Diagnostic accuracy of a CNN diagnosis from SRH images was compared with conventional frozen section or smear techniques. Final clinical diagnosis was used as ground truth with the SRH arm providing a slightly higher overall accuracy (264/278) than the control arm (261/278). Interestingly of the 17 errors in the control arm, SRH images correctly classified all of them, whilst the reverse was true of the 14 cases for SRH. Details of the clinical trials carried out with the NIO system are summarised in Table 1.
Table 1 Results from clinical trials of the NIO system
Study DOI |
Year |
Sample size |
Diagnosis |
Critera evaluated |
SRH vs. permanent section accuracy |
10.1038/s41551-016-0027 |
2017 |
30 |
Human |
Lesional from non-lesional |
98% |
|
|
|
|
Glial from non-glial |
100.0% |
|
|
|
|
Predicting diagnosis |
92.2% |
|
|
|
Computational |
Lesional from non-lesional |
100% |
|
|
|
|
Glial from non-glial |
90% |
|
|
|
|
Predicting diagnosis |
90% |
10.1158/0008-5472.CAN-17-1974 |
2018 |
25 |
Human |
Lesional from non-lesional |
97.3% |
|
|
|
|
Low-grade from high-grade |
98% |
|
|
|
|
Predicting diagnosis |
93.3% |
|
|
|
Computational |
Lesional from non-lesional |
93.8% |
|
|
|
|
Low-grade from high-grade |
89.4% |
10.3171/2019.9.JNS192075. |
2019 |
82 |
Human |
Lesional from non-lesional |
97.5% |
|
|
|
|
Predicting diagnosis |
91.5% |
10.1038/s41591-019-0715-9 |
2020 |
278 |
Computational |
Predicting diagnosis |
94.6% |
3. Advances in imaging technology
The NIO system has been vital in showing that SRH is possible in an operating theatre environment but there are a number of potential pathways to increase the imaging speed by altering the optical design which allows faster imaging, as shown in Fig. 4B.
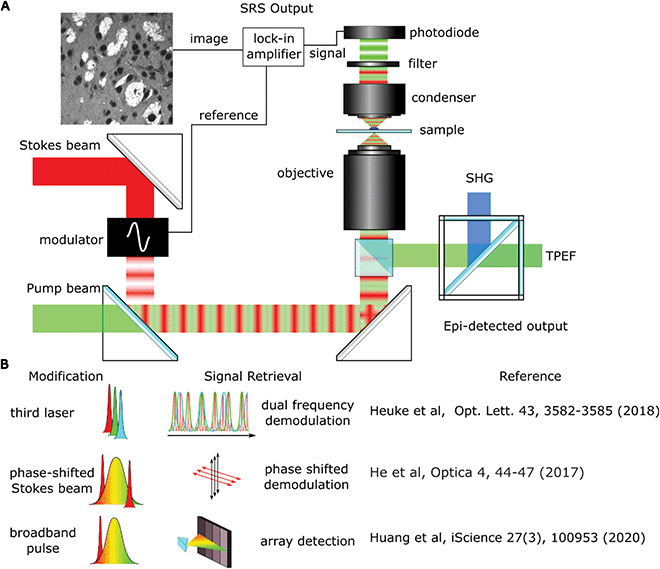 |
| Fig. 4 Advances in SRS imaging technology. (A) Experimental setup of a single frequency SRS system with epi detection of other signals. A modulated Stokes beam and pump beam are directed on to a sample, with the forward scattered light being filtered and demodulated by a lock-in amplifier to generate SRS signals. Back scattered light can be used to generate images for other non-linear imaging modalities. (B) Methods for simultaneous multifrequency imaging with SRS include using the third laser modulated at a second frequency and two lock in amplifiers. For dual phase detection, the Stokes beam is split into two with them being modulated with a 90° phase difference. The SRS signals can then be detected as the in-phase and in-quadrature components of the lock-in amplifier. Finally, multiple Raman modes can be detected simultaneously by using a diffraction grating to disperse a broadband pump beam onto a 32-channel photodetector array. | |
Heuke et al. designed a system that expanded a typical SRS setup with another optical parametric oscillator (OPO) creating a second independently tunable pump beam that can be used over the full range of molecular vibrations.19 By modulating at a second frequency, multiple lock-in amplifiers can separate the individual signals from a single Stokes beam. A modification of this setup was used by Sarri et al.7 to record a difference image between the 2930 cm−1 and 2845 cm−1 frequencies as a frequency modulated (FM) SRS technique alongside coherent anti-Stokes Raman scattering (CARS) to provide the second Raman shift wavelength. This setup halved the acquisition time compared to sequential scanning.20
Using a third laser can be an expensive solution and increases the complexity of alignment and optical design. He et al. showed that you could also create an image at a second frequency using a broadband femtosecond laser with spectral focusing.21 In this setup the Stokes beam was split by a polarising beam splitter to create a second Stokes beam. By careful manipulation of the optics, this second Stokes beam was phase shifted so that the induced modulation could be picked up as the quadrature component of a lock-in amplifier. By using a longer duration pump pulse, the Stokes laser could interact with both the CH2 and CH3 frequencies with little cross talk, also halving the time required for imaging. Another advantage of using broader pulses was shown by Francis et al.22 who investigated the optimal laser pulse width. As the pulse widths became broader they found the signal-to-noise ratio increased due to the broad peaks in the C–H stretch region. Subsequent unmixing of the images showed only small decreases in contrast.
Using a broadband laser can also be used to capture simultaneous spectral data by using a grating to project the broadband pulses onto an array of detectors. Huang et al. used a 32 element photodiode array to allow them to record channels with a spectral resolution of 13.4 cm−1.23 Using an unsupervised algorithm they were then able to cluster cellular compartments based on their signature Raman spectra. Although this approach was used for cytometry it could also be applied to pathological tissue samples.
When scanning large tissue samples the tiling of fields of view together is very inefficient due to the need to scan overlapping regions required for image stitching. This stop-start method of scanning also adds downtime due to the need to move the sample and re-initialize the system. Zhang et al.,24 introduced a strip mosaicing method where strips of the sample were scanned in a continuous fashion by translating the sample whilst the scan mirrors operate in 2D. This reduced the scanning downtime and when combined with their dual phase detection, reduced the imaging time for the whole brain from 70 to 8 minutes.
Hyperspectral imaging, where many different wavelengths are scanned to build up SRS spectra could be used to measure more subtle shifts in the vibrational spectra which have traditionally been used for spontaneous Raman diagnostics of tumours.25 Whilst manually tuning a laser is possible, this approach is often time consuming and so the selective filtering of a broadband source is often preferred. Alshaykh et al., used a combination of acousto-optic programmable dispersive filters and a spectral focusing method to scan a spectral width of 200 cm−1 which covers the C–H stretch region almost completely in 12.5 μs.26 Audier et al.,27 subsequently showed that using this technique on biopsy samples could generate images with suitable quality for SRH. Further utilisation of the spectral data encoded in each pixel could allow for far more detailed feature extraction to be carried out than image recognition algorithms alone are capable of.
4. Quantitative imaging
Quantification of Raman signals with SRS is possible as there is a linear dependence of the signal intensity with chemical concentration, however, this can be hampered and due to irregular light scattering within the tissue, as well as overlapping chemical species. Oh et al.,28 developed a technique they termed Normalized Raman Imaging (NoRI), to computationally remove the effect of tissue light scattering during spectral decomposition to calculate the absolute concentrations of cellular components. By assuming that the sum of protein, lipid and water was constant in a tissue sample the attenuation caused by the sample itself could be removed. Following linear decomposition of SRS scans taken at methyl, methylene and oxygen–hydrogen stretching peaks, the sum of these three components also serves as the normalisation mask for the tissue. The decomposition process was calibrated using bovine serum albumin, dioleoyl-phosphocholine and pure water as the standards for protein, lipid and water respectively, with mass concentrations estimated by multiplying the volume fraction of the components with an estimated density of pure protein, lipid or water. The method was validated by comparing NoRI calculations against known concentrations of BSA solutions, and in situ NoRI quantification of total protein and lipid mass density in chondrocytes. This showed good agreement with dry mass density measured by refractive index tomography, although small differences could also be explained by the contribution of other chemical species such as nucleic acids or glycoproteins which are not accounted for. Apart from acquiring high quality contrast for the identification of a variety of histological features in mouse cerebellum and pancreas, the utility of NoRI in quantitative histopathology was demonstrated by imaging the brain of mice from a model of Alzheimer's disease, where protein rich amyloid plaques were visualised in the extracellular space, surrounded by high lipid concentration, which were distinct from the patterns of protein and lipid organisation observed in normal mouse brain.
5. Computer aided diagnosis
Computer aided diagnosis (CAD) is well integrated in radiology but its adoption in histopathology has been slower.29 While CAD is used to diagnose the presence of a tumour, histopathology is required to classify such tumours and inform the course of treatment, consequently requiring more complex systems. Furthermore, the use of CAD in histopathology requires digital images but histopathology has traditionally relied on the pathologist viewing glass slides at the microscope. Recently advances have been made with the introduction of whole slide imaging (WSI) and digital pathology and its adoption in some hospitals for all primary histopathological diagnosis.30,31 With the increased use of digital pathology, machine learning (ML) approaches to classifying histopathological samples are being investigated as reviewed by Komura et al.32 The motivation for developing automated processes for interpreting slides is driven by a shortage of specialist pathologists and the potential to decrease the time to determine a preliminary diagnosis as discussed above.7,33,34 The large data sets generated from SRH can lend themselves to ML approaches to rapidly classify tissue. Orringer et al., have developed ML approaches to enable automated diagnosis of CNS tumours from their SRH images – as reviewed by Khalsa et al.35 Their approach has evolved from using vector-based multilayer perceptron (MLP) which are trained using specialised knowledge of CNS tumours to manually define important features (nuclear morphology, cell density, vascularity, etc.) and can result in biasing of data,10 to using deep CNN that retain spatial information and learn what image features are important in determining diagnosis and predicting patient outcomes.12
All ML approaches require access to large numbers of classified and unclassified images to train the networks. However, once trained deep neural networks have been shown to accurately differentiate neoplastic specimens from normal tissue in laryngeal squamous cell carcinoma36 and in brain tumours (Fig. 5) and to have a diagnostic accuracy of 94.6%, comparable with H&E samples used by specialist pathologists at 93.9%.12 Interestingly, Hollon et al.,12 found that the CNN correctly classified those misclassified by pathologists and pathologists correctly classified those misclassified by the CNN, which suggests that pathologists aided by automated classification systems will improve diagnostic accuracy, particularly in difficult cases. Additionally, insight into important features used to classify images can be extracted latterly from the classification programme.
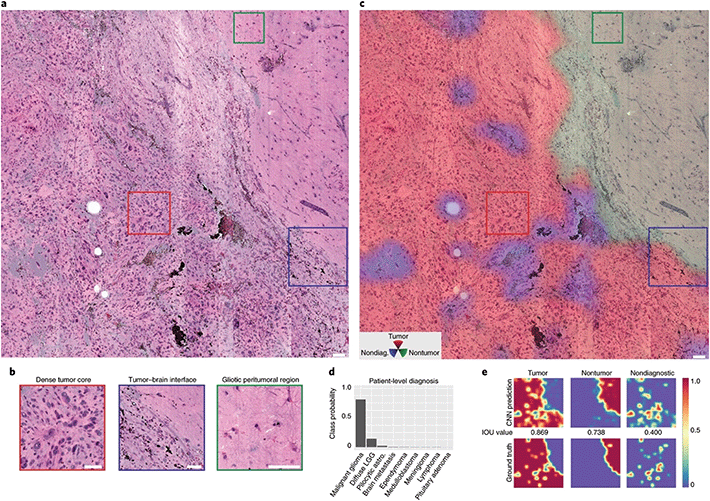 |
| Fig. 5 Example of SRS histopathology using a CNN (ResNet 34) to aid with diagnosis. (a) Full SRH mosaic of a brain-tumour interface of a patient diagnosed with glioblastoma. (b) Dense hypercellular glial tumour with nuclear atypia is seen diffusely on the left and peritumoral gliotic brain with reactive astrocytes on the right of the specimen. (c) Three channel RGB CNN prediction transparency overlaid on the SRH image. (d) Associated patient level diagnostic class probabilities. (e) Inference class probability heatmaps for tumour, non-tumour and non-diagnostic regions within the SRH image. Adapted and reproduced from ref. 12 with permission from Springer Nature Publishing Group, copyright 2020. | |
6. Beyond H&E
Shin et al., highlighted some of the limitations of using just SRH for the detection of complex pathologies.34 Assessing a broad range of skull base tumours, where cytoarchitecture can be lost during freezing and sectioning, pseudo-H&E coloured SRH images achieved a relative accuracy of 87% compared to permanent sections. The high accuracy was mainly driven by a large number of easy to diagnose meningiomas. Other tumour types like chondosarcoma (0% accuracy) and schwannoma (44% accuracy) proved more challenging due to the absence of H&E features in the SRH images. The authors posited that in some tumour types lipid and protein contrast did not offer enough diagnostic features. By imaging at other SRS frequencies or using more label-free imaging modes additional diagnostic contrast can be added to images than just revealed by CH3/CH2 imaging alone. Some of these virtual ‘stains’ that either have been used in conjunction with SRH or could easily be added due to the similarity of microscope setup are discussed below and summarised in Fig. 6.
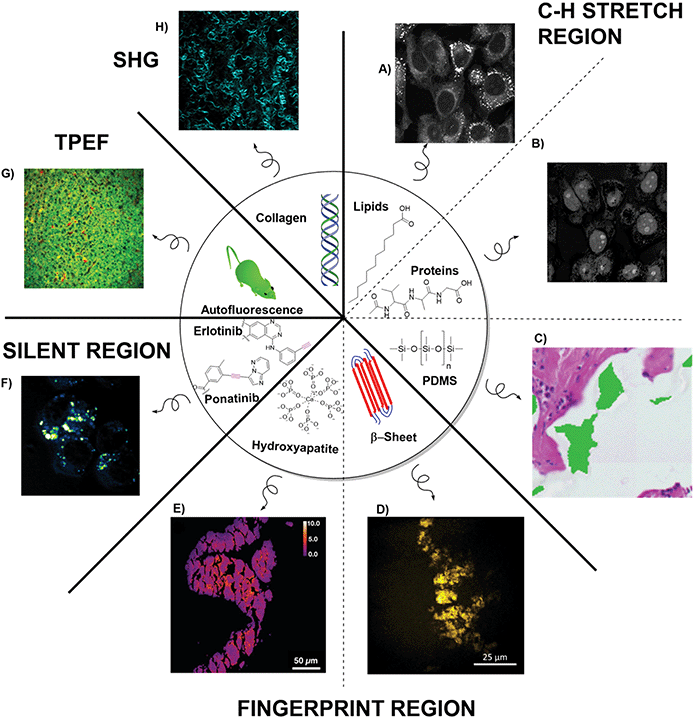 |
| Fig. 6 Overview of the different imaging ‘stains’ that multimodal histology can provide. Stimulated Raman Scattering in the C–H stretch region can image (A) cytoplasm and organelles of cells using the CH2 stretch, (B) isolated nuclei using the difference of CH3 and CH2 images, (C) silicon from implants (image reproduced from ref. 42 with permission from Wiley Online Library, copyright 2020). Imaging in the fingerprint region can show (D) build-up of amyloid plaques (image reproduced from48 with permission from Springer Nature Publishing Group, copyright 2015), or (E) the formation of calcifications (image reproduced from ref. 47 with permission from Ivyspring International, copyright 2020). Imaging in the silent region can allow (F) the direct visualisations of alkyne containing drugs such as ponatinib. Using multiphoton imaging can give details on (G) autofluorescence life-time imaging for metabolic tissue profiling (two-photon-excited fluorescence: TPEF), as well as (H) SHG imaging to image collagen fibres. | |
6.1 Second harmonic generation and other non-linear modalities
A number of studies have incorporated the use of second harmonic generation (SHG) to visualise collagen fibres within tissue samples. For example, working on laryngeal cancers Zhang et al.,36 first compared 80 pairs of frozen sections imaged with SRS at the CH2 and CH3 wavelengths and SHG, with H&E staining to demonstrate that pathologists could use the combined SRH and SHG images for diagnosis with high accuracy (94.2% vs. 94.6% accuracy SRH vs. frozen section H&E). Unlike other work, the SRH images were not pseudo-coloured to an H&E like stain but a brief overview was given to the pathologists. Like Hollon et al.,12 a CNN was trained on 45 fresh samples from patients and used to classify 33 independent surgical specimens with 100% accuracy compared to standard H&E paraffin sections.
A saffron stain, which marks collagen in connective tissue, can be added to an H&E stain (HES) to image gastrointestinal tumours as the macro and microscopic organisation of collagen can be an important indicator of cancer development and also help to guide intraoperative decision making. Sarri et al.,33 presented direct one-to-one comparisons of cryosections imaged first by SRH and SHG, then processed for HES staining. The combined SRH/SHG images were recoloured with look-up tables used to match those that are produced with traditional staining. SHG and SRH were coloured orange, pink and dark purple to match saffron, eosin and haematoxylin staining. The recoloured images showed a high image correlation coefficient to the HES images, with differences often attributed to the inherent sectioning ability of SRH or slight variations in colouration. Features that were characteristic of both healthy areas of tissue and dysplasia could be readily visualised and although the intrinsic SRH z-sectioning ability was responsible for a few missing nuclei compared to HES, the overall image was sharper.
In addition to recording SHG images simultaneously, SRS microscope setups are often amenable to collecting other label-free non-linear signals that could be used to provide more contrast for the area being scanned. Whilst using frequency modulation-SRS (FM-SRS) to image nuclei, Sarri et al., were able to use either coherent anti-Stokes Raman scattering (CARS) or two-photon auto fluorescence to image the cellular body.7 Although CARS exhibits a non-resonant background which usually makes SRS preferable, the signal from the C–H stretch region is well defined and provides a strong backwards scattered signal that can be more easily detected in thick samples.
Autofluorescence from the cell is typically from oxidative chain respiratory proteins NADH and FAD and high levels of NADH have been associated with glycolysis or regions of hypoxia.37,38 Bower et al., have demonstrated that incorporation of fluorescence lifetime imaging microscopy (FLIM) is able to separate free and bound NADH in living samples, and developed a video rate FLIM system which could add information on metabolic activity to the images.39
6.2 Imaging in the C–H region
6.2.1 DNA Imaging.
Imaging DNA in the fingerprint region has previously been reported but only in cells with condensed chromatin/chromosomes to allow sufficiently concentrated DNA to generate an SRS signal.40 An approach that would allow DNA imaging even in interphase was later demonstrated by using a distinct spectral feature of DNA in the C–H stretching region which when coupled with linear decomposition allowed a higher sensitivity DNA imaging than that found in the fingerprint region which could help add better nuclear morphology and architecture than current SRH techniques.15 Hill et al.,41 were able to further enhance imaging of DNA within this region by using a polarisation encoded FM-SRS technique designed to isolate spectral contributions from low-signal species. By tuning the frequency difference so that there is a roughly equivalent contribution of protein at each vibrational transition measured during the frequency modulation it can be cancelled out, highlighting any contribution from DNA C–H stretches in the ‘on-resonance’ portion.
6.2.2 Imaging silicone implants.
Currently, silicone detection in histopathological slides from women with silicone breast implant failures relies on morphological features. Haasterecht et al., developed an SRS technique to specifically detect micrometer (≥2 μm) sized polydimethylsiloxane (PDMS; the filling of modern implants) fragments that have a C–H stretch vibrational frequency at 2905 cm−1 which is distinct from that of the major tissue constituents.42 Histopathological samples collected following removal of failed implants identified large aggregates of PDMS. In one case, localization within vacuoles was detected in an enlarged axillary lymph node from a patient with a ruptured implant. Deparaffinised H&E stained slides did not interfere with the SRS signal for silicone distribution and quantification. It is anticipated that the scope of this technique can be broadened to thicker samples and simultaneous tissue component analysis for improved pathophysiological understanding of silicone implant failures.
6.3 Imaging in the silent region
The absence of any competing inherent biological signals allows SRS to be used to image relatively low concentrations of molecules that contain Raman peaks in the silent region. Whilst this has usually been used to add a small Raman active label such as an alkyne to a target molecule43,44 there are a number of clinical drugs that already contain this bond in their structure. Sepp et al.,45 were able to image ponatinib, an alkyne containing tyrosine kinase inhibitor used to treat chronic myeloid leukaemia at biologically relevant concentrations. This demonstrates a potential to visualise drug distributions within tissues simultaneously with SRH.
6.4 Imaging in the fingerprint region
There is a Raman spectral change in the amide I band (to 1670 cm−1) of the β-sheets within misfolded proteins that form in amyloid plaques, compared to native proteins (1658 cm−1). The deposition of amyloid plaques is a hallmark of Alzheimer's disease and this allowed Ji et al. to rapidly detect and quantify misfolded amyloid plaques in brain tissue taken from a mouse model of Alzheimer's disease. Using a hyperspectral picosecond narrow band laser-based SRS set up to collect images at three Raman frequencies (1640, 1658, and 1670 cm−1), together with a linear decomposition algorithm, allowed extraction of spectral information from misfolded proteins in the fingerprint region. Interestingly, unlike other imaging methods, three-color SRS revealed that the plaques are surrounded by lipid-rich halo structures whose relationship with the pathophysiology of amyloid plaques is yet unknown. Fresh tissue samples gave the best images, as tissue processing induced protein conformational changes that reflected directly on the amide I spectral signature.46
Shin et al., demonstrated the clinical value of hyperspectral SRS imaging for breast cancer diagnosis via provision of spatially resolved qualitative and quantitative information on tissue calcification.47 A broadband femtosecond dual-beam laser system was used to quantify the carbonate content of hydroxyapatite, the main calcification species associated with cancer. The ratio of carbonate (∼1070 cm−1) to phosphate (∼960 cm−1) peaks mapped the intra- and inter-tissue calcification heterogeneity among breast cancer tissue samples. A variety of calcifications were identified whose chemical composition could be correlated to the local malignancy, degree of advancement and type of breast cancer. SHG was incorporated to visualize calcified organic matrix components to bridge the information gap where carbonate content was insufficient for diagnosis such as the differentiation between fibroadenoma and invasive ductal carcinoma. Of a total 214 breast calcifications imaged for separating benign from neoplastic processes, >85% sensitivity and specificity were reached. The feasibility for combining calcium imaging and SHG with SRH will enable comprehensive diagnosis and potentially minimize inter-pathologist discordance for effective prognosis.
7. Correlated imaging with SRS histopathology
As SRS histopathology only involves light interacting with the sample, and no sample preparation steps, it is well placed to be used alongside other analytical processes that require unprocessed tissue. This can either be done on the same piece of tissue, or using an adjacent section where correlation of features is eased due to the lack of distortions introduced during normal tissue processing. Randall et al.,49 co-registered SRS histopathology images of brain slices with a serial section using quantitative MALDI-MSI by an automatic t-distributed stochastic neighbour embedding based registration method to image the distribution of the tyrosine kinase inhibitor erlotinib. Images showed that the protein rich tumour core contained higher drug concentrations but the tumour edge had an inverse relationship between higher lipid content and drug content. Erlotinib showed limited diffusion across regions of intact blood brain barrier within regions of normal brain matter.
8. 3D histopathology
Current histopathological techniques rely on the examination of thin (5 μm) sections for light microscopy, with fewer than 5 sections typically examined during a frozen section procedure. Imaging the whole volume of a centimetre-sized tissue block would require the examination of 2000 serial sections, which is not feasible. Imaging the tissue block by sectioning it into a few thicker slices (250 μm–2 mm) would allow the generation of 3D images, removing the inherent sampling error of the conventional approach and potentially identifying focal abnormalities not present in the initial, superficial, sections. As SRS imaging requires no labelling, this would also save time and resources during sample preparation, and avoid variation in staining intensity among traditionally labelled slices.
The most limiting factor of imaging in 3D using SRS microscopy is imaging depth. Hill et al. explored SRS imaging depth in four different tissue types – brain, kidney, liver and lung.50 Using ex vivo murine samples at 1 mm thickness, they determined maximum imaging depths for each tissue type (kidney 165 μm, liver 170 μm, brain 185 μm, lung 70 μm). Lung tissue had the poorest penetration depth (70 μm), due to its strong light scattering properties. For brain tissue, they also compared penetration depth with varying sample thickness (250 μm, 500 μm, 1 mm, 2 mm), and found that sample thickness plays a role in how deep SRS can image. Penetration depth was best for thinner samples (205 μm for 250 μm sample) and decreased as sample thickness increased (130 μm for 2 mm sample).
To improve penetration depth, Li et al., optimised tissue clearing methods in brain samples.51 Using formamide as a clearing agent improved SRS imaging depth and allowed penetration up to 500 μm in brain slices. The same method was also successfully applied to kidney, liver and lung tissue imaging. This demonstrates that SRS can be successfully used in 3D histopathology. However, further research is required to explore how the 3D data could be used to add value in pathology.
9. Conclusions and future perspectives
Conventional histopathology methods are limited by the requirement for time-consuming and expensive laboratory processing, and are restricted to the analysis of thin 2D sections by light microscopy. Optical imaging methods based on Raman scattering offer the prospect of not only generating equivalent information using a label-free approach but also producing additional information based on chemical signatures and 3D structure. Coupled with artificial intelligence approaches that can derive molecular information from histopathological images,52 this paves the way for enhanced histopathology by providing pathologists with additional diagnostics tools. This has the potential to enhance patient outcomes, the former through improved diagnostic accuracy and the latter by obviating the need for tissue biopsy, with consequent improvement in clinical efficiency.
Conflicts of interest
There are no conflicts of interest to declare.
Acknowledgements
This work was supported by Cancer Research UK grants (C8618/A27574 and C157/A25140), EPSRC and MRC (OPTIMA CDT Studentship to KS, EP/L016559/1).
References
- S. B. Sams and J. A. Wisell, Discordance Between Intraoperative Consultation by Frozen Section and Final Diagnosis, Int. J. Surg. Pathol., 2017, 25(1), 41–50 CrossRef PubMed
.
- E. Mahe, S. Ara and M. Bishara,
et al., Intraoperative pathology consultation: error, cause and impact, Can. J. Surg., 2013, 56(3), E13–E18 CrossRef PubMed
.
- H. Jaafar, Intra-operative frozen section consultation: concepts, applications and limitations, Malays. J. Med. Sci., 2006, 13(1), 4–12 Search PubMed
.
- D. A. Novis and R. J. Zarbo, Interinstitutional comparison of frozen section turnaround time. A College of American Pathologists Q-Probes study of 32868 frozen sections in 700 hospitals, Arch. Pathol. Lab. Med., 1997, 121(6), 559–567 CAS
.
- H. J. Butler, J. M. Cameron and C. A. Jenkins,
et al., Shining a light on clinical spectroscopy: Translation of diagnostic IR, 2D-IR and Raman spectroscopy towards the clinic, J. Clin. Spectrosc., 2019, 1, 100003 CrossRef
.
- W. Min, C. W. Freudiger, S. Lu and X. S. Xie, Coherent nonlinear optical imaging: beyond fluorescence microscopy, Annu. Rev. Phys. Chem., 2011, 62, 507–530 CrossRef CAS PubMed
.
- B. Sarri, R. Canonge and X. Audier,
et al., Fast stimulated Raman and second harmonic generation imaging for intraoperative gastro-intestinal cancer detection, Sci. Rep., 2019, 9(1), 10052 CrossRef PubMed
.
- M. T. Cicerone and C. H. Camp, Histological coherent Raman imaging: a prognostic review, Analyst, 2017, 143(1), 33–59 RSC
.
- Y. F. Yang, L. C. Chen and M. B. Ji, Stimulated Raman scattering microscopy for rapid brain tumor histology, J. Innovative Opt. Health Sci., 2017, 10(5), 1730010 CrossRef CAS
.
- T. C. Hollon, S. Lewis and B. Pandian,
et al., Rapid Intraoperative Diagnosis of Pediatric Brain Tumors Using Stimulated Raman Histology, Cancer Res., 2018, 78(1), 278–289 CrossRef CAS PubMed
.
- D. G. Eichberg, A. H. Shah and L. Di,
et al., Stimulated Raman histology for rapid and accurate intraoperative diagnosis of CNS tumors: prospective blinded study, J. Neurosurg., 2019, 1–7 Search PubMed
.
- T. C. Hollon, B. Pandian and A. R. Adapa,
et al., Near real-time intraoperative brain tumor diagnosis using stimulated Raman histology and deep neural networks, Nat. Med., 2020, 26(1), 52–58 CrossRef CAS PubMed
.
- C. W. Freudiger, W. Yang, G. R. Holtom, N. Peyghambarian, X. S. Xie and K. Q. Kieu, Stimulated Raman Scattering Microscopy with a Robust Fibre Laser Source, Nat. Photonics, 2014, 8(2), 153–159 CrossRef CAS PubMed
.
- D. A. Orringer, B. Pandian and Y. S. Niknafs,
et al., Rapid intraoperative histology of unprocessed surgical specimens via fibre-laser-based stimulated Raman scattering microscopy, Nat. Biomed. Eng., 2017, 1 Search PubMed
.
- F. K. Lu, S. Basu and V. Igras,
et al., Label-free DNA imaging in vivo with stimulated Raman scattering microscopy, Proc. Natl. Acad. Sci. U. S. A., 2015, 112(37), 11624–11629 CrossRef CAS PubMed
.
- K. Bae, W. Zheng and K. Lin,
et al., Epi-Detected Hyperspectral Stimulated Raman Scattering Microscopy for Label-Free Molecular Subtyping of Glioblastomas, Anal. Chem., 2018, 90(17), 10249–10255 CrossRef CAS PubMed
.
- C.-S. Liao, P. Wang and C. Y. Huang,
et al., In Vivo and in Situ Spectroscopic Imaging by a Handheld Stimulated Raman Scattering Microscope, ACS Photonics, 2018, 5(3), 947–954 CrossRef CAS
.
- A. Lombardini, V. Mytskaniuk and S. Sivankutty,
et al., High-resolution multimodal flexible coherent Raman endoscope, Light: Sci. Appl., 2018, 7, 10 CrossRef PubMed
.
- S. Heuke, B. Sarri, X. Audier and H. Rigneault, Simultaneous dual-channel stimulated Raman scattering microscopy demultiplexed at distinct modulation frequencies, Opt. Lett., 2018, 43(15), 3582–3585 CrossRef CAS PubMed
.
- D. Zhang, M. N. Slipchenko, D. E. Leaird, A. M. Weiner and J. X. Cheng, Spectrally modulated stimulated Raman scattering imaging with an angle-to-wavelength pulse shaper, Opt. Express, 2013, 21(11), 13864–13874 CrossRef PubMed
.
- R. Y. He, Y. K. Xu and L. L. Zhang,
et al., Dual-phase stimulated Raman scattering microscopy for real-time two-color imaging, Optica, 2017, 4(1), 44–47 CrossRef CAS
.
- A. Francis, K. Berry, Y. Chen, B. Figueroa and D. Fu, Label-free pathology by spectrally sliced femtosecond stimulated Raman scattering (SRS) microscopy, PLoS One, 2017, 12(5), e0178750 CrossRef PubMed
.
- K. C. Huang, J. Li, C. Zhang, Y. Tan and J. X. Cheng, Multiplex Stimulated Raman Scattering Imaging Cytometry Reveals Lipid-Rich Protrusions in Cancer Cells under Stress Condition, iScience, 2020, 23(3), 100953 CrossRef CAS PubMed
.
- B. Zhang, M. Sun and Y. Yang,
et al., Rapid, large-scale stimulated Raman histology with strip mosaicing and dual-phase detection, Biomed. Opt. Express, 2018, 9(6), 2604–2613 CrossRef CAS PubMed
.
- R. O. Draga, M. C. Grimbergen and P. L. Vijverberg,
et al., In vivo bladder cancer diagnosis by high-volume Raman spectroscopy, Anal. Chem., 2010, 82(14), 5993–5999 CrossRef CAS PubMed
.
- M. S. Alshaykh, C. S. Liao and O. E. Sandoval,
et al., High-speed stimulated hyperspectral Raman imaging using rapid acousto-optic delay lines, Opt. Lett., 2017, 42(8), 1548–1551 CrossRef CAS PubMed
.
- X. Audier, N. Forget and H. Rigneault, High-speed chemical imaging of dynamic and histological samples with stimulated Raman micro-spectroscopy, Opt. Express, 2020, 28(10), 15505–15514 CrossRef CAS PubMed
.
- S. Oh, C. Lee and D. Fu,
et al., In situ measurement of absolute concentrations by Normalized Raman Imaging, bioRxiv, 2019, 629543 Search PubMed
.
- S. Nam, Y. Chong and C. K. Jung,
et al., Introduction to digital pathology and computer-aided pathology, J. Pathol. Transl. Med., 2020, 54(2), 125–134 CrossRef PubMed
.
- J. Griffin and D. Treanor, Digital pathology in clinical use: where are we now and what is holding us back?, Histopathology, 2017, 70(1), 134–145 CrossRef PubMed
.
- J. A. Retamero, J. Aneiros-Fernandez and R. G. Del Moral, Complete Digital Pathology for Routine Histopathology Diagnosis in a Multicenter Hospital Network, Arch. Pathol. Lab. Med., 2020, 144(2), 221–228 CrossRef PubMed
.
- D. Komura and S. Ishikawa, Machine Learning Methods for Histopathological Image Analysis, Comput. Struct. Biotechnol. J., 2018, 16, 34–42 CrossRef CAS PubMed
.
- B. Sarri, F. Poizat and S. Heuke,
et al., Stimulated Raman histology: one to one comparison with standard hematoxylin and eosin staining, Biomed. Opt. Express, 2019, 10(10), 5378–5384 CrossRef CAS PubMed
.
- K. S. Shin, A. T. Francis and A. H. Hill,
et al., Intraoperative assessment of skull base tumors using stimulated Raman scattering microscopy, Sci. Rep., 2019, 9(1), 20392 CrossRef CAS PubMed
.
- S. S. S. Khalsa, T. C. Hollon and A. Adapa,
et al., Automated histologic diagnosis of CNS tumors with machine learning, CNS Oncol., 2020, 9(2), CNS56 CrossRef CAS PubMed
.
- L. Zhang, Y. Wu and B. Zheng,
et al., Rapid histology of laryngeal squamous cell carcinoma with deep-learning based stimulated Raman scattering microscopy, Theranostics, 2019, 9(9), 2541–2554 CrossRef CAS PubMed
.
- S. You, Y. Sun and E. J. Chaney,
et al., Slide-free virtual histochemistry (Part I): development via nonlinear optics, Biomed. Opt. Express, 2018, 9(11), 5240–5252 CrossRef CAS PubMed
.
- J. H. Lee, J. J. Rico-Jimenez and C. Zhang,
et al., Simultaneous label-free autofluorescence and multi-harmonic imaging reveals in vivo structural and metabolic changes in murine skin, Biomed. Opt. Express, 2019, 10(10), 5431–5444 CrossRef CAS PubMed
.
- A. J. Bower, J. Li, E. J. Chaney, M. Marjanovic, D. R. Spillman Jr. and S. A. Boppart, High-speed imaging of transient metabolic dynamics using two-photon fluorescence lifetime imaging microscopy, Optica, 2018, 5(10), 1290–1296 CrossRef CAS PubMed
.
- X. Zhang, M. B. Roeffaers and S. Basu,
et al., Label-free live-cell imaging of nucleic acids using stimulated Raman scattering microscopy, ChemPhysChem, 2012, 13(4), 1054–1059 CrossRef CAS PubMed
.
- A. H. Hill, E. Munger, A. T. Francis, B. Manifold and D. Fu, Frequency Modulation Stimulated Raman Scattering Microscopy through Polarization Encoding, J. Phys. Chem. B, 2019, 123(40), 8397–8404 CrossRef CAS PubMed
.
- L. van Haasterecht, L. Zada and R. W. Schmidt,
et al., Label-free stimulated Raman scattering imaging reveals silicone breast implant material in tissue, J. Biophotonics, 2020, 13(5), e201960197 Search PubMed
.
- W. J. Tipping, M. Lee, A. Serrels, V. G. Brunton and A. N. Hulme, Stimulated Raman scattering microscopy: an emerging tool for drug discovery, Chem. Soc. Rev., 2016, 45(8), 2075–2089 RSC
.
- Z. Zhao, Y. Shen, F. Hu and W. Min, Applications of vibrational tags in biological imaging by Raman microscopy, Analyst, 2017, 142(21), 4018–4029 RSC
.
- K. Sepp, M. Lee, M. T. J. Bluntzer, G. V. Helgason, A. N. Hulme and V. G. Brunton, Utilizing Stimulated Raman Scattering Microscopy To Study Intracellular Distribution of Label-Free Ponatinib in Live Cells, J. Med. Chem., 2020, 63(5), 2028–2034 CrossRef CAS PubMed
.
- M. Ji, M. Arbel and L. Zhang,
et al., Label-free imaging of amyloid plaques in Alzheimer's disease with stimulated Raman scattering microscopy, Sci. Adv., 2018, 4(11), eaat7715 CrossRef CAS PubMed
.
- K. S. Shin, M. Laohajaratsang, S. Men, B. Figueroa, S. M. Dintzis and D. Fu, Quantitative chemical imaging of breast calcifications in association with neoplastic processes, Theranostics, 2020, 10(13), 5865–5878 CrossRef CAS PubMed
.
- J. Kiskis, H. Fink, L. Nyberg, J. Thyr, J. Y. Li and A. Enejder, Plaque-associated lipids in Alzheimer's diseased brain tissue visualized by nonlinear microscopy, Sci. Rep., 2015, 5, 13489 CrossRef CAS PubMed
.
- E. C. Randall, K. B. Emdal and J. K. Laramy,
et al., Integrated mapping of pharmacokinetics and pharmacodynamics in a patient-derived xenograft model of glioblastoma, Nat. Commun., 2018, 9(1), 4904 CrossRef PubMed
.
- A. H. Hill, B. Manifold and D. Fu, Tissue imaging depth limit of stimulated Raman scattering microscopy, Biomed. Opt. Express, 2020, 11(2), 762–774 CrossRef CAS PubMed
.
- J. Li, P. Lin, Y. Tan and J. X. Cheng, Volumetric stimulated Raman scattering imaging of cleared tissues towards three-dimensional chemical histopathology, Biomed. Opt. Express, 2019, 10(8), 4329–4339 CrossRef CAS PubMed
.
- J. N. Kather, A. T. Pearson and N. Halama,
et al., Deep learning can predict microsatellite instability directly from histology in gastrointestinal cancer, Nat. Med., 2019, 25(7), 1054–1056 CrossRef CAS PubMed
.
|
This journal is © The Royal Society of Chemistry 2021 |