DOI:
10.1039/D0AN01482F
(Critical Review)
Analyst, 2021,
146, 770-788
Discrimination of bacteria using whole organism fingerprinting: the utility of modern physicochemical techniques for bacterial typing
Received
27th July 2020
, Accepted 18th November 2020
First published on 18th November 2020
Abstract
Rapid and accurate classification and discrimination of bacteria is an important task and has been highlighted recently for rapid diagnostics using real-time results. Coupled with a recent report by Jim O'Neill [https://amr-review.org] that if left unaddressed antimicrobial resistance (AMR) in bacteria could kill 10 million people per year by 2050, which would surpass current cancer mortality, this further highlights the need for unequivocal identification of microorganisms. Whilst traditional microbiological testing has offered insights into the characterisation and identification of a wide range of bacteria, these approaches have proven to be laborious and time-consuming and are not really fit for purpose, considering the modern day speed and volume of international travel and the opportunities it creates for the spread of pathogens globally. To overcome these disadvantages, modern analytical methods, such as mass spectrometry (MS) and vibrational spectroscopy, that analyse the whole organism, have emerged as essential alternative approaches. Currently within clinical microbiology laboratories, matrix assisted laser desorption ionisation (MALDI)-MS is the method of choice for bacterial identification. This is largely down to its robust analysis as it largely measures the ribosomes which are always present irrespective of how the bacteria are cultured. However, MALDI-MS requires large amounts of biomass and infrared spectroscopy and Raman spectroscopy are attractive alternatives as these physicochemical bioanalytical techniques have the advantages of being rapid, reliable and cost-effective for analysing various types of bacterial samples, even at the single cell level. In this review, we discuss the fundamental applications, advantages and disadvantages of modern analytical techniques used for bacterial characterisation, classification and identification.
| Najla AlMasoud obtained her BSc in Chemistry from Princess Nourah bint Abdulrahman University (PNU), Saudi Arabia. Najla was also awarded her Masters and PhD degrees from the University of Manchester, UK and is currently an Assistant Professor in Chemistry and a member of the IRB community at PNU. Prior to this, she managed the community services at the college of science at PNU from 2016 to 2019. Najla published a number of scientific papers in international journals with regard to modern analytical techniques for bacterial characterization including discrimination, rapid detection of foodborne bacteria, environmental metabolomics, and analysis of pharmaceutical compounds using enhanced Raman scattering. Najla is interested in nanotechnology, in line with analytical techniques. |
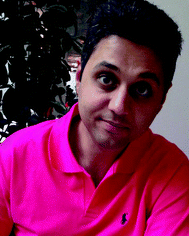 Howbeer Muhamadali | Howbeer Muhamadali received first class honours in Microbiology at the Manchester Metropolitan University in 2010. In 2011, he successfully completed an MPhil in geomicrobiology at the University of Manchester and continued with a PhD program (Biotechnology/Metabolomics) working on metabolomics investigations of different microbial bioprocesses using various analytical techniques and multivariate statistical analysis approaches. In Dec 2019, he started his own group at the University of Liverpool as a tenure track fellow, working on the applications of molecular imaging and metabolomics techniques for better understanding of antimicrobial resistance at single cell and community (biofilm) levels. |
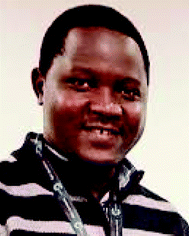 Malama Chisanga | Malama Chisanga graduated with top class distinction in Chemistry from Copperbelt University, Zambia in 2012, before completing an MSc at the University of Manchester in 2014. In 2020, he successfully completed his PhD in Analytical Chemistry under the mentorship of Professor Roy Goodacre and Professor Nick Lockyer at the University of Manchester. Malama's project involved the development of SERS, Raman spectroscopy and FT-IR spectroscopy combined with multivariate statistical techniques to probe metabolic functions and phenotypic characteristics of clinically and industrially relevant bacteria within complex microbial communities. He re-joined Roy Goodacre's research laboratories at the University of Liverpool in 2020 where he worked as an honorary research associate. Malama's current research involves the investigation of kinetics of substrate uptake and cross-feeding experiments of metabolites among microbes residing in mixed microbial consortia using several analytical approaches including SERS, Raman spectroscopy, and GC-MS platforms. |
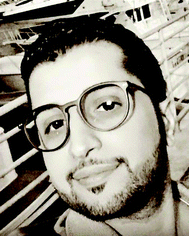 Haitham AlRabiah | Haitham AlRabiah is an Associate Professor in the Department of Pharmaceutical Chemistry at King Saud University, where he has been a faculty member since 2006. Haitham completed his PhD at the University of Manchester in metabolomics under the supervision of Professor Roy Goodacre, his MSc in Pharmaceutical Analysis at the University of Strathclyde and his undergraduate studies at the College of Pharmacy at KSU. His research interests lie in the area of pharmaceutical analysis, drug metabolism, biomarker diagnostics and metabolomics. He has co-authored more than 60 original research articles in his field of interest, and supervised several PhD students. |
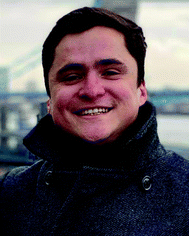 Cassio A. Lima | Cassio Lima obtained his B.Sc. in Physics from the Federal University of Uberlandia (Brazil) in 2013 and M.Sc. (2015) and PhD (2019) degrees from the University of Sao Paulo (Brazil). He joined Prof. Roy Goodacre's group at the University of Liverpool (UK) in 2019, where he currently works as a research associate. His main area of research involves characterisation of biological systems via vibrational spectroscopy including infrared (FTIR and O-PTIR) and Raman spectroscopy (classical Raman, CARS, SRS, and SERS). |
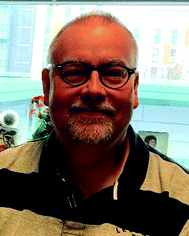 Royston Goodacre | Roy Goodacre is Professor of Biological Chemistry within ISMIB and director of the Centre for Metabolomics Research. He has extensive experience in mass spectrometry-based metabolomics and Raman and infrared spectroscopy, as well as advanced multivariate data analysis. Amongst other things he develops these physicochemical methods for bacterial typing. Full Bio: https://en.wikipedia.org/wiki/Roy_Goodacre. |
Introduction
Unequivocal identification of bacteria is essential for several clinical and biological applications, such as the treatment of disease in animals and plants, where accurate diagnosis is required for an efficient and effective strategy to eradicate infectious microorganisms.1 Accurate diagnosis is also important in limiting the possibility of toxicity to host cells as well as reducing the risk of developing antimicrobial resistance (AMR). In addition, detection and identification of bacteria play a key role in quality control in the food industry,2 while adequate control of bacteria and fungi is important with food biotechnology, for example for the fermentation of foodstuffs and production of microbial metabolites used as food ingredients such as acetic acid and citric acid.3
A desirable technique for bacterial characterisation and identification should fulfil the following criteria: provision and identification within universal bacterial detection, the ability to detect and identify emerging and uncharacterised bacteria unequivocally, high throughput analysis, low operation cost and minimal sample preparation time.4
Traditional bacterial typing
The first step for bacterial identification is the isolation of the bacteria from the complex sample that they reside in. For human samples this may be something relatively non-complex like urine or blood, to faeces where many commensal bacteria reside as part of the natural microflora; for environmental samples this could be for example a soil specimen. In order to achieve isolation of bacteria, microbiologists use selective and/or differential media. As the names suggested these media first enrich for a particular bacterial species. This requires the clinician/microbiologist to have prior information as to what the infective organism may be. In addition, some media are also differential in nature so that the microorganism that a microbiologist is looking for becomes coloured in some particular way. This is usually due to a specific enzyme reaction turning a substrate into a chromogenic product that can be easily seen by eye.5 Following isolation of the bacteria a range of tests are used for identification purposes. It should be stressed that isolation of bacteria is a relatively slow process which is dependent on the growth rate of the microbes: taking days for fast-growing bacteria like Escherichia coli, to several weeks for slow growing bacteria like Mycobacterium tuberculosis.
A great deal of research has demonstrated the application of a wide range of laboratory techniques to classify and identify bacteria;6 these include physiological and biochemical tests, such as cell morphology assessment and analytical profile index (API),7 immuno-assays such as the enzyme-linked immunosorbent assay (ELISA),8 as well as genetic analysis (DNA sequencing) using polymerase chain reaction (PCR)4 and pulsed-field gel electrophoresis (PFGE).9 However, the majority of these standard microbiological approaches tend to be time-consuming, cannot differentiate between dead and live cells and are labour and cost-intensive (Table 1). Therefore, this highlights the need for new alternative and/or complementary approaches that offer lower turnaround time, high reliability and cost-effective bacterial analyses.10
Table 1 Advantages and disadvantages of traditional methods used to characterise bacteria
Methods |
Advantages |
Disadvantages |
PCR |
• Sensitive |
• Target DNA sequence must be known |
• Precise |
• Small amounts of contaminants within samples interfere with experiments |
• Accurate |
• Cannot differentiate between live versus dead bacteria |
|
• Time-consuming |
|
• Requires expertise |
ELISA |
• Specificity |
• Need for highly specific antibodies and prior knowledge |
• Sensitivity |
• Labour-intensive |
• Reliability |
|
PFGE |
• DNA restriction patterns generated by PFGE are stable and reproducible |
• Time-consuming |
• PFGE has proven extremely powerful in the analysis of large DNA molecules from a variety of sources |
• Bands are not independent |
|
• High cost |
Physicochemical methods for bacterial typing
Modern analytical techniques, including mass spectrometry11 and spectroscopic techniques,12 have demonstrated great potential in classification, identification and characterisation of bacteria. Analytical techniques such as matrix-assisted laser desorption/ionisation mass spectrometry (MALDI-MS),13 electrospray ionisation mass spectrometry (ESI-MS),14 Raman spectroscopy15 and Fourier-transform infrared (FT-IR) spectroscopy16 have undoubtedly gained increasing importance in many microbiology and clinical laboratories due to their efficiency in laboratory diagnosis of microbial infections. A simple literature search covering the last 18 years using suitable keywords (e.g. [‘bacterial typing’ AND {‘mass spectrometry’ OR ‘vibrational spectroscopy’}]) in different bibliometric repositories shows the rapid and steady increase in the number of publications in bacterial typing, as shown in Fig. 1. A Web of Science search showed a six-fold increase in publications in this period, suggesting that more biological and clinical laboratories are opting for the use of these analytical methods in bacterial classification and identification, rather than routine old-fashioned traditional methods.
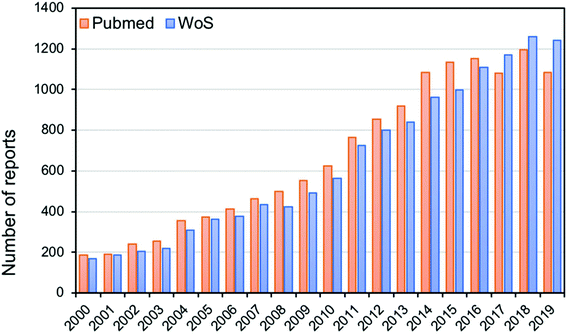 |
| Fig. 1 Bar chart showing the number of publications from 2000 to 2019 in PubMed and Web of Science (WoS) bibliometric repositories using the search terms [“bacterial typing” AND (“mass spectrometry” OR “vibrational spectroscopy”)] for modern analytical techniques used in bacterial typing, classification or identification. | |
In this review, we discuss the use of mass spectrometric and vibrational spectroscopic methods as biomolecular analytical tools, with a specific focus on their application in identifying and classifying bacterial samples – a technique referred to as ‘whole organism fingerprinting’.17,18Fig. 2 shows a schematic summary of the various methods used for bacterial characterization, including traditional methods, mass spectrometry and vibrational spectroscopy. It is noteworthy that MS, Raman spectroscopy and infrared spectroscopy probe the whole cell, which is in contrast to the traditional methods that target specific molecular species. Moreover, as these methods generate whole organism fingerprints, they measure the phenotype of the bacterial cell, which reflects the interaction of the microorganism′s genotype within the environment it inhabits.
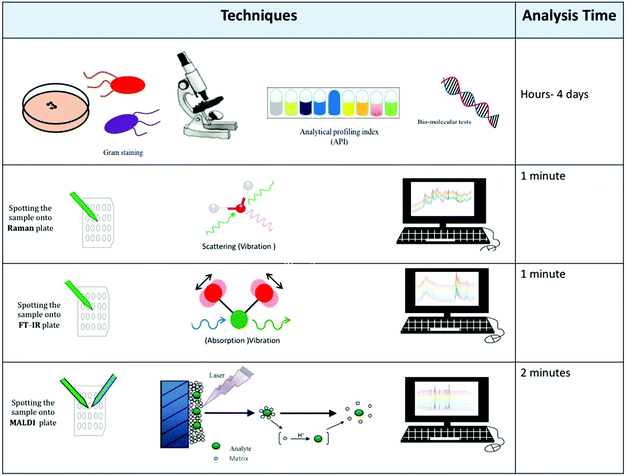 |
| Fig. 2 Illustration of various methods used for bacterial characterisation, including traditional methods, vibrational spectroscopy, and MALDI-mass spectrometry. | |
Vibrational spectroscopic techniques
Vibrational spectroscopy has attracted considerable interest over the past decades as a high-throughput screening technique for rapid analysis of various microbial and biological samples.19–21 FT-IR spectroscopy and Raman spectroscopy are the most popular vibrational spectroscopic platforms used for identification and classification of bacteria based on whole organism biomolecular fingerprints.22–24 Therefore, these spectroscopic techniques provide compelling evidence and useful data for investigating the intrinsic biochemical composition and variations in the bacterial phenotype.25 Interestingly, the spectral data and information obtained from these vibrational techniques contain a wealth of useful biochemical information from the whole cell and can be employed to identify, discriminate and characterise different bacterial species, including clinically and industrially relevant strains.26–28 In addition, these spectroscopic methods can be used to quantify the levels of biological products in microbial fermentations,29 or small molecule biomarkers (e.g.) dipicolinic acid for quantification of bacterial spores.30,31
Fourier-transform infrared spectroscopy
An introduction to FT-IR spectroscopy for the microbiologist.
FT-IR spectroscopy is a versatile analytical tool which is widely utilised for food quality control and pharmaceutical and medical research, in addition to bacterial classification and identification.32–35 FT-IR spectroscopy allows for rapid and high-throughput analysis without being destructive to bacterial samples.12,36 A wide range of research studies have indicated the application of FT-IR spectroscopy in combination with chemometrics to discriminate between different types of bacteria obtained from various sources, such as dietary and clinical products.37,38
In principle, FT-IR spectroscopy is based on the fundamental premise that when infrared light interacts with an investigated sample, there may be an increase in the amplitude of the vibrations of molecules such as stretching and bending vibrations as a result of absorption of this infrared radiation.28,39 The resultant vibrational modes of various molecular bonds are detected and measured simultaneously and can be directly matched to the biochemical species that give rise to them; hence, a precise fingerprint of infrared-active biomolecules is presented by peak intensities and wavenumbers (units of cm−1 and being defined as 1/λ of the absorbing IR, which for mid-IR is 2.5 to 25 µm) in the infrared spectrum. The peak intensities provide quantitative information about the amounts of detected biochemical molecules whilst peak positions (wavenumbers) provide qualitative measurements related to the identity of investigated bacteria. It is also worth mentioning that for a molecule to absorb IR radiation, its molecular bonds should undergo a change in the permanent dipole moment as they vibrate at higher frequency. This implies that many polar biochemical bonds (e.g., C
O, C–N, N–H, etc.) present in proteins and peptides, carbohydrates, and fatty acids/lipids are frequently detected by FT-IR spectroscopy. In bacterial classification and identification, most analyses of bacterial samples have been reported to fall within the mid-IR region (4000 to 600 cm−1) mainly because absorption patterns of functional groups in biological molecules are observed in this particular region as sharp fundamental vibrations, rather than broad overtones or harmonics which are found in the near-IR. In relation to applications and to facilitate unambiguous spectral band assignments of biologically relevant compounds, the mid-IR region is further divided into sub-regions that represent:36,40,41
(1) Fatty acids (3050–2800 cm−1): CH2 and CH3 stretching vibrations,
(2) Proteins and peptides (1750–1500 cm−1): C
O, C–N and N–H, vibrations and
(3) Polysaccharides (1200–900 cm−1): C–O and C–O–C vibrational modes.
A growing resurgence of interest in the application of IR spectroscopy emerged in 1991 when Naumann and colleagues utilised this technique for analysing microbiological samples.42 Following this breakthrough, the past four decades have witnessed an exponential increase in the published literature which is clear evidence of the crucial role that the FT-IR technique plays in microbial analysis. Interestingly, FT-IR spectroscopy achieves successful discrimination between various taxonomic levels, growth conditions,43 detection of contaminants and prediction of antibiotic resistance as demonstrated by several researchers performing bacterial typing.44–46
FT-IR spectroscopy for bacterial typing.
Application of the combination of FT-IR spectroscopy and chemometrics47,48 has been shown to enable accurate classification and identification of different clinical pathogens such as bacteria49,50 and fungi51,52 at different taxonomic levels. Lee and colleagues analysed four different strains of Pseudomonas spp., three different strains of Escherichia spp. and two different type strains of Bacillus spp. The results demonstrated clear discrimination between bacterial strains and the main differences were found to be in the frequency regions of amide I, amide II and PO2− ionised asymmetric and symmetric stretching, and this was confirmed by partial least squares discriminant analysis (PLS-DA) and hierarchical clustering analysis (HCA) of the FT-IR data.53 In another study, Rebuffo and colleagues applied an advanced multivariate analysis that involved the use of artificial neural networks (ANN) to identify Listeria at species levels. Analysis of FT-IR spectral data showed that 277 isolates from five Listeria species could be differentiated with 96% accuracy within 25 h. These findings suggest that this technique is potentially suitable for routine analysis of foodborne pathogens in the laboratory.54 Another investigation carried out by Janbu et al.55 involved rapid analysis of five Listeria species using FT-IR micro- and macro-spectroscopy combined with chemometric techniques based on canonical discriminant analysis (CDA) and PLS-DA. Interestingly, the results obtained demonstrated a discrimination accuracy of 93% and 100% of all the screened species for FT-IR micro- and macro-spectroscopic techniques respectively. It is very clear in this study that despite using smaller biomass (from microcolonies with dimensions 250–300 μm), FT-IR micro-spectroscopy was capable of differentiating Listeria species accurately. This is very important in modern clinical and environmental microbiology where there is a shift towards microbial colony and single cell (with the emergence of nano-IR) level analyses for unequivocal identification, differentiation and characterisation of clinical pathogens even below the infective dose. The most recent study carried out by Wang et al. illustrated the ability of FT-IR to discriminate between 16 types of foodborne pathogenic bacterial strains, and was supported by multivariate analysis such as principal component analysis (PCA) and HCA of FT-IR data. In this study the authors found that a specific spectral region from 1300 to 1000 cm−1 which corresponds to phosphate and polysaccharide vibrations was successfully employed to discriminate bacterial strains.56
In other studies, Shapaval et al.57 developed a micro-cultivation protocol for FT-IR spectroscopy to identify various species of molds based on the spectral libraries combined with ANN models, resulting in an identification accuracy of 95% at the genus and species levels. Furthermore, Shapaval and coworkers58 established a library-independent method based on FT-IR spectroscopy and samples were accurately identified (from 80% to 100%) at the genus or species levels. In an attempt to demonstrate the applicability of FT-IR spectroscopy as a diagnostic tool in clinical setting, Maquelin et al.59 employed FT-IR and multivariate analysis to identify bacteria and yeast pathogens such as Staphylococcus aureus, Escherichia coli, Enterococcus spp., Pseudomonas aeruginosa, Streptococcus spp., and Candida spp., which are frequently detected in the bloodstream of hospitalised patients. According to LDA and ANN analyses of FT-IR spectral data, a clinically acceptable identification accuracy of >98% was clearly demonstrated and compared favorably with standard phenotypic identification tools (API and Vitek systems) routinely applied in hospital laboratory setting. In the same study,59 FT-IR analysis exhibited far superior turnaround time (6–8 h) than standard microbiological tools that took ∼48 h to obtain identification results.
Gram-positive bacteria, such as those belonging to the Bacillus genera60 and Listeria species,61 show good discrimination using FT-IR techniques, with a number of investigations focusing particularly on discriminating between different species. Listeria species are implicated as causative pathogens of Listeriosis, a foodborne microbial infection which has recently gained notoriety in South Africa, where a significant number of victims died following ingestion of food predominantly contaminated with Listeria monocytogenes.62,63 There is no doubt that rapid identification and classification of Listeria species is very desirable to guide effective therapeutic strategies, and thus to reduce mortality rates. Similarly, the discrimination of Bacillus genus was first attempted by Lin et al.64 and Beattie et al.65 Lin and colleagues analysed Bacillus cereus isolates using IR, which involved the identification and differentiation of ten B. cereus group isolates, including B. cereus, B. mycoides, and B. thuringiensis strains, five Bacillus spp. isolates and five non-Bacillus spp. Furthermore, two different types of media were used in this study to analyse these isolates. The results clearly indicated that species-specific peaks appeared between 1738 and 1740 cm−1 in the IR data for the B. cereus isolates. In addition, other distinctive peaks of various band shapes and sizes were observed between 1800 and 1500 cm−1 for the analysed bacterial isolates. The results suggest that FT-IR spectroscopy can indeed be useful for quick identification of species within the B. cereus group. To illustrate the capability of the FT-IR tool for microbial analysis further, Beattie and colleagues65 expanded the study conducted by Lin and coworkers64 where they probed a variety of Bacillus species this time including B. cereus, B. circulans, B. firmus, B. licheniformis, B. megaterium and B. pumilus. In this study, the FT-IR method was supported by canonical variate analysis (CVA) which proved to be effective in discriminating between different types of Bacillus bacteria with a success rate of 95%. Other authors have also illustrated the ability of FT-IR spectroscopy coupled with robust chemometrics to discriminate between different infectious Listeria spp.66 lactic acid bacteria,67Salmonella68 and Pectobacterium and Dickeya spp.69 These studies clearly demonstrate that FT-IR spectroscopy is capable of classifying and differentiating between medically and industrially important bacteria and other microorganisms at various taxonomic levels, which are important prerequisites for appropriate patient management and protection of public health.
Although FT-IR spectroscopy does not provide detailed information regarding specific molecules due to the molecular complexity of biological samples, and the fact that the information provided in FT-IR spectra is related to functional groups, compared to some other techniques such as MALDI-MS, it is recognized as an important tool that can be employed for rapid screening of complex mixtures (e.g., bacterial samples) composed of small biomolecules and biopolymers such as proteins, carbohydrates, lipids, nucleic acids and amino acids,70,71 with only a minimal amount of sample and sample preparation required.
One of the drawbacks of FT-IR spectroscopy is that it registers strong broad bands for water molecules in the mid-IR region which are likely to mask some vital diagnostic peaks which may potentially lead to misinterpretation of spectral data. This problem is usually overcome by drying the samples at appropriate temperatures, using heavy water (D2O), attempting to subtract H2O signals or using attenuated total reflectance (ATR) as a different IR sampling method.72 Another alternative to overcome these limitations is to employ Raman spectroscopy.
Raman spectroscopy
Introductory Raman spectroscopy for the microbiologist.
Over the last decade, Raman spectroscopy has been used successfully as an alternative and complementary physicochemical analytical technique to FT-IR spectroscopy. Different microbial samples such as bacteria, yeast and fungi can be identified and differentiated at the species and subspecies levels. This is mainly because Raman spectroscopy has clearly demonstrated high accuracy, speed and spatial resolution for microbial analysis in the past decades. Another attribute that sets Raman spectroscopy apart from other microbial typing tools is that the Raman signals of water are very weak, making Raman spectroscopy more appropriate for detecting microbes directly within aqueous habitats in which microbes naturally reside.73,74
Like FT-IR spectroscopy, Raman spectroscopy is a non-destructive and portable technique, potentially enabling its application within or outside centralised clinical settings when analysing bacterial samples at the point of care – through so called ‘point-and-shoot’ analysis.75 Although Raman spectroscopy was traditionally used for analytical chemistry applications, there has recently been an increase in biological and pharmaceutical studies that utilise this technique.76–78 The fundamental principle behind Raman spectroscopy lies in its ability to use monochromatic light delivered by powerful lasers, mainly in the near-IR (NIR) to visible, or within UV regions, which exploits the Raman effect (inelastic scattering). The Raman scattering effect occurs in a very small fraction of scattered photons (relative to the Rayleigh process which is the dominant effect) after excitation of molecules.71 The Raman effect is caused when there is a difference between the energy of the scattered photon and the incident photon following interaction between the electric field of incident radiation and sample molecules being analysed. This interaction causes distortion of electron clouds in symmetrical Raman-active covalent chemical bond vibrations which results in increased induced dipole moment (polarizability). This scattering event causes energy exchange due to collisions between photons and molecules resulting in the molecules either gaining or losing a minimal amount of energy. An increase in molecular energy of sample molecules causes a decrease in the scattered photons resulting in Stokes scattering whereas the decrease in molecular energy due to the transfer of quantum energy to the scattered radiation leads to anti-Stokes scattering. In contrast, Rayleigh scattering (also known as elastic scattering) does not change the quantum state of the sample molecules under investigation significantly; that is to say it does not provide useful qualitative or quantitative information about the sample molecules. Despite being very useful, Raman scattering is an inherently weak effect since typically only about 1 in 106–108 photons scatter in an inelastic manner leading to lower sensitivity and longer spectral collection times.79
To overcome this weakness, a number of improved sample preparation methods and techniques are used in conjunction with Raman spectroscopy, including surface enhanced Raman scattering (SERS)80–83 resonance Raman spectroscopy (RRS),84,85 coherent anti-Stokes Raman spectroscopy (CARS)86,87 and stimulated Raman spectroscopy (SRS)88 and are shown to enhance Raman signals by several orders of magnitude which is often needed for bacterial classification and identification.
The continuous development of instrumentation and computing has increased the popularity of Raman spectroscopy, thereby increasing the scope of application in bacterial classification and identification. Table 2 shows the main Raman bands that are seen in bacterial samples and their corresponding assignments.71,89,90
Table 2 Main peaks that are observed in Raman spectra from bacteria, along with their corresponding assignments
Peak wavenumber (cm−1) |
Band assignment |
∼3064 |
C–H stretching in proteins, lipids, etc. |
2800–3100 |
CH3 and CH2 stretching from lipids, carbohydrates, proteins, etc. |
∼1655 |
C O stretching from amide I |
1575–1590 |
Ring vibrations in guanine and adenine |
∼1550 |
N–H bending and C–N stretching in amide II (weaker than amide I and III) |
1453 |
CH2 deformations in proteins, lipids, etc. |
1339 |
Adenine, guanine, tyrosine ring vibrations |
1230–1295 |
C–N stretch and N–H bend in amide III |
∼1170 |
Tyrosine, phenylalanine vibrations |
∼1002 |
Ring vibrations in phenylalanine |
∼1087 |
C–O stretching from amides, proteins or P O stretching in phosphodiesters, flavin adenine dinucleotide, etc. |
∼781 |
Cytosine, uracil (ring stretching) |
Raman spectroscopy for bacterial typing.
Raman spectroscopy provides a high degree of flexibility for a number of applications in bacterial identification and classification, enabling data to be recorded and analysed for bacterial samples from a variety of biological sources,89 including directly from colonies.25 Advances in Raman spectroscopy enables the discrimination of intact bacteria without the need for complex sample preparation, providing reproducible and distinct biochemical fingerprints for each bacterial strain,91 and allowing reliable identification to be carried out.
Raman spectroscopy has been applied for the detection and characterisation of various microbes as well as assessment of the response of microbes to external perturbations. For example, Athamneh et al.92 demonstrated the ability of Raman spectroscopy to profile the phenotypic response and susceptibility testing of E. coli to antibiotics using 532 nm excitation laser wavelength. In this study, E. coli cultures were subjected to 15 different antibiotics (including amongst others ciprofloxacin, ampicillin, and kanamycin) with known mechanisms of action for 30 min. The results showed that Raman spectra contained promising biochemical information to differentiate between profiles induced by individual antibiotics belonging to the same class. Other researchers used UV resonance Raman spectroscopy for the characterisation of bacteria,93 and went on to demonstrate that when bacteria were challenged with different concentrations of the antibiotic amikacin, which inhibits protein synthesis, quantitative changes could be seen in the protein and nucleic acid vibrations when excitation was in the deep UV at 244 nm.94 Another study was carried out by Zhou et al.95 in which the authors illustrated the utility of novel nanostructures, AgNPs coating bacteria structure, for quick counting and differentiation of live and dead bacteria via SERS. Furthermore, Kusić et al.96 used Raman micro-spectroscopy (that is to say, Raman technique coupled with a microscope) in combination with support vector machines (SVMs) to identify water pathogens at the single cell level. The authors further illustrated that Raman spectroscopy can be employed as a fast and reliable technique to discriminate between Legionella species and to identify unknown samples.
Assaf at el.97 distinguished between two closely related species, Salmonella enterica and E. coli, inoculated and examined with six of the most frequently used buffered peptone water brands using Raman spectroscopy. The results illustrated that bacterial cells behaved inversely depending on the water brand used in terms of biomass production and the spectral fingerprint. The accuracy of the analysed bacteria was achieved between 85% and 100%, and this was similar to the findings of another study conducted by Wang et al.98 where the detection of pathogenic S. aureus was successfully reported using Raman spectroscopy. In another study, the successful classification of six bacterial species (B. cereus, Enterobacter aerogenes, E. coli, Streptococcus pyrogenes, Enterococcus faecalis and Streptococcus salivarius) was carried out by Xie et al. using Raman spectroscopy.99 In addition, Harz et al.100 reported the successful discrimination of several Staphylococcus species both at the species and strain levels. A further study carried out by Schmilovitch et al.101 enabled clear distinction between Gram-positive and Gram-negative bacteria using Raman spectroscopy with a diode laser of 785 nm excitation. These examples provide evidence that Raman spectroscopy can be used for the analysis of bacterial samples using various sample preparation methods, instrumentation parameters and chemometric tools.
Although Raman spectroscopy exhibits poor quantum efficiency in comparison with IR spectroscopic techniques, SERS is often employed to enhance Raman signals, resulting in increased sensitivity down to single molecule detection.102 SERS involves the measurement of the interactions between plasmonic metallic nanoparticles (NPs) and sample molecules; these molecules can be in direct contact to or reside in close proximity to the NP surface.103,104 Although the SERS enhancement mechanisms are still inconclusive, and that the debate is still ongoing within the SERS community,105 the enhancement of the Raman scattering observed via the SERS method is largely due to the incident excitation frequency being resonant with the surface plasmon resonance in metallic nanostructures, the so-called localised surface plasmon resonance (LSPR). Silver (Ag) and gold (Au) based colloidal NPs are most widely used as enhancement substrates due to their low cost, ease of synthesis and high stability under normal laboratory condition. For more detailed description of SERS principles, readers are directed to the following excellent reviews.104,106,107 The first demonstration of SERS for the identification of bacteria was reported by Jarvis & Goodacre, who showed that the signal arose from the surface features of the cell due to the interaction of the LSPR with the bacterial cell surface.108 Some bacteria can naturally reduce Ag(I) and Au(III) to Ag and Au NPs, respectively and thus the signal can be made to arise from within bacterial cells.109 This so-called in situ production of Ag or Au NPs, where metallic particles are formed in the presence of bacterial cells and attached to either the cell surface or cytoplasmic biomolecules, was recently employed to differentiate among five near isogenic cell wall mutants of a single strain of Campylobacter jejuni foodborne pathogen.80 Interestingly, the SERS findings were consistent with the results obtained from Raman spectroscopy and matrix-assisted laser desorption/ionisation mass spectrometry data applied as confirmatory techniques in this study, clearly demonstrating excellent reproducibility and robustness of SERS. It is thus clear that due to the plasmonic enhancement effect it is not surprising that SERS is a highly sensitive, fast and reliable analytical tool that can detect low concentration of bacterial cells, differentiate closely related pathogens and more importantly can probe single prokaryotic cells and highly infectious biowarfare agents.104 This makes SERS very attractive and useful in clinical diagnostics, food and water quality and safety control measurements where rapid, sensitive and unequivocal detection of infectious pathogens is absolutely important.110
All the above studies analysed the whole organism. By contrast, a recent study carried out by Kearns et al.111 developed a new bionanosenser to detect specific bacterial pathogens (E. coli, Salmonella typhimurium, and methicillin-resistant S. aureus) simultaneously by SERS that was targeted to specific DNA sequences. This enabled the authors to multiplex the pathogen detection, and was shown to be highly quantitative when multivariate analyses were made. For information on quantification of analytes and bacteria by SERS the reader is direct to these recent reviews.83,104,112
Finally, perhaps the most exciting aspect of Raman spectroscopy is that it is possible to measure single bacteria. This is because the excitation source used for Raman spectroscopy is a UV to near IR laser that is highly focused and thus possesses similar dimensions to bacterial cells (ca. 1 µm). Schuster and colleagues were perhaps the first to demonstrate that Raman spectroscopy had sufficient spatial resolution and generated chemical information about single cells of the industrially-relevant Clostridium beijerinckii.113 Single cell analysis has been demonstrated by several groups, including for measuring metabolic activity of cells,28,103 and opens up the tantalising realisation that bacteria could be analysed without the need for a culture step.114 Thus Raman spectroscopy would appear to possess this major advantage over infrared spectroscopy. However, with the recent developments of atomic force microscopy-infrared (AFM-IR)115 and optical photothermal infrared (O-PTIR) spectroscopy,116 infrared microspectroscopy is starting to rival Raman spectroscopy for analysis of single bacterial cells. By way of example Fig. 3 shows an infrared spectrum collected using O-PTIR from a single E. coli cell with typical dimensions of 1 × 2 µm weighing just 1 pg.
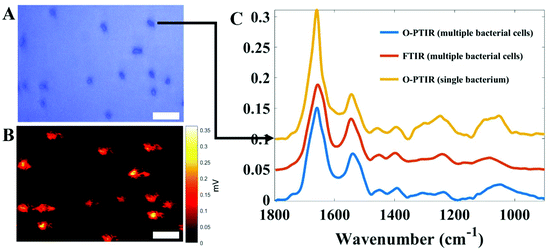 |
| Fig. 3 Infrared spectral signatures acquired from E. coli cells via O-PTIR using a mIRage infrared microscope from Photothermal Spectroscopy Corp and FT-IR spectroscopy operating in transmission mode on a Bruker Equinox 55 infrared spectrometer. (A) Optical image displaying several E. coli cells. (B) Single frequency image obtained through O-PTIR imaging for the amide I band (1655 cm−1). (C) Infrared spectra acquired from single E. coli cells via O-PTIR (yellow line) as well as from multiple bacterial cells (bulk) of the same strain using traditional FT-IR (red line) and O-PTIR (blue line) technologies. In A and B, the scale bar is 3 µm. | |
Mass spectrometry
Mass spectrometry (MS) is regularly used in biological studies for qualitative and quantitative purposes. This analytical technique exploits the use of a core physical property, the mass-to-charge ratio (m/z) of molecules as well as their fragmentation patterns that can be used for analyte identification. Prior to the use of this analytical technique, conventional methods were employed for detection of biomolecules in bacterial samples based on direct chemical or electron ionisation techniques,117 or through the use of pyrolysis as a sample introduction technique.118,119 The main disadvantages of these conventional techniques were that the energy associated with their operation caused considerable decomposition of samples, which led to very complex and often uninterpretable mass spectra. Thus, conventional methods were gradually replaced by more advanced hyphenated mass spectrometric methods. Gas chromatography in combination with mass spectrometry (GC-MS) is one such example of common hyphenated techniques that can be used to analyse volatile and non-volatile (after sample derivatisation) small molecules both qualitatively and quantitatively;120 however, the use of chromatography necessitates relatively long analysis times (30–60 min is typical) and complex deconvolution steps are involved, thus making GC-MS both time-consuming and laborious, and the same can be said for liquid chromatography-mass spectrometry (LC-MS).121 This highlights the need for other more rapid and facile methods of analysis. The introduction of soft ionisation techniques such as matrix-assisted laser desorption/ionisation (MALDI)122 and electrospray ionisation (ESI)123 in the late 1980s solved the problems associated with harsh ionisation techniques. Many MS methods have since been developed for use in a wide range of applications, such as biological, chemical and life sciences. MALDI and ESI are highly accurate methods that have been employed for the identification and characterisation of proteins, lipids, sugars and nucleic acids in various biological samples, and as such are ideal for the analysis of bacteria.124 Whilst some researchers have developed ESI-MS via direct infusion for bacterial classification,125,126 the vast majority of mass spectrometry-based bacterial identification has been undertaken using MALDI-MS.
An introduction to matrix-assisted laser desorption/ionisation mass spectrometry
Although FT-IR spectroscopy and Raman spectroscopy are regarded as powerful physicochemical tools, the introduction of MALDI-MS proved to be a preeminent advancement in the identification of bacteria within clinical microbiology laboratories due to the speed, accuracy, simplicity and cost-effectiveness of this technique.127
MALDI-MS works by producing molecular ions when a laser beam is applied to analytes, which are mixed prior to analysis with a matrix, and air dried. Analyte and matrix mixtures are analysed on a MALDI target metal plate with the most common ones being stainless steel, aluminium and gold.128 The matrix is a highly concentrated solution of low molecular weight, generally acidic, organic molecules that contain a conjugated double bond system capable of absorbing the UV laser energy used in MALDI.129 Examples of matrices that absorb in the UV (337 nm excitation is typical of MALDI) include sinapinic acid (SA),130 α-cyano-4-hydroxycinnamic acid (HCCA)131,132 and 2,5-dihydroxybenzoic acid (DHB).133,134 There is no universal matrix or a standard method for the deposition of the analyte/matrix mixture on the MALDI plate that can be used for routine analysis, nor a set of guidelines in the literature to assist in choosing the optimal matrix and protocol for the analysis of microorganisms and other sample analytes. Hence, the matrix and preparation method most suitable for a particular application are typically chosen on the basis of trial and error, although some researchers have used the design of experiments to optimise the matrix and preparation method,135 that can be applied for bacterial characterisation.136 The suitability of the matrix used in any research study is highly dependent on certain factors, including the solubility of the matrix in different solvents and its ability to absorb laser energy at the wavelength used in the MALDI-MS device. In MALDI-MS, sample and matrix molecules co-crystallize on the plate and subsequently different types of laser beams, with the most popular being in the UV with a nitrogen laser (337 nm) or sometimes in the infrared with an erbium doped yttrium aluminium garnet (Er:YAG) laser (2940 nm), can be used to excite the analyte/matrix crystals. Irradiation of the analyte/matrix system by a laser pulse leads to desorption of the embedded analyte molecules.131 The mechanism of desorption is not well-understood and the desorbed molecules are ionised forming predominantly singly charged ions, which are separated and counted by the time-of-flight (TOF) mass analyser.4
MALDI, being a very soft ionisation technique, also allows the direct measurements of proteins in whole bacterial cells, without any fragmentation.137,138 In these methods, the majority of proteins that can be identified are ribosomal proteins, which are detected quite easily due to their smaller size and high abundance. Moreover, the fact that amino acid sequences of ribosomal proteins are conserved to a great extent at the genus and sometimes at the species level, the slight variations in these sequences can be used to distinguish bacteria at the strain level.139 One of the benefits of the ribosomes being measured is that they are always produced under any growth condition and so are not susceptible to variable phenotypic effects during bacterial cultivation; this may be why the method is very stable and used routinely in many clinical microbiology laboratories. Identification of bacteria at the genus, species or strain level is usually performed using the obtained protein mass patterns together with a library based approach or a bioinformatics enabled analysis.140 In both of these methods, the analyst is comparing and matching distinguished and discriminated peak (biomarker) in the MALDI-MS profiles of the bacteria under investigation with publicly available databases.141,142
The use of MALDI-MS has been extended to a variety of research areas, including the direct analysis of organisms and biological tissue samples and the characterisation of peptide and protein components.143–145 In addition to its qualitative applications, MALDI-MS is also a popular quantitative tool due to its ability to analyse molecules over a wide range of mass, its high sensitivity, easy and fast sample preparation, and short analysis time. Quantification can be relative to a reference sample or based on the use of internal isotopically labelled standards. Challenging samples have been successfully characterised and quantified using MALDI-MS, including intact peptides and proteins measured directly in biological tissues and fluids.4
Despite the numerous reported quantitative MALDI-MS applications, there still remain some limitations that restrict the widespread use of MALDI-MS and these are thought to be mainly associated with analyte/matrix mixture heterogeneity, which results in variable analyte signal intensities. Other limitations include the difficulty in coupling MALDI-MS to separation techniques, such as liquid chromatography, and limitations of the dynamic range that arise due to saturation of the detector. Together, these factors most likely compromise the use and reliability of making quantitative determinations using MALDI-MS, with the majority of studies focusing on analytes of low molecular mass, for example food products,146 while other investigations utilised MALDI-MS as a relatively quantitative tool in biological applications, such as the analysis of proteins,147 neuropeptides,148 antibiotics,149 and metabolites in biological tissues or fluids.150
Bacterial typing with MALDI-MS
Drucker and Fenselau were the first to report the typing of microbes using MALDI-MS in 1993, where the identification of microbial samples was based on their cellular lipid compositions. Since then, the identification of microbes has developed so that they can be identified based on their protein profiles, which can be directly obtained from whole cell organisms,151 with the first application of rapid identification of bacteria from protein profiles reported by Claydon and co-workers in 1996,152 which was closely followed by very similar findings by another research group later the same year.153 Since 2016, Korea has introduced the use of MALDI-MS as a new clinical analytical technique in healthcare for reliable routine analysis. This has the potential to identify bacterial infections correctly, leading to timely prescription of appropriate antibiotics to treat infections effectively and limit resistance. Lange et al. analysed different types of bacteria (108 Klebsiella spp. isolates) using MALDI-MS in the presence of meropenem; in this work a sensitivity and specificity of 97% and 94% respectively were achieved.154 A recent study was carried out by Axelsson et al. where the authors showed the ability of MALDI to distinguish between susceptible and resistant strains of E. coli and K. pneumoniae with 90 min of incubation at 37 °C for three different types of antibiotics (cefotaxime, meropenem and ciprofloxacin). In this study, overall 841 positive blood culture analyses of 14 reference strains were achieved, and the results showed high sensitivity (99%), specificity (99%) and accuracy (97%).155 DeMarco et al. demonstrated clear detection and identification of bacteria directly from urine samples without the need for bacterial culture steps, using the diafiltration MALDI-MS technique.156 Similarly, Nakano et al. carried out a study using MALDI-MS to discriminate between 10 major serotypes of S. pneumoniae.157 Woods et al. went further to differentiate a large number of S. anginosus group using MALDI-MS with a high sensitivity of 93%.158 Moreover, discrimination between 35 Enterococcus faecium strains was carried out in clinical samples using MALDI-MS at the strain level with high accuracy (78%).159 Finally in a recent study Zhu and co-workers showed that bacteria isolated from blood culture could be identified from as little as 500 cells per mL in blood serum and 8000 cells per mL in whole blood.160
As MALDI-MS currently dominates clinical microbiology labs for bacterial typing, and in some areas replacing traditional microbiological methods for bacterial typing, we discuss the methods that people have used to conduct MALDI-MS on bacteria for bacterial characterisation and identification.
MALDI-MS: sample preparation for bacterial analyses
Researchers have assessed several MALDI-MS sample preparation methods for many different microorganisms. Whilst direct cell profiling can be used to identify some microbes, some researchers have developed methods for the generation of whole cell lysates or crude cell extracts. When using direct cell profiling, a single bacterial colony can be gathered using a plastic loop and placed onto a MALDI sample plate, which is then overlaid directly with the matrix solution (see below for a discussion of common matrices used) before analysis. MALDI-MS of intact bacteria has been used to identify Gram-negative bacteria, such as Neisseria spp.161 and Vibrio spp.162 By contrast, as the cell wall of Gram-positive species is tougher ‘preparatory extraction’ of microbes with formic acid (FA) is preferred for bacterial identification. However, Gram-negative bacteria cannot be identified using this extraction method.163,164
Aerobic actinomycetes such as Nocardia and Mycobacterium species require specialized processing procedures prior to the MALDI-TOF analysis due to the composition of their cell walls which contain mycolic acids. Verroken et al.165 developed a modified procedure for the identification of Nocardia spp. by MALDI-MS, in which bacteria are first lysed in boiling water and the proteins are then precipitated using ethanol. The proteins are dried and resuspended in 70% FA and acetonitrile (ACN). This was followed by analysis using MALDI-MS. Several different procedures have been reported regarding sample preparation protocols used for the identification of mycobacteria by MALDI-MS; some of these examples include direct bacterial profiling and treatments using formic acid. However, due to the pathogenicity of some Mycobacterium spp. safety issues have been a concern during investigations. A procedure that combined both bacterial inactivation and process methods was described by El Khéchine et al.166 Colonies from mycobacteria were collected using screw-cap tubes, with water and 0.5% Tween 20, which were then inactivated by heating for 1 h at 95 °C. The inactivated samples were then subjected to centrifugation and then vortexed with glass beads, which led to the disruption of the mycobacterial cell wall. The resultant pellet was resuspended in FA and followed by centrifugation. Finally, the supernatant was spotted onto the MALDI plate and was overlaid with a suitable matrix.166
MALDI-MS: choosing the best matrix
It can be challenging to choose the most suitable matrix for the analysis of specific bacteria. This is because there are currently no agreed guidelines documented (so called Standard Operating Procedures (SOPs)) to assist the process of choosing the most suitable matrix and deposition protocol for the analysis of all possible microorganisms. Thus, the matrix is generally chosen through trial and error. The matrix used is usually determined by looking at a number of different factors, including the solubility of the matrix in different solvents, as well as its ability for enhanced desorption and ionisation by having the correct laser excitation frequency. An appropriate matrix selection is a vital phase in the development of the sample preparation protocol for the MALDI-MS and the preferred matrices for direct analysis of bacteria for proteins include CHCA, SA, DHB, with the addition of FA. On the other hand, with lipid or phospholipid ionisation, 2-mercaptobenzothiazole (MBT) or CMBT is favoured.167 Unfortunately, there is no agreement on what the best matrix for the MALDI-MS bacteria profiling is, because of the various factors that contribute to the ionisation process and thus the MALDI-MS profile quality. These include the variability of sample preparation procedures and the analyte itself, the chemicals used, etc. It has been found that when using a different matrix for the same type of bacteria, a substantial change occurs in the MALDI mass spectrum. For example, Demirev et al.168 noted that CHCA generated very different signals from the SA/4-methoxycinnamic acid (MCA) mixture when used for E. coli cells.
The use of alpha-cyano-4-hydroxycinnamic acid (CHCA) has been reported in nearly half of the studies in the field of MALDI bacteria profiling for analysis of peptides or small proteins. CHCA is generally chosen instead of other matrices, due to its high detection rate, signal intensity in the lower mass range169–171 and its sensitivity.172 It was concluded that a higher frequency of signals resulted in the formation of doubly protonated ions.173 Despite the numerous advantages that CHCA has to offer in MALDI-MS, there are also a number of disadvantages, such as its lower signal resolution in comparison with FA and SA, it has a higher degree of peak broadening and the lack of signals in the high-mass range, compared to FA.174
SA is another well-known protein matrix, which has also been widely used in studies carried out on bacteria profiling using MALDI-MS. Many studies reported better ionisation of high molecular weight proteins,175 in turn benefiting from less frequent peak broadenings, improved spectral quality, sample homogeneity, greater mass accuracy, and shot-to-shot reproducibility, as well as better signal resolution. This matrix has also been found to have the best signal-to-noise ratio and signal intensity among other matrices.176–178 Furthermore, other researchers preferred the use of FA as the matrix for bacteria profiling, because of its ability to ionize compounds with higher molecular weight,179 its good signal resolution and shot-to-shot reproducibility.
As there are many matrices to choose from, some researchers have decided to use combinations of matrices for bacterial analysis. A binary 1
:
1 mixture of 2-(4-hydroxyphenylazo)benzoic acid (HABA)/MBT was found to produce peaks in the high mass range from extracted proteinaceous materials extracted from E. coli.180
Finally, choosing an appropriate solvent is another vital step for sample preparation prior to analysing samples using MALDI-MS. Solvent volatility is frequently modified to attain large biomolecule ionisation; this is because slow crystallisation is thought to generate larger matrix crystals. Alternatively, the homogeneity of the matrix layer is composed of small crystals through fast solvent evaporation, enhancing shot-to-shot reproducibility. Conway et al.181 described that the SA matrix dissolved in 30% ACN (0.1% TFA) and mixed with an Enterobacteriaceae suspension in water led to a wider range and higher signal intensity in comparison with when samples were dissolved in isopropyl alcohol. Moreover, Ruelle et al.182 stated that CHCA dissolved in 0.1% TFA/ACN (60
:
40) resulted in the formation of peaks from E. coli suspension only in the low-mass region, while additional peaks in the high-mass range were detected when dissolved in ACN/isopropanol/0.1% TFA.
As is clear from the above there is still much room for development in the best matrix and solvent combinations to use for MALDI-MS analysis of intact bacteria as well as solvent extracts for peptide, protein or lipid analysis.
MALDI-MS: sample deposition approaches
Several sample deposition methods can be used for MALDI-MS analysis. When the matrix and analyte are homogenized, co-crystallisation occurs, prompting the formation of sweet or hot spots. The methods for sample deposition are generally easy to perform. The most frequently used ones for sample preparation are mixing the bacteria and matrix together, overlay, underlay and sandwich methods. These methods are illustrated within Fig. 4.
 |
| Fig. 4 Generalised scheme showing the role of MALDI-MS, FT-IR and Raman spectroscopy for addressing four different microbiological roles. Also shown are the main bacterial sample preparation methods, along with matrix deposition approaches for MALDI-MS (blue represents the UV absorbing matrix and red the bacteria), as well as the need for robust multivariate analysis. | |
Vaidyanathan et al.178 analysed B. sphaericus, E. coli, and Brucella laterosporus and observed difference in low- and high-mass ranges of MALDI-MS profiles when three separate sample deposition methods were investigated. These included:
(a) Mixed method – a pre-mix of the bacterial suspension with the matrix solution was used,
(b) Modified underlay method – where the matrix was first spotted on a MALDI plate, then a pre-mix of sample/matrix was added as an additional layer,
(c) Overlay method – where the bacterial sample was first spotted on a MALDI plate, allowed to dry, and was then covered with the matrix solution.
The last approach provided the best spectra in terms of the number of detected signals and signal-to-noise ratio. Despite this finding other workers have shown that the mix method was preferred for the analysis of Gram-positive bacteria.13 This clearly highlights that one sample analysis method does not exist for the analysis of all bacteria.
Multivariate analysis
In this review we have introduced and discussed three different physicochemical bioanalytical techniques that are used for bacterial typing. All of these produce a large number of characteristics about the sample under analysis. For FT-IR and Raman these consist of thousands of complex vibrational modes either from absorption of infrared light at a particular wavelength (expressed as wavenumber), or from the shift in the frequency of light from a laser excitation source in Raman spectroscopy. For MALDI these data are representative of the relative amounts of particular analytes (peptides, proteins, lipids, etc.) with particular m/z. Thus, multivariate analysis is needed in order to transform these spectra into useful outputs that aid clinical microbiology.
The central principle of multivariate analysis (MVA) relies on simplifying the data to a smaller number of variables without compromising the information content within the original data input, with the hope that this helps enhance scientific reasoning. For bacterial characterisation and typing there are generally two approaches: unsupervised methods that perform exploratory analysis; and supervised learning methods that aim to effect identification of bacteria from a spectral input. In general, unsupervised methods use only the spectral input variables (X-data), whilst supervised methods aim to take the X-data as input variables and associate these data with a set of target output variables (Y-data) which encode the identity of a set of bacteria of interest.83
Perhaps the most popular unsupervised method is principal component analysis (PCA) which is used to reduce data dimensionality and as an exploratory analysis shows structure in the data by clustering spectra into groups with similar characteristics, as well as being used to discover outliers. PCA is not used to identify bacteria from their spectral profiles and so in general supervised models are used for bacterial classification from MS and spectroscopy data. The most popular ordination models are based on Fisher's linear discriminant analysis (LDA), and include discriminant function analysis (DFA; also termed canonical variate analysis (CVA)) and partial least squares-discriminant analysis (PLS-DA).183,184
There are various nonlinear equivalents to these LDA methods that perform nonlinear mapping from X-data (MS, IR, Raman) to Y-data (bacterial identity). These methods are generally referred to as machine learning techniques, with the most well-known referred to as: support vector machines (SVMs), random forests (RFs) and kernel PLS (kPLS) and for the interested reader a recent tutorial review highlights these alogorithms.185 Recently the interest in artificial intelligence (AI) and artificial neural networks (ANNs) has grown and in turn given rise to in depth learning. A variety of layers are used in convolution neural networks, which are essentially different to the single layer neural networks developed by Rumelhart and colleagues.186,187 Finally, whilst supervised learning methods are very powerful, they are not infallible and so need to be robustly calibrated. It is vital that the number of latent variables is selected carefully, and that supervised models are validated through (e.g.) bootstrapping or n-fold cross-validation, preferably tested with data not used at all in model construction.185 Only then can precise prediction, increased and reliable quality control, and assurance in disease diagnostics be provided.188
Comparative assessment of these analytical techniques used in bacterial typing
This review has highlighted several physicochemical techniques that can be used for bacterial characterisation. Each of these whole organism fingerprinting methods has advantages and disadvantages, and these must be explored prior to an analyst making an informed decision as to which tool to use.
Although different analytical techniques tend to provide a specific type of information, the choice of which to employ has so far been mostly guided by availability, cost and intended applications. Table 3 provides a comparative assessment of the performance of analytical techniques whilst Table 4 highlights the advantages and disadvantages of these techniques. Fig. 4 also emphasises the overall scheme of sample preparation and in particular the reasons for choosing one method over another. This is provided by a series of microbiological questions which are highlighted below:
• Typing to species level: for routine bacterial typing where the genus and species identification is needed then MALDI-MS is the technique of choice.
• Species/sub-species typing: whilst MALDI-MS is an excellent identification method for sub-species analysis, vibrational spectroscopy and in particular FT-IR spectroscopy is generally preferred, although we note that Raman spectroscopy can also be used for this purpose.
• Phenotypic characterisation: bacteria often adapt to their environment and this will be by changing the physiology and biochemistry of the sample. This is best measured using FT-IR spectroscopy, and Raman spectroscopy can also play a role here.
• Single cell analysis: for the analysis of single bacterial cells the preferred method of analysis is currently Raman spectroscopy. However new optical, photothermal, and infrared based spectroscopy methods are starting to emerge as illustrated in Fig. 3.
Table 3 Comparison of the characteristics of various vibrational and mass spectrometric techniques
Analytical technique |
|
|
|
Techniques characteristics |
FT-IR spectroscopy |
Raman spectroscopy |
MALDI-MS |
AFM-IR, atomic force microscopy coupled with infrared; O-PTIR, optical photothermal infrared.
|
Cost of running per sample |
Low |
Low |
Low |
Automation |
Yes |
Yes |
Yes |
Sample preparation |
Minimum |
Minimum |
Minimum/moderate (depending on biomolecule/matrix) |
Amount of sample required |
1–2 μL |
1 μL |
2 μL |
Single cell analysis possible? |
No (but possible with AFM-IR and O-PTIR)a |
Yes |
No |
Analysis time |
1 min |
1 min |
2 min |
Reproducibility |
Good |
Medium |
Medium |
Sensitivity |
High |
High |
High |
Destructive of sample |
No |
No |
Yes |
Size of generated data |
Small |
Small |
Average |
Complexity of data |
Low |
Average |
Average |
Table 4 Advantages and disadvantages of vibrational and mass spectrometric techniques used in characterisation of bacteria
Method |
Advantages |
Disadvantages |
FT-IR |
• Easy sample preparation |
• May need expertise in chemometric analysis of data |
• Simple to use |
• The water band is very strong so the sample needs to be dried |
• Sensitive technique |
• Different conditions (e.g. growth time and culture medium) can cause variations in spectra |
• Provides general information about whole-bacterial cells |
|
• Rapid analysis |
|
• Portability |
|
Raman |
• Provides information on biological structures |
• The Raman effect is weak leading to low sensitivity and long collection times |
• Able to analyse small quantities of samples |
• Interference with fluorescence |
• Single cell analysis |
• Spectral bands affected by environmental conditions (e.g. pH, growth stage, etc.) |
• The water band is very weak |
|
• Rapid |
|
• Whole-cell molecular fingerprinting |
|
• Portability |
|
MALDI-MS |
• Rapid and specific detection of whole bacteria |
• MALDI matrix cluster ions obscure low m/z species (<600 amu) leading to matrix interference with small molecules |
• Ability to analyse high molecular weight compounds (e.g. proteins) in a wide mass range |
• Homogeneity from spot to spot is variable |
• Generally unaffected by growth conditions, as ribosomes are key discriminatory features in spectra |
• Compromised quantification |
• Soft ionisation technique |
|
• Sub-picomole sensitivity |
|
• Wide array of matrices |
|
This information can aid experimental design in bacterial typing and identification applications, with the tantalising prospect of some techniques being able to measure single bacterial cells, which means that the clinician no longer needs to isolate the microorganism prior to testing. Importantly, several of these techniques provide complementary and confirmatory data, offering highly reliable phenotyping results and more comprehensive analysis of bioprocesses. The characteristics of the various analytical techniques that can be used to analyse bacteria as discussed in this review should be considered along with the type of sample and preparation method in order to ensure validity and reliability of the generated information.
Conclusion and outlook
The development in the analysis of bacterial samples using modern analytical techniques has been on the rise over recent decades due to several advantages, such as rapid analysis and accurate, and readily interpretable results, with more future improvements expected in sample preparation and methods of analysis from bulk bacterial samples to single cells. In this review we have aimed to provide insights into the applications of infrared and Raman spectroscopy as well as mass spectrometry for the characterisation and identification of bacterial samples from different sources. Currently within clinical microbiology laboratories MALDI-MS is the method of choice for bacterial identification. The success of this particular mass spectrometry technique is largely down to its robust analysis because it measures a small proportion of the bacterial cell – namely the ribosomes, which are always present irrespective of how the bacteria are cultured.
One of the main challenges for the future is the desire to have even more rapid analysis by removing the need to enrich and isolate the bacteria or fungi prior to analysis. Here the high spatial resolution offered by both Raman spectroscopy and infrared micro-spectroscopy means that there is potential for single cell analysis, as illustrated in Fig. 3. The remaining challenge here will be the necessity to locate the microorganism prior to analysis. For some highly complex samples like soil or faeces this will be very problematic without the successful implementation of mechanical capture mechanisms.
The whole organism fingerprinting methods discussed within this review generate large amounts of high dimensional phenotypic data about the sample analysis. Therefore, it is essential that these techniques are combined with suitable chemometrics and machine learning to provide easily interpretable outputs. In the wake of the novel coronavirus (COVID-19) global pandemic, IR spectroscopy and Raman spectroscopy in parallel with multivariate chemometrics may play a crucial role in rapid and sensitive screening of RNA or glycoproteins for severe acute respiratory coronavirus-2 (SARS-CoV-2),189 the causative agent of COVID-19, that would potentially allow for timely intervention of appropriate patient care, reduction of mortality rate and continuous monitoring of COVID-19.
In summary, we believe that the powerful combination of whole organism fingerprinting methods with robust informatics will provide simple and efficient diagnostic and monitoring tools in many different areas including within the fields of medicine, the environment and food, and by biotechnological processes.
Conflicts of interest
There are no conflicts to declare.
Acknowledgements
This research was funded by the Deanship of Scientific Research at Princess Nourah bint Abdulrahman University through the Fast-track Research Funding Program to NA. HM, CAL and RG are grateful to the University of Liverpool for support.
References
- R. W. Peeling, P. G. Smith and P. M. M. Bossuyt, Nat. Rev. Microbiol., 2006, 4, S2–S6 CrossRef.
- J. Wilkinson, Nat. Toxins, 1999, 7, 221–232 CrossRef CAS.
- M. Sauer, D. Porro, D. Mattanovich and P. Branduardi, Trends Biotechnol., 2008, 26, 100–108 CrossRef CAS.
- S. Sauer and M. Kliem, Nat. Rev. Microbiol., 2010, 8, 74–82 CrossRef CAS.
- J. Perry, Clin. Microbiol. Rev., 2017, 30, 449–479 CrossRef CAS.
- V. Sintchenko, J. R. Iredell and G. L. Gilbert, Nat. Rev. Microbiol., 2007, 5, 464–470 CrossRef CAS.
- B. Holmes, W. Willcox and S. Lapage, J. Clin. Pathol., 1978, 31, 22–30 CrossRef CAS.
- E. Engvall, Med. Biol., 1977, 55, 193–200 CAS.
- T. F. O'Sullivan and G. F. Fitzgerald, FEMS Microbiol. Lett., 1998, 168, 213–219 CrossRef.
- H. AlRabiah, E. Correa, M. Upton and R. Goodacre, Analyst, 2013, 138, 1363 RSC.
-
J. H. Gross, Mass spectrometry: a textbook, Springer Science and Business Media, 2006 Search PubMed.
- M. J. Baker, H. J. Byrne, J. Chalmers, P. Gardner, R. Goodacre, A. Henderson and J. Sulé-Suso, Analyst, 2018, 143, 1735–1757 RSC.
- N. AlMasoud, Y. Xu, N. Nicolaou and R. Goodacre, Anal. Chim. Acta, 2014, 840, 49–57 CrossRef CAS.
- J. W. Allwood, H. AlRabiah, E. Correa, A. Vaughan, Y. Xu, M. Upton and R. Goodacre, Metabolomics, 2015, 11, 438–453 CrossRef CAS.
- S. Pahlow, S. Meisel, D. Cialla-May, K. Weber, P. Rösch and J. Popp, Adv. Drug Delivery Rev., 2015, 89, 105–120 CrossRef CAS.
- C. Quintelas, E. C. Ferreira, J. A. Lopes and C. Sousa, Biotechnol. J., 2018, 13, 1700449 CrossRef.
- R. Goodacre, E. M. Timmins, R. Burton, N. Kaderbhai, A. M. Woodward, D. B. Kell and P. J. Rooney, Microbiology, 1998, 144, 1157–1170 CrossRef CAS.
-
J. T. Magee, Whole-organism fingerprinting, in Handbook of New Bacterial Systematics, ed. M. Goodfellow and A. G. O'Donnell, Academic Press, London, 1996, pp. 383–427 Search PubMed.
- X. N. Lu, H. M. Al-Qadiri, M. S. Lin and B. A. Rasco, Food Bioprocess Technol., 2011, 4, 919–935 CrossRef.
- J. P. Harrison and D. Berry, Front. Microbiol., 2017, 8, 675 CrossRef.
- L. B. Kong, P. F. Zhang, P. Setlow and Y. Q. Li, Anal. Chem., 2010, 82, 3840–3847 CrossRef CAS.
- J. P. Maity, S. Kar, C. M. Lin, C. Y. Chen, Y. F. Chang, J. S. Jean and T. R. Kulp, Spectrochim. Acta, Part A, 2013, 116, 478–484 CrossRef CAS.
- D. I. Ellis, D. P. Cowcher, L. Ashton, S. O'Hagan and R. Goodacre, Analyst, 2013, 138, 3871–3884 RSC.
- G. Sharma and A. Prakash, J. Microbiol., Biotechnol. Food Sci., 2020, 9, 310–314 Search PubMed.
- K. Maquelin, L. P. Choo-Smith, T. van Vreeswijk and H. P. Endtz, Anal. Chem., 2000, 72, 12–19 CrossRef CAS.
- H. Muhamadali, D. Weaver, A. Subaihi, N. AlMasoud, D. K. Trivedi, D. I. Ellis, D. Linton and R. Goodacre, Analyst, 2016, 141, 111–122 RSC.
- K. Maquelin, L. Dijkshoorn, T. J. K. van der Reijden and G. J. Puppels, J. Microbiol. Methods, 2006, 64, 126–131 CrossRef CAS.
- H. Muhamadali, M. Chisanga, A. Subaihi and R. Goodacre, Anal. Chem., 2015, 87, 4578–4586 CrossRef CAS.
- A. C. McGovern, D. Broadhurst, J. Taylor, N. Kaderbhai, M. K. Winson, D. A. Small, J. J. Rowland, D. B. Kell and R. Goodacre, Biotechnol. Bioeng., 2002, 78, 527–538 CrossRef CAS.
- D. P. Cowcher, Y. Xu and R. Goodacre, Anal. Chem., 2013, 85, 3297–3302 CrossRef CAS.
- M. Cheung, W. W. Y. Lee, D. P. Cowcher, R. Goodacre and S. E. J. Bell, Chem. Commun., 2016, 52, 9925–9928 RSC.
- L. C. Thomas and J. E. S. Greenstreet, Spectrochim. Acta, 1954, 6, 302–319 CrossRef.
- L. Mariey, J. P. Signolle, C. Amiel and J. Travert, Vib. Spectrosc., 2001, 26, 151–159 CrossRef CAS.
- D. Helm, H. Labischinski, G. Schallehn and D. Naumann, Microbiology, 1991, 137, 69–79 CrossRef CAS.
-
D. Naumann, in Encyclopedia of Analytical Chemistry, John Wiley & Sons, Ltd, 2006 Search PubMed.
- D. I. Ellis, W. B. Dunn, J. L. Griffin, J. W. Allwood and R. Goodacre, Pharmacogenomics, 2007, 8, 1243–1266 CrossRef CAS.
- R. Gurbanov, A. G. Gozen and F. Severcan, Spectrochim. Acta, Part A, 2018, 189, 282–290 CrossRef CAS.
- H. Muhamadali, A. Subaihi, M. Mohammadtaheri, Y. Xu, D. I. Ellis, R. Ramanathan, V. Bansal and R. Goodacre, Analyst, 2016, 141, 5127–5136 RSC.
-
B. H. Stuart, in Encyclopedia of Analytical Chemistry, John Wiley & Sons, Ltd, 2006 Search PubMed.
-
B. Stuart, Modern infrared spectroscopy, Chichester, 1996 Search PubMed.
- C. Berthomieu and R. Hienerwadel, Photosynth. Res., 2009, 101, 157–170 CrossRef CAS.
- D. Naumann, D. Helm and H. Labischinski, Nature, 1991, 351, 81–82 CrossRef CAS.
- H. AlRabiah, J. W. Allwood, E. Correa, Y. Xu and R. Goodacre, PLoS One, 2018, 13, e0200272 CrossRef.
- H. AlRabiah, Y. Xu, N. J. Rattray, A. A. Vaughan, T. Gibreel, A. Sayqal and R. Goodacre, Analyst, 2014, 139, 4193–4199 RSC.
- N. M. Amiali, M. R. Mulvey, B. Berger-Bächi, J. Sedman, A. E. Simor and A. A. Ismail, J. Antimicrob. Chemother., 2008, 61, 95 CrossRef CAS.
- G. D. Sockalingum, W. Bouhedja, P. Pina, P. Allouch, C. Mandray, R. Labia, J. M. Millot and M. Manfait, Biochem. Biophys. Res. Commun., 1997, 232, 240 CrossRef CAS.
- N. Jin, D. Zhang and F. L. Martin, Integr. Biol., 2017, 9, 406–417 CrossRef CAS.
- N. Jin, M. Paraskevaidi, K. T. Semple, F. L. Maryin and D. Zhang, Anal. Chem., 2017, 89, 9814 CrossRef CAS.
- A. Novais, A. R. Freitas, C. Rodrigues and L. Peixe, Eur. J. Clin. Microbiol., 2019, 38, 427–448 CrossRef.
- P. Zarnowiec, L. Lechowicz, G. Czerwonka and W. Kaca, Curr. Med. Chem., 2015, 22, 1710–1718 CrossRef CAS.
- A. Salman, L. Tsror, A. Pomerantz, R. Moreh, S. Mordechai and M. Huleihel, Spectroscopy, 2101, 24, 261–267 CrossRef.
- K. Forfang, B. Zimmermann, G. Kosa, A. Kohler and V. Shapaval, PLoS One, 2017, 12, e0170611 CrossRef.
- J. Lee, M. S. Ahn, Y. L. Lee, E. Y. Jie, S. G. Kim and S. W. Kim, J. Appl. Microbiol., 2019, 126, 864–871 CrossRef CAS.
- C. A. Rebuffo, J. Schmitt, M. Wenning, F. von Stetten and S. Scherer, Appl. Environ. Microbiol., 2006, 72, 994–1000 CrossRef CAS.
- A. O. Janbu, T. Møretrø, D. Bertrand and A. Kohler, FEMS Microbiol. Lett., 2008, 278, 164 CrossRef CAS.
- Y. D. Wang, X. L. Li, J. Hu and J. H. Lü, Nucl. Sci. Tech., 2019, 30, 25 CrossRef.
- V. Shapaval, J. Schmitt, T. Møretrø, H. Suso, I. Skaar, D. Lillehaug and A. Kohler, J. Appl. Microbiol., 2013, 114, 788–796 CrossRef CAS.
- V. Shapaval, T. Møretrø, A. Wold, H. Suso, J. Schmitt, D. Lillehaug and A. Kohler, Lett. Appl. Microbiol., 2016, 64, 335–34259 CrossRef.
- K. Maquelin, C. Kirschner, L. P. Choo-Smith, N. A. Ngo-Thi, T. van Vreeswijk, M. Stämmler, H. P. Endtz, H. A. Bruining, D. Naumann and G. J. Puppels, J. Clin. Microbiol., 2003, 41, 324–329 CrossRef CAS.
- M. Bağcıoğlu, M. Fricker, S. Johler and M. Ehling-Schulz, Front. Microbiol., 2019, 10, 902 CrossRef.
- A. Hornemann, D. Sinning, S. Cortes, L. Campino, P. Emmer, K. Kuhls, G. Ulm, M. Frohme and B. Beckhoff, Anal. Bioanal. Chem., 2017, 409, 6907–6923 CrossRef CAS.
- C. D. Kaptchouang Tchatchouang, J. Fri, M. De Santi, G. Brandi, G. F. Schiavano, G. Amagliani and C. N. Ateba, Microorganisms, 2020, 8, 135 CrossRef.
- K. F. Romanolo, L. Gorski, S. Wang and C. R. Lauzon, PLoS One, 2015, 10, e0143425 CrossRef CAS.
- S. F. Lin, H. Schraft and M. W. Griffiths, J. Food Prot., 1998, 61, 921–923 CrossRef CAS.
- S. H. Beattie, C. Holt, D. Hirst and A. G. Williams, FEMS Microbiol. Lett., 1998, 164, 201 CrossRef CAS.
- A. Al-Mariri, L. Ramadan, A. Younes and A. Al-Laham, Bulg. J. Vet. Med., 2019, 22, 285–296 Search PubMed.
- S. Treguier, C. Couderc, H. Tormo, D. Kleiber and C. Levasseur-Garcia, J. Microbiol. Methods, 2019, 165, 105693 CrossRef CAS.
- H. Oberreuter and J. Rau, FEMS Microbiol. Lett., 2019, 366, fnz193 CrossRef CAS.
- G. Abu-Aqil, L. Tsror, E. Shufan, S. Mordechai, M. Huleihel and A. Salman, J. Biophotonics, 2020, 13, e201960156 CrossRef.
- K. Kochan, C. Nethercott, J. Taghavimoghaddam, Z. Richardson, E. Lai, S. Crawford, A. Y. Peleg, B. R. Wood and P. Heraud, Anal. Chem., 2020, 92, 8235–8243 CrossRef CAS.
- M. J. Baker, J. Trevisan, P. Bassan, R. Bhargava, H. J. Butler, K. M. Dorling, P. R. Fielden, S. W. Fogarty, N. J. Fullwood, K. A. Heys, C. Hughes, P. Lasch, P. L. Martin-Hirsch, B. Obinaju, G. D. Sockalingum, J. Sulé-Suso, R. J. Strong, M. J. Walsh, B. R. Wood, P. Gardner and F. L. Martin, Nat. Protocols, 2014, 9, 1771–1791 CAS.
- M. J. Walsh, M. N. Singh, H. M. Pollock, L. J. Cooper, M. J. German, H. F. Stringfellow, N. J. Fullwood, E. Paraskevaidis, P. L. Martin-Hirsch and F. L. Martin, Biochem. Biophys. Res. Commun., 2007, 352, 213–219 CrossRef CAS.
- C. S. Ho, N. Jean, C. A. Hogan, L. Blackmon, S. S. Jeffrey, M. Holodniy, N. Banaei, A. A. Saleh, S. Ermon and J. Dionne, Nat. Commun., 2019, 10, 1–8 CrossRef.
- P. E. Fournier, M. Drancourt, P. Colson, J. M. Rolain, B. L. Scola and D. Raoult, Nat. Rev. Microbiol., 2013, 11, 574–585 CrossRef CAS.
- D. I. Ellis, H. Muhamadali, S. A. Haughey, C. T. Elliott and R. Goodacre, Anal. Methods, 2015, 7, 9401–9414 RSC.
- H. J. Butler, L. Ashton, B. Bird, G. Cinque, K. Curtis, J. Dorney, K. Esmonde-White, N. J. Fullwood, B. Gardner, P. L. Martin-Hirsch, M. J. Walsh, M. R. McAinsh, N. Stone and F. L. Martin, Nat. Protocols, 2016, 11, 664–687 CAS.
- T. Vankeirsbilck, A. Vercauteren, W. Baeyens, G. Van der Weken, F. Verpoort, G. Vergote and J. P. Remon, Trends Anal. Chem., 2002, 21, 869–877 CrossRef CAS.
- S. Pahlow, S. Meisel, D. Cialla-May, K. Weber and J. P. P. Röschac, Adv. Drug Delivery Rev., 2015, 89, 105–120 CrossRef CAS.
- S. Lohumi, H. Lee and B.-K. Cho, Trends Food Sci. Technol., 2015, 46, 85–98 CrossRef CAS.
- M. Chisanga, D. Linton, H. Muhamadali, D. I. Ellis, R. L. Kimber, A. Mironov and R. Goodacre, Analyst, 2020, 145, 1236–1249 RSC.
- A. V. Vlasov, N. L. Maliar, S. V. Bazhenov, E. I. Nikelshparg, N. A. Brazhe, A. D. Vlasova, S. D. Osipov, V. V. Sudarev, Y. L. Ryzhykau, A. O. Bogorodskiy and E. V. Zinovev, Crystals, 2020, 10, 38 CrossRef CAS.
- S. D. Dryden, S. Anastasova, G. Satta, A. J. Thompson, D. R. Leff and A. Darzi, Int. Soc. Opt. Photonics, 2020, 11247, 1124705 Search PubMed.
- M. Chisanga, H. Muhamadali, D. I. Ellis and R. Goodacre, Appl. Sci., 2019, 9, 1163 CrossRef CAS.
- J. R. Baena and B. Lendl, Curr. Opin. Chem. Biol., 2004, 8, 534–539 CrossRef CAS.
- J. De Gelder, K. De Gussem, P. Vandenabeele and L. Moens, J. Raman Spectrosc., 2007, 38, 1133–1147 CrossRef CAS.
- T. Hellerer, C. Axäng, C. Brackmann, P. Hillertz, M. Pilon and A. Enejder, Proc. Natl. Acad. Sci. U. S. A., 2007, 104, 14658–14663 CrossRef CAS.
- H. G. Breunig, R. Bückle, M. Kellner-Höfer, M. Weinigel, J. Lademann, W. Sterry and K. König, Microsc. Res. Tech., 2012, 75, 492–498 CrossRef.
- C. W. Freudiger, W. Min, B. G. Saar, S. Lu, G. R. Holtom, C. He, J. C. Tsai, J. X. Kang and X. S. Xie, Science, 2008, 322, 1857–1861 CrossRef CAS.
- G. Clemens, J. R. Hands, K. M. Dorling and M. J. Baker, Analyst, 2014, 139, 4411–4444 RSC.
-
W. E. Huang, M. Li, R. M. Jarvis, R. Goodacre and S. A. Banwart, in Advances in applied microbiology, Academic Press, 2010, vol. 70, pp. 153–186 Search PubMed.
- S. Kumar, R. Gopinathan, G. K. Chandra, S. Umapathy and D. K. Saini, Anal. Bioanal. Chem., 2020, 412, 2505–2516 CrossRef CAS.
- A. I. M. Athamneh, R. A. Alajlouni, R. S. Wallace, M. N. Seleem and R. S. Senger, Antimicrob. Agents Chemother., 2014, 58, 302–1314 CrossRef.
- E. C. López-Díez and R. Goodacre, Anal. Chem., 2014, 76, 585–591 CrossRef.
- E. C. López-Díez, C. L. Winder, L. Ashton, F. Currie and R. Goodacre, Anal. Chem., 2005, 77, 2901–2906 CrossRef.
- H. Zhou, D. Yang, N. P. Ivleva, N. E. Mircescu, S. Schubert, R. Niessner, A. Wieser and C. Haisch, Anal. Chem., 2015, 87, 6553–6561 CrossRef CAS.
- D. Kusić, B. Kampe, P. Rösch and J. Popp, Water Res., 2014, 48, 179–189 CrossRef.
- A. Assaf, E. Grangé, C. B. Y. Cordella, D. N. Rutledge, M. Lees, A. Lahmar and G. Thouand, Anal. Bioanal. Chem., 2020, 412, 595–3604 CrossRef.
- J. Wang, X. Wu, C. Wang, N. Shao, P. Dong, R. Xiao and S. Wang, ACS Appl. Mater. Interfaces, 2015, 7, 20919–20929 CrossRef CAS.
- C. Xie, J. Mace, M. A. Dinno, Y. Q. Li, W. Tang, R. J. Newton and P. J. Gemperline, Anal. Chem., 2005, 77, 4390–4397 CrossRef CAS.
- M. Harz, P. Rösch, K. D. Peschke, O. Ronneberger, H. Burkhardt and J. Popp, Analyst, 2005, 130, 1543–1550 RSC.
- Z. Schmilovitch, A. Mizrach, V. Alchanatis, G. Kritzman, R. Korotic and J. Irudayaraj, Trans. ASAE, 2005, 48, 1843–1850 Search PubMed.
- A. Subaihi, L. Almanqur, H. Muhamadali, N. AlMasoud, D. I. Ellis, D. K. Trivedi, K. A. Hollywood, Y. Xu and R. Goodacre, Anal. Chem., 2016, 88, 10884–10892 CrossRef CAS.
- M. Chisanga, H. Muhamadali, R. Kimber and R. Goodacre, Faraday Discuss., 2017, 205, 331–343 RSC.
- M. Chisanga, H. Muhamadali, D. I. Ellis and R. Goodacre, Appl. Spectrosc., 2018, 72, 987–1000 CrossRef CAS.
- D. Graham, R. Goodacre, H. Arnolds, J. F. Masson, G. Schatz, J. Baumberg, D. H. Kim, J. Aizpurua, W. Lum, A. Silvestri and B. De Nijs, Faraday Discuss., 2017, 205, 173–211 RSC.
- P. L. Stiles, J. A. Dieringer, N. C. Shah and R. P. Van Duyne, Annu. Rev. Anal. Chem., 2008, 1, 601–621 CrossRef CAS.
- S. Schlucker, Surface-Enhanced Raman Spectroscopy: Concepts and Chemical Applications, Angew. Chem., Int. Ed., 2014, 53, 4756–4795 CrossRef.
- R. M. Jarvis and R. Goodacre, Anal. Chem., 2004, 76, 40–47 CrossRef CAS.
- R. M. Jarvis, N. Law, I. T. Shadi, P. O'Brien, J. Lloyd and R. Goodacre, Anal. Chem., 2008, 80, 6741–6746 CrossRef CAS.
- J. Baumberg, S. Bell, A. Bonifacio and R. Chikkaraddy,
et al.
, Faraday Discuss., 2017, 205, 429–456 RSC.
- H. Kearns, R. Goodacre, L. E. Jamieson, D. Graham and K. Faulds, Anal. Chem., 2017, 89, 12666–12673 CrossRef CAS.
- R. Goodacre, D. Graham and K. Faulds, TrAC, Trends Anal. Chem., 2018, 102, 359–368 CrossRef CAS.
- K. C. Schuster, I. Reese, E. Urlaub, J. R. Gapes and B. Lendl, Anal. Chem., 2000, 72, 5529–5534 CrossRef CAS.
- B. Lorenz, C. Wichmann, S. Stöckel, P. Rösch and J. Popp, Trends Microbiol., 2017, 25, 413–424 CrossRef CAS.
- A. Dazzi, C. B. Prater, Q. Hu, D. B. Chase, J. F. Rabolt and C. Marcott, Appl. Spectrosc., 2012, 66, 1365–1384 CrossRef CAS.
- D. Zhang, C. Li, C. Zhang, M. N. Slipchenko, G. Eakins and J. X. Cheng, Sci. Adv., 2016, 2, e1600521 CrossRef.
-
M. Hesse, H. Meier and B. Zeeh, Spectroscopic Methods in Organic Chemistry, Thieme, Stuttgart, 2nd edn, 2008 Search PubMed.
- R. Goodacre and D. B. Kell, Curr. Opin. Biotechnol., 1996, 7, 20–28 CrossRef CAS.
- R. Goodacre, E. M. Timmins, R. Burton, N. Kaderbhai, A. M. Woodward, D. B. Kell and P. J. Rooney, Microbiology, 1998, 144, 1157–1170 CrossRef CAS.
- A. Fox, J. Clin. Microbiol., 2006, 44, 2677–2680 CrossRef CAS.
- W. B. Dunn, A. Erban, R. J. M. Weber, D. J. Creek, M. Brown, R. Brietling, T. Hankemeier, R. Goodacre, S. Neumann, J. Kopka and M. R. Viant, Metabolomics, 2013, 9, S44–S66 CrossRef.
- M. Karas and F. Hillenkamp, Anal. Chem., 1988, 60, 2299–2303 CrossRef CAS.
- L. Yin, Z. Zhang, Y. Liu, Y. Gao and J. Gu, Analyst, 2019, 144, 824–845 RSC.
- S. Sauer, M. H. Bodo Lange, G. Johan, N. Lajos, S. Harald and L. Hans, Nat. Rev. Genet., 2005, 6, 465–476 CrossRef CAS.
- S. Vaidyanathan, D. B. Kell and R. Goodacre, J. Am. Soc. Mass Spectrom., 2002, 13, 118–128 CrossRef CAS.
- S. Vaidyanathan, J. J. Rowland, D. B. Kell and R. Goodacre, Anal. Chem., 2001, 73, 4134–4144 CrossRef CAS.
- T. Luzzatto-Knaan, A. V. Melnik and P. C. Dorrestein, Analyst, 2015, 140, 4949–4966 RSC.
- B. Fuchs, R. Süß and J. Schiller, Prog. Lipid Res., 2010, 49, 450–475 CrossRef CAS.
- R. Giebel, C. Worden, S. M. Rust, G. T. Kleinheinz, M. Robbins and T. R. Sandrin, Adv. Appl. Microbiol., 2010, 71, 149–184 CAS.
- N. AlMasoud, Y. Xu, N. Nicolaou and R. Goodacre, Anal. Chim. Acta, 2014, 840, 49–57 CrossRef CAS.
- P. Lasch, W. Beyer, H. Nattermann, M. Stammler, E. Siegbrecht, R. Grunow and D. Naumann, Appl. Environ. Microbiol., 2009, 75, 7229–7242 CrossRef CAS.
- A. Mewara, M. Sharma, T. Kaura, K. Zaman, R. Yadav and R. Sehgal, Parasites Vectors, 2018, 11, 281 CrossRef.
- M. Kussmann, E. Nordhoff, H. Rahbek-Nielsen, S. Haebel, M. Rossel-Larsen, L. Jakobsen, J. Gobom, E. Mirgorodskaya, A. Kroll-Kristensen, L. Palm and P. Roepstorff, J. Mass Spectrom., 1997, 32, 593–601 CrossRef CAS.
- S. Bourcier, S. Bouchonnet and Y. Hoppilliard, Int. J. Mass Spectrom., 2001, 210, 59–69 CrossRef.
- N. AlMasoud, E. Correa, D. K. Trivedi and R. Goodacre, Anal. Chem., 2016, 88, 6301–6308 CrossRef CAS.
- N. AlMasoud, Y. Xu, D. K. Trivedi, S. Salivo, T. Abban, N. J. W. Rattray, E. Szula, H. AlRabiah, A. Sayqal and R. Goodacre, Anal. Bioanal. Chem., 2016, 408, 7865–7878 CrossRef CAS.
- W. Yan, J. Qian, Y. Ge, K. Ye, C. Zhou and H. Zhang, Anal. Biochem., 2020, 592, 113582 CrossRef CAS.
- X. Xu, N. Xiao, M. Yang, Y. Su and Y. Guo, Talanta, 2020, 208, 120361 CrossRef CAS.
- A. E. Clark, E. J. Kaleta, A. Arora and D. M. Wolk, Clin. Microbiol. Rev., 2013, 26, 547–603 CrossRef CAS.
- T. R. Sandrin, J. E. Goldstein and S. Schumaker, Mass Spectrom. Rev., 2013, 32, 188–217 CrossRef CAS.
- A. Freiwald and S. Sauer, Nat. Protocols, 2009, 4, 732–742 CAS.
- B. Feng, H. Shi, F. Xu, F. Hu, J. He, H. Yang, C. Ding, W. Chen and S. Yu, Anal. Chim. Acta, 2020, 1111, 75–82 CrossRef CAS.
- A. Mukherjee, G. Banerjee, P. Mukherjee, A. K. Ray, G. Chandra and K. Ghosh, Microb. Pathog., 2019, 130, 146–155 CrossRef CAS.
- S. S. Rubakhin and J. V. Sweedler, Anal. Chem., 2008, 80, 7128–7136 CrossRef CAS.
- F. Calderón-Celis, J. R. Encinar and A. Sanz-Medel, Mass Spectrom. Rev., 2018, 37, 715–737 CrossRef.
- R. Liyanage, J. Gidden, C. L. Wilkins and J. O. Lay Jr., Rapid Commun. Mass Spectrom., 2019, 1–10 Search PubMed.
- Y. Li, M. Shan, Z. Zhu, X. Mao, M. Yan, Y. Chen, Q. Zhu, H. Li and B. Gu, BMC Infect. Dis., 2019, 19, 941 CrossRef.
- Y. Liu, Q. Cao and L. Li, Methods Enzymol., 2019, 626, 147–202 CAS.
- D. Zhang, Y. Yang, Q. Qin, J. Xu, B. Wang, J. Chen, B. Liu, W. Zhang and L. Qiao, Anal. Chem., 2019, 91, 2352–2359 CrossRef CAS.
-
J. Krismer, J. Sobek, R. F. Steinhoff, R. Brönnimann, M. Pabst and R. Zenobi, in Single Cell Metabolism, Humana, New York, NY, 2020, pp. 113–124 Search PubMed.
-
C. Fenselau, Mass spectrometry for characterization of microorganisms, in ACS Symposium Series, American Chemical Society, Washington, DC, USA, 1993, ch. 1, vol. 541, pp. 1–7 Search PubMed.
- M. A. Claydon, S. N. Davey, V. Edwards-Jones and D. B. Gordon, Nat. Biotechnol., 1996, 14, 1584–1586 CrossRef CAS.
- R. D. Holland, J. G. Wilkes, F. Rafii, J. B. Sutherland, C. C. Persons, K. J. Voorhees and J. O. Lay, Rapid Commun. Mass Spectrom., 1996, 10, 1227–1232 CrossRef CAS.
- C. Lange, S. Schubert, J. Jung, M. Kostrzewa and K. Sparbier, J. Clin. Microbiol., 2014, 52, 4155–4162 CrossRef.
- C. Axelsson, A. S. Rehnstam-Holm and B. Nilson, Infect. Dis., 2020, 52, 45–53 CrossRef CAS.
- M. L. DeMarco and C. A. D. Burnham, Am. J. Clin. Pathol., 2014, 141, 204–212 CrossRef.
- S. Nakano, Y. Matsumura, Y. Ito, T. Fujisawa, B. Chang, S. Suga, K. Kato, T. Yunoki, G. Hotta and T. Noguchi, Eur. J. Clin. Microbiol. Infect. Dis., 2015, 34, 2191–2198 CrossRef CAS.
- K. Woods, D. Beighton and J. L. Klein, J. Med. Microbiol., 2014, 63, 1143–1147 CrossRef.
- N. AlMasoud, Y. Xu, D. I. Ellis, P. Rooney, J. F. Turton and R. Goodacre, Anal. Methods, 2016, 8, 7603–7613 RSC.
- Y. Zhu, L. Qiao, M. Prudent, A. Bondarenko, N. Gasilova, S. B. Möller, N. Lion, H. Pick, T. Gong, Z. Chen, P. Yang, L. T. Lovey and H. H. Girault, Chem. Sci., 2016, 7, 2987–2995 RSC.
- E. N. Ilina, A. D. Borovskaya, M. M. Malakhova, V. A. Vereshchagin, A. A. Kubanova and A. N. Kruglov, J. Mol. Diagn., 2009, 11, 75–86 CrossRef CAS.
- R. Eddabra, P. Gilles and S. Jean-Michel, Microbiol. Res., 2012, 167, 226–230 CrossRef CAS.
- A. A. Alatoom, C. J. Cazanave, S. A. Cunningham, S. M. Ihde and R. Patel, J. Clin. Microbiol., 2012, 50, 160–163 CrossRef CAS.
- R. T. Saffert, S. A. Cunningham, S. M. Ihde, K. E. M. Jobe, J. Mandrekar and R. Patel, J. Clin. Microbiol., 2011, 49, 887–892 CrossRef.
- A. Verroken, M. Janssens, C. Berhin, P. Bogaerts, T. D. Huang and G. Wauters, J. Clin. Microbiol., 2010, 48, 4015–4021 CrossRef CAS.
- A. El Khéchine, C. Couderc, C. Flaudrops, D. Raoult and M. Drancourt, PLoS One, 2011, 6, e24720 CrossRef.
- O. Šedo, I. Sedláček and Z. Zdráhal, Mass Spectrom. Rev., 2010, 30, 417–434 CrossRef.
- P. A. Demirev, Y. P. Ho, V. Ryzhov and C. Fenselau, Anal. Chem., 1999, 71, 2732–2738 CrossRef CAS.
- E. Elhanany, R. Barak, M. Fisher, D. Kobiler and Z. Altboum, Rapid Commun. Mass Spectrom., 2001, 15, 2110–2116 CrossRef CAS.
- V. Horneffer, J. Haverkamp, H. G. Janssen and R. Notz, J. Am. Soc. Mass Spectrom., 2004, 15, 1444–1454 CrossRef CAS.
- E. I. Shaw, H. Moura, A. R. Woolfitt, M. Ospina, H. A. Thompson and J. R. Barr, Anal. Chem., 2004, 76, 4017–4022 CrossRef CAS.
- C. J. Keys, D. J. Dare, H. Sutton, G. Wells, M. Lunt, T. McKenna, M. McDowall and H. N. Shah, Infect., Genet. Evol., 2004, 4, 221–242 CrossRef CAS.
- F. J. Pineda, M. D. Antoine, P. A. Demirev, A. B. Feldman, J. Jackman, M. Longenecke and J. S. Lin, Anal. Chem., 2003, 75, 3817–3822 CrossRef CAS.
- J. Ramirez and C. Fenselau, J. Mass Spectrom., 2001, 36, 929–936 CrossRef CAS.
- H. Moura, A. R. Woolfitt, M. G. Carvalho, A. Pavlopoulos, L. M. Teixeria, G. A. Satten and J. R. Barr, FEMS Immunol. Med. Microbiol., 2008, 53, 333–342 CrossRef CAS.
- M. L. Ochoa and P. B. Harrington, Anal. Chem., 2005, 77, 5258–5267 CrossRef CAS.
- J. J. Jones, M. J. Stump, R. C. Fleming, J. O. Lay and C. L. Wilkins, Anal. Chem., 2003, 75, 1340–1347 CrossRef CAS.
- S. Vaidyanathan, C. L. Winder, S. C. Wade, D. B. Kell and R. Goodacre, Rapid Commun. Mass Spectrom., 2002, 16, 1276–1286 CrossRef CAS.
- A. J. Madonna, F. Basile, I. Ferrer, M. A. Meetani, J. C. Rees and K. J. Voorhes, Rapid Commun. Mass Spectrom., 2002, 14, 2220–2229 CrossRef.
- M. A. Domin, K. J. Welham and D. S. Ashton, Rapid Commun. Mass Spectrom., 1999, 13, 222–226 CrossRef CAS.
- G. C. Conway, S. C. Smole, D. A. Sarracino, R. D. Arbeit and P. E. Leopold, J. Mol. Microbiol. Biotechnol., 2001, 3, 103–112 CAS.
- V. Ruelle, B. El Moualij, W. Zorzi, P. Ledent and E. De Pauw, Rapid Commun. Mass Spectrom., 2004, 18, 2013–2019 CrossRef CAS.
- S. Wold, K. Esbensen and P. Geladi, Chemom. Intell. Lab. Syst., 1987, 2, 37–52 CrossRef CAS.
- D. Granato, J. S. Santos, G. B. Escher, B. L. Ferreira and R. M. Maggio, Trends Food Sci. Technol., 2018, 72, 83–90 CrossRef CAS.
- P. S. Gromski, H. Muhamadali, D. I. Ellis, Y. Xu, E. Correa, M. L. Turner and R. Goodacre, Anal. Chim. Acta, 2015, 879, 10–23 CrossRef CAS.
- J. Schmidhuber, Neural Netw., 2015, 61, 85–117 CrossRef.
-
D. E. Rumelhart, J. L. McClelland and P. R. Group, Parallel Distributed Processing, Experiments in the Microstructure of Cognition, MIT Press, Cambridge, MA, USA, 1986, pp. 1–2 Search PubMed.
- D. I. Broadhurst and D. B. Kell, Metabolomics, 2006, 2, 171–196 CrossRef CAS.
- R. S. Khan and I. U. Rehman, Expert Rev. Mol. Diagn., 2020, 647–649 CrossRef CAS.
Footnote |
† Present address: School of Mathematics and Natural Sciences, Department of Chemistry, Copperbelt University, Kitwe, Zambia. |
|
This journal is © The Royal Society of Chemistry 2021 |
Click here to see how this site uses Cookies. View our privacy policy here.