DOI:
10.1039/D4LC00533C
(Paper)
Lab Chip, 2024, Advance Article
Sample-to-answer centrifugal microfluidic droplet PCR platform for quantitation of viral load†
Received
21st June 2024
, Accepted 30th August 2024
First published on 11th September 2024
Abstract
Droplet digital polymerase chain reaction (ddPCR) stands out as a highly sensitive diagnostic technique that is gaining traction in infectious disease diagnostics due to its ability to quantitate very low numbers of viral gene copies. By partitioning the sample into thousands of droplets, ddPCR enables precise and absolute quantification without relying on a standard curve. However, current ddPCR systems often exhibit relatively low levels of integration, and the analytical process remains dependent on elaborate workflows for up-front sample preparation. Here, we introduce a fully-integrated system seamlessly combining viral lysis, RNA extraction, emulsification, reverse transcription (RT) ddPCR, and fluorescence readout in a sample-to-answer format. The system comprises a disposable microfluidic cartridge housing buffers and reagents required for the assay, and a centrifugal platform that allows for pneumatic actuation of liquids during rotation, enabling automation of the workflow. Highly monodisperse droplets (∼50 μm in diameter) are produced using centrifugal step emulsification and automatically transferred to an integrated heating module for target amplification. The platform is equipped with a miniature fluorescence imaging system enabling on-chip read-out of droplets after RT-ddPCR. We demonstrate sample-to-answer detection of SARS-CoV-2 N and E genes, along with RNase P endogenous reference, using hydrolysis probes and multiplexed amplification within single droplets for concentrations as low as 0.1 copy per μL. We also tested 14 nasopharyngeal swab specimens from patients and were able to distinguish positive and negative SARS-CoV-2 samples with 100% accuracy, surpassing results obtained by conventional real-time amplification.
1 Introduction
Infectious diseases continue to challenge societies globally, with timely and accurate diagnosis serving as a cornerstone in disease management, epidemiological tracking, and public health interventions.1,2 The recent COVID-19 pandemic caused by severe acute respiratory syndrome coronavirus 2 (SARS-CoV-2)3 has underscored the need for innovative methods and technologies that enable not only detection, but also sensitive quantification of viral pathogens.4 For example, several public health initiatives relied on the relationship between viral load and the potential for SARS-CoV-2 transmission. Assessment of viral load was also key to monitoring disease progression and therapy response for infected individuals. Although the level of SARS-CoV-2 can fluctuate over the course of an infection, increased disease severity and mortality have been shown to correlate with higher SARS-CoV-2 levels.5
Nucleic acid (NA) testing based on quantitative reverse transcriptase polymerase chain reaction (RT-qPCR) has become the standard method for detecting SARS-CoV-2, as recommended by the Centers for Disease Control and Prevention.6 The concentration of RNA in the sample is thereby inferred from amplification rates relative to a standard curve. While valuable and robust, RT-qPCR encounters limitations in terms of sensitivity and consistency with clinical specimens, especially when the viral load is low.4,7 RT-qPCR tests for SARS-CoV-2 require upfront sample lysis and RNA purification, which rely on workflows that are time- and labour-intense.8 Moreover, the presence of contamination may affect the efficiency of the amplification process and therefore the accuracy of quantification.9,10
Droplet digital polymerase chain reaction (ddPCR)11 has emerged as a transformative technique that offers unparalleled precision and sensitivity in the detection of SARS-CoV-2.12,13 Conceptually, ddPCR partitions the sample into thousands of individual droplets, each of which then serves as an independent reaction vessel. Once PCR cycling is performed, positive droplets are enumerated to count target NA strands. In this way, absolute quantification can be achieved without a standard curve. Its high sensitivity makes ddPCR particularly useful for detecting low target concentrations, even in complex sample matrices. Commercial instruments such as the QX600™ Droplet Digital PCR System from Bio-Rad Laboratories and the Naica® Crystal Digital PCR system from Stilla Technologies harness much of the potential ddPCR offers as an analytical method. They are particularly well suited for centralized diagnostic laboratories as they are compatible with multiwell plate formats utilized by automated liquid handlers and allow processing of large numbers of samples within several hours. However, these instruments require upstream sample preparation, sophisticated infrastructure, trained operators, and significant upfront investment. Moreover, from the technological standpoint, with pressure-driven flow remaining the primary method for sample emulsification in ddPCR systems, auxiliary pumps and world-to-chip connectivity remain important components of instrument design. Ongoing research and development efforts are directed toward the development of compact, multifunctional systems compatible with sample-to-answer workflows for diagnostic applications at the point of need, including clinical settings and remote locations.
Centrifugation has gained increasing attention as a convenient alternative to emulsification using pressure-driven flow.14 The dynamic forces generated upon rotation of a microfluidic disk or cartridge allow for controlling the displacement of fluid inside micro-scale channels with high precision. Since external pumps are not required for interfacing the fluidic circuit, a relatively low level of instrument complexity can be maintained for many applications. Centrifugal-driven emulsification has been shown to produce droplets at very high rates while maintaining a low coefficient of variation (CV), and is being used increasingly to conduct digital assays on rotating platforms.15–21 For example, Schuler et al. have employed centrifugal step emulsification to produce droplets of 120–170 μm in diameter for performing recombinase polymerase amplification (RPA)15 and ddPCR.16 Li et al. have shown centrifugal-based emulsification for multiple displacement amplification (MDA) at the single-cell level.17 Peng et al. have developed a centrifugal microfluidic emulsification system for DNA analysis using droplet digital loop-mediated isothermal amplification (ddLAMP).18 Wang et al. have demonstrated a centrifugal microfluidic pressure regulator where the droplet generation process is controlled by the rotation speed to perform cell transfection.19 Schlenker et al. have implemented a four-plex ddPCR assay on a centrifugal microfluidic platform to quantify cancer-associated mutations.20 Schulz et al. have explored dual-volume centrifugal step emulsification as a means of extending the dynamic range in NA quantification using ddLAMP.21
However, air bubble formation and coalescence of droplets during thermal cycling have been frequently reported as issues that can potentially impede the performance of digital assays on centrifugal systems.16,18,20 Air bubbles arising from the degassing of the oil phase can disrupt water-in-oil emulsions inside a shallow chamber, which complicates the read-out. To mitigate this problem, Schuler et al. have designed a tapered ddPCR chamber that allows unwanted gas bubbles to be eliminated from the system.16 Peng et al. have incorporated specially designed oil storage structures for redistributing pressure, reducing the coalescence between neighbouring droplets.18
Herein, we describe a centrifugal sample-to-answer system for quantitative detection of viral pathogens using RT-ddPCR (Fig. 1) where we address some of the technical limitations that so far have prevented wide-spread applicability of ddPCR in clinical settings. We solve the problem of air bubble formation and droplet coalescence in a pragmatic, yet effective way by relocating the water-in-oil emulsion generated on a microfluidic cartridge to an external thermocycling compartment attached to the rotating stage. This transfer between the microfluidic cartridge and the heating module takes advantage of a previously developed platform equipped with a programmable, on-board active pneumatic pumping and pressure control system.22–26 The additional degree of freedom provided by this platform allows for conducting fluid manipulation steps that are difficult or impossible to perform using conventional rotating systems. Moreover, it allows for full automation of the ddPCR protocol from sample preparation to detection using a single integrated microfluidic cartridge. The platform also features a three-channel miniature epi-fluorescence imaging module for visualization and read-out. The potential of this system is demonstrated by conducting a fully automated sample-to-answer workflow that combines sample preparation and multiplexed amplification of N and E genes of SARS-CoV-2 along with RNase P endogenous reference in single droplets using hydrolysis probes.
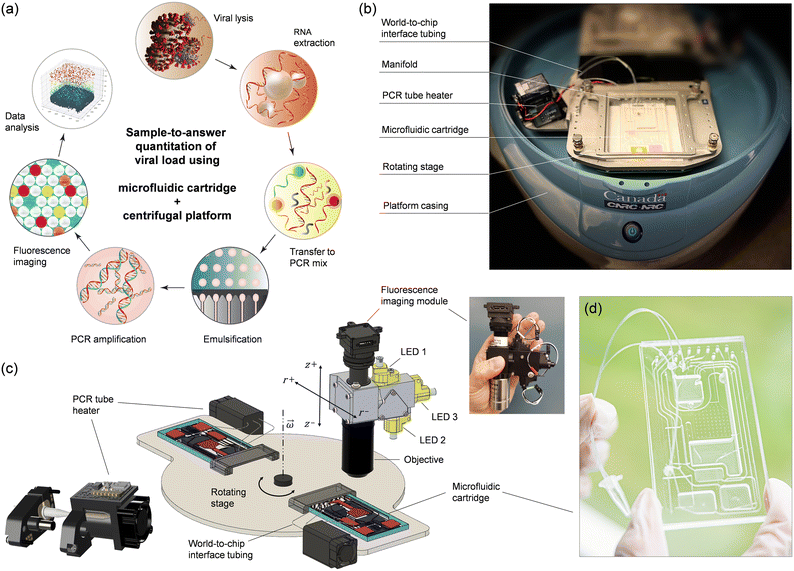 |
| Fig. 1 Implementation of the automated ddPCR-based sample-to-answer detection process. (a) The workflow comprises viral lysis, RNA extraction, preparation and partitioning of the reaction mixture, and PCR amplification, followed by fluorescence imaging and data analysis. The process is integrated on a polymer-based microfluidic cartridge that is operated on a centrifugal platform with active pneumatic pumping. (b) Photograph of a microfluidic cartridge installed on the rotating stage of the platform. World-to-chip interface tubing connects the cartridge to the PCR tube inside the tube heater. (c) Schematic representation showing the rotating stage of the platform with the mounted PCR tube heater and the setup used for fluorescence imaging of droplet monolayers on the cartridge. Insets show a 3D model of the PCR tube heater and a photograph of the compact multispectral fluorescence imaging system used in this work. (d) Photograph of an assembled cartridge. | |
2 Concept and design implementation
The sample-to-answer process (Fig. 1a) starts with a nasopharyngeal swab (NPS) specimen suspended in viral transport medium (VTM). The automated workflow includes viral lysis, RNA extraction, transfer to PCR mix, emulsification, thermal cycling, and fluorescence imaging of a droplet monolayer conducted on a centrifugal platform (Fig. 1b and c) using a disposable multifunctional microfluidic cartridge (Fig. 1d). Integration of these steps required several technical implementations that are detailed below.
2.1 Centrifugal microfluidic platform
Active pneumatic pumping in centrifugal microfluidics offers several advantages for managing complex workflows, enabling multiple assay steps to be conducted either in parallel or sequentially.23–29 With this technology, liquids can be transported reliably in any direction, allowing reservoirs to be placed anywhere across the entire surface of the cartridge. Moreover, active pneumatic pumping can be used to mediate the transfer of liquid to and from external vials, promoting advanced world-to-chip interfacing.27,28 The pneumatic system originally designed for the platform comprised 8 pressure ports which allowed us to successfully integrate and automate several sample preparation and detection assays. However, 8 pressure ports were insufficient for conducting the more elaborate sample-to-answer workflow developed here. For this reason, we upgraded the pneumatic module and increased the number of independent pneumatic lines to 12 per cartridge (Fig. S1†).
The platform also integrates planar thermoelectric elements located on the rotating stage underneath the cartridge. While this temperature control method proved suitable for on-chip PCR temperature cycling24 and isothermal amplification,25 it presented unique challenges when applied to ddPCR. The merging of droplets during the thermal cycling process necessitated the exploration of alternative strategies. By taking advantage of the world-to-chip interfacing capabilities of the platform, we implemented a small, programmable heating module on the rotating stage to perform thermal cycling (Fig. 1b and c). The heater is adapted for accommodating a disposable PCR microtube connected to the cartridge through laboratory tubing (Fig. S2 and associated text in the ESI†). As part of the integrated process, the emulsion is transferred from the cartridge to the microtube and back in an automated and reproducible manner using the pneumatic control system of the platform. The heating module is composed of five key elements (Fig. S3 and S4†): (i) two heating coils; (ii) a cooling fan; (iii) a pinch valve mechanism, (iv) a microcontroller, and (v) a wireless communication board. One of the heating coils is used for temperature cycling of the microtube body (between 60 and 95 °C) while the other coil is heating the cap of the microtube at a constant temperature (e.g., ∼100 °C) to prevent evaporation/condensation issues, much like a conventional PCR thermocycler. During the PCR amplification, the tubing is closed using a programmable pinching mechanism (Fig. S5†) as an additional strategy to prevent evaporation. The module is powered through the rotor of the microfluidic platform while programming and communication with the main computer is done wirelessly using the integrated microcontroller board. The miniature PCR module can provide heating/cooling ramps of ∼0.7 °C s−1, allowing the entire thermal cycling protocol to be performed in less than 2 h (Fig. S6 and S7†).
Finally, we outfitted the platform with a miniature epi-fluorescence imaging module (Fig. 1c and S8†) comprising single-color excitation LEDs and proprietary optical filters for three spectral channels (488, 555, and 645 nm) to enable detection of hydrolysis probes labeled with FAM, ROX and Cy5 fluorophores. The miniaturized module uses the multiband dichroic beam splitter and multiband pass emission filter approach which eliminates optomechanical complexity and bulkiness associated with filter wheels, providing a compact and economical solution to multicolor fluorescence imaging. The three channels have been selected to provide sufficient wavelength separation (>60 nm) and minimize overlap of the emission spectra. Using individual LEDs for each channel, only one fluorophore is excited at a time, allowing fluorescence images to be recorded sequentially with a monochrome CCD camera. We opted for a 2× objective to provide a large field-of-view (8 mm × 8 mm) with the capacity to capture over 10
000 droplets simultaneously.
2.2 Microfluidic cartridge
The microfluidic circuit (Fig. 2a) adopts a configuration that is adequate for conducting the analytical protocol in a reliable, fully-automated fashion. Storage compartments are equipped with exit channels designed to hold liquids until needed. Openings for the pressure ports (#1 to 12) are located on the bottom of the cartridge, interfacing with the pneumatic manifold (Fig. S1†). These ports are used to apply controlled air pressure to push liquids toward the exit channels. Downstream transfer occurs once the applied pneumatic pressure is high enough to displace the fluid front within the exit channel toward the target reservoir.
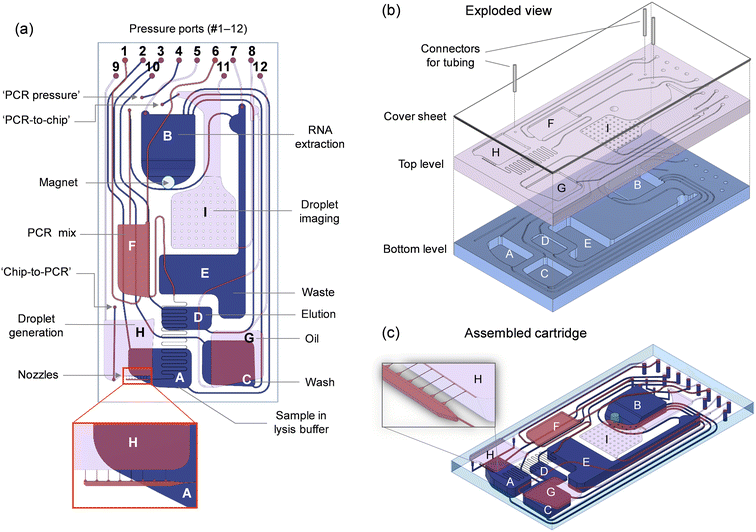 |
| Fig. 2 Microfluidic cartridge used for implementation of the analytical process. (a) Cartridge design (top view). Color coding is used to represent two different levels of the cartridge, with the bottom level in dark blue and the top level in light pink. The inset shows the droplet generation unit. (b and c) 3D renderings of the cartridge configuration revealing the microfluidic features implemented on the top and bottom levels (b) before and (c) after assembly. | |
An important consideration in the distribution of channels and reservoirs is their size, which, in turn, has an impact on the manufacturability of the cartridge. Combining both small (μm) and large (mm) microfluidic elements on a single plane represents a challenge for cartridge fabrication. Here, the dimensions of channels and reservoirs implemented in the fluidic design (Table S1†) vary considerably in width and depth (from 10 μm to 4.5 mm) depending on their intended purpose. For example, the volumes to be accommodated (Table S2†) range from tenths of microliters (for the elution buffer) to hundreds of microliters (for the sample, oil and wash buffer) and more than 1 mL (for the waste reservoir). Also, the droplet generation structure features narrow channels (15 μm in width, 10 μm in depth) to achieve a droplet diameter of ∼50 μm at relatively low rotation speeds. For this reason, we separated larger from smaller features in the design and considered a two-level microfluidic architecture (Fig. 2b and c) where the bottom level contains deep reservoirs (for RNA extraction, storage of wash buffer, and collection of waste) and large channels, while the top level consists of smaller microfluidic components (for droplet generation and processing). In doing so, we were able to fabricate the bottom level in Zeonor using CNC machining, while the top level was produced using rapid prototyping in poly(dimethyl siloxane) (PDMS).
A second consideration is related to the design and positioning of key microfluidic components to achieve optimal performance. Since the centrifugal force plays a role in the droplet formation process (see section 4.1), the droplet generation structure was placed as far as possible from the rotation center to maximize control over this parameter. Additionally, to achieve higher degree of control over flow rates and the droplet generation process, we included a serpentine channel before the nozzle distributor. This serves to decelerate the flow of the dispersed phase when no pressure is applied, while the desired flow rate can be reached by increasing the pressure applied to reservoir (F) containing the master mix. Finally, as the rotor is stopped successively at predetermined angular positions for the droplet imaging step, the imaging chamber (I) has been positioned within the spatial range of the microscope setup, while ensuring that no other features (e.g., channels or reservoirs) interfere with the readout. This chamber was also designed to distribute droplets in the form of a close-packed monolayer using an implementation strategy that, we believe, is original and unique. The compartment has been divided in two distinct segments: (i) a deep, elongated part at the top for receiving the emulsion from the PCR module, and (ii) a wide, shallow portion (∼50 μm in depth) at the bottom to spread out droplets for imaging (see Fig. S9 and S10 and associated text in the ESI†).
3 Experimental section
3.1 Cartridge fabrication
Large reservoirs and communication microchannels were carved into a block (6 mm in thickness) of Zeonor 1060R (Zeon Chemicals, Louisville, KY) using precision machining (Q350 CNC Mill; Menig Automation, Morgan Hill, CA). The top layer was fabricated in PDMS (Sylgard 184; Dow Corning, Midland, MI) using replica molding. The device was assembled by permanently bonding the Zeonor and PDMS layers through a combination of O2 plasma activation (HI RF power, 900 mTorr for 30 s; Harrick Plasma, Ithaca, NY) and treatment of the Zeonor substrate with a 1% (v/v) solution of (3-aminopropyl)triethoxysilane (APTES; Sigma-Aldrich, Oakville, ON) for 15 min.30 Subsequently, the Zeonor substrate was rinsed with water, dried with a stream of nitrogen gas and put in contact with the activated PDMS surface. Finally, a thin flat PDMS substrate was placed on the top layer to close the chambers and channels. Sealing was achieved using O2 plasma activation (30 s), allowing irreversible PDMS bonding.31 Interconnects between the features on the top and the bottom layer were implemented using through-hole vias that were punched manually. An all-thermoplastic fabrication method was also developed (see Fig. S11 and associated text in the ESI†).
3.2 Viral RNA extraction
Viral RNA was extracted from 100 μL sample volumes deriving from remnant COVID-positive or COVID-negative NPS specimens (iSpecimen, Lexington, MA). For analytical performance assessment, VTM (Fisher Scientific, Waltham, MA) was spiked with SARS-CoV-2 synthetic RNA (Twist Bioscience, South San Francisco, CA). RNAdvance Viral kit (Beckman Coulter, Mississauga, ON) was used following manufacturer's recommendations. NPS samples were placed in tubes containing lysis buffer and incubated at room temperature for 10 min. Lysate was then combined with binding buffer for manual extraction or loaded onto the cartridge for the automated protocol. The lysis step was omitted for experiments utilizing synthetic SARS-CoV-2 RNA to prevent degradation. For manual extraction in tubes, a DynaMag magnetic rack (Thermo Fisher Scientific) was used to capture magnetic nanoparticles (MNPs). The elution was performed in 25 μL of nuclease-free water (Sigma-Aldrich). On-chip extraction of viral RNA was conducted using the automated protocol with the same reagents and volumes as for manual extraction. For on-chip capture of MNPs, the external magnetic field was provided by a nickel-plated neodymium alloy disk magnet (D201, 1/8′′ in diameter, 1/32′′ in thickness; K&J Magnetics, Pipersville, PA) which remained inserted in the designated area on the cartridge for the entire duration of the workflow. The extracted RNA was subsequently used in downstream RT-qPCR for assessment of RNA extraction efficiency as well as in on-chip ddPCR for determination of viral copy number and assay sensitivity.
3.3 RT-qPCR
Extracted RNA samples were analyzed using multiplexed RT-qPCR with primer–probe sequences for N and E regions of the SARS-CoV-2 genome and RNase P as an internal control for RNA extraction. Primer and probe sequences (Table S3†) were synthesized by Integrated DNA Technologies (Coralville, IA). Each RT-qPCR reaction consisted of 5 μL 4× TaqPath 1-Step Multiplex Master Mix (No ROX) (Thermo Fisher Scientific), 2 μL 10× primer/probe mix (final concentration of 1 μM and 0.5 μM, respectively), 10 μL template, and 3 μL nuclease-free water (Sigma-Aldrich), for a total volume of 20 μL. Samples were tested in duplicate (n = 2). A no-template control (NTC) reaction was included to test for PCR inhibition. Thermal cycling was performed using a CFX96 Touch Real-Time PCR Detection System (Bio-Rad, Hercules, CA). To quantify the copies of SARS-CoV-2 genes, each RT-qPCR run included serial dilutions of synthetic RNA (Twist Bioscience) from 106 to 0.1 copy per μL. Ct values were plotted against RNA copy numbers and linear regression was used to determine standard curves.
3.4 ddPCR
The ddPCR reaction master mix consisted of 15 μL 5× QuantiTect Virus Master Mix (No ROX) (Qiagen, Hilden, Germany), 7.5 μL 100× QuantiTect Virus RT Mix (Qiagen), 7.5 μL HotStar Taq Plus DNA Polymerase (Qiagen), 7.5 μL 10× primer/probe mix (final concentration of 1 μM and 0.5 μM, respectively), and 12.5 μL nuclease-free water (Sigma-Aldrich). Template input consisted of 25 μL eluant from on-chip RNA extraction and comprised samples of isolated synthetic SARS-CoV-2 RNA (MT007544.1; Twist Bioscience) in VTM, isolated viral RNA from NPS samples (iSpecimen), or nuclease-free water for NTC. On-chip ddPCR was performed on the centrifugal platform using the automated protocol. Droplets containing ddPCR reaction master mix with template input were generated on-chip using centrifugal step emulsification in a designated reservoir containing fluorinated carrier oil (5% (v/v) 00-8 FluoroSurfactant in HFE7500; RAN Biotechnologies, Beverly, MA). The emulsion was then transferred to the platform heater. Temperature cycling was as follows: 20 min at 50 °C, 5 min at 95 °C, and 40 cycles of 15 s at 95 °C and 45 s at 60 °C using a ramp rate of ∼0.7 °C s−1. Following thermal cycling, the emulsion was transferred back to the cartridge for fluorescence imaging. The experiments were repeated in triplicate (n = 3).
3.5 Image acquisition and analysis
The centrifugal platform was equipped with a miniature epi-fluorescence imaging module (Etaluma, Carlsbad, CA) mounted inside a custom-built cover lid. An embedded integrated circuit board was used for communication and control of the device. The module comprised multiband-pass optical filters, a 2× objective (Nikon, Melville, NY), an LED ring (NeoPixel; Adafruit, New York, NY) with a custom diffuser for brightfield illumination and a CMOS camera (daA3840-45um; Basler, Ahrensburg, Germany) for image acquisition. The images for each spectral channel, including the white light illumination, were recorded at rest. Four sets of images were taken at different locations on the imaging chamber thus capturing a full field-of-view for analysis. For each sample, 30
000 to 40
000 droplets were analyzed. Custom software implemented in LabView (NI Vision; National Instruments, Austin, TX) was used for droplet image analysis and sample quantification. A mask was generated from the brightfield image and applied to the fluorescence images prior to analysis. Mean fluorescence intensity of droplets was computed for each fluorophore and the values were displayed in a scatter intensity map. The mean droplet intensity threshold was determined based on the ‘definetherain’ algorithm,32,33 classifying the droplets into positive and negative responses. Poisson distribution was used to fit the fraction of positive droplets and obtain the absolute quantity of viral RNA gene targets of the input sample.
4 Results and discussion
4.1 Droplet generation
Step emulsification involves the movement of only one liquid phase (e.g., the aqueous phase), which simplifies design configurations and makes it well-suited for implementation on centrifugal platforms. The mechanism underlying droplet formation is well understood,34,35 and accurate models for predicting flow regimes and droplet size both theoretically36 and empirically37 for different experimental conditions and design parameters have been proposed. The capillary number Ca = ηdud/σ has been identified as a critical parameter for differentiating flow regimes by considering the viscosity of the dispersed phase ηd, the average velocity of the dispersed phase in the nozzle channel ud, and the surface tension σ at the interface between dispersed and continuous phases. A capillary number <0.05 is generally recommended to prevent jetting and mediate steady dripping governed mainly by the geometry of the microfluidic device.34
With respect to this theoretical framework, the microfluidic device we developed for droplet generation is characterized by a capillary number on the order of O(−3), that is one order of magnitude smaller than the critical value recommended for the dripping regime. To confirm the dripping regime experimentally, we recorded droplet formation in real-time using a miniature microscope and a high-speed camera mounted on the rotating stage (Fig. S12†). This allowed visualization of three different stages of the droplet generation process (Fig. 3a), which agree with literature reports.34 In the initial stage, the dispersed phase reaches the nozzle at t = 0 ms and begins forming a disk-shaped tongue, gradually spreading over the terrace by t = 8 ms. Subsequently, in the second stage, as the dispersed phase reaches the edge of the terrace, it initiates droplet formation in the oil reservoir, leading to a break up process around t ≅ 10 ms. Finally, in the third stage, surface tension and buoyancy cause detachment of the droplet from the terrace within the time range t ∈ [10 ms, 42 ms]. The duration of this phase is a critical limiting factor, as the arrival of new droplets at the terrace before the detachment of previous droplets can lead to their collision and an increased likelihood of merging. The following experimental considerations address this fundamental limitation.
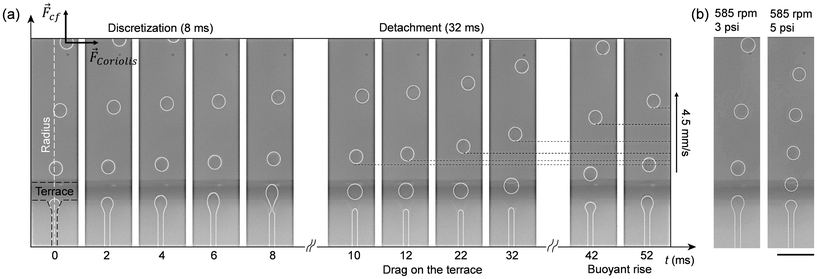 |
| Fig. 3 Droplet generation on the centrifugal platform observed in real-time. (a) Timeline of the droplet generation process. cf and Coriolis are the centrifugal and Coriolis forces, respectively, acting on the droplets. (b) Droplet generation at lower and higher flow rate. Video clips are available in the ESI.† The scale bar denotes 100 μm. | |
By using active pneumatic pumping,22 it is possible to decouple the flow rate of the dispersed phase through the nozzles from the buoyancy force acting on the droplets in the continuous phase by independently controlling the applied pressure and the rotation speed. To highlight this key feature of our platform, we present images of the droplet generation processes (Fig. 3b) for two applied pressures (e.g., 3 and 5 psi) at a constant rotation speed (e.g., 585 rpm). While the size of the droplets is only marginally affected by an increased flow rate (e.g., ∼10% difference in the droplet diameter), a smaller spacing (e.g., on the order of one droplet diameter) is observed in high pressure regimes. Consequently, the increase of the droplet generation frequency due to a higher pressure-induced flow rate should always be accompanied by an increase in the rotation frequency to accelerate the drag on the terrace and the buoyancy rise of the droplets in the reservoir. Once these parameters are set, a larger number of nozzles can be employed in parallel to further increase droplet generation frequencies. In the present work, we use an 8-nozzle droplet generator operated at a flow rate of 1 μL min−1 for a rotation speed of 500 rpm and a pneumatic air pressure of 3.5 psi, a parameter space that represents a good compromise between the size distribution of the droplets and the overall experiment time. These settings provide a maximum frequency at one nozzle of ∼25 Hz, corresponding to an overall frequency of 200 Hz for 8 nozzles working in parallel.
4.2 Sample-to-answer workflow
The workflow conducted on the cartridge is described in detail in the ESI† (Fig. S13 and associated text). It comprises a timed sequence of spinning and pressure actuation steps performed by the platform to displace fluids according to the requirements of the assay. Table S4,† in addition, details operational parameters used to execute the protocol.
4.3 Analytical performance
We determined the limit of detection (LOD) achievable with the centrifugal ddPCR assay using serial dilutions of synthetic SARS-CoV-2 RNA in VTM at 0, 0.1, 1, 10, 100, and 1000 copies μL−1. Image acquisition at the end of the process yields both optical and fluorescence micrographs of droplet monolayers as depicted in Fig. 4a, S14 and S15.† The analysis software uses the brightfield image to create a mask that identifies accepted droplets based on their type (dark or light object selection), size and morphology. This mask excludes droplets that have merged during thermal cycling and any artifacts present on the substrate. The generated mask is automatically applied by the software to the fluorescence images to plot the intensity map, apply the threshold for each fluorophore (Fig. 4b) and measure input sample copy numbers for each concentration. The linear regression curve (Fig. 4c) was produced by plotting copy number concentrations measured by ddPCR against input values of serially diluted synthetic SARS-CoV-2 RNA. The automated ddPCR assay showed excellent linearity for both N and E gene regions of SARS-CoV-2 RNA between the target input amounts and measured values in the dynamic range of five orders of magnitude, slightly better than what we determined for manual RNA extraction and downstream RT-qPCR (Fig. S16a†). The estimated copy number using ddPCR correlated well with the RT-qPCR Ct values (Fig. S16b†) for the range detectable by RT-qPCR (1–1000 copies per μL). However, the sensitivity of the microfluidic ddPCR assay was found to be ∼0.1 copy per μL for both N and E genes, which was more sensitive than the experimentally determined LOD of 1 copy per μL for RT-qPCR.
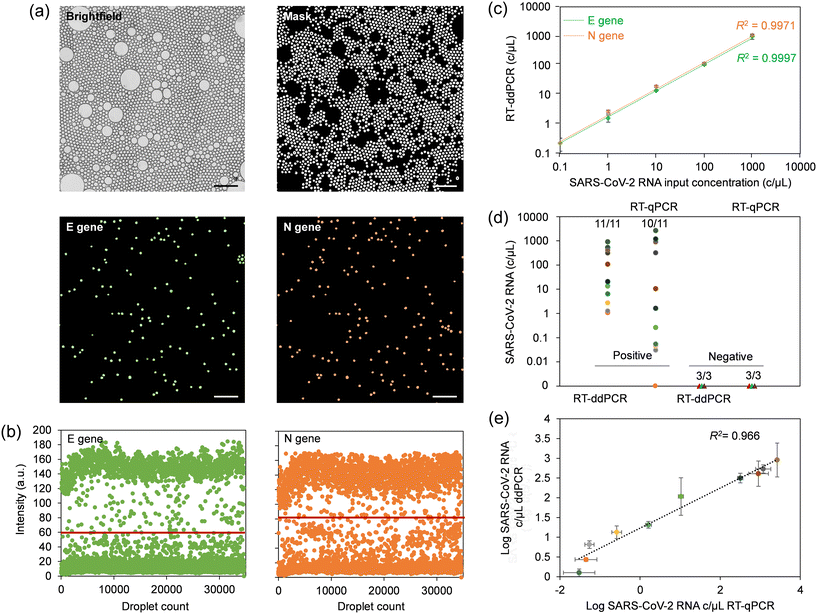 |
| Fig. 4 Assessment of analytical performance using the sample-to-answer microfluidic ddPCR platform. (a) Brightfield and fluorescence images showing a droplet monolayer in the imaging chamber. Here, ddPCR has been conducted using synthetic SARS-CoV-2 RNA diluted in VTM at a concentration of 1000 copies per μL. Only a zoomed-in portion of the imaging chamber is shown to increase visibility of droplets. The scale bars in the images are 400 μm. (b) Intensity maps for FAM and ROX fluorophores, representing E and N genes, respectively. Horizontal lines denote the threshold for positive and negative counts. (c) Standard curve constructed using different input concentrations of synthetic SARS-CoV-2 RNA. (d) Validation of the ddPCR platform using 14 clinical COVID-19 NPS samples benchmarked against RT-qPCR. (e) Correlation of viral load estimates for patient samples detected as positive (P2–P11) using centrifugal ddPCR and RT-qPCR. | |
It is noteworthy that the sensitivity of the target amplification reaction greatly relies on efficient extraction and purification of viral RNA. To optimize yield, we used commercially available viral RNA extraction reagents which allowed us to alleviate the need for two separate wash buffers and thus implement a single wash chamber in the design. This approach resulted in more efficient magnetic extraction while minimizing the footprint required on the cartridge for this step. A comparative RNA extraction test with a manual assay resulted in an extraction efficiency close to 100% for both N and E gene regions using this device configuration (Fig. S16c†). This test also confirmed largely similar extraction efficiencies for manual and on-chip methods.
4.4 Assay validation using clinical samples
NPS specimens comprising 11 COVID-positive and three COVID-negative patient samples were used to validate the performance of the microfluidic platform, which we benchmarked against a commercial benchtop RT-qPCR assay. The multiplex benchtop RT-qPCR assay for N and E genes was preceded by manual RNA extraction for which the RNase P gene was included as an endogenous control. Standard curves for estimating viral load were produced by performing RT-qPCR with serial dilutions of synthetic SARS-CoV-2 RNA in nuclease-free water. The standard curves exhibited a slope between −3.6 and −3.3, indicating efficiency of RT-qPCR reactions between 90 and 100%. Interception with Ct values was in the range of 36–39, suggesting high reproducibility between runs.38 RT-qPCR Ct values for the cohort (Table S5†) were cross-referenced with those provided by the supplier. The data suggests that the primer/probe set for the N gene had higher sensitivity, and was able to correctly identify 10 out of 11 positive patient samples, compared to 6 out of 11 for the E gene. Thus, only the N gene was subsequently considered for comparison with ddPCR. Negative patient swabs showed only amplification for RNase P, with no amplification for N and E genes (for up to 40 amplification cycles), confirming the absence of SARS-CoV-2 RNA. A direct comparison of the SARS-CoV-2 diagnostic test results obtained using RT-qPCR and microfluidic sample-to-answer ddPCR is shown in Fig. 4d. The microfluidic assay was able to correctly identify all 11 positive COVID-19 samples, while all the 3 negative samples were correctly identified as negative. The validity of the RNA extraction and resulting quality of eluted RNA for both, the manually performed RT-qPCR assay and automated sample-to-answer ddPCR were validated using RNase P control gene (Fig. S16d†). A correlogram for samples identified as positives using both RT-qPCR and ddPCR (Fig. 4e) suggests comparable results when viral loads are elevated. Conversely, ddPCR provided higher precision in quantifying patient samples with low viral load, whereas RT-qPCR severely underestimated these values. This observation agrees with literature reports noting shortcomings of qPCR in relation to multiplexed amplification of gene targets at low copy numbers.39–41
5 Conclusion
In this work, we describe an integrated ddPCR system that makes use of a polymer-based microfluidic cartridge and a centrifugal platform with active pneumatic pumping to conduct a sample-to-answer assay for the quantification of SARS-CoV-2 from NPS specimens. The analytical workflow comprises on-chip viral lysis, RNA extraction, emulsification, amplification of target-specific gene markers, and read-out of droplets using an embedded epi-fluorescence imaging module. Displacement of fluids within the integrated system is performed using a pre-determined sequence of spinning and pneumatic actuation steps executed by the platform in a fully automated fashion. As such, the ddPCR system requires only minimal user intervention, limited to the onboarding of sample and assay components, and securing the cartridge on the rotating stage of the platform. The relatively high level of integration shown here minimizes the need for off-chip sample preparation, which constitutes an advantage over other centrifugal technologies used to conduct digital assays (Table S6†). Also, the system is easy to use, thus promoting operation by non-specialized personnel and potential deployment in areas where laboratory-grade infrastructure is not available.
In comparison to conventional RT-qPCR, the sample-to-answer ddPCR system presented here provides several practical and analytical advantages. A significant limitation of RT-qPCR is that it requires a calibration curve for absolute quantification. This process is often laborious and time-consuming, which can increase the overall cost of the assay. The massive partitioning afforded by ddPCR makes calibration obsolete as quantification is derived directly from the number of positive droplets. With an LOD of 0.1 copy per μL, the ddPCR assay investigated here also exhibits higher sensitivity than RT-qPCR, which is consistent with literature reports.42 Partitioning reactions into sub-nanoliter droplets reduces interference from PCR inhibitors that may be present in the eluted sample. The ddPCR technique therefore is advantageous for the detection and quantification of SARS-CoV-2 at the onset of an infection when the viral load is low, which enables timely implementation of appropriate mitigation efforts to prevent further spread of the disease. Coupling image acquisition by the platform with machine learning algorithms constitutes a powerful solution to extract clinically relevant information more efficiently from ddPCR assays43,44—an option that could be envisaged as future development with this technology.
Data availability
The authors confirm that the data supporting the findings of this study are available within the paper and its ESI† files. Should any raw data files be needed they are available from the corresponding author, L. M., upon reasonable request.
Author contributions
L. M. and L. C. conceptualized the work. L. C. created the cartridge design with input from D. B. T. V. and D. C. acquired funding. L. M., T. V. and D. C. supervised the project. L. L. fabricated microfluidic cartridges used in analytical performance testing. K. M. developed the fabrication method for all-thermoplastic microfluidic cartridges. L. M., L. C. and B.-U. M. performed microfluidic testing and validation. C. N. and D. D. F. conducted analytical assays. L. M. analyzed the data. L. M., L. C. and M. G. wrote the manuscript with input from all authors.
Conflicts of interest
There are no conflicts to declare.
Acknowledgements
This work was supported by the Canadian Space Agency and the National Research Council of Canada through the joint initiative “Viral Molecular Identification System (ViMIS)” within the Space Analytics Program. We thank our colleagues Harold Hébert, Alex Ko, Andrew Risdale, Lucas Poncelet, Caroline-Miville Godin, François Normandin, Aaron Bessoff, Émilie Leblanc Gaudreau and Simon Geissbuehler for their help with instrumentation, CNC machining and software implementation. We are grateful to Amaya Arcelus for her support.
References
- D. M. Morens, G. K. Folkers and A. S. Fauci, Nature, 2004, 430, 242–249 CrossRef CAS PubMed.
- R. E. Baker, A. S. Mahmud, I. F. Miller, M. Rajeev, F. Rasambainarivo, B. L. Rice, S. Takahashi, A. J. Tatem, C. E. Wagner, L. F. Wang, A. Wesolowski and C. J. E. Metcalf, Nat. Rev. Microbiol., 2022, 20, 193–205 CrossRef CAS PubMed.
- B. Hu, H. Guo, P. Zhou and Z. L. Shi, Nat. Rev. Microbiol., 2021, 19, 141–154 CrossRef CAS PubMed.
- F. Yu, L. Yan, N. Wang, S. Yang, L. Wang, Y. Tang, G. Gao, S. Wang, C. Ma, R. Xie, F. Wang, C. Tan, L. Zhu, Y. Guo and F. Zhang, Clin. Infect. Dis., 2020, 71, 793–798 CrossRef CAS PubMed.
- J. Fajnzylber, J. Regan, K. Coxen, H. Corry, C. Wong, A. Rosenthal, D. Worrall, F. Giguel, A. Piechocka-Trocha, C. Atyeo, S. Fischinger, A. Chan, K. T. Flaherty, K. Hall, M. Dougan, E. T. Ryan, E. Gillespie, R. Chishti, Y. Li, N. Jilg, D. Hanidziar, R. M. Baron, L. Baden, A. M. Tsibris, K. A. Armstrong, D. R. Kuritzkes, G. Alter, B. D. Walker, X. Yu and J. Z. Li, et al., Nat. Commun., 2020, 11, 5493 CrossRef CAS PubMed.
- U.S. National Center for Immunization and Respiratory Diseases (NCIRD) & Department of Veterinary Disease Research and Development Branch, Real-Time RT-PCR Panel for Detection, 2019-Novel Coronavirus, Centers for Disease Control and Prevention, Atlanta, GA, 2020, https://stacks.cdc.gov/view/cdc/84526/cdc_84526_DS1.pdf, (accessed December 29, 2023) Search PubMed.
- V. Pecoraro, A. Negro, T. Pirotti and T. Trenti, Eur. J. Clin. Invest., 2022, 52, e13706 CrossRef CAS PubMed.
- T. G. W. Graham, C. Dugast-Darzacq, G. M. Dailey, X. H. Nguyenla, E. Van Dis, M. N. Esbin, A. Abidi, S. A. Stanley, X. Darzacq and R. Tjian, PLoS One, 2021, 16, e0246647 CrossRef CAS PubMed.
- S. C. Taylor, G. Laperriere and H. Germain, Sci. Rep., 2017, 7, 2409 CrossRef PubMed.
- C. Schrader, A. Schielke, L. Ellerbroek and R. Johne, J. Appl. Microbiol., 2012, 113, 1014–1026 CrossRef CAS PubMed.
- B. J. Hindson, K. D. Ness, D. A. Masquelier, P. Belgrader, N. J. Heredia, A. J. Makarewicz, I. J. Bright, M. Y. Lucero, A. L. Hiddessen, T. C. Legler, T. K. Kitano, M. R. Hodel, J. F. Petersen, P. W. Wyatt, E. R. Steenblock, P. H. Shah, L. J. Bousse, C. B. Troup, J. C. Mellen, D. K. Wittmann, N. G. Erndt, T. H. Cauley, R. T. Koehler, A. P. So, S. Dube, K. A. Rose, L. Montesclaros, S. Wang, D. P. Stumbo, S. P. Hodges, S. Romine, F. P. Milanovich, H. E. White, J. F. Regan, G. A. Karlin-Neumann, C. M. Hindson, S. Saxonov and B. W. Colston, Anal. Chem., 2011, 83, 8604–8610 CrossRef CAS PubMed.
- H. N. Vasudevan, P. Xu, V. Servellita, S. Miller, L. Liu, A. Gopez, C. Y. Chiu and A. R. Abate, Sci. Rep., 2021, 11, 780 CrossRef CAS PubMed.
- A. Marchio, C. Batejat, J. Vanhomwegen, M. Feher, Q. Grassin, M. Chazal, O. Raulin, A. Farges-Berth, F. Reibel, V. Esteve, A. Dejean, N. Jouvenet, J. C. Manuguerra and P. Pineau, Arch. Virol., 2021, 166, 2529–2540 CrossRef CAS PubMed.
- J. Azimi-Boulali, M. Madadelahi, M. J. Madou and S. O. Martinez-Chapa, Micromachines, 2020, 11, 603 CrossRef PubMed.
- F. Schuler, F. Schwemmer, M. Trotter, S. Wadle, R. Zengerle, F. von Stetten and N. Paust, Lab Chip, 2015, 15, 2759–2766 RSC.
- F. Schuler, M. Trotter, M. Geltman, F. Schwemmer, S. Wadle, E. Domínguez-Garrido, M. López, C. Cervera-Acedo, P. Santibáñez, F. von Stetten, R. Zengerle and N. Paust, Lab Chip, 2016, 16, 208–216 RSC.
- X. Li, D. Zhang, W. Ruan, W. Liu, K. Yin, T. Tian, Y. Bi, Q. Ruan, Y. Zhao, Z. Zhu and C. Yang, Anal. Chem., 2019, 91, 13611–13619 CrossRef CAS PubMed.
- H. Peng, M. Zhu, Z. Gao, C. Liao, C. Jia, H. Wang, H. Zhou and J. Zhao, Biomed. Microdevices, 2020, 22, 18 CrossRef PubMed.
- Y. Wang, S. Liu, T. Zhang, H. Cong, Y. Wei, J. Xu, Y.-P. Ho, S.-K. Kong and H.-P. Ho, Lab Chip, 2019, 19, 3870–3879 RSC.
- F. Schlenker, E. Kipf, N. Borst, N. Paust, R. Zengerle, F. von Stetten, P. Juelg and T. Hutzenlaub, Processes, 2021, 9, 97 CrossRef CAS.
- M. Schulz, J. Ruediger, E. Landmann, M. Bakheit, S. Frischmann, D. Rassler, A. R. Homann, F. von Stetten, R. Zengerle and N. Paust, Anal. Chem., 2021, 93, 2854–2860 CrossRef CAS PubMed.
- L. Clime, D. Brassard, M. Geissler and T. Veres, Lab Chip, 2015, 15, 2400–2411 RSC.
- D. Brassard, M. Geissler, M. Descarreaux, D. Tremblay, J. Daoud, L. Clime, M. Mounier, D. Charlebois and T. Veres, Lab Chip, 2019, 19, 1941–1952 RSC.
- M. Geissler, D. Brassard, L. Clime, A. V. C. Pilar, L. Malic, J. Daoud, V. Barrère, C. Luebbert, B. W. Blais, N. Corneau and T. Veres, Analyst, 2020, 145, 6831–6845 RSC.
- L. Malic, D. Brassard, D. Da Fonte, C. Nassif, M. Mounier, A. Ponton, M. Geissler, M. Shiu, K. J. Morton and T. Veres, Lab Chip, 2022, 22, 3157–3171 RSC.
- M. Geissler, D. Brassard, N. Adam, N. Nasheri, A. V. C. Pilar, K. Tapp, L. Clime, C. Miville-Godin, M. Mounier, C. Nassif, L. Lukic, L. Malic, N. Corneau and T. Veres, Lab Chip, 2024, 24, 668–679 RSC.
- L. Clime, D. Brassard, J. Daoud, C. Miville-Godin and T. Veres, Proceedings of 20th International Conference on Miniaturized Systems for Chemistry and Life Sciences, Dublin, Ireland, 2016 Search PubMed.
- D. Brassard, J. Daoud, L. Clime, M. Geissler, L. Malic, D. Charlebois and T. Veres, Proceedings of 23rd International Conference on Miniaturized Systems for Chemistry and Life Sciences, Basel, Switzerland, 2019 Search PubMed.
- L. Clime, J. Daoud, D. Brassard, L. Malic, M. Geissler and T. Veres, Microfluid. Nanofluid., 2019, 23, 29 CrossRef.
- V. Sunkara, D.-K. Park, H. Hwang, R. Chantiwas, S. A. Soper and Y.-K. Cho, Lab Chip, 2011, 11, 962–965 RSC.
- A. Borók, K. Laboda and A. Bonyár, Biosensors, 2021, 11, 292 CrossRef PubMed.
- M. Jones, J. Williams, K. Gärtner, R. Phillips, J. Hurst and J. Frater, J. Virol. Methods, 2014, 202, 46–53 CrossRef CAS PubMed.
- L. Malic, J. Daoud, M. Geissler, A. Boutin, L. Lukic, M. Janta, A. Elmanzalawy and T. Veres, Analyst, 2019, 144, 6541–6553 RSC.
- M. Schulz, F. von Stetten, R. Zengerle and N. Paust, Langmuir, 2019, 35, 9809–9815 CrossRef CAS PubMed.
- P. Zhu and L. Wang, Lab Chip, 2017, 17, 34–75 RSC.
- W. Zhou, K. Jiang, T. Zhang, C. Liu, H. Li, Z. Zhang and Y. Tang, Phys. Fluids, 2022, 34, 122069 Search PubMed.
- L. Clime, L. Malic, J. Daoud, L. Lukic, M. Geissler and T. Veres, Lab Chip, 2020, 20, 3091–3095 RSC.
- P. S. Adams, Data analysis and reporting, in Real-Time PCR, ed. T. Dorak, Taylor & Francis, New York, NY, 2007, pp. 65–88 Search PubMed.
- J. F. Huggett and A. Whale, Clin. Chem., 2013, 59, 1691–1693 CrossRef CAS PubMed.
- C. Liu, Q. Shi, M. Peng, R. Lu, H. Li, Y. Cai, J. Chen, J. Xu and B. Shen, Aging, 2020, 12, 20997–21003 CrossRef CAS PubMed.
- R. Nyaruaba, C. Mwaliko, D. Dobnik, P. Neužil, P. Amoth, M. Mwau, J. Yu, H. Yang and H. Wei, Clin. Microbiol. Rev., 2022, 35, e0016821 CrossRef PubMed.
- R. Hayden, Z. Gu, J. Ingersoll, D. Abdul-Ali, L. Shi, S. Pounds and A. Caliendo, J. Clin. Microbiol., 2013, 51, 540–546 CrossRef CAS PubMed.
- C. Liu, B. Li, H. Lin, C. Yang, J. Guo, B. Cui, W. Pan, J. Feng, T. Luo, F. Chu, X. Xu, L. Zheng and S. Yao, Biosens. Bioelectron., 2021, 194, 113615 CrossRef CAS PubMed.
- Y. S. Lee, J. W. Choi, T. Kang and B. G. Chung, BioChip J., 2023, 17, 112–119 CrossRef CAS PubMed.
|
This journal is © The Royal Society of Chemistry 2024 |