Quantitative structure–activity relationships for primary aerobic biodegradation of organic chemicals in pristine surface waters: starting points for predicting biodegradation under acclimatization†
Received
13th August 2017
, Accepted 7th November 2017
First published on 14th November 2017
Abstract
Microbial biomass and acclimation can affect the removal of organic chemicals in natural surface waters. In order to account for these effects and develop more robust models for biodegradation, we have compiled and curated removal data for un-acclimated (pristine) surface waters on which we developed quantitative structure–activity relationships (QSARs). Global analysis of the very heterogeneous dataset including neutral, anionic, cationic and zwitterionic chemicals (N = 233) using a random forest algorithm showed that useful predictions were possible (Qext2 = 0.4–0.5) though relatively large standard errors were associated (SDEP ∼0.7). Classification of the chemicals based on speciation state and metabolic pathway showed that biodegradation is influenced by the two, and that the dependence of biodegradation on chemical characteristics is non-linear. Class-specific QSAR analysis indicated that shape and charge distribution determine the biodegradation of neutral chemicals (R2 ∼ 0.6), e.g. through membrane permeation or binding to P450 enzymes, whereas the average biodegradation of charged chemicals is 1 to 2 orders of magnitude lower, for which degradation depends more directly on cellular uptake (R2 ∼ 0.6). Further analysis showed that specific chemical classes such as peptides and organic halogens are relatively less biodegradable in pristine surface waters, resulting in the need for the microbial consortia to acclimate. Additional literature data was used to verify an acclimation model (based on Monod-type kinetics) capable of extrapolating QSAR predictions to acclimating conditions such as in water treatment, downstream lakes and large rivers under μg L−1 to mg L−1 concentrations. The framework developed, despite being based on multiple assumptions, is promising and needs further validation using experimentation with more standardised and homogenised conditions as well as adequate characterization of the inoculum used.
Environmental significance
In this study quantitative structure–property relationship (QSAR) models were developed capable of predicting aerobic biodegradation of both neutral and charged chemicals. The developed models, in combination with Monod-type acclimation kinetics, can be used to predict biodegradation in various surface waters. The methods developed in our study are the first of its kind that allow for robust predictions that were not possible previously.
|
1. Introduction
The detection and monitoring of micropollutants (MP) in surface and wastewaters has increased in the last decade and levels have been detected posing a threat to aquatic organisms.1,2 Biodegradation is an important process for the fate of micropollutants (MPs) in the aquatic environment, involving e.g. hydrolysis, oxidation, and reduction of the chemical structure to ultimately produce carbon dioxide and water. For most assessments, the initial biodegradation step is of prime importance (i.e., the removal of the parent chemical from the environment).3,4 However, MP biodegradation is often not considered explicitly in most models of sewer systems and/or recipient water bodies. Instead, often simple heuristic sink terms (i.e. the fraction of MP eliminated) based on loads are used to approximate MP biodegradation.5 In order to predict the contaminant biodegradation in centralised water treatment works, mechanistic approaches are needed to complement conventional, heuristic and other fate models.5 The field of biodegradation modelling has been reviewed repeatedly.6,7 Recent prediction tools for surface waters include the CATALOGIC framework by Dimitrov et al. (2011)8 and the artificial neural network (ANN) by Tamm et al. (2012) both capable of predicting primary transformation of diverse organic pollutants (e.g. aromatic, aliphatic and cyclic amines, ketones, alcohols, esters, etc.).9,10 In turn, QSARs predicting biodegradation in terms of mineralization are also widely available.11,12 In spite of their satisfactory performance, such models often lack detailed descriptions of the data and test media used, limiting their use to generic conditions only.
Factors heavily influencing biodegradation are the pollutant concentration, microbial biomass and enzymatic activity.13–15 Additionally, biodegradation is a complex function of pH, temperature, dissolved oxygen, available nutrients, the presence of other organic chemicals (synthetic or naturally occurring) that may serve as co-metabolites or alternative energy sources, and the populations and types of organisms capable of transforming the chemical.16,17 It has been noted that biodegradation processes are too complex to reliably predict a rate constant using current approaches as available for abiotic processes.7,15,18,19 Nevertheless, it is possible to make generalisations based on the relative importance of the involved factors. For example, at the low concentrations of MPs in the environment (<1 ppm), the rates of biodegradation may be expected to follow second-order kinetics, being first order in both chemical and biomass. Also, microorganism growth due to biodegradation (i.e. consumption) of the chemical may not be significant.20 In most assessments, the microbial inoculum is left uncharacterized, providing only limited insight into pathways of degradation as is the case for a suite of OECD tests.15,21 However, microbial adaptation may significantly enhance their capacity towards biodegrading MPs.22 The assessment of specific microbial strains and enzymatic reactions responsible for MP biodegradation are examples of studies to better assess biodegradation of MPs under different redox and pH conditions, in pristine and acclimated water bodies.5
In order to address the current knowledge gaps, we aimed to develop robust models capable of predicting biodegradation rate constants for a range of surface waters. Acknowledging the importance of biomass and microbial adaptation in surface water and realistic pollutant concentrations, we collected data for the removal of chemicals in ‘relatively pristine’ surface waters as proxies for second-order biodegradation rate constants. Using quantitative structure–activity relationship (QSAR) analysis in combination with enzymatic pathway analysis and chemical speciation we developed methods for prediction of biodegradation rates in un-acclimated surface waters. Subsequently, an acclimation framework based on Monod-type kinetics was used to extrapolate the un-acclimated rate constants to predict biodegradation under acclimatizing conditions.
2. Methods
Data collection and selection
Biodegradation data were obtained from the open literature using Web of Science and Google Scholar search engines. Keywords included biodegradation, biotransformation, microbial, primary, surface water, river, pond, lake and OECD309 (OECD test for aerobic biodegradation in surface water23). Ionizable pharmaceuticals and pesticides were specifically but not exclusively included because they represent an upcoming class of pollutants. Moreover, their hydrophobicity is generally low, thus potentially increasing the chemical space and applicability of the QSARs. Significant data sources are given in ref. 24–27. Endpoints collected were first-order rate constants and half-lives (the majority of the data), second-order rate constants and percentages of removal. Studies were included if biodegradation experiments were conducted using mixed microbial consortia in ‘relatively pristine’ (un-acclimated) aerobic surface waters, i.e. ponds, creeks, lakes, rivers. Importantly, biodegradation data was not to be acquired using media with deliberate previous exposure to the respective chemicals, though there may be background concentrations. Die-away data were only allowed either if direct products were CO2/H2O, or if it can be assumed that the first transformation step is rate limiting (no intermediates, no incomplete mineralisation). Unless mentioned otherwise, only experiments conducted in absence of light were included to exclude the influence of photolysis. Highly hydrophobic chemicals and surfactants were omitted (omitted if log
Kow > 4 as a cut-off which has been used before in hazard classification28) to limit the potential influence of water solubility and sorption on biodegradation29 (groundwater media were included for substances with log
Kow < 1.5). If more data sources were available for the same compound, the orders of preference were: low DOC > high DOC (to limit sorption); ponds > creeks > small lakes > small rivers > large lakes > large rivers; long > short test duration; low compound concentration > high compound concentration (to limit microbial acclimation); 10 °C < temperature < 20 °C, and the inoculum 106 < cells L−1 < 109 (for standardization). If more than one suitable datapoint was available for a compound, the geometric average was used. The final dataset containing 233 unique organic chemicals including their dominant speciation states and other metadata is given in the ESI.†
Derivation of second-order biodegradation rate constants
The widely-used Monod kinetic equation, describing the relation between growth-limiting pollutant (substrate) concentration P (mg L−1) and the specific growth rate μ (h−1) in a well-mixed system with microbial maintenance m and decay (considered negligible), is expressed as:30,31 |  | (1) |
where X (cells L−1) is the biomass per unit volume, μm (h−1) the maximum specific growth rate, and Ks (mg L−1) the concentration of the substrate supporting half-maximum specific growth rate (0.5μm). Conversely, the rate of substrate utilisation equals:20,32 |  | (2) |
where kb is the substrate utilization constant or biodegradation constant (also known as Vmax), equal to μm/Y and Y (cells mg−1) is the biomass produced from a unit amount of substrate consumed. The constants μm, Ks, and Y depend on the characteristics of the microbes and conditions (e.g., pH, concentrations of other nutrients). For many pollutants in the environment, concentrations are very low and biomass X does not change significantly from consumption of the pollutant, such that P ≪ Ks, and eqn (2) can be rewritten:20 | 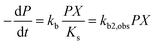 | (3) |
which shows that the biodegradation rate is first-order in biomass and in pollutant concentration, with kb2,obs (L cell−1 h−1) being a second-order rate constant. For many of the biodegradation studies as well as for OECD 309 tests, a realistic concentration of inoculum is e.g. 106–109 cells L−1 in surface water.33 Recommended concentrations for the closed bottle and OECD screening studies are 107–109 and 108, respectively.34 Hence, unless the concentration of the inoculum was reported, half-lives and first-order degradation rate constants were converted to second-order rate constants assuming a default of 108 cells L−1 for X. If half-lives and/or rate constants were not explicitly reported in literature but removal percentages were, the latter were converted to rate constants based on linear extrapolation, i.e. by dividing the fraction removed during the lag phase by the duration of the lag phase (i.e., prior to acclimation). In other cases, no (significant) biodegradation was observed, e.g. because the test duration was relatively short or substances were poorly biodegradable. If no significant biodegradation was observed within the test period or lag phase, the biodegradation half-life was taken as 10-fold the test duration or lag phase, corresponding to an observed half-life of >28 days for ∼30% of the dataset (ESI†).35,36 The observed biodegradation rate constants (kb2,obs) were corrected for sorption to DOC similar to Tebes-Stevens and Jones (2004)29 according to: | 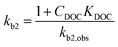 | (4) |
where kb2 (L cell−1 h−1) is the second-order rate constant in the water phase. KDOC was estimated using the relationship KDOC = 0.08KOW.37 If the concentration of DOC (CDOC) was unknown, 7 mg L−1 was used as a default.34 No DOC corrections were applied to esters since abiotic hydrolysis may play a role. In turn, abiotic hydrolysis is influenced (inhibited) by steric substituents, and less by sorption to DOC.
QSAR modelling
Biodegradation rate constants (kb2), in logarithmic scale, were used to build QSAR models describing the association between molecular descriptors representing diverse structural or physicochemical properties of the compounds and the log
kb2. The structures were extracted in SMILES format, representing the main ionisation state expected at experimental pH (most at ∼7.4) and normalised using standardizer38 software. Then, reasonable three-dimensional structures were obtained using the CORINA v3.494 (ref. 39) program. The full dataset was divided randomly into a training series, which contained 70% (163) of the compounds and a test series with the remaining 30% (70). Pentacle,40–42 PaDEL43 and VolSurf44 were used to compute GRIND (‘pharmacophore-like’ MD), PaDEL (‘generic’ MD)35 and VolSurf (‘ADME-type’ MD) molecular descriptors, respectively, based on the chemical structures in the training series. Descriptor selection was automatized using a variable selection algorithm.45 The respective matrices obtained, together with the log
kb2 values, were submitted to two machine learning methods: Partial Least Squares (PLS)46 and Random Forest (RF).47 Both leave-one-out cross validation (LOOCV) and external validation were performed to evaluate the model quality. The best model was selected based on the lowest standard error for external prediction (i.e. the test set, SDEP), see Fig. 1, and descriptor importance was calculated to allow for interpretation.45,48,49 More detailed information on the method and results for all descriptor packages and algorithms is given in the ESI.†
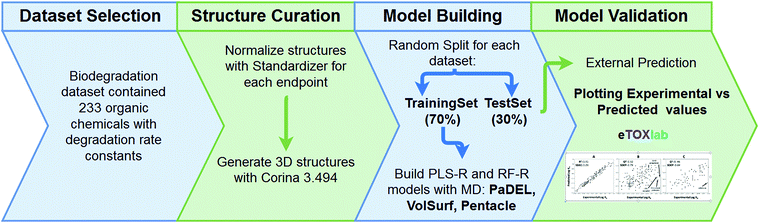 |
| Fig. 1 The modelling workflow used to generate the global biodegradation QSAR. | |
Classification and class-specific QSAR
In order to investigate the influence of the natural enzymatic activity of ‘relatively pristine’ (un-adapted) surface waters, the dataset was split according to the enzymatic family involved in bacterial transformation of the parent compound. However, bacteria have hundreds of enzymes in every living cell, catalysing many reactions. Hydrolases, oxidoreductases and transferases occur frequently, whereas lyases, isomerases and ligases are less abundant.50 Hydrolase enzymes catalyse the breaking of bonds through the addition of water (esterase, peptidases and lipases). Oxidoreductases catalyse redox reactions which are vital for many metabolic processes, particularly in aerobic/anaerobic respiration usually requiring cofactors such as NAD(P)H (e.g. for alcohol dehydrogenase). In turn, transferases catalyse the transfer of functional groups (e.g. phosphate, methyl and glycosyl).50 For the majority of the chemicals in the dataset, the enzymatic families involved in the primary biodegradation step are unknown. For this reason, the primary enzymatic family involved was predicted using the Pathway Prediction System (PPS) of the EAWAG-Biocatalysis/Biodegradation Database (EAWAG-BBD).51 This PPS predicts microbial catabolic reactions using substructure searching, a rule-base, and atom-to-atom mapping. The system is able to recognize organic functional groups and predict transformations based on biotransformation rules. The rules are based on reactions found in the EAWAG-BBD database or in the scientific literature. The same system was recently used for predicting biodegradation in soil.52 Subclasses of enzymes assigned by the EAWAG-BBD PPS were ‘unspecific’ esterase, mono- and dioxygenase, alcohol dehydrogenase, aldehyde dehydrogenase, dehalogenase, nitroreductase, amidohydrolase, amine dehydrogenase, demethylase, epoxide hydrolase, isomerase and hydrolases (i.e. general groups, not classified as a specific category of enzymes). In case multiple enzyme classes were assigned to one compound by the EAWAG-BBD PPS, only the most likely enzyme class was considered, in order of relative likelihood: aldehyde dehydrogenase > monooxygenase > alcohol dehydrogenase > dioxygenase > dehalogenase > amine dehydrogenase/N-demethylase > amidohydrolase > specific hydrolases, as determined from preliminary investigation using the EAWAG-BBD PPS and compounds with only a single enzyme class assigned.
The dataset acquired contained a significant proportion organic chemicals which are largely ionised at experimental pH (N = 97, i.e. >40%). It is known that the persistence of ionizable organic chemicals is probably attributable to the lack of uptake in cells, whereas the presumed electrostatic attraction appeared to have no measurable influence on membrane passage.53–55 For this reason, chemicals were also classified according to the chemical's speciation state (at pH ∼ 7.4 using pKa values from Drugbank or Chemaxon estimation). To investigate further the influence of specific chemical characteristics on the biodegradation rate constant, class-specific QSARs were derived using linear regression and aforementioned descriptors.42–44,56 Subsets were chosen based on dataset size and internal variance (SD ≥ 0.6). The available data permitted QSAR analysis of (1) neutral chemicals transformed via unspecific monooxygenase, and (2) charged chemicals transformed via oxygenase and alcohol/aldehyde dehydrogenase, see Table 1. Correlation-based feature selection57 was used to select statistically relevant descriptors for the two data subsets selected based on enzymatic pathway and speciation. To prevent overfitting, the number of selected descriptors was limited to one per 15 substances in the dataset, i.e. N ≥ 15 per descriptor. QLOOCV2 and rss (root sum of squares) were used to evaluate the class-specific QSARs.
Modelling biodegradation under acclimatizing conditions
Chemical pollutants have been detected in various surface waters and concentrations range from 10−9 to 10−3 g L−1 between sites depending on emissions and fate processes.58 Under such variable concentrations, biodegradation may be affected by adaptation of the microbial consortia towards the pollutant(s). According to Monod kinetics (eqn (1) and (2)), the biomass X and hence enzymatic activity towards a specific transformation process is a function of pollutant concentration, time, biomass and the specific growth rate of the native microbial consortium utilising the chemical pollutant. For low pollutant and DOC concentrations, the growth in biomass is negligible so that we can write: |  | (5) |
for which kmax is the rate constant expressing the maximum enzymatic activity, taken as 10−9 (L per cell per h) (see Table 1). kmax is to be normalised for the microbial activity towards a specific pollutant (Ap) relative to the microbial activity due to the presence of organic nutrients (An) as Ap/(Ap + An) with Ap ≤ An. We anticipate that Ap can vary as a function of time (t) and assume that the enzymatic activity towards the pollutant is equivalent to its concentration, i.e. Ap(t) ∼ P(t). Also, we assume that An ∼ Pn, being the concentration of organic nutrients (Pn, set to a value of 7 mg L−1) to reflect the concentration of dissolved organic carbon (CDOC) potentially consumable by bacteria.59 We thereby write: |  | (6) |
If we further assume the change in pollutant concentration is negligible compared to the change in the rate constant dP/dt ≪ kb2(t)X, with X the biomass, which is a good approximation for the acclimation phase, and Pn is constant, eqn (6) retakes the form of
for which the general solution (to eqn (6)) is:
|  | (7) |
For high kb2,t=t0 and Pt=t0, we return to pseudo first-order kinetics. The validity of this relationship was tested using observed kinetic biodegradation data for primary removal of several organic chemicals in surface waters with different levels of adaptation.60–64kb2,t=t0 was set to represent the QSAR-derived initial un-acclimated rate constant, and the specific growth rate μ was used as a fitting parameter. Eqn (7) is able to incorporate the influence of co-metabolism since it includes terms for both the chemical pollutant (through Pt=t and kb2,t=t0) and other available organic nutrients (through Pn). However, in order to more accurately address co-metabolism further distinction of Pn (and An) into separate terms for different types of organic nutrients or microorganisms may be needed.65
In many polluted surface waters, the concentration of the chemical pollutant is often relatively constant66,67 and the bacterial consortia have sufficient time to acclimate68 (see also the data sources in the ESI†). Assuming short acclimation times as well as the sole dependence of enzymatic activity on the pollutant concentration, the second order biodegradation rate constant is linear in P:
| 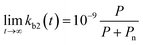 | (8) |
In order to investigate whether the limiting rate constant could be proportional to concentration, we collected removal data for three chemicals from Dutch wastewater treatment plants.69 Measurements in which a concentration of zero was reported in either the influent or effluent were omitted because of potential involvement of measurement uncertainty at the limit of detection. Removal was related to the pollutant concentration assuming the rate of biodegradation is first-order in biomass (taken to be ∼1 × 1010 cells L−1 in activated sludge).
3. Results and discussion
Global QSAR
The total dataset contained 233 organic chemicals, with degradation rate constants generally ranging from 10−9 to 10−12 L cell−1 h−1. The global QSARs performed reasonably, with goodness-of-fit statistics up to 0.35 (Table S1†), and giving Q2 up to 0.46 for the external prediction (Fig. 2C). For full results see ESI (Table S1†). The RF algorithm in combination with ‘generic’ PaDEL descriptors43 performed best based on external prediction. Among the outliers were 2,4-D propylester, di-n-propylphthalate and 17b-estradiol (Fig. 2B) for which the experimental biodegradation was higher than expected. For these compounds, the inaccurate prediction may be due to abiotic hydrolysis (an ester group being hydrolyzed by water, instead of esterase enzymes) or the presence of specific metabolic pathways in surface water. The results of the importance analysis for the RF model show that PaDEL descriptors characterizing molecular topology/accessibility, the presence of nitrogen and other heteroatoms, and energetic features were relatively important (see ESI† for details).
 |
| Fig. 2 Global biodegradation QSAR built using random forest method and PaDEL descriptors. Predicted and experimental values are represented on the X and Y axis, respectively. (A) Calculated values versus experimental values for the training set. (B) Internal prediction values were shown using LOO cross-validation. (C) External prediction for the test series. Four ‘outliers’ are shown, i.e. 2-4-D propylester, 17b-estradiol, di-n-propyl phthalate and cellobiose. | |
Classification
Prediction of the enzymatic class involved in the primary biodegradation resulted in distinct subsets of data with varying rate constants. The observation that biodegradation is class-specific (Table 1) is in line with the finding that the RF allows for better predictions compared to the PLS algorithm (Table S1;† see previous section). Compared to PLS, classification and RF are better able to capture the nonlinearities resulting from the presence/absence of certain functional groups and connectivity within the molecule (Table S1†).70 As compared to the RF method used (i.e. an ensemble of decision trees), group contribution methods (GCM) are based solely on the number and type of molecular groups that form the compound.71 Predicting biodegradation using GCM may falsely identify readily bio-transformable chemical functionalities, whereas steric influences, region-selectivity, or bond polarity can inhibit enzymatic activity.72,73 Biodegradation is likely to depend on functional groups as well as on connectivity within the molecule.51 Hence, in this study, the potential contribution of organic functional groups or enzymatic families was investigated by combining experimental data with expert rules, and atom-to-atom mapping using the EAWAG-BBD PPS51 (e.g. monooxygenation of tert-butanol and 1,4-dioxane).
Table 1 Classification of ‘relatively pristine’ primary second-order biodegradation rate constants (in L per cell per h) according to transformation process51 and speciation state. Colors indicate the median of relative biodegradability: green is ‘readily biodegradable’, i.e. log
kb2 > −10.0, yellow is ‘biodegradable’, i.e. −10.0 > log
kb > −11.0, and red is ‘non-biodegradable’, i.e. (log
kb < −11.0). Data outlined in green and blue denote the class-specific QSARs for non-ionic chemicals transformed via monooxygenase enzyme families (Fig. 3) and various charged chemicals (Fig. 4), respectively
The average biodegradation rate constants for distinct chemical classes transformed by enzyme families are given in Table 1. The influence of speciation is visualized by the far-right columns. Significant differences in biodegradation exist between neutral and charged chemical species. The differences were largest for large neutral and cationic compounds in the unspecific monooxygenase enzyme class (∼102), ESI.† While quantitative estimates of the relative speeds of cell uptake by ionised and non-ionized basic compounds are lacking, studies on acidic moieties show uptake speeds 103–104 lower for the ionic forms relative to the neutral species.53 Uptake studies by eukaryotes (animals and plants) have reported that it is the non-ionized form of the chemicals that show greatest partitioning into cells.53,74 Recently, the extent of bacterial transformation was shown to be generally proportional to the fraction of unionised, neutral species present.54 It may be stated that the observed differences in biodegradation rate constants between neutral and charged species are due to cellular uptake. As far as we know, there are no studies on the relative importance of transport versus enzymatic transformation beyond a few substances. From an evolutionary point of view, it can be argued that for an “natural” substance, both are optimized towards each other. As the picture may be even more complex for synthetic compounds, we do not beforehand assume one process to dominate degradation. In any case, the upper limit of enzymatic transformation rate is often diffusion-limited, being controlled by interaction of the substrate with either the cell membrane or associated enzyme(s).75 The relative differences in biodegradation rates between enzymatic classes strongly indicates that of all the possibly involved enzymes, it is the non-specific biodegradation of the neutral or weakly ionised compound fraction that is most likely to occur in surface waters, irrespective of cell type. For compounds with a pKa/pKb close to the experimental pH, the observed biodegradation rate may be determinable via the Henderson–Hasselbalch equation.
Also noticeable is the influence of the enzymatic family associated with the primary biodegradation step, bottom-four rows in Table 1. Non-ionic chemicals classified as bio-transformed via unspecific monooxygenases, isomerases as well as alcohol- and aldehyde dehydrogenases showed high rate constants. This is fully attributable to the common presence of enzymes in freshwater ecosystems such as cytochrome P450 monooxygenases for short (<4 C) linear alkyl chains and phenyl rings,76,77 methanol dehydrogenase,78 and formaldehyde dehydrogenase.79,80 Low biodegradation is expected for compounds primarily transformed by unspecific amidohydrolase, amine dehydrogenase and dehalogenase. Such compounds are persistent in ‘relatively pristine’ surface waters which can be attributed to the low natural microbial activity of the associated enzyme classes and the need for adaptation.81,82 Illustratively, although acrylamide was readily degraded in aerobic fresh water by bacteria with a half-life of 55–70 h, it had a significant lag phase of 33–50 h
83 in which amidohydrolase activity was induced. Biodegradation of non-ionic esters may be high due to the abundance of unspecific esterase activity in unpolluted as well as polluted surface water.84 Previously Wolfe et al. (1980) found that for esters, 93% of the variance in the data could be explained by alkaline hydrolysis.85 Thus, the variance in biodegradability of esters may be due to the steric effect of ester sidechains, hindering either hydrolytic enzymes from binding or attack by water directly.73 Aspirin, being negatively charged, has a hydrolysis half-life of ∼40 days at pH ∼ 7 and room temperature.86 Similar to esters, degradation of epoxides in surface water also depends on abiotic hydrolysis. If resistant to abiotic hydrolysis, bacterial uptake and hence biodegradation should depend on hydrophobicity, charge, and size87 as is observed for other chemical classes (see the RF model in Fig. 2, Table 1 and eqn (9)).
Class-specific QSARs
Class-specific QSAR analysis was performed for both charged and neutral organic chemicals. The chemicals used to derive the relationships were selected based on the classification in Table 1. For neutral organic chemicals biotransformed via unspecific monooxygenase, statistically significant descriptors were identified and used to develop a QSAR (R2 = 0.64, eqn (9); Fig. 3). For the multiple linear regression (MLR) model for neutral chemicals, molecular eccentricity (MEcc) and a MOE-type van der Waals surface area descriptor encoding atomic partial charges (PEOE_VSA9) were selected (co-linearity R2 < 0.01), which characterize molecular shape and charge distribution. These descriptors selected possibly represent membrane permeation and substrate binding to active enzymatic sites (e.g. P450); two important processes in biodegradation. One outlier was removed from the dataset, ethinylestradiol, due to its unusually low biodegradation in surface water.88,89 In contrast with 17b-estradiol and estrone, which were not outliers, the ethinyl group in position C17 of the steroid is known to prevent it from normal enzymatic (P450) oxidation in the human liver.90 | log kb2 = 6.31 MEcc + 0.12 PEOE_VSA9 − 16.42 (non-ionics, monooxygen.) N = 33, R2 = 0.64, QLOOCV2 = 0.58, rss = 2.64 | (9) |
 |
| Fig. 3 Class-specific biodegradation QSAR for un-acclimated surface waters for neutral, non-halogenated chemicals. Chemicals included were predicted to be primary bio-transformed via unspecific mono-oxygenase enzyme families, R2 = 0.64; QLOOCV2 = 0.51; N = 33. | |
The geometrical descriptor selected for the model for charged chemicals was the accessible molecular surface area (ASA). In the QSAR developed (Fig. 4), ASA denotes molecular size, while shape and flexibility may also be relevant. Since size is a prime descriptor for this data subset, a power function was used to fit the data.91 The QSAR developed for charged compounds (eqn (10)) indicates that cellular uptake via diffusion though the cellular membrane or pores may be driving biodegradation. Generally, for large, branched and charged molecules, it is more difficult to move across the cellular membrane than it is for small and compact molecules. Moreover, it is often observed that low molecular weight organic acids (LMWOAs) are more readily biodegradable.92–95
| kb2 = 0.0087 ASA3.48 (charged, monooxygen/dioxygen/alcoholdehydr) N = 72, R2 = 0.59, QLOOCV2 = 0.57; rss = 23.17 | (10) |
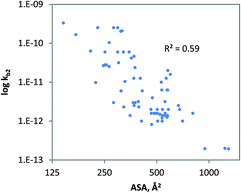 |
| Fig. 4 The relationship between ‘un-acclimated’ biodegradation of charged organic chemicals and the accessible molecular surface area, R2 = 0.59; QLOOCV2 = 0.57; N = 72. Chemicals were included if predicted to be bio-transformed via monooxygenase, dioxygenase and alcohol dehydrogenase enzyme families. | |
Analysis showed that the radius of gyration (Rg) was also statistically significant (R2 = 0.57) which supports the relevance of cellular uptake. However, further validation of eqn (10) is needed using additional tests with low pollutant concentrations and longer durations. Also, QSAR statistics may be improved using more intuitive descriptors such as the liposome–water partitioning and diffusion coefficients. From class-specific QSAR analyses it is plausible that the RF model developed (see previous sections) also includes aspects such as molecular branching, mass and steric features.48,96 Comparison of rate constants between anionic and cationic chemicals suggests that cationic chemicals may be slightly less biodegradable (Table 1), though standard deviations are large. Cations may be less biodegradable due to e.g. ion-trapping effects,97–100 sorption to DOM (both lowering their bioavailability) or antimicrobial activity. The potential relevance of ion-trapping and sorption for biodegradation requires further experimental investigation.
Acclimation
Generally, biodegradation is rapid when adapted bacteria are present.101 Hence, enrichment is a well-known strategy for the development and utilisation of specific bacterial strains capable of biodegrading chemical pollutants. For example, a crude enzyme extract obtained from a mixed microbial culture that was grown on parathion showed exceptionally high activity for phosphatase hydrolysis of at least ten organophosphates.102 In contrast, biodegradation of organophosphates in ‘relatively pristine’ waters is low (Table 1, ESI†), and the general absence of specific phosphatase enzymatic activity in surface waters, as well as potential antibacterial properties of organophosphates, may be the cause. Also, for some halogenated compounds biodegradation must be induced, since the antimicrobial activities of these compounds require that the bacteria adapt. Oxygenase enzymes cannot easily attack halogenated compounds since the aromatic ring is deactivated due to lower electron density103 and the same applies to nitrated compounds. As shown in Table 1, the average biodegradation rate of halogenated compounds was lower than non-halogenated compounds, though significance is low in some cases. Compounds in our dataset with high variance in kb values are 4-chloro-m-cresol (log
kb = −11.5 and −9.9
104,105) and propanil (log
kb = −10.6 and −9.0).106,107 Moreover, lamivudine and emtricitabine (log
kb = −10.1 and −10.7) are both likely biotransformed by unspecific alcohol dehydrogenase activity but differ by one fluorine group.64 In several cases the test media were largely left uncharacterized, hence, the data used to develop the QSAR for un-acclimated biodegradation may represent microbial inoculums which are at least partially acclimated. This may result in overestimations for biodegradation for pristine surface waters and their naive bacterial communities. Apart from the influence of halogenation, variance in biodegradation rates has also been reported for compounds transformed by unspecific dioxygenase and demethylase enzyme classes. For aniline, a ∼10-fold variance in biodegradation rates has been observed using oligotrophic lake, pond and river waters.61,108 Also, adaptation of river water microorganisms to relatively high doses of caffeine81 is likely the principal reason for the extreme variances in observed removal between media.109 Though caffeine removal in wastewater treatment is efficient,110 in pristine waters the compound is relatively inert. In line with the data for caffeine, low removal was also observed for levamisole which likely has a similar biotransformation pathway (via amine dehydrogenase, ESI†). Clearly, bacterial communities in ‘relatively pristine’ surface waters are also capable of adapting to (and expressing enzymatic activity towards) chemical pollutants without selection based on antimicrobial activity.
It is known that under certain conditions, the a priori assumption of first-order kinetics is not valid and a Monod-type approach is more appropriate.111,112 Hence, biodegradation with microbial acclimation was modelled using eqn (7) and appropriate removal data.60–64 Biodegradation in surface water media was modelled for three chemical classes: aniline (neutral), chlorotoluron (chlorinated herbicide) and metoprolol (cationic beta-blocker drug). Fig. 5 shows the concentration changes over time as a function of initial kb (kb,t=t0), μ and P. Using the input parameters, the acclimation model (eqn (7)) shows the lag times for aniline and chlorotoluron of ∼10 and ∼60 days. No lag time was modelled using the data for metoprolol, but the acclimation model gave a better fit than using pseudo first-order kinetics (Fig. 5). Though substrate concentrations used were relatively low, biodegradation is not well described using first-order kinetics (i.e. using only 1 parameter). Instead, the data60,61 could be fitted better using the adaptation model and lag phases can be estimated using two parameters: the initial second order biodegradation rate constant (kb,t=t0) and specific growth rate μ, see Fig. 5. The high value for μ needed to fit the model to the aniline data reflects the abundance of microorganisms (i.e. enzymes) capable of using aniline as the sole source of carbon and nitrogen.113–115 Conversely, the low value of μ for chlorotoluron needed may reflect the antimicrobial properties of the herbicide. Since in ‘relatively pristine’ surface water the biodegradation potential for metoprolol, and cationic organic chemicals in general, is very low (Tables 1 and S2†),116 one might expect to find a lag phase when monitoring the removal of propranolol over time. However, the biodegradation data for metoprolol from63 was obtained using a microcosm with water from an open-water wetland cell receiving wastewater effluent from an adjacent treatment plant. This results in the high initial rate constant used in fitting the acclimation model for the observed removal (Fig. 5) as the microbial consortium is already acclimated to metoprolol or structurally similar pollutants (i.e. co-metabolism). Noticeably, after t ≥ 7 days, the biodegradation rate at 2 μg L−1 is significantly lowered compared to pseudo first-order kinetics, which indicates that the bacterial consortium may be able to re-adapt to ‘pristine’ (i.e. un-acclimated) conditions.
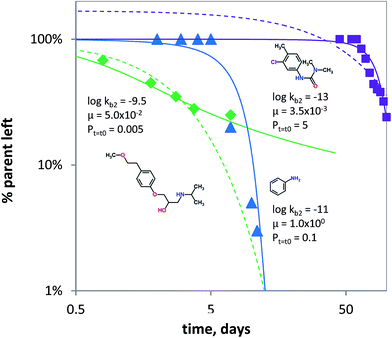 |
| Fig. 5 Biodegradation for metoprolol (green diamonds, Jasper et al. 2014), aniline (blue triangles, Torang 2003), chlorotoluron (purple squares, El-Dib et al. 1998). The solid lines denote biodegradation according to the acclimation kinetics model (eqn (7)). log kb2 in L per cell per h, μ in h−1 and Pt=t0 in mg L−1. The dashed lines denote biodegradation fits according to pseudo first-order kinetics. | |
‘Steady state’ acclimation and removal in wastewater
Removal of chemical pollutants during wastewater treatment was modelled as a function of concentration using the linear ‘steady state’ acclimation model (eqn (8)). For naphthalene and metformin useful predictions can be achieved as shown by the dependence of removal on pollutant concentration, explaining more than a third of the variation in the data for metformin and naphthalene (Fig. 6). Naphthalene is likely primarily bio-transformed due to unspecific dioxygenase activity in pristine surface water, and hence ‘pristine’ log
kb is likely between −10 and −11 L per cell per h (Table 1). Since the chemical carries no charge it can readily be taken up by microorganisms which, depending on the substrate concentration, will express higher unspecific dioxygenase activity. This is visualized in Fig. 6 in which naphthalene shows relatively high removal at relatively low concentrations. In contrast to naphthalene, naproxen (pKa = 4.2) carries a negative charge (∼0.1% neutral and ∼99.9% charged at pH 7.4) and hence is less bioavailable with pristine log
kb likely between −11 and −12 L cell−1 h−1 (Table 1). The high variance in the observed removal of naproxen compared to naphthalene and metformin may be explained by its acidic character in combination with variable medium characteristics like pH and TOC69 which influence sorption–desorption kinetics and bioavailability in the heterogeneous set of WWTPs. For naproxen other removal processes may dominate in WWTPs, like sorption (log
Kd values of 2.16 and 1.38
117) to activated sludge. Variation in biomass between the WWTPs may also significantly affect removal. Though a biomass of 1010 cells L−1 for activated sludge is often used, the actual biomass may differ by an order of magnitude, explaining much of the variance seen in Fig. 6. The cationic metformin (pKa = 12.4) has a neutral fraction of 1 × 10−5 at pH 7.4, making it relatively non-bioavailable. Metformin may be even less biodegradable due to the metabolic pathway required for its degradation (likely N-demethylation) which may need high substrate concentrations to be induced. Though N-demethylase activity can be induced by high influent concentrations of the neutral caffeine118,119 (i.e. potential co-metabolism of caffeine/metformin), bacterial uptake of the cationic metformin may be 103 to 105 times lower than its neutral form.
 |
| Fig. 6 The fraction of chemical pollutants left after biological treatment in wastewater treatment plants (blue triangles: naphthalene, red squares: naproxen, green diamonds: metformin) versus the pollutant concentration. The rate constant as estimated from the ‘steady state’ acclimation model (eqn (8)) has a linear dependence on the pollutant concentration. | |
Limitations and future recommendations
Several important assumptions and limitations have been taken in the presented approach which need to be considered in order to generate reliable estimations. The global QSAR for biodegradation under un-acclimated conditions (Fig. 2) is built on ‘quality’ data since we were able to apply selection criteria (see Methods). However, a significant portion of data ‘meta-data’ was unavailable, e.g. oxygen content, pollutant concentration, microbial biomass, temperature, medium chemistry and nutrients. Removal data from WWTPs69 especially lacked appropriate meta-data which complicates model development. Discrepancies in microbial consortia between e.g. ‘relatively pristine’ lakes and downstream rivers have been shown to heavily affect biodegradation. For the development of more robust biodegradation models, long term test assays are needed that include more extensive characterization of the inoculum used. The incorporation of molecular microbial ecology methods (i.e., ‘omics’) could improve the underlying mechanistic understanding of biodegradation processes, and enable better extrapolation of data from laboratory based test systems to the relevant environment.21 Such an approach may be able to distinguish between the inherent chemical biodegradability, and induced enzymatic activity in surface waters. Importantly, the acclimation kinetics model used does not consider the growth of biomass and influence thereof. The microbial biomass in surface waters may be considered constant with respect to the half-life of readily biodegradable compounds (t1/2 ∼ 1–10 days). However, for more persistent compounds variation in microbial biomass should influence removal in natural waters since biomass may differ as much as an order of magnitude120,121 between winter and summer conditions. The acclimation kinetics model may not be applicable to aquatic systems with low biomass since substrate saturation can play a role. For biodegradation in deep waters, different methods are needed involving an analysis of anaerobically relevant enzyme families. Though the influence of co-metabolism can be accounted for by the acclimation kinetics model, further distinction between different types of pollutants, nutrients and microorganisms may be needed to allow for more accurate and robust prediction. Conversely, biodegradation may be inhibited by specific chemicals, e.g. antimicrobial compounds or heavy metals, which were also not considered in the development of our models. For this purpose, including a an ‘inhibition’ or ‘maintenance’ term in eqn (1) may be needed.
4. Conclusion
Global QSPRs were built capable of producing useful predictions of biodegradation for a wide range of compound classes including ionizable organic chemicals though relatively large standard errors were associated (SDEP ∼0.7). Using class-specific QSAR better statistics were obtained, showing that charged chemicals are inherently less biodegradable, an effect which is more pronounced for ‘high-molecular-weight’ chemicals. The class-specific size-dependence agrees with the widely-known dependence of bilayer permeability and cellular uptake on permeate volume for organic solutes.91 Though, the size dependence can vary significantly depending on charge (distribution),122,123 lipophilicity (in the case of surfactants),124 molecular weight cut-offs,122 saturation kinetics and active uptake processes.125 For neutral chemicals, shape and charge distribution affect the biodegradation, e.g. through membrane permeation or binding to P450 enzyme families. Depending on the (sub)set of chemicals used in the QSAR analysis, different (families of) descriptors were found relevant. This, along with the non-linearity observed, illustrates the large amount of processes that underlie biodegradation in surface waters. Although microbial consortia are able to degrade a wide range of organic pollutants, biodegradation is inhibited in cases in which molecules show more aspects of complexity. For both global and class-specific QSAR analyses, the heterogeneity in available literature ‘meta-data’ contributed to the suboptimal statistics. Likely a significant factor in this was the difference in levels of microbial acclimation in surface waters, which can be accounted for by using Monod-type kinetics. In the risk assessment of chemicals explicit assumptions are often needed. Using several assumptions as well as Monod-type kinetics, the QSAR predictions can be extrapolated to acclimated conditions such as in water treatment, in downstream lakes and large rivers. The framework developed needs further validation using experimentation with standardised and homogenised conditions as well as adequate microbial characterization. Despite these uncertainties and limitations in this study, the presented methodology is the first of its kind that allows for robust predictions of biodegradation for very heterogeneous substances in a variety of surface waters.
Conflicts of interest
There are no conflicts to declare.
Acknowledgements
The research leading to these results has received support from the Innovative Medicines Initiative Joint Undertaking under iPiE grant agreement no. 115735, resources of which are composed of financial contribution from the European Union's Seventh Framework Programme (FP7/2007-2013) and EFPIA companies' in kind contribution.
References
- B. Petrie, R. Barden and B. Kasprzyk-Hordern, A review on emerging contaminants in wastewaters and the environment: Current knowledge, understudied areas and recommendations for future monitoring, Water Res., 2015, 72, 3–27 CrossRef CAS PubMed.
- S. U. Gerbersdorf,
et al., Anthropogenic Trace Compounds (ATCs) in aquatic habitats – Research needs on sources, fate, detection and toxicity to ensure timely elimination strategies and risk management, Environ. Int., 2015, 79, 85–105 CrossRef CAS PubMed.
- D. Dabrowska, A. Kot-Wasik and J. Namiesnik, The importance of degradation in the fate of selected organic compounds in the environment. Part II. Photodegradation and biodegradation, Pol. J. Environ. Stud., 2004, 13(6), 617–626 CAS.
- S. Berkner and C. Thierbach, Biodegradability and transformation of human pharmaceutical active ingredients in environmentally relevant test systems, Environ. Sci. Pollut. Res., 2014, 21(16), 9461–9467 CrossRef CAS PubMed.
- B. G. Plosz,
et al., Modelling micro-pollutant fate in wastewater collection and treatment systems: status and challenges, Water Sci. Technol., 2013, 67(1), 1–15 CAS.
-
A. Sabljic and Y. Nakagawa, Biodegradation and Quantitative Structure–Activity Relationship (QSAR), in Non-First Order Degradation and Time-Dependent Sorption of Organic Chemicals in Soil, American Chemical Society, 2014, pp. 57–84 Search PubMed.
- T. M. Nolte and A. M. J. Ragas, A review of quantitative structure–property relationships for the fate of ionizable organic chemicals in water matrices and identification of knowledge gaps, Environ. Sci.: Processes Impacts, 2017, 19(3), 221–246 CAS.
- S. Dimitrov,
et al., Simulation of chemical metabolism for fate and hazard assessment. II CATALOGIC simulation of abiotic and microbial degradation, SAR QSAR Environ. Res., 2011, 22(7–8), 719–755 CrossRef CAS PubMed.
- V. Ruusmann, S. Sild and U. Maran, QSAR DataBank repository: open and linked qualitative and quantitative structure–activity relationship models, J. Cheminf., 2015, 7, 32 CAS.
-
T. Tamm, G. Karelson, D. Dobchev, K. Tämm, J. Jänes and M. Karelson, QSARDB entry in QMRF format, QSAR ANN model for Persistence: Biotic degradation in water, 2012 Search PubMed.
- S. Vorberg and I. V. Tetko, Modeling the Biodegradability of Chemical Compounds Using the Online CHEmical Modeling Environment (OCHEM), Mol. Inf., 2014, 33(1), 73–85 CrossRef CAS PubMed.
- R. S. Boethling and J. Costanza, Domain of EPI suite biotransformation models, SAR QSAR Environ. Res., 2010, 21(5–6), 415–443 CrossRef CAS PubMed.
- E. Dindar,
et al., Effect of wastewater sludge application on enzyme activities in soil contaminated with crude oil, J. Soil Sci. Plant Nutr., 2017, 17(1), 180–193 Search PubMed.
- P. C. Sangave and A. B. Pandit, Enhancement in biodegradability of distillery wastewater using enzymatic pretreatment, J. Environ. Manage., 2006, 78(1), 77–85 CrossRef CAS PubMed.
- C. Rucker and K. Kummerer, Modeling and predicting aquatic aerobic biodegradation – a review from a user's perspective, Green Chem., 2012, 14(4), 875–887 RSC.
- J. P. M. Vink and S. E. A. T. M. VanderZee, Pesticide biotransformation in surface waters: Multivariate analyses of environmental factors at field sites, Water Res., 1997, 31(11), 2858–2868 CrossRef CAS.
- V. Rajani and J. K. Reshma, Factors affecting phenol degradation potential of microbes – a review, World J. Pharm. Pharmaceut. Sci., 2016, 5(11), 691–706 Search PubMed.
- Y. Lee and U. von Gunten, Quantitative structure–activity relationships (QSARs) for the transformation of organic micropollutants during oxidative water treatment, Water Res., 2012, 46(19), 6177–6195 CrossRef CAS PubMed.
-
C. Burgis, Predicting Biological Removal of Contaminants in Wastewater Treatment: QSBR Modeling, in School of Engineering and Applied Science, University of Virginia, Virginia, US, 2012 Search PubMed.
-
M. Callahan and M. W. Slimak, Office of Water Planning and Standards, Water-related environmental fate of 129 priority pollutants, Environmental Protection Agency, United States, 1980 Search PubMed.
- A. Kowalczyk,
et al., Refinement of biodegradation tests methodologies and the proposed utility of new microbial ecology techniques, Ecotoxicol. Environ. Saf., 2015, 111, 9–22 CrossRef CAS PubMed.
- J. R. van der Meer, Environmental pollution promotes selection of microbial degradation pathways, Front. Ecol. Environ., 2006, 4(1), 35–42 CrossRef.
-
OECD, Aerobic Mineralisation in Surface Water – Simulation Biodegradation Test, OECD Guideline for the Testing of Chemicals, 2004 Search PubMed.
-
P. H. Howard, R. S. Boethling, W. F. Jarvis, W. M. Meylan and E. M. Michalenko, Handbook of Environmental Degradation Rates, CRC Press, Boca Raton, New York, 1991 Search PubMed.
- P. D. Capel and S. J. Larson, A Chemodynamic Approach for Estimating Losses of Target Organic-Chemicals from Water during Sample Holding Time, Chemosphere, 1995, 30(6), 1097–1107 CrossRef CAS.
-
M. Richardson, Environmental Xenobiotics, Taylor & Francis, Rickmansworth, Hertfordshire, UK, 1996 Search PubMed.
-
D. Mackay, W. Y. Shiu, K.-C. Ma and S. C. Lee, Physical–Chemical Properties and Environmental Fate for Organic Chemicals, CRC Press Taylor & Francis Group, Boca Raton, FL, 2006, pp. 33487–2742 Search PubMed.
-
United Nations, Globally Harmonized System of Classification and Labelling of Chemicals (GHS), New York and Geneva, 4th edn, 2011 Search PubMed.
- C. L. Tebes-Stevens and W. J. Jones, Estimation of microbial reductive transformation rates for chlorinated benzenes and phenols using a quantitative structure–activity relationship approach, Environ. Toxicol. Chem., 2004, 23(7), 1600–1609 CrossRef CAS PubMed.
-
J. Monod, Recherches sur la croissance des Cultures Bactériennes, Hermann, Paris, 1942 Search PubMed.
- P. van Bodegom, Microbial maintenance: A critical review on its quantification, Microb. Ecol., 2007, 53(4), 513–523 CrossRef PubMed.
- G. C. Okpokwasili and C. O. Nweke, Microbial growth and substrate utilization kinetics, Afr. J. Biotechnol., 2006, 5(4), 305–317 CAS.
-
OECD, Harmonised Integrated Classification System for Human health and Environmental Hazards of Chemical Substances and Mixtures, Organisation for Economic Co-operation and Development, 2001 Search PubMed.
-
OECD, OECD Guideline for Testing of Chemicals: Ready Biodegradability, OECD test 301, 1992 Search PubMed.
-
OECD, Introduction to the OECD guidelines for testing of chemicals, section 3. Principles and strategies related to the testing of degradation of organic chemicals, Organisation for Economic Cooperation and Development, Paris, 2006 Search PubMed.
-
OECD, OECD guidelines for the testing of chemicals. Guideline No. 301, Ready Biodegradability, Organisation for Economic Cooperation and Development, 1992 Search PubMed.
- L. P. Burkhard, Estimating dissolved organic carbon partition coefficients for nonionic organic chemicals, Environ. Sci. Technol., 2000, 34(22), 4663–4668 CrossRef CAS.
-
F. Atkinson, Standardiser, 2014, https://github.com/flatkinson/standardiser Search PubMed.
- J. Sadowski, J. Gasteiger and G. Klebe, Comparison of Automatic 3-Dimensional Model Builders Using 639 X-Ray Structures, J. Chem. Inf. Comput. Sci., 1994, 34(4), 1000–1008 CrossRef CAS.
- A. Duran, I. Zamora and M. Pastor, Suitability of GRIND-Based Principal Properties for the Description of Molecular Similarity and Ligand-Based Virtual Screening, J. Chem. Inf. Model., 2009, 49(9), 2129–2138 CrossRef CAS PubMed.
-
Molecular Discovery, Pentacle 1.0.6, 2017, http://www.moldiscovery.com/software/pentacle/ Search PubMed.
- M. Pastor,
et al., GRid-INdependent descriptors (GRIND): A novel class of alignment-independent three-dimensional molecular descriptors, J. Med. Chem., 2000, 43(17), 3233–3243 CrossRef CAS PubMed.
- C. W. Yap, PaDEL-Descriptor: An Open Source Software to Calculate Molecular Descriptors and Fingerprints, J. Comput. Chem., 2011, 32(7), 1466–1474 CrossRef CAS PubMed.
- G. Cruciani, M. Pastor and W. Guba, VolSurf: a new tool for the pharmacokinetic optimization of lead compounds, Eur. J. Pharm. Sci., 2000, 11, S29–S39 CrossRef CAS PubMed.
- P. Carrio,
et al., eTOXlab, an open source modeling framework for implementing predictive models in production environments, J. Cheminf., 2015, 7, 8 Search PubMed.
- S. Wold, M. Sjostrom and L. Eriksson, PLS-regression: a basic tool of chemometrics, Chemom. Intell. Lab. Syst., 2001, 58(2), 109–130 CrossRef CAS.
- F. Pedregosa,
et al., Scikit-learn: Machine Learning in Python, J. Mach. Learn. Res., 2011, 12, 2825–2830 Search PubMed.
- P. Polishchuk, Interpretation of Quantitative Structure−Activity Relationship Models: Past, Present, and Future, J. Chem. Inf. Model., 2017 DOI:10.1021/acs.jcim.7b00274.
- P. Carrio,
et al., Applicability Domain Analysis (ADAN): A Robust Method for Assessing the Reliability of Drug Property Predictions, J. Chem. Inf. Model., 2014, 54(5), 1500–1511 CrossRef CAS PubMed.
-
G. Moss, Enzyme Nomenclature, 2011, http://www.chem.qmul.ac.uk/iubmb/enzyme/ Search PubMed.
-
Eawag, Eawag Biocatalysis/Biodegradation Database and Pathway Prediction System, 7 December 2016, http://eawag-bbd.ethz.ch/predict/ Search PubMed.
- D. A. R. S. Latino,
et al., Eawag-Soil in enviPath: a new resource for exploring regulatory pesticide soil biodegradation pathways and half-life data, Environ. Sci.: Processes Impacts, 2017, 19(3), 449–464 CAS.
- C. Rendal, K. O. Kusk and S. Trapp, Optimal Choice of pH For Toxicity And Bioaccumulation Studies of Ionizing Organic Chemicals, Environ. Toxicol. Chem., 2011, 30(11), 2395–2406 CrossRef CAS PubMed.
- R. Gulde,
et al., pH-Dependent Biotransformation of Ionizable Organic Micropollutants in Activated Sludge, Environ. Sci. Technol., 2014, 48(23), 13760–13768 CrossRef CAS PubMed.
- A. D. Tappin,
et al., Unexpected removal of the most neutral cationic pharmaceutical in river waters, Environ. Chem. Lett., 2016, 14(4), 455–465 CrossRef CAS.
- D. S. Cao,
et al., ChemoPy: freely available python package for computational biology and chemoinformatics, Bioinformatics, 2013, 29(8), 1092–1094 CrossRef CAS PubMed.
- K. Michalak and H. Kwasnicka, Correlation based feature selection method, Int. J. Bio-Inspired Comput., 2010, 2(5), 319–332 CrossRef.
- H. T. Trinh, P. Adriaens and C. M. Lastoskie, Fate factors and emission flux estimates for emerging contaminants in surface waters, AIMS Environ. Sci., 2016, 3(1), 21–44 CrossRef CAS.
- H. M. Freese,
et al., Dissolved inorganic nutrients and organic substrates in the River Warnow (North-Eastern Germany) – Utilisation by bacterioplankton, Limnologica, 2007, 37(3), 264–277 CrossRef CAS.
- M. A. El-Dib and H. F. Abou-Waly, Biodegradation of some triazines and phenylureas in surface waters, Water Res., 1998, 32(6), 1881–1887 CrossRef CAS.
-
L. Toräng, Biodegradation rates of chemicals in surface water and groundwater assessed in batch simulation tests, Technical University of Denmark, Lyngby, Denmark, 2003 Search PubMed.
- C. Accinelli,
et al., Dissipation and removal of oseltamivir (Tamiflu) in different aquatic environments, Chemosphere, 2010, 79(8), 891–897 CrossRef CAS PubMed.
- J. T. Jasper,
et al., Biotransformation of Trace Organic Contaminants in Open-Water Unit Process Treatment Wetlands, Environ. Sci. Technol., 2014, 48(9), 5136–5144 CrossRef CAS PubMed.
- C. Prasse,
et al., Co-occurrence of Photochemical and Microbiological Transformation Processes in Open-Water Unit Process Wetlands, Environ. Sci. Technol., 2015, 49(24), 14136–14145 CrossRef CAS PubMed.
- A. Nzila, Update on the cometabolism of organic pollutants by bacteria, Environ. Pollut., 2013, 178, 474–482 CrossRef CAS PubMed.
- A. M. Veach and M. J. Bernot, Temporal variation of pharmaceuticals in an urban and agriculturally influenced stream, Sci. Total Environ., 2011, 409(21), 4553–4563 CrossRef CAS PubMed.
- M. Ivesic,
et al., Monitoring of selected pharmaceuticals in surface waters of Croatia, Environ. Sci. Pollut. Res. Int., 2017, 24(29), 23389–23400 CrossRef CAS PubMed.
- R. M. Baena-Nogueras, E. Gonzalez-Mazo and P. A. Lara-Martin, Degradation kinetics of pharmaceuticals and personal care products in surface waters: photolysis vs. biodegradation, Sci. Total Environ., 2017, 590, 643–654 CrossRef PubMed.
-
RIVM, Watson-database: Microverontreinigingen in influent en effluent van RWZI's, 2015, http://www.emissieregistratie.nl/erpubliek/erpub/wsn/default.aspx Search PubMed.
- L. Auret and C. Aldrich, Interpretation of nonlinear relationships between process variables by use of random forests, Miner. Eng., 2012, 35, 27–42 CrossRef CAS.
-
M. Pavan and A. P. Worth, Review of QSAR Models for Ready Biodegradation, European Commission Directorate General Joint Research Centre, Ispra (VA) Italy, 2006 Search PubMed.
-
N. Cnubben, Quantitative structure activity relationships for the biotransformation and toxicity of halogenated benzene-derivatives: Implications for enzyme catalysis and reaction mechanisms, University of Wageningen, Wageningen, Netherlands, 1996 Search PubMed.
- D. W. Liang,
et al., Phthalates biodegradation in the environment, Appl. Microbiol. Biotechnol., 2008, 80(2), 183–198 CrossRef CAS PubMed.
- D. C. Warhurst,
et al., Hydroxychloroquine is much less active than chloroquine against chloroquine-resistant Plasmodium falciparum, in agreement with its physicochemical properties, J. Antimicrob. Chemother., 2003, 52(2), 188–193 CrossRef CAS PubMed.
-
J. M. Berg, J. L. Tymoczko and L. Stryer, Enzymes: Basic Concepts and Kinetics, in Biochemistry, W.H. Freeman & Co Ltd, New York, US, 2002, ch. 8 Search PubMed.
- R. H. Muller, T. Rohwerder and H. Harms, Carbon conversion efficiency and limits of productive bacterial degradation of methyl tert-butyl ether and related compounds, Appl. Environ. Microbiol., 2007, 73(6), 1783–1791 CrossRef PubMed.
-
NRC, Physicochemical Properties and Environmental Fate, in A Framework to Guide Selection of Chemial Alternatives, N.R.C.C.o.t.D.a.E.o.S.C. Substitutions, The National Academic Press, Washington, D.C, 2014, ch. 5 Search PubMed.
- A. Ramachandran and D. A. Walsh, Investigation of XoxF methanol dehydrogenases reveals new methylotrophic bacteria in pelagic marine and freshwater ecosystems, FEMS Microbiol. Ecol., 2015, 91(10) CrossRef CAS PubMed.
- Y. M. Kim, E. W. Lee, S. J. Kim and M. S. Lee, Characterization of Formaldehyde-degrading Bacteria Isolated from River Sediment, Korean J. Fish. Aquat. Sci., 2008, 41(2), 84–88 CAS.
- O. Bruneel,
et al., Characterization of the Active Bacterial Community Involved in Natural Attenuation Processes in Arsenic-Rich Creek Sediments, Microb. Ecol., 2011, 61(4), 793–810 CrossRef PubMed.
- P. M. Bradley,
et al., Biotransformation of caffeine, cotinine, and nicotine in stream sediments: Implications for use as wastewater indicators, Environ. Toxicol. Chem., 2007, 26(6), 1116–1121 CrossRef CAS PubMed.
- E. Sugrue,
et al., Evolutionary Expansion of the Amidohydrolase Superfamily in Bacteria in Response to the Synthetic Compounds Molinate and Diuron, Appl. Environ. Microbiol., 2015, 81(7), 2612–2624 CrossRef CAS PubMed.
-
E. J. Conway, R. J. Peterson, R. F. Collingsworth, J. G. Graca and J. W. Carter, Assessment of the need for and character of limitations on acrylamide and its compounds (Report prepared for the Offic of Pesticides and Toxic Substances), US Environmental Protection Agency, Washington DC, 1979 Search PubMed.
- N. Yamaguchi and M. Nasu, Flow cytometric analysis of bacterial respiratory and enzymatic activity in the natural aquatic environment, J. Appl. Microbiol., 1997, 83(1), 43–52 CAS.
- N. L. Wolfe,
et al., Correlation of Microbial-Degradation Rates with Chemical-Structure, Environ. Sci. Technol., 1980, 14(9), 1143–1144 CrossRef CAS.
- S. K. Bakar and S. Niazi, Stability of Aspirin in Different Media, J. Pharm. Sci., 1983, 72(9), 1024–1026 CrossRef CAS PubMed.
- A. R. Tehrani-Bagha,
et al., Cationic ester-containing gemini surfactants: Chemical hydrolysis and biodegradation, J. Colloid Interface Sci., 2007, 312(2), 444–452 CrossRef CAS PubMed.
- M. D. Jurgens,
et al., The potential for estradiol and ethinylestradiol degradation in English rivers, Environ. Toxicol. Chem., 2002, 21(3), 480–488 CrossRef CAS PubMed.
- G. Cormier,
et al., The degradation behaviour of nine diverse contaminants in urban surface water and wastewater prior to water treatment, Environ. Sci.: Processes Impacts, 2015, 17(12), 2051–2065 CAS.
- S. Subramanian,
et al., Oral feeding with ethinyl estradiol. suppresses and treats experimental autoimmune encephalomyelitis in SJL mice and inhibits the recruitment of inflammatory cells into the central nervous system, J. Immunol., 2003, 170(3), 1548–1555 CrossRef CAS.
-
R. P. Schwarzenbach, G. P. M. and D. M. Imboden, Environmental Organic Chemistry, Wiley, New York, 2003 Search PubMed.
- J. J. Yang,
et al., Retention Mechanisms of Citric Acid in Ternary Kaolinite-Fe(III)-Citrate Acid Systems Using Fe K-edge EXAFS and L-3, L-2-edge XANES Spectroscopy, Sci. Rep., 2016, 6, 617–620 Search PubMed.
- G. Renella, L. Landi and P. Nannipieni, Degradation of low molecular weight organic acids complexed with heavy metals in soil, Geoderma, 2004, 122(2–4), 311–315 CrossRef CAS.
- K. Fujii, M. Aoki and K. Kitayama, Biodegradation of low molecular weight organic acids in rhizosphere soils from a tropical montane rain forest, Soil Biol. Biochem., 2012, 47, 142–148 CrossRef CAS.
- P. A. W. van Hees, D. L. Jones and D. L. Godbold, Biodegradation of low molecular weight organic acids in coniferous forest podzolic soils, Soil Biol. Biochem., 2002, 34(9), 1261–1272 CrossRef CAS.
- E. Estrada, Spectral moments of the edge adjacency matrix in molecular graphs. 1. Definition and applications to the prediction of physical properties of alkanes, J. Chem. Inf. Comput. Sci., 1996, 36(4), 844–849 CrossRef CAS.
- K. Horikoshi, Alkaliphiles: Some applications of their products for biotechnology, Microbiol. Mol. Biol. Rev., 1999, 63(4), 735–750 CAS.
- T. A. Krulwich, G. Sachs and E. Padan, Molecular aspects of bacterial pH sensing and homeostasis, Nat. Rev. Microbiol., 2011, 9(5), 330–343 CrossRef CAS PubMed.
-
K. H. Neumann, W. Barz and E. Reinhard, Primary and Secondary Metabolism of Plant Cell Cultures, Springer-verlag, Berlin Heidelberg, 1985 Search PubMed.
-
S. T. Weiss, High-Yield Pharmacology, Wolters Kluwer, Philadelphia, 2009 Search PubMed.
- P. K. Arora and H. Bae, Bacterial degradation of chlorophenols and their derivatives, Microb. Cell Factories, 2014, 13, 31 CrossRef PubMed.
- D. M. Munnecke, Enzymatic-Hydrolysis of Organophosphate Insecticides, a Possible Pesticide Disposal Method, Appl. Environ. Microbiol., 1976, 32(1), 7–13 CAS.
- J. Thiele, R. Muller and F. Lingens, Enzymatic Dehalogenation of Chlorinated Nitroaromatic Compounds, Appl. Environ. Microbiol., 1988, 54(5), 1199–1202 CAS.
-
W. R. Mabey, J. H. Smith, R. T. Podoll, H. L. Johnson, T. Mill, T. W. Chou, C. J. Gates, I. Waight Partridge, H. Jaber and D. Vandenberg, Aquatic fate process data for organic priority pollutants, United States Environmental Protection Agency, Washington DC, 1982 Search PubMed.
-
M. Möndel, 14C-Preventol CMK: Aerobic degradation of 14C-Preventol CMK in two different aquatic sediment systems, RLP AgroScience GmbH, Neustadt a.d. Weinstraße, Gemany, 2009 Search PubMed.
- D. F. Paris and J. E. Rogers, Kinetic Concepts for Measuring Microbial Rate Constants – Effects of Nutrients on Rate Constants, Appl. Environ. Microbiol., 1986, 51(2), 221–225 CAS.
- W. C. Steen and T. W. Collette, Microbial degradation of seven amides by suspended bacterial populations, Appl. Environ. Microbiol., 1989, 55(10), 2545–2549 CAS.
- D. F. Paris and N. L. Wolfe, Relationship between Properties of a Series of Anilines and Their Transformation by Bacteria, Appl. Environ. Microbiol., 1987, 53(5), 911–916 CAS.
- M. W. Lam,
et al., Aquatic persistence of eight pharmaceuticals in a microcosm study, Environ. Toxicol. Chem., 2004, 23(6), 1431–1440 CrossRef CAS PubMed.
- J. Bagnall,
et al., Stereoselective biodegradation of amphetamine and methamphetamine in river microcosms, Water Res., 2013, 47(15), 5708–5718 CrossRef CAS PubMed.
- P. J. Alvarez, P. J. Anid and T. M. Vogel, Kinetics of aerobic biodegradation of benzene and toluene in sandy aquifer material, Biodegradation, 1991, 2(1), 43–51 CrossRef CAS PubMed.
- B. A. Bekins, E. Warren and E. M. Godsy, A comparison of zero-order, first-order, and Monod biotransformation models, Ground Water, 1998, 36(2), 261–268 CrossRef CAS.
- H. Radianingtyas, G. K. Robinson and A. T. Bull, Characterization of a soil-derived bacterial consortium degrading 4-chloroaniline, Microbiology, 2003, 149, 3279–3287 CrossRef CAS PubMed.
- J. L. Wang, Z. Y. Mao and W. Z. Wu, Microbial degradation of aniline by bacterial consortium, Biomed. Environ. Sci., 2003, 16(4), 398–404 Search PubMed.
- R. S. Bose, S. Dey, S. Saha, C. K. Ghosh and M. G. Chaudhuri, Enhanced removal of dissolved aniline from water under combined system of nano zero-valent iron and Pseudomonas putida, Sustainable Water Resources Management, 2016, 2, 143 CrossRef.
- Q. T. Liu, R. I. Cumming and A. D. Sharpe, Photo-induced environmental depletion processes of beta-blockers in river waters, Photochem. Photobiol. Sci., 2009, 8(6), 768–777 CAS.
- L. Berthod,
et al., Quantitative structure–property relationships for predicting sorption of pharmaceuticals to sewage sludge during waste water treatment processes, Sci. Total Environ., 2016, 1512–1520 Search PubMed.
- R. M. Summers,
et al., Genetic characterization of caffeine degradation by bacteria and its potential applications, Microb. Biotechnol., 2015, 8(3), 369–378 CrossRef CAS PubMed.
- H. Al Qarni,
et al., Investigating the removal of some pharmaceutical compounds in hospital wastewater treatment plants operating in Saudi Arabia, Environ. Sci. Pollut. Res., 2016, 23(13), 13003–13014 CrossRef CAS PubMed.
- M. Winder and J. E. Cloern, The annual cycles of phytoplankton biomass, Philos. Trans. R. Soc., B, 2010, 365(1555), 3215–3226 CrossRef PubMed.
- J. S. Anissi, K. and O. A. Olapade, Seasonal Shifts in the Bacterioplankton Assemblages of High Altitude Middle Atlas Lakes, J. Water Resour. Protect., 2014, 6(1), 7 Search PubMed.
- B. L. Timney,
et al., Simple rules for passive diffusion through the nuclear pore complex, J. Cell Biol., 2016, 215(1), 57–76 CrossRef CAS PubMed.
- E. M. Johnson and W. M. Deen, Electrostatic effects on the equilibrium partitioning of spherical colloids in random fibrous media, J. Colloid Interface Sci., 1996, 178(2), 749–756 CrossRef CAS.
- S. Stolte,
et al., Primary biodegradation of ionic liquid cations, identification of degradation products of 1-methyl-3-octylimidazolium chloride and electrochemical wastewater treatment of poorly biodegradable compounds, Green Chem., 2008, 10(2), 214–224 RSC.
- R. M. Krupka, Kinetics of Transport-Systems Dependent on Periplasmic Binding-Proteins, Biochim. Biophys. Acta, Bioenerg., 1992, 1110(1), 1–10 CrossRef CAS.
Footnote |
† Electronic supplementary information (ESI) available. See DOI: 10.1039/c7em00375g |
|
This journal is © The Royal Society of Chemistry 2018 |