Design and engineering of whole-cell biocatalytic cascades for the valorization of fatty acids
Received
6th September 2019
, Accepted 14th November 2019
First published on 19th November 2019
Abstract
One of the key factors to construct a productive whole-cell biocatalytic cascade may include the design of the biotransformation pathways, discovery and engineering of the cascade enzymes, and functional and balanced expression of the cascade enzymes in microbial cells as well as systematic engineering of the whole-cell biocatalytic cascades. Such issues are covered in this review exemplified for the biotransformation of renewable fatty acids into industrially relevant oleochemicals (e.g., medium-chain fatty acids such as n-nonanoic acid, 9-hydroxynonanoic acid, 9-aminononanoic acid, and 1,9-nonanedioic acid) as model systems.
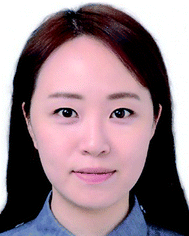 Ji-Won Song | Ji-Won Song received her Ph.D. degree from the Department of Food Science & Engineering, Ewha Womans University (Seoul, Republic of Korea), in 2016 under the supervision of Prof. Jin-Byung Park. She is now carrying out postdoctoral research in Prof. Park's research group at Ewha Womans University. She has been working on the design of biotransformation pathways (e.g., renewable fatty acids) and engineering of recombinant bacterial biocatalysts. Her current research interest focuses on the development of nano-scale bioreactors based on bacterial outer-membrane vesicles for the biotransformation of fatty acids. |
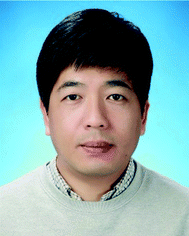 Joo-Hyun Seo | Joo-Hyun Seo received his Ph.D. degree in the development of a computational enzyme identification system from Seoul National University (Seoul, Republic of Korea; supervisor: Prof. Byung-Gee Kim) in 2009. After that, he moved to the University of California (San Diego, CA, USA) as a postdoctoral fellow working on multi-omic analysis of bacterial metabolism (research advisor: Prof. Bernhard Palsson). Currently, he is a professor in the Department of Bio and Fermentation Convergence Technology at Kookmin University (Seoul, Republic of Korea). His research interests are mainly focused on (1) development of an enzymatic process for value-added compounds, (2) computational enzyme identification and evolution for the development of industrial enzymes using bioinformatics and machine learning, (3) sequence analysis of antibody libraries for in vivo-evolved antibodies, and (4) development of therapeutic proteins. |
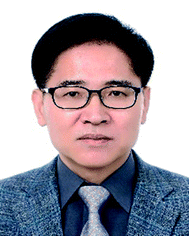 Deok-Kun Oh | Deok-Kun Oh received his Ph.D. degree from the Department of Biotechnology, Korea Advanced Institute of Science and Technology. He studied at Osaka University (Japan) as a visiting researcher. Currently, he is a professor in the Department of Bioscience and Biotechnology at Konkuk University (Seoul, Republic of Korea). He has published 280 international papers. His research interests are mainly focused on structure-based enzyme engineering and his main research topic is hydroxylation of fatty acids by hydratases, lipoxygenases, and diol synthases. He is studying the enzymatic synthesis of lipid mediators and specialized pro-resolving mediators (C20, C22 di- and tri-hydroxy polyunsaturated fatty acids in humans) such as lipoxins, resolvins, protectins, and maresins. |
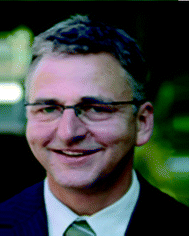 Uwe T. Bornscheuer | Uwe T. Bornscheuer studied chemistry and completed his doctorate in 1993 at the University of Hannover under the supervision of K. Schügerl and T. Scheper. He then carried out research at the University of Nagoya (Japan) with T. Yamane. In 1998, he completed his habilitation at the University of Stuttgart with R. D. Schmid on new strategies for the use of lipases and esterases in organic synthesis. He has been a professor at the Institute of Biochemistry at the University of Greifswald since October 1999. Bornscheuer received several awards such as the BioCat2008 Award for his innovative work on tailored biocatalysts for industrial applications and several awards (Normann Medal, Chevreul Medal, Stephen S. Chang Award) for his achievements in the area of enzymatic modifications of lipids, fats and oils. His current research interest focuses on the discovery and protein engineering of enzymes from various classes for applications in organic synthesis, lipid modification, and enzymatic degradation of plastics or algal polysaccharides. The methods used range from rational protein design to high-throughput screening methods for biocatalyst discovery including the development of enzyme-cascade reactions. |
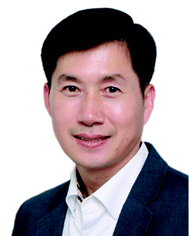 Jin-Byung Park | Jin-Byung Park received his Ph.D. degree from the Institute of Biotechnology, ETH (Zurich, Switzerland), in 2005. After that, he moved to the University of California (Berkeley, USA) as a postdoctoral fellow working on biocatalysis in organic/aqueous emulsions and in non-aqueous media. Currently, he is a professor in the Department of Food Science & Engineering at Ewha Womans University (Seoul, Republic of Korea). His research interests are mainly focused on whole-cell biotransformations (regiospecific oxygenation and/or oxidative cleavage of fatty acids, design and construction of whole-cell biocatalysts, metabolic engineering of microbial cells) and enzyme engineering (examination of enzyme structure and function relationship, design and construction of biotransformation pathways, engineering of enzymes for biocatalysis). |
1. Introduction
Enzymes are able to catalyze a variety of biological and chemical, natural and artificial, single to multistep reactions generating a versatile array of biologically active and structurally diverse molecules.1–7 The synthetic performance of the enzymes is steadily increasing due to not only the discovery of new active enzymes but also by rational and evolutionary engineering of them, including de novo design of stable protein structures.8,9
The metabolic networks or enzyme reaction networks in living cells represent a highly efficient system of cascade reactions. Natural and engineered metabolic networks have been extensively used for the production of a variety of chemicals including alcohols, aldehydes, acids, esters, ketones, and hydrocarbons.10–18 This basic principle is also frequently used not only in biocatalysis with isolated enzymes but also in whole-cell biotransformations.2,12,19–26 This cascade biocatalysis consisting of isolated enzymes is often shown in cofactor-independent biotransformations and/or for enzymes having high KM values. For instance, conversion of ethanol to acetoin, 2,3-butanediol, and 2-butanol by ethanol dehydrogenase, formolase, 2,3-butanediol dehydrogenase, diol dehydratase, and NADH oxidase was exploited in a cell-free system because of the large KM value of formolase for acetaldehyde (i.e., 58 mM).27 A newer trend is the combination of enzymes with various chemical catalysts.28–32 Furthermore, microorganism consortia-mediated chemoenzymatic multistep biotransformations in a one-pot process have also been investigated.33
Obvious advantages of cascade biocatalysis are that there is no need for purification/isolation of intermediates, operating time, production costs and waste are reduced, and concomitantly, overall yields are improved.12,19,21,23,25,34–38 Additionally, the problem of low stability and toxicity of the reaction intermediates can be attenuated by balanced expression of the cascade enzymes. If needed, reactivity and selectivity can be enhanced as unfavorable reaction equilibria can be overcome. Consequently, the past decade has seen a substantial increase in successful examples for the combination of enzymes for multistep syntheses offering higher yields, decreased costs, environmental benefits and high selectivity.28,31 For example, biocatalytic cascades for limonene, vanillin, and chiral lactone synthesis, which are interesting commercial products, have been reported.39–41 Thereby, this review will focus on major contributions in the field of cascade biocatalysis, particularly for whole-cell biocatalytic cascades.
2. Design of cascade enzyme-based whole-cell biocatalysts
2.1 Design of the biotransformation pathways
Simplicity of the biotransformation pathways, availability of the starting materials, transport of the reactants, functional expression of the cascade enzymes, toxicity of the reactants and products, equilibrium thermodynamics, and cofactor dependency as well as background reactions by metabolic enzymes and/or by-product formations can be included as the major concerns in the design of multi-step whole-cell biotransformation pathways (Fig. 1).12,19,20 Here, we will discuss the design of such pathways by using the biotransformation of hydrocarbons and fatty acids into value-added products as model systems. One of the representative examples is the biosynthesis of industrially relevant medium-chain fatty acids (e.g., 9-hydroxynonanoic acid (10), 9-aminononanoic acid (23), and 1,9-nonanedioic acid (11)) from renewable fatty acids (Schemes 1–5).20,42 These compounds can be produced via terminal oxyfunctionalization of medium-chain fatty acids (e.g., n-nonanoic acid) and hydrocarbons43–47 or via oxidative cleavage of a C–C bond within long-chain fatty acids (e.g., oleic acid (5), linoleic acid (12), and ricinoleic acid (40)).48–52 The biotransformation pathway of n-nonanoic acid methyl ester (NAME) (1) is simpler than that of oleic acid (5) (Schemes 1 and 2). However, oleic acid is the cheaper and renewable substrate as compared to NAME. Regarding reactant toxicity, NAME and its derivatives are much more toxic to microbial cells than long-chain fatty acids. Thereby, attenuation of the reactant toxicity should be considered to reach high product concentrations. NAME can be hydrolyzed by native esterases in microbial cells, so that host cell engineering to remove the ester hydrolysis activity is required as a prerequisite. On the other hand, transport of the reactants should be considered in the oleic acid biotransformation.51,53n-Nonanoic acid (9), which is produced simultaneously with 9-hydroxynonanoic acid (10) from oleic acid (5) (Scheme 2), may inhibit activity of the cascade enzymes and make isolation of the target products complicated.54,55
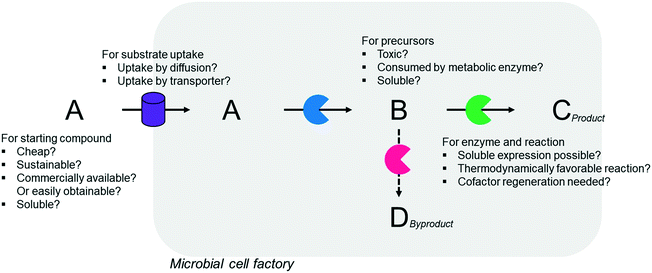 |
| Fig. 1 Factors that influence whole-cell multi-step biotransformation. | |
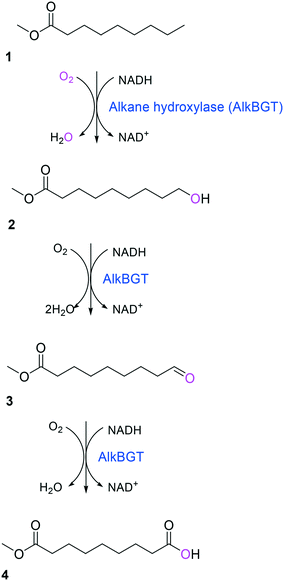 |
| Scheme 1 Biotransformation pathway of n-nonanoic acid methyl ester (1) into C9 chemicals.43,44 | |
 |
| Scheme 2 Biotransformation pathway of oleic acid (5) into C9 chemicals.49,51 | |
Another way to produce the industrially relevant C9 chemicals from renewable long-chain fatty acids may include a multiple enzyme cascade reaction involving a 9(S)-lipoxygenase and a 9-hydroperoxide lyase52 (Scheme 3). This biotransformation pathway is rather simple but functional expression of the cascade enzymes in microbial cells turned out to be difficult. Moreover, the lipoxygenases are susceptible to deactivation via suicide reactions caused by reactive reaction intermediates (e.g., hydroperoxy fatty acid (13)),56–60 limiting the product concentration in the range of less than a few grams per liter.
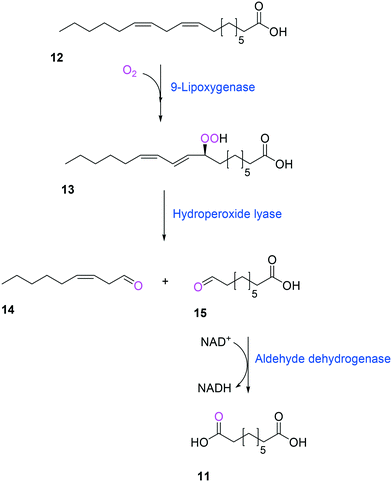 |
| Scheme 3 Biotransformation pathway of linoleic acid (12) into 1,9-nonanedioic acid (11) by lipoxygenase, hydroperoxide lyase, and aldehyde dehydrogenase.52 | |
Whole-cell biotransformation of ω-hydroxy fatty acids into ω-amino fatty acids is rather complicated with respect to the pathway design because equilibrium thermodynamics, amine donor supply, cofactor regeneration, and by-product formation must be considered to obtain a high product concentration along with high conversion and yield.20,36,61–64 One approach to solve these issues would be to introduce an amino acid dehydrogenase65–69 or to feed an appropriate amine donor.61,64 Ladkau et al. (2016)68 have established a coupling system for amine donor supply by coexpressing a heterologous alanine dehydrogenase (Scheme 4). This enzyme allowed regeneration of the amine donor (i.e., alanine) for ω-transaminase by catalyzing reductive amination of pyruvate into alanine. Another way was to use benzylamine as an amine donor.64 Benzaldehyde, which is then produced as a by-product from benzylamine, was reduced to benzyl alcohol by metabolic enzymes present in E. coli cells, generating NAD+ for the alcohol dehydrogenase and shifting the equilibrium to the amination of ω-oxo fatty acids (Scheme 5). On the other hand, formation of by-products (e.g., α,ω-dicarboxylic acids from ω-oxo fatty acids) should be minimized by removing unspecific whole-cell oxidation activity with ω-oxo fatty acids and/or by increasing the transamination activity.
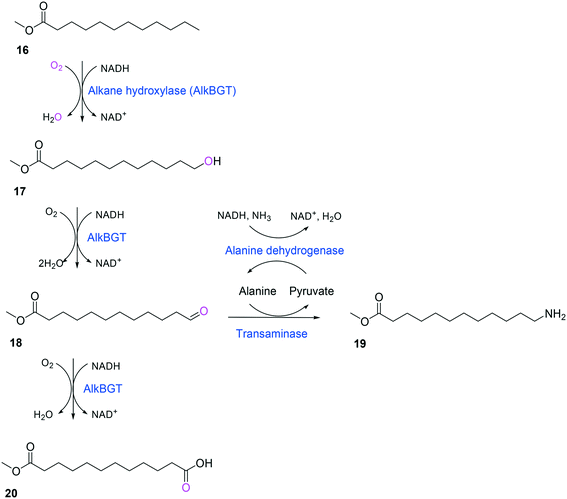 |
| Scheme 4 Biotransformation pathway of n-dodecanoic acid methyl ester (16) into 12-aminododecanoic acid methyl ester (19).68 | |
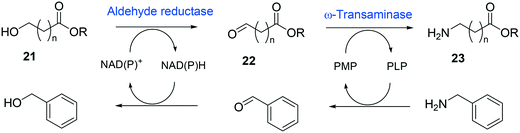 |
| Scheme 5 Biotransformation pathway of ω-hydroxy fatty acids (21) into ω-amino fatty acids (23).64 | |
In summary, a number of factors, including availability of the starting materials, transport of the reactants, functional expression and stability of the cascade enzymes, toxicity of the reactants and products, equilibrium thermodynamics, cofactor regeneration, and background reactions and by-product formation, should be considered in designing multi-step whole-cell biotransformation pathways to achieve high product concentrations and product yields.
2.2 Discovery and engineering of the cascade enzymes
Two of the key enzymes in multi-step biotransformations of aromatic and aliphatic hydrocarbons including fatty acids are oxygenases and transaminases (Schemes 1–6). Thereby, discovery and engineering of these enzymes will be focused in this section.
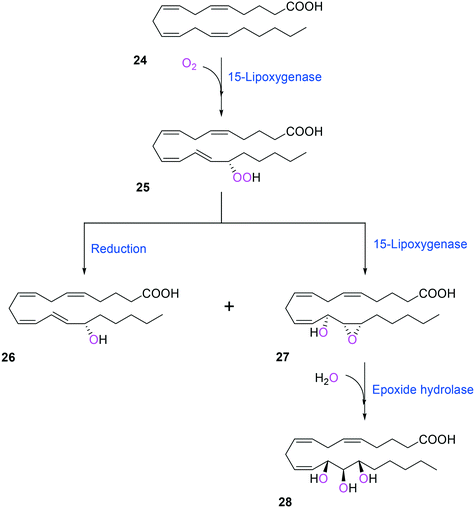 |
| Scheme 6 Biotransformation pathway of arachidonic acid (24) into a hepoxilin (27) and a trioxilin (28) via 15-hydroperoxyeicosapentaenoic acid (25).88 | |
2.2.1 Discovery of suitable cascade enzymes.
Substrate scope-based screening.
Several excellent reviews have previously described the discovery of novel enzymes based on sequence and structure information.70–72 In particular, Slabu et al. (2017)70 summarized a sequence-driven approach, homology-based search, and motif-based in silico strategy in addition to a traditional enrichment cultivation-based function-driven approach for the discovery of transaminases. Thereby, this section will focus on substrate scope-based screening of oxygenases.
One representative example for oxygenases is the Baeyer-–Villiger monooxygenase (BVMO), which catalyzes the enzymatic counterpart of the chemical Baeyer–Villiger oxidation first published in 1899. Instead of peracids used for chemical reactions, BVMOs use molecular oxygen and the cofactor NAD(P)H to convert ketones into esters.73–77 The catalytic activity and regioselectivity of BVMOs are difficult to predict based on sequence and structure information because the enzymes undergo conformational changes during biocatalysis.78 Thereby, substrate scope-based screening can be used to find new enzyme candidates for the desired reactions. For instance, the BVMOs, which were classified to catalyze the oxygenation of long-chain aliphatic ketones (e.g., 2-decanone and 4-decanone), might be used as candidates for the oxygenation of keto fatty acids such as 10-keto-octadecanoic acid (7) (Scheme 2) and 10-keto-octadec-12-enoic acid. The BVMOs, which are able to oxygenate 2-decanone and 4-decanone, may accept 10-keto-octadecanoic acid as a substrate because these compounds have a carbonyl group in the linear aliphatic hydrocarbon backbone. Based on the reaction mechanism, the carbonyl group should interact with the peroxyflavin and catalytic residues in the active site of enzymes, while the aliphatic hydrocarbon backbone may have hydrophobic interactions with amino acid residues sitting in the substrate-binding region (Fig. 2), as previously reported for fatty acid-converting enzymes.79–82 If the candidate enzymes have a space for a carboxyl group of the reaction substrates and/or possess positively charged amino acid residues (e.g., arginine and lysine), which may interact with the negatively charged carboxyl group, the enzyme candidates would be active with the target substrates. Based on this concept, the BVMOs from Pseudomonas putida KT2440 and Pseudomonas fluorescens DSM50106, which had been shown to accept long-chain aliphatic ketones (e.g., 2- and 4-decanone) as substrates, were found to also catalyze the oxygenation of the long-chain keto fatty acids (e.g., 10-keto-octadecanoic acid (7), 10-keto-octadec-12-enoic acid, 12-keto-octadec-9-enoic acid, and 10-keto-12-hydroxyoctadecanoic acid (3)) (Fig. 2).49,62,83–85
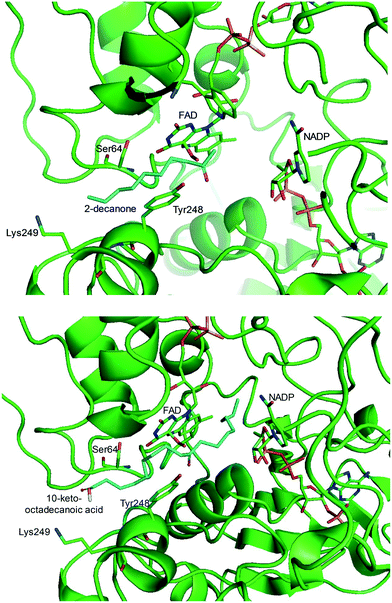 |
| Fig. 2 Model structure of the substrate-BVMO from P. putida KT2440. The homology model of the BVMO was constructed using a previously reported method.120,181 Docking simulation was performed using Autodock 3.0.182 The best docking pose was selected among 100 docking poses based on the distance between two reaction centers and binding energy.183 Two reaction centers mean the oxygen atom attached to flavin peroxide and the carbon atom of the carbonyl group in the substrates. The figures were drawn using PyMol184 (http://www.pymol.org/). | |
Another class of enzymes, for which the substrate scope is hardly predictable from sequence and structure information, are lipoxygenases (LOXs). These enzymes catalyze regio- and stereo-specific dioxygenation of polyunsaturated fatty acids with one or more Z,Z-1,4-pentadiene units by introducing molecular oxygen at one end of the pentadiene to form hydroperoxy fatty acids.52,86–88 Some mammalian LOXs may catalyze further conversion of the hydroperoxy fatty acids (25) into epoxy fatty acids or epoxy hydroxy fatty acids (hepoxilins) (27) by epoxidation89 (Scheme 6). Recently, microbial LOXs that are able to transform polyunsaturated fatty acids (e.g., arachidonic acid (24), eicosapentaenoic acid, and docosahexaenoic acid) to epoxy hydroxy fatty acids via hydroperoxy fatty acids (Scheme 6) were discovered for the first time. The enzymes are the LOXs from Myxococcus xanthus87,90 and Burkholderia thailandensis.88 The microbial LOXs were screened based on their catalytic activities towards conversion of polyunsaturated fatty acids into hydroperoxy fatty acids.
Biosensor-based screening.
A variety of ligand-inducible transcription factors in nature may specifically recognize small molecules and alter gene transcription at their targeted promoters. Thereby, a number of natural and synthetic transcription factor-based biosensors with ligand specificity and dynamic detection ranges have been applied for sensing various small molecules.91–96 For instance, a synthetic microbial biosensor (Fig. 3), based on the Nit from Alcaligenes faecalis, was applied for the highly specific detection of lactam compounds that had been produced from ω-amino fatty acids.97 The lactam-detecting biosensor, termed ε-caprolactam-detectable genetic enzyme-screening system, was successfully used for high-throughput screening of ε-caprolactam-converting cyclases from diverse metagenomes. Therefore, the biosensor-based screening systems, which are straightforward and powerful tools for detecting target molecules, can be used for high-throughput screening of enzymes and pathways of interest.
 |
| Fig. 3 Schematic representation of the ε-caprolactam-detectable genetic enzyme-screening system with cyclase in E. coli.97 Intracellular ε-caprolactam compounds were generated by 3-hydroxybutyrate dehydrogenase from ω-fatty acids, such as 5-aminovaleric acid or 6-aminocaproic acid, and were visualized by superfolder GFP, whose expression was induced by the ε-caprolactam-nitrilase regulator complex. This figure has been adapted from ref. 97 with permission from Springer Nature. | |
2.2.2 Enzyme engineering.
Oxygen uncoupling is one of the major issues in protein engineering of oxygenases, including P450 monooxygenases and flavin monooxygenases (e.g., BVMOs), because they may generate reactive oxygen species (ROS) (e.g., H2O2) consuming molecular oxygen and electrons from expensive nicotinamide cofactors.35,73,98–104 The flavin-dependent reductases (e.g., old yellow enzymes) may also generate H2O2via an electron uncoupling reaction under aerobic conditions.105 In addition, dioxygenases (e.g., lipoxygenases) produce hydroperoxy fatty acids (13, 25) as the major products and/or intermediates52,86–88 (Schemes 3 and 6). Notably, the ROS may deactivate not only the oxygen-utilizing enzymes but also other cascade enzymes by oxidizing low-redox potential amino acids such as methionine, cysteine, tryptophan, and histidine.106,107 Thereby, this section will focus on oxygen uncoupling and protein engineering of oxygenases to improve oxidative stability.
In the case of the flavin monooxygenases (e.g., BVMOs), hydrogen peroxide can be generated from the reaction intermediate (i.e., flavin-C4α-(hydro)peroxide) (31) during catalysis102,105 (Scheme 7). The H2O2 formation was reported to be influenced by a number of factors including stability of the peroxyflavin intermediate (31). Interestingly, in a recent study dealing with the switch of the cofactor specificity of a BVMO from the natural NADPH to the cheaper NADH, it was noted that substantial uncoupling occurred and the amount of H2O2 formed changed considerably upon introducing mutations.100 As commonly only cofactor (NAD(P)H) consumption is measured in spectrophotometric assays, it remains unclear how much cofactor is used for the desired product formation and how much cofactor is ending up in H2O2. To overcome this problem, the Bornscheuer group recently reported a versatile spectrophotometric assay in which both NAD(P)H consumption and H2O2 formation can be quantified simultaneously.103
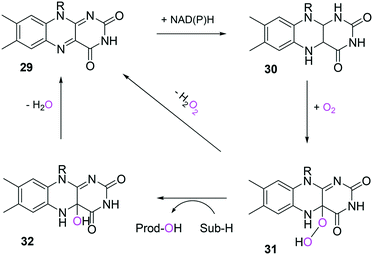 |
| Scheme 7 Simplified mechanism of flavin-dependent monooxygenases.102,105 It consists of NAD(P)H-dependent reduction of the flavin prosthetic group, followed by activation of molecular oxygen as a (hydro)peroxyflavin (31), and substrate oxygenation. The catalytic cycle is closed after elimination of water and reformation of the oxidised flavin (29). Alternatively, the (hydro)peroxyflavin can eliminate H2O2 spontaneously (uncoupling reaction) into the oxidised flavin. | |
In general, the active site of BVMOs is located in a cleft at the domain interface. The ribose group of NADP+ and an arginine residue, which lies above the flavin ring, are critical for stabilizing the anion of flavin peroxide (31) and/or the characteristic Criegee intermediates. They may generate various interactions including hydrogen bonding networks to the intermediate peroxyflavin species.108,109 The arginine residue may also act as an anchoring element for proper binding of the ketone substrate. Its positively charged guanidinium group can enhance the propensity of the substrate to undergo a nucleophilic attack by the flavin peroxide intermediate.108 As a result, such intensive networks allow the BVMOs to be less susceptible to oxygen uncoupling as compared to the flavoprotein hydroxylases.102,103,105,110
A number of studies have reported stabilization of the BVMOs against oxidative stress. One example was engineering of the cyclohexanone monooxygenase (CHMO) from Acinetobacter sp. NCIMB 9871.111 The enzyme stability was substantially improved by a single mutation such as M5I, M291L, M481A, C376L, or C520V. In particular, the C376L mutant has shown the highest activity in the presence of low concentrations of hydrogen peroxide. Oxidation of C376 by H2O2, situated close to the predicted NADPH binding site, might negatively affect the positioning of NADP+, which is important to stabilize the flavin-peroxide intermediate.112 Another interesting point was that substitution of C330L, which is present within the active site of CHMO, led to a significant loss of activity in the presence of H2O2. This might be ascribed to destruction of hydrogen bonding networks in the active site by the C330L mutation, which is also critical for stability of the flavin peroxide intermediate. The contribution of other amino acid residues (i.e., M5I, M291L, M481A, or C520V) to oxidative stability, which are mostly found on the enzyme surface, remains to be investigated.
Another study was engineering of the BVMO from P. putida KT2440 to improve its oxidative stability.113,114 The enzyme was subjected to diverse single mutations including the amino acid residues buried in the NADPH binding domain (e.g., C302, M212, M203) and the surface-exposed residues (M453, C261, M340). The C302L and C302I variants have shown the greatest activity after incubation with H2O2. Among the double mutants tested, the C302L/M340L variant exhibited 2 to 3 fold-higher activity as compared to the C302L and C302I mutants, suggesting that both the C302L and the M340L mutations are involved in tolerance of the BVMO to oxidative stress. Notably, the position of the C302 residue corresponds to that of the C330 residue in the Acinetobacter sp. CHMO, which is present within the active site.111 However, mutation of C330L in CHMO resulted in a significant decrease of oxidative stability. According to the structural models, L302 of the BVMO is surrounded by hydrophobic amino acid residues such as A194 and V197, generating hydrophobic interaction, whereas A56, T80, and I215 are found in the vicinity of L330 of CHMO (Fig. 4). Therefore, it was assumed that the microenvironment of the amino acid residues targeted is important to acquire oxidative stability.
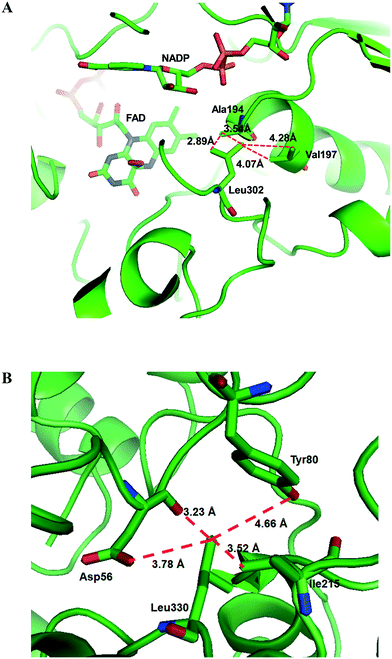 |
| Fig. 4 Model structure of the BVMO from P. putida KT2440 (A) and the CHMO from Acinetobacter sp. NCIMB 9871 (B), showing the microenvironment of residues L302 and L330, respectively.113 These figures have been adapted from ref. 113 with permission from Springer Nature. | |
Protein engineering based on H2O2-driven enzyme inactivation mechanisms has also been intensively investigated with many other classes of enzymes. For instance, the protein energy landscape exploration (PELE) approach has been used for deciphering the pathways for the reaction substrate, O2, and H2O2 in vanillyl alcohol oxidase.115 The PELE simulation suggested that O2 and H2O2 might share the same pathway to enter or exit the active site of the enzyme, which is different from that of vanillyl alcohol. Thereby, O2 reactivity and H2O2 formation could be controlled by introducing an amino acid residue, which may facilitate or prevent the O2 positioning in the active site cavity.116,117
Recently, protein engineering of the lipase from Penicillium camembertii to improve its tolerance against H2O2 was reported.118 Molecular dynamics (MD) simulation of a number of H2O2 molecules in the enzyme active site led to the assumption that the rate of H2O2-related enzyme inactivation correlates with the amount and probability of H2O2 in the catalytic site. Further analysis using the MD trajectory of the enzyme revealed that the hydrogen peroxide molecules were preferentially located close to the residues Y21 and H144 forming hydrogen bonds with Y21, H144 and H259. The three nonpolar amino acid residues (i.e., G82, F256, and I260) in the vicinity of the H2O2 binding site were replaced by more hydrophilic amino acids to restrict access of H2O2 to the active site. One of the mutants (i.e., I260R) exhibited marked enhancements in H2O2 tolerance while maintaining the catalytic activity. These results suggested that H2O2 binding is still thermodynamically feasible but kinetically impeded thereby reducing the number of H2O2 molecules in the active site.
In summary, understanding of the H2O2-driven enzyme inactivation mechanisms and the major factors that affect the stability of the oxygenated intermediates will allow rational protein engineering to generate more robust enzymes for cascade biocatalysis under aerobic conditions.
2.3 Functional and balanced expression of the cascade enzymes
Oxygenases such as P450 monooxygenases, flavin monooxygenases, and lipoxygenases are usually difficult to express in a functional form in a bacterial cell because of the large complexity in their three-dimensional structure.20,90,119–124 Moreover, the turnover frequency and total turnover number of most oxygenases are significantly lower than those of other classes of enzymes. For instance, the kcat/KM value of the BVMO from P. putida KT2440 for 10-ketooctadecanoic acid (7) was approximately 1000-fold lower than that of the long-chain secondary alcohol dehydrogenase from Micrococcus luteus for 10-hydroxyoctadecanoic acid (6) (Scheme 2).113,114 As a result, oxygenation is found as a frequent rate-limiting step in the enzyme cascade reactions. Thereby, this section will focus on soluble and functional expression of oxygenases (e.g., BVMOs) in microbial cells.
A number of studies have been conducted to improve the functional expression of the BVMOs, in particular, from P. putida KT2440 and P. fluorescens DSM50106 in E. coli strains. For instance, directed evolution, fusion with soluble tags and enzymes, and coexpression with molecular chaperones as well as optimization of gene expression at both the transcriptional and the translational levels51,54,113,114,120,122,123,125–127 have been investigated to improve the soluble expression of the Pseudomonas sp. BVMOs. One of the striking results was obtained from application of the stable and tunable plasmid (STAPL) system.128 The STAPL system is based on a synthetic promoter and a synthetic auxotrophy using infA, encoding a translation initiation factor, as a selection marker to modulate plasmid copy numbers without antibiotics (Fig. 5). The STAPL system allowed fine-tuning of the expression level of the target enzymes (e.g., the BVMOs from P. putida KT2440) to an optimal level in E. coli.114 Moreover, the STAPL system appeared to increase the stability of the recombinant plasmid and thus the homogeneity of the recombinant E. coli population expressing the BVMOs.114 After all, the STAPL system allowed recombinant E. coli expressing the BVMO from P. putida KT2440 in addition to a fatty acid double-bond hydratase, a long-chain secondary alcohol dehydrogenase, and a long-chain fatty acid transporter, to ultimately produce C9 chemicals (i.e., n-nonanoic acid (9) and 9-hydroxynonanoic acid (10)) from oleic acid (Scheme 2), with a conversion of up to 20 μmol g−1 dry cells per min. This value was 2.4-fold greater than that of the control strain before engineering.
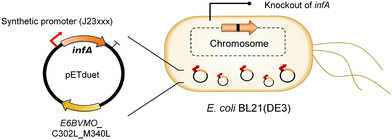 |
| Fig. 5 Schematic representation of the STAPL system-based E. coli biocatalysts.114 This figure has been adapted from ref. 114 with permission from Elsevier. | |
The balanced expression of the cascade enzymes is also important for the productive cascade enzyme-based whole-cell biocatalysis. Otherwise, the reaction intermediates may accumulate, resulting in an inhibition of the enzymes and/or toxification of the microbial cells. For instance, a toxic intermediate (i.e., 3-hydroxypropionaldehyde (34)) may accumulate during the biotransformation of glycerol into 3-hydroxypropionic acid (36) or 1,3-propanediol (35) (Schemes 8 and 9), if the total catalytic activity of glycerol dehydratases (GDHts) in a cell is greater than that of the aldehyde dehydrogenases or the alcohol dehydrogenases. To prevent the accumulation of 3-hydroxypropionaldehyde and to increase the productivity, Sankaranarayanan et al. have optimized the promoter strength in the GDHt expression system by using various synthetic promoters.129 3-Hydroxypropionaldehyde accumulation could be completely abolished when the aldehyde dehydrogenases were expressed to an approximately 8-fold higher level than that of GDHt. Another elegant approach was to improve the specific activity of the aldehyde dehydrogenases by applying a directed evolution approach.130 Seok et al. (2018)130 constructed a synthetic selection device using a 3-hydroxypropionic acid-responsive transcription factor to screen a hyper-producing E. coli cell. The method was applied to an aldehyde-binding site library of α-ketoglutaric semialdehyde dehydrogenase. The synthetic selection device allowed enrichment of the strains having a 2.8-fold greater active enzyme variant toward its aldehyde substrate than the wild-type one.
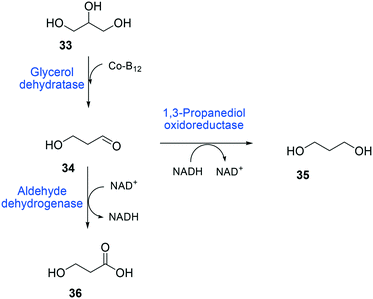 |
| Scheme 8 Biotransformation pathway of glycerol (33) into 3-hydroxypropionic acid (36).178 | |
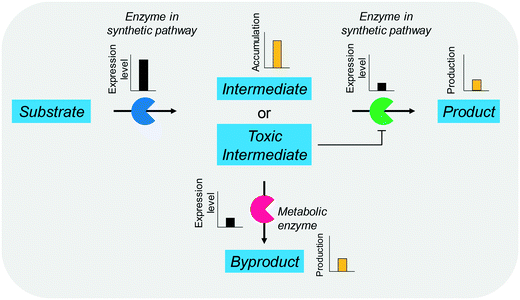 |
| Scheme 9 Influence of unbalanced expression of cascade enzymes on the yield of the final product. | |
2.4 Systematic engineering of the cascade enzyme-based whole-cell biocatalysts
The whole-cell biotransformation rates are influenced not only by the activity and stability of the cascade enzymes but also by transport of the reactants, regeneration of the cofactors, and toxic effects of the reactants and products on the microbial cells10,19,20,25,36,83 (Fig. 1). Thereby, all of these factors should be considered to reach high productivities and high product concentrations.
2.4.1 Transport of the reactants.
Transport of large hydrophobic compounds such as long-chain fatty acids and hydrocarbons into microbial cells is generally difficult because of their poor solubility and dispersibility in the aqueous medium and of low permeability of the cellular membranes. For instance, the outer layer of the outer membrane of Gram-negative bacteria was known as a major barrier to transport of large hydrophobic substances into the microbial cells. There could be a number of approaches to improve the transport efficiency of the hydrophobic molecules. One approach was to use surfactants to increase the dispersion of the hydrophobic substrates into the reaction medium.49,131,132 Another way was to increase cell membrane permeability by treatment of the microbial cells with organic solvents.133,134 In particular, the recently reported biocompatible natural based solvents such as deep eutectic mixtures (NADES)135,136 appear to be promising as solvents.
Julsing et al. (2012)137 reported an elegant method to improve mass transport efficiency of the hydrophobic substrates into E. coli cells. They used a transporter protein for hydrophobic molecules (i.e., AlkL from P. putida GPo1) through the cellular membrane. The AlkL system was able to facilitate transport of not only aliphatic alkanes (i.e. C7–C16 alkanes) but also fatty acid methyl esters (i.e. n-nonanoic acid methyl ester (1), dodecanoic acid methyl ester (16)) into E. coli cells.43,137–139 The long-chain fatty acid transporter FadL was successfully used to improve the transport efficiency of long-chain fatty acids such as oleic acid (5), linoleic acid (12), and ricinoleic acid (40) into E. coli cells.51,53 FadL expression was especially critical for the transport of fatty acids, which are solid under reaction conditions (e.g., 10-hydroxyoctadecanoic acid (6)) (Scheme 10).
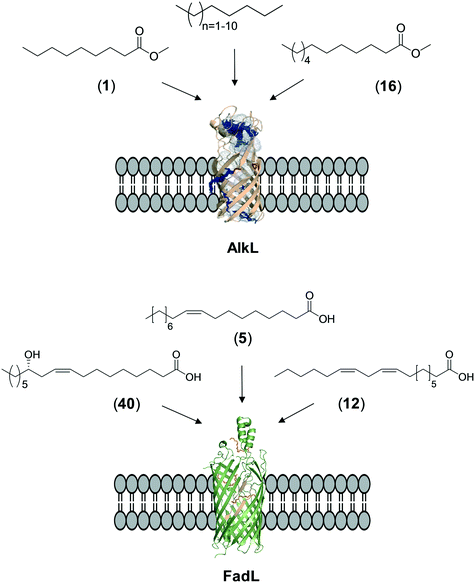 |
| Scheme 10 Transport of aliphatic alkanes, fatty acid methyl esters, and long-chain fatty acids by the AlkL138 and FadL (PDB code: 1T16)179 systems. | |
One of the key points in the introduction of a transporter protein in the cellular membrane would be optimization of the expression level. However, too high expression of a transporter protein may generate a negative effect on balanced expression of the cascade enzymes in microbial cells. For instance, excessive expression of FadL resulted in a decrease of the expression level of the BVMO of P. putida KT2440 in E. coli.53 In addition, the excessive expression may lead to too much uptake of the toxic reactants, generating undesired side effects. For instance, excessive expression of AlkL in the outer membrane has led to extensive uptake of dodecanoic acid methyl ester (16) and thus to toxification and deactivation of the recombinant E. coli-based biocatalysts140 (Scheme 11).
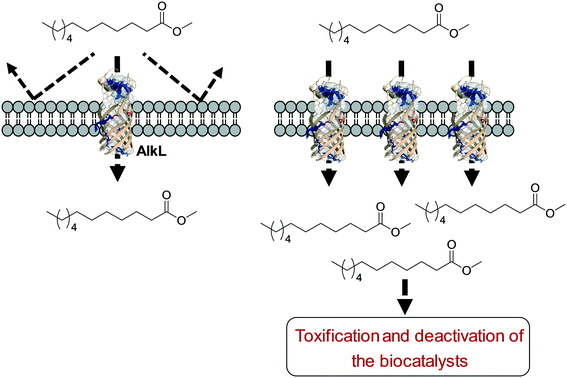 |
| Scheme 11 Influence of excessive expression of AlkL on the biocatalysts.138 | |
2.4.2 Regeneration of the cofactors.
The nicotinamide cofactors (e.g., NAD(P)H) are usually involved not only in reduction and oxidation of the reactants but also in activation of molecular oxygen for oxygenase-based biocatalysis (Schemes 1 and 2); they reduce other enzyme-bound cofactors (typically heme or flavin), which in turn react with molecular oxygen and thereby activate it for the oxygenation reaction. One of the best ways to regenerate the nicotinamide cofactors during whole-cell biotransformations would be to use cellular glucose metabolism (Fig. 6). Theoretically, 10 to 11 molecules of NAD(P)H can be regenerated from complete oxidation of one molecule of glucose into CO2.141,142 Transhydrogenases (e.g., PntAB of E. coli) may allow making NAD(P)H interconvertible in cells.143 Otherwise, glucose dehydrogenase or formate dehydrogenase can be used for the regeneration of NADPH and NADH, respectively, by using glucose or formate as a reductant.144 On the other hand, NAD(P)+ can be regenerated by NAD(P)H oxidase using O2 as an electron acceptor145–147 or by NAD(P)H dehydrogenases in the electron transport chain of microbial cells.
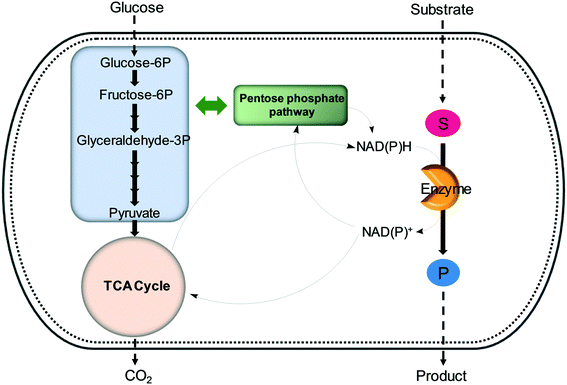 |
| Fig. 6 Regeneration of nicotinamide cofactors through cellular glucose metabolism during whole-cell biotransformations. | |
One of the elegant methods for cofactor regeneration in enzyme cascades may reside in the construction of a redox neutral multi-enzyme network by applying a suitable cofactor recycling system.12,37,64,69,148,149 For instance, a NAD+-dependent alcohol dehydrogenase from Bacillus stearothermophilus and an ω-transaminase from Chromobacterium violaceum were successfully coupled with a NADH-dependent L-alanine dehydrogenase from Bacillus subtilis for the production of amine products from the corresponding alcohols69 (Scheme 12). The L-alanine dehydrogenase served for recycling of the NADH cofactor as well as the amine donor (i.e., alanine) from pyruvate.150–152 Another elaborated example is a two-step enzyme cascade in parallel and “anti-sense” to transform ω-hydroxy fatty acids and α,ω-diols to the corresponding ω-amino fatty acids and α,ω-diamines, respectively, by recombinant E. coli expressing an aldehyde reductase and ω-transaminase64 (Scheme 5). Benzylamine served as an amine donor while its deamination product benzaldehyde served as an electron acceptor, which is released from the oxidation of ω-hydroxy fatty acids and α,ω-diols. C6 to C16 substrates (100 mM) could be converted into the corresponding products with >90% yield by this redox-neutral multi-step biotransformation.
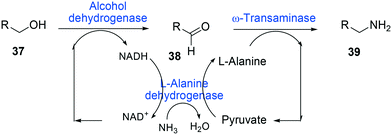 |
| Scheme 12 Biotransformation pathway of primary alcohols (37) into amines (39).69 | |
The self-sustaining redox neutral reaction systems are empowered by enzyme engineering to switch the cofactor affinity of the enzymes and/or by fusion with a cofactor-recycling system.145–147 The NADPH-dependent cyclohexanone monooxygenase (CHMO) from Acinetobacter sp. NCIMB 9871 has been changed to accept NADH as an electron donor by combining structure analysis, sequence alignment, and literature data.100 Two combinatorial variants having three or four mutations exhibited significantly greater conversion of cyclohexanone with NADH compared to NADPH. These variants may allow coupling with NAD+-dependent alcohol dehydrogenases.
2.4.3 Attenuation of the reactant and product toxicity.
Organic chemicals and hydrocarbons may cause damage (e.g., solvent stress) to the cellular membranes of microbial cells.153–159 For instance, toluene was reported to lead to formation of transient or permanent pores through interaction with the cellular membranes.153,160 These compounds may also disrupt the electron transport chain (ETC) and thus disturb energy generation by uncoupling phosphorylation. Carboxylic acids and fatty acids (e.g., n-heptanoic acid and n-octanoic acid) may cause not only solvent stress but also acid stress via intracellular acidification by generating protons within the cells, thereby resulting in the destabilization of proteins and DNA.141,154,155,157,158
Tolerance of microbial cells to the toxic fatty acids and hydrocarbons can be enhanced by metabolic and/or evolutionary engineering of the microbial solvent and acid resistance systems.156,161–164 For instance, the acid tolerance of E. coli BL21 to n-heptanoic acid was improved by engineering the glutamic acid-dependent acid resistance (GDAR) system, which is responsible for scavenging intracellular protons by catalyzing decarboxylation of glutamic acid.141,165 Activation of the GDAR system resulted in an increase of acid tolerance of E. coli BL21 to n-heptanoic acid. Furthermore, activation of the GDAR system allowed the recombinant E. coli BL21 cells expressing the long-chain alcohol dehydrogenase of M. luteus and the BVMO of P. putida to reach 60% greater product concentration in the biotransformation of ricinoleic acid into n-heptanoic acid (43) and 11-hydroxyundec-9-enoic acid (44) (Scheme 13).
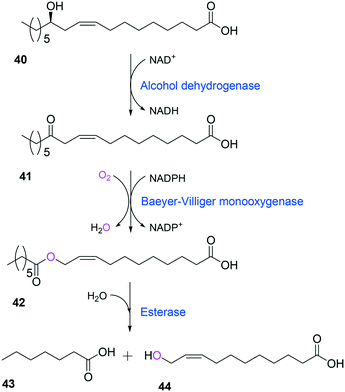 |
| Scheme 13 Biotransformation pathway of ricinoleic acid (40) into n-heptanoic acid (43) and 11-hydroxyundec-9-enoic acid (44).49,180 | |
Another approach was to express the human Caveolin-1 (CAV1) in E. coli, which is involved in eukaryotic endocytosis, to induce the formation of heterologous caveolae in the cytoplasmic membrane.166 The bacterial endocytosis led to alleviation of cytotoxicity of medium- to long-chain fatty acids in E. coli BL21 in addition to increase of fatty acid transport. The caveolae-forming E. coli biocatalysts showed greater biotransformation activity of ricinoleic acid or castor oil into (Z)-11-(heptanoyloxy)undec-9-enoic acid (Scheme 13) as compared to wild-type E. coli-based biocatalysts. Therefore, tolerance of whole-cell biocatalysts to the hydrophobic reactants and products is one of the key factors to determine productivities and product concentrations in the multi-step enzyme biotransformations.
3. Design and engineering of the cascade enzyme-based biocatalytic processes
3.1 Design of the biocatalysis systems
The biocatalysis systems for the multi-step syntheses may consist of multiple whole-cell systems,41,62 a combination of free enzymes and whole-cell biocatalysts,48,49,55 and a combination of enzyme and chemo-catalysts28–32,54,167 in addition to a typical single-cell system, depending on the nature of the reactants and the reactions. One of the representative examples for the combination of free enzymes and whole-cell biocatalysts may include the simultaneous enzyme/whole-cell biotransformation of 10,12-dihydroxyoctadecanoic acid (45) into 3-hydroxynonanoic acid (47) and 1,9-nonanedioic acid (11)48 (Scheme 14). The enzyme/whole-cell biotransformation was initiated by adding the dihydroxy fatty acid (45) and an esterase from P. fluorescens into the culture medium of the recombinant E. coli expressing the long-chain fatty acid transporter FadL and the cascade enzymes (i.e., long-chain secondary alcohol dehydrogenase (SADH), BVMO, primary alcohol dehydrogenase (PADH), and aldehyde dehydrogenase (AlDH)) (Scheme 14). The esterase from P. fluorescens was directly added into the reaction medium because the ester compound (46) was excreted out of the cells. The reactions proceeded as follows: the dihydroxy fatty acid (45) is transformed into the ester (46) by intracellular SADH and BVMO, which is then subjected to hydrolysis by the extracellular esterases. The hydrolysis products (10) enter into the E. coli cells and are further oxidized to 1,9-nonanedioic acid (11) by the intracellular PADH and AlDH. Ultimately, 3-hydroxynonanoic acid (47) and 1,9-nonanedioic acid (11) were produced to an overall rate of over 20 μmol product per g dry cells per min (U g−1 dry cells).48
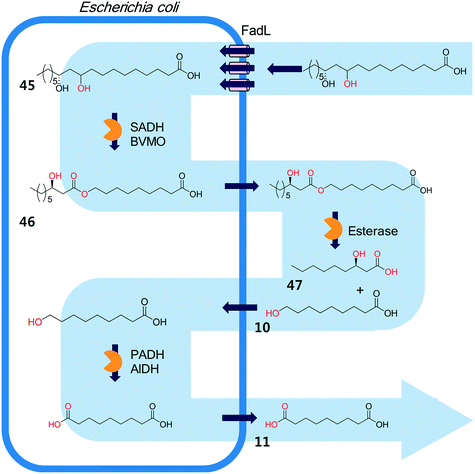 |
| Scheme 14 The simultaneous enzyme/whole-cell biotransformation of 10,12-dihydroxyoctadecanoic acid (45) into 3-hydroxynonanoic acid (47) and 1,9-nonanedioic acid (11).48 This scheme has been reproduced from ref. 48 with permission from John Wiley and Sons. | |
Combinations of enzymatic and chemical transformations can be useful to conduct multi-step reactions, in which the reactants and/or products are toxic and thus able to inactivate the whole-cell biocatalysts.28,29,32,54,167 One example is the chemo-enzymatic synthesis of n-nonanoic acid (10) and 1,9-nonanedioic acid (11) (Scheme 2). Since n-nonanoic acid (9) has not only strong inhibition to the enzymatic hydrolysis of the ester (8) but is also toxic to microbial cells (see section 1.4.3 for details), it was difficult to produce the target products at a high concentration. Thereby, the ester (8) was first over-produced from oleic acid (5) by the recombinant E. coli expressing a fatty acid double bond hydratase, SADH, and BVMO. The esters were then subjected to chemical hydrolysis and oxidation into n-nonanoic acid (9) and 1,9-nonanedioic acid (11).54 Other many interesting examples as well as opportunities and challenges for combining chemo- and biocatalysis have been reviewed recently by Rudroff et al.28 and by Gröger.29
3.2 Attenuation of the reactant and product toxicity
One of the engineering targets for the whole-cell biotransformation processes may include increase of mass transport and alleviation of reactant and product toxicity to reach high productivities and high product concentrations. It is especially true for the cascade enzyme whole-cell biocatalytic processes having hydrophobic substances as starting materials, as discussed in section 1.4. This section will thus focus on attenuation of the reactant and product toxicity at the process engineering level.
3.2.1 Organic/aqueous two-liquid phase system.
Non-toxic organic solvents can be used for in situ extraction of toxic products from an aqueous reaction medium and/or controlled release of the reactants. In particular, non-toxic deep eutectic mixtures have been recently applied for enzymatic and whole-cell mediated bioprocesses.135,168–172 Moreover, screening of a suitable organic solvent for the bioprocesses using aqueous–organic two phase systems was recently reviewed by Grundtvig et al. (2018).170 One of the representative examples is the terminal oxyfunctionalization of medium-chain fatty acid methyl esters (e.g., nonanoic acid methyl ester and dodecanoic acid methyl ester (DAME)) by recombinant E. coli expressing AlkBGT of P. putida GPo1 (ref. 43, 44 and 68) (Schemes 1 and 4). The use of bis(2-ethylhexyl) phthalate (BEHP) as an organic carrier solvent allowed controlled supply of the reaction substrate (16) and in situ extraction of the acid product (i.e., dodecanedioic acid methyl ester (DDAME) (20)) from the aqueous reaction medium (Scheme 4) (Fig. 7). As a result, the recombinant E. coli expressing AlkBGT was able to produce DDAME (20) to a concentration of 117 mM.43
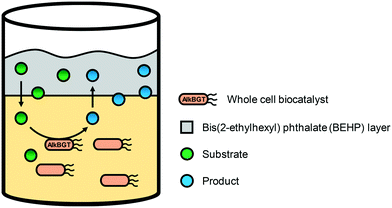 |
| Fig. 7 Schematic diagram of an organic/aqueous two-liquid phase reaction system. | |
On the other hand, the major product of the recombinant E. coli expressing AlkBGT was changed from DDAME (20) to 12-hydroxydodecanoic acid methyl ester (HDAME) (17) when the reaction substrate (DAME) was used as the bulk organic phase in stirred tank bioreactors.140 Low acid (20) formation and predominant HDAME (17) accumulation was explained by the saturation of AlkB with DAME (16) and/or the extraction of HDAME (17) and especially 12-oxododecanoic acid methyl ester (18) to the organic phase. Overall, the organic/aqueous two-liquid phase system enabled one not only to improve the stability of the E. coli-based whole-cell biocatalyst but also to direct the multistep oxyfunctionalization of DAME towards the exclusive formation of alcohol (17) or acid (20).
3.2.2 Adsorbent resin-based biotransformation system.
Another approach may include in situ recovery of the hydrophobic products and controlled release of the reactants via adsorption and diffusion onto a hydrophobic absorbent resin.173–175 For example, the cyclic ketone bicyclo[3.2.0]hept-2-ene-6-one was converted into a mixture of the two corresponding lactones to a high concentration by recombinant E. coli expressing the CHMO from Acinetobacter sp. NCIMB 9871 in the presence of a hydrophobic absorbent resin.176 The resin-based whole-cell biotransformation process allowed high product concentration and bioconversion yield of ca. 16 g L−1 and 83%, respectively, by attenuating product toxicity via in situ product recovery with 100 g L−1 hydrophobic absorbent resins.
The hydrophobic absorbent resins were also successfully used to alleviate the toxic effects of oleic acid and its derivatives as well as n-nonanoic acid and 9-hydroxynonanoic acid during oleic acid biotransformation by recombinant E. coli-based biocatalysts (Scheme 2). The in situ recovery of n-nonanoic acid and controlled release of the reactants allowed the whole-cell biocatalysts to produce n-nonanoic acid and 9-hydroxynonanoic acid to over 200 mM in the aqueous medium.177
4. Conclusions
The productivities of cascade enzyme whole-cell biocatalysis can be influenced by a number of factors, including activity and stability of the cascade enzymes in whole cells, transport of the reactants, regeneration of the cofactors, equilibrium thermodynamics, background reactions by metabolic enzymes and/or by-product formation as well as cellular toxicity of the reactants and products. These factors can be optimized by multi-layer engineering of the cascade enzymes, whole cells, reaction conditions and bioreaction engineering strategies. The discovery of new enzymes and the design of novel biotransformation pathways play a key role in the performance of cascade enzyme whole-cell biocatalytic processes. Synthetic biology, systems biology, and bioinformatics can further facilitate the multi-layer engineering and discovery of new enzymes needed for efficient novel enzyme-based processes.
Conflicts of interest
The authors declare no conflict of interest.
Acknowledgements
This study was supported by the C1 Gas Refinery Research Center (NRF Grant Number: 2018M3D3A1A01055735) of the National Research Foundation (NRF) of Korea funded by the Ministry of Science and ICT. J.-W. Song was partially supported by RP-Grant 2019 of Ewha Womans University. J.-H. Seo was supported by the Basic Science Research Program through the National Research Foundation of Korea (NRF) funded by the Ministry of Education (2017R1D1A1B03033525).
References
- N. J. Turner and E. O'Reilly, Nat. Chem. Biol., 2013, 9, 285 CrossRef CAS.
- S. P. France, L. J. Hepworth, N. J. Turner and S. L. Flitsch, ACS Catal., 2017, 7, 710–724 CrossRef CAS.
- F. H. Arnold, Angew. Chem., Int. Ed., 2019, 58, 14420–14426 CrossRef CAS PubMed.
- H. A. Bunzel, H. Kries, L. Marchetti, C. Zeymer, P. R. E. Mittl, A. J. Mulholland and D. Hilvert, J. Am. Chem. Soc., 2019, 141, 11745–11748 CrossRef CAS.
- Y. Wang, H. Ren and H. Zhao, Crit. Rev. Biochem. Mol. Biol., 2018, 53, 115–129 CrossRef CAS.
- L. Schmermund, V. Jurkaš, F. F. Özgen, G. D. Barone, H. C. Büchsenschütz, C. K. Winkler, S. Schmidt, R. Kourist and W. Kroutil, ACS Catal., 2019, 9, 4115–4144 CrossRef CAS.
- L. Chuaboon, T. Wongnate, P. Punthong, C. Kiattisewee, N. Lawan, C.-Y. Hsu, C.-H. Lin, U. T. Bornscheuer and P. Chaiyen, Angew. Chem., Int. Ed., 2019, 58, 2428–2432 CrossRef CAS.
- R. A. Langan, S. E. Boyken, A. H. Ng, J. A. Samson, G. Dods, A. M. Westbrook, T. H. Nguyen, M. J. Lajoie, Z. Chen, S. Berger, V. K. Mulligan, J. E. Dueber, W. R. P. Novak, H. El-Samad and D. Baker, Nature, 2019, 572, 205–210 CrossRef CAS.
- A. H. Ng, T. H. Nguyen, M. Gomez-Schiavon, G. Dods, R. A. Langan, S. E. Boyken, J. A. Samson, L. M. Waldburger, J. E. Dueber, D. Baker and H. El-Samad, Nature, 2019, 572, 265–269 CrossRef CAS.
- S. Pontrelli, T. Y. Chiu, E. I. Lan, F. Y. H. Chen, P. C. Chang and J. C. Liao, Metab. Eng., 2018, 50, 16–46 CrossRef CAS.
- X. L. Chen, C. Gao, L. Guo, G. P. Hu, Q. L. Luo, J. Liu, J. Nielsen, J. Chen and L. M. Liu, Chem. Rev., 2018, 118, 4–72 CrossRef CAS.
- J. Muschiol, C. Peters, N. Oberleitner, M. D. Mihovilovic, U. T. Bornscheuer and F. Rudroff, Chem. Commun., 2015, 51, 5798–5811 RSC.
- K. R. Choi, W. D. Jang, D. Yang, J. S. Cho, D. Park and S. Y. Lee, Trends Biotechnol., 2019, 37, 817–837 CrossRef CAS.
- S. Y. Lee, H. U. Kim, T. U. Chae, J. S. Cho, J. W. Kim, J. H. Shin, D. I. Kim, Y.-S. Ko, W. D. Jang and Y.-S. Jang, Nat. Catal., 2019, 2, 18–33 CrossRef CAS.
- E. K. R. Hanko, C. M. Denby, I. N. V. Sanchez, W. Lin, K. J. Ramirez, C. A. Singer, G. T. Beckham and J. D. Keasling, Metab. Eng., 2018, 48, 52–62 CrossRef CAS.
- A. V. Lis, K. Schneider, J. Weber, J. D. Keasling, M. K. Jensen and T. Klein, Microb. Cell Fact., 2019, 18, 50 CrossRef.
- T. U. Chae, J. H. Ahn, Y. S. Ko, J. W. Kim, J. A. Lee, E. H. Lee and S. Y. Lee, Metab. Eng., 2019 DOI:10.1016/j.ymben.2019.03.005 , in press.
- Q. Yan and B. F. Pfleger, Metab. Eng., 2019 DOI:10.1016/j.ymben.2019.04.009 , in press.
- F. Rudroff, Curr. Opin. Chem. Biol., 2019, 49, 84–90 CrossRef CAS.
-
Y. J. Yeon and J.-B. Park, in Lipid Modification by Enzymes and Engineered Microbes, AOCS Press, 2018, pp. 139–155 Search PubMed.
- J. M. Sperl and V. Sieber, ACS Catal., 2018, 8, 2385–2396 CrossRef CAS.
- P.-W. Seo, H.-J. Cho, H.-Y. Jeong, J.-H. Kim, J.-W. Kim, E.-Y. Lee, J.-B. Park and J.-S. Kim, Catal. Sci. Technol., 2019 10.1039/C9CY01566C , in press.
- J. H. Schrittwieser, S. Velikogne, M. Hall and W. Kroutil, Chem. Rev., 2018, 118, 270–348 CrossRef CAS.
- H. H. Sun, H. F. Zhang, E. L. Ang and H. M. Zhao, Bioorg. Med. Chem., 2018, 26, 1275–1284 CrossRef CAS.
- S. Wu and Z. Li, ChemCatChem, 2018, 10, 2164–2178 CrossRef CAS.
- H. J. Cha, J. B. Park and S. Park, Biotechnol. Bioprocess Eng., 2019, 24, 41–47 CrossRef CAS.
- L. Zhang, R. Singh, S. Dakshinamurthy, Z. Guo, J. Li, F. Chen, Y. He, X. Guan, Y. C. Kang and J.-K. Lee, Green Chem., 2018, 20, 230–242 RSC.
- F. Rudroff, M. D. Mihovilovic, H. Groger, R. Snajdrova, H. Iding and U. T. Bornscheuer, Nat. Catal., 2018, 1, 12–22 CrossRef.
-
H. Gröger, in Modern Biocatalysis: Advances Towards Synthetic Biological Systems, The Royal Society of Chemistry, 2018, pp. 439–472 Search PubMed.
- H. Groger and W. Hummel, Curr. Opin. Chem. Biol., 2014, 19, 171–179 CrossRef PubMed.
- C. A. Denard, J. F. Hartwig and H. M. Zhao, ACS Catal., 2013, 3, 2856–2864 CrossRef CAS.
- Z. C. Litman, Y. J. Wang, H. M. Zhao and J. F. Hartwig, Nature, 2018, 560, 355–359 CrossRef CAS.
- F. Dumeignil, M. Guehl, A. Gimbernat, M. Capron, N. L. Ferreira, R. Froidevaux, J.-S. Girardon, R. Wojcieszak, P. Dhulster and D. Delcroix, Catal. Sci. Technol., 2018, 8, 5708–5734 RSC.
-
U. T. Bornscheuer, in Lipid Modification by Enzymes and Engineered Microbes, AOCS Press, 2018 Search PubMed.
-
M. W. Fraaije and D. B. Janssen, in Modern Biooxidation: Enzymes, Reactions Applications, Wiley-VCH, 2007, pp. 77–97 Search PubMed.
- S. K. Kim and Y. C. Park, Appl. Microbiol. Biotechnol., 2019, 103, 191–199 CrossRef CAS.
- A. Kohl, V. Srinivasamurthy, D. Böttcher, J. Kabisch and U. T. Bornscheuer, Enzyme Microb. Technol., 2018, 108, 53–58 CrossRef CAS.
- J. Wachtmeister and D. Rother, Curr. Opin. Biotechnol., 2016, 42, 169–177 CrossRef CAS.
- F.-L. Du, H.-L. Yu, J.-H. Xu and C.-X. Li, Bioresour. Bioprocess., 2014, 1, 10 CrossRef.
- T. Furuya, M. Miura and K. Kino, ChemBioChem, 2014, 15, 2248–2254 CrossRef CAS.
- N. Oberleitner, A. K. Ressmann, K. Bica, P. Gärtner, M. W. Fraaije, U. T. Bornscheuer, F. Rudroff and M. D. Mihovilovic, Green Chem., 2017, 19, 367–371 RSC.
- S. Schaffer and T. Haas, Org. Process Res. Dev., 2014, 18, 752–766 CrossRef CAS.
- M. Schrewe, M. K. Julsing, K. Lange, E. Czarnotta, A. Schmid and B. Buhler, Biotechnol. Bioeng., 2014, 111, 1820–1830 CrossRef CAS.
- M. Schrewe, A. O. Magnusson, C. Willrodt, B. Bühler and A. Schmid, Adv. Synth. Catal., 2011, 353, 3485–3495 CrossRef CAS.
- B. Buhler and A. Schmid, J. Biotechnol., 2004, 113, 183–210 CrossRef PubMed.
- W. Lu, J. E. Ness, W. Xie, X. Zhang, J. Minshull and R. A. Gross, J. Am. Chem. Soc., 2010, 132, 15451–15455 CrossRef CAS PubMed.
- D. L. Craft, K. M. Madduri, M. Eshoo and C. R. Wilson, Appl. Environ. Microbiol., 2003, 69, 5983–5991 CrossRef CAS PubMed.
- H.-J. Cha, E.-J. Seo, J.-W. Song, H.-J. Jo, A. R. Kumar and J.-B. Park, Adv. Synth. Catal., 2018, 360, 696–703 CrossRef CAS.
- J.-W. Song, E.-Y. Jeon, D.-H. Song, H.-Y. Jang, U. T. Bornscheuer, D.-K. Oh and J.-B. Park, Angew. Chem., Int. Ed., 2013, 52, 2534–2537 CrossRef CAS.
- P. D. V. N. Sudheer, J. Yun, S. Chauhan, T. J. Kang and K. Y. Choi, Biotechnol. Bioprocess Eng., 2017, 22, 717–724 CrossRef CAS.
- E.-Y. Jeon, J.-H. Seo, W.-R. Kang, M.-J. Kim, J.-H. Lee, D.-K. Oh and J.-B. Park, ACS Catal., 2016, 6, 7547–7553 CrossRef CAS.
- K. B. Otte, M. Kirtz, B. M. Nestl and B. Hauer, ChemSusChem, 2013, 6, 2149–2156 CrossRef CAS.
- E.-Y. Jeon, J.-W. Song, H.-J. Cha, S.-M. Lee, J. Lee and J.-B. Park, J. Biotechnol., 2018, 281, 161–167 CrossRef CAS.
- S. Koppireddi, J.-H. Seo, E.-Y. Jeon, P. S. Chowdhury, H.-Y. Jang, J.-B. Park and Y.-U. Kwon, Adv. Synth. Catal., 2016, 358, 3084–3092 CrossRef CAS.
- E.-J. Seo, Y. J. Yeon, J.-H. Seo, J.-H. Lee, J. P. Boñgol, Y. Oh, J. M. Park, S.-M. Lim, C.-G. Lee and J.-B. Park, Bioresour. Technol., 2018, 251, 288–294 CrossRef CAS.
- B. Hartel, P. Ludwig, T. Schewe and S. M. Rapoport, Eur. J. Biochem., 1982, 126, 353–357 CrossRef CAS PubMed.
- M. R. Kim, S. H. Kim and D. E. Sok, Biochem. Biophys. Res. Commun., 1989, 164, 1384–1390 CrossRef CAS PubMed.
- S. Rapoport, B. Hartel and G. Hausdorf, Eur. J. Biochem., 1984, 139, 573–576 CrossRef CAS PubMed.
- K. Kishimoto, M. Nakamura, H. Suzuki, T. Yoshimoto, S. Yamamoto, T. Takao, Y. Shimonishi and T. Tanabe, Biochim. Biophys. Acta, 1996, 1300, 56–62 CrossRef.
- R. Wiesner, H. Suzuki, M. Walther, S. Yamamoto and H. Kuhn, Free Radical Biol. Med., 2003, 34, 304–315 CrossRef CAS.
- D.-S. Lee, J.-W. Song, M. Voß, E. Schuiten, R. K. Akula, Y.-U. Kwon, U. Bornscheuer and J.-B. Park, Adv. Synth. Catal., 2019, 361, 1359–1367 CrossRef CAS.
- J.-W. Song, J.-H. Lee, U. T. Bornscheuer and J.-B. Park, Adv. Synth. Catal., 2014, 356, 1782–1788 CrossRef CAS.
- M. Genz, C. Vickers, T. Van den Bergh, H.-J. Joosten, M. Dörr, M. Höhne and U. T. Bornscheuer, Int. J. Mol. Sci., 2015, 16, 26953–26963 CrossRef CAS.
- S. Sung, H. Jeon, S. Sarak, M. M. Ahsan, M. D. Patil, W. Kroutil, B.-G. Kim and H. Yun, Green Chem., 2018, 20, 4591–4595 RSC.
- O. Mayol, K. Bastard, L. Beloti, A. Frese, J. P. Turkenburg, J.-L. Petit, A. Mariage, A. Debard, V. Pellouin, A. Perret, V. de Berardinis, A. Zaparucha, G. Grogan and C. Vergne-Vaxelaire, Nat. Catal., 2019, 2, 324–333 CrossRef CAS.
- V. Tseliou, M. F. Masman, W. Bohmer, T. Knaus and F. G. Mutti, ChemBioChem, 2019, 20, 800–812 CrossRef CAS.
- X. J. Yin, Y. Y. Liu, L. J. Meng, H. S. Zhou, J. P. Wu and L. R. Yang, Adv. Synth. Catal., 2019, 361, 803–812 CrossRef CAS.
- N. Ladkau, M. Assmann, M. Schrewe, M. K. Julsing, A. Schmid and B. Buhler, Metab. Eng., 2016, 36, 1–9 CrossRef CAS.
- J. H. Sattler, M. Fuchs, K. Tauber, F. G. Mutti, K. Faber, J. Pfeffer, T. Haas and W. Kroutil, Angew. Chem., Int. Ed., 2012, 51, 9156–9159 CrossRef CAS PubMed.
- I. Slabu, J. L. Galman, R. C. Lloyd and N. J. Turner, ACS Catal., 2017, 7, 8263–8284 CrossRef CAS.
- A. Romero-Rivera, M. Garcia-Borràs and S. Osuna, Chem. Commun., 2017, 53, 284–297 RSC.
- F. Steffen-Munsberg, C. Vickers, H. Kohls, H. Land, H. Mallin, A. Nobili, L. Skalden, T. van den Bergh, H. J. Joosten, P. Berglund, M. Hohne and U. T. Bornscheuer, Biotechnol. Adv., 2015, 33, 566–604 CrossRef CAS PubMed.
- K. Balke, A. Beier and U. T. Bornscheuer, Biotechnol. Adv., 2018, 36, 247–263 CrossRef CAS PubMed.
- M. Bucko, P. Gemeiner, A. Schenkmayerova, T. Krajcovic, F. Rudroff and M. D. Mihovilovic, Appl. Microbiol. Biotechnol., 2016, 100, 6585–6599 CrossRef CAS PubMed.
- H. Leisch, K. Morley and P. C. K. Lau, Chem. Rev., 2011, 111, 4165–4222 CrossRef CAS PubMed.
- G. de Gonzalo, M. D. Mihovilovic and M. W. Fraaije, ChemBioChem, 2010, 11, 2208–2231 CrossRef CAS PubMed.
- M. D. Mihovilovic, B. Muller and P. Stanetty, Eur. J. Org. Chem., 2002, 3711–3730 CrossRef CAS.
- B. J. Yachnin, T. Sprules, M. B. McEvoy, P. C. Lau and A. M. Berghuis, J. Am. Chem. Soc., 2012, 134, 7788–7795 CrossRef CAS PubMed.
- R. M. Demming, M. P. Fischer, J. Schmid and B. Hauer, Curr. Opin. Chem. Biol., 2018, 43, 43–50 CrossRef CAS PubMed.
- M. Engleder, T. Pavkov-Keller, A. Emmerstorfer, A. Hromic, S. Schrempf, G. Steinkellner, T. Wriessnegger, E. Leitner, G. A. Strohmeier, I. Kaluzna, D. Mink, M. Schurmann, S. Wallner, P. Macheroux, K. Gruber and H. Pichler, ChemBioChem, 2015, 16, 1730–1734 CrossRef CAS PubMed.
- J. Ortega-Anaya and A. Hernández-Santoyo, Biochim. Biophys. Acta, Biomembr., 2015, 1848, 3166–3174 CrossRef CAS PubMed.
- A. Volkov, S. Khoshnevis, P. Neumann, C. Herrfurth, D. Wohlwend, R. Ficner and I. Feussner, Acta Crystallogr., Sect. D: Biol. Crystallogr., 2013, 69, 648–657 CrossRef CAS PubMed.
- J. H. Seo, S. M. Lee, J. Lee and J. B. Park, J. Biotechnol., 2015, 216, 158–166 CrossRef CAS PubMed.
- S. U. Kim, K. R. Kim, J. W. Kim, S. Kim, Y. U. Kwon, D. K. Oh and J. B. Park, J. Agric. Food Chem., 2015, 63, 2773–2781 CrossRef CAS PubMed.
- H.-J. Oh, S.-U. Kim, J.-W. Song, J.-H. Lee, W.-R. Kang, Y.-S. Jo, K.-R. Kim, U. T. Bornscheuer, D.-K. Oh and J.-B. Park, Adv. Synth. Catal., 2015, 357, 408–416 CrossRef CAS.
- Y. C. Joo and D. K. Oh, Biotechnol. Adv., 2012, 30, 1524–1532 CrossRef CAS PubMed.
- J.-U. An, Y.-S. Song, K.-R. Kim, Y.-J. Ko, D.-Y. Yoon and D.-K. Oh, Nat. Commun., 2018, 9, 128 CrossRef PubMed.
- I.-G. Lee, J.-U. An, Y.-J. Ko, J.-B. Park and D.-K. Oh, Green Chem., 2019, 21, 3172–3181 RSC.
-
J. Rapp, Master of Science, University of Toledo, 2006.
- J. U. An, S. H. Hong and D. K. Oh, Biochim. Biophys. Acta, Mol. Cell Biol. Lipids, 2018, 1863, 823–833 CrossRef CAS PubMed.
- L. Eggeling, M. Bott and J. Marienhagen, Curr. Opin. Biotechnol., 2015, 35, 30–36 CrossRef CAS PubMed.
- S. Raman, J. K. Rogers, N. D. Taylor and G. M. Church, Proc. Natl. Acad. Sci. U. S. A., 2014, 111, 17803–17808 CrossRef CAS PubMed.
- M. Schallmey, J. Frunzke, L. Eggeling and J. Marienhagen, Curr. Opin. Biotechnol., 2014, 26, 148–154 CrossRef CAS PubMed.
- S. L. Choi, E. Rha, S. J. Lee, H. Kim, K. Kwon, Y. S. Jeong, Y. H. Rhee, J. J. Song, H. S. Kim and S. G. Lee, ACS Synth. Biol., 2014, 3, 163–171 CrossRef CAS PubMed.
- T. van Rossum, S. W. M. Kengen and J. van der Oost, FEBS J., 2013, 280, 2979–2996 CrossRef CAS PubMed.
- E. van der Helm, H. J. Genee and M. O. A. Sommer, Nat. Chem. Biol., 2018, 14, 752–759 CrossRef CAS PubMed.
- S.-J. Yeom, M. Kim, K. K. Kwon, Y. Fu, E. Rha, S.-H. Park, H. Lee, H. Kim, D.-H. Lee, D.-M. Kim and S.-G. Lee, Nat. Commun., 2018, 9, 5053 CrossRef PubMed.
- S. Chakraborty, M. Ortiz-Maldonado, B. Entsch and D. P. Ballou, Biochemistry, 2010, 49, 372–385 CrossRef CAS PubMed.
- M. Ortiz-Maldonado, B. Entsch and D. P. Ballou, Biochemistry, 2004, 43, 15246–15257 CrossRef CAS PubMed.
- A. Beier, S. Bordewick, M. Genz, S. Schmidt, T. Van Den Bergh, C. Peters, H. J. Joosten and U. T. Bornscheuer, ChemBioChem, 2016, 17, 2312–2315 CrossRef CAS PubMed.
- W. P. Dijkman, G. de Gonzalo, A. Mattevi and M. W. Fraaije, Appl. Microbiol. Biotechnol., 2013, 97, 5177–5188 CrossRef CAS PubMed.
- D. Holtmann and F. Hollmann, ChemBioChem, 2016, 17, 1391–1398 CrossRef CAS PubMed.
- L. K. Morlock, D. Bottcher and U. T. Bornscheuer, Appl. Microbiol. Biotechnol., 2018, 102, 985–994 CrossRef CAS PubMed.
- W. J. H. van Berkel, N. M. Kamerbeek and M. W. Fraaije, J. Biotechnol., 2006, 124, 670–689 CrossRef CAS PubMed.
- E. Romero, J. R. Gómez Castellanos, G. Gadda, M. W. Fraaije and A. Mattevi, Chem. Rev., 2018, 118, 1742–1769 CrossRef CAS PubMed.
- E. R. Stadtman and R. L. Levine, Amino Acids, 2003, 25, 207–218 CrossRef CAS PubMed.
- M. Finnegan, E. Linley, S. P. Denyer, G. McDonnell, C. Simons and J. Y. Maillard, J. Antimicrob. Chemother., 2010, 65, 2108–2115 CrossRef CAS PubMed.
- R. Orru, H. M. Dudek, C. Martinoli, D. E. Torres Pazmiño, A. Royant, M. Weik, M. W. Fraaije and A. Mattevi, J. Biol. Chem., 2011, 286, 29284–29291 CrossRef CAS PubMed.
- A. Alfieri, E. Malito, R. Orru, M. W. Fraaije and A. Mattevi, Proc. Natl. Acad. Sci. U. S. A., 2008, 105, 6572–6577 CrossRef CAS PubMed.
- C. A. McDonald, R. L. Fagan, F. Collard, V. M. Monnier and B. A. Palfey, J. Am. Chem. Soc., 2011, 133, 16809–16811 CrossRef CAS PubMed.
- D. J. Opperman and M. T. Reetz, ChemBioChem, 2010, 11, 2589–2596 CrossRef CAS PubMed.
- O. B. Fordwour and K. R. Wolthers, Arch. Biochem. Biophys., 2018, 654, 85–96 CrossRef CAS PubMed.
- J.-M. Woo, E.-Y. Jeon, E.-J. Seo, J.-H. Seo, D.-Y. Lee, Y. J. Yeon and J.-B. Park, Sci. Rep., 2018, 8, 10280 CrossRef PubMed.
- E. J. Seo, C. W. Kang, J. M. Woo, S. Jang, Y. J. Yeon, G. Y. Jung and J. B. Park, Metab. Eng., 2019, 54, 137–144 CrossRef CAS PubMed.
- G. Gygli, M. F. Lucas, V. Guallar and W. J. H. van Berkel, PLoS Comput. Biol., 2017, 13(10), e1005787 CrossRef PubMed.
- A. Hernández-Ortega, F. Lucas, P. Ferreira, M. Medina, V. Guallar and A. T. Martínez, Biochemistry, 2012, 51, 6595–6608 CrossRef PubMed.
- A. Hernández-Ortega, F. Lucas, P. Ferreira, M. Medina, V. Guallar and A. T. Martínez, J. Biol. Chem., 2011, 286, 41105–41114 CrossRef PubMed.
- Z. Zhao, D. Lan, X. Tan, F. Hollmann, U. T. Bornscheuer, B. Yang and Y. Wang, ACS Catal., 2019, 9, 2916–2921 CrossRef CAS.
- Y. Wei, E. L. Ang and H. Zhao, Curr. Opin. Chem. Biol., 2018, 43, 1–7 CrossRef CAS PubMed.
- J. H. Seo, H. H. Kim, E. Y. Jeon, Y. H. Song, C. S. Shin and J. B. Park, Sci. Rep., 2016, 6, 28223 CrossRef CAS PubMed.
- M. T. Lundemo and J. M. Woodley, Appl. Microbiol. Biotechnol., 2015, 99, 2465–2483 CrossRef CAS PubMed.
- A. Kirschner, J. Altenbuchner and U. T. Bornscheuer, Appl. Microbiol. Biotechnol., 2007, 73, 1065–1072 CrossRef CAS PubMed.
- J. Rehdorf, A. Kirschner and U. T. Bornscheuer, Biotechnol. Lett., 2007, 29, 1393–1398 CrossRef CAS PubMed.
- J. U. An and D. K. Oh, J. Lipid Res., 2018, 59, 2153–2163 CrossRef CAS PubMed.
- J.-W. Song, J.-M. Woo, G. Y. Jung, U. T. Bornscheuer and J.-B. Park, Sci. Rep., 2016, 6, 29406 CrossRef PubMed.
- A. Kirschner and U. T. Bornscheuer, Appl. Microbiol. Biotechnol., 2008, 81, 465–472 CrossRef CAS PubMed.
- A. H. Baek, E. Y. Jeon, S. M. Lee and J. B. Park, Biotechnol. Bioeng., 2015, 112, 889–895 CrossRef CAS PubMed.
- C. W. Kang, H. G. Lim, J. Yang, M. H. Noh, S. W. Seo and G. Y. Jung, Metab. Eng., 2018, 48, 121–128 CrossRef CAS PubMed.
- M. Sankaranarayanan, A. Somasundar, E. Seol, A. S. Chauhan, S. Kwon, G. Y. Jung and S. Park, J. Biotechnol., 2017, 259, 140–147 CrossRef CAS PubMed.
- J. Y. Seok, J. Yang, S. J. Choi, H. G. Lim, U. J. Choi, K. J. Kim, S. Park, T. H. Yoo and G. Y. Jung, Metab. Eng., 2018, 47, 113–120 CrossRef CAS.
- H. Pfruender, R. Jones and D. Weuster-Botz, J. Biotechnol., 2006, 124, 182–190 CrossRef CAS PubMed.
- E.-Y. Jeon, J.-H. Lee, K.-M. Yang, Y.-C. Joo, D.-K. Oh and J.-B. Park, Process Biochem., 2012, 47, 941–947 CrossRef CAS.
- H. J. Oh, K. C. Shin and D. K. Oh, Biotechnol. Lett., 2013, 35, 1487–1493 CrossRef CAS PubMed.
- R. Leon, P. Fernandes, H. M. Pinheiro and J. M. S. Cabral, Enzyme Microb. Technol., 1998, 23, 483–500 CrossRef CAS.
- Á. Mourelle-Insua, I. Lavandera and V. Gotor-Fernández, Green Chem., 2019, 21, 2946–2951 RSC.
- T.-X. Yang, L.-Q. Zhao, J. Wang, G.-L. Song, H.-M. Liu, H. Cheng and Z. Yang, ACS Sustainable Chem. Eng., 2017, 5, 5713–5722 CrossRef CAS.
- M. K. Julsing, M. Schrewe, S. Cornelissen, I. Hermann, A. Schmid and B. Buhler, Appl. Environ. Microbiol., 2012, 78, 5724–5733 CrossRef CAS PubMed.
- C. Grant, D. Deszcz, Y. C. Wei, R. J. Martinez-Torres, P. Morris, T. Folliard, R. Sreenivasan, J. Ward, P. Dalby, J. M. Woodley and F. Baganz, Sci. Rep., 2014, 4, 5844 CrossRef CAS PubMed.
- D. Scheps, S. H. Malca, S. M. Richter, K. Marisch, B. M. Nestl and B. Hauer, Microb. Biotechnol., 2013, 6, 694–707 CAS.
- M. Kadisch, M. K. Julsing, M. Schrewe, N. Jehmlich, B. Scheer, M. von Bergen, A. Schmid and B. Buhler, Biotechnol. Bioeng., 2017, 114, 874–884 CrossRef CAS.
- J.-M. Woo, J.-W. Kim, J.-W. Song, L. M. Blank and J.-B. Park, PLoS One, 2016, 11, e0163265 CrossRef PubMed.
- L. M. Blank, B. E. Ebert, B. Buhler and A. Schmid, Biotechnol. Bioeng., 2008, 100, 1050–1065 CrossRef CAS PubMed.
- C. Rathnasingh, S. M. Raj, Y. Lee, C. Catherine, S. Ashok and S. Park, J. Biotechnol., 2012, 157, 633–640 CrossRef CAS PubMed.
- M. A. F. Delgove, M. T. Elford, K. V. Bernaerts and S. M. A. De Wildeman, Org. Process Res. Dev., 2018, 22, 803–812 CrossRef CAS PubMed.
- E.-J. Seo, H.-J. Kim, M.-J. Kim, J.-S. Kim and J.-B. Park, Chem. Commun., 2019, 55, 14462–14465 RSC.
- G. Rehn, A. T. Pedersen and J. M. Woodley, J. Mol. Catal. B: Enzym., 2016, 134, 331–339 CrossRef CAS.
- C. Nowak, B. Beer, A. Pick, T. Roth, P. Lommes and V. Sieber, Front. Microbiol., 2015, 6, 957 Search PubMed.
- G. Grogan, Curr. Opin. Chem. Biol., 2018, 43, 15–22 CrossRef CAS PubMed.
- S. Schmidt, C. Scherkus, J. Muschiol, U. Menyes, T. Winkler, W. Hummel, H. Groger, A. Liese, H. G. Herz and U. T. Bornscheuer, Angew. Chem., Int. Ed., 2015, 54, 2784–2787 CrossRef CAS PubMed.
- M. D. Truppo, J. D. Rozzell, J. C. Moore and N. J. Turner, Org. Biomol. Chem., 2009, 7, 395–398 RSC.
- D. Koszelewski, I. Lavandera, D. Clay, G. M. Guebitz, D. Rozzell and W. Kroutil, Angew. Chem., Int. Ed., 2008, 47, 9337–9340 CrossRef CAS.
- F. G. Mutti, C. S. Fuchs, D. Pressnitz, N. G. Turrini, J. H. Sattler, A. Lerchner, A. Skerra and W. Kroutil, Eur. J. Org. Chem., 2012, 2012, 1003–1007 CrossRef CAS.
- A. Segura, L. Molina, S. Fillet, T. Krell, P. Bernal, J. Munoz-Rojas and J. L. Ramos, Curr. Opin. Biotechnol., 2012, 23, 415–421 CrossRef CAS PubMed.
- L. A. Royce, J. M. Yoon, Y. Chen, E. Rickenbach, J. V. Shanks and L. R. Jarboe, Metab. Eng., 2015, 29, 180–188 CrossRef CAS PubMed.
- L. A. Royce, E. Boggess, Y. Fu, P. Liu, J. V. Shanks, J. Dickerson and L. R. Jarboe, PLoS One, 2014, 9, e89580 CrossRef PubMed.
- M. P. Brynildsen and J. C. Liao, Mol. Syst. Biol., 2009, 5, 277 CrossRef PubMed.
- A. P. Desbois and V. J. Smith, Appl. Microbiol. Biotechnol., 2010, 85, 1629–1642 CrossRef CAS PubMed.
- L. R. Jarboe, L. A. Royce and P. Liu, Front. Microbiol., 2013, 4, 272 Search PubMed.
- P. Liu, A. Chernyshov, T. Najdi, Y. Fu, J. Dickerson, S. Sandmeyer and L. Jarboe, Appl. Microbiol. Biotechnol., 2013, 97, 3239–3251 CrossRef CAS.
- M. J. de Smet, J. Kingma and B. Witholt, Biochim. Biophys. Acta, 1978, 506, 64–80 CrossRef CAS.
- P. Lund, A. Tramonti and D. De Biase, FEMS Microbiol. Rev., 2014, 38, 1091–1125 CrossRef CAS.
- L. M. Maurer, E. Yohannes, S. S. Bondurant, M. Radmacher and J. L. Slonczewski, J. Bacteriol., 2005, 187, 304–319 CrossRef CAS PubMed.
- G. Kannan, J. C. Wilks, D. M. Fitzgerald, B. D. Jones, S. S. BonDurant and J. L. Slonczewski, BMC Microbiol., 2008, 8, 37 CrossRef PubMed.
- L. W. Marzan, C. M. M. Hasan and K. Shimizu, Arch. Microbiol., 2013, 195, 161–171 CrossRef CAS PubMed.
- J.-H. Seo, S.-W. Baek, J. Lee and J.-B. Park, Biotechnol. Bioprocess Eng., 2017, 22, 231–238 CrossRef CAS.
- J. Shin, J. Yu, M. Park, C. Kim, H. Kim, Y. Park, C. Ban, E. Seydametova, Y.-H. Song, C.-S. Shin, K.-H. Chung, J.-M. Woo, H. Chung, J.-B. Park and D.-H. Kweon, ACS Synth. Biol., 2019, 8, 1055–1066 CrossRef CAS.
- H.-Y. Jang, K. Singha, H.-H. Kim, Y.-U. Kwon and J.-B. Park, Green Chem., 2016, 18, 1089–1095 RSC.
- I. Juneidi, M. Hayyan and M. A. Hashim, Process Biochem., 2018, 66, 33–60 CrossRef CAS.
- K. Radosevic, I. Canak, M. Panic, K. Markov, M. C. Bubalo, J. Frece, V. G. Srcek and I. R. Redovnikovic, Environ. Sci. Pollut. Res., 2018, 25, 14188–14196 CrossRef CAS.
- I. P. R. Grundtvig, S. Heintz, U. Kruhne, K. V. Gernaey, P. Adlercreutz, J. D. Hayler, A. S. Wells and J. M. Woodley, Biotechnol. Adv., 2018, 36, 1801–1814 CrossRef.
- W. Van Hecke, G. Kaur and H. De Wever, Biotechnol. Adv., 2014, 32, 1245–1255 CrossRef CAS.
- D. Kuhn, M. K. Julsing, E. Heinzle and B. Buhler, Green Chem., 2012, 14, 645–653 RSC.
- V. Alphand, G. Carrea, R. Wohlgemuth, R. Furstoss and J. M. Woodley, Trends Biotechnol., 2003, 21, 318–323 CrossRef CAS PubMed.
- I. Hilker, M. C. Gutierrez, R. Furstoss, J. Ward, R. Wohlgemuth and V. Alphand, Nat. Protoc., 2008, 3, 546–554 CrossRef CAS PubMed.
- H. D. Simpson, V. Alphand and R. Furstoss, J. Mol. Catal. B: Enzym., 2001, 16, 101–108 CrossRef CAS.
- I. Hilker, V. Alphand, R. Wohlgemuth and R. Furstoss, Adv. Synth. Catal., 2004, 346, 203–214 CrossRef CAS.
- T.-H. Kim, S.-H. Kang, J.-E. Han, E.-J. Seo, E.-Y. Jeon, G.-E. Choi, J.-B. Park and D.-K. Oh, Angew. Chem., Int. Ed., 2019 Search PubMed , under review.
- L. Matsakas, K. Hrůzová, U. Rova and P. Christakopoulos, Fermentation, 2018, 4, 13 CrossRef.
- B. van den Berg, P. N. Black, W. M. Clemons, Jr. and T. A. Rapoport, Science, 2004, 304, 1506–1509 CrossRef CAS PubMed.
- H.-Y. Jang, E.-Y. Jeon, A. H. Baek, S.-M. Lee and J.-B. Park, Process Biochem., 2014, 49, 617–622 CrossRef CAS.
- J.-H. Seo, G.-S. Lee, J. Kim, B.-K. Cho, K. Joo, J. Lee and B.-G. Kim, Enzyme Microb. Technol., 2009, 45, 218–225 CrossRef CAS.
- G. M. Morris, D. S. Goodsell, R. S. Halliday, R. Huey, W. E. Hart, R. K. Belew and A. J. Olson, J. Comput. Chem., 1998, 19, 1639–1662 CrossRef CAS.
- J.-H. Seo, D. Kyung, K. Joo, J. Lee and B.-G. Kim, Biotechnol. Bioeng., 2011, 108, 253–263 CrossRef CAS PubMed.
-
W. L. DeLano, Ccp4 Newsletter Protein Crystallogr, 2002, vol. 40, p. 11 Search PubMed.
Footnote |
† J.-W. Song and J.-H. Seo equally contributed to this work. |
|
This journal is © The Royal Society of Chemistry 2020 |
Click here to see how this site uses Cookies. View our privacy policy here.