DOI:
10.1039/D4LC00118D
(Paper)
Lab Chip, 2024,
24, 2658-2668
Microfluidic particle counter visualizing mucosal antibodies against SARS-CoV-2 in the upper respiratory tract for rapid evaluation of immune protection†
Received
6th February 2024
, Accepted 8th April 2024
First published on 8th April 2024
Abstract
Mucosal antibodies in the upper respiratory tract are the earliest and most critical responders to prevent respiratory infections, providing an indication for the rapid evaluation of immune protection. Here, we report a microfluidic particle counter that directly visualizes mucosal antibody levels in nasal mucus. The mucosal anti-SARS-CoV-2 spike receptor binding domain (RBD) antibodies in nasal secretions first react with magnetic microparticles (MMPs) and polystyrene microparticles (PMPs) that are surface-modified to form a “MMPs-anti-spike RBD IgG-PMPs” complex when RBD is present. After magnetic separation and loading into the microfluidic particle counter, the free PMPs, which are reduced with increasing anti-spike RBD IgG antibody levels, are trapped by a microfluidic particle dam and accumulate in the trapping channel. A sensitive mode [limit of detection (LOD): 14.0 ng mL−1; sample-to-answer time: 70 min] and an equipment-free rapid mode (LOD: 37.4 ng mL−1; sample-to-answer time: 20 min) were achieved. Eighty-seven nasal secretion (NS) samples from vaccinees were analyzed using our microfluidic particle counter, and the results closely resemble those of the gold-standard enzyme-linked immunosorbent assay (ELISA). The analysis shows that higher antibody levels were found in convalescent volunteers compared to noninfected volunteers. Together, we demonstrate a rapid kit that directly indicates immune status, which can guide vaccine strategy for individuals and the government.
Introduction
The coronavirus disease 2019 (COVID-19) pandemic, caused by severe acute respiratory syndrome coronavirus 2 (SARS-CoV-2) continues to pose a major threat to public health.1–3 As a respiratory infectious disease, vaccination is believed to be an effective way to achieve herd immunity, which ultimately promotes individual immune protection by eliciting high levels of specific antibodies that neutralize SARS-CoV-2 viruses and eventually slowing the spread of the virus in the community.4–6 However, the emerging variant contains mutations that confer the ability to evade neutralization by several antibodies,7 making it extremely unstoppable. Moreover, the antibody level after vaccination decays rapidly,8 which worsens the situation. Fortunately, 80% of recipients with three doses of mRNA vaccines exhibited sufficient immunization to neutralize the omicron variant. In contrast, the same level of protection was only observed in 3% of inactivated vaccines with three doses.9 The fact that mRNA vaccines are reported to have much higher immunogenicity10 suggests that not all antibodies lose their activities against omicron,7 and effective immune protection can still be achieved as long as the antibody level is sufficiently high. Thus, monitoring antibody levels becomes of the utmost importance to assess the level of community protection and provide guidance for vaccination strategy.11,12
Inhalation of virus-laden aerosols at short and long range is the main mode of SARS-CoV-2 infection.13 Aerosols deposit primarily in nasal and nasopharyngeal cavities, suggesting that the upper respiratory tract is the primary site of virus infection.14 Therefore, antibody responses in nasal mucus provide the earliest and most significant protection against the entry of respiratory viruses, including SARS-CoV-2.11,15–18 Studies based on nonhuman primates revealed that high levels of anti-S-specific and anti-RBD antibodies lead to a reduction of viral replication and inflammation in the lungs, providing a good correlation with immune protection.19 More importantly, a mucosal antibody level of 645 IU mL−1 of nasal swab S-specific Immunoglobulin G (IgG) was established as a protection threshold that ensures that the amount of SARS-CoV-2 nucleocapsid (N)-specific subgenomic RNA is less than 100
000 copies per swab.19 Thus, the measurement of mucosal antibody levels in nasal mucus is expected to provide a good evaluation of immune protection against viral infection.
The most common antibody detection method is lateral flow immunoassays (LFIAs), which are popular among the public due to their easy-to-use platform and simple result interpretation. However, LFIAs only provide qualitative (positive/negative) results.20 They are mostly used as an auxiliary tool for virus detection in case antigen tests are not accurate. For the quantitative analysis of antibodies, we can only rely on traditional immunoassays, such as enzyme-linked immunosorbent assay (ELISA) and chemiluminescence immunoassay.21,22 Although accurate, their high cost and requirements for professional and sophisticated inspection equipment make these methods impossible to implement in limited-resource areas. They are particularly unfeasible in the context of overwhelmed healthcare systems during the COVID-19 pandemic. Thus, the exploration of an equipment-free platform for quantitative antibody detection is urgently needed.
Here, we report a fast, low-cost, equipment-free and non-invasive microfluidic particle counter that integrates microparticles and a microfluidic chip for rapid evaluation of immune protection against SARS-CoV-2. Rather than using serum-based specimens, which require centrifugation to extract blood plasma,23 this device directly visualizes the levels of anti-spike RBD IgG antibodies in the nasal mucus. We chose the anti-spike RBD IgG antibody as the detection target due to its function of targeting S1 RBD to prevent binding between RBD and angiotensin-converting enzyme 2 (ACE2) of human cells.24–27 Magnetic microparticles (MMPs) coated with SARS-CoV-2 spike RBD and polystyrene microparticles (PMPs) coated with anti-human IgG were used for target detection. When target IgG antibodies were present, the “MMPs-anti-spike RBD IgG-PMPs” complex would form; thus, the quantity of the free PMPs is inversely proportional to the antibody concentration. After magnetic separation, the supernatant containing only the free PMPs was transferred to a microfluidic particle counter to visualize the PMP accumulation length to interpret the antibody level (Fig. 1).
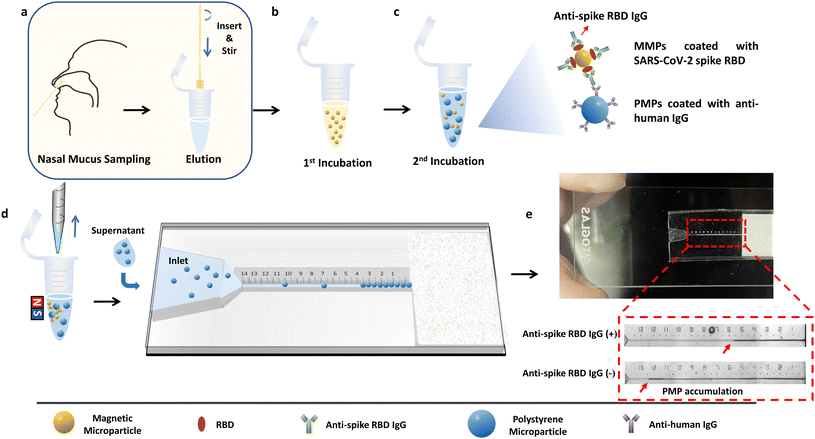 |
| Fig. 1 Overview of the microfluidic particle counter for rapid evaluation immune protection. (a) Nasal secretion sampling and elution in the elution buffer. (b) RBD-modified MMP solution first reacting with nasal secretion samples. (c) Injection of PMP solution in the tube to form the “MMPs-anti-spike RBD IgG” complex after rinsing. (d) Extraction of the supernatant with the free PMPs after magnetic separation, followed by loading into the microfluidic particle counter to trap and visually count the free PMPs via the PMP accumulation length, which reflects the concentration of anti-spike RBD. (e) Optical image of the microfluidic chip showing the PMP accumulation length, which is visible to the naked eye. | |
Experimental section
Design and fabrication of polydimethylsiloxane (PDMS)-NOA63 chip
The first version of the microfluidic chip used for the optimization of the microparticle and antibody concentration was made of a PDMS layer embedded with a sample loading inlet 2 mm in height and a NOA 63 layer with a solution-wicking channel 25 μm in height (Fig. S1†). The PDMS layer was cast using a mold printed with a 3D printer (Phrozen Sonic Mini 8K, Hsinchu City 30091, Taiwan R.O.C.) with an ultraviolet (UV) sensitive resin (ANYCUBIC, Shenzhen, Guangdong, China). After washing the printed mold in alcohol under ultrasonication for 5 min to remove the residual liquid resin on the surface, 3 min of UV post-curing was performed to solidify the resin inside the mold. Subsequently, the mold was treated with hexamethyldisilane (HMDS; Sigma-Aldrich, New Jersey, USA) for 5 min through chemical vapor deposition (CVD) in a fume hood to prevent the adhesion of PDMS to the printed mold. The PDMS precursor (elastomer base
:
curing agent = 10
:
1; Sylgard TM 184, Dow Corning, USA) mixed with dimethyl siloxane (60–70% ethylene oxide) block copolymer 20 cSt (Gelest, 30 Pennsylvania, USA) with a ratio of 1
:
0.0025 was poured onto the 3D-printed mold and cured at 80 °C for 2 hours. The function of the copolymer is to permanently change the hydrophilicity of PDMS to guarantee the required flow rate. After peeling it from the mold, all edges were cut to ensure a flat surface.
The NOA 63 channel layer was fabricated based on photolithography. SU8 2015 (Gersteltec Sarl, Switzerland) was first spin-coated onto a silicon wafer (Suzhou Crystal Silicon Electronic & Technology Co. Ltd.) at 1200 rpm to obtain a 25 ± 0.3 μm thickness SU8 master. After 5 min of soft baking at 95 °C, UV exposure and development were performed to remove unwanted photoresist. After hard baking at 125 °C for 6 h, the PDMS precursor was poured onto the SU8 master mold and cured at 80 °C for 2 hours to obtain the first PDMS. After being peeled off, the first PDMS layer was treated with plasma (Harrick Plasma, 400 mTorr) for 5 min and later coated with trichloro (1H,1H,2H,2H-perfluorooctyl) saline (97%) by gas-phase deposition at room temperature for 8 h in the fume hood. Next, a secondary PDMS was obtained by casting the PDMS precursor on the first PDMS. After 2 h of curing and demolding, the secondary PDMS was used as the master to make the NOA 63 layer. Briefly, liquid NOA 63 was smeared onto the patterned surface of the secondary PDMS, covered with a commercial polypropylene film (KOKUYO, Japan) and UV cured for 30 s. After peeling the sample off and cutting the edges of the slide, the patterned NOA 63 layer was bonded with the PDMS layer using plasma treatment (400 mTorr) for 5 min.28,29 Nail polish was applied on both sides of the inlet on the PDMS layer to build dams to avoid solution leakage.
Design and fabrication of PDMS-glass chip
The new version of the microfluidic chip consisted of a PDMS layer with embedded channels and a sealing glass layer designed to achieve sample detection (Fig. S2†). A filter paper acted as a capillary pump to ensure a fast flow as well as to trap the PMPs to form the visualized accumulation length. The PDMS layer was cast using a 3D-printed mold, and the fabrication steps were the same as above. After being printed with UV-sensitive resin, the mold was cleaned in ethanol for 5 min and UV cured for 3 min, followed by 5 min of HMDS coating using CVD. Next, a mixture of the PDMS precursor with dimethyl siloxane copolymer in a ratio of 1
:
0.0025 was cast on the 3D-printed mold and cured at 80 °C for 2 hours. After peeling it off from the mold, all the edges were cut to ensure a flat surface. Then, the copolymer PDMS layer and an alcohol-cleaned glass slide were treated with plasma (Harrick Plasma PDC-001, New York, USA) for 5 min at 400 mTorr and further bonded via slight compression to create an enclosed device. The chip was then heated at 125 °C for 3 min to ensure a tight binding. After inserting the filter paper into the designed slot, the PDMS-glass chip was ready to use.
Microparticle activation and modification
Carboxyl magnetic microparticles (MMPs) and polystyrene microparticles (PMPs) were activated using 1-ethyl-3-(3-dimethylaminopropyl) carbodiimide (EDC) and N-hydroxysuccinimide (NHS) in activation buffer (0.1 M MES in deionized water, 0.2% Tween 20, pH 5.0). In detail, 1.2 μL of MMP stock solution (25 mg mL−1) and 0.8 μL of PMP stock solution (100 mg mL−1) were washed twice with 200 μL of activation buffer. A magnetic rack and centrifuge were used separately for solid–liquid separation of MMP and PMP solutions. After removing the supernatant from the MMP and PMP solutions in the final washing step, 100 μL of freshly prepared EDC (50 mg mL−1) and NHS (50 mg mL−1) in the activation buffer were added to resuspend the MMPs and PMPs, followed by ultrasonication, vertexing and shaking for 15 min. Next, the microparticles were rinsed three times with coupling buffer (0.2% Tween 20 in 0.01 M PBS, pH 8.5) and finally resuspended in 4 μL of coupling buffer.
The MMPs and PMPs were next mixed with 2.4 μL of SARS-CoV-2 spike RBD (200 μg mL−1) and 2.4 μL of anti-human IgG antibodies (2.5 mg mL−1), respectively, based on optimized results (Fig. S5†). After overnight incubation in a 4 °C refrigerator with constant shaking, the modified microparticles were washed three times with 200 μL of coupling buffer. Then, the microparticles were resuspended with 200 μL of casein blocker and mixed for 30 min at room temperature with vortex shaking. After that, the MMPs and PMPs were washed with 200 μL of reaction buffer (1% Tween 20 in 0.01 M PBS, pH 8.5) three times, followed by resuspension in 4 μL of reaction buffer, respectively.
Sample detection with microfluidic chips
The workflow for the microfluidic particle counter is as follows: for the detection of standard solutions and human samples, 4 μL of modified MMP solution was first mixed with 16 μL of a standard solution (6.4 μL of standard samples eluted in 9.6 μL of elution buffer) or eluted human samples and constantly shaken for 30 min for sensitive mode or occasionally hand-stirred for 5 min for rapid mode. After the first incubation, the MMPs as well as the complex “MMPs-anti-spike RBD IgG” were washed 3 times with 200 μL of reaction buffer to remove interfering factors in the samples, especially other IgG antibodies that are not targeted to SARS-CoV-2 spike RBD but can bind with secondary antibodies modified on the PMPs. For the second stage, after the MMPs were resuspended in 8 μL of reaction buffer, 4 μL of the PMPs were added, and another 30 min of constant shaking or 5 min of occasional hand-stirring was performed to promote the reaction.
After all processes were finished, the reaction tube was placed on a magnetic rack to separate the MMPs and MMPs–anti-spike RBD IgG–PMPs from the liquid. After around 30 s, 3 μL of the supernatant was withdrawn and loaded on the microfluidic chip to measure the PMP accumulation length.
Unit conversion
A commercial pre-coated ELISA kit (catalog no. 41A235, ImmunoDiagnostics Limited, Hong Kong) was used to achieve the unit conversion of anti-spike RBD IgG antibody from IU mL−1 to ng mL−1. In this experiment, the standard solutions were created by serial dilutions of two sets of anti-spike RBD IgG antibody in the unit formats of IU mL−1 and ng mL−1 in the commercial assay buffer. One hundred microliters of the standard solutions were added to appropriate wells with duplication. After 1 h incubation at room temperature, the solutions were removed. Next, the washing step was performed by adding 300 μL of wash buffer three times, with 1 min incubation each time. After that, 100 μL of HRP-conjugated detection solution was added to each well and incubated at room temperature for 1 h. After washing 4 times with 300 μL of wash buffer, 100 μL of substrate solution was added and incubated in the dark at room temperature for 15 min. After adding 100 μL of stop solution to each well and gently tapping the kit, the color of the solution immediately changed from blue to yellow. Finally, using the microplate reader, the optical density at a wavelength of 450 nm was measured within 10 min. The standard curve for absorbance results for different concentrations of standard solutions in IU mL−1 is shown in Fig. S10a.† The linear regression equation was calculated to be y = 0.0874 + 0.5925 × x, (R2 = 0.9964) using linear fitting. The absorbance results based on different concentrations of standard solutions in ng mL−1 (1, 5, and 10 ng mL−1) (Fig. S10b†) were then used to calculate corresponding concentrations in IU mL−1 based on eqn (4). Finally, based on the two sets of data, the equation for unit conversion from ng mL−1 to IU mL−1 was obtained as y(IU mL−1) = −0.0056 + 0.2538 × x(ng mL−1) (R2 = 0.9999) (Fig. S10c†).
Results and discussion
Correlation of anti-spike RBD IgG levels in nasal secretions and plasma
We first studied the anti-spike RBD IgG levels present in blood plasma and nasal secretions. At present, most studies of anti-SARS-CoV-2 antibodies rely on plasma samples.12,23,30,31 However, there is not an established safety threshold of serological antibody level against viral infection. Instead, the immunity of mucous membranes in the upper respiratory tract has been reported to be the primary barrier against foreign airborne matter.11,13,16,32 A recent report suggests that mucosal antibodies are derived primarily from transudation of the antibodies from the blood instead of local production by tissue-resident B cells or plasma cells.33,34 To validate this, we performed correlation analyses using ELISA results from 10 pairs of plasma and nasal secretion samples collected from the same volunteers. First, two standard curves were created using serial dilutions of anti-spike RBD IgG protein in a nasal secretion model solution (commercial nasal fluid containing 1/50 of commercial serum) to mimic the total IgG concentration in human NS35,36 and in the commercial human serum (Fig. S3†). Then, using inverse regression referenced to calibration curves, the concentrations of the antibody in plasma (Fig. 2a) and eluted nasal secretion solution were determined. The anti-spike RBD IgG levels of the pre-elution nasal mucus were further calculated based on the recorded dilution factor. We found that the level of anti-spike RBD IgG in the nasal secretions was correlated with that in plasma (Fig. 2b), and the regression equation was calculated to be
using linear fitting (Fig. 2b). As expected, a positive correlation was observed between plasma and nasal mucus anti-spike RBD IgG levels, reflecting the fact that the IgG antibodies measured in the nasal mucus were originally from the serum that seeped into the mucosa from circulation.
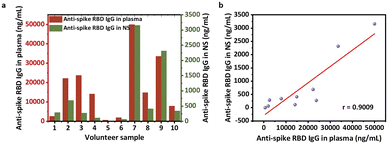 |
| Fig. 2 Correlation of anti-spike RBD IgG levels in NS and blood plasma. (a) Original anti-spike RBD IgG concentrations in the human plasma and nasal secretions of 10 volunteers based on ELISA. (b) Comparison of anti-spike RBD IgG levels in blood plasma (x-axis) and nasal secretion (y-axis). Data points represent results for each individual participant (10 volunteers). | |
Microfluidic particle counter for quantitative detection of anti-spike RBD IgG antibodies in nasal mucus model solution
Next, to provide a diagnostic device for rapid evaluation of the mucosal antibody levels, we designed a microfluidic particle counter combining microparticles in a microfluidic chip. For the microparticle system, the PMPs are surface-modified with anti-human IgG, while the surface of the MMPs is modified with SARS-CoV-2 spike RBD to achieve an accurate connection between the MMPs and PMPs mediated by anti-spike RBD IgG (Fig. 3a). When the target IgG antibodies are present, the complex “MMPs-anti-spike RBD IgG-PMPs” will be formed.
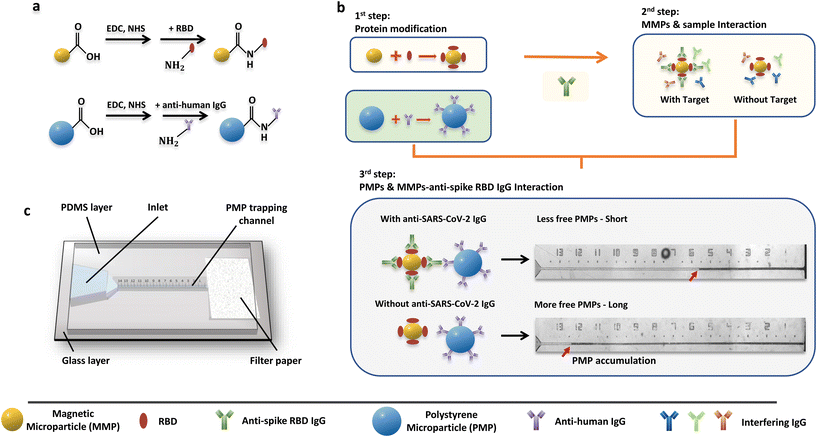 |
| Fig. 3 Layout of the microfluidic visual assay. (a) EDC-NHS chemistry activating the available carboxylic acid groups of microparticles. The resulting NHS ester reacts with amine groups of proteins in activation buffer. (b) Schematic illustration showing the detection principle and incubation steps of the microparticles and their accumulation in the microfluidic chip. (c) Layout of the microfluidic chip where the free PMPs are trapped and accumulate for visual counting. | |
Notably, while RBD can only react with anti-spike RBD IgG in sample solutions, interfering substances such as other human IgG antibodies may compete to bind with the anti-human IgG modified on the PMPs when present. To prevent this, the MMP solution was first reacted with the sample solution (first incubation) to form “MMPs-anti-spike RBD IgG” when the target antibodies were present. Then, rinsing was performed to remove other interfering factors from the nasal secretion samples, such as other IgG antibodies. Next, PMP solution was injected into the MMP solution and incubated to form the “MMPs–anti-RBD spike IgG–PMPs” complex (second incubation) (Fig. 3b). Finally, the sandwiched “MMPs–anti-spike RBD IgG–PMPs” and free MMPs were attracted to the side by the magnet rack, leaving only free the PMPs in the supernatant.
To visually quantify the amount of the free PMPs in the supernatant, a capillary-driven microfluidic chip with a trapping channel and a capillary pump region was used (Fig. 3c and S4†). The use of PDMS containing 0.25% w/w copolymer can maintain the long-term hydrophilicity of the microfluidic device after plasma treatment.37 Of note, the use of capillary force allows a low sample volume requirement and automated operation without external power sources. After loading 3 μL of the supernatant solution onto the chip, the free PMPs were accumulated at the microfluidic particle dam using either the 8 μm nozzle (Fig. S4a,† PDMS-NOA 63 chip) or a filter paper (Fig. S4b,† PDMS-glass chip). Therefore, the concentration of anti-spike RBD IgG, which is inversely proportional to the amount of the free PMPs, will be converted to the PMP accumulation length that is formed in the trapping channel and can be visualized using the naked eye (Fig. 3b). Thus, the level of anti-spike RBD IgGs can be calculated using inverse regression with reference to the calibration curve.
To explore the limit of detection (LOD) of the overall system, we systematically optimized experimental conditions (Fig. S5†) using the PDMS-NOA 63 chip. A PMP concentration of 20 mg mL−1 was selected based on the longest accumulation length of the microfluidic chip, which was then used to optimize the MMP concentration and protein immobilization on the microparticles (RBD on the MMPs and anti-human IgG on the PMPs) to achieve the maximum signal-to-noise ratio. After testing all conditions with/without target antibodies, values of 75 ng anti-human IgG per mg PMPs, 7.5 mg mL−1 MMPs and 16 ng RBD per mg MMPs were chosen as optimized conditions. Then, to study the combined effect of viscosity and reaction volume, different ratios of model solution
:
elution buffer to reaction buffer (2
:
3
:
0; 2
:
3
:
1; 2
:
3
:
3; and 2
:
3
:
7) were tested with/without the target antibody (Fig. S6†), while the volume ratio of model sample to elution buffer was set consistently to the ratio used in the human sample collection as approximately 2
:
3 (80
:
120 μL). The result at a ratio of 2
:
3
:
0 shows the best signal-to-noise ratio, meaning that no additional reaction buffer was needed in the incubation.
Furthermore, the reaction time for the first and second incubation was optimized using a series of concentrations of anti-spike RBD IgG antibodies spiked in commercial simulated nasal fluid as the model solution (Fig. S7†). The result shows that 30 min is required for both the first and second incubation to quantitatively differentiate the target concentration from 0 to 500 ng mL−1. In addition, we discovered that 5 min is already sufficient for the detection of antibody concentration above 100 ng mL−1. This is further clarified by the difference in PMP trapping length of 6.5 mm vs. 5.8 mm between the control and 100 ng mL−1 of the sample.
The selectivity of this assay was then verified by testing high concentrations of other interfering factors with a 30 min reaction time for the first and second incubation. The interfering factors included Mucin-5 AC (MUC5AC) (2.3 mg mL−1), albumin (2.13 mg mL−1), Middle East respiratory syndrome coronavirus (MERS-CoV) antibody (1 mg mL−1), and influenza A antibody (1 mg mL−1) (Fig. S8†). While a relatively short PMP accumulation length (around 6 mm) was observed with anti-spike RBD IgG (1 μg mL−1), the potential interfering factors with much higher concentrations (1000–2000 times higher) all resulted in long PMP accumulation lengths (around 11.5 mm) comparable to that of the blank control sample (p > 0.2 for all cases). Notably, on the basis of the 95% confidence interval of the two-tailed hypothesis (α = 0.05), the acceptable tolerance of the blank control was calculated to be 11.621 ± 0.696 mm, which covers measured results from all the interfering factors. Together, these findings suggest that the binding between the MMPs and PMPs is specific to antibodies against SARS-CoV-2 spike RBD.
Detection of anti-spike RBD IgG in human NS samples based on sensitive mode
For the PDMS-NOA 63 chip, some PMPs were trapped on the side wall of the PDMS layer without entering microchannels, which required occasional handshaking to assist them to flow into chips. To solve this technical problem, we designed a new channel that utilizes gravity to ensure the complete and unmanned loading of all microparticles into the trapping channel (Fig. S4b,† PDMS-glass chip). Additionally, this new design uses filter paper to drive capillary flow and trap PMPs, which is also compatible with the specifications of our 3D printer. Thus, we were able to ensure similar microparticle behaviour in our new device while simplifying the fabrication procedure.
With the new device and optimized experimental conditions, the sensitive mode was designed using 30 min for first and second incubations. A time of 30 min was chosen because it was sufficient to quantitatively differentiate a target concentration below 500 ng mL−1 (Fig. S7†). The standard curve for the sensitive mode based on the microfluidic chips was achieved using a series of concentrations of anti-spike RBD IgG antibodies spiked in the model solution. The non-linear regression equation in the dynamic range of 0 to 800 ng mL−1 is
| y(0–800ng mL−1) = 5.5181 + 6.0077e−0.00265×x, R2 = 0.9710 | (1) |
(
Fig. 4a, left). Based on the linear range near the blank sample (0 to 40 ng mL
−1) (
Fig. 4a, right), the linear regression was determined to be
|  | (2) |
using least-squares regression while incorporating the variance of model parameters,
i.e., the uncertainties of intercept
sb0 and slope
sb1. A LOD of 14.0 ng mL
−1 was achieved, which prevents type I and type II errors (less than 5% false positive/negative rate; see the ESI
† for details).
38–40
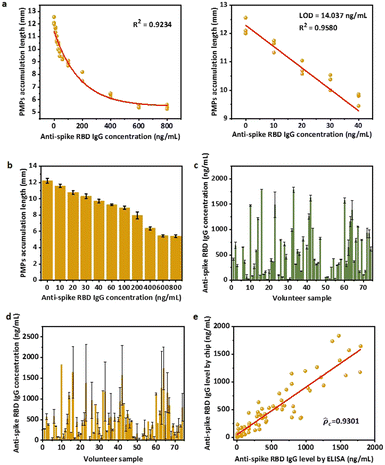 |
| Fig. 4 Sensitive mode to quantify anti-spike RBD IgG in human NS samples in the microfluidic chip. (a) Standard curve of the equipment-based sensitive mode based on serial concentrations of anti-spike RBD IgG antibodies spiked in model solution and the linear regression from 0 to 40 ng mL−1 (n = 3). (b) Measured PMP accumulation length showed an inverse proportion to the target antibody concentration (mean ± SD, n = 3). (c) Original anti-spike RBD IgG concentration of 74 NS samples based on ELISA. (d) Original anti-spike RBD IgG concentration in 74 human NS samples measured by our counter using the calibration curve from Fig. 4a, left. (e) Comparison of anti-spike RBD IgG concentrations in human NS samples measured by an ELISA kit (x-axis) and our system (y-axis) (n = 74). | |
To verify the compatibility with human samples, 87 volunteers with at least two doses of vaccination were recruited in Hong Kong. The anti-spike RBD IgG concentrations of the 87 total NS samples were first measured using ELISA (Fig. S3c†). Notably, the volume ratio of NS to elution buffer was set as approximately 80
:
120 μL. However, because the natural NS volume collected may not be exactly 80 μL (±10%), the actual amount of NS was weighed to determine the elution factor [(NS volume + elution buffer volume)/NS volume], and the original concentration of the NS sample was back-converted based on the factor (Fig. 4c). For detection on the microfluidic chips, after obtaining PMP accumulation length results, the anti-spike RBD IgG levels in the NS sample were calculated according to non-linear regression equations (eqn (1)) and multiplied by the elution factor of the individual sample (Fig. 4d). Because of the dynamic range of the sensitive mode (0 to 800 ng mL−1), only calculated concentrations below 2000 ng mL−1 in the NS sample, which would be roughly diluted to 800 ng mL−1 in the eluted sample with an average elution factor ∼2.5, were considered valid. Therefore, the results of ELISA (Fig. 4c) and sensitive mode for 74 human samples of anti-spike RBD IgG concentrations below 2000 ng mL−1 were compared (Fig. 4d). A good correlation was achieved on the basis of Lin's concordance correlation coefficient (
c = 0.9301) was obtained when our assay results were compared with gold standard ELISA test results one-by-one,41 indicating the accuracy and reliability of our system as a quantitative detection assay (Fig. 4e).
Anti-spike RBD IgG antibody detection in human NS samples based on equipment-free rapid mode
We next explored the possible rapid mode to achieve point-of-care testing. While accurate, the sensitive mode still relies on laboratory procedures such as vortexing to complete the reaction, which is not convenient for home use. Here, a rapid mode was designed to be fast and equipment-free, utilizing only 5 min of incubation for first and second interactions and a handheld pipetting mixing procedure that consumers can follow. A series of concentrations of anti-spike RBD IgG antibodies spiked in model solution were used to obtain the standard curve for the equipment-free rapid-mode assay (Fig. 5a and b). The non-linear regression equation of the standard curve for equipment-free rapid mode with a dynamic range from 0 to 1200 ng mL−1 is: | y(0–1200ng mL−1) = 5.6654 − 6.6576 × (1 − e−0.002×x), R2 = 0.9928 | (3) |
(Fig. 5a). An LOD of 37.4 ng mL−1 was achieved using the linear range near the blank sample [Fig. 5a; linear regression]: |  | (4) |
Then, the 87 volunteer NS samples were tested using the rapid mode protocol. After measuring the PMP accumulation lengths, the anti-spike RBD IgG levels were calculated according to the non-linear regression equations (Fig. 5c). Note that when concentrations are high, the response becomes nonlinear in both standard curves (Fig. 4a and 5a), so a slight variation in measured PMP accumulation length can represent a considerable fluctuation in the calculated anti-spike RBD IgG concentration. As a result, calculated values with low concentrations are more comparable and show smaller error bars than those with high concentrations. For NS samples with an antibody concentration below 4000 ng mL−1 (n = 84, 96% of all the samples), a good correlation was achieved on the basis of Lin's concordance correlation coefficient (
c = 0.9318) when compared to the gold-standard ELISA test (Fig. 5d), indicating the accuracy and reliability of our system even in equipment-free rapid mode.
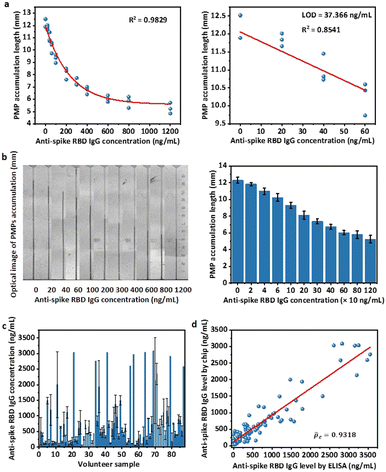 |
| Fig. 5 Equipment-free rapid mode to quantify anti-spike RBD IgG in human NS samples in the microfluidic chip. (a) Standard curve of equipment-free rapid mode based on the serial concentrations of anti-spike RBD IgG antibodies spiked in model solution and linear regression from 0 to 60 ng mL−1 (n = 3). (b) Optical image and bar chart of the PMP accumulation lengths with different target antibody concentrations (mean ± SD, n = 3). (c) Original concentrations of anti-spike RBD IgG antibodies in all 87 human NS samples measured with our counter using the calibration curve from Fig. 5a, left. (d) Comparison of anti-spike RBD IgG concentrations in human NS samples measured using an ELISA kit (x-axis) and our system (y-axis) (n = 84). | |
Analysis of the level of anti-spike RBD IgG correlated with infection status, gender and vaccine type
With the success of the microparticle assay in antibody detection, we further explored the antibody levels in human NS in relationship to infection status, gender and vaccine type. We first grouped data based on infection status. The anti-spike RBD IgG level was found to be significantly higher in the infected group (mean value: 1604.71 ng mL−1; median: 1294.73 ng mL−1; Fig. 6a) compared to the non-infected group (mean value: 617.64 ng mL−1; median: 421.78 ng mL−1; P < 0.0001, two-tailed t-test). For uninfected volunteers, those with at least one dose of mRNA vaccine had higher antibody levels (mean value: 760.45 ng mL−1; median: 544.01 ng mL−1; Fig. 6b) than others who only received two or three doses of inactivated vaccines (mean value: 267.75 ng mL−1; median: 172.55 ng mL−1; P = 0.0040, two-tailed t-test). For all the 87 samples, the anti-spike RBD IgG level was independent of gender (P = 0.3193; Fig. 6c). The same conclusions were obtained from analysis of the results based on the ELISA test (Fig. S9†).
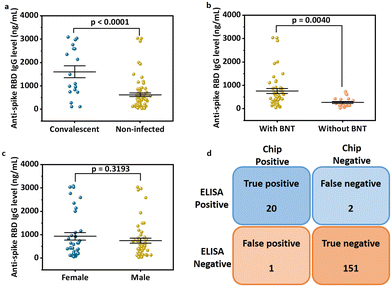 |
| Fig. 6 Correlation between the anti-spike RBD IgG level with infection, vaccine type and gender, as analyzed in rapid mode. (a–c) Level of anti-spike RBD IgG in NS based on infection (a), vaccine type (b), and gender (c). (d) Sensitivity analysis and specificity analysis of rapid mode. | |
We next explored the possibility of using the rapid mode as a semi-quantitative assay that can provide a relatively precise value of the antibody concentration and help to determine whether the antibody level is higher than the threshold or not. Based on the research on non-human primates, a concentration of 2541.5 ng mL−1 (645 IU mL−1, calculated based on the linear regression equation of unit conversion: y(IU mL−1) = −0.0056 + 0.2538 × x(ng mL−1), Fig. S10†) of the concentration of anti-spike RBD IgG in NS was selected as a threshold for protection.19 In other words, when 2.5 is used as the elution factor, immune protection is gained in the subject when the PMP accumulation length is lower than 5.66 mm. Using the ELISA results as the ground truth, 11 positive (protected) results were achieved by ELISA, while the rest 76 were negative (unprotected). Each sample was tested twice by the assay with equipment-free rapid mode, and 174 results were obtained. Finally, the assay showed 91% sensitivity (positive predictive agreement =
) and 99% specificity (negative predictive agreement =
) for rapid mode (Fig. 6d).
Notably, the protection threshold is 2541.5 ng mL−1 in NS, which is equivalent to 1016.6 ng mL−1 after dilution. Thus, the threshold for protection is already in the nonlinear region of the standard curve, leading to a high probability of false negatives and positives. To reduce such discrepancies, increasing the elution factor may be a good strategy in the future. With a higher elution factor, the target concentration would be reduced to the linear region of the standard curve, which is more tolerable in terms of the fluctuation of the PMP accumulation length, and thus, the accuracy of the semi-quantitative assay could be further improved.
Comparison to other methods
Based on the results (Table 1), our microfluidic particle counter achieves equipment-free quantitative detection of anti-SARS-CoV-2 antibody, and the sample-to-answer time is 20 min, which is much more efficient than the commercial ELISA kit, which requires 2–4 h. This type of device is urgently needed in resource-limited areas, especially in times of shortage of inspection equipment when the pandemic occurs. Additionally, the detection range of the rapid mode of the microfluidic counter system is 0–3000 ng mL−1, which covers the range of anti-SARS-CoV-2 IgG concentrations in most people and the protection threshold. Under these conditions, users can obtain accurate antibody concentration data to evaluate their immune protection status.
Table 1 Comparison with other detection methods
Method |
Measurement |
External equipment |
Reaction time |
Sample volume for each test |
LOD |
Commercial rapid test kit |
Colorimetry |
No |
15–20 min |
About 50 μL |
100 ng mL−1 (used in our lab) |
LFIA based on SeNPs42 |
Colorimetry |
No |
10 min |
About 50 μL |
5 ng mL−1 |
Commercial ELISA kit |
Optical absorbance |
Spectrophotometer |
Mostly 2–4 h |
1 μL |
0.15 IU mL−1 (0.61 ng mL−1) |
Electrochemical detection platform43 |
Current |
Potentiostat; smartphone |
13 min |
1 μL |
10.1 ng mL−1 |
Fluorescent immunochroma-tographic assay44 |
Fluorescence |
Fluorescence reader |
15 min |
20 μL |
38.3 IU mL−1 (150.93 ng mL−1) |
Paper microfluidic chip45 |
Fluorescence; velocimetry |
Smartphone-based fluorescence microscope; smartphone-based video capture |
27–32 min or 12 min |
5 μL |
1–5 ng mL−1 |
Microfluidic chip (this work) |
Distance |
No |
20 min |
1.6 μL |
37.4 ng mL−1 (rapid); 14.0 ng mL−1 (sensitive) |
Additionally, compared to previous designs reported by us,23,37,46 here, we leverage gravity and filter paper into the design of chip. Gravity is used to ensure that all the PMPs are loaded to prevent data fluctuations. Filter paper is used to drive capillary flow and PMP accumulation, which eliminates the requirement for micro-sized structures, such as the array of micro-pillars for capillary pumping and an 8 μm nozzle to trap the PMPs. With the simplification of fabrication procedures, the cost of our chip is around 2 USD for each test, including the chip body and microparticle part, which is much lower than that of the commercial ELISA kit (around 10–20 USD for each well).
Conclusion
During the outbreak of the COVID-19 pandemic, vaccination is regarded as the safest and most effective way to prevent infection, block transmission and contribute to reducing the spread of SARS-CoV-2. However, mutations of new variants may confer evasion of neutralization.7 Moreover, the antibody level elicited by vaccination or infection decays with time. Thus, effective assessment of immune protection is crucial for deciding vaccination strategy. Using nasal mucus, which represents the earliest and most significant protection against infection, our microfluidic chip has achieved high sensitivity (LOD: 14.0 ng mL−1; sample-to-answer time: 70 min; dynamic range: 0 to 800 ng mL−1) with the equipment-based sensitive mode and home-use rapid tests (LOD: 37.4 ng mL−1; sample-to-answer time: 20 min; dynamic range: 0 to 1200 ng mL−1). Tests of 87 clinical specimens show good accuracy compared to the gold standard ELISA (concordance correlation coefficients
c = 0.9301 and
c = 0.9318), indicating the reliability of our quantitative assay. Finally, based on the given protection threshold in NHPs, we adapted the equipment-free rapid mode to a semi-quantitative method that provides a true/false determination of immune protection with a sensitivity of 91% and specificity of 99%. This work will allow a precise and personalized rapid-test kit to evaluate immune protection against the next wave of the pandemic and speed up the resumption of normality.
Author contributions
Jiaheng Li: conceptualization, methodology, investigation, data curation, writing-original draft preparation. Lok Ting Chu: conceptualization, methodology, writing reviewing and editing. Hogi Hartanto: methodology, writing reviewing and editing. Guihuan Guo, Lu Liu, Jianpeng Wu, Minghui Wu, Chenyu Cui, Gaobo Wang, Wengang Liu, Hoi Kwan Kwong, Siying Wu: methodology. Ting-Hsuan Chen: conceptualization, methodology, supervision, funding acquisition, writing reviewing and editing.
Conflicts of interest
There are no conflicts to declare.
Acknowledgements
We thank Cami Yuk Ting Cham for providing her professional experience in assisting the sample collection. This work was supported in part by The Science, Technology and Innovation Commission of Shenzhen Municipality (JCYJ20210324134006017), in part by City University of Hong Kong (9678242 and 7020072), in part by Tung Biomedical Sciences Centre at City University of Hong Kong (9609336), and in part by InnoHK Project at Hong Kong Centre for Cerebro-cardiovascular Health Engineering (COCHE).
Notes and references
-
W. Coronavirus, 2021.
- F. Wu, S. Zhao, B. Yu, Y.-M. Chen, W. Wang, Z.-G. Song, Y. Hu, Z.-W. Tao, J.-H. Tian and Y.-Y. Pei, Nature, 2020, 579, 265–269 CrossRef CAS PubMed.
- R. Filip, R. Gheorghita Puscaselu, L. Anchidin-Norocel, M. Dimian and W. K. Savage, J. Pers. Med., 2022, 12, 1295 CrossRef PubMed.
- A. Fontanet and S. Cauchemez, Nat. Rev. Immunol., 2020, 20, 583–584 CrossRef PubMed.
- H. E. Randolph and L. B. Barreiro, Immunity, 2020, 52, 737–741 CrossRef CAS PubMed.
- F. Amanat, M. Thapa, T. Lei, S. M. S. Ahmed, D. C. Adelsberg, J. M. Carreño, S. Strohmeier, A. J. Schmitz, S. Zafar, J. Q. Zhou, W. Rijnink, H. Alshammary, N. Borcherding, A. G. Reiche, K. Srivastava, E. M. Sordillo, H. Van Bakel, The Personalized Virology Initiative, J. S. Turner, G. Bajic, V. Simon, A. H. Ellebedy and F. Krammer, Cell, 2021, 184, 3936–3948 CrossRef CAS PubMed.
- X. Shen, Nat. Med., 2022, 28, 445–446 CrossRef CAS PubMed.
- F. J. Ibarrondo, J. A. Fulcher, D. Goodman-Meza, J. Elliott, C. Hofmann, M. A. Hausner, K. G. Ferbas, N. H. Tobin, G. M. Aldrovandi and O. O. Yang, N. Engl. J. Med., 2020, 383, 1085–1087 CrossRef PubMed.
- S. M. S. Cheng, C. K. P. Mok, Y. W. Y. Leung, S. S. Ng, K. C. K. Chan, F. W. Ko, C. Chen, K. Yiu, B. H. S. Lam, E. H. Y. Lau, K. K. P. Chan, L. L. H. Luk, J. K. C. Li, L. C. H. Tsang, L. L. M. Poon, D. S. C. Hui and M. Peiris, Nat. Med., 2022, 28, 486–489 CrossRef CAS PubMed.
- W. W. Lim, L. Mak, G. M. Leung, B. J. Cowling and M. Peiris, Lancet Microbe, 2021, 2, E423 CrossRef CAS PubMed.
- V. Mouro and A. Fischer, Mucosal Immunol., 2022, 15, 584–594 CrossRef CAS PubMed.
- T. Takano, T. Sato, R. Kotaki, S. Moriyama, S. Fukushi, M. Shinoda, K. Kabasawa, N. Shimada, M. Kousaka, Y. Adachi, T. Onodera, K. Terahara, M. Isogawa, T. Matsumura, M. Shinkai and Y. Takahashi, Nat. Commun., 2023, 14, 1451 CrossRef CAS PubMed.
- C. C. Wang, K. A. Prather, J. Sznitman, J. L. Jimenez, S. S. Lakdawala, Z. Tufekci and L. C. Marr, Science, 2021, 373, eabd9149 CrossRef CAS PubMed.
- N. H. L. Leung, Nat. Rev. Microbiol., 2021, 19, 528–545 CrossRef CAS PubMed.
- M. Guerrieri, B. Francavilla, D. Fiorelli, M. Nuccetelli, F. M. Passali, L. Coppeta, G. Somma, S. Bernardini, A. Magrini and S. Di Girolamo, Vaccines, 2021, 9, 1499 CrossRef CAS PubMed.
- M. W. Russell, Z. Moldoveanu, P. L. Ogra and J. Mestecky, Front. Immunol., 2020, 11 DOI:10.3389/fimmu.2020.611337.
- K. Sano, D. Bhavsar, G. Singh, D. Floda, K. Srivastava, C. Gleason, PARIS Study Group, J. M. Carreño, V. Simon and F. Krammer, Nat. Commun., 2022, 13, 5135 CrossRef CAS PubMed.
- D. Fiorelli, B. Francavilla, A. Magrini, S. Di Girolamo, S. Bernardini and M. Nuccetelli, Int. Immunopharmacol., 2023, 115, 109615 CrossRef CAS PubMed.
- K. S. Corbett, M. C. Nason, B. Flach, M. Gagne, S. O'Connell, T. S. Johnston, S. N. Shah, V. V. Edara, K. Floyd, L. Lai, C. Mcdanal, J. R. Francica, B. Flynn, K. Wu, A. Choi, M. Koch, O. M. Abiona, A. P. Werner, J. I. Moliva, S. F. Andrew, M. M. Donaldson, D. R. Flebbe, E. Lamb, A. T. Noe, S. T. Nurmukhambetova, S. J. Provost, A. Cook, A. Dodson, A. Faudree, J. Greenhouse, S. Kar, L. Pessaint, M. Porto, K. Steingrebe, D. Valentin, S. Zouantcha, K. W. Bock, M. Minai, B. M. Nagata, R. V. D. Wetering, S. Boyoglu-Barnum, K. Leung, W. Shi, E. S. Yang, Y. Zhang, J. M. Todd, L. Wang, G. S. Alvarado, H. Andersen, K. E. Foulds, D. K. Edwards, J. R. Mascola, I. N. Moore, M. G. Lewis, A. Carfi, D. Montefiori, M.S. Suthar, A. Mcdermott, M. Roederer, N. J. Sullivan, D. C. Douek, B. S. Graham and R. A. Seder, Science, 2021, 373, eabj0299 CrossRef CAS PubMed.
- P. I. Kontou, G. G. Braliou, N. L. Dimou, G. Nikolopoulos and P. G. Bagos, Diagnostics, 2020, 10, 319 CrossRef CAS PubMed.
- J. Zhao, Q. Yuan, H. Wang, W. Liu, X. Liao, Y. Su, X. Wang, J. Yuan, T. Li, J. Li, S. Qian, C. Hong, F. Wang, Y. Liu, Z. Wang, Q. He, Z. Li, B. He, T. Zhang, Y. Fu, S. Ge, L. Liu, J. Zhang, N. Xia and Z. Zhang, Clin. Infect. Dis., 2020, 71, 2027–2034 CrossRef CAS PubMed.
- R. A, H. Wang, W. Wang and W. Tan, Virol. Sin., 2020, 35, 699–712 CrossRef CAS PubMed.
- M. Wu, S. Wu, G. Wang, W. Liu, L. T. Chu, T. Jiang, H. K. Kwong, H. L. Chow, I. W. S. Li and T.-H. Chen, Sci. Adv., 2022, 8, eabn6064 CrossRef PubMed.
- J. Fröberg, J. Gillard, R. Philipsen, K. Lanke, J. Rust, D. Van Tuijl, K. Teelen, T. Bousema, E. Simonetti, C. E. Van Der Gaast-De Jongh, M. Bos, F. J. Van Kuppeveld, B.-J. Bosch, M. Nabuurs-Franssen, N. Van Der Geest-Blankert, C. Van Daal, M. A. Huynen, M. I. De Jonge and D. A. Diavatopoulos, Nat. Commun., 2021, 12, 5621 CrossRef PubMed.
- M. F. Bachmann, M. O. Mohsen, L. Zha, M. Vogel and D. E. Speiser, npj Vaccines, 2021, 6, 2 CrossRef CAS PubMed.
- J. Lan, J. Ge, J. Yu, S. Shan, H. Zhou, S. Fan, Q. Zhang, X. Shi, Q. Wang, L. Zhang and X. Wang, Nature, 2020, 581, 215–220 CrossRef CAS PubMed.
- A. Cristiano, M. Pieri, S. Sarubbi, M. Pelagalli, G. Calugi, F. Tomassetti, S. Bernardini and M. Nuccetelli, Clin. Immunol., 2022, 234, 108918 CrossRef CAS PubMed.
- G. Wang, L. T. Chu, H. Hartanto, W. B. Utomo, R. A. Pravasta and T.-H. Chen, ACS Sens., 2019, 5, 19–23 CrossRef PubMed.
- M. Wu, G. Wang, L. T. Chu, H. Huang and T.-H. Chen, Sens. Actuators, B, 2020, 324, 128727 CrossRef CAS.
- D. J. Sullivan, M. Franchini, M. J. Joyner, A. Casadevall and D. Focosi, Nat. Commun., 2022, 13, 6478 CrossRef CAS PubMed.
- G. Uzun, R. Müller, K. Althaus, M. Becker, P. Marsall, D. Junker, S. Nowak-Harnau, N. Schneiderhan-Marra, H. Klüter, H. Schrezenmeier, P. Bugert and T. Bakchoul, Viruses, 2023, 15, 1357 CrossRef CAS PubMed.
- J. Holmgren and C. Czerkinsky, Nat. Med., 2005, 11, S45–S53 CrossRef CAS PubMed.
- J. I. Cohen, L. Dropulic, K. Wang, K. Gangler, K. Morgan, K. Liepshutz, T. Krogmann, M. A. Ali, J. Qin, J. Wang, J. S. Vogel, Y. Lei, L. P. Suzuki-Williams, C. Spalding, T. N. Palmore and P. D. Burbelo, Clin. Infect. Dis., 2023, 76, 1391–1399 CrossRef CAS PubMed.
- A. Ishizaka, M. Koga, T. Mizutani, R. Uraki, S. Yamayoshi, K. Iwatsuki-Horimoto, S. Yamamoto, M. Imai, T. Tsutsumi, Y. Suzuki, Y. Kawaoka and H. Yotsuyanagi, Virol. J., 2023, 20, 146 CrossRef CAS PubMed.
- S. R. Khan, L. Chaker, M. A. Ikram, R. P. Peeters, P. M. van Hagen and V. A. S. H. Dalm, J. Clin. Immunol., 2021, 41, 1902–1914 CrossRef CAS PubMed.
- M. Yoshida, S. M. Claypool, J. S. Wagner, E. Mizoguchi, A. Mizoguchi, D. C. Roopenian, W. I. Lencer and R. S. Blumberg, Immunity, 2004, 20, 769–783 CrossRef CAS PubMed.
- G. Wang, J. Li, S. Wu, T. Jiang and T.-H. Chen, Anal. Chem., 2022, 94, 15925–15929 CrossRef CAS PubMed.
- C. A. Clayton, J. W. Hines and P. D. Elkins, Anal. Chem., 1987, 59, 2506–2514 CrossRef CAS.
- I. Lavagnini and F. Magno, Mass Spectrom. Rev., 2007, 26, 1–18 CrossRef CAS PubMed.
- C. Cui, C.-H. Lau, L. T. Chu, H. K. Kwong, C. Tin and T.-H. Chen, Biosens. Bioelectron., 2023, 220, 114859 CrossRef CAS PubMed.
- I. Lawrence and K. Lin, Biometrics, 1989, 255–268 Search PubMed.
- Z. Wang, Z. Zheng, H. Hu, Q. Zhou, W. Liu, X. Li, Z. Liu, Y. Wang and Y. Ma, Lab Chip, 2020, 20, 4255–4261 RSC.
- R. Peng, Y. Pan, Z. Li, Z. Qin, J. M. Rini and X. Liu, Biosens. Bioelectron., 2022, 197, 113762 CrossRef CAS PubMed.
- X. Duan, Y. Shi, X. Zhang, X. Ge, R. Fan, J. Guo, Y. Li, G. Li, Y. Ding, R. A. Osman, W. Jiang, J. Sun, X. Luan and G. Zhang, Biosens. Bioelectron., 2022, 199, 113883 CrossRef CAS PubMed.
- Y. Liang, B. C. Buchanan, B. Khanthaphixay, A. Zhou, G. Quirk, M. Worobey and J.-Y. Yoon, Biosens. Bioelectron., 2023, 229, 115221 CrossRef CAS PubMed.
- G. Wang, Y. Zhang, H. K. Kwong, M. Zheng, J. Wu, C. Cui, K. W. Y. Chan, C. Xu and T. H. Chen, Adv. Sci., 2024, 2306188 CrossRef PubMed.
|
This journal is © The Royal Society of Chemistry 2024 |