DOI:
10.1039/D2NA00339B
(Paper)
Nanoscale Adv., 2022,
4, 3987-3995
A high-performance wearable pressure sensor based on an MXene/PVP composite nanofiber membrane for health monitoring†
Received
29th May 2022
, Accepted 14th August 2022
First published on 15th August 2022
Abstract
Flexible and wearable pressure sensors have attracted extensive attention in domains, such as electronic skin, medical monitoring and human–machine interaction. However, developing a pressure sensor with high sensitivity, mechanical stability and a wide detection range remains a huge challenge. In this work, a flexible capacitive pressure sensor, based on a Ti3C2Tx (MXene)/polyvinyl pyrrolidone (PVP) composite nanofiber membrane (CNM), prepared via an efficient electrospinning process, is presented. The experimental results show that even a small mass fraction of MXene can effectively decrease the compression modulus of the PVP nanofiber membrane, thus enhancing the sensing performance. Specifically, the sensor based on (0.1 wt% MXene)/PVP CNM has a high sensitivity (0.5 kPa−1 at 0–1.5 kPa), a fast response/recovery time (45/45 ms), a wide pressure detection range (0–200 kPa), a low detection limit (∼9 Pa) and an excellent mechanical stability (8000 cycles). Due to its superior performance, the sensor can monitor subtle changes in human physiology and other signals, such as pulse, respiration, human joint motions and airflow. In addition, a 4 × 4 sensor array is fabricated that can accurately map the shape and position of objects with good resolution. The high-performance flexible pressure sensor, as developed in this work, shows good application prospects in advanced human–computer interface systems.
1. Introduction
In recent years, flexible pressure sensors exhibit promising prospects in advanced applications, especially in the fields of electronic skin, flexible display screens, medical monitoring, and human–machine interaction.1–12 In general, the practical applications of flexible pressure sensors mainly involve the tactile pressure interaction between the users and the devices, ranging from slight stimuli to heavy touches.13 Therefore, it is crucial for flexible pressure sensors to be highly sensitive and operate within a wide detection pressure range, thereby exhibiting good sensing capability, similar to the human skin. According to the signal conversion mechanism, flexible pressure sensors can be mainly divided into four types: piezoresistive, piezoelectric, capacitive, and triboelectric pressure sensors.14–22 Among them, capacitive pressure sensors have the advantages of relatively high sensitivity, fast response time and low power consumption, thereby attracting high research interest.
Many research studies reveal that the introduction of microstructures into dielectric layers of capacitive pressure sensors can further improve their sensing performance. For example, Cui et al. presented a pressure sensor with a 1D pyramidal micrographic structure on a polydimethylsiloxane (PDMS) substrate and a dielectric layer of polystyrene (PS) microspheres, with a sensitivity of 0.741 kPa−1 in the 0–1 kPa pressure range, a pressure range of 0–50 kPa and a response time of 150 ms.23 Ji et al. proposed a novel dielectric layer based on the gradient microsphere structure (GDA) to achieve a high sensitivity (0.065 kPa−1) of capacitive sensors with an ultra-wide linear range (0–1700 kPa) and a fast response time (100 ms).24 Although microstructures can effectively enhance sensitivity, the manufacturing processes involved, such as photolithography, are usually expensive and complicated, failing to meet the requirements of most practical applications. In comparison, the electrospinning process is simple, efficient and inexpensive, while the fabricated nanofiber membranes have high porosity, large specific surface area and a low compression modulus, making them suitable for practical applications on a large scale.25,26
The selection of the dielectric layer material is also particularly vital. Polymer materials, which are commonly used as dielectric layers, generally have a high compression modulus, inhibiting the optimization of the sensor sensitivity. In order to solve this problem, conductive fillers can be added to polymer materials to form nanocomposites, thus decreasing the compression modulus while maintaining the flexibility. For example, sensors based on carbon nanotubes (CNTs)/polyvinylidene fluoride (PVDF), silver nanowires (AgNWs)/thermoplastic polyurethane (TPU) and zinc oxide nanowires (ZnONWs)/polydimethylsiloxane (PDMS) elastomer composite membranes, have been studied.27–29 However, these one-dimensional (1D) materials such as CNTs, AgNWs and ZnONWs, have low surface areas and tend to aggregate into bundles, which will partly affect the sensing performance. In order to solve the above problems, two-dimensional (2D) materials can be used.
MXene is a kind of metal carbide and nitride with a 2D layered structure. The surface functional groups, such as O, F and OH, lead to the formation of semiconductor functionalized MXene (Mn+1XnTx), where M is an early transition metal (Ti, Mo, V, etc.), X is carbon or nitrogen, x is the termination number and T is a functional group (O, F or OH).30,31 Thanks to its abundant functional groups, MXene can be dispersed in water or other organic materials. Compared to other 2D conductive nanofillers, MXene has the advantages of high electrical conductivity, large specific surface area and high aspect ratio.32,33 Ti3C2Tx is the most widely studied MXene and is used in many applications, including energy storage, electromagnetic interference shielding, catalysis, optoelectronics and sensors.30,34,35 Therefore, it is an ideal additive for the nanocomposite dielectric layer.
In this work, a flexible capacitive pressure sensor, based on an MXene (Ti3C2Tx)/polyvinyl pyrrolidone (PVP) composite nanofiber membrane (CNM), was fabricated using a simple and efficient electrospinning process. The PVP micro-structure network has excellent physiological inertness and bio-compatibility and is widely studied for its antihemorrhagic and antibacterial wound protection effects.36,37 Furthermore, a small mass fraction of MXene is added into the PVP nanofiber membrane, effectively reducing its compressive modulus and improving the sensing performance. The results indicate that the produced capacitive pressure sensor, based on f(MXene)/PVP (f = 0.1 wt%) CNM, has a high sensitivity of 0.5 kPa−1 at 0–1.5 kPa, a fast response/recovery time of 45/45 ms, and a low detection limit (∼9 Pa) and it shows an excellent mechanical stability during 8000 operation cycles. In addition, the sensor can be used to detect human health signals, such as pulse, respiration and human joint bending, among others. Finally, a 4 × 4 sensor microarray is constructed for mapping the pressure distribution of objects placed on a flat surface.
2. Experimental section
2.1 Preparation of the MXene/PVP solution
MXene/PVP composites were chosen as dielectric materials, while N,N-dimethylformamide (DMF) (99.5%) was used as the organic solvent. 5 g 9.48 mg mL−1 DMF solution was added in a 20 mL vial, followed by adding 1 g PVP particles (Mr = 1
300
000) into the DMF solution, at a ratio of 1
:
5. 5 mg mL−1 MXene solution was added into the PVP/DMF suspension solution, while the mass fractions of MXene and PVP were set at 0.05
:
99.95, 0.1
:
99.9 and 0.15
:
99.85, respectively. Finally, the solutions were kept under magnetic stirring for 24 hours, until they became homogeneous.
2.2 Preparation of the MXene/PVP CNM
MXene/PVP CNM was prepared using the electrospinning process. First, a high voltage electric field of 15 kV was applied at the end of the syringe needle. The syringe's propulsion speed was 10 mL h−1. The distance between the aluminum foil of the receiving device and the needle was set at 15 cm. The ambient humidity was less than 20%. Afterwards, f(MXene)/PVP (f = 0, 0.05, 0.1 and 0.15 wt%) mixed solution portions were drawn with a 1 mL syringe and used for electrospinning. The prepared films were obtained by drying for 10 min on a heating table, at 150 °C. Finally, the MXene/PVP CNMs were prepared by UV irradiation (UV-2550, Shimadzu) for 40 minutes.
2.3 Preparation of the sensor
The prepared MXene/PVP CNM was used as the dielectric layer, while the polyimide (PI) film sputtered with Cu was used as the electrode. The MXene/PVP CNM was sandwiched between two layers of Cu-sputtered PI electrodes, forming a flexible capacitive pressure sensor. The physical appearance of the fabricated device is shown in Fig. S1.† Silver paste was spread on the edge of the electrode, while the copper wire was put through subsequent performance testing. Finally, the device was packaged with transparent tape to isolate noise and prevent signal distortion.
2.4 Microstructure characterization of the sensor
The microstructure and morphology of the PVP, MXene particles and MXene/PVP CNMs were characterized using a field emission scanning electron microscope (FESEM, Hitachi S4800). A transmission electron microscope (TEM, FEI Talos F200X) was employed to further characterize the microstructure of MXene. Elemental dispersion analysis of the MXene/PVP CNM was performed using an Energy Dispersive Spectrometer (EDS).
2.5 Electromechanical characterization of the sensor
Regarding electromechanical characterization, a computer-controlled stepper motor (ZQ-9908) was used to apply static and dynamic loading to the sensor. The capacitive response was measured using an LCR (inductance, capacitance, resistance) meter (CYCLETEST ECA200) at a frequency of 1 MHz and a voltage of 1 V. To measure human physiological signals, the sensors were fixed on the wrist, mask, muscle, finger joint and wrist joint, respectively. The copper wires were used to connect the sensors to the LCR meter. The real-time change of the capacitance value was recorded by a computer. Similarly, to test the perception of the external physical pressure, the sensors were fixed on a table and beaker, respectively. Pressure was generated by squeezing a rubber balloon or holding a beaker, and the capacitance signal was recorded using an LCR meter and a computer.
2.6 Preparation and measurement of the 4 × 4 sensor array
The fabrication process of a 4 × 4 sensor array was as follows. The patterned mask was placed on the surface of a PI film for Cu target sputtering to prepare the upper and lower electrodes. The width and spacing of each electrode were fixed at 0.5 cm. Next, the MXene/PVP CNM, prepared by electrospinning, was cut into 16 equally sized pieces of 0.5 × 0.5 cm2, to prevent crosstalk between adjacent capacitors. The middle MXene/PVP CNM dielectric layer was assembled face to face with the upper and lower electrodes, to form a 4 × 4 sensor array. As shown in Fig. S2,† the capacitance of the sensor array was measured orderly using a dual comparator (LM393), and wirelessly transmitted to a computer through the WIFI module (ESP8266). Finally, the capacitance cloud map can be directly displayed on the screen of C++ programmed software.
3. Results and discussion
The fabrication procedure of the proposed flexible capacitive pressure sensor, based on MXene/PVP CNM, is schematically illustrated in Fig. 1. The process starts with thorough mixing of the MXene solution and the PVP particles. Next, the MXene/PVP CNM was prepared by electrospinning, followed by UV treatment.38,39 Finally, the MXene/PVP CNM was sandwiched between Cu-sputtered PI electrodes to obtain a complete pressure sensor. The detailed experimental steps can be viewed in the “Experimental section”. Fig. 2(a) and (b) show the FESEM images of the PVP and MXene particles. It can be obtained from the size distribution that the average sizes of the PVP and MXene particles are 108 μm and 18 μm, respectively. Most importantly, the organ-like MXene particles are assembled by multilayer MXene nanosheets, as shown in Fig. 2(b). As further analysis of the MXene nanosheet, the high-resolution TEM image and selected-area electron diffraction (SAED) pattern (Fig. 2(c)) indicate the hexagonal lattice characteristics of the MXene nanosheet, which is consistent with other reported studies.40,41Fig. 2(d) shows the FESEM image of the f(MXene)/PVP (f = 0.1 wt%) CNM, where the fibrous scaffolds appear intertwined, forming a fiber network. Observing the selected area through an enlarged magnification (Fig. 2(e)), some light-colored nanosheets appear densely attached to each fiber. Further analysis of the selected area using energy dispersive spectroscopy (EDS) indicates that the area contains chemical elements of C, O, N, F and Ti, verifying that the light-colored nanosheets are actually MXene. It can be seen from Fig. 2(f) that the assembled sensor is a typical sandwich structure in a cross-sectional view. The Cu-sputtered PI electrode has a thickness of 50 μm, while the MXene/PVP CNM dielectric layer has a thickness of 23 μm.
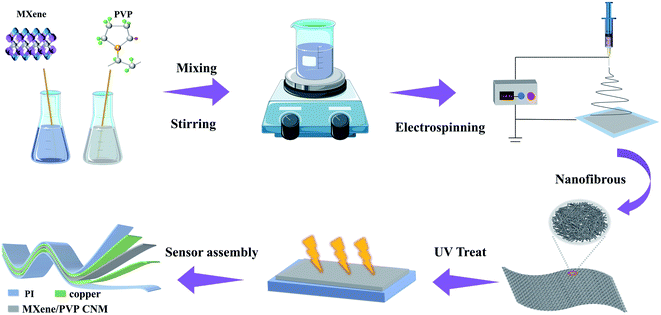 |
| Fig. 1 Schematic diagram of the fabrication process of the MXene/PVP CNM-based pressure sensor. | |
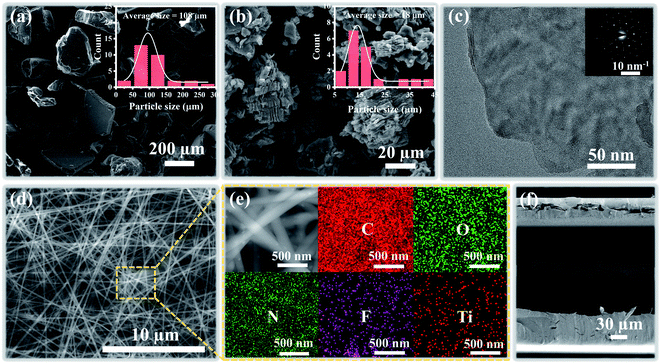 |
| Fig. 2 FESEM images of the (a) PVP and (b) MXene particles. (c) TEM image of the MXene nanosheet and its SAED pattern. (d) FESEM image of the MXene/PVP CNM. (e) Partial enlarged view of the MXene/PVP CNM and corresponding EDS elemental mapping of C, O, N, F and Ti. (f) Cross-sectional SEM image of the assembled sensor. | |
The corresponding capacitance C of the pressure sensor, based on the MXene/PVP CNM, equal to that of a traditional parallel-plate capacitor, is given by:
|  | (1) |
where
A is the overlapping area of the two electrodes,
d is the distance between the two electrodes, and
εr and
ε0 are the dielectric constants of the CNM and free space, respectively.
42 When a vertical pressure
P is applied on the sensor, the ratio of the variation of the capacitance Δ
C to the initial capacitance
C0 can be expressed as:
| 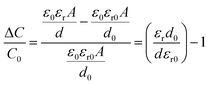 | (2) |
where
εr/
εr0 represents the dielectric constant of the CNM with/without pressure, while
d/
d0 is the distance between the two electrodes with/without pressure. In addition, the sensitivity of the sensor is defined as:
where
S can be obtained by calculating the slope of the pressure–capacitance curve. On the one hand, the MXene/PVP CNM, prepared by electrospinning, contains a large number of air gaps, which are progressively filled by MXene/PVP fibers as the pressure increases, thus increasing the dielectric constant
εr. On the other hand, the introduction of a small fraction of MXene into PVP can not only decrease its compression modulus, but also have a small impact on its dielectric constant
εr0. Based on the synergy of the above two aspects, the sensing performance can be effectively improved.
The dielectric layer plays a great role in improving the overall performance of the capacitive pressure sensor. First, MXene/PVP CNMs electrospun at different times (20, 40 and 60 min) were prepared, and the influence of the dielectric layer thickness (electrospinning time) on the sensing performance is discussed. As shown in Fig. 3(a), at a constant pressure of 200 kPa, the pressure sensor based on the MXene/PVP CNM electrospun at 20 min has a ΔC/C0 of 1.419, which is larger than that of the sensors electrospun at 40 min (0.977) and 60 min (0.750). Since the thin dielectric layer (electrospun at 20 min) exhibits more pores, εr and C will increase rapidly under an applied pressure, leading to excellent sensing performance. However, the ultrathin dielectric layer is easily damaged under high pressure, resulting in a short circuit. Therefore, the optimal electrospinning time for the MXene/PVP CNM dielectric layer is 20 min.
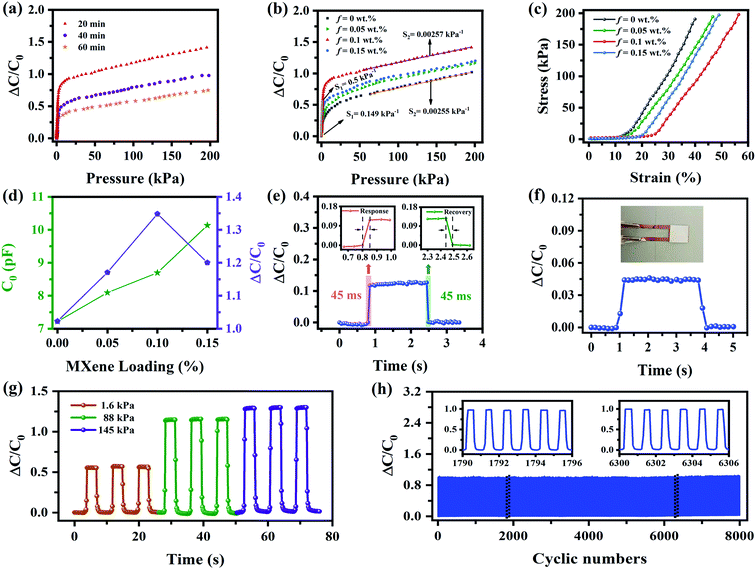 |
| Fig. 3 Experimental sensing performance of the MXene/PVP CNM-based pressure sensor. (a) Relative capacitance change (ΔC/C0) of the pressure sensors based on the MXene/PVP CNMs electrospun at different times (20, 40 and 60 min). (b) Relative capacitance change (ΔC/C0) of the pressure sensors based on f(MXene)/PVP (f = 0, 0.05, 0.1 and 0.15 wt%) CNM dielectric layers. (c) Compressive stress–strain performance of the sensors. (d) Relation of the initial capacitance C0 and the relative change in capacitance ΔC/C0 of the sensors with the MXene fraction amount. (e) Response and recovery time at a pressure of 240 Pa. (f) Demonstration of the lower limit of detection sensitivity, under sequential loading/unloading of a piece of tissue paper (∼9 Pa). (g) Relative change in capacitance response, under repeatedly applied pressures of 1.6 kPa, 88 kPa, and 145 kPa. (h) Cycling stability test for 8000 loading and unloading cycles, at 34 kPa. The insets present the selected cycles at the beginning and end of the stability test. | |
In order to evaluate the effect of the addition of MXene on the sensing performance, S of the sensors, based on f(MXene)/PVP (f = 0, 0.05, 0.1 and 0.15 wt%) CNMs, is illustrated in Fig. 3(b). S first increases, with the increase of f (0 ≤ f ≤ 0.1 wt%), followed by a decrease, as f (0.1 < f ≤ 0.15 wt%) further increases, exhibiting a maximum value at f = 0.1 wt%. S of the sensor with f = 0.1 wt%, in the low-pressure region (0–1.5 kPa), is 0.5 kPa−1, whereas in the high-pressure region (60–200 kPa), it is 2.57 × 10−3 kPa−1. This occurrence can be explained by the strain–stress analysis plot in Fig. 3(c) and the force-displacement analysis plot in Fig. S3.† As shown in Fig. 3(c), the compression modulus of the MXene/PVP CNM, which can be obtained by the slope of the curve, decreases from 7.22 to 5.93, as f increases from 0 to 0.1 wt%. However, as f further increases from 0.1 to 0.15 wt%, the compression modulus rises from 5.93 to 6.72. A similar phenomenon can also be seen in Fig. S3.† The compressive stiffness, obtained by the slope of the curve, decreases from 86.11 to 61.64 as f increases from 0 to 0.1 wt%, and then rises to 72.70 as f further increases to 0.15 wt%. In other words, the compressive flexibility first decreases (0 ≤ f < 0.1 wt%), and then increases (0.1 < f ≤ 0.15 wt%). This can be attributed to the fact that a small fraction of MXene can weaken the fluidity of the PVP chain and soften the PVP nanofiber membrane, resulting in a lower compression modulus/stiffness.43 Nevertheless, an excess of MXene will easily agglomerate inside the PVP nanofiber and tend to create a 3D physical network, thus increasing the compression modulus/stiffness.44,45 Consequently, the sensor with f = 0.1 wt% possesses the maximum S value.
As shown in Fig. 3(d), the initial capacitance C0 of the sensors based on f(MXene)/PVP (f = 0, 0.05, 0.1 and 0.15 wt%) CNMs slightly increases along f, as a result of the elevation of the effective dielectric constant caused by the addition of MXene, which is consistent with the principle of formula (1). However, the relative capacitance ΔC first increases and then decreases with f, matching the variation of the compressive modulus, as illustrated in Fig. 3(c). Similar to most of the dielectric composites, the results shown in Fig. S4† demonstrate that C0 of the sensors based on f(MXene)/PVP (f = 0, 0.05, 0.1 and 0.15 wt%) CNMs decreases as the frequency increases, which is caused by the Maxwell–Wagner–Sillar interface polarization inside the material.46 Furthermore, the conductivity of the sensors, as a function of frequency, is shown in Fig. S5.† The sensors show conductive behavior when the frequency is greater than 105 Hz, while the conductivity is proportional to f. This indicates that the MXene can form a stable conductive seepage bridge in the PVP matrix at high frequencies.
Regarding other performance aspects of the sensor with f = 0.1 wt%, Fig. 3(e) shows its response and recovery time. When a force of 240 Pa is applied, the relative capacitance ΔC/C0 increases rapidly and the response time is 45 ms. After unloading, the capacitor returns to its initial level rapidly, and the recovery time is also 45 ms. The device shows not only a fast response/recovery time but also an excellent advantage in sensing tiny objects. In Fig. 3(f), a piece of paper (∼9 Pa) is placed lightly on the sensor. The relative capacitance increases rapidly from 0 to 0.043, returning to 0 after the paper is picked up. Based on the demonstrated excellent performance, the sensor appears to have a similar perception ability to human skin. The pressure stability under repeated iterations is also studied. Fig. 3(g) displays the stability tests of the sensor at different levels of pressure. Forces of 1.6 kPa, 88 kPa and 145 kPa are repeatedly applied three times, while observations show that the pressure response is stable, without obvious fluctuations. In addition, the sensor is subjected to fatigue tests up to 8000 times, as shown in Fig. 3(h), where the inset represents an enlarged view of selected cycles. According to the illustrations, the device shows an excellent mechanical stability throughout the test interval, which proves the long endurance of the proposed device. Overall, the performance of the sensor in this work is comparable to those of other reported state-of-the-art pressure sensors, as shown in Table 1.47–53
Table 1 Sensing performance, including the sensitivity, response time, pressure range and stability of the MXene/PVP CNM-based capacitive pressure sensor and other reported state-of-the-art current pressure sensors
Nanofiber material |
Sensitivity |
Response time |
Pressure range |
Stability [cycle] |
Ref. |
ACG-M hydrogel |
— |
500 ms |
— |
— |
47
|
RGO coated PU foam |
0.67/kPa |
10 ms |
0–30 kPa |
100 |
48
|
CNFs/PDMS@Ni foam |
0.6/kPa |
30 ms |
0–20 kPa |
10 000 |
49
|
PLGA–PCL nanofiber |
0.863/kPa |
251 ms |
0–5 kPa |
— |
50
|
Poly (ionic liquid) nanofiber |
0.49/kPa |
30 ms |
0–11 kPa |
300 |
51
|
PDMS ion gel/PVDF-HFP nanofiber |
0.43/kPa |
— |
0–1.6 kPa |
— |
52
|
RCU nanofiber |
0.174/kPa |
— |
0–10 kPa |
1000 |
53
|
PVP/MXene nanofiber |
0.5/kPa |
45ms |
0–200 kPa |
8000 |
This work |
4. Applications
Wearable miniature electronic devices have gained interest in recent years. Specifically, heart rate detection has become an attractive feature of such devices.54,55Fig. 4(a) shows the ability of the proposed sensor to detect the heart rate. The designed sensor is fixed on the volunteer's wrist, along with medical tape to detect his pulse signal. The real-time pulse of the volunteer is comparable to the pulse rate of 90 beats per min, usually detected in healthy adults. In addition, respiratory monitoring is also used, as an indispensable part of medical diagnosis. As shown in Fig. 4(b), the designed sensor is installed on the mask to monitor the normal and deep breathing of the volunteer, so as to obtain important biological signals regarding human health based on the detected breathing frequency. As the volunteer exhales, the air pressure pushes the sensor to compress, causing the relative capacitance to increase. As the volunteer inhales, the air pressure disappears and the relative capacitance gradually decreases, returning to its original value. During a deep breath, the change of the relative capacitance is larger due to a higher air pressure.
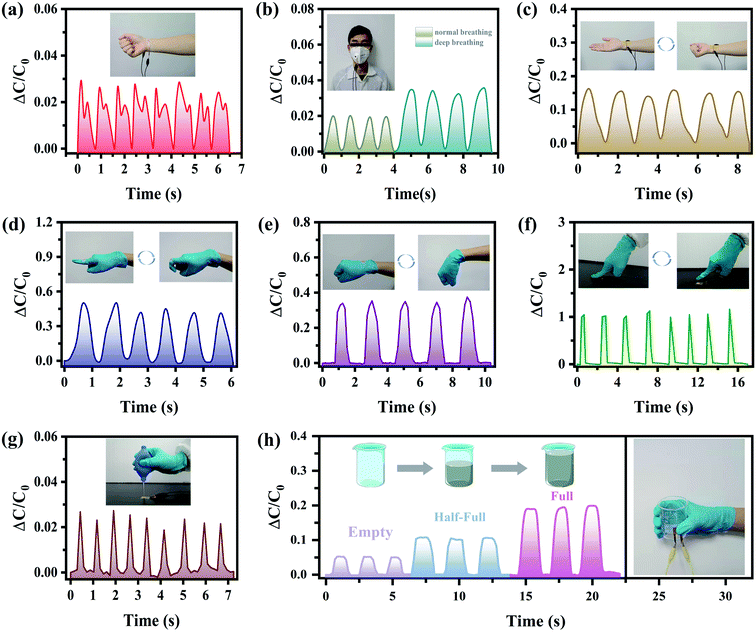 |
| Fig. 4 Applications of the MXene/PVP CNM-based sensor. (a) Real-time monitoring of human pulse wave. (b) Breath detection of normal and deep breathing. (c) The capacitance response to the muscle contraction and relaxation by opening the palm and clenching the fist. (d) The capacitance response to a finger bending at 90 degrees. (e) The capacitance response to the wrist bending at 60 degrees. (f) The capacitance response to the repeated pressing with the finger. (g) The capacitive response to a light airflow. (h) The capacitive response to holding an empty, half-full and full beaker. | |
In a further evaluation of the practical and intelligent application of the designed sensor, it is attached to body joints to detect the physiological signals of movements, providing the user with motion related feedback and guidance.56,57 Specifically, the contraction and relaxation of the wrist muscles are illustrated in Fig. 4(c). As the palm makes a fist, the muscle protrudes, exerting a pressure on the sensor, thereby increasing the relative capacitance. Monitoring muscle movements and receiving clear feedback enhance the development of healthy exercise habits in a short period of time, while improving efficiency and reducing the risk of injury. Following this, in order to monitor the joint motion of human hands, the sensor is connected to the finger (Fig. 4(d)) and wrist (Fig. 4(e)). The relative capacitance increases, as the finger/wrist bends, while it returns to the original value, when the finger/wrist relaxes. These signals show a fast and steady response to various joint motions, reflecting the sensor's ability to monitor movements in both small joints (such as fingers) and large joints (such as wrists). Next, the sensor's response to other physical stimuli is also tested. As shown in Fig. 4(f), when a finger repeatedly applies force on the sensor, the variation of the relative capacitance can quite accurately reflect the size and duration of that force. Furthermore, the sensor successfully demonstrates its ability to sense tiny airflow, as shown in Fig. 4(g). When a rubber balloon is squeezed, the generated airflow is distributed on the surface of the sensor, resulting in an obvious wave peak, within a rapid response time. This behavior will be of great help to prove its usefulness in the monitoring of atmospheric airflow and wind direction in the future. Moreover, the sensor can distinguish objects of different weights. During the respective experiment, one holds a beaker in his hand, while the sensor is fixed between the thumb and the beaker. As an empty/half-full/full beaker of water is picked up and put down repeatedly, the capacitance signal increases with the weight of the object, indicating that more force of the hand is required (Fig. 4(h)).
In order to verify the ability of the pressure sensor to obtain load information in space, a 4 × 4 sensor array is designed, as shown in Fig. 5(a). The upper and lower parts of the sensing matrix are Cu-sputtered PI electrodes, while the middle part is the MXene/PVP CNM dielectric layer. When 3D printed letters (“T”, “L”, “O”, “P”) are placed on different pixels of the sensor matrix, the device can identify the overall pressure distribution. The magnitude of the pressure applied by different weights can also be obtained based on the value of the relative capacitance change, as illustrated in Fig. 5(b)–(e). The detailed data can be found in the ESI.† These results indicate the application potential of the sensing matrix in the human–machine interface field.
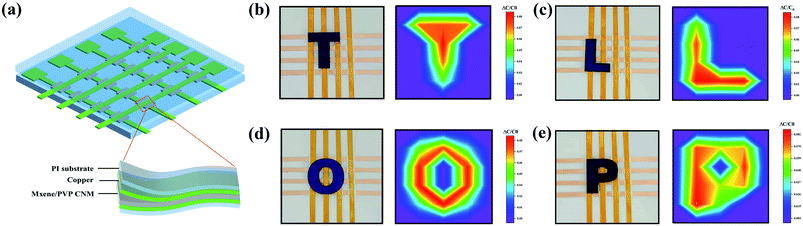 |
| Fig. 5 Spatial pressure distribution based on the sensor array. (a) Schematic diagram of the 4 × 4 sensor array. (b–e) Placement of 3D letters T, L, O and P on the sensor matrix. On the left side there is the actual shape, while on the right there is the capacitance mapping. | |
5. Conclusion
In conclusion, a high-performance flexible capacitive pressure sensor, based on MXene/PVP CNM, was developed. Different mass fractions of MXene (f = 0, 0.05, 0.1, 0.15 wt%) were added to the PVP membrane to fabricate composite dielectric layers, via electrospinning. Due to the high porosity and low compression modulus, the pressure sensor with f = 0.1 wt% demonstrated the best mechanical and electrical properties, including a high sensitivity (0.5 kPa−1 at 0–1.5 kPa), a fast response/recovery time (45/45 ms), a wide operating pressure range (0–200 kPa), an ultra-low detection limit (∼9 Pa) and an excellent stability (8000 cycles). In addition, due to its outstanding electromechanical properties, the designed sensor can be used in various pressure monitoring applications, such as pulse, respiration, human joint bending and hand-holding a beaker. Finally, a pressure mapping test of the 4 × 4 sensing array was performed, showing its great potential in future wearable medical devices and health monitoring. The developed high-performance sensor has broad application prospects in generic advanced wearable electronic devices.
Conflicts of interest
There are no conflicts to declare.
Acknowledgements
This work was supported by the National Natural Science Foundation of China (Grant No. 61904092 and 62181240278) and Natural Science Foundation of Shandong Province (No. ZR2021ME052).
References
- Q. Ma, L. Sun and B. Wang, Matter, 2022, 5, 782–784 CrossRef.
- X. Wei, H. Li, W. Yue, S. Gao, Z. Chen, Y. Li and G. Shen, Matter, 2022, 5, 1481–1501 CrossRef.
- H. B. Choi, J. Oh, Y. Kim, M. Pyatykh, J. C. Yang, S. Ryu and S. Park, ACS Appl. Mater. Interfaces, 2020, 12, 16691–16699 CrossRef CAS PubMed.
- D. J. Lipomi, M. Vosgueritchian, B. C.-K. Tee, S. L. Hellstrom, J. A. Lee, C. H. Fox and Z. Bao, Nat. Nanotechnol., 2011, 6, 788–792 CrossRef CAS PubMed.
- J. Chen, L. Li, Z. Zhu, Z. Luo, W. Tang, L. Wang and H. Li, Mater. Today Chem., 2022, 23, 100718 CrossRef CAS.
- Q. Wu, Y. Qiao, R. Guo, S. Naveed, T. Hirtz, X. Li, Y. Fu, Y. Wei, G. Deng, Y. Yang, X. Wu and T.-L. Ren, ACS Nano, 2020, 14, 10104–10114 CrossRef CAS PubMed.
- K. Meng, X. Xiao, W. Wei, G. Chen, A. Nashalian, S. Shen, X. Xiao and J. Chen, Adv. Mater., 2022, 34, 2109357 CrossRef CAS PubMed.
- W. Wang, S. Gao, Y. Li, W. Yue, H. Kan, C. Zhang, Z. Lou, L. Wang and G. Shen, Adv. Funct. Mater., 2021, 31, 2101201 CrossRef CAS.
- S. Sundaram, P. Kellnhofer, Y. Li, J.-Y. Zhu, A. Torralba and W. Matusik, Nature, 2019, 569, 698–702 CrossRef CAS PubMed.
- Y. Zheng, K. S. Beloshenko, S. Shulga, R. Wojnarowska and S. Prokhorenko, Ukr. J. Phys. Opt., 2017, 18, 102–108 CrossRef.
- Y. Zheng, K. S. Beloshenko, M. Makarovskiy, Y. Guliyova and S. Prokhorenko, Ukr. J. Phys. Opt., 2017, 18, 225–231 CrossRef.
- X. Liao, W. Wang, L. Zhong, X. Lai and Y. Zheng, Nano Energy, 2019, 62, 410–418 CrossRef CAS.
- H. Niu, S. Gao, W. Yue, Y. Li, W. Zhou and H. Liu, Small, 2020, 16, 1904774 CrossRef CAS PubMed.
- L. Bi, Z. Yang, L. Chen, Z. Wu and C. Ye, J. Mater. Chem. A, 2020, 8, 20036 Search PubMed.
- B. Chen, L. Zhang, H. Li, X. Lai and X. Zeng, J. Colloid Interface Sci., 2022, 617, 478–488 CrossRef CAS PubMed.
- E. S. Hosseini, L. Manjakkal, D. Shakthivel and R. Dahiya, ACS Appl. Mater. Interfaces, 2020, 12, 9008–9016 CrossRef CAS PubMed.
- Z. Chen, Z. Wang, X. Li, Y. Lin, N. Luo, M. Long, N. Zhao and J. B. Xu, ACS Nano, 2017, 11, 4507–4513 CrossRef CAS PubMed.
- Y. Guo, S. Gao, W. Yue, C. Zhang and Y. Li, ACS Appl. Mater. Interfaces, 2019, 11, 48594–48603 CrossRef CAS PubMed.
- M. Yue, Y. Wang, H. Guo, C. Zhang and T. Liu, Compos. Sci. Technol., 2022, 220, 109263 CrossRef CAS.
- J. Zhang, L. Wan, Y. Gao, X. Fang, T. Lu, L. Pan and F. Xuan, Adv. Electron. Mater., 2019, 5, 1900285 CrossRef.
- D. Yang, H. Guo, X. Chen, L. Wang, P. Jiang, W. Zhang, L. Zhang and Z. L. Wang, J. Mater. Chem. A, 2020, 8, 23827–23833 RSC.
- H. J. Lee, K.-Y. Chun, J. H. Oh and C.-S. Han, ACS Sens., 2021, 6, 2411–2418 CrossRef CAS PubMed.
- J. Cui, B. Zhang, J. Duan, H. Guo and J. Tang, Materials, 2017, 10, 1439 CrossRef PubMed.
- B. Ji, Q. Zhou, M. Lei, S. Ding, Q. Song, Y. Gao, S. Li, Y. Xu, Y. Zhou and B. Zhou, Small, 2021, 17, 2103312 CrossRef CAS PubMed.
- X. Li, Y. Zhao, Y. Bai, X. Zhao, R. Wang, Y. Huang, Q. Liang and Z. Huang, Electrochim. Acta, 2017, 230, 445–453 CrossRef CAS.
- H. Matsumoto and A. Tanioka, Membranes, 2011, 1, 249–264 CrossRef CAS PubMed.
- X. Yang, Y. Wang and X. Qing, Sens. Actuators, A, 2019, 299, 111579 CrossRef CAS.
- S. Zhao, W. Ran, D. Wang, R. Yin, Y. Yan, K. Jiang, Z. Lou and G. Shen, ACS Appl. Mater. Interfaces, 2020, 12, 32023–32030 CrossRef CAS PubMed.
- G.-W. Hsieh, L.-C. Shih and P.-Y. Chen, Nanomaterials, 2022, 12, 256 CrossRef CAS PubMed.
- L. Li, X. Fu, S. Chen, S. Uzun, A. Levitt, C. E. Shuck, W. Han and Y. Gogotsi, ACS Appl. Mater. Interfaces, 2020, 12, 15362–15369 CrossRef CAS PubMed.
- M. Mao, K.-X. Yu, C.-F. Cao, L.-X. Gong, G.-D. Zhang, L. Zhao, P. Song, J.-F. Gao and L.-C. Tang, Chem. Eng. J., 2022, 427, 131615 CrossRef CAS.
- J. Xu, T. Peng, X. Qin, Q. Zhang, T. Liu, W. Dai, B. Chen, H. Yu and S. Shi, J. Mater. Chem. A, 2021, 9, 14147–14171 RSC.
- S. Zhao, W. Ran, D. Wang, R. Yin, Y. Yan, K. Jiang, Z. Lou and G. Shen, ACS Sens., 2021, 6, 2630–2641 CrossRef PubMed.
- S. Sharma, A. Chhetry, M. Sharifuzzaman, H. Yoon and J. Y. Park, ACS Appl. Mater. Interfaces, 2020, 12, 22212–22224 CrossRef CAS PubMed.
- C. Jiang, C. Wu, X. Li, Y. Yao, L. Lan, F. Zhao, Z. Ye, Y. Ying and J. Ping, Nano Energy, 2019, 59, 268–276 CrossRef CAS.
- X. Liu, M. Hou, X. Luo, M. Zheng, X. Wang, H. Zhang and J. Guo, Biomacromolecules, 2021, 22, 319–329 CrossRef CAS PubMed.
- S. K. Das, T. Parandhaman and M. D. Dey, Green Chem., 2021, 23, 629 RSC.
- F. Hassouna, S. Therias, G. Mailhot and J.-L. Gardette, Polym. Degrad. Stab., 2009, 94, 2257–2266 CrossRef CAS.
- E. Yilgor, O. Kaymakci, M. Isik, S. Bilgin and I. Yilgor, Appl. Surf. Sci., 2012, 258, 4246–4253 CrossRef CAS.
- Q. Yu, B. Zhao, X. Ren, C. Zhu, Q. Wang, Y. Lin, W. Zeng, Z. Chen and S. Wang, Langmuir, 2022, 38, 5494–5501 CrossRef CAS PubMed.
- L. Ding, Y. Wei, L. Li, T. Zhang, H. Wang, J. Xue, L.-X. Ding, S. Wang, J. Caro and Y. Gogotsi, Nat. Commun., 2018, 9(1–7), 155 CrossRef PubMed.
- X. Zeng, Z. Wang, H. Zhang, W. Yang, L. Xiang, Z. Zhao, L.-M. Peng and Y. Hu, ACS Appl. Mater. Interfaces, 2019, 11, 21218–21226 CrossRef CAS PubMed.
- Y. Zhang, Y. Zhao, S. Tan and Z. Zhang, Polym. Chem., 2017, 8, 1840–1849 RSC.
- H. Zhang, Z. Zhang, K. Friedrich and C. Eger, Acta Mater., 2006, 54, 1833–1842 CrossRef CAS.
- W. Yang, N.-W. Li, S. Zhao, Z. Yuan, J. Wang, X. Du, B. Wang, R. Cao, X. Li, W. Xu, Z. L. Wang and C. Li, Adv. Mater. Technol., 2018, 3, 1700241 CrossRef.
- Prateek, V. K. Thakur and R. K. Gupta, Chem. Rev., 2016, 116, 4260–4317 CrossRef CAS PubMed.
- S.-N. Li, Z.-R. Yu, B.-F. Guo, K.-Y. Guo, Y. Li, L.-X. Gong, L. Zhao, J. Bae and L.-C. Tang, Nano Energy, 2021, 90, 106502 CrossRef CAS.
- L. Zhao, F. Qiang, S.-W. Dai, S.-C. Shen, Y.-Z. Huang, N.-J. Huang, G.-D. Zhang, L.-Z. Guan, J.-F. Gao, Y.-H. Song and L.-C. Tang, Nanoscale, 2019, 11, 10229 RSC.
- S.-W. Dai, Y.-L. Gu, L. Zhao, W. Zhang, C.-H. Gao, Y.-X. Wu, S.-C. Shen, C. Zhang, T.-T. Kong, Y.-T. Li, L.-X. Gong, G.-D. Zhang and L.-C. Tang, Composites, Part B, 2021, 225, 109243 CrossRef CAS.
- H. B. K. Muhammad, M. Ali, A. M. Soomroa, S. W. Kima, H. B. Kima, B. G. Lee and K. H. Choi, Sens. Actuators, A, 2019, 294, 140–147 CrossRef.
- Z. H. Wang, Y. Si, C. Y. Zhao, D. Yu, W. Wang and G. Sun, ACS Appl. Mater. Interfaces, 2019, 11, 27200–27209 CrossRef CAS PubMed.
- M. F. Lin, J. Q. Xiong, J. X. Wang, K. S. Parida and P. S. Lee, Nano Energy, 2018, 44, 248–255 CrossRef CAS.
- Y. B. Shin, C. J. Han, Y. M. Kim and J. W. Kim, Adv. Eng. Mater., 2021, 24, 2100903 CrossRef.
- X. Qian, M. Su, F. Li and Y. Song, Acta Chim. Sin., 2016, 74, 565–575 CrossRef CAS.
- Y. Khan, A. E. Ostfeld, C. M. Lochner, A. Pierre and A. C. Arias, Adv. Mater., 2016, 28, 4373–4395 CrossRef CAS PubMed.
- S. Park, J. Y. Cho, B. J. Lee, J.-M. Hwang, M. Lee, S. Y. Hwang, K. M. Kim, K. H. Lee and D. Park, Sci. Rep., 2020, 10, 11772 CrossRef CAS PubMed.
- M. Yang, Y. Cheng, Y. Yue, Y. Chen, H. Gao, L. Li, B. Cai, W. Liu, Z. Wang, H. Guo, N. Liu and Y. Gao, Adv. Sci., 2022, 9, 2200507 CrossRef CAS PubMed.
|
This journal is © The Royal Society of Chemistry 2022 |