DOI:
10.1039/D0NA00371A
(Paper)
Nanoscale Adv., 2020,
2, 2853-2858
Nanoscale structure detection and monitoring of tumour growth with optical coherence tomography†
Received
25th February 2020
, Accepted 22nd May 2020
First published on 25th May 2020
Abstract
Approximately 90% of cancers originate in epithelial tissues leading to epithelial thickening, but the ultrastructural changes and underlying architecture are less well known. Depth resolved label free visualization of nanoscale tissue morphology is required to reveal the extent and distribution of ultrastructural changes in underlying tissue, but is difficult to achieve with existing imaging modalities. We developed a nanosensitive optical coherence tomography (nsOCT) approach to provide such imaging based on dominant axial structure with a few nanometre detection accuracy. nsOCT maps the distribution of axial structural sizes an order of magnitude smaller than the axial resolution of the system. We validated nsOCT methodology by detecting synthetic axial structure via numerical simulations. Subsequently, we validated the nsOCT technique experimentally by detecting known structures from a commercially fabricated sample. nsOCT reveals scaling with different depth of dominant submicron structural changes associated with carcinoma which may inform the origins of the disease, its progression and improve diagnosis.
Introduction
Many early disease processes in living tissues exhibit changes at the nanometre scale. Non-invasive, label-free detection of submicron structural alterations with nanoscale accuracy in biological tissue poses significant challenges to researchers and healthcare professionals. Therefore, it is highly desirable to be able to detect these nanoscopic changes in submicron structures during physiological processes in real time. Most of the nanoscopic techniques rely on labelling and are limited to superficial imaging which is generally not suitable for in vivo applications.1–6 In the fight against cancer, nanoscopy and nanotechnology can provide molecular level contrast to detect disease earlier and more accurately.7–13 At the same time, it can help us to monitor any patient improvements during treatment. In a recent development, partial wave spectroscopy based approach demonstrated that it can detect nano-sensitive structure and its alteration in cells.14 Although, this method can only detect overall averaged structure, but not a structure hiding within scattering tissue depth. There has been progression in the development of true colour depth resolved imaging, which is based on absorption contrast of oxy and de-oxy haemoglobin and provides molecular contrast present there rather than submicron structural morphology.15 There is another recent work claiming depth resolved nanoscale mapping for cancer detection16 which we believe is based on overall average length scale present through the depth rather than depth resolved sub-voxel morphological scales. In early development, optical coherence tomography demonstrated nano-sensitive structural alteration in biological tissue, however this was again based on statistical mass distribution throughout the accessible depth.17 These techniques can only detect overall nanoscopic changes rather than depth resolved sub-voxel nanoscopic information which is crucial to visualise subtle alteration of local submicron structure for better diagnosis. In this direction, our research group recently developed nano-sensitive optical coherence tomography (nsOCT) techniques which can detect depth resolved submicron dominant structure with nanometre scale accuracy.18–20 Recently, we have demonstrated nsOCT-based correlation mapping imaging to visualize few nanometre structural changes without any labelling21 and application of the nsOCT for cornea cross-linking study.22 Another research group recently applied nsOCT for in vivo detection of nanometre scale structural changes of the human tympanic membrane in otitis media.23 The theoretical approach of nsOCT can be found in our previous published paper.19 In this present research, we have numerically validated an nsOCT approach on synthetic submicron axial structure with few nanometre accuracies. Results of numerical simulation have been confirmed via experimental nsOCT imaging of two fabricated samples (repeating axial structure 431.56 nm and 441.67 nm respectively) and by detecting structures with ∼5 nm accuracy. Finally, we have applied our developed nsOCT method to murine tumour and healthy mammary fat pad (MFP) and found interesting changes in depth resolved dominant submicron structures as the tumour progressed. Initial experimental results demonstrated new possibilities for the label free nano-sensitive detection of sub-voxel ultra-structure related to tumour progress in the MFP. Thus, nsOCT offers exciting depth resolved optical nanoscopy and far-reaching opportunities for early disease diagnosis and monitoring of treatment response.
Materials and method
Periodic submicron structure
We received two experimental samples from OptiGrate Corp. USA, with different axial periodic structure, where the corresponding periods are 431.56 nm and 441.67 nm (Fig. 1(c)). Each periodic layer fabricated with sinusoidal refractive index varies 1.483 ± 0.001. No variation in periodicity vs. time if temperature of usage will be <400 °C (periods are stable better than 1.0 pm). Thickness of each sample is ∼3 mm. We have also synthesized three axial periodic structure with 424.8 nm, 431.56 nm and 442.67 nm for numerical study (Fig. 1(a)). In axial periodic structure synthetization, we have considered same sinusoidal refractive index variation to mimic experimental sample. In additional numerical study, we have synthesized submicron structure with 444.70 nm, 451.90 nm, 462.77 nm, 473.61 nm and 488.10 nm (Fig. 2(a) and (c)). Here, we have considered sinusoidal refractive index variation 1.383 ± 0.001 to mimic tissue like refractive index variation. In an advance simulation, we have synthesized randomized submicron structure with different depths to mimic tissue (Fig. 2(e) and (f)). Here again structure varies from 444 nm to 488 nm, and we have considered sinusoidal refractive index variation 1.383 ± 0.001 over each submicron structure.
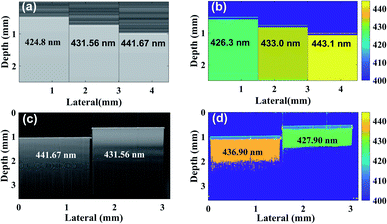 |
| Fig. 1 Simulated (a) OCT (present submicron scale structure mentioned) (b) nsOCT (detected submicron scale structure with colour bar), experimental (c) OCT (present submicron scale structure mentioned), (d) nsOCT (colour bar represents detected submicron scale structure). | |
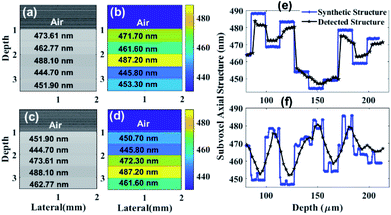 |
| Fig. 2 (a) and (c) represents OCT images with different submicron scale structure in different depth (simulated submicron scale structures mentioned at each layer). (b) and (d) represents maximum spatial period images with different submicron scale structure in different depth (detected sub-micron scale structures mentioned at each layer). Detection of randomised submicron structure with few nanometre accuracies. (e) Blue colour line (diamond) displays synthetic submicron structures throughout the depth. Black colour plot displayed detected axial sub-voxel dominant structures (maximum or most existing spatial period) throughout the depth using nsOCT methodology. (f) Blue colour line (diamond) displays synthetic submicron structures (synthetic size varies randomly over the depth) throughout the depth. Black colour plot displayed detected axial sub-voxel dominant structures (maximum spatial period) throughout the depth using nsOCT methodology. | |
Tissue samples preparation
All animal procedures were performed in accordance with the Guidelines for Care and Use of Laboratory Animals of the “Animal Care Research Ethics Committee (ACREC), National University of Ireland Galway (NUIG)” and approved by the “Health Product Regularity Authority (HPRA), Ireland”.
The acquisition of the tissue samples does not affect any ethical, health, or privacy concerns and was performed according to the ethical regulations and safety standards of NUI Galway. Female BALB/c mice (Charles River Laboratories Ltd.) aged between 6 and 8 weeks were employed. Mice received a mammary fat pad (MFP, 4th inguinal) injection of 1 × 105 4T1 breast cancer cells suspended in 100 μl RPMI medium. The tumours were detected by palpation after seven days following injection. Tumour growth was monitored using callipers measurement, and at the appropriate time animals were sacrificed by CO2 inhalation. The tumour size was 715 mm3. Tumour and healthy MFP were harvested and placed in PBS solution separately before transferring to the nsOCT imaging facility for ex vivo study. The sample was removed from PBS and mounted on a glass slide to bring the sample under the objective of spectral domain OCT system (Telesto III, Thorlabs Inc.) for nsOCT acquisition.
Analysis and detection methodology of nano-sensitive optical coherence tomography (nsOCT)
We and another research group have previously demonstrated a method, Fourier domain nano-sensitive optical coherence tomography (nsOCT), for detection of depth resolved submicron dominant structure, non-invasively with nanoscale accuracy.18,19,21 Our approach is based on finding maximum contributed spatial period (equivalent to size of dominant axial structure), calculated from corresponding OCT signal, at all accessible depth (voxels). This spatial period carries information about dominant submicron (444 nm–488 nm corresponding to the wavelength range available in our OCT system) structure within each voxel which are extremely sensitive to structural alterations. Here, we have described the principles of this approach and demonstrated its feasibility by clearly differentiating different submicron (444 nm–488 nm) structure. Initially, we have validated our approach by numerically detecting three synthetic submicron structure with ∼1.5 nm accuracy (see Fig. 1(a) and (b)).
For spectral interference data acquisition, the Telesto III (Thorlabs Inc.), a commercial spectral-domain optical coherence tomography (sdOCT) system utilized with an objective lens LSM03 (NA = 0.055, lateral resolution ≈ 13 μm). Schematic of the OCT system can be found in ESI (Fig. S1).† This sdOCT system operating with a wavelength range of λ = 1176 nm–1413 nm with centre wavelength λc ≈ 1300 nm and sensitivity 96 dB @ 76 kHz rate, can achieve an axial resolution of 5.5 μm and imaging depth up to 3.6 mm in air. Detectable spatial period (HZ = λ/2n; n = refractive index of the medium) depends on wavelength range of the broadband source.19 Therefore, in the case of biological tissue, theoretically we can detect 420 nm to 504 nm structure with few nanometre uncertainty depending upon spectrometer resolution.19 It must be noted that the range of detectable axial dominant structure depends on wavelength range of the OCT system.19 The possible detection error also depends on how closely we can scan those windows over the spectral range.19 A detailed flowchart of nsOCT analysis can be found in the ESI (Fig. S2)† and theoretical discussion can be found in our previous publication.19
Numerical simulations to construct nsOCT from synthesized submicron structures
We have followed standard FD-OCT theory and simulation strategy to implement proposed nsOCT simulation.24,25 We have used MATLAB 2019a (MathWorks®) for its implementation. For validation purpose, we performed a numerical simulation for periodicity with 424.8 nm in addition to 431.56 nm and 441.67 nm. Each periodic layer fabricated with sinusoidal refractive index varies from 1.483 ± 0.001. Each layer divided into many sub layers with thickness 0.01 nm to make it smooth enough in sinusoidal index variation to mimic fabricated layer sample from OptiGrate Corp. USA. For example, if periodicity is 424.8 nm, the number of sublayers in each periodic layer is 42
480. The OCT signal was formed as an interference of the reflected light from the layer sample with the reference wave from a gold mirror. We have also introduced suitable noise in source and detector spectra to mimic experimental reality in our simulation. The signal to noise ratio, SNR ≈ 86 dB in our simulation. The inverse Fourier transform was used to form a B-scan of these samples, presented in Fig. 1(a). For OCT simulation, similar sinusoidal index variation is considered for two different layers of thickness 441.67 nm and 431.56 nm. In addition, we have simulated for 424.8 nm periodic and repeating structure (Fig. 1(a) and (b)) to demonstrate our state of art methodology for depth resolved submicron dominant structural detection with few nanometre accuracies.
To check the capability of the nsOCT further to detect even more structural differences, we have synthesized axial submicron structure with layer thickness as 444.70 nm, 451.90 nm, 462.77 nm, 473.61 nm, 488.10 nm and with average index 1.383 ± 0.001 (Fig. 2(a) and (c)). We then numerically simulated optical coherence tomography by illuminating broadband light with wavelength range from 1176 nm to 1413 nm. We constructed nsOCT (Fig. 2(b) and (d)) following our developed methodology (see nsOCT flowchart in ESI: Fig. S2†).
In another advanced approach, we have numerically demonstrated that we can detect randomised submicron dominant synthetic structure at different depth with ∼5 nm error (see Fig. 2(e) and (f)).
Results and discussion
It is a general practice of the research community when testing any new technique to apply it in a system which is close to human and does not involve human sample study initially. Application of a new technique on animal samples also provides the opportunity to perform the required numbers of experiment to optimize experimental protocols, post processing, image analysis etc. Therefore, we have decided to use mouse mammary fat pad (MFP) to test the applicability of the comparably new nsOCT technique instead of moving straight into human tissue characterization. Here efforts were to demonstrate new technical capabilities which has proved can be deployed in the future to access human skin via a hand-held probe or can be adopted with an endoscopic system for imaging of the intestine wall for early disease diagnosis based on nano-sensitive structural detection.
Validation of nano-sensitive optical coherence tomography (nsOCT) numerically and experimentally in submicron axial structure
For validation purposes, we have numerically constructed OCT images from different synthetic axial structures (axial structure size mentioned on each OCT B-Scan, Fig. 1(a)). Fig. 1(b) displays numerically constructed nsOCT following nsOCT methodology (see nsOCT flowchart in ESI: Fig. S2†) and detected submicron axial structure mentioned on each OCT B-Scan. It is clearly demonstrated that our proposed numerical technique can detect axial submicron structure with ∼1.5 nm accuracy (compare Fig. 1(a) and (b)). As a proof of concept experiment, state of the art nsOCT approach was applied on spectral OCT signal from two fabricated samples with two different repeating axial periodic submicron structures of 441.67 nm and 431.56 nm, respectively. Interference spectra was obtained using spectral domain OCT (SDOCT) system (TELESTO III, Thorlabs, Inc.) and constructed OCT images (B-Scan) are presented in Fig. 1(c). Subsequently, we have applied the nsOCT approach on spectral OCT signal to extract dominant spatial period at each voxel of each Aline. These nsOCT images (B-Scan) were formed as colour maps of detected spatial periods and displays in Fig. 1(b) (numerically constructed) and Fig. 1(d) (experimentally). Hence, all areas with similar axial structure are visualized with the same colour. The depth of the preliminary nsOCT image was limited to ∼1.0 mm by thresholding, and further optimization could increase the depth of access. Note that, in this experimental study, our detection accuracy is ∼ 5 nm. Therefore, we can confirm that numerical simulation and experimental approach can detect and differentiate dominant nanostructures in different samples through state of the art nsOCT methodology with 5 nm accuracy. Although we have validated our approach numerically and experimentally, still it may be required as to whether our proposed technique is applicable if we have different submicron structures at different depths? To check applicability of our proposed nsOCT method, we have synthesized five layers with thickness ∼ 0.5 mm each and composed with five different submicron structures like 444.70 nm, 451.90 nm, 462.77 nm, 473.61 nm, 488.10 nm and placed in five different depth to construct a B-Scan.
In Fig. 2(a), we have displayed constructed B-Scan OCT image of these synthesized layers (structural composition of each layers mentioned on the B-Scan) and corresponding dominant spatial period (B-Scan) where we can see detected submicron structures with ∼1.5 nm accuracy (compare Fig. 2(a) and (b)). Now, if we interchange each layer with their respective submicron structures throughout the depth, we can again detect them with ∼1.5 nm accuracy (see results in Fig. 2(c) and (d)). Therefore, we have demonstrated that different dominant submicron structures at different depths can be detected through proposed state of the art nsOCT technique. However, further confirmation is required regarding applicability of nsOCT in practical situations, for example biological tissue samples composed of randomized submicron structure throughout the depth and does not expect to follow layer structure what we have demonstrated in Fig. 2(a) – (d). Therefore, in an advanced numerical simulation approach (see Fig. 2(e) and (f)), we have demonstrated the detection of randomised synthetic submicron structures. We have displayed synthetic submicron structures (Y-axis: sub-voxel axial structure) through the depth (X-axis) in blue colour line. Here, synthetic submicron axial structures vary from 0.445 μm to 0.488 μm. Subsequently, we have displayed detected axial sub-voxel dominant structures with ∼5 nm accuracy in black colour plot throughout the depth using the proposed nsOCT methodology. This demonstration confirmed the applicability and reliability of our proposed nsOCT technique to detect depth resolved submicron structures with few nanometre accuracies.
Detection of sub-voxel informative nano-sensitive submicron structure in healthy MFP and tumour murine tissue
After successful validation of the nsOCT approach via numerical simulation and experimental approach, we have applied our nsOCT techniques on freshly dissected tissue samples with healthy MFP and tumour portion. Fig. 3(a) and (b) represents conventional H&E stained histological images from healthy MFP and tumour tissue surface. We can notice qualitative structural differences between them but are unable to quantify based on structural changes and it is also not a label free technique. Fig. 3(c) and (d) represents conventional OCT volume constructed from healthy MFP and tumour tissue, respectively. Note that, it is impossible to differentiate from healthy MFP to tumour tissue visually in OCT as it loses submicron structural information in conventional OCT construction (axial resolution in tissue is ∼4.0 μm, lateral resolution ∼13.0 μm). Note that, resolution might be degraded over depth due to dispersion mismatch and other issues. However, we are not expecting resolution change to have an effect in our study much as nsOCT detection is based on spectral features of the interference signal and can detect submicron dominant structures which is much smaller than resolution itself.
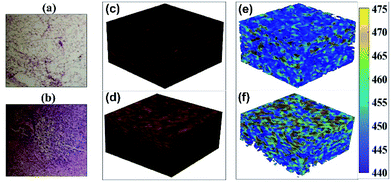 |
| Fig. 3 H&E stained histological images (200 μm × 200 μm) of (a) normal and (b) tumour tissue. (c) and (d) 3D volume (250 μm × 250 μm × 180 μm) OCT images of healthy and tumour tissue. (e) and (f) Dominant submicron axial structural mapped 3D volume OCT images of healthy and tumour tissue, respectively. Colour bar represents size of dominant submicron axial structures. | |
It is also worthy to mention here that lateral resolution simply can be improved by using high NA objective but will reduce the depth of access. Axial resolution also can be improved by using higher wavelength band OCT system. In this present research, the lateral resolution is far larger than detected dominant axial structure and it is expected to have a different dominant axial structure at different lateral (2D) location within resolution itself. In principle, we can detect different dominant axial structural information at different locations within resolution by performing dense scanning and accruing different A-lines. In this study, we have also performed dense scanning over 1.0 μm interval providing 13 × 13 different values of dominant structure within a lateral resolution. Note that, we have also axial resolution around 5.0 μm and even more in nsOCT images (∼20.0 μm considering whole spectral range divided into four windows for nsOCT construction) as we are doing windowing over interference spectra to construct nsOCT. Here, the main theme is that we have been able to detect submicron scale dominant structure within a 13 μm × 13 μm × 20 μm voxel. Regarding imaging data volume, it is essential to mention that nsOCT technique required reduced amount of memory to process and store data compared to other nanoscale imaging technique with relatively the same amount of information from the same volume of interest. For example, with 10 nm resolution imaging technique, it required 13
000 × 13
000 × 20
000 voxels to process and store images compared to single voxel in nsOCT with similar amount of information and with the same volume of interest. Fig. 3(e) and (f) displays volume nsOCT where dominant axial submicron structure (most existing spatial period in a voxel or maximum spatial period) mapped onto healthy MFP and tumour tissue, respectively. Notice that, these dominant nano structural maps provide clear differences between healthy MFP and tumour tissue. Observation: lower submicron structure (445 nm–455 nm) was more prominent in healthy MFP tissue where higher submicron structure (460 nm–470 nm) was more prominent on tumour tissue. Details of enface vs. depth scan video with comparison between healthy MFP and tumour submicron structure can be found in our ESI video files (ESI_video1: EnfaceHZvs Depth_Healthy.avi, ESI_video2: EnfaceHZvsDepth_Tumour.avi).† Although, it is clear from enface images that there is an alteration in submicron dominant structure, it is essential to quantify overall structural distribution over enface images to differentiate tumour from healthy portion clinically. Fig. 4(a) and (b) displays histogram of dominant structure over superficial enface for healthy and tumour tissue region, respectively. Fig. 4(c) displays distribution of axial submicron structure throughout the volume of interest. Similar trends were obvious (smaller and larger than 455 nm structure dominating in healthy and tumour tissue respectively) as observed in (Fig. 4(a) and (b)) axial structural distribution in the first enface image from healthy and tumour tissue. We can clearly see alteration in dominant structural distribution from healthy MFP to tumour: structure bellow 455 nm is more populated in healthy tissue in contrast to tumour tissue where axial structure larger than 455 nm is more populated. Note that, we have a limitation of our detection from 444 nm to 488 nm which is limited by wavelength range. We have found that 455 nm structure are more populated in tumour tissue compare to healthy MFP within our detection range (444 nm to 488 nm). There may be other axial structures which are also populated in tumour tissue beyond our detection range and this requires further investigation with an OCT system with a broader wavelength band In line with this, our research group is developing a new ultra-high wavelength band OCT system for further investigation. For statistical significance and clinical applicability, it is advisable to look at statistics or size distribution at each depth (enface) and their alteration as the tumour progresses in multiple samples. In future studies, we will perform the nsOCT study on as many samples as possible to find consistent and statistically significant differences if any.
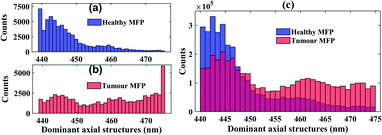 |
| Fig. 4 (a) and (b) Histogram of dominant structure over superficial enface slice for healthy and tumour tissue regions, respectively. (c) Volume (250 μm × 250 μm × 180 μm) histogram represents overall distribution of dominant submicron structure throughout healthy (blue colour) and tumour (red colour) tissue samples. | |
In ESI Fig. S3,† we have displayed a means of dominant submicron structure over each enface corresponding to each depth of access. We have performed averaging of dominant structure over eight healthy tissue spots and ten tumour spots with area (250 μm × 250 μm) each. Higher trends of mean dominant submicron axial structure through analysed depth indicates increasing trends of size of submicron structures in tumour tissue compare to healthy MFP. Observations: overall ∼10 nm changes occur close to tissue surface in transition from healthy to tumour. Possible reason may be due to swelling of cells, cell organelles during tumour progression.
Conclusions
In conclusion, a Fourier domain nano-sensitive optical coherence tomography (nsOCT) method is validated, adapted, and explored for extraction and quantification of submicron scale axial structure with few nanometre accuracies. This adapted nsOCT technique was successfully applied in depth wise randomised synthetic submicron axial structure with ∼5 nm detection accuracy. Experimental validation of this nsOCT construction method was performed on fabricated samples with different submicron structure and its detection with similar accuracy. The agreement between the synthesized and nsOCT extracted structure, establishes self-consistency. We have also demonstrated that subtle changes (few nanometre) in depth resolved submicron structure can indeed be probed and quantified from OCT interference spectra, despite numerous complexities associated with the wide range of axial scaling at different depth. Subsequently, the developed methodology was applied in biological tissues to extract depth wise sub-voxel axial submicron structure. Results suggested that size of the overall submicron dominant structure increased by ∼10 nm as the tumour progressed. The demonstrated ability to delineate submicron scale axial structural properties may provide a valuable non-invasive tool for characterization of tissue and a wide variety of other complex scattering media of non-biological origin with few nanometre accuracies. Observed differences in the submicron scale axial morphology distribution in tissue volume between healthy and tumour MFP shows considerable promise as a potential label free biomarker for early cancer detection and monitoring treatment response. These abilities to probe and quantify nano-sensitive morphological and structural alterations associated with cancer development using the nsOCT in backscattering mode bodes well for in vivo deployment, because the backscattering geometry is clinically convenient, and the measurement technique is straight-forward. Exploiting the elastic backscattering spectra (singly or weakly scattered) recorded from tissue depths by interfering with reference signal, in vivo applications of this approach can be realized with a suitably designed fibre optic probe. Finally, adapted nsOCT method represents as a fundamentally new and novel approach with great potential, but its actual benefits for in vivo detection of cancer initiation remains to be rigorously evaluated.
Ethical statement
All animal procedures were performed in accordance with the Guidelines for Care and Use of Laboratory Animals of the “Animal Care Research Ethics Committee (ACREC), National University of Ireland Galway (NUIG)” and approved by the “Health Product Regularity Authority (HPRA), Ireland”.
Conflicts of interest
There are no conflicts to declare.
Acknowledgements
This project received funding from Irish Research Council (IRC), under Government of Ireland postdoctoral fellowship with project ID: GOIPD/2017/837. Nandan Das acknowledge National University of Ireland Galway (NUIG) for research facilities. Also, this project has received funding from the European Union's Horizon 2020 research and innovation program under grant agreements no. 761214 and no. 779960. The materials presented and views expressed here are the responsibility of the author(s) only. The EU Commission takes no responsibility for any use made of the information set out. This work was supported by NUI Galway, Galway University Foundation, the University of Limerick Foundation, the National Biophotonics Imaging Platform (NBIP) Ireland funded under the Higher Education Authority PRTLI Cycle 4 and co-funded by the Irish Government and the European Union.
Notes and references
- P. C. Montgomery and A. Leong-Hoi, Emerging optical nanoscopy techniques, Nanotechnol., Sci. Appl., 2015, 8, 31–44 CrossRef CAS PubMed.
- S. J. Sahl, S. W. Hell and S. Jakobs, Fluorescence nanoscopy in cell biology, Nat. Rev. Mol. Cell Biol., 2017, 18, 685 CrossRef CAS PubMed.
- U. V. Nägerl and T. Bonhoeffer, Imaging Living Synapses at the Nanoscale by STED Microscopy, J. Neurosci., 2010, 30, 9341 CrossRef PubMed.
- R. P. J. Nieuwenhuizen,
et al., Measuring image resolution in optical nanoscopy, Nat. Methods, 2013, 10, 557 CrossRef CAS PubMed.
- C. A. Wurm,
et al., Novel red fluorophores with superior performance in STED microscopy, Opt. Nanoscopy, 2012, 1, 7 CrossRef.
- E. Rittweger, K. Y. Han, S. E. Irvine, C. Eggeling and S. W. Hell, STED microscopy reveals crystal colour centres with nanometric resolution, Nat. Photonics, 2009, 3, 144–147 CrossRef CAS.
- S. W. Hell, Far-Field Optical Nanoscopy, Science, 2007, 316, 1153 CrossRef CAS PubMed.
- S. Suran, K. Bharadwaj, S. Raghavan and M. M. Varma, Bright-field Nanoscopy: Visualizing Nano-structures with Localized Optical Contrast Using a Conventional Microscope, Sci. Rep., 2016, 6, 25011 CrossRef CAS PubMed.
- S. W. Hell, R. Schmidt and A. Egner, Diffraction-unlimited three-dimensional optical nanoscopy with opposing lenses, Nat. Photonics, 2009, 3, 381–387 CrossRef CAS.
- G. Zanini, K. Korobchevskaya, T. Deguchi, A. Diaspro and P. Bianchini, Label-Free Optical Nanoscopy of Single-Layer Graphene, ACS Nano, 2019, 13, 9673–9681 CrossRef CAS PubMed.
- Y. Zhao,
et al., Nanoscale imaging of clinical specimens using pathology-optimized expansion microscopy, Nat. Biotechnol., 2017, 35, 757 CrossRef CAS PubMed.
- O. Bucur and Y. Zhao, Nanoscale Imaging of Kidney Glomeruli Using Expansion Pathology, Front. Med., 2018, 5, 322 CrossRef PubMed.
- I. Øie Cristina,
et al., New ways of looking at very small holes – using optical nanoscopy to visualize liver sinusoidal endothelial cell fenestrations, Nanophotonics, 2018, 7, 575 Search PubMed.
- S. Gladstein,
et al., Multimodal interference-based imaging of nanoscale structure and macromolecular motion uncovers UV induced cellular paroxysm, Nat. Commun., 2019, 10, 1652 CrossRef PubMed.
- F. E. Robles, C. Wilson, G. Grant and A. Wax, Molecular imaging true-colour spectroscopic optical coherence tomography, Nat. Photonics, 2011, 5, 744–747 CrossRef CAS PubMed.
- S. Uttam,
et al., Early Prediction of Cancer Progression by Depth-Resolved Nanoscale Mapping of Nuclear Architecture from Unstained Tissue Specimens, Cancer Res., 2015, 75, 4718–4727 CrossRef CAS PubMed.
- J. Yi,
et al., Can OCT be sensitive to nanoscale structural alterations in biological tissue?, Opt. Express, 2013, 21, 9043–9059 CrossRef PubMed.
- S. A. Alexandrov, H. M. Subhash, A. Zam and M. Leahy, Nano-sensitive optical coherence tomography, Nanoscale, 2014, 6, 3545–3549 RSC.
- S. Alexandrov, H. Subhash and M. Leahy, Nanosensitive optical coherence tomography for the study of changes in static and dynamic structures, Quantum Electron., 2014, 44, 657–663 CrossRef CAS.
-
S. Alexandrov, H. Subhash, M. Leahy and A. Zam, A nano-sensitive Fourier-domain optical coherence tomography inspection system, US Pat. US20160238370 A1, 2014, issued July 3, 2018 EP3055643 B1, 2014, issued July 31, 2019.
- S. Alexandrov,
et al., Spatial frequency domain correlation mapping optical coherence tomography for nanoscale structural characterization, Appl. Phys. Lett., 2019, 115, 121105 CrossRef.
- Y. Zhou,
et al., Noninvasive detection of nanoscale structural changes in cornea associated with cross-linking treatment, J. Biophotonics, 2020, e201960234 CAS.
- R. Dsouza,
et al., In vivo detection of nanometer-scale structural changes of the human tympanic membrane in otitis media, Sci. Rep., 2018, 8, 8777 CrossRef PubMed.
- Y. Rao, N. P. Sarwade and R. Makkar, Modeling and Simulation of Optical Coherence Tomography on Virtual OCT, Procedia Comput. Sci., 2015, 45, 644–650 CrossRef.
-
P. M. d. S. Carvalho, Optical coherence tomography Layout Simulation Using MATLAB®, FCTUC Física - Teses de Mestrado (Master Dissertation), University of Coimbra, APPENDIX B, 2016, pp. 133–135.
Footnote |
† Electronic supplementary information (ESI) available: OCT schematic; nsOCT flowchart; maximum spatial period vs. depth video: healthy and tumour. See DOI: 10.1039/d0na00371a |
|
This journal is © The Royal Society of Chemistry 2020 |