Predictive quantitative structure–property relationship (QSPR) modeling for adsorption of organic pollutants by carbon nanotubes (CNTs)†
Received
22nd September 2018
, Accepted 16th November 2018
First published on 16th November 2018
Abstract
Nanotechnology has introduced a new generation of adsorbents like carbon nanotubes (CNTs), which have drawn a widespread attention due to their outstanding ability for the removal of various inorganic and organic pollutants. The goal of this study was to develop regression-based quantitative structure–property relationship (QSPR) models for organic pollutants and organic solvents using only easily computable 2D descriptors to explore the key structural features essential for adsorption to multi-walled CNTs and improve the dispersibility index of single-walled CNTs. The statistical results of the developed models showed good quality and predictivity based on both internal and external validation metrics (dataset 1: R2 range of 0.893–0.920, Q2(LOO) range of 0.863–0.895, Q2F1 range of 0.887–0.919; dataset 2: R2 range of 0.793–0.845, Q2(LOO) range of 0.743–0.798, Q2F1 range of 0.783–0.890; dataset 3: R2 = 0.830, Q2(LOO) = 0.775, Q2F1 = 0.945). We have also tried to explore whether the quality of the predictions of test set compounds can be enhanced through an “intelligent” selection of multiple models using the “Intelligent consensus predictor” tool. The consensus results suggested that the consensus predictivity of the test set compounds gave better results than those from the individual MLR models based on different criteria (dataset 1: Q2F1 = 0.935, Q2F2 = 0.935, MAE(95%) = good; dataset 2: Q2F1 = 0.887, Q2F2 = 0.879, MAE(95%) = good). The contributed descriptors obtained from different models suggested that the organic pollutants may adsorb to the CNTs through hydrogen bonding interactions, π–π interactions, hydrophobic interactions and electrostatic interaction. Based on the observations obtained from the developed models, we have inferred that the adsorption of the organic pollutants onto the CNTs can be enhanced by the following factors: a higher number of aromatic rings, high unsaturation or electron richness of molecules, the presence of polar groups substituted in the aromatic ring, the presence of oxygen and nitrogen atoms, the size of the molecules, and the hydrophobic surface of the molecules. On the other hand, the presence of C–O groups, aliphatic primary alcohols and the presence of chlorine atoms may retard the adsorption of organic pollutants. The results also suggest that the organic solvents bearing the >N- fragment, a higher degree of branching (compactness), polar solvents with low donor number and lower ionization potential may be better solvents for enhancing the dispersibility of single-walled CNTs.
Environmental significance
Nanotechnology has introduced a new generation of adsorbents such as carbon nanotubes (CNTs), which have drawn widespread attention due to their outstanding ability for the removal of various inorganic and organic pollutants. The goal of this study was to develop quantitative structure–property relationship (QSPR) models to explore the key structural features of organic pollutants, which are essential for adsorption to multi-walled CNTs. We have also developed models to investigate the characteristics that can improve the dispersibility of single-walled CNTs. This information may be helpful in the process of removal of the harmful and toxic contaminants/disposal of the by-products from various industries by increasing the adsorption of pollutants and the dispersibility of CNTs, thus making a pollution-free environment.
|
1. Introduction
A noticeable amount of organic pollutants is released into the environment via various routes like the burning of fossil fuels, wastes from incineration, exhausts from automobiles, agricultural processes and industrial sectors. The disposal of the by-products from the various industries is a challenging job for environmentalists and industries. The major problem with pollutants is their effective and safe disposal without further affecting the environment adversely. The organic pollutants (phenols, cresols, alkyl benzene sulfonates, nitro chlorobenzene, chlorinated paraffins, butadiene, synthetic dyes, insecticides, fungicides and pesticides, etc.) accumulate in the food chain and persist in nature and cause a significant threat to the environment.1–4 The United States Environmental Protection Agency (EPA) has set maximum contamination levels (MCLs) and maximum contamination level goals (MCLG) for each pollutant, with no ill health effects. Sometimes the MCL level goes beyond the MCLG level because of the problem in determining small quantities of contaminants and due to lack of availability of treatment technologies and analytical methods.5–14 Thus, for the protection of the environment, the use of new and advanced materials is important. In recent years, greater focus has been placed on nanostructures as adsorbents and catalysts for removing the harmful and toxic contaminants from the environment.15–17 Among the various nanomaterial adsorbents, carbon nanotubes (CNTs) have been thoroughly investigated because they have a large surface area to volume ratio, inertness towards chemicals, light mass density, porous structure, great physical and chemical properties, small diameter, extraordinary optical and electrical properties, high tensile strength and efficient affinity towards pollutants. The possibility of surface modification with different functional groups makes CNTs good adsorbents18–20 and enhances their reactivity and dispersibility for environmental protection applications.
SWCNTs have some unique mechanical, electrical and thermal properties but possess poor solubility as well as poor dispersibility in aqueous and other common organic solvents.21 They possess high polarizability along with van der Waals interactions and hydrophobic surface, so they are able to form aggregates with each other and with other biological and chemical systems to produce mixtures of aggregates, specifically in water.22,23 This bundling or entangling feature of SWCNTs causes difficulties in the dispersion of CNTs in various solvents or matrices.24–26 This also prevents the exploration of the chemistry of CNTs at a molecular level and hinders their applications27 as well as limits the availability of adsorption sites for the adsorption of pollutants on the CNT surface.28 The morphology variation of CNTs may also result in a difference in their aggregation tendencies, which may additionally impact their adsorption capability. The major interactions are van der Waals interactions, π–π stacking, and hydrophobic interactions for dispersibility, as suggested by many researchers.29
Hyung et al.30 reported that organic contaminants can interact with carbon nanotubes in aquatic systems and increase their stability and transport and thus, the mobility of the adsorbed organic matters on CNTs can be enhanced. The popularity of CNTs has increased since Long and Yang first reported that they can efficiently remove dioxins as compared to activated carbon.31 The sorption studies performed on CNTs for metal ions32 and organic contaminants, such as butane,33 trihalomethanes,34 dioxin,31 xylenes,35 chlorophenols,36 1,2-dichlorobenzene,37 resorcinol38 and polycyclic aromatic hydrocarbons (PAHs),15,39 suggest that CNTs can remove both organic and inorganic pollutants from water and gases.
Although a large number of pollutants are reported in the literature, adsorption data is available for only around 70
000 pollutants.40 The determination of experimental data for a large number of pollutants is time-consuming as well as laborious and costly. The surface properties of CNTs can be modified by treating them with some active chemicals so that the CNTs do not aggregate or form bundles and hence, the dispersion of CNTs can be enhanced. QSPR modeling of organic pollutants/solvents using adsorption properties/dispersibility index by CNTs can, therefore, be of great importance for researchers and practitioners. The quantitative structure–property relationship (QSPR) approach is easier than the thermodynamic model since the input parameters of QSPR can be more easily obtained as compared to the thermodynamic models.41 QSPR not only reduces the experimental work but also predicts the features based on the chemical structures. Thus, the rationalization ideas obtained from such models provide the researchers with a conceptual framework upon which a firm discussion can be based. Recently, a great deal of work has been done with QSPR and linear surface energy relationship (LSER) modeling to develop predictive models for CNTs, including the adsorption of organic chemicals (OCs) by CNTs,41–47 dispersibility of CNTs in organic solvents48–51 and other properties similar to CNTs. In the past, some work has been done by researchers, for example, linear LSER models were developed by Xia et al.43 using the biological surface index (BSAI) for the prediction and characterization of the intermolecular adsorption of OCs by CNTs. Apul et al.45 reported a 3D-QSPR modeling applying the same data sets for the adsorption of aromatic compounds by CNTs and compared it with MLR, ANN and SVM methods. Another QSPR model was reported by Yilmaz et al.48 using additive descriptors and quantum-chemical descriptors for the determination of the dispersibility of CNTs in different organic solvents.
The objective of the present study has been to develop statistically significant QSPR models of organic pollutants with multiple-endpoints using only easily computable 2D descriptors to explore the key structural features that are essential for adsorption to MWCNTs. We have also developed a QSPR model for organic solvents to investigate the characteristics of molecules that can improve the dispersibility of SWCNTs and may overcome the drawbacks of SWCNTs. A variable selection strategy was also employed prior to the development of final models to reduce noise in the input. We have also tried to explore whether the quality of predictions of test set compounds can be enhanced through the “intelligent” selection of multiple MLR models using an “Intelligent consensus predictor” tool.
2. Methods and materials
2.1. Dataset
We have developed QSPR models separately, using three different data sets for diverse organic contaminants with multiple-endpoints of carbon nanotubes reported in the literature.41,44,52 The first dataset involves the defined adsorption affinity properties (k∞) of 59 organic contaminants by multi-walled carbon nanotubes (MWCNTs). The second dataset involves the adsorption affinity of 69 organic contaminants related to the specific surface area (kSA) of multi-walled carbon nanotubes (MWCNTs), and the third data set involves 29 organic solvents with defined dispersibility index values (Cmax) for single-walled carbon nanotubes (SWCNTs). We have not excluded any compound of individual data sets in our modeling analysis. All the endpoint values were taken in the logarithmic scale for the modeling purposes. The first two data sets mainly involve adsorption data for synthetic organic compounds like pyrene, naphthalene, phenol, benzene, aniline, benzoate, chloroanisole, alcohol, acetophenone, isophoron, phenanthrene dicamba, atrazine, carbamazepine, pyrimidinone, acetamide, piperidine, propionitrile, acrylic acid, thiodiethanol, ethanolamine, cyclopentanone, acetone and ethylene glycol derivatives, while the third data set is related to different types of solvents. The dispersibility of single-walled carbon nanotubes (SWCNTs) was measured in different solvent ranges. Here, Cmax (mg mL−1) represents the maximum dispersibility of single-walled carbon nanotubes, K∞ and KSA are both adsorption coefficients that can be obtained from isotherm data. K∞ is the ratio of qe and Ce (solid and liquid phase equilibrium concentrations, respectively, at infinite dilution conditions with an average of 0.2% aqueous solubility). KSA is the normalized value of K∞ and the specific surface area of multi-walled carbon nanotubes (MWCNTs). The data sets are given in Tables S1, S2 and S3 in the ESI† section.
2.2. Descriptor calculation
“The molecular descriptor is the final result of a logic and mathematical procedure which transforms chemical information encoded within a symbolic representation of a molecule into a useful number or the result of some standardized experiments”. All the dataset compounds were drawn using the Marvin Sketch software.53 The descriptors were calculated using two software tools, namely, Dragon software version 6,54 and PaDEL-descriptor55 software. In this work, we have calculated only 2D descriptors covering constitutional, ring descriptors, connectivity index, functional group counts, atom centered fragments, atom type E-states, 2D atom pairs, molecular properties (using Dragon software version 6) and ETA indices (using PaDEL-Descriptor software).
2.3. Data set division
Division of the dataset is a very important step for QSPR. The present work deals with three datasets containing diverse organic pollutants or solvents. In each case, all the dataset compounds were divided into a training set and a test set using the “Modified k-medoid” clustering technique. The clustering technique categorizes a set of compounds into clusters so that the compounds present in the same cluster are similar to each other. On the other hand, when two compounds belong to two different clusters, they are said to be dissimilar in nature. The indicative compounds within a cluster are called medoids. This technique tends to select k from most middle objects or compounds as the initial medoid. Three clusters were generated for the dataset containing 59 and 29 compounds, while six clusters were generated for the dataset containing 69 compounds. We have selected approximately 25% of compounds from each data set for the test set and the remaining 75% of compounds were selected for the training set. The purpose of the training set was to develop the model and the test set was used to validate the model for prediction purposes. The same strategy was applied in the case of all three datasets for training and test set division.
2.4. Variable selection and model development
After the dataset division step, we performed data pretreatment to remove intercorrelated descriptors from all three sets of datasets. Prior to the development of final models, we tried to extract the important descriptors from the large pool of initial descriptors using various variable selection strategies.56,57 In case of the dataset containing 59 and 69 organic pollutants, we separately ran a stepwise regression and selected some descriptors in each case. After removing the selected descriptors obtained from the first stepwise regression run, we ran the stepwise regression again using the remaining pool of descriptors, and we repeated the same procedure. In this way, we selected some manageable numbers of descriptors and made a reduced pool of descriptors. In the case of the dataset containing 29 compounds, we developed GA equations and made a descriptor pool using the descriptors obtained from the GA (genetic algorithm) equations. After that, we ran the best subset selection for all three datasets using the reduced pools of descriptors. For this, we used a tool developed in our laboratory.58 Five (three models were selected) and four (two models were selected) descriptor models were generated in the case of the dataset containing 59 organic pollutants, whereas six (three models were selected) and five (two models were selected) descriptor models were generated for the dataset containing 69 organic pollutants. Among the equations generated from the best subset selection, we selected five models, five models and four models for 59, 69 and 29 compounds, respectively, based on MAE criteria.59 Descriptors were selected from the GA and stepwise regression models and a descriptor pool was generated. Finally, the selected models were run using the intelligent consensus predictor (ICP) tool developed in our laboratory60 to explore whether the quality of predictions of external compounds could be enhanced through an “intelligent” selection of multiple models (in this report, five models were selected).
The multilayered strategies like data pretreatment,58 stepwise regression,61 genetic method62 and best subset selection58 were involved for the selection of variables prior to the development of the final models and different steps are discussed separately in the ESI† section.
2.4.1. Intelligent consensus predictor (ICP).
60
This software was used to judge the performance of consensus predictions in comparison to their quality obtained from the individual (MLR) models based on the MAE based criteria (95%). It is obvious that a single model might not be equally useful for prediction for the whole test set compounds, which means that one QSPR model may be the best model for prediction of a test compound while the other model may be the best predictor for another test compounds. For this reason, we have selected five models in the case of a dataset containing 59 (M1–M5) and 69 (N1–N5) organic contaminants, and performed consensus prediction using the “Intelligent consensus predictor” tool to explore whether the quality of the predictions of the test set compounds could be enhanced through an “intelligent” selection of multiple models. The steps involved in the development of both MLR and PLS models are represented schematically in Fig. 1.
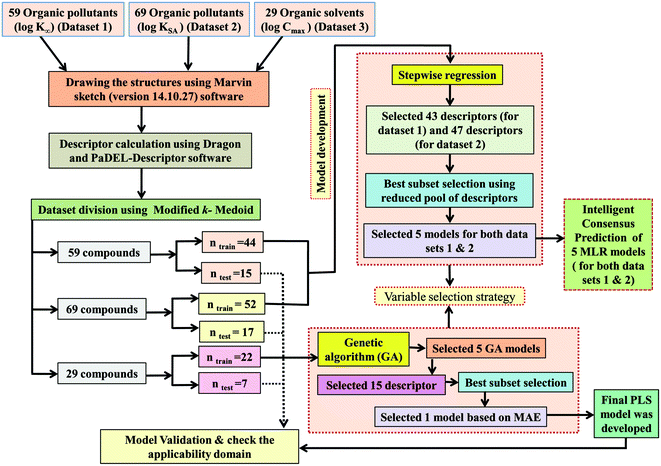 |
| Fig. 1 Schematic representation of the steps involved in the development of QSPR models. | |
2.5. Statistical validation metrics
In order to judge the predictivity and reliability of the developed QSPR models, we have examined the statistical quality, applying both internal and external validation metrics. In this work, we have used various statistical parameters like determination coefficient R2, explained variance R2a, variance ratio (F), and standard error of estimate (s). These parameters are not sufficient to evaluate the predictive potential of the model, so we have used some other classical parameters for validation of the models. The internal predictivity parameters like the leave-one-out cross-validated correlation coefficient (Q2LOO), and external predictivity parameters like R2pred or Q2F1, Q2F2 and concordance correlation coefficient (CCC), were also calculated. We also calculated some r2m parameters like r2m(LOO) and Δr2m(LOO) for internal validation and r2m(test) and Δr2m(test) for external validation.63 The basic objective of the predictive performance of QSPR models is to investigate the prediction errors of an external set, which should be within the chemical and response-based domain of the internal set (i.e., training set). The Q2ext-based metrics (i.e., R2pred and Q2F2) are not always able to provide the correct indication of the prediction quality because of the influence of the response range as well as the distribution of the values of response in both the training and test set compounds.59 Thus, we have also validated the models using the mean absolute error (MAE) criteria for both external and internal validation.59 The error based metrics were used to determine the true indication of the prediction quality in terms of prediction error since they do not evaluate the performance of the model in comparison with the mean response (Roy et al., 2016 (ref. 59)). The threshold values of Q2, Q2F2, R2pred, r2m(test), r2m(LOO) are 0.5 and for CCC, it is 0.750.64,65 The limit for Δr2m(test) and Δr2m(LOO) is 0.2. Recently, Roy et al. reported that a single model might not be equally useful in the prediction for the whole test set compounds, i.e., one QSPR model may be the best model for prediction of a test compound while the other model may be the best predictor for another test compound. For this reason, we have also performed Intelligent consensus prediction (ICP) using multiple QSPR models to determine whether the quality of the predictions of test set compounds can be enhanced through an “intelligent” selection. Here, a simple average of predictions from all the models is not considered; only ‘qualified models’ are taken into account.
2.6. Applicability domain
“The applicability domain of a (Q)SAR is the physicochemical, structural, or biological space, knowledge or information on which the training set of the model has been developed, and for which it is applicable to make predictions for new compounds. The applicability domain of a (Q)SAR should be described in terms of the most relevant parameters, i.e., usually those that are descriptors of the model. Ideally, the (Q)SAR should only be used to make predictions within that domain by interpolation not extrapolation”. The AD of the QSAR model is characterized by the molecular properties of the training set compounds. The AD criteria help to check whether the test/query compound under consideration is inside the AD or not. Here, we have checked the applicability domain of test set compounds of the developed models, employing the standardization approach (for first two data sets) using the software developed in our laboratory66 and a DModX (distance to model X) approach67 at 99% confidence level using SIMCA-P software68 (for the third data set). The predictability of a QSPR model is good if the molecules are present within the domain of the chemical space of the training set molecules.
2.7. Software used
Marvin Sketch version 5.5.0.1 (ref. 53) was used to draw chemical structures. Descriptors were calculated by the PADEL-Descriptor software55 and Dragon software version 6.54 Clustering of each data set was done by the “Modified K-Medoid” tool version 1.3 (ref. 58) for its splitting into a training set and a test set. Data Pretreatment version 1.2 was used to remove intercorrelated descriptors. Stepwise regression analysis was done by the MINITAB software version13.14.69 Genetic Algorithm was done by using the Genetic Algorithm tool version 4.1.58 Best subset selection58 and intelligent consensus predictor tool60 were used to generate the QSPR models.
3. Results and discussion
We have developed QSPR models (five MLR models for each of the datasets containing 59 and 69 organic contaminants, and one PLS model for the dataset containing 29 organic contaminants) for three datasets containing diverse organic pollutants with defined adsorption affinities for MWCNTs (for datasets 1 and 2), and the dispersibility index of SWCNTs (for dataset 3), using reduced descriptors pools obtained by different strategies as discussed in the Materials and methods section. We checked the statistical quality of all the individual models using both internal and external validation parameters, which showed that the models are statistically significant (Table 1). We also checked the MAE-based criteria for all the models.59 All the models passed the MAE-based criteria.59 Besides the routinely used validation parameters, we also checked the consensus predictions (for datasets 1 and 2 only) using the developed MLR models employing a newly developed “Intelligent consensus predictor” tool60 to check whether the quality of the predictions of the test set compounds can be enhanced through an “intelligent” selection of multiple MLR models. We found that the consensus predictions of multiple MLR models are better (based on MAE based criteria) than the results obtained from the individual models as shown in Table 1 (here, in both cases, the winner model is CM3). It was also found that the consensus predictions of the test set compounds are better as compared to the individual MLR models based on not only the MAE-based criteria but also the other external validation metrics used in this work as shown in Table 1. All the individual models are mentioned below and the descriptors are discussed elaborately. In the equation, ntraining is the number of compounds used to develop the models and ntest is the number of compounds used for the external prediction of the developed models. The values of leave-one-out (LOO) cross-validated correlation coefficient (Q2) (Q2 in the range of 0.863–0.895 for dataset 1; 0.743–0.798 for data set 2 and 0.775 for dataset 3) above the critical value of 0.5 signify the statistical reliability of the models. The predictability of the models was judged by means of predictive R2 (R2pred) or Q2F1 (Q2F1 range of 0.887–0.919 for dataset 1; 0.783–0.890 for data set 2 and 0.945 for dataset 3) and Q2F2 (Q2F2 range of 0.886–0.919 for dataset 1; 0.768–0.882 for data set 2 and 0.938 for dataset 3), which show the good predictive ability of the models. The statistical results of all the models are summarized in Table 1. The PLS model developed from dataset 3 was also validated using a randomization test through randomly reordering (100 permutations) the dependent variable (log
Cmax) using the SIMCA-P software.68 Here, the intercept values for both R2 and Q2 are below the stipulated values (R2int < 0.4 and Q2int < 0.05), which confirmed that the developed model was not obtained by chance (Fig. S1 in ESI†). We have also checked the intercorrelation among the modeled descriptors for MLR models based on the Pearson correlation coefficient using the SPSS software.70 The results showed that there is no intercorrelation between the modeled descriptors.
Table 1 Statistical quality and validation parameters obtained from the developed MLR and PLS models
Dataset |
Type of model |
Training set statistics |
Test set statistics |
Model R2 |
Model Q2(LOO) |
MAE_train |

|
Δr2m(LOO) |
R
2pred or Q2F1 |
Q
2F2
|
CCC |

|
Δr2m(test) |
MAE (100%) |
MAE (95%) |
MAE |
CM0 = Ordinary consensus predictions. CM1 = Average of predictions from individual models IM1 through IM5. CM2 = Weighted average predictions from individual models IM1 through IM5. CM3 = Best selection of predictions (compound-wise) from individual models IM1 through IM5. *Note that we have run the “Intelligent consensus predictor tool” using the options, AD: No; Dixon Q-test: No; Euclidean distance: No.
|
59 organic contaminants |
Individual models (M1–M5) |
IM1 |
0.920 |
0.895 |
Good |
0.851 |
0.078 |
0.887 |
0.886 |
0.934 |
0.745 |
0.104 |
0.271 |
0.240 |
Good |
IM2 |
0.912 |
0.892 |
Good |
0.848 |
0.079 |
0.916 |
0.915 |
0.952 |
0.817 |
0.072 |
0.221 |
0.197 |
Good |
IM3 |
0.905 |
0.880 |
Good |
0.832 |
0.075 |
0.919 |
0.919 |
0.954 |
0.825 |
0.069 |
0.213 |
0.189 |
Good |
IM4 |
0.893 |
0.872 |
Good |
0.821 |
0.092 |
0.918 |
0.917 |
0.953 |
0.806 |
0.074 |
0.213 |
0.187 |
Good |
IM5 |
0.893 |
0.863 |
Good |
0.808 |
0.086 |
0.915 |
0.914 |
0.950 |
0.798 |
0.076 |
0.222 |
0.199 |
Good |
Consensus models |
CM0 |
— |
— |
— |
— |
— |
0.917 |
0.916 |
0.952 |
0.800 |
0.074 |
0.227 |
0.203 |
Good |
CM1 |
— |
— |
— |
— |
— |
0.917 |
0.916 |
0.952 |
0.800 |
0.074 |
0.227 |
0.203 |
Good |
CM2 |
— |
— |
— |
— |
— |
0.919 |
0.919 |
0.953 |
0.803 |
0.073 |
0.221 |
0.196 |
Good |
CM3
|
—
|
—
|
—
|
—
|
—
|
0.935
|
0.935
|
0.962
|
0.812
|
0.059
|
0.187
|
0.163
|
Good
|
69 organic contaminants |
Individual models (N1–N5) |
IM1 |
0.845 |
0.798 |
Moderate |
0.709 |
0.087 |
0.809 |
0.795 |
0.908 |
0.783 |
0.048 |
0.319 |
0.271 |
Moderate |
IM2 |
0.842 |
0.790 |
Moderate |
0.723 |
0.114 |
0.830 |
0.818 |
0.918 |
0.805 |
0.050 |
0.359 |
0.323 |
Good |
IM3 |
0.842 |
0.788 |
Good |
0.714 |
0.081 |
0.783 |
0.768 |
0.890 |
0.712 |
0.140 |
0.340 |
0.265 |
Good |
IM4 |
0.829 |
0.785 |
Good |
0.709 |
0.087 |
0.812 |
0.799 |
0.903 |
0.748 |
0.044 |
0.330 |
0.286 |
Moderate |
IM5 |
0.793 |
0.743 |
Good |
0.709 |
0.087 |
0.890 |
0.882 |
0.940 |
0.836 |
0.090 |
0.273 |
0.247 |
Good |
Consensus models |
CM0 |
— |
— |
— |
— |
— |
0.862 |
0.852 |
0.929 |
0.818 |
0.002 |
0.284 |
0.245 |
Good |
CM1 |
— |
— |
— |
— |
— |
0.862 |
0.852 |
0.929 |
0.818 |
0.002 |
0.284 |
0.245 |
Good |
CM2 |
— |
— |
— |
— |
— |
0.865 |
0.855 |
0.930 |
0.820 |
0.014 |
0.279 |
0.241 |
Good |
CM3
|
—
|
—
|
—
|
—
|
—
|
0.887
|
0.879
|
0.941
|
0.851
|
0.040
|
0.263
|
0.235
|
Good
|
29 organic contaminants |
|
P1 |
0.830 |
0.775 |
Good |
0.689 |
0.115 |
0.945 |
0.938 |
0.991 |
0.909 |
0.048 |
0.152 |
— |
Good |
From the observations obtained from the modeled descriptors, it has been found that the organic pollutants may interact with the MWCNTs through different mechanisms like hydrogen bonding interactions, hydrophobic interactions, π–π interactions and electrostatic interactions as discussed below.
3.1. Dataset 1
:
59 organic pollutants
The significant descriptors obtained from the five MLR models (see Models M1–M5) for the adsorption properties (log
Kα) of 59 organic chemicals on MWCNTs are X0v, nArOH, B01[C–O], B06[C–Cl], Ui, F03[O–O], F04[N–O], ETA_BetaP, minsCH3, B03[O–O] and nHBint4, which regulate the adsorption properties of the organic pollutants. The contribution of the descriptors can be easily identified from the regression coefficient of the independent variables. In this case, all the descriptors contributed positively (positive regression coefficients), except the B01[C–O] descriptor (negative regression coefficient). The definition, contribution and frequency of the contributed descriptors are shown in Table S4 in the ESI.† We have checked the applicability domain of the developed MLR models using the standardization approach to confirm whether there is any compound present outside the applicability domain or not. It was found that one compound (compound number 41) for model M1 is situated outside the applicability domain, while compound number 56 is situated outside the domain of applicability in case of models M2, M3, M4 and M5; however, these compounds showed good predictivity based on the models. The scatter plot of the observed vs. predicted adsorption coefficient for all the MLR models are shown in Fig. 2.
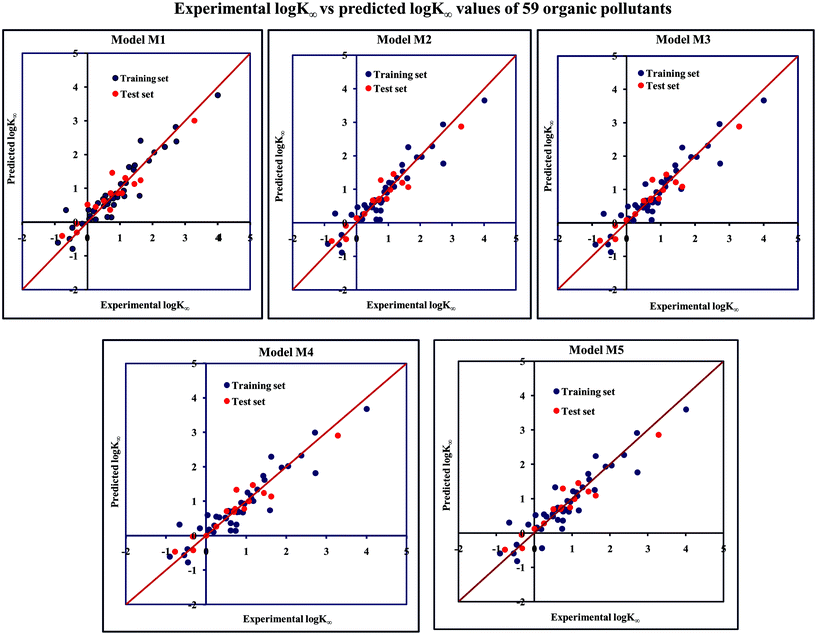 |
| Fig. 2 The scatter plot of the observed and the predicted adsorption coefficient property (log K∞) of the developed MLR models (models M1–M5). | |
Model M1.
log k∞ = −4.62(±0.337) + 0.834(±0.155) × Ui + 0.663(±0.220) × B06[C–Cl] + 0.641(±0.057) × X0v + 0.600(±0.091) × nArOH − 0.611(±0.121) × B01[C–O] |
Model M2.
log k∞ = −8.51(±0.722) + 0.803(±0.048) × X0v + 0.681(±0.146) × F03[O–O] + 0.415(±0.144) × F04[N−O] + 3.27(±0.491) × ETA_BetaP + 0.204(±0.067) × minsCH3 |
Model M3.
log k∞ = −8.68(±0.746) + 0.802(±0.050) × X0v + 0.603(±0.272) × B03[O–O] + 3.39(±0.503) × ETA_BetaP + 0.213(±0.069) × minsCH3 + 0.412(±0.148) × nHBint4 |
Model M4.
log k∞ = −8.72(±0.782) + 0.785(±0.052) × X0v + 0.650(±0.158) × F03[O–O] + 3.51(±0.527) × ETA_BetaP + 0.202(±0.073) × minsCH3 |
Model M5.
log k∞ = −8.42(±0.773) + 0.785(±0.052)X0v + 3.29(±0.526)ETA_BetaP + 0.199(±0.072)minsCH3 + 0.566(±0.137)nHBint4 |
3.1.1. The descriptors related to hydrogen bonding interactions.
The functional group count descriptor, nArOH, represents the number of aromatic hydroxyl groups present in the compound. This descriptor influences the adsorption properties of organic pollutants by MWCNTs as indicated by its positive regression coefficient. Thus, the compounds containing a large number of aromatic hydroxyl groups may enhance the adsorption properties of organic pollutants by MWCNTs as shown in compounds 13 (pyrogallol) (containing 3-OH groups), 5 (2-phenyl phenol) (containing 1-OH group) and 14 (2,4,6 trichlorophenol) (containing 1-OH group). On the other hand, the compounds containing no aromatic hydroxyl groups are detrimental for the adsorption affinity of organic pollutants by MWCNTs as shown in compounds 18 (4-chloroaniline), 36 (benzyl alcohol) and 42 (phenethyl alcohol) (these compounds contain no aromatic hydroxyl groups). Although some compounds containing no aromatic hydroxyl groups still show high adsorption affinity for the organic pollutants by MWCNTs, it is due to some other dominating descriptors present in the model. Thus, the substitution of electron donating groups like hydroxyl groups in the aromatic ring of organic pollutants could enhance the adsorption on MWCNTs.
A 2D atom pair descriptor, F04[N–O], indicates the frequency of the N–O fragment at topological distance 4. The positive regression coefficient of the descriptor suggests that an increase in N–O fragments at topological distance 4 is directly proportional to the adsorption affinity of organic pollutants. The greater number of fragments correlates to higher adsorption properties as observed in the case of compounds 19 (2-nitroaniline) and 27 (3-nitrophenol), while the absence of such fragments at topological distance 4 has no influence on the adsorption by MWCNTs as shown in compounds 18 (4-chloroaniline), 36 (benzyl alcohol) and 42 (phenethylalcohol). This descriptor also indicates that the frequency of two electronegative atoms of organic pollutants (electron donating or electron withdrawing groups) should be situated at topological distance 4 for better adsorption on MWCNTs. In the case of compound number 19, nitrogen (–NH2 group) acts as an electron donor and oxygen (–NO2 group) acts as an electron withdrawing group, whereas in the case of compound number 27, nitrogen (–NO2 group) acts as an electron withdrawing group, and oxygen (–OH group) acts as an electron donating group.
The E-state descriptor, nHBint4 indicates the count of potential internal hydrogen bonds separated by four edges. The positive regression coefficient suggests that hydrogen bonds of organic pollutants have the propensity to play a dominant role in enhancing the adsorption properties. Thus, the organic pollutants bearing hydrogen-bonded groups separated by four path lengths are conducive to adsorption as shown in compounds 13 (pyrogallol), 19 (2-nitroaniline) and 48 (3-chlorophenol), whereas the absence of such fragment in organic pollutants are detrimental to the adsorption affinity as shown in compounds 6 (benzene), 11 (phenol) and 42 (phenethyl alcohol).
B03[O–O] is a 2D atom pair descriptor that indicates the presence or absence of the O–O fragment at topological distance 3. The positive regression coefficient of the descriptor indicates that the higher the frequency of this fragment, the higher is the adsorption affinity. Thus, the presence of the O–O fragment at topological distance 3 favors the adsorption of organic pollutants by MWCNTs as shown in compounds no. 12 (catechol) and 13 (pyrogallol), while compounds no. 6 (benzene), 42 (phenethyl alcohol) and 36 (benzyl alcohol) show low adsorption because these compounds have no such fragments at topological distance 3.
Hydrogen bonding is one of the key mechanisms for the adsorption of organic contaminants on CNTs. The information obtained from the descriptors nArOH, F04[N–O], nHBint4, F03[O–O] and B03[O–O] suggested that there may be some hydrogen bonding interactions between organic pollutants and MWCNTs, which regulate the adsorption affinity (Fig. 3) of organic pollutants toward MWCNTs. In the case of the descriptor nArOH, the aromatic hydroxyl group may form hydrogen bonds with the hydroxy/carboxylic groups on the CNTs surface and the hydrogen bonds may also form between the surface-adsorbed aromatic hydroxyl group-containing organic pollutants (phenolics) and dissolved phenolics. Here, the hydroxyl group is always connected to an aromatic ring. Thus, it is obvious that this aromatic ring of organic pollutants themselves can interact with CNTs by π–π interactions. The descriptor, F04[N–O], also suggested that besides the hydrogen bonding interactions, there may also be a chance to form electrostatic interactions. The electron-withdrawing groups like NO2 may also strengthen the π–π interactions formed between the benzene derivatives (acting as π-acceptor) and CNTs (acting as π-donor). In the case of B03[O–O], two oxygen atoms (hydroxyl groups) are separated by topological distance 3 and can interact with CNTs by hydrogen bonding interactions. These two electronegative atoms of organic pollutants could also interact electrostatically with CNTs and strengthen the π–π interactions formed between the organic pollutants and MWCNTs.39,71 It is worth noting that although the C–O bond is detrimental to the adsorption of organic pollutants on CNTs, the frequency of the O–O fragment at topological distance 3 can suppress the detrimental effect of the C–O group and influence the adsorption affinity of organic pollutants on MWCNTs. The descriptors involved in the hydrogen bonding interactions between the organic pollutants and MWCNTs are depicted in Fig. 3.
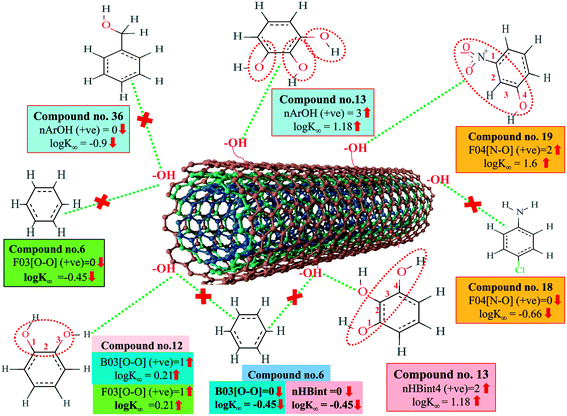 |
| Fig. 3 Mechanistic interpretation of the descriptors related to hydrogen bonding interactions between organic pollutants and MWCNTs (dataset 1). | |
3.1.2. The descriptors related to hydrophobic interactions.
A 2D atom pair descriptor, B06[C–Cl], represents the presence or absence of the C–Cl bond at topological distance 6. The positive regression coefficient of this parameter suggests that the presence of such a fragment at topological distance 6 enhances the adsorption affinity of organic pollutants towards the MWCNTs as shown in compounds 50 (4-chloroacetophenone) and 57 (2-chloronapthlene). On the other hand, compounds like 11 (phenol), 22 (4-methylphenol) and 43 (3-methylbenzyl alcohol) show poor adsorption affinity for the MWCNTs due to the absence of such a fragment.
The descriptor X0v indicates a valence connectivity index of the order 0, which can be calculated through Kier and Hall's connectivity index as shown below. This descriptor contributed positively to the adsorption affinity of organic pollutants for the MWCNTs. Thus, the size of the organic pollutants plays a crucial role in regulating the adsorption affinity of organic pollutants to MWCNTs. It has been found that on increasing the numerical value of this descriptor, the adsorption affinity of organic pollutants for MWCNTs also increases, as shown in the case of compounds 1 (pyrene), 58 (azobenzene) and 5 (2-phenyl phenol) (bigger in size), while the adsorption affinity of organic pollutants for MWCNTs decreases in the case of compounds 6 (benzene), 11 (phenol) and 36 (benzyl alcohol) (smaller in size).
The valence connectivity index of the zeroth order can be calculated by the following:
In the above equation, δvi = the valence vertex degree, Zvi = valence electrons in the ith atom, hi = the number of hydrogen atoms connected to the ith atom, Zi = the number of electrons in the ith atom.
The E-state indices of a particular atom in a certain molecule provide information on its electronic state of that particular atom, which in turn depends on π bonds, the lone pair of electrons and ∂ bonds that inform the quantitative availability of the valence electrons.72 The descriptor minsCH3 indicates the minimum atom type E-state CH3. The positive regression coefficient of this descriptor indicates that the presence of the CH3 group has an important role in influencing the adsorption properties of organic pollutants. The numerical value of this descriptor is directly proportional to the adsorption property, which suggests that with increasing the numerical value of this descriptor, the adsorption affinity of the organic pollutants also increases as evidenced by compounds 10 (2,4-dinitrotoluene), 50 (4-chloroacetophenone) and 52 (1-methylnaphtalene). On the other hand, the adsorption affinity of organic pollutants decreases with the absence of the CH3 group as shown in compounds 6 (benzene), 11 (phenol) and 36 (benzyl alcohol).
Hydrophobic interactions between organic pollutants and CNTs are also an important mechanism for better adsorption. The descriptors, B06[C–Cl], X0v and minsCH3 suggest that the organic pollutants may be adsorbed onto the MWCNTs by hydrophobic interactions. In the case of B06[C–Cl] and X0v, the size of the molecules (for B06[C–Cl], the distance between C and Cl atoms is six, which reflects the size of the molecules) plays an important role in the adsorption affinity. The size enhances the surface area of molecules, which can regulate the hydrophobic interactions between organic pollutants and MWCNTs. The methyl group (information obtained from minsCH3 descriptor) and CNTs are hydrophobic in nature. Thus, an increase in the minsCH3 value would indicate a higher degree of unsaturation and would enhance the reactivity. There is, therefore, a chance for hydrophobic interactions between organic pollutants and MWCNTs, which reflects better adsorption. The descriptors involved in hydrophobic interactions between organic pollutants and CNTs are depicted in Fig. 4.
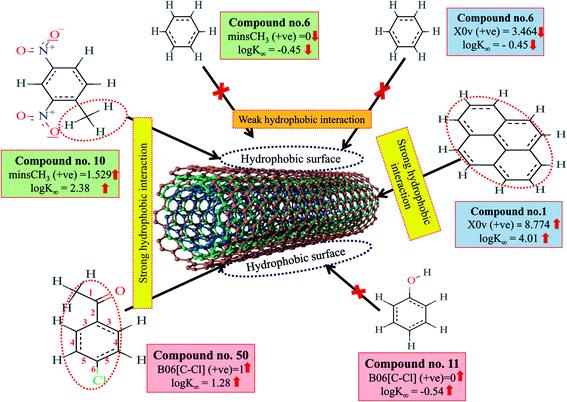 |
| Fig. 4 Mechanistic interpretation of the descriptors related to the hydrophobic interaction between organic pollutants and MWCNTs (dataset 1). | |
3.1.3. The descriptors related to π–π interactions.
The descriptor, Ui, gives information about the unsaturation index, which contributes positively to the adsorption affinity of organic pollutants by MWCNTs as indicated by the positive regression coefficient. From this descriptor, it has been suggested that the presence of unsaturated inorganic pollutants plays a crucial role in enhancing the adsorption affinity. This was demonstrated in compounds 1 (pyrene), 10 (2,4-dinitrotoluene) and 58 (azobenzene) (the numerical values of this descriptor are 3.392, 3 and 3, respectively), and vice versa in the case of compounds 11 (phenol), 36 (benzyl alcohol) and 42 (phenethyl alcohol) (the numerical values of this descriptor are 2 in each compound). Here, the compounds, 1 (pyrene), 10 (2,4-dinitrotoluene) and 58 (azobenzene) have a higher range of unsaturation index values due to the presence of a large number of double bonds.
The ETA index, ETA_BetaP, gives a measure of sigma, pi and non-bonded (i.e., lone pairs capable of forming resonance with the aromatic system) electrons relative to the molecular size. Therefore, electron-richness (unsaturation) relative to the molecular size of organic pollutants is an important parameter for regulating the adsorption properties. The positive regression coefficient of this parameter indicates that the electron densities of the molecules should be higher for increasing the adsorption affinity of organic pollutants for MWCNTs, as found in compounds 1 (pyrene), 28 (1,3-dinitrobenzene) and 58 (azobenzene), whereas the compounds with low electron density show a lower range of adsorption affinities as shown in compounds 36 (benzyl alcohol), 42 (phenethyl alcohol) and 43 (3-methylbenzyl alcohol). Thus, it can be concluded that the molecules should be electron-rich for higher adsorption properties of organic pollutants.
The π–π interaction is another important mechanism involved in the adsorption of organic pollutants to CNTs. The information obtained from Ui and ETA_BetaP descriptors suggested that the organic pollutants can adsorb to MWCNTs by strong π–π interactions. The descriptors B03[O–O], F03[O–O] and F04[N–O] suggested that the [O–O] fragments at topological distance 3 and the [N–O] fragments at the topological distance 4 may strengthen the π–π interactions formed between organic pollutants and MWCNTs. The descriptor Ui suggested that unsaturation plays a crucial role for the adsorption of organic pollutants to MWCNTs. CNTs also contain a large number of double bonds (unsaturation), so there is a chance to form strong π–π interactions between organic pollutants and MWCNTs, which reflects the better adsorption of these pollutants to MWCNTs; hence, a higher number of double bonds of organic pollutants enhance the adsorption affinity to MWCNTs. The descriptor, ETA_BetaP suggested that unsaturation (electron-richness) relative to the molecular size of organic pollutants plays a crucial role in regulating the adsorption properties. From this descriptor, it can be inferred that the adsorption affinity of organic pollutants to MWCNTs is increased due to the π–π interactions. The descriptors involved in π–π interactions between organic pollutants and CNTs are described graphically in Fig. 5.
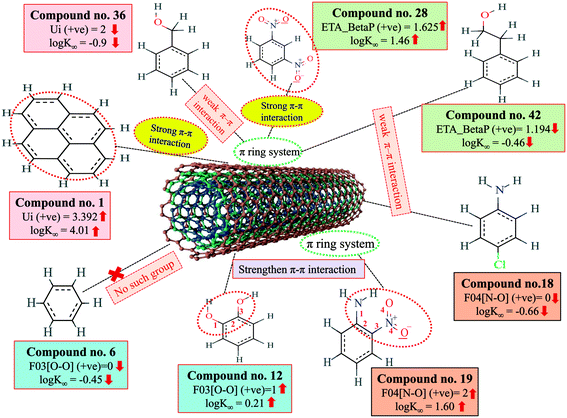 |
| Fig. 5 Mechanistic interpretation of the descriptors related to the π–π interactions between organic pollutants and MWCNTs (dataset 1). | |
3.1.4. The descriptors related to electrostatic interactions.
F03[O–O], a 2D atom pair descriptor, indicates the frequency of the O–O fragment at topological distance 3. The positive regression coefficient of this descriptor suggests that presence of a greater number of O–O bonds at the topological distance 3 might be beneficial for the adsorption affinity of organic pollutants for MWCNTs as shown in compounds 12 (catechol) and 13 (pyrogallol), whereas the opposite happens in the case of compounds 6 (benzene), 42 (phenethyl alcohol) and 43 (3-methylbenzyl alcohol) (where, no O–O fragment is present at topological distance 3). This fragment may also strengthen the π–π interactions formed between organic pollutants and MWCNTs.73,74 Like B03[O–O], this descriptor also suppresses the detrimental effect of the C–O group as discussed earlier in this section.
The information obtained from the descriptors, F03[O–O], B03[O–O] and F04[N–O] suggests that the organic pollutants can adhere to the surface of the MWCNTs by strong electrostatic interactions. The descriptors F03[O–O] and B03[O–O] indicate that the frequency or presence/absence of two electronegative atoms (electron donating group) at the topological distance 3 is essential to enhance the adsorption affinity of organic pollutants to MWCNTs. Thus, there may be a chance to form electrostatic interactions between organic pollutants (negatively charged atom like oxygen atom of the hydroxyl group) and MWCNTs (the sidewall of the CNTs are electrically polarizable and thus polar molecules can easily adhere to their surface). The descriptors involved for electrostatic interactions between organic pollutants and CNTs are represented graphically in Fig. 6.
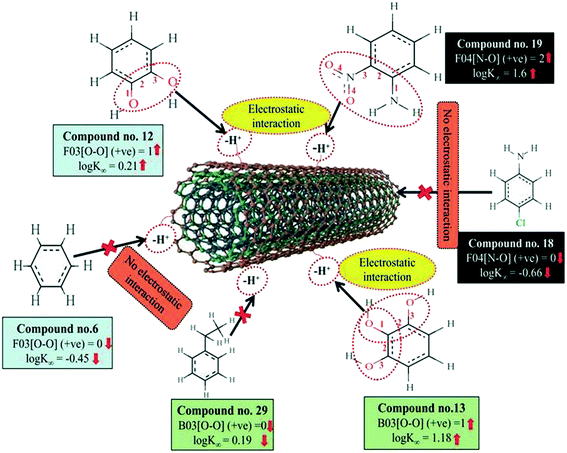 |
| Fig. 6 Mechanistic interpretation of the descriptors related to the electrostatic interactions between organic pollutants and MWCNTs (dataset 1). | |
The 2D atom pair descriptor, B01[C–O], indicates the presence or absence of the C–O bond at topological distance 1. The negative regression coefficient of the descriptor supports that the presence of this fragment at topological distance one is detrimental to the adsorption affinity of organic pollutants by MWCNTs, though it can form hydrogen bonds with MWCNTs. For example, compounds like 1 (pyrene), 57 (2-chloronaphthalene) and 58 (azobenzene) have higher adsorption affinity value due to the absence of such fragments at topological distance 1, whereas compounds like 11 (phenol), 36 (benzyl alcohol) and 42 (phenethyl alcohol) have lower adsorption affinity due to the presence of one C–O bond in each compound.
3.2. Dataset 2
:
69 organic pollutants
The significant descriptors obtained from the five MLR models using the adsorption properties (log
KSA) of 69 organic pollutants related to the specific surface area of MWCNTs are Eta_Epsilon_3, X1A, X2A, nOHp, VAdjMat, F04(O–Cl), B05(O–Cl), MLOGP2, T(N⋯N), O%, and T(O⋯Cl). We have discussed here all the significant descriptors, which are the key properties for altering the adsorption properties of organic pollutants. The definition, contribution and frequency of the modeled descriptors are shown in Table S5 in the ESI.† The applicability domain of the developed models using the standardization approach showed that one test set compound (compound number 10) for model N1, two test set compounds (compound number 10 and 21) for model N2, one test set compound (compound number 21) for model N3 are situated outside the applicability domain, while in the case of model nos. 4 and 5, all the test set compounds are situated within the domain of applicability. The scatter plot of observed vs. predicted adsorption coefficient related to the specific surface area of MWCNTs for all the MLR models are shown in Fig. 7.
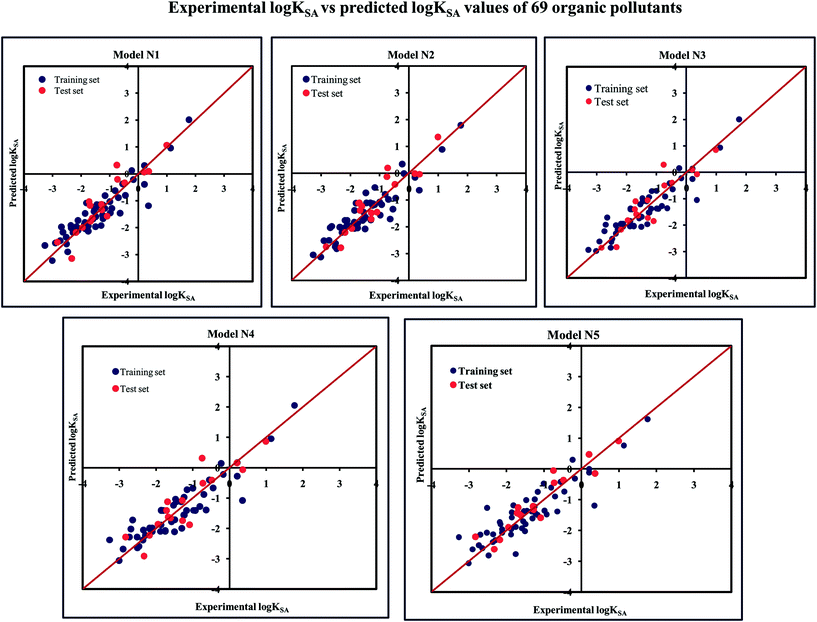 |
| Fig. 7 The scatter plots of the observed and the predicted adsorption coefficient properties related to the specific surface area of MWCNTs (log KSA) of the developed MLR models (models N1–N5). | |
Model N1.
log KSA = 4.29(±2.194) + 0.0965(±0.014) × O% − 16.4(±4.397) × X1A + 0.145(±0.032) × T(N⋯N) − 0.0279(±0.009) × T(O⋯Cl) − 1.01(±0.294) × B05(Cl⋯Cl) + 0.203(±0.022) × MLOGP2 |
Model N2.
log KSA = −7.19(±0.571) + 0.0805(±0.015) × O% − 0.662(±0.323) × nOHp − 0.0358(±0.009) × T(O⋯Cl) − 0.943(±0.294) × B05(Cl⋯Cl) + 0.185(±0.019) × MLOGP2 + 0.958(±0.144) × VAdjMat |
Model N3.
log KSA = −42.3(±7.527) + 0.0973(±0.013) × O% − 0.622(±0.323) × nOHp + 0.154(±0.031) × T(N⋯N) − 0.0407(±0.008) × T(O⋯Cl) + 0.160(±0.20) × MLOGP2 + 89.8(±17.51) × ETA_Epsilon_3 |
Model N4.
log KSA = −42.0(±7.743) + 0.101(±0.014) × O% + 0.159(±0.032) × T(N⋯N) − 0.0411(±0.008) × T(O⋯Cl) + 0.168(±0.021) × MLOGP2 + 88.9(±18.01) × ETA_Epsilon_3 |
Model N5.
log KSA = 2.49(±1.36) + 0.0757(±0.016) × O% − 17.3(±3.773) × X2A + 0.145(±0.036) × T(N⋯N) − 0.721(±0.144) × F04(O⋯Cl) + 0.158(±0.023) × MLOGP2 |
3.2.1. The descriptors related to the hydrophobic interaction.
The descriptor, X1A, indicates an average connectivity index of the order one, it encodes the ‘chi’ value across one bond, which can be calculated on the basis of Kier and Hall's connectivity index and defined as follows:
In this equation, b runs over the 1st order subgraphs having n vertices with B edges; δi and δj are the number of other vertices attached to vertex i and j, respectively. The negative regression coefficient of this descriptor implies that the higher numerical values of this descriptor are not favorable to enhance the adsorption properties of organic pollutants related to the specific surface area of MWCNTs as shown in compounds 3 (benzene), 56 (ethylbenzene) and 57 (benzyl alcohol) (the corresponding numerical values of these compounds are 0.5, 0.491, 0.491, respectively, showing a lower range of adsorption affinity). On the other hand, compounds like 35 (tetracycline), 22 (pyrene) and 26 (phenanthrene) show better adsorption affinity (log
KSA) due to their lower numerical values of this descriptor.
Another significant descriptor, X2A, indicates an average connectivity index of the order 2, and encodes the ‘chi’ value across two bonds, which can be calculated on the basis of Kier and Hall's connectivity index, defined in the following equation:
Here, b runs over the 2nd order subgraphs having n vertices with B edges, δi and δj are the numbers of other vertices attached to vertex i and j, respectively. This descriptor also has a negative contribution towards the adsorption profile (log
KSA) of organic pollutants by MWCNTs as evidenced by the negative regression coefficient. This indicates that the adsorption properties of organic pollutants decrease with an increase in the numerical value of this descriptor as shown in compounds 3 (benzene), 18 (aniline) and 40 (bromobenzene), and vice versa in the case of compounds 22 (pyrene), 26 (phenanthrene) and 35 (tetracycline).
The VAdjMat descriptor represents the vertex adjacency information and gives information about molecular dimension and hydrophobicity. This descriptor can be calculated by using the following formula:
Here, m depicts the number of heavy–heavy bonds. This descriptor contributed positively towards the adsorption properties (log
KSA) of organic pollutants as indicated by the positive regression coefficient. Thus, the higher numerical value of this descriptor is influential toward the adsorption affinity of organic pollutants. This indicates that hydrophobicity plays a crucial role in altering the adsorption properties of organic pollutants by MWCNTs. For example, compounds 22 (pyrene), 26 (phenanthrene) and 35 (tetracycline) show a higher range of adsorption properties as these compounds contain higher numerical values of this descriptor. Compounds 3 (benzene), 55 (iodobenzene) and 46 (chlorobenzene) show a lower range of adsorption properties as these compounds contain higher numerical values of this descriptor. It is therefore suggested that the hydrophobic organic pollutants can easily be adsorbed by MWCNTs through hydrophobic interactions between the pollutants and CNTs.
The next descriptor, MLOGP2, represents the squared Moriguchi octanol–water partition coefficient, calculated from the regression equation of the Moriguchi log
P model75,76 consisting of 13 parameters as depicted in the following equation.
log P = −1.244(CX)0.6 − 1.017(NO)0.9 + 0.406PRX − 0.145(UB)0.8 + 0.511HB + 0.268POL − 2.215AMP + 0.912ALK − 0.392RNG − 3.684QN + 0.474NO2 + 1.582NCS + 0.773BLM − 1.041 |
‘CX’ depicts the summation of the weighted number of carbon atoms; ‘NO’ depicts the total number of N and O atoms; ‘PRX’ represents the proximity effect of N/O; ‘UB’ represents the number of unsaturated bonds including semi-polar bonds; ‘POL’ depicts the number of aromatic polar substituents; ‘AMP’ depicts the amphoteric property; ‘ALK’ represents the dummy variable for alkanes and alkenes; ‘RNG’ depicts the indicator variable for the presence of a ring structure, except for benzene and its condensed ring; ‘QN’ represents quaternary nitrogen; ‘NO2’ represents the number of nitro groups; ‘HB’ represents a dummy variable for the presence of intermolecular hydrogen bonds; ‘NCS’ depicts isothiocyanato or thiocyanato; ‘BLM’ represents a dummy variable for the presence of β-lactam.
The positive regression coefficient of this descriptor indicates that hydrophobicity plays a crucial role in regulating the adsorption properties of organic pollutants. The highly hydrophobic organic pollutants can easily be adsorbed by MWCNTs as evidenced by compounds 22 (pyrene), 26 (phenanthrene) and 34 (azobenzene) as their corresponding MLOG2 values are 22.653, 18.762 and 10.539, respectively, whereas hydrophilic molecules are poorly adsorbed by MWCNTs as evidenced by compounds 18 (aniline), 57 (benzylalcohol) and 63 (3-nitroaniline) as their corresponding MLOGP2 values are 2.268, 2.532 and 1.816 respectively. Therefore, it can be inferred that the organic pollutants are adsorbed onto the CNTs through hydrophobic interactions. Thus, for proper adsorption, organic pollutants should be hydrophobic in nature. Note that this was also observed in the case of the VAdjMat descriptor as discussed previously. MLOGP2 is not strictly a 2D descriptor. Here, the term ‘intramolecular H-bonds’ is used to calculate the MLOGP value, which is conformation dependent.
The information obtained from the descriptors X1A, X2A, VAdjMat and MLOGP2 suggested that the adsorption of organic pollutants related to the specific surface area of MWCNTs may occur through hydrophobic interactions. The molecular connectivity index (X1A and X2A) has a direct relationship with the count of interacting C–H bonds present in a molecule. The number of C–H bonds in a molecule is equal to the number of H atoms. As the C–H bond increases, the hydrophobicity of the molecule increases. The δ value (depends on the number of H atoms, the definition of a δ value for a carbon atom in a molecular graph is: δ = 4 − H) decreases with the average connectivity index. Thus, the hydrophobic interactions between the organic contaminants and MWCNTs are reduced and the adsorption of organic pollutants related to the specific surface area of MWCNTs may also be reduced.77
The descriptors VAdjMat and MLOGP2 give information about the hydrophobicity of molecules. It is obvious that the hydrophobic organic pollutants will interact with hydrophobic CNTs through hydrophobic interactions. This implies that the hydrophobic organic pollutants can be easily adsorbed by MWCNTs through hydrophobic interactions. The descriptors involved for hydrophobic interaction are graphically depicted in Fig. 8.
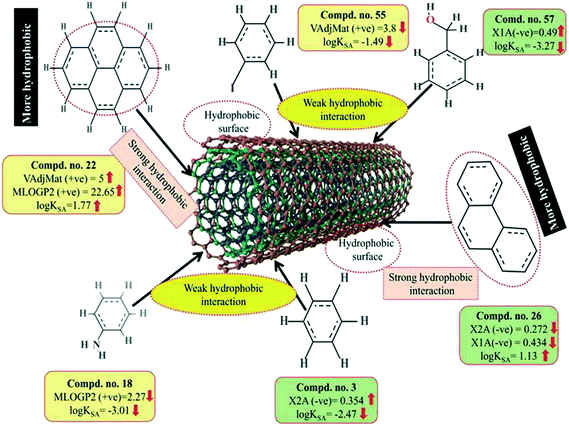 |
| Fig. 8 Mechanistic interpretation of the descriptors related to the hydrophobic interactions between organic pollutants and MWCNTs (dataset 2). | |
3.2.2. The descriptors related to the π–π interactions.
A functional group count descriptor, nOHp, describes the number of primary alcohols. The negative regression coefficient of this descriptor points out that the primary alcoholic group is not favored to enhance the adsorption properties (log
KSA) of organic pollutants as found in compounds 13 (3-methyl benzyl alcohol) and 57 (benzyl alcohol). On the contrary, organic pollutants that do not contain any primary alcoholic groups have higher adsorption affinities (log
KSA) as shown in compounds 22 (pyrene), 26 (phenanthrene) and 34 (azobenzene). Thus, the organic pollutants that do not contain any primary alcoholic groups may be highly adsorbed by MWCNTs.
F04[O–Cl] is a 2D atom pair descriptor that indicates the number of (O–Cl) fragments at a topological distance of 4. The negative regression coefficient of this descriptor indicates that the frequency of the O–Cl fragment at the topological distance 4 is inversely proportional to the adsorption properties of organic pollutants. A higher number for this fragment correlates to lower adsorption properties of organic pollutants, as observed in compounds 7 (dicamba), 61 (3-chlorophenol) and 66 (2,4,5-trichlorophenoxyacetic acid) (these compounds contain 3, 1 and 1 such fragments, respectively, at a topological distance of 4), while a lower numerical value of this descriptor correlates to a higher adsorption property of organic pollutants as observed in compounds 22 (pyrene), 26 (phenanthrene), 34 (azobenzene) and 69 (2,4-dinitrotoluene) (these compounds contain no such fragments at topological distance 4). Thus, the presence of this fragment at the topological distance 4 may hinder the adsorption of the organic pollutants by MWCNTs. The adsorption of organic contaminants to the CNTs decreases when the frequency of the (O–Cl) fragment at topological distance 4 increases. Compound 2 (2,4,6-trichlorophenol) also contains a O–Cl fragment but not at topological distance 4. Therefore, the adsorption affinity related to the specific surface area of the MWCNTs value of compound 2 is (log
KSA value = −0.81) not low as compared to compounds 7 (dicamba), 61 (3-chlorophenol) and 66 (2,4,5-trichlorophenoxyacetic acid) (these compounds contain 3, 1 and 1 such fragments, respectively, at topological distance 4 and the log
KSA values are −2.64, −1.75 and −2.51, respectively).
T(O⋯Cl), a 2D atom pair descriptor, indicates the sum of the topological distance between oxygen and chlorine. The negative regression coefficient of this descriptor suggests that a higher numerical value of this descriptor is detrimental to enhancing the adsorption properties of organic pollutants related to the specific surface area of MWCNTs as shown in compounds 2 (2,4,6-trichlorophenol), 7 (dicamba) and 66 (2,4,6-trichlorophenoxyacetic acid). On the other hand, the organic pollutants containing no such fragments have higher adsorption properties as shown in compounds 22 (pyrene), 26 (phenanthrene) and 34 (azobenzene). From this observation, it can be inferred that the organic pollutants without (O⋯Cl) fragments may be better adsorbed onto the MWCNTs surface.
A 2D atom pair descriptor, B05(Cl–Cl), describes the presence or absence of Cl–Cl fragments at topological distance 5. The negative regression coefficient of this descriptor indicates that the presence of the Cl–Cl fragment at the topological distance 5 may reduce the adsorption property of organic pollutants related to the specific surface area of MWCNTs (log
KSA). A higher number of this fragment correlates to lower adsorption property of organic pollutants as observed in compounds 7 (dicamba), 41 (1,2,4-trichlorobenzene) and 66 (2,4,5-trichlorophenoxyacetic acid) (containing one such fragment each) while absence of this fragment in organic pollutants correlates to higher adsorption property as evidenced from compounds 22 (pyrene), 26 (phenanthrene) and 34 (azobenzene). From this descriptor, it can be suggested that the presence of this fragment at topological distance 5 may retard adsorption of the organic pollutants by MWCNTs.
Another 2D atom pair descriptor, T(N⋯N), indicates the sum of the topological distances between two nitrogen atoms. A positive contribution towards the adsorption properties of organic pollutants related to the specific surface area of MWCNTs (log
KSA) indicates that for better adsorption of organic pollutants by MWCNTs, the topological distance between two nitrogen atoms should be greater, as shown in compounds 4 (oxytetracycline), 35 (tetracycline) and 69 (2,4-dinitrotoluene) (as their corresponding topological distances between two nitrogen atoms are 5, 5 and 4, respectively), and vice versa in the case of compounds 42 (isophorone), 43 (4-fluorophenol) and 44 (acetophenone). Thus, it can be inferred that the topological distances between two nitrogen atoms should be greater for the better adsorption of organic pollutants by MWCNTs.
As discussed earlier in the introduction section, π–π interactions are one of the key mechanisms for the adsorption of organic pollutants to CNTs. The information obtained from these descriptors, nOHp, F04[O–Cl], B05[Cl–Cl], T(N⋯N) and T(O⋯Cl), strongly support this statement. The descriptor nOHp weakens the π–π interaction that occurs between the organic pollutants and CNTs. In this case, the hydroxyl group is alcoholic in nature (aliphatic hydroxyl group) and cannot donate the lone pair of electrons to the aromatic ring (not directly bonded to the aromatic carbon) and ultimately weaken the π–π interactions of the aromatic ring, though it can form hydrogen bonds with the surface modified CNTs. On the other hand, the phenolic hydroxyl group can donate the lone pair of electrons to the aromatic ring (bonded directly to the aromatic carbon atom) as discussed previously (section 3.1), thus strengthening the π–π interactions between organic pollutants and CNTs. In the case of the phenolic hydroxyl group, it can also act as a π donor, but this is not possible in case of the alcoholic hydroxyl group. From this observation, it can be suggested that the aliphatic hydroxyl (alcoholic) group is not favorable for the adsorption affinity of organic pollutants to the CNTs. In case of the descriptors B05[Cl–Cl], T(O⋯Cl) and F04[O–Cl], the chlorine atom has an electron inductive effect and decreases the electron density in the benzene ring, which compensates for the electron-donating effect of the oxygen atom (in the case of compounds 7 and 66), even after –OH dissociated into –O−. The withdrawing inductive character of chlorine substituents decreases the electron density of the p-chlorophenol ring as compared with that of the phenol ring. Thus, when the O–Cl or Cl–Cl fragment is present in an aromatic molecule, it decreases the electron density of that aromatic ring (as compared with that of the –OH substituted benzene ring (phenolic) or the benzene ring itself) and ultimately, electron donor–acceptor interactions do not occur easily between CNTs and organic contaminants. Hence, the compound could not be easily adsorbed to the MWCNTs. In case of the descriptor T(N⋯N), the lone pair of electrons of the nitrogen atom can be donated to the ring system (when directly attached) and enhance the π–π interaction with the CNTs. The nitrogen can be present as the amino form (electron donating) or in the nitro form (electron withdrawing). Both forms strengthen the π–π interactions between the organic pollutants and CNTs by increasing or decreasing the π-electron density of the aromatic ring system and act as π electron donor or acceptor, respectively. If the nitrogen is not directly attached to the aromatic ring system, then adsorption happens through electrostatic interactions between the nitrogen of the pollutants and the hydrogen of CNTs by forming dipoles when they are close to each other; the position of the nitrogen atom hardly matters here. The descriptors influencing the π–π interaction are graphically represented in Fig. 9.
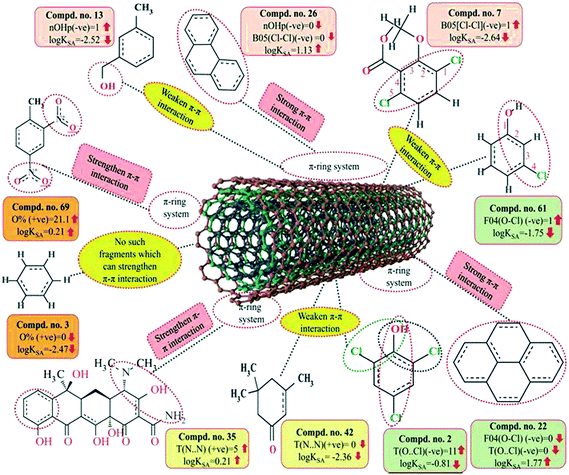 |
| Fig. 9 Mechanistic interpretation of the descriptors related to π–π interactions between organic pollutants and MWCNTs (dataset 2). | |
3.2.3. The descriptors related to hydrogen bonding interactions.
The descriptor, O%, indicates the percentage of oxygen atoms present in a particular molecule. The positive regression coefficient of this descriptor suggests that the presence of oxygen atom is highly influential in the adsorption of the organic pollutants on the surface of MWCNTs. For example, compounds 4 (oxytetracycline), 35 (tetracycline) and 69 (2,4-dinitrotoluene) show better adsorption affinity as their corresponding percentages of oxygen atoms are 15.8, 14.3 and 21.1, respectively. In contrast, compounds 3 (benzene), 18 (aniline) and 24 (4-chloroaniline) show poor adsorption affinity as these compounds do not contain any oxygen atoms. The oxygen atom may be present in different organic pollutants in keto, phenolic (favorable for adsorption) or alcoholic forms (not favorable for adsorption as discussed previously). These different types of oxygen may interact with CNTs in different ways, e.g., hydrogen bonding, strengthening the π–π interactions and electrostatic interactions. On the other hand, a high percentage of oxygen atoms may enhance the polarity of the pollutants. Since the sidewalls of the CNTs are also electrically polarized, the polar group of organic pollutants can easily adhere to the surface of the CNTs. The descriptor involved for hydrogen bonding interactions is given in Fig. 10.
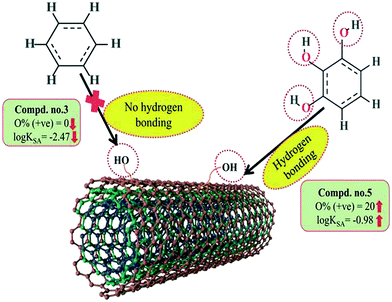 |
| Fig. 10 Mechanistic interpretation of the descriptors related to hydrogen bonding interactions between organic pollutants and MWCNTs (dataset 2). | |
3.2.4. The descriptors related to the electrostatic interactions.
The descriptor, Eta_Epsilon_3, indicates the summation of epsilon values relative to the total number of atoms including hydrogen in the connected molecular graph of the reference alkane, which can be calculated by the following equation.
ε denotes electronegativity, NR denotes the number of atoms present in the reference alkane. This descriptor has a positive contribution towards the adsorption properties of organic pollutants related to the specific surface area of MWCNTs. This indicates that the electron-rich organic pollutants will be highly adsorbed by MWCNTs. Thus, the higher numerical value (due to strong electrostatic interactions between organic pollutants and CNTs) of this descriptor is required to increase the adsorption properties of organic pollutants by MWCNTs as shown in compounds 22 (pyrene), 26 (phenanthrene) and 35 (tetracycline) and vice versa in the case of compounds 7 (dicamba), 13 (3-methylbenzyl alcohol) and 18 (aniline) (due to weak electrostatic interactions between these organic pollutants and CNTs).
The information obtained from the descriptor O% suggests that the organic pollutants can adhere to the surface of MWCNTs by electrostatic interactions. There may be a chance to form electrostatic interactions between organic pollutants (negatively charged atoms like the oxygen atom of the hydroxyl group) and MWCNTs (sidewalls of the CNTs are electrically polarizable, thus polar molecules can easily adhere to their surface). The descriptors involved in electrostatic interactions are shown graphically in Fig. 11.
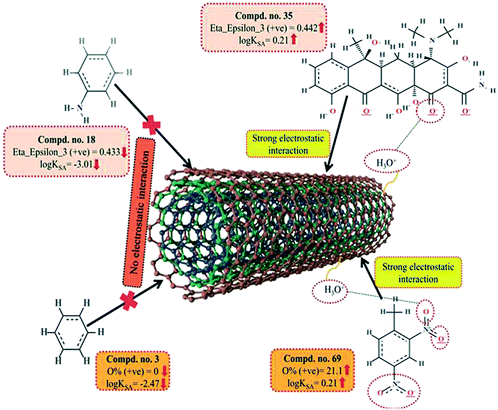 |
| Fig. 11 Mechanistic interpretation of the descriptors related to the electrostatic interactions between organic pollutants and MWCNTs (dataset 2). | |
3.3. Dataset 3
:
29 organic solvents
The significant descriptors obtained from the PLS model using the dispersibility index (log
Cmax) values of 29 organic solvents to SWCNTs are minsssN, SpMin3_Bhe, VPC-6 and SpMin6_Bhi (arranged according to the variable importance plot, Fig. S2 in ESI†). The modeled descriptors, which are the key properties altering the dispersibility indexes of organic solvents, are discussed below. We have also checked the applicability domain of test set compounds using the DModX approach (99% confidence level) to find out whether any test set compounds lie outside of the AD (D-critical = 4.559). The results suggested that the entire test set compounds lie within the AD, except for compound number 29 (Fig. S3 in ESI†). The scatter plot of the observed vs. predicted dispersibility index of SWCNTs in different solvents are presented in Fig. 12.
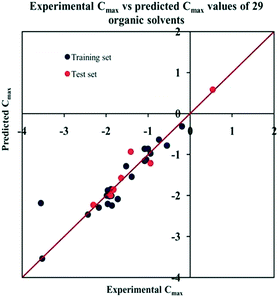 |
| Fig. 12 The scatter plot of the observed and the predicted dispersibility index of SWCNTs (log Cmax) of the developed PLS model (model P1). | |
Model P1.
log Cmax = −1.379 + 1.379 × VPC-6 − 0.949 × SpMin3_Bhe + 0.659 × minsssN − 0.375 × SpMin6_Bhi |
The most significant descriptor, minsssN, indicates the minimum atom type E-state >N-. The E-state variable encodes the intrinsic electronic state of each atom present in the molecular graph. The intrinsic electronic state of the atom is changed by the electronic influence of all other atoms in the molecule within the context of the topological character of the molecule. Atoms that posses π and lone pairs of electrons or are terminal atoms possess higher positive values for the E-state index. Atoms that do not have π and lone pairs of electrons and are present at the interior part of a molecule possess lower E-state values. An increase in the minsssN value would indicate the higher electronegativity of the organic solvents, which is beneficial for the dispersibility of SWNTs. The positive regression coefficient of this descriptor indicates that nitrogen atoms connected to other heavy atoms play an important role in influencing the dispersibility of SWNTs in different organic solvents. The numerical values of this descriptor are directly proportional to the dispersibility of SWCNTs, suggesting that the dispersibility index of the SWNTs will increase with increasing the number of such fragments as evidenced by the compounds 1 (1,3-dimethyltetrahydro-2(1H)-pyrimidinone), 2 (1-butylpyrrolidin-2-one) and 5 (3-(2-oxo-1-pyrrolidinyl)propanenitrile). On the other hand, the absence of such fragments in different organic solvents decreases the dispersibility index of SWCNTs as shown in compounds 24 (cyclohexanone), 27 (formamide) and 28 (benzyl alcohol). Thus, from this descriptor, it can be suggested that the dispersibility of CNTs may be enhanced through electrostatic interactions.
The second highest significant descriptor, SpMin3_Bhe, is defined as the smallest absolute eigenvalue of Burden modified matrix-n3/weighted by the relative Sanderson electronegativities.78 The negative contribution shown by SpMin3_Bhe indicates that the dispersibility index of SWCNTs in various solvents can be increased by decreasing the numerical value of SpMin3_Bhe as shown in compounds 9 (dimethyl-imidazolidinone), 10 (dimethyl acetamide) and 16 (acrylic acid). On the other hand, the dispersibility of SWCNTs can be decreased by increasing the numerical value of SpMin3_Bhe as shown in compounds 22 (benzyl benzoate) and 26 (triethyleneglycol). The SpMin3_Bhe descriptor weighted by the relative Sanderson electronegativity suggests that the electronegativity of the solvents and polar interactions with CNTs play an important role in the dispersibility of the SWCNTs. It can be concluded that polar interactions can have an optimum value. Thus, polar solvents with low donor number are preferred for the dispersibility of the CNTs or it would be better to state that solvents with medium polarity are satisfactory.
The third highest significant descriptor, VPC-6, is a type of topological descriptor, which indicates the chi valance path cluster of order 6. This descriptor differentiates the molecules according to their size, degree of branching, flexibility and overall shape. Chi cluster descriptor (VPC-6) is an indicator of the nth degree of branching and thus implicates the effect of substitution in a molecule. The organic solvent molecules that are relatively compact have higher values of this descriptor,79 suggesting that a small sized molecule with compactness is most probably a better solvent for SWCNTs. It has a positive contribution toward the dispersibility index of SWCNTs in different organic solvents. This indicates that the degree of branching of organic solvents increases the dispersibility index of SWCNTs as shown in compounds 1 (1,3-dimethyltetrahydro-2(1H)-pyrimidinone), 3 (1-benzylpyrrolidin-2-one), and 9 (dimethyl-imidazolidinone), and vice versa in case of compounds 10 (dimethyl acetamide), 16 (acrylic acid) and 17 (2,2′-thiodiethanol).
The least significant descriptor, SpMin6_Bhi indicates the smallest absolute eigenvalue of Burden modified matrix – n6/weighted by the relative first ionization potential.
A modified Burden matrix Q is defined as follows:
[Q]ij = Zi + 0.1δi + 0.01 × nπi and [Q]ij = 0.4/dij |
where,
Zi depicts the atomic number of the
ith atom,
di depicts the number of non-hydrogen neighbors of the
ith atom (
i.e., the vertex degree),
nπi depicts the number of π electrons, and
dij depicts the topological distance between the
ith and
jth atoms.
78 A larger ionization potential of a molecule suggests that higher energy is required to convert the molecule into cationic form, whereas a smaller ionization potential can easily convert the molecule into cationic form, which helps in the easy interaction of the cationic form of the molecule to the π-system of the carbon nanotube through π-cationic interactions. This descriptor is inversely proportional to the dispersibility of SWNTs, suggesting that with increasing the ionization potential, the dispersibility index of the SWNTs decreases as evidenced by compounds
27 (formamide),
16 (acrylic acid), and
9 (dimethyl-imidazolidinone). On the other hand, the dispersibility index of organic solvents increases in the case of compounds
2 (1-butylpyrrolidin-2-one) and
5 [3-(2-oxo-1-pyrrolidinyl)propanenitrile]. The effects of the contributed descriptors on the dispersibility of SWCNTs in diverse organic solvents are summarized graphically in
Fig. 13.
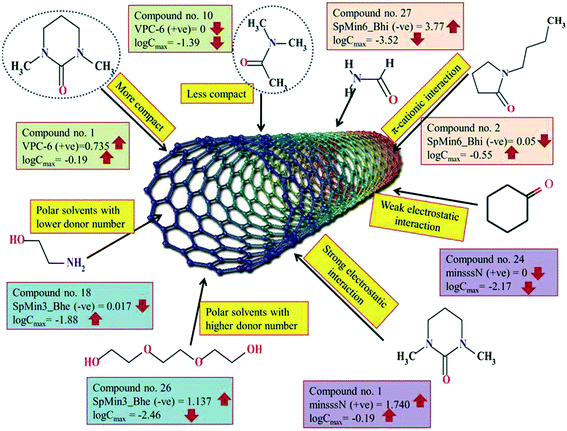 |
| Fig. 13 The effects of the contributed descriptors on the dispersibility of SWCNTs in diverse organic solvents. | |
4. Overview and conclusions
MLR and PLS regression-based strategies were employed to develop QSPR models of organic pollutants (datasets 1 & 2) and organic solvents (dataset 3). Multiple endpoints related to CNTs (adsorption coefficient, adsorption coefficient related to specific surface area of MWCNTs and dispersibility index) were used to explore the key structural features that influence the adsorption and dispersibility of the investigated molecules towards MWCNTs and SWCNTs, respectively. The models were developed using 2D descriptors only. Prior to the development of the final models, different strategies for variable selection were performed to extract the most significant descriptors for the generation of the final MLR (5 models for both datasets 1 and 2) and PLS (a single model for dataset 3) models. Extensive validation of the developed models was performed, which showed good predictibility and robustness. The QSPR models were developed in compliance with the OCED principles. We also used the “Intelligent consensus predictor” tool to explore whether the quality of the predictions of test set compounds could be enhanced through an “intelligent” selection of multiple MLR models (in the case of datasets 1 and 2). The results showed that based on the MAE-based criteria, the consensus predictions of multiple MLR models are better than the results obtained from the individual models. In both cases, the winning model was CM3. The insights obtained from the developed MLR models for datasets 1 and 2 are as follows: (i) the descriptors like Ui, F03[O–O], F04[N–O], ETA_BetaP, nOHp, O%, T(N⋯N), T(O⋯Cl) and F04[O–Cl] influence the adsorption of organic pollutants either by π–π interactions or by strengthening π–π interactions. (ii) nArOH, F03[O–O], B03[O–O], nHBint, F04[N–O], Eta_Epsilon_3 and O% descriptors favor the adsorption of organic pollutants through electrostatic interactions. (iii) The organic pollutants adsorbed through hydrogen bonding interactions are indicated by nArOH, F03[O–O], B03[O–O], nHBint, F04[N–O] and O%. (iv) The descriptors minsCH3, B06[C–Cl], X0v, VAdjMat, MLOGP2, X2A and X1A are essential for the adsorption of organic pollutants through hydrophobic interactions. These observations were further supported by the following discussion: the organic adsorbates of CNTs were mostly aromatic compounds, confirming that aromatic compounds have a better interaction with CNTs than the non-aromatic pollutants, due to their π electron richness and flat conformation. The systematic understanding of aromatic contaminants is therefore critical since aromaticity plays an important role in adsorption. Several studies have suggested that π–π interactions are crucial for the adsorption of organic compounds to CNTs,71,80,81 which in turn depends on the size and shape of the molecules, due to the curvature of the CNTs and its substituents. The π-system of the organic pollutants interacts with the π-system of the CNTs through π–π interactions and the interactions increase with the number of aromatic rings in the adsorbates.39,82 Both electron withdrawing groups (e.g. –NO2 and –Cl) and electron donating groups (e.g. –NH2, –OH) strengthen the π–π interactions between the pollutants and MWCNTs73,74 by acting as π-electron acceptors and π-electron donors, respectively. The hydroxyl group was investigated as an electron donating substituent on adsorptive interactions among pollutants and MWCNTs, since the hydroxyls, by dissociating to –O− (which has stronger electron donating ability), strengthen the n–π electron donor–acceptor (EDA) mechanism. Compounds with no aromatic ring (no π electrons) interact through hydrophobic forces. A study also suggested that CNTs act as strong adsorbents for hydrophobic compounds due to hydrophobic interactions.15,16,33,83–85 Hydroxyl groups (phenolic form) can interact through various means, such as (i) hydrophobic interactions (ii) electrostatic interactions (both attraction and repulsion) (iii) hydrogen bonding interactions and (iv) enhancing π–π interactions. As the number of hydroxyl groups (phenolics) in the pollutants increases, the hydrophobicity decreases. Thus, it can be considered as a major factor in the adsorption of phenolics to CNTs. Hydrogen bonding can also be a major interaction between hydroxyl-containing pollutants and substituted carbon nanotubes.86,87 Hydroxyl and amino group interactions can be related to the electronic features. In one experiment, it was observed that 1-naphthylamine has better adsorption to treated CNTs than the untreated CNTs, and there was an additional observation that although both 2,4-dichlorophenol and 2-naphthol contain an –OH group, the adsorption of 2-naphthol was more significant with variation in the functionality of CNTs.88 This indicates that when the adsorbates possess electronic properties, the functionality of nanotubes helps with the improvement of adsorption.88 Chen et al.89 reported that nitro group containing pollutants show stronger adsorption than non-polar aromatics. This indicates that along with hydrophobic interactions, there is some other essential interaction that controls the adsorption, which is comparable to the π-electron polarizability that is related to aromatic compounds and electron donating as well as accepting properties, similar to compounds having more than two nitro groups. Nitroaromatic compounds, besides being polar in nature, have electron accepting capacity when interacting with adsorbents having high electron polarizability properties and also have high electron conjugation with the π-electrons of CNTs. Thus, the higher affinity of nitro aromatic compounds as compared to other pollutants is due to π–π electron donor–acceptor interactions; since nitrogen is a strong electron-withdrawing atom, it acts as a π-acceptor and carbon nanotubes act as the π-donor.90–93 Hydrogen bonding is also possible between nitro groups of the pollutants, which act as H-acceptors and functional group-substituted carbon nanotubes. The presence of two chlorine atoms causes the electron inductive effect, which may cause a reduction in the electron density of the aromatic ring attached to it, as suggested by Sulaymon and Ahmed et al.;94 the electron donating effect of the hydroxyl atom attached to the aromatic ring compensates for this by dissociating into the stronger electron donor like –O− (oxygen). We can, therefore, conclude that the adsorption of the organic pollutants to the CNTs can be enhanced by the following: a greater number of aromatic rings, high unsaturation or electron richness of the molecule, the presence of polar groups substituted on the aromatic ring, the presence of two oxygen atoms at a topological distance of 3, the presence of nitrogen and oxygen atoms at the topological distance of 4, the size of the molecules, and the hydrophobic surface of the molecules. On the other hand, the presence of carbon and oxygen atoms at a topological distance of 1, aliphatic primary alcohols, the presence of two chlorine atoms at topological distance 5 and the presence of oxygen and chlorine atoms at topological distance 4 may be detrimental and can retard the adsorption of organic pollutants. From the insights obtained from the PLS model for dataset 3, we have interpreted that the organic solvents bearing the >N- fragment, polar solvents with low donor number, compact molecules and lower ionization potential may be better solvents to enhance the dispersibility of SWCNTs. Dispersibility is directly correlated to the adsorption properties of molecules to CNTs. This PLS model and contributed descriptors can help with the understanding of the mechanism of the dispersion process and predict organic solvents that improve the dispersibility of SWCNTs and may overcome the drawbacks of SWCNTs. This work may, therefore, be helpful in the removal of the harmful and toxic contaminants/disposal of the by-products from the various industries, making it possible to achieve a pollution-free environment.
Conflicts of interest
There are no conflicts to declare.
Acknowledgements
Financial assistance from the AICTE, New Delhi in the form of a fellowship to JR and SG is thankfully acknowledged. PKO thanks the UGC, New Delhi for financial assistance in the form of a fellowship (Letter number and date: F./PDFSS-2015-17-WES-11996; dated: 06/04/2016). KR thanks CSIR, New Delhi for financial assistance under a Major Research project (CSIR Project No. 01(2895)/17/EMR-II).
References
- U. K. Garg, M. P. Kaur, V. K. Garg and D. Sud, Removal of hexavalent Cr from aqueous 19 solutions by agricultural waste biomass, J. Hazard. Mater., 2007, 140, 60–68 CrossRef CAS PubMed.
-
J. M. Randall, E. Hautala and A. C. Waiss Jr, Removal and recycling of heavy metal ions from mining and industrial waste streams with agricultural by-products, in Proceedings of the fourth mineral waste utilization symposium, Chicago, 1974 Search PubMed.
- D. J. Ferner, Toxicity, heavy metals, Med. J., 2001, 2(5), 1 Search PubMed.
- Y. Lu, S. Song, R. Wang, Z. Liu, J. Meng, A. J. Sweetman, A. Jenkins, R. C. Ferrier, H. Li, W. Luo and T. Wang, Impacts of soil and water pollution on food safety and health risks in China, Environ. Int., 2015, 77, 5–15 CrossRef CAS PubMed.
-
L. B. Franklin, Wastewater engineering: treatment, disposal and reuse, McGraw Hill, New York, 1991 Search PubMed.
-
R. L. Droste, Theory and practice of water and wastewater treatment, Wiley, New York, 1997 Search PubMed.
- R. N. Goyal, V. K. Gupta, A. Sangal and N. Bachheti, Voltammetric determination of uric acid at a fullerene-C60-modified glassy carbon electrode, Electroanalysis, 2005, 17(24), 2217–2223 CrossRef CAS.
- R. N. Goyal, V. K. Gupta and N. Bachheti, Voltammetric determination of adenosine and guanosine using fullerene-C60-modified glassy carbon electrode, Talanta, 2007, 71(3), 1110–1117 CrossRef CAS PubMed.
- R. N. Goyal, V. K. Gupta and N. Bachheti, Fullerene-C60-modified electrode as a sensitive voltammetric sensor for detection of nandrolone, Anal. Chim. Acta, 2007, 597, 82–89 CrossRef CAS PubMed.
- R. N. Goyal, V. K. Gupta, N. Bachheti and R. A. Sharma, Electrochemical sensor for the determination of dopamine in presence of high concentration of ascorbic acid using a fullerene-C60 coated gold electrode, Electroanalysis, 2008, 20, 757–764 CrossRef CAS.
- R. N. Goyal, M. Oyama, V. K. Gupta, S. P. Singh and S. Chatterjee, Sensors for 5-hydroxytryptamine and 5-hydroxyindole acetic acid based on nanomaterial modified electrodes, Sens. Actuators, B, 2008, 134, 816–821 CrossRef CAS.
- R. N. Goyal, V. K. Gupta and S. Chatterjee, Fullerene–C60–modified edge plane pyrolytic graphite electrode for the determination of dexamethasone in pharmaceutical formulations and human biological fluids, Biosens. Bioelectron., 2009, 24, 1649–1654 CrossRef CAS PubMed.
-
D. Z. John, Handbook of drinking water quality: standards and controls, Van Nostrand Reinhold, New York, 1990 Search PubMed.
-
E. A. Laws, Aquatic pollution: an introductory text, Wiley, New York, 3rd edn, 2000 Search PubMed.
- K. Yang, L. Z. Zhu and B. S. Xing, Adsorption of polycyclic aromatic hydrocarbons by carbon nanomaterials, Environ. Sci. Technol., 2006, 40, 1855–1861 CrossRef CAS PubMed.
- K. Yang, X. Wang, L. Zhu and B. Xing, Competitive sorption of pyrene, phenanthrene, and naphthalene on multiwalled carbon nanotubes, Environ. Sci. Technol., 2006, 40, 5804–5810 CrossRef CAS PubMed.
- Y. H. Li, Z. Di, J. Ding, D. Wu, Z. Luan and Y. Zhu, Adsorption thermodynamic, kinetic and desorption studies of Pb2+ on carbon nanotubes, Water Res., 2005, 39(4), 605–609 CrossRef CAS PubMed.
- H. M. Al-Saidi, M. A. Abdel-Fadeel, A. Z. El-Sonbati and A. A. El-Bindary, Multi-walled carbon nanotubes as an adsorbent material for the solid phase extraction of bismuth from aqueous media: kinetic and thermodynamic studies and analytical applications, J. Mol. Liq., 2016, 216, 693–698 CrossRef CAS.
- S. Kumar, G. Bhanjana, N. Dilbaghi and A. Umar, Multi walled carbon nanotubes as sorbent for removal of crystal violet, J. Nanosci. Nanotechnol., 2014, 14, 7054–7059 CrossRef CAS PubMed.
- S. Mosayebidorcheh and M. Hatami, Heat transfer analysis in carbon nanotube-water between rotating disks under thermal radiation conditions, J. Mol. Liq., 2017, 240, 258–267 CrossRef CAS.
- N. Nakashima, Soluble carbon nanotubes: Fundamental and applications, Int. J. Nanosci., 2005, 4, 119–137 CrossRef CAS.
- D. A. Britz and A. N. Khlobystov, Noncovalent interactions of molecules with single walled carbon nanotubes, Chem. Soc. Rev., 2006, 35(7), 637–659 RSC.
- L. A. Girifalco, M. Hodak and R. S. Lee, Carbon nanotubes, buckyballs, ropes, and a universal graphitic potential, Phys. Rev. B: Condens. Matter Mater. Phys., 2000, 62(19), 13104 CrossRef CAS.
- E. Hammel, X. Tang, M. Trampert, T. Schmitt, K. Mauthner, A. Eder and P. Pötschke, Carbon nanofibers for composite applications, Carbon, 2004, 42(5–6), 1153–1158 CrossRef CAS.
- T. Liu, I. Y. Phang, L. Shen, S. Y. Chow and W. D. Zhang, Morphology and mechanical properties of multiwalled carbon nanotubes reinforced nylon-6 composites, Macromolecules, 2004, 37(19), 7214–7222 CrossRef CAS.
- Y. S. Song and J. R. Youn, Influence of dispersion states of carbon nanotubes on physical properties of epoxy nanocomposites, Carbon, 2005, 43(7), 1378–1385 CrossRef CAS.
- K. E. Geckeler and T. Premkumar, Carbon nanotubes: are they dispersed or dissolved in liquids?, Nanoscale Res. Lett., 2011, 6(1), 136 CrossRef PubMed.
- A. Abbas, A. M. Al-Amer, T. Laoui, M. J. Al-Marri, M. S. Nasser, M. Khraisheh and M. A. Atieh, Heavy metal removal from aqueous solution by advanced carbon nanotubes: critical review of adsorption applications, Sep. Purif. Technol., 2016, 157, 141–161 CrossRef.
- O. V. Kharissova, B. I. Kharisov and E. G. de Casas Ortiz, Dispersion of carbon nanotubes in water and non-aqueous solvents, RSC Adv., 2013, 3(47), 24812–24852 RSC.
- H. Hyung, J. D. Fortner, J. B. Hughes and J. H. Kim, Natural organic matter stabilizes carbon nanotubes in the aqueous phase, Environ. Sci. Technol., 2007, 41, 179–184 CrossRef CAS PubMed.
- R. Q. Long and R. T. Yang, Carbon nanotubes as superior sorbent for dioxin removal, J. Am. Chem. Soc., 2001, 123, 2058–2059 CrossRef CAS PubMed.
- G. P. Rao, C. Lu and F. Su, Sorption of divalent heavy metal ions from aqueous solution by carbon nanotubes: a review, Sep. Purif. Technol., 2007, 58, 224–231 CrossRef CAS.
- J. Hilding, E. A. Grulke, S. B. Sinnott, D. Qian, R. Andrews and M. Jagtoyen, Sorption of butane on carbon multiwall nanotubes at room temperature, Langmuir, 2001, 17, 7540–7544 CrossRef CAS.
- C. S. Lu, Y. L. Chung and K. F. Chang, Adsorption of trihalomethanes from water with carbon nanotubes, Water Res., 2005, 39, 1183–1189 CrossRef CAS PubMed.
- C. J. M. Chin, L. C. Shih, H. J. Tsai and T. K. Liu, Adsorption of o-xylene and p-xylene from water by SWCNTs, Carbon, 2007, 45, 1254–1260 CrossRef CAS.
- Q. Liao, J. Sun and L. Gao, Adsorption of chlorophenols by multiwalled carbon nanotubes treated with HNO3 and NH3, Carbon, 2008, 46, 553–555 CrossRef CAS.
- X. J. Peng, Y. H. Li, Z. K. Luan, Z. C. Di, H. Y. Wang, B. H. Tian and Z. P. Jia, Adsorption of 1, 2-dichlorobenzene from water to carbon nanotubes, Chem. Phys. Lett., 2003, 376, 154–158 CrossRef CAS.
- Q. Liao, J. Sun and L. Gao, The adsorption of resorcinol from water using multi-walled carbon nanotubes, Colloids Surf., 2008, 312, 160–165 CrossRef CAS.
- S. Gotovac, H. Honda, Y. Hattori, K. Takahashi, H. Kanoh and K. Kaneko, Effect of nanoscale curvature of single-walled carbon nanotubes on adsorption of polycyclic aromatic hydrocarbons, Nano Lett., 2007, 7, 583–587 CrossRef CAS PubMed.
- D. C. Luehrs, J. P. Hickey, P. E. Nilsen, K. A. Godbole and T. N. Rogers, Linear solvation energy relationship of the limiting partition coefficient of organic solutes between water and activated carbon, Environ. Sci. Technol., 1996, 30, 143–152 CrossRef CAS.
- O. G. Apul, Q. Wang, T. Shao, J. R. Rieck and T. Karanfi, Predictive model development for adsorption of aromatic contaminants by multi-walled carbon nanotubes, Environ. Sci. Technol., 2012, 47, 2295–2303 CrossRef PubMed.
- M. Rahimi-Nasrabadi, R. Akhoondi, S. M. Pourmortazavi and F. Ahmadi, Predicting adsorption of aromatic compounds by carbon nanotubes based on quantitative structure property relationship principles, J. Mol. Struct., 2015, 1099, 510–515 CrossRef CAS.
- X. R. Xia, N. A. Monteiro-Riviere and J. E. Riviere, An index for characterization of nanomaterials in biological systems, Nat. Nanotechnol., 2010, 5, 671–675 CrossRef CAS PubMed.
- V. Chayawan, Quantum-mechanical parameters for the risk assessment of multiwalled carbon-nanotubes: a study using adsorption of probe compounds and its application to biomolecules, Environ. Pollut., 2016, 218, 615–624 CrossRef PubMed.
- O. G. Apul, P. Xuan, F. Luo and T. Karanfil, Development of a 3D QSPR model for adsorption of aromatic compounds by carbon nanotubes: comparison of multiple linear regression, artificial neural network and support vector machine, RSC Adv., 2013, 3, 23924–23934 RSC.
- O. G. Apul, Y. Zhou and T. Karanfil, Mechanisms and modeling of halogenated aliphatic contaminant adsorption by carbon nanotubes, J. Hazard. Mater., 2015, 295, 138–144 CrossRef CAS PubMed.
- Z. Hassanzadeh, M. Kompany-Zareh, R. Ghavami, S. Gholami and A. Malek-Khatabi, Combining radial basis function neural network with genetic algorithm to QSPR modeling of adsorption on multi-walled carbon nanotubes surface, J. Mol. Struct., 2015, 1098, 191–198 CrossRef CAS.
- H. Yilmaz, B. Rasulev and J. Leszczynski, Modeling the dispersibility of single walled carbon nanotubes in organic solvents by quantitative structure-activity relationship approach, Nanomaterials, 2015, 5, 778–791 CrossRef CAS PubMed.
- M. Salahinejad and E. Zolfonoun, QSAR studies of the dispersion of SWNTs in different organic solvents, J. Nanopart. Res., 2013, 15, 2028 CrossRef.
- M. Rofouei, M. Salahinejad and J. B. Ghasemi, An alignment independent 3D-QSAR modeling of dispersibility of single-walled carbon nanotubes in different organic solvents, Fullerenes, Nanotubes, Carbon Nanostruct., 2014, 22, 605–617 CrossRef CAS.
- A. Heidari and M. H. Fatemi, Hybrid docking-Nano-QSPR: an alternative approach for prediction of chemicals adsorption on nanoparticles, NANO, 2016, 11, 1650078 CrossRef CAS.
- S. D. Bergin, Z. Sun, D. Rickard, P. V. Streich, J. P. Hamilton and J. N. Coleman, Multicomponent solubility parameters for single-walled carbon, nanotube−solvent mixtures, ACS Nano, 2009, 3, 2340–2350 CrossRef CAS PubMed.
-
http://www.chemaxon.com
.
-
http://www.talete.mi.it/products/dragon description.htm
.
-
http://www.yapcwsoft.com/dd/padeldescriptor
.
- S. Das, P. K. Ojha and K. Roy, Multilayered variable selection in QSPR: a case study of modeling melting point of bromide ionic liquids, Int. J. Quant. Struct.-Prop. Relat., 2017, 2(1), 106–124 Search PubMed.
- P. K. Ojha and K. Roy, Comparative QSARs for antimalarial endochins: importance of descriptor-thinning and noise reduction prior to feature selection, Chemom. Intell. Lab. Syst., 2011, 109(2), 146–161 CrossRef CAS.
-
http://teqip.jdvu.ac.in/QSAR_Tools/DTCLab
.
- K. Roy, R. N. Das, P. Ambure and R. B. Aher, Be aware of error measures. Further studies on validation of predictive QSAR models, Chemom. Intell. Lab. Syst., 2016, 152, 18–33 CrossRef CAS.
- K. Roy, P. Ambure, S. Kar and P. K. Ojha, Is it possible to improve the quality of predictions from an “intelligent” use of multiple QSAR/QSPR/QSTR models?, J. Chemom., 2018, 32(4), 2992 CrossRef.
-
R. B. Darlington, in Regression and linear models, New York, McGraw-Hill, 1990 Search PubMed.
- D. Rogers and A. J. Hopfinger, Application of genetic
function approximation to quantitative structure-activity relationships and quantitative structure-property relationships, J. Chem. Inf. Comput. Sci., 1994, 34, 854–866 CrossRef CAS.
- P. K. Ojha, I. Mira, R. N. Das and K. Roy, Further exploring rm2 metrics for validation of QSPR models, Chemom. Intell. Lab. Syst., 2011, 107(1), 194–205 CrossRef CAS.
- I. Lawrence and K. Lin, Assay validation using the concordance correlation coefficient, Biometrics, 1992, 599–604 Search PubMed.
- N. Chirico and P. Gramatica, Real external predictivity of QSAR models: how to evaluate it? Comparison of different validation criteria and proposal of using the concordance correlation coefficient, J. Chem. Inf. Model., 2011, 51(9), 2320–2335 CrossRef CAS PubMed.
- K. Roy, S. Kar and P. Ambure, On a simple approach for determining applicability domain of QSAR models, Chemom. Intell. Lab. Syst., 2015, 145, 22–29 CrossRef CAS.
- S. Wold, M. Sjöström and L. Eriksson, PLS-regression: a basic tool of chemometrics, Chemom. Intell. Lab. Syst., 2001, 58, 109–130 CrossRef CAS.
-
UMETRICS, UMETRICS SIMCA-P 10.0, Umea, Sweden, 2002, info@umetrics.com, www.umetrics.com Search PubMed.
-
http://www.minitab.com/en-US/default.aspx
.
- SPSS is statistical software of SPSS Inc., USA, 1999.
- Y. Zhang, S. L. Yuan, W. W. Zhou, J. J. Xu and Y. Li, Spectroscopic evidence and molecular simulation investigation of the pi-pi interaction between pyrene molecules and carbon nanotubes, J. Nanosci. Nanotechnol., 2007, 7, 2366–2375 CrossRef CAS PubMed.
- L. H. Hall and L. B. Kier, Electrotopological state indices for atom types: a novel combination of electronic, topological, and valence state information, J. Chem. Inf. Comput. Sci., 1995, 35(6), 1039–1045 CrossRef CAS.
- L. M. Woods, S. C. Bǎdescu and T. L. Reinecke, Adsorption of simple benzene derivatives on carbon nanotubes, Phys. Rev. B: Condens. Matter Mater. Phys., 2007, 75(1–9), 155415 CrossRef.
- A. Star, T. R. Han, J. C. P. Gabriel, K. Bradley and G. Gruner, Interaction of aromatic compounds with carbon nanotubes: correlation to the Hammett parameter of the substituent and measured carbon nanotube FET response, Nano Lett., 2003, 3, 1421–1423 CrossRef CAS.
- I. Moriguchi, S. Hirono, I. Nakagome and H. Hirano, Comparison of reliability of log P values for drugs calculated by several methods, Chem. Pharm. Bull., 1994, 42(4), 976–978 CrossRef CAS.
- P. K. Ojha and K. Roy, Development of a robust and validated 2D-QSPR model for sweetness potency of diverse functional organic molecules, Food Chem. Toxicol., 2017, 112, 551–562 CrossRef PubMed.
- L. B. Kier and L. H. Hall, The meaning of molecular connectivity: A bimolecular accessibility model, Croat. Chem. Acta, 2002, 75(2), 371–382 CAS.
-
R. Todeschini and V. Consonni, Molecular Descriptors for Chemoinformatics: volume I: alphabetical listing/volume II: appendices, references, John Wiley & Sons, vol. 41, 2009 Search PubMed.
- K. P. Singh and S. Gupta, Nano-QSAR modeling for predicting biological activity of diverse nanomaterials, RSC Adv., 2014, 4(26), 13215–13230 RSC.
- F. S, Su and C. S. Lu, Adsorption kinetics, thermodynamics and desorption of natural dissolved organic matter by multiwalled carbon nanotubes, J. Environ. Sci. Health, Part A: Toxic/Hazard. Subst. Environ. Eng., 2007, 42, 1543–1552 CrossRef PubMed.
- Z. W. Wang, C. L. Liu, Z. G. Liu, H. Xiang, Z. Li and Q. H. Gong, π-π interaction enhancement on the ultrafast third-order optical nonlinearity of carbon nanotubes/polymer composites, Chem. Phys. Lett., 2005, 407, 35–39 CrossRef CAS.
- F. Tournus, S. Latil, M. I. Heggie and J. C. Charlier, π- stacking interaction between carbon nanotubes and organic molecules, Phys. Rev. B: Condens. Matter Mater. Phys., 2005, 72(1–5), 75431 CrossRef.
- S. B. Fagan, A. G. S. Filho, J. O. G. Lima, J. M. Filho, O. P. Ferreira, I. O. Mazali, O. L. Alves and M. S. Dresselhaus, 1, 2- Dichlorobenzene interacting with carbon nanotubes, Nano Lett., 2004, 4, 1285–1288 CrossRef CAS.
- S. Gotovac, Y. Hattori, D. Noguchi, J. Miyamoto, M. Kanamaru, S. Utsumi, H. Kanoh and K. Kanek, Phenanthrene adsorption from solution on single wall carbon nanotubes, J. Phys. Chem. B, 2006, 110, 16219–16224 CrossRef CAS PubMed.
- J. Zhao and J. Lu, Noncovalent functionalization of carbon nanotubes by aromatic organic molecules, Appl. Phys. Lett., 2003, 82, 3746–3748 CrossRef CAS.
- X. J. Li, W. Chen, Q. W. Zhan, L. M. Dai, L. Sowards, M. Pender and R. R. Naik, Direct measurements of interactions between polypeptides and carbon nanotubes, J. Phys. Chem. B, 2006, 110, 12621–12625 CrossRef CAS PubMed.
- A. M. Li, Q. X. Zhang, H. S. Wu, Z. C. Zhai, F. Q. Liu, Z. H. Fei, C. Long, Z. L. Zhu and J. L. Chen, A new amine-modified hypercrosslinked polymeric adsorbent for removing phenolics compounds from aqueous solutions, Adsorpt. Sci. Technol., 2004, 22, 807–819 CrossRef CAS.
- W. Chen, L. Duan, L. Wang and D. Zhu, Adsorption of hydroxyl-and amino-substituted aromatics to carbon nanotubes, Environ. Sci. Technol., 2008, 42(18), 6862–6868 CrossRef CAS PubMed.
- W. Chen, L. Duan and D. Zhu, Adsorption of polar and nonpolar organic chemicals to carbon nanotubes, Environ. Sci. Technol., 2007, 41(24), 8295–8300 CrossRef CAS PubMed.
- L. R. Radovic, C. Moreno-Castilla and J. Rivera-Utrilla, Carbon materials as adsorbents in aqueous solutions, Chem. Phys. Carbon, 2001, 227–406 CAS.
- C. A. Hunter and J. K. M. Sanders, The nature of π-π interactions, J. Am. Chem. Soc., 1990, 112, 5525–5534 CrossRef CAS.
- J. C. Ma and D. A. Dougherty, The cation-π interaction, Chem. Rev., 1997, 97, 1303–1324 CrossRef CAS PubMed.
- C. A. Hunter, K. R. Lawson, J. Perkins and C. J. Urch, Aromatic interactions, J. Chem. Soc., Perkin Trans. 1, 2001, 651–669 RSC.
- A. H. Sulaymon and K. W. Ahmed, Competitive adsorption of furfural and phenolic compounds onto activated carbon in fixed bed column, Environ. Sci. Technol., 2008, 42, 392–397 CrossRef CAS PubMed.
Footnotes |
† Electronic supplementary information (ESI) available. See DOI: 10.1039/c8en01059e |
‡ These authors contributed equally. |
|
This journal is © The Royal Society of Chemistry 2019 |