DOI:
10.1039/D4NR00825A
(Paper)
Nanoscale, 2024,
16, 10675-10681
Sensing nature's alarm: SnO2/MXene gas sensor unveils methyl jasmonate signatures of plant insect stress†
Received
27th February 2024
, Accepted 30th April 2024
First published on 3rd May 2024
Abstract
The incorporation of artificial intelligence into agriculture presents challenges, particularly due to hardware limitations, especially in sensors. Currently, pest detection relies heavily on manual scouting by humans. Therefore, the objective of this study is to create a chemoresistive sensor that enables early identification of the characteristic volatile compound, viz., methyl jasmonate, released during pest infestations. Given the lower reactivity of esters, we have fine-tuned a composite consisting of SnO2 nanoparticles and 2D-MXene sheets to enhance adsorption and selective oxidation, resulting in heightened sensitivity. The optimized composite demonstrated a notable response even at concentrations as low as 120 ppb, successfully confirming pest infestations in tomato crops.
1. Introduction
The indiscriminate use of pesticides in agriculture has led to a significant impact on human health.1 This problem worsens with the worldwide population increase, which directly fuels the demand for food production, and as a result pesticide use increases.2 Early prediction of the damage caused to crops using a real-time sensor incorporated with Internet of Things (IoT) technology will facilitate pest management at the right time, avoiding economic losses. Therefore, in recent times, several initiatives have emerged to create sensors aimed at identifying different stresses in plants by leveraging the unique signatures of volatile organic compounds (VOCs), drawing inspiration from health science.3–5 Detecting VOCs under ambient circumstances is time-consuming as it depends on methods like gas chromatography, mass spectrometry, and fluorescence spectroscopy, which need sophisticated pre-concentration steps.6–10 Chemoresistive sensors present a potential remedy for this concern, as their function is based on the alteration of their electrical resistance upon exposure to specific gases or chemicals in their environment. Among various n-type semiconducting metal oxides, SnO2 stands out as a preferred choice for gas sensor applications due to its broad band-gap of 3.6 eV at 27 °C.11–14
Furthermore, two-dimensional (2D) nanomaterials are drawing considerable interest owing to their distinct microstructure, electrical properties, quantum optics, spillover effect, and outstanding gas adsorption capabilities, with MXenes emerging as a standout choice among them.15–18 The abundance of active sites, commendable conductivity, customizable surface chemistry, and remarkable stability make MXenes highly appealing for applications in gas sensing.19,20 However, other materials such as porous MOFs typically have very low electrical conductivity, which is partly due to the insulating nature of the organic ligands and the lack of overlap between their p orbitals and the d orbitals of metal ions, making them inappropriate candidates for gas sensing materials.21,22
MXene-based chemoresistive sensors exhibited a strong reactivity to vapors of acetone, ethanol, ammonia, and propanal. The exceptional signal-to-noise ratio (S/N) observed in MXene-based sensors underscores their distinct advantage over other 2D materials, making them an outstanding option for detecting volatile organic compounds (VOCs) at concentrations as low as parts per billion (ppb).23 Similar to other 2D nanomaterials, MXenes also face problems such as low selectivity, poor as well as delayed sensor response, instability, etc.24–26 The incorporation of a heterojunction between a p-type MXene and n-type SnO2 can address the limitations of 2D material-based gas sensors by improving charge separation and catalytic activity.27–29
Plants protect themselves against herbivory by deploying a wide array of chemical defences. Methyl jasmonate (MeJA) is an odorous volatile ester crucial for phyto-defence systems that protect crops against herbivory by inducing the production of downstream toxic compounds.30–34 MeJA triggers the release cascade of other terpenes; hence, MeJA is emitted in significantly higher quantities than the previously targeted β-myrcene reported in previous studies.35–37 Injury to leaf tissues triggers the activation of the octadecanoid/lipoxygenase (LOX) pathway in the chloroplast, which oxidizes linolenic acid, an unsaturated fatty acid in the membrane, to 13-hydroperoxylinolenic acid. This activation leads to the buildup of 12-oxyphytodienoic acid and 7-isojasmonic acid. Subsequently, MeJA is synthesized in the cytoplasm through the esterification by JA methyltransferases (JMT).30,38–40
In this study, we have created a gas sensor using a composite of a metal oxide embedded in a 2D-MXene for the identification of the stress-induced signature molecule MeJA. MeJA is released by plants in response to insect stress and possesses multiple oxidation sites. The integration of a p–n junction is anticipated to enhance the sensor's response, selectivity, as well as response and recovery times.
2. Results and discussion
2.1 Characterization of the synthesized nanomaterials
The synthesized SnO2, MXene and SnO2/MXene nanocomposite were characterized using spectroscopy techniques such as UV absorption, XRD and Raman spectroscopy. In Fig. 1a, the SnO2 absorbance spectra show two maxima around 240 and 300 nm that are caused by the valence band (VB) and conduction band (CB) transitions. They could be associated with the Sn4+/Sn2+ intervalence charge transfer and surface Sn4+ species.41 The MXene spectra show two peak maxima around 225 and 275 nm, which might be due to the band gap energy and inter-band transitions.42 In the SnO2/MXene spectra, the SnO2 peak shifted from 300 nm to 310 nm, which may be due to the formation of a heterojunction between SnO2 and the MXene. There was no separate peak observed for the MXene, which may be due to the lower concentration.
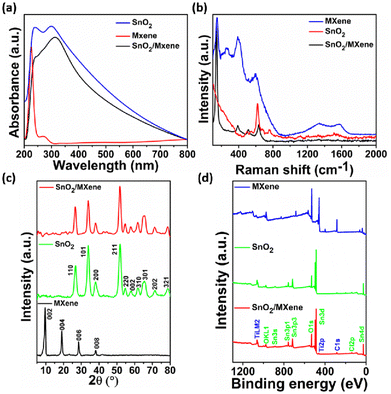 |
| Fig. 1 Characterization of the SnO2, MXene and SnO2/MXene nanocomposite. (a) UV-Vis spectra, (b) Raman spectra, (c) XRD spectra and (d) XPS survey spectra of the SnO2, MXene and SnO2/MXene nanocomposite. | |
The Raman spectra of SnO2 shows the rutile structure of SnO2 corresponding to 2 SnO2 molecules per unit cell and 15 optical phonons.43 A smaller broad peak around 470 to 520 cm−1, an intense peak around 625 cm−1 and a broader peak 750 to 775 cm−1 confirm the rutile tetragonal structure of SnO2. The peak near 670–690 cm−1 suggests that size reduction and disorder in nanoparticles activate some IR modes. Raman spectra of the MXene shows peaks around 150 and 260 cm−1, attributed to the collective vibration of two titanium layers and carbon; the peaks around 390 and 590 cm−1 can be attributed to surface group vibrations.44 Broad peaks around 1340 and 1575 cm−1 represent the D and G peaks of carbon, indicating the presence of sp2 carbon and its defects. Furthermore, in the SnO2/MXene spectra, the peak near 140 cm−1 can be attributed to Ti in the sample, and is shifted towards a lower wavenumber compared to that for the pristine MXene. This shift can be due to the formation of oxygen vacancies in the structure, which will be highly favourable for sensing volatile compounds (Fig. 1b).45,46
XRD analysis confirmed that the SnO2 nanoparticles have a rutile tetragonal structure, which matched with JCPDA file 41-1445. In the MXene spectra, the 61° peak was absent, which indicates that the delaminated Ti3C2Tx sheets were stacked planarly and that the aluminium was completely eliminated.47 Furthermore, the peak around 9.6° confirms the successful synthesis of MXene sheets. However, in the SnO2/MXene composite, no separate peak was observed for the MXene, which indicates that the MXene does not form any cluster but disperses well forming a huge number of Schottky barriers that may favor VOC detection at low concentrations (Fig. 1c).48
Furthermore, for the confirmation of elements and their oxidation state, XPS was performed as shown in ESI Fig. S1a,† where two peaks at 486 eV and 495 eV in the SnO2 Sn 3d spectrum correspond to Sn 3d5/2 and Sn 3d3/2, respectively. Both peaks could be further deconvolved into four peaks, representing Sn2+ and Sn4+. Fig. S2b† shows the O 1s spectrum of the MXene. The peaks of the oxygen at 529 and 530 eV were attributed to the lattice and adsorbed oxygen. Fig. S2a† shows the deconvoluted spectra of Ti 2p, where the 2p3/2 and 2p1/2 pair of peaks that are present at around 458 and 464 eV are attributed to the Ti4+ 2p1/2 and Ti4+ 2p3/2 bonds of the MXene. In Fig. S2c,† the C 1s peak is further deconvoluted into four peaks, ascribed to C–Ti, C–C, CHx/CO, and –COO. In the SnO2/MXene spectra, all of the Sn 3d peaks moved to higher binding energies (Fig. S3a and c†). The peak of the oxygen in the SnO2/MXene nanocomposite shifted to a higher energy. The intensity of the Ti 2p peak from SnO2/MXene at 454 eV increased compared to that of the MXene (Fig. S3b†). Additionally, a new peak appeared at 461 eV, which indicates the formation of a nano-heterojunction between SnO2 and the MXene. The hydrothermal reaction causes the C–Ti peak in the C 1s spectra of SnO2/MXene to weaken.23,49,50
Following the confirmation of the materials through various spectroscopy techniques, the morphologies of the samples were analyzed using SEM and AFM. The MAX phase after HF etching confirms successful aluminium removal, and the layers are clearly separated from each other. Similar sheets were also observed in the AFM image (ESI Fig. S4†). In SnO2/MXene, the MXene sheets are not clearly visible due to the lower concentration (Fig. 2a–c). The transmission electron micrograph (TEM) of SnO2 samples shows particles <5 nm in size, which is less than twice the Debye length (Fig. 2d–f). The high-resolution transmission electron microscopy (HRTEM) images reveal the “d” spacing to be 0.34 nm and 0.26 nm, which correspond to the 110 plane of SnO2 nanoparticles and the 006 plane of the MXene, respectively (Fig. 2d and e inset).
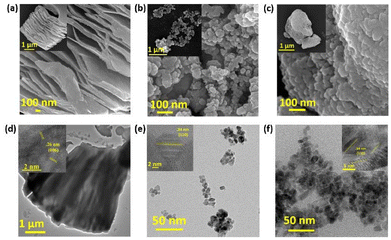 |
| Fig. 2 Scanning electron microscopy images of the (a) MXene, (b) SnO2 and (c) SnO2/MXene nanocomposite. Transmission electron microscopy images of (d) MXene, (e) SnO2 and (f) SnO2/MXene. | |
2.2 Gas sensor optimization
Following the material confirmation through the above characterization, the synthesized material (≈5 mg) was dropcasted on sensor electrodes, with reference to our previous optimization.51,52 To obtain maximum sensitivity, an electrode having a 200 μm interdigit gap and a platinum heating electrode at the back for temperature control have been used. Following this, the electrodes were stabilized thermally in a furnace at ≈500 °C for ≈5 hours. The SEM image of the coated electrode is shown in ESI Fig. S5.†
Finding an optimum working temperature is a very crucial step, as the increase in the surface potential barrier of semiconducting metal oxides, due to the extraction of electrons by the volatile environment, can be overcome by the heating-assisted electron density. This phenomenon enhanced electron tunnelling through the barrier, promoting reactions with adsorbed molecules in a reversible chemisorption fashion that influences sensor performance.53 As the temperature was increased from 50 °C to 175 °C with a gradient of 25 °C using a DC power source, the SnO2 and MXene gave the maximum response at 125 °C and 50 °C, respectively. In case of SnO2, at 50 °C the response was close to negligible; hence, it could not be recorded. However, the MXene, which is widely used as a room-temperature sensor because of its high conductivity and large number of active sites, was able to show a maximum response at 50 °C (Fig. 3a and b and ESI Fig. S7†).24 Interestingly, the SnO2/MXene nanocomposite shows significantly enhanced response at 125 °C, which is approximately 10 times and 5 times greater than the maximum response recorded from SnO2 and the MXene, respectively.
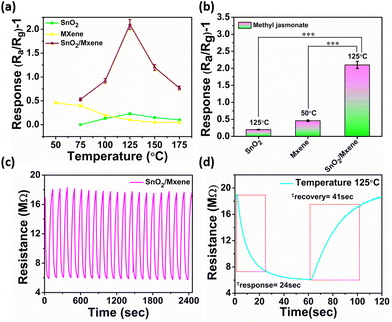 |
| Fig. 3 Characterization of the sensor. (a and b) The effect of temperature and the highest response achieved with SnO2, MXene, and the SnO2/MXene sensor. (c) Reproducibility of the SnO2/MXene sensor for MeJA. (d) Response and recovery times of the SnO2/MXene sensor in the presence of MeJA. Experiments were conducted in triplicate; the SnO2/MXene sensor was compared with SnO2 and MXene sensors and the results are given as mean ± SD. Statistical significance is shown by ***p ≤ 0.001. | |
In the nanocomposite, an increase in the barrier due to the heterojunction may have favoured an increase in response with an increase in temperature up to 125 °C. On the other hand, highly rapid atomic species desorption at high temperatures or the decomposition of the molecules may have caused a decline in response with further increase in temperature.54
In order to check the repeatability of the sensor, the response of the electrode was recorded for 20 on/off cycles for MeJA. In this attempt, the sensor showed high repeatability with very quick response and recovery times (Fig. 3c and d). This indicates that these nanostructures remain stable for a long time under these working conditions, as the temperature is much below their decomposition temperature.
2.3 Selectivity and stability of the sensor
Selectivity of a sensor is crucial to avoid the crosstalk from multiple stress signature molecules, which could provide false information. In this study, we checked the MeJA selectivity of the SnO2/MXene gas sensor in the presence of β-ionone, β-caryophyllene, and β-myrcene. We found that the SnO2/MXene-based gas sensor shows the highest response for MeJA at 125 °C and a negligible response to other analytes (Fig. 4a and ESI Fig. S8†). Furthermore, the limits of detection and sensor response at varying concentrations were examined. It was found that the sensor based on SnO2/MXene can clearly distinguish between MeJA and other analytes at ≥120 ppb (Fig. 4b). The linear plot and R2 of the SnO2/MXene sensor response to a proportional change in concentration are given in ESI Fig. S10.†
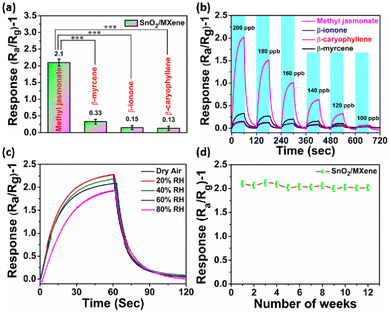 |
| Fig. 4 (a and b) Selectivity of the SnO2/MXene sensor for MeJA against different analytes with a concentration gradient. The stability of the sensor (c) under different RHs (d) for continuous 12 weeks. Three independent experiments were conducted, and the response obtained with methyl jasmonate was compared with those of β-ionone, β-caryophyllene, and β-myrcene. Results are given as mean ± SD. Statistical significance is shown by ***p ≤ 0.001. | |
Humidity is a real challenge for gas sensors because water vapor adsorbed on the surface will result in a decreased sensor response. Hence, the sensor was examined for its response in dry air to 80% RH, with 20% gradient. Compared to 60% humidity, at which all the above optimizations were carried out, lower humidity caused a higher response and higher humidity caused a lower response. Thus, the response was found to be inversely proportional to humidity. 80% humidity is limited to very sparse locations, and hence ignoring it causes the response to have only a ±7% deviation, which can be corrected using humidity data. Furthermore, long-term stability was also checked and it was found that the sensor gave almost similar responses for up to 12 weeks with a stable baseline (Fig. 4c and d).
2.4 Real-time experiment
Tuta absoluta, commonly known as the tomato leaf miner, is a major pest of tomato plants that causes a huge yield loss as it damages both leaves and fruits. For real-time detection, tomato plants were incubated in two separate glass jars: one for the control plant and another for the treatment plant (a digital image of the infected plant with Tuta absoluta is provided in ESI Fig. S12†). The volatile compounds released from the T. absoluta infested plant were collected using a carbotrap, the eluate was concentrated and the compounds were determined using GC-MS (Fig. 5a, ESI Fig. S11†). The air samples from both control and treated plants (Fig. 5b) were exposed to the gas sensor, and it was observed that the treated plant gave a 10 times higher response than the control plant at 36 hours. In the control plant, the highest response was achieved at 12 hours and afterwards it started to decline, but in case of the treated plant, the response slowly increased up to 36 hours, and after that, it started to decline (Fig. 5c and d).
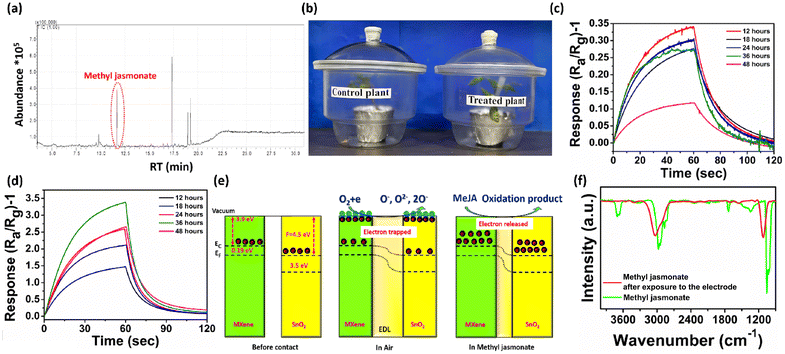 |
| Fig. 5 (a) GC-MS chromatogram obtained from the head-space volatiles of the tomato plant infested with Tuta-absoluta. (b) Photographs of the incubated control and treated plants. Sensor response to the (c) control plant and (d) treated plant at different time periods. (e) Energy diagram of the SnO2/MXene nanocomposite. (f) FTIR spectra of MeJA before and after exposure to the electrode. | |
2.5 Mechanism and reason behind selectivity
SnO2 aids in detecting volatile organic compounds (VOCs), and the metallic MXene will aid in the adsorption of analytes in the heterojunction's interface region. Furthermore, the presence of the semiconducting p-type MXene with the n-type SnO2 together results in a p–n junction barrier that holds more electrons and improves the sensitivity to the incidence of reducing gas. The SnO2 and MXene have work functions of 4.5 eV and 3.9 eV, respectively, which cause discrepancy in the Fermi level as depicted in Fig. 5e. The difference in the Fermi level will lead to the movement of electrons from the MXene to SnO2 until the Fermi level equilibrates, causing band bending, which contributes to better sensitivity.55 Oxygen from the open environment will adsorb onto the sensor surface, which will capture free electrons from the conduction band of SnO2 and increase the resistance. MeJA, with its numerous oxidation sites, will consume the adsorbed oxygen, releasing electrons that influence the electron depletion layer and reduce electrical resistance. Upon re-exposure to regular air, oxygen will once again adsorb onto the surface, capturing free electrons and increasing resistance.
The SnO2/MXene-based gas sensor has demonstrated good selectivity toward MeJA. The selective catalytic oxidation of MeJA may result from the high catalytic activity of Ti at the optimum temperature, which may not be advantageous for other analytes.56 The adsorption of a functional group is another important aspect. MeJA contains an ester group, which promotes a higher degree of physisorption on the surface.50 Such functional groups are absent in β-myrcene, β-caryophyllene, and β-ionone, which may have led to a weak interaction and a decreased sensor response.57–59
Furthermore, FTIR analysis of the adsorbed volatile products eluted from the carbotrap X placed at the outlet of the chamber was performed in the presence and absence of the electrode (Fig. 5f). The peak at 2970–2830 cm−1 corresponds to the asymmetrical and symmetrical stretching vibrations of CH2 groups, which appeared in the samples not exposed to the electrode. This peak shifted to a higher wavenumber in the sample exposed to the electrode, due to its oxidation. Another peak around 1740 cm−1, corresponding to the ν(C
O) stretching vibration of the esters in the MeJA spectra, completely disappeared due to the oxidation of MeJA.60–62
3. Conclusions
A hybrid composite comprising nanoparticles of SnO2/MXene was synthesized and assessed for its efficacy in sensing the phyto-volatile MeJA. The advantage of high sensitivity, coupled with excellent selectivity against other phyto-volatiles, was realized by elevating the working temperature to 125 °C with an optimized MXene concentration of 1%. The formation of a heterojunction between the MXene and SnO2 facilitated the accumulation of more electrons, enhancing sensitivity and yielding a response to a minimal concentration of 120 ppb. Furthermore, the ability of the MXene to adsorb esters complements the heterojunction in terms of sensitivity and selective oxidation. The stability of the transducer was confirmed through multiple on/off cycles over several months and ultimately validated in the detection of pest-infested tomato crops.
Author contributions
P. K. performed the experiments, analyzed the data and wrote the initial draft. S. K. helped in the execution of the sensor validation in the crop. K. S. assisted in designing the plant experiment and AFM. M. C. performed the Raman spectroscopy, GC-MS and XPS analysis. B. K. S. performed XRD and UV analysis and data interpretation. P. S. performed BET and GC-MS analysis. V. S. conceived the idea, supervised the experiments and wrote the manuscript.
Conflicts of interest
There are no conflicts to declare.
Acknowledgements
P. K. is thankful to the UGC for a fellowship. V. S. thanks the Government of India DBT (BT/PR36476/NNT/28/1723/2020) and DST (BT/PR36476/NNT/28/1723/2020). The authors are thankful to Dr Ujjal K Gautam, IISER Mohali, for the AFM access and Director, ICAR NBAIR for extending the facilities.
References
- J. Pretty, Science, 2018, 362, 6417 CrossRef PubMed.
- W. J. Ntow, H. J. Gijzen, P. Kelderman and P. Drechsel, Pest Manage. Sci., 2006, 62, 356–365 CrossRef CAS PubMed.
- M. Chandel, P. Kumar, A. Arora, S. Kataria, S. C. Dubey, M. Djanaguiraman, K. Kaur, B. K. Sahu, A. De Sarkar and V. Shanmugam, Anal. Chem., 2022, 94, 11081–11088 CrossRef CAS PubMed.
- A. T. Güntner, S. Abegg, K. Königstein, P. A. Gerber, A. Schmidt-Trucksäss and S. E. Pratsinis, ACS Sens., 2019, 4, 268–280 CrossRef PubMed.
- W. K. Son, Y. S. Choi, Y. W. Han, D. W. Shin, K. Min, J. Shin, M. J. Lee, H. Son, D. H. Jeong and S. Y. Kwak, Nat. Nanotechnol., 2023, 18, 205–216 CrossRef CAS PubMed.
- C. Bur, M. Bastuck, D. Puglisi, A. Schütze, A. Lloyd Spetz and M. Andersson, Sens. Actuators, B, 2015, 214, 225–233 CrossRef CAS.
- X. Guan, Z. Zhao, S. Cai, S. Wang and H. Lu, J. Chromatogr. A, 2019, 1587, 227–238 CrossRef CAS PubMed.
- C. Massie, G. Stewart, G. McGregor and J. R. Gilchrist, Sens. Actuators, B, 2006, 113, 830–836 CrossRef CAS.
- J. H. Lee, S. M. Hwang, D. W. Lee and G. S. Heo, Bull. Korean Chem. Soc., 2002, 23, 488–496 CrossRef CAS.
- Z. Zhou, W. Xiong, Y. Zhang, D. Yang, T. Wang, Y. Che and J. Zhao, Anal. Chem., 2017, 89, 3814–3818 CrossRef CAS PubMed.
- H. Ji, W. Zeng and Y. Li, Nanoscale, 2019, 1–5 Search PubMed.
- S. Wang, J. Yang, H. Zhang, Y. Wang, X. Gao, L. Wang and Z. Zhu, Sens. Actuators, B, 2015, 207, 83–89 CrossRef CAS.
- X. Liu, N. Chen, B. Han, X. Xiao, G. Chen, I. Djerdj and Y. Wang, Nanoscale, 2015, 7, 14872–14880 RSC.
- S. Xu, S. Chu, L. Yang, Y. Chen, Z. Wang and C. Jiang, New J. Chem., 2018, 42, 19557–19563 RSC.
- Y. Z. Zhang, K. H. Lee, D. H. Anjum, R. Sougrat, Q. Jiang, H. Kim and H. N. Alshareef, Sci. Adv., 2018, 4, 1–8 Search PubMed.
- B. Anasori, M. R. Lukatskaya and Y. Gogotsi, Nat. Rev. Mater., 2017, 2, 1–17 Search PubMed.
- C. Anichini, W. Czepa, D. Pakulski, A. Aliprandi, A. Ciesielski and P. Samorì, Chem. Soc. Rev., 2018, 47, 4860–4908 RSC.
- M. Singh, E. D. Gaspera, T. Ahmed, S. Walia, R. Ramanathan, J. v. Embden, E. Mayes and V. Bansal, 2D Mater., 2017, 4, 025110 CrossRef.
- R. Bhardwaj and A. Hazra, J. Mater. Chem. C, 2021, 9, 15735–15754 RSC.
- S. Radhakrishnan and C. S. Rout, Nanoscale
Adv., 2023, 5, 4649–4669 RSC.
- X. Keng, C. Zhan, W. Zhao, X. Yu, Q. Zhu and Li Yang, J. Hazard. Mater., 2023, 416, 125906 Search PubMed.
- S. Lei, M. G. Campbell and M. Dincă, Angew. Chem., Int. Ed., 2016, 55, n3566–n3579 CrossRef PubMed.
- S. Liu, M. Wang, C. Ge, S. Lei, S. Hussain, M. Wang, G. Qiao and G. Liu, Sens. Actuators, B, 2022, 365, 131919 CrossRef CAS.
- S. J. Kim, H. J. Koh, C. E. Ren, O. Kwon, K. Maleski, S. Y. Cho, B. Anasori, C. K. Kim, Y. K. Choi, J. Kim, Y. Gogotsi and H. T. Jung, ACS Nano, 2018, 12, 986–993 CrossRef CAS PubMed.
- W. Yuan, K. Yang, H. Peng, F. Li and F. Yin, J. Mater. Chem. A, 2018, 6, 18116–18124 RSC.
- E. Lee, A. Vahidmohammadi, B. C. Prorok, Y. S. Yoon, M. Beidaghi and D. J. Kim, ACS Appl. Mater. Interfaces, 2017, 9, 37184–37190 CrossRef CAS PubMed.
- T. He, W. Liu, T. Lv, M. Ma, Z. Liu, A. Vasiliev and X. Li, Sens. Actuators, B, 2021, 329, 129275 CrossRef CAS.
- T.-C. Huang, H.-C. Tsai, Y.-C. Chin, W.-S. Huang, Y.-C. Chiu, T.-C. Hsu, Z.-C. Chia, T.-C. Hung, C.-C. Huang and Y.-T. Hsieh, ACS Appl. Nano Mater., 2021, 4, 10103–10115 CrossRef CAS.
- Y.-H. Chen, K.-A. Tsai, T.-W. Liu, Y.-J. Chang, Yu.-C. Wei, M.-W. Zheng, S.-H. Liu, M.-Y. Liao, P.-Yu Sie, J.-H. Lin, S.-W. Tseng and Y.-C. Pu, J. Phys. Chem. Lett., 2023, 14, 122–131 CrossRef CAS PubMed.
- C. Rodriguez-Saona, S. J. Crafts-Brandner, P. W. Paré and T. J. Henneberry, J. Chem. Ecol., 2001, 27, 679–695 CrossRef CAS PubMed.
- R. Meyer, G. F. Rautenbach and I. A. Dubery, Phytochem. Anal., 2003, 14, 155–159 CrossRef CAS PubMed.
- O. S. Kulkarni, M. Mazumder, S. Kini, E. D. Hill, J. S. B. Aow, S. M. L. Phua, U. Elejalde, S. Kjelleberg and S. Swarup, Nat. Chem. Biol., 2023, 20, 473–483 CrossRef PubMed.
- N. C. Weber, J. S. Ana, L. R. Redaelli and E. F. Dawud, Entomol. Exp. Appl., 2023, 171, 162–171 CrossRef CAS.
- X. Han, S. Shao, X. Han and Y. Zhang, Molecules, 2021, 27(15), 4728 CrossRef PubMed.
- A. L. Schilmiller and G. A. Howe, Curr. Opin. Plant Biol., 2005, 8, 369–377 CrossRef CAS PubMed.
- K. Luck, J. Jirschitzka, S. Irmisch, M. Huber, J. Gershenzon and T. G. Köllner, BMC Plant Biol., 2016, 16, 1–15 CrossRef PubMed.
- G. A. Howe and G. Jander, Annu. Rev. Plant Biol., 2008, 59, 41–66 CrossRef CAS PubMed.
- C. Wasternack, Ann. Bot., 2007, 100, 681–697 CrossRef CAS PubMed.
- N. C. Avanci, D. D. Luche, G. H. Goldman and M. H. Goldman, Genet. Mol. Res., 2010, 9, 484–505 CrossRef CAS PubMed.
- M. Reyes-Díaz, T. Lobos, L. Cardemil, A. Nunes-Nesi, J. Retamales, L. Jaakola, M. Alberdi and A. Ribera-Fonseca, Molecules, 2016, 21, 1–18 CrossRef PubMed.
- D. A. Popescu, J. M. Herrmann, A. Ensuque and F. Bozon-Verduraz, Phys. Chem. Chem. Phys., 2001, 3, 2522–2530 RSC.
- X. Chen, X. Sun, W. Xu, G. Pan, D. Zhou, J. Zhu, H. Wang, X. Bai, B. Dong and H. Song, Nanoscale, 2018, 10, 1111–1118 RSC.
- R. N. Mariammal, K. Ramachandran, B. Renganathan and D. Sastikumar, Sens. Actuators, B, 2012, 169, 199–207 CrossRef CAS.
- A. Thakur, N. Chandran B. S, K. Davidson, A. Bedford, H. Fang, Y. Im, V. Kanduri, B. C. Wyatt, S. K. Nemani, V. Poliukhova, R. Kumar, Z. Fakhraai and B. Anasori, Small Methods, 2023, 7, 1–16 Search PubMed.
- M. Al-Hashem, S. Akbar and P. Morris, Sens. Actuators, B, 2019, 301, 126845 CrossRef CAS.
- Z. Wang, F. Wang, A. Hermawan, Y. Asakura, T. Hasegawa, H. Kumagai, H. Kato, M. Kakihana, J. Zhu and S. Yin, J. Mater. Sci. Technol., 2021, 73, 128–138 CrossRef CAS.
- Y. Wang, Z. Niu, Y. Dai, P. Mu and J. Li, Nanoscale, 2023, 15, 4170–4194 RSC.
- O. Chmela, J. Sadílek, G. Domènech-Gil, J. Samà, J. Somer, R. Mohan, A. Romano-Rodriguez, J. Hubálek and S. Vallejos, Nanoscale, 2018, 10, 9087–9096 RSC.
- P. Wu, Y. Li, S. Xiao, D. Chen, J. Chen, J. Tang and X. Zhang, ACS Appl. Mater. Interfaces, 2022, 14, 48200–48211 CrossRef CAS PubMed.
- V. Adepu, A. Kunchur, C. S. R. Kolli, S. Siddhartha, V. Mattela and P. Sahatiya, ACS Appl. Nano Mater., 2022, 5, 6852–6863 CrossRef CAS.
- S. Kataria, M. Chandel, P. Kumar, M. Palanisami, N. Moun, S. Kanagarajan and V. Shanmugam, Sens. Actuators, B, 2023, 403, 134975 CrossRef.
- P. Kumar, M. Chandel, S. Kataria, K. Swami, K. Kaur, B. K. Sahu, A. Dadhich, R. R. Urkude, K. Subaharan, N. Koratkar and V. Shanmugam, ACS Sens., 2024, 9(1), 81–91 CrossRef CAS PubMed.
- S. H. Wang, T. C. Chou and C. C. Liu, Sens. Actuators, B, 2003, 94, 343–351 CrossRef CAS.
- P. H. Suman, A. A. Felix, H. L. Tuller, J. A. Varela and M. O. Orlandi, Sens. Actuators, B, 2015, 208, 122–127 CrossRef CAS.
- R. Vishnuraj, K. K. Karuppanan, M. Aleem and B. Pullithadathil, Nanoscale Adv., 2020, 2, 4785–4797 RSC.
- S. T. Navale, Z. B. Yang, C. Liu, P. J. Cao, V. B. Patil, N. S. Ramgir, R. S. Mane and F. J. Stadler, Sens. Actuators, B, 2018, 255, 1701–1710 CrossRef CAS.
- N. Liu, X. F. Wang, G. Zhang, H. Liang, T. Li, Y. Zhao, T. Zhang, Z. Tan and X. Z. Song, ACS Appl. Nano Mater., 2022, 5, 14320–14327 CrossRef CAS.
- Y. Fan, W. Wang, J. Zhang, Y. Lu, C. Liu, S. Adimi and Y. Chen, Sens. Actuators, B, 2021, 344, 130129 CrossRef CAS.
- W. Yang, B. Fang, X. Xiao, H. Meng and S. Liu, Sens. Actuators, B, 2022, 358, 131457 CrossRef CAS.
- C. Li, P. Wang, N. W. Menzies, E. Lombi and P. M. Kopittke, J. Plant Nutr. Soil Sci., 2018, 181, 409–418 CrossRef CAS.
- P. Guzmán, V. Fernández, J. Graça, V. Cabral, N. Kayali, M. Khayet and L. Gil, Front. Plant Sci., 2014, 5, 1–12 Search PubMed.
- J. A. Heredia-Guerrero, J. J. Benítez, E. Domínguez, I. S. Bayer, R. Cingolani, A. Athanassiou and A. Heredia, Front. Plant Sci., 2014, 5, 1–14 Search PubMed.
|
This journal is © The Royal Society of Chemistry 2024 |